DOI:
10.1039/D1NR04996H
(Paper)
Nanoscale, 2022,
14, 2617-2627
Revealing the heterogeneity in neuroblastoma cells via nanopillar-guided subnuclear deformation†
Received
31st July 2021
, Accepted 29th November 2021
First published on 8th February 2022
Abstract
Neuroblastoma is a hard-to-treat childhood cancer that is well known for the heterogeneity of its clinical phenotypes. Although the risk levels of neuroblastoma have been defined from a complex matrix of clinical and tumor biological factors to guide treatment, the accuracy in predicting cancer relapse and related fatality is still poor in many cases, where heterogeneity with subpopulations in highly malignant or drug-resistant tumors is believed to be underestimated by the current analysis methods. Therefore, new technologies to probe neuroblastoma heterogeneity are needed for the improvement of risk stratification. In this study, we introduce the nanopillar-guided subnuclear morphology as an effective indicator for heterogeneity evaluation among individual neuroblastoma cells. Nuclear polymorphisms, especially the generation of subnuclear irregularities, are well-known markers of high cancer metastasis risk and poor prognosis. By quantitatively evaluating the orientation of nanopillar-guided nuclear envelope features in neuroblastoma cells, we identified two subpopulations with differential motilities and EMT marker levels. Moreover, with endogenous expression, cells with high levels of the nuclear structure protein lamin A exhibit anisotropic deformation on nanopillars and migrate faster than low-lamin A cells, indicating a greater potential for metastasis. Overexpression of lamin A, however, reduces both the coherency and migration speed, suggesting that subpopulations with similar lamin A levels may have different metastatic potentials. We further verified that nanopillar-generated nuclear deformation patterns can quantitatively reveal individual cells’ responses to anti-cancer drug treatment. Overall, we envision that the nanopillar-based assessment of subnuclear irregularities brings new additions to our toolkits for both precise risk stratification in neuroblastoma and the evaluation of related anti-cancer therapeutics.
Introduction
As a devastating childhood cancer, neuroblastoma is widely known for its high relapse rate and significant drop of survival rate to ∼10% once relapse occurs.1,2 One of the key contributing factors is its significant heterogeneity in cellular phenotypes, where a subset of the cells may survive or not respond to treatment and may cause relapse. In clinical practice, risk stratification that is based on a series of clinical and molecular characterizations,3 including tumor histology,4 MYCN amplification state,5,6 and DNA ploidy,7 is critically conducted to tailor the treatment plan for individual patients. However, poor clinical outcomes are still observed in tumors irrespective of risk factors such as MYCN amplification,8 suggesting that new aspects of the heterogeneity in neuroblastoma are waiting to be revealed to improve the accuracy of risk stratification for better therapeutic guidance.
Both genetic markers and morphological features are used in the risk stratification of neuroblastoma;3 the genetic markers have gained more attention owing to their well-established sequencing technologies.9 Morphological changes observed in histology, however, are still evaluated according to description-based grading systems,10 despite their widely recognized strong implications for cancer progression and high prevalence across diverse cancer types.11–13 Nevertheless, some interesting facts have been observed, such as the heterogeneity results from the integration of different metabolic and genetic conditions; meanwhile, similar morphological alterations throughout cancer progression are shared among different cancer cells, with substantial metabolic and genetic variation. It is intriguing to see whether technologies to improve the precision in morphological analysis can bring new insights for better understanding of the heterogeneity in neuroblastoma.
Among all the cellular components, the nucleus serves as the central location to host all the genetic regulation activities and often displays dramatic morphological changes in many types of cancers, including neuroblastoma.10,11 Especially, subnuclear shape irregularities in tens to hundreds of nanometers, such as grooves and folds on the nuclear envelope, nucleoplasmic reticulum, and nucleo-cytoplasmic inclusions, have long been used as robust diagnostic and prognostic markers in histological assessment; they are associated with many oncogenetic changes, such as genomic instability and cancer metastasis.14–16 However, whether they can be used to characterize heterogeneity in neuroblastoma and thus for risk stratification is still unclear. Quantitative characterization of these subnuclear irregularities remains technically challenging for conventional image segmentation methods, as they mostly target whole nuclear features, such as size, circularity and aspect ratio. Thus, technologies to probe subcellular features in cells will be highly beneficial.
Recently, vertically aligned nanostructures have been introduced to effectively probe a variety of subcellular properties, including electrophysiological signals,17 cell adhesion forces,18–20 intracellular enzyme activity,21 cytosolic pH,22 gene transcription,23–25 immune cell behavior26 and protein dynamics on the plasma membrane.27–32 More importantly, protruding nanostructures can reach deeply into the cell interior and directly interrogate the subnuclear morphology to probe nuclear deformability,33 nuclear mechanotransduction34 and nuclear shape irregularities35 in individual cells. We therefore hypothesized that nanopillar-enabled evaluation of subnuclear shape irregularities can be used to probe heterogeneity in neuroblastoma. Specifically, we will study the nanopillar-guided nuclear deformation patterns in neuroblastoma cells with the expression of different nuclear lamina proteins and how they correlate with metastatic cellular properties such as cell migration and EMT transition. The potential of using nanopillar-based characterization for anti-cancer drug evaluation will also be validated in neuroblastoma cells.
Results and discussion
Nanopillar-guided subnuclear patterns associated with heterogeneity of lamin A expression level in SK-N-SH cells
Neuroblastoma cells are known to have different expression levels of lamin proteins36 that critically impact the morphology and deformability of the nuclei.37–39 Here, we take SK-N-SH cells as a model system and probe, firstly, the heterogeneous expression levels of lamin A and lamin B1 via immunostaining. Lamin B1 is known to be constitutively expressed in all neuroblastoma cells, while lamin A levels vary significantly and are more associated with differentiated cells.40–42 A-type lamins, including lamin A and lamin C, are known to dominate the mechanical properties of the cell nucleus and determine the nuclear morphology.37 As shown in Fig. 1a, all the cells expressed lamin B1 as expected, but only some of them showed detectable lamin A levels. More interestingly, the nuclear morphology showed a strong correlation with the endogenous level of lamin A; high-lamin A cells displayed an abnormal nuclear morphology with obvious grooves and invaginations, in contrast with low-lamin A cells, which normally show a clean nuclear contour (Fig. 1a). However, when characterized using conventional nuclear morphometric parameters such as nuclear area and circularity (Fig. 1b), the two populations are not always distinguishable (nuclear area: 115.2 ± 29.7 μm2, N = 89 cells (low); 165.5 ± 73.1 μm2, N = 44 cells (high), p value <0.0001. Circularity: 0.7720 ± 0.0926, N = 89 cells (low); 0.7974 ± 0.0992, N = 44 cells (high), p value = 0.1595). More importantly, the subnuclear irregularities shown in high-lamin A cells but not in low-lamin A cells (Fig. 1c) are overlooked in the conventional methods, which target the overall morphology of the entire nucleus instead of the subnuclear features.
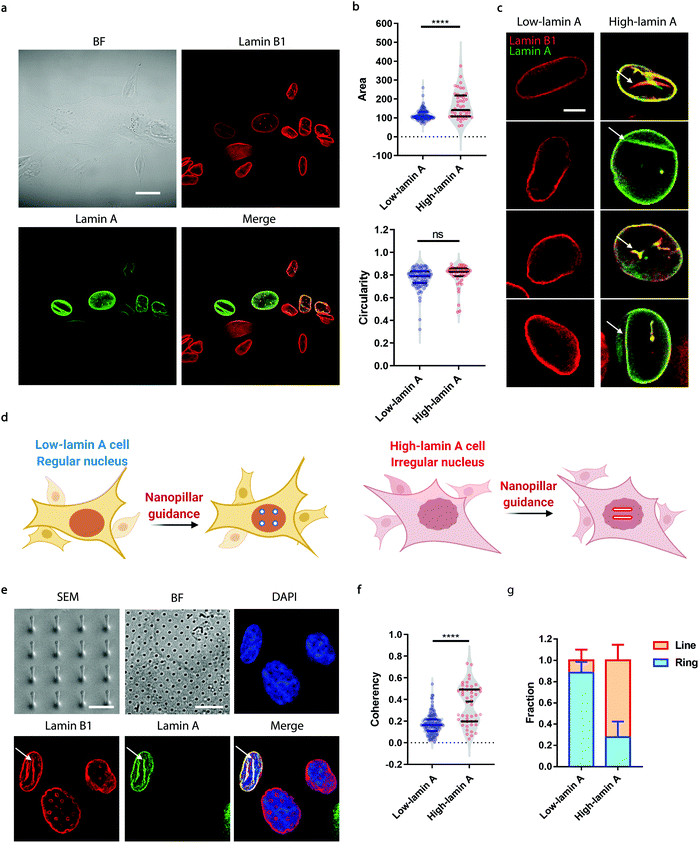 |
| Fig. 1 The degree of subnuclear shape irregularities is dependent on the lamin A level in SK-N-SH cells. (a) Fluorescence images showing that cells with high lamin A levels show abnormal nuclear morphology. Scale bar, 20 μm. (b) Characterization of the whole-nucleus morphology between low-lamin A cells and high-lamin A cells. (c) Raw images showing that high-lamin A cells exhibit an abnormal nuclear morphology while low-lamin A cells display a regular nuclear contour. Arrows indicate the locations of the subnuclear irregularities. Scale bar, 5 μm. (d) Schematics showing differential nanopillar-guided features in low- and high-lamin A cells. (e) SEM images of the nanopillar arrays and fluorescence images showing that the subnuclear grooves are guided by nanopillars in high-lamin A cells, whereas ring-like features can be observed in low-lamin A cells. The arrows indicate the locations of the guided subnuclear irregularities. Scale bars, 2 μm (SEM); 10 μm (BF). (f) Anisotropy measurements of the nanopillar-guided subnuclear features in low-lamin A cells (N = 122 pillars) and high-lamin A cells (N = 48 pillars). (g) Fractions of ring deformation and line deformation in low-lamin A cells (N = 64 cells) and high-lamin A cells (N = 24 cells). Statistical significance was evaluated by an unpaired t-test with Welch's correction. **** P < 0.0001; ns > 0.05. | |
Taking advantage of the nanopillar-guided subnuclear deformation reported earlier,33,35 we hereby investigated whether the guided patterns on nanopillars can effectively reflect neuroblastoma heterogeneity. As illustrated in Fig. 1d, the SK-N-SH cells were classified into low-lamin A and high-lamin A groups to evaluate their subnuclear features on the nanopillars separately. Nanopillar arrays with 500 nm in diameter, 3 μm in pitch, and 1.5 μm in height were used to provide effective guidance of the nuclear grooves in cancer cells, similar to the geometry optimized in an earlier study.35 The scanning electron micrograph (SEM) is shown in Fig. 1e. When visualizing the nucleus by immunostaining both lamin B1 and lamin A, it is interesting to see that the cells with low lamin A showed clear ring deformation; meanwhile, those high in lamin A displayed aligned lines across nearby nanopillars, suggesting a nanopillar-guided alignment of their subnuclear irregular features (Fig. 1e). It is noteworthy that heterogeneity still exists among the cells with similar lamin A levels. For example, both line and ring features have been observed in high-lamin A cells (Fig. S1†). By characterizing the orientation of nanopillar-guided features using the Orientation J plug-in43 in ImageJ,44 we obtained a quantified coherency value on each nanopillar. The coherency ranges from 0 to 1. 0 refers to fully isotropic features without preferred orientation, suggesting fewer irregularities; meanwhile, 1 refers to completely anisotropic features with a dominant orientation angle, indicating more irregularities. Comparing cells with low and high lamin A, it is obvious that high-lamin A cells showed a significantly higher pillar coherency value (0.3525 ± 0.1838, n = 48 pillars) than low-lamin A cells (0.1727 ± 0.0961, n = 122 pillars) (Fig. 1f), consistent with the fact that high-lamin A cells generally have more subnuclear shape irregularities than low-lamin A cells. More interestingly, two subpopulations with different coherency values can be observed in high-lamin A cells according to the two regions with high probability density (pillar coherency ∼0.2 and 0.5, respectively) (Fig. 1f). This implies that heterogeneity exists even within the high-lamin A cells, with one subpopulation showing a high degree of subnuclear shape irregularities while the other is similar to low-lamin A cells. By averaging the pillar coherency values in each cell, we obtained cell coherency values as a single-cell readout for quantitative analysis. Setting the coherency value at 0.3 as a cutoff for differentiating cells with dominant isotropic ring or anisotropic line deformations, we found that most of the low-lamin A cells (0.885 ± 0.101, n = 65 cells) did not show nanopillar-guided abnormal nuclear features. However, the majority of high-lamin A cells (0.722 ± 0.147, n = 24 cells) exhibited aligned subnuclear irregularities on their nanopillars (Fig. 1g).
Correlation of migration speed with lamin A heterogeneity in neuroblastoma cells
To correlate the lamin A heterogeneity with metastatic potential, we examined the cell migration between high- and low-lamin A cells using two established assays, the wound healing assay and the transwell assay. In the wound healing assay, high-lamin A cells exhibited a greater migration rate than low-lamin A cells. At the beginning (0 h) of the wound healing, the high-lamin A cells showed a random distribution across the cell monolayer, with no preference for the wound region (Fig. 2a, 0 h). Intriguingly, after 24 hours of migration, the majority of the cells closer to the wound show high lamin A intensity (Fig. 2a, 24 h), suggesting that the high-lamin A cells are more migratory than the low-lamin A cells. Similarly, when plotting the lamin A intensity along the distance from the wound healing edge, a flat curve was obtained at the 0 h time point; however, after 24-h migration, a significant increase of lamin A was observed closer to the scratch edge of the migration front (Fig. 2b). To further demonstrate the correlation between lamin A heterogeneity and cell motility, we performed live cell imaging to track the cell migration at single-cell resolution (ESI Movie S1†) and conducted immunostaining at the last point of the live cell tracking to correlate the lamin A levels of the individual cells (Fig. 2c). Consistently, at the single cell level, most of the cells near the wound edge showed significantly higher lamin A levels compared to those further away from the wound after overnight migration (0–150 μm: 1187.24 ± 425.20, N = 113 cells; 150–300 μm: 827.14 ± 311.58, N = 271 cells; p value <0.0001) (Fig. 2c and d). When correlating the migration rates and lamin A levels of individual cells, we found that high-lamin A cells showed higher migration rates (0.3132 ± 0.1629 μm min−1, n = 18 cells) than low-lamin A cells (0.1965 ± 0.0944 μm min−1, n = 18 cells) (Fig. 2e). More interestingly, similar to the nanopillar-guided subnuclear deformation coherency measurement in Fig. 1f, two high probability density regions with distinct migration rates were observed among the high-lamin A cells (Fig. 2e), which implies that the high-lamin A cells are also heterogeneous and possibly contain two subpopulations with different migration abilities.
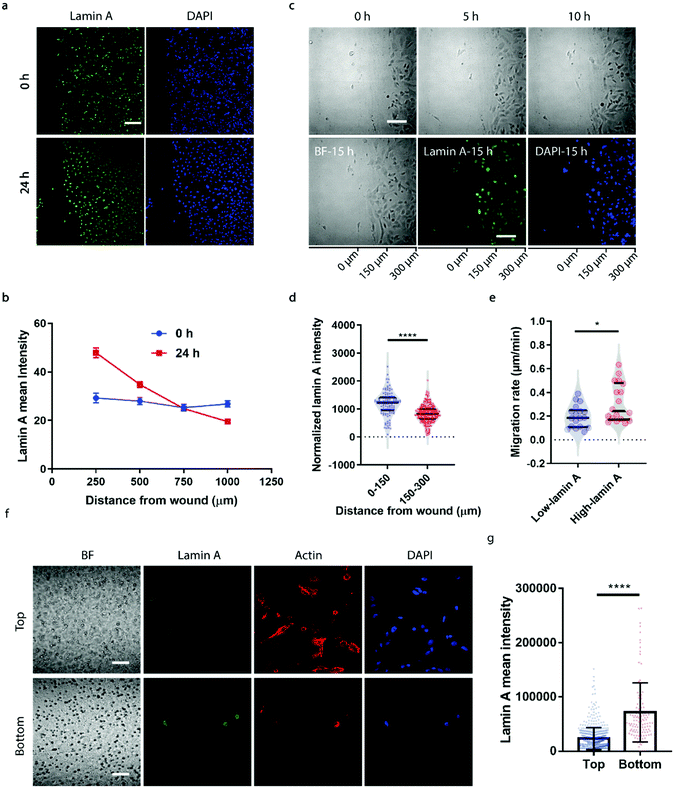 |
| Fig. 2 High lamin A levels are associated with greater metastatic potential in SK-N-SH cells. (a) Fluorescence images showing the lamin A levels of cells near the wound before and after 24-hour migration. Scale bar, 200 μm. (b) Correlation between lamin A intensity and distance from the wound before and after 24-hour migration. (c) Cell migration overnight in the wound healing assay and fluorescence images showing the lamin A levels of cells at the last time point of cell migration. The horizontal axis indicates the distance from the edge. Scale bars, 100 μm. (d) Cells closer to the edge area of the wound after overnight migration show higher lamin A intensity. (e) Comparison of the migration rates of cells showing different levels of lamin A. (f) Fluorescence images showing the lamin A levels of the cells at the top and bottom surfaces of the transwell device. Scale bars, 50 μm. (G) Cells at the bottom surface of the permeable membrane show higher lamin A levels compared with the cells remaining at the top surface. Statistical significance was evaluated by an unpaired t-test with Welch's correction. **** P < 0.0001; * P < 0.05. | |
Next, the transwell assay was performed to verify the invasion ability of cells with different lamin A-expression levels, which correlates with the metastatic potential of the cell. SK-N-SH cells were seeded on the top of a transwell device with 5 μm pore size. Cells were driven to migrate through the porous membrane by the differential serum concentrations and were allowed to penetrate the membrane for 2 hours, where cells reaching the bottom membrane are regarded as more invasive compared to cells that are retained on the top membrane. Interestingly, as shown in Fig. 2f and g, cells located at the bottom membrane show significantly higher lamin A levels than those staying at the top membrane, suggesting that the SK-N-SH cells with high motility and increased potential for metastasis have higher lamin A expression. This is consistent with our observations from the wound healing assay.
Heterogeneity of high-lamin A cells probed by nanopillar-guided subnuclear patterns
Earlier studies have shown that transient overexpression of lamin A often leads to decreased cell migration.45 Interestingly, we observed the opposite trend. Higher migration capacity was seen in cells with endogenously high lamin A levels, as shown in Fig. 2. This raises the question of whether this inconsistency is caused by heterogeneity within the population of high-lamin A cells. As shown in Fig. 1f, two subpopulations among the high-lamin A cells can be clearly differentiated based on their nanopillar-guided deformation coherency. Therefore, we hypothesized that the migratability can be more faithfully reflected by the deformation pattern on the nanopillars. To prove this, we compared the identified high-lamin A cells with and without transient overexpression of lamin A/C-EGFP (Fig. 3a). Interestingly, the cells with lamin A/C overexpression generated isotropic ring deformations on the nanopillars, which has been observed to result in slow migration in breast cancer cells.35 Statistical analysis further showed a significant decrease (p value = 0.0230) of deformation coherency in the transfected cells (0.2735 ± 0.1703, n = 62 pillars), so that no more of the distinguishable subpopulation with higher coherency was left compared to non-transfected cells (0.3525 ± 0.1838, n = 48 pillars) (Fig. 3b). This strongly implies that the different motilities in high-lamin A cells are better correlated with the deformation coherency on the nanopillars, where cells with low coherency, i.e. ring deformation on the nanopillars, are slow to migrate. To be consistent with the results from the immunostaining of lamin A instead of lamin A/C, we overexpressed lamin A-GFP in SK-N-SH as well and characterized the deformation coherency. As shown in Fig. S2a,† lamin A overexpression leads to formation of ring-like subnuclear patterns on the nanopillars that are similar to those of the low-lamin A cells and lamin A/C-overexpressed cells. Quantitative measurement of the deformation coherency (Fig. S2b†) further confirms that the lamin A overexpression decreases the coherency compared with non-transfected high-lamin A cells, similarly to lamin A/C overexpression.
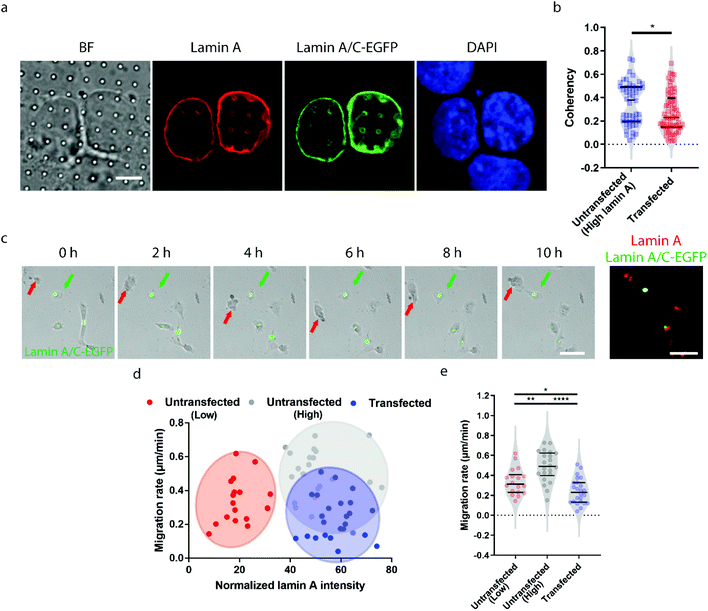 |
| Fig. 3 Heterogeneity in high-lamin A cells revealed by nanopillar-guided subnuclear patterns. (a) Fluorescence images showing ring deformation patterns in SK-N-SH cells transfected with lamin A/C-EGFP. Scale bar, 5 μm. (b) Anisotropy measurements of the nanopillar-guided subnuclear features in both untransfected and transfected cells. (c) Live cell imaging showing overnight migration of transfected and untransfected cells and fluorescence images showing lamin A and lamin A/C-EGFP signals in cells at the last point of cell migration. The green arrows indicate transfected cells, whereas the red arrows indicate untransfected cells. Scale bars, 50 μm. (d) Correlation between the migration rate and lamin A level at the single cell level for both untransfected and transfected cells. (e) Comparison of the migration rate between untransfected cells, including low and high lamin A cells, and transfected cells. Statistical significance was evaluated by an unpaired t-test with Welch's correction. **** P < 0.0001; ** P < 0.01; * P < 0.05. | |
To verify the effects of lamin A/C overexpression on cell motility, live cell imaging was performed overnight to track the migration of both untransfected and transfected cells, and the lamin A level of individual cells were probed by immunostaining at the last time point (Fig. 3c and ESI Movie S2†). By correlating the migration rate with the lamin A level in individual cells, we found that non-transfected cells with endogenous high lamin A levels tended to migrate faster than those with low lamin A levels (Fig. 3d), which is in agreement with the results shown in Fig. 2. In comparison, most of the transfected cells with overexpressed high lamin A migrate slower than those with endogenous high lamin A without transfection (Fig. 3d and e). More interestingly, the transfected cells (0.2407 ± 0.1275 μm min−1, n = 24 cells) even migrated slower compared with untransfected cells with endogenously low lamin A levels (0.3382 ± 0.1310 μm min−1, n = 18 cells) (Fig. 3e); this suggests the strong impact of lamin A overexpression on cell motility, which is consistent with the coherency measurement on the nanopillars. These results demonstrated that the nanopillar-probed deformation coherency reveals better heterogeneity among neuroblastoma cells, and it also correlates with one of the key indicators of metastasis, the cell migration speed.
Subnuclear deformation patterns on nanopillar arrays correlates with EMT
It has been well established that cancer cells typically acquire a migratory phenotype via epithelial-to-mesenchymal transition (EMT) throughout metastatic progression.46 We hypothesize that EMT is responsible for the increased cell motility and invasiveness in high-lamin A cells. Vimentin was selected among the EMT markers in this study due to its reported contribution to preserving nuclear integrity.47 On the flat surface, the vimentin level shows a strong dependence on the lamin A level, as obvious vimentin filaments were observed in cells with high lamin A, whereas negligible vimentin signals could be found in low-lamin A cells (Fig. 4a). In agreement with this observation, vimentin intensity was positively correlated with lamin A level in neuroblastoma cells (Fig. 4b). Similarly, the cell lamin A level was positively correlated with another well-established EMT marker, SNAIL + SLUG, on a flat surface (Fig. 4a and c). On nanopillar arrays, strong vimentin as well as SNAIL + SLUG signals were found in cells with aligned deformation patterns across nearby pillars, while a weak vimentin signal was observed in cells showing subnuclear ring features (Fig. 4d). Coherency measurements further revealed a monotonic correlation with the vimentin and SNAIL + SLUG intensities in the same cell (Fig. 4e and f). Interestingly, this suggests that the nanopillar-guided deformation pattern is a valuable indicator of EMT during metastasis development.
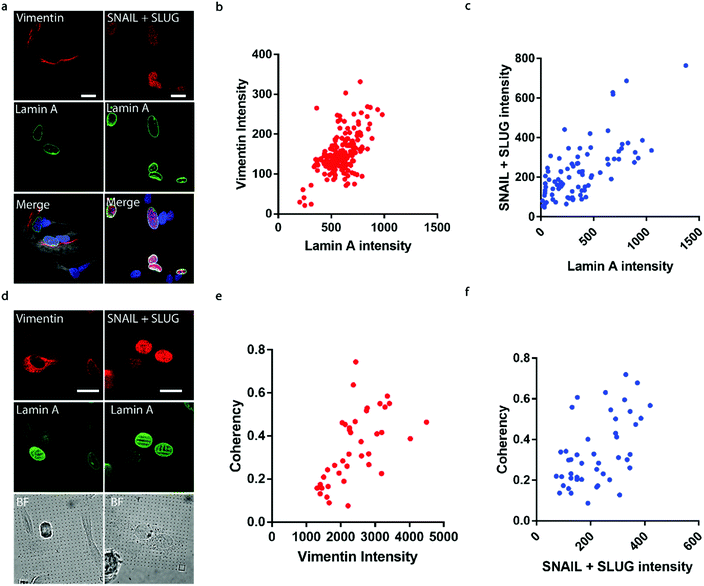 |
| Fig. 4 Subnuclear irregularities and lamin A levels correlate with EMT. (a) Fluorescence images showing that cells with high lamin A show strong vimentin and SNAIL + SLUG signals. Scale bar, 10 μm. (b) Correlation between the lamin A levels and vimentin levels in individual SK-N-SH cells. (c) Correlation between the lamin A levels and SNAIL + SLUG levels in individual SK-N-SH cells. (d) Fluorescence images showing that cells with guided nuclear grooves show stronger vimentin and SNAIL + SLUG signals than those exhibiting subnuclear ring features on nanopillar arrays. Scale bar, 10 μm. (e) Correlation between the anisotropicity of guided nuclear irregularities and the vimentin levels in individual SK-N-SH cells. (f) Correlation between the anisotropicity of guided nuclear irregularities and the SNAIL + SLUG levels in individual SK-N-SH cells. | |
Assessing anti-cancer drug effects via subnuclear anisotropicity on nanopillars
A growing demand for probing heterogeneity in neuroblastoma cells comes from anti-cancer drug development, as the precise characterization of the drug response among different subpopulations may facilitate the identification of drugs more effectively targeting metastatic cells to improve prognosis and prevent relapse. Here, we chose two drugs widely used for the treatment of neuroblastoma, doxorubicin (DOX, 1 μM) and etoposide (Etop, 10 μM).48,49 When neuroblastoma cells with various levels of endogenous lamin A were treated with either DOX or Etop for 24 h, it was interesting to observe that most of the cells that remained attached on the surface exhibited significantly high lamin A levels (Fig. 5a and b). It may be that the drug treatment upregulated the lamin A expression. However, it is not clear whether individual cells with potentially upregulated lamin A after drug treatment formed similar ring deformation patterns to those of the over-expressed cells shown in Fig. 3a, or line patterns similar to those of the endogenous high-lamin A cells shown in Fig. 1e. We therefore examined the nanopillar-guided subnuclear deformation patterns upon treatment. Strikingly, compared to the more common line features observed in the control group, cells treated with either DOX or Etop tended to develop more isotropic ring features on nanopillar arrays despite their high lamin A levels (Fig. 5c). As a result, the pillar coherency of the treated cells (DOX: 0.3057 ± 0.1862, n = 38 pillars; Etop: 0.2606 ± 0.1564, n = 44 pillars) is significantly lower (DOX: p value = 0.0344; Etop: p value = 0.0002) than that of the DMSO control group (0.3853 ± 0.1448, n = 47 pillars) (Fig. 5d). Consistently, the fraction of cells with dominant isotropic ring features and low deformation coherency increased upon anti-cancer drug treatment (DMSO: 0.262 ± 0.160, n = 30 cells; DOX: 0.607 ± 0.152, n = 22 cells; Etop: 0.722 ± 0.079, n = 24 cells) (Fig. 5e). Since isotropic ring fractions are more associated with fewer EMT markers and slower migration, as shown earlier, their increased fraction upon drug treatment suggests that although the high-lamin A cells survived the drug treatment, their metastasis ability was significantly reduced. Together, this demonstrates that deformation coherency can be employed as a highly sensitive indicator of anti-cancer drug efficacy in neuroblastoma cells.
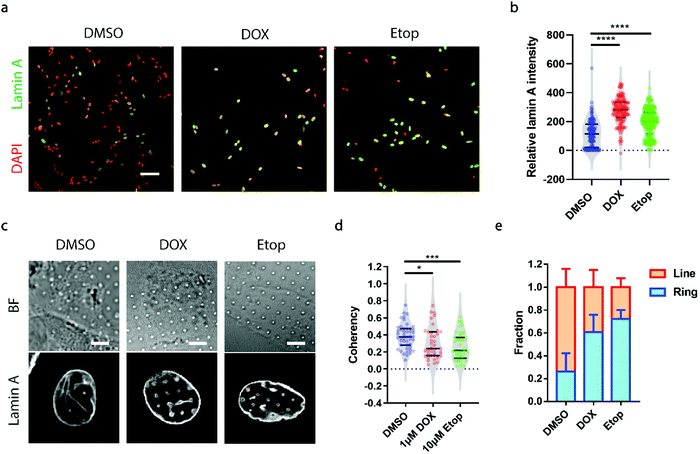 |
| Fig. 5 Probe of the effects of anti-cancer drugs on neuroblastoma cells via subnuclear anisotropicity on nanopillars. (a) Fluorescence images showing that anti-cancer drug treatment leads to increased lamin A levels in SK-N-SH cells. Scale bar, 100 μm. (b) Lamin A intensity measurements revealed that cells treated with anti-cancer drugs had significantly higher lamin A levels than those treated with DMSO. (c) Nanopillar-guided subnuclear grooves decreased upon anti-cancer drug treatment. Scale bars, 5 μm. (d) Anisotropy measurements of the nanopillar-guided subnuclear features in cells treated with DMSO, 1 μM DOX and 10 μM Etop. (e) Fractions of ring deformation and line deformations in cells treated with DMSO, 1 μM DOX and 10 μM Etop. Statistical significance was evaluated by an unpaired t-test with Welch's correction. *** P < 0.001; * P < 0.05. | |
Conclusion
In this study, we demonstrated that nanopillar-guided subnuclear deformation patterns can be used as an effective marker to characterize the heterogeneity in neuroblastoma cells. In addition to various lamin A expression levels widely reported in neuroblastoma, we successfully further differentiated cells with similar lamin A levels into subpopulations depending on the orientation coherency of their deformation features on nanopillars. High deformation coherency was found to exist at a higher percentage in high-lamin A cells with faster migration speed, and it was positively correlated with EMT marker increase. These results demonstrated that risk stratification of individual neuroblastoma cells can be achieved via quantitative assessment of the subnuclear irregularities guided by nanopillar arrays. More importantly, the subtle response of drug-resistant high-lamin A cells can be sensitively reflected through the changes in deformation patterns and can be quantitatively read out using coherency value measurements. We envision that this nuclear irregularities-based risk stratification of neuroblastoma cells will open up new avenues to improve cancer therapy.
Materials and methods
Fabrication and characterization of nanopillar arrays
SiO2 nanopillar arrays were fabricated via electron-beam lithography (EBL) and anisotropic dry etching. The surface was firstly rinsed with acetone and isopropyl alcohol (IPA). Thin layers of polymethylmethacrylate (PMMA) (MicroChem) and a conductive polymer, AR-PC 5090.02 (Allresist), were then respectively spin-coated on the cleaned chip. EBL (FEI Helios NanoLab 650) was subsequently conducted to write different patterns, which decides the diameter and pitch of the nanopillars, on the chip surface. The PMMA at the exposed areas was dissolved in the development solution (3
:
1 isopropanol
:
methylisobutylketone), followed by chromium (Cr) deposition by thermal evaporation (UNIVEX 250 Benchtop). Cr masks at the written areas remained at the chip surface after lift-off with acetone, and nanostructures were fabricated through reactive ion etching with a mixture of CF4 and CHF3 (Oxford Plasmalab 80). SEM characterization (FEI Helios NanoLab 650) of the nanostructures was carried out after 10 nm Cr deposition.
Cell culture and drug treatment
The nanopillar arrays were cleaned and sterilized by air plasma and UV exposure before cell culture. Each nanochip was then coated with fibronectin (2 μg ml−1, Sigma-Aldrich) for 30 minutes at 37 °C. The SK-N-SH cells were seeded on the nanostructures after coating and grown in Eagle's minimum essential medium (MEM) supplemented with 2 mM L-glutamine (Gibco), 1% MEM non-essential amino acids (NEAA) (Gibco), 10% fetal bovine serum (FBS) (Life Technologies) and 100 U ml−1 penicillin and 100 mg ml−1 streptomycin (Life Technologies) in a standard incubator at 37 °C with 5% CO2. Drug treatment was performed after overnight incubation; the SK-N-SH cells on the nanostructures were treated with etoposide (ETOP) (Sigma), doxorubicin (DOX) (Sigma) or dimethyl sulfoxide (DMSO) (Sigma). After 24-hour incubation, the treated cells and untreated cells were fixed with 4% paraformaldehyde (PFA) solution in PBS (Boster Biological Technology, AR1068) for 15 minutes for subsequent immunostaining.
Immunostaining
The nuclear morphologies of the SK-N-SH cells were visualized by immunostaining with lamin A and lamin B1. The lamin A and vimentin levels in the cells were characterized by immunostaining of lamin A and vimentin. Cells were cultured on nanopillars and incubated overnight before fixation with pre-warmed 4% paraformaldehyde (PFA) in PBS (Boster biological technology AR1068) for 15 minutes at room temperature. After washing with PBS three times, the cells were permeabilized in 0.5% Triton X-100 (Sigma) in PBS for 15 minutes, followed by blocking with 5% bovine serum albumin (BSA) (Sigma) in PBS for 1 hour. Subsequently, the samples were incubated with primary antibodies (anti-lamin A, Abcam, ab26300; anti-lamin B1, Abcam, ab16048; anti-vimentin, Sigma, V5255; anti-SNAIL + SLUG, Abcam, ab224731) at 1
:
400 dilution at room temperature for 1 hour or 4 °C overnight. The samples were again washed with PBS for three times and then incubated with secondary antibodies (chicken anti-rabbit IgG Alexa 488, Invitrogen, A21441; anti-mouse IgG Alexa 555, Cell Signaling Technology, 4409s) at 1
:
600 dilution at room temperature for 1 hour. After washing with PBS three times, the samples were stained with DAPI (Sigma), phalloidin (Cytoskeleton, Inc.) or cellmask (Invitrogen) in different experiments.
Confocal imaging
Fluorescence imaging and live cell imaging were performed by confocal microscopy (Zeiss LSM 800 with Airyscan or a spinning disc confocal microscope (SDC) that was built around a Nikon Ti2 inverted microscope equipped with a Yokogawa CSU-W1 confocal spinning head). Specifically, the nuclear morphologies of individual cells and the cells in the transwell experiments were captured in the Zeiss LSM 800 with Airyscan. The live cell imaging and subsequent fluorescence imaging were performed using the SDC, and the image acquisition and processing were controlled by MetaMorph (Molecular Device) software.
Quantification of nanopillar-guided subnuclear irregularities
The guided subnuclear irregularities were characterized by measurement of their anisotropicity. Firstly, one image with the sharpest guided features in one stack was selected for subsequent characterization. Square masks (2.75 μm × 2.75 μm, 11 pixels × 11 pixels) were manually drawn based on the location of the nanopillars in the brightfield channel. After thresholding the cropped images of the lamin A channel using the masks, the coherency, an indicator of the anisotropy properties, of the nanopillar-guided nuclear features was measured using orientationJ (a plugin function in ImageJ).
Transfection
For lamin A-GFP and lamin A/C-EGFP transfection, SK-N-SH cells were firstly starved in Opti-MEM (Gibco) medium for 30 min at 37 °C. The transfection mixture was prepared by mixing 1 μg plasmid and 1.5 μl Lipofectamine 3000 (Life Technologies) in Opti-MEM and incubating for 20 min at room temperature. After 4-hour incubation with the transfection mixture, the Opti-MEM medium was replaced with culture medium, and the cells were allowed to recover overnight before imaging.
Wound healing assay
SK-N-SH cells were first cultured in 35 mm dishes until they were approximately 90% confluent. Scratches were then made in the confluent monolayer of cells using a sterile 200 μl pipette tip, and the culture medium was replaced. Brightfield microscopic pictures were taken of the same field at 0 hours and 24 hours. The migration rate was measured by the difference in the closure area within the same time period using Image J.
Transwell assay
The SK-N-SH cells were seeded on the top membrane with 5 μm pore size of a transwell device (Corning) and maintained in culture medium without serum. Complete medium with 10% FBS (Life Technologies) was used as the chemoattractant and placed in the lower chamber. After incubation for 2 hours, the cells were fixed and stained, and the distribution of the lamin A levels of the cells was examined under confocal microscopy.
Statistical analysis
Welch's t tests (unpaired, 2 tailed, not assuming equal SD) were used to evaluate the significance. All tests were performed using Prism (GraphPad Software). Data are presented as mean ± SEM or mean ± SD as stated in the figure captions. All experiments were repeated at least twice.
Data availability
The data that support the findings of this study are available from the corresponding authors upon reasonable request.
Author contributions
Y. P. Z., R. P. D. and W. Z. conceived the idea and designed the experiments. Y. P. Z. and R. P. D. performed most of the experiments and data analysis. Y. P. Z. and W. G. performed fabrication and SEM experiments. Y. P. Z. and W. Z. drafted the manuscript. All of the authors discussed and commented on the manuscript.
Conflicts of interest
Y. P. Z. and W. Z. are inventors on a pending patent related to this work filed by Nanyang Technological University (no. PCT/SG2021/050687, filed on 10 November 2021). The authors declare that they have no other competing interests.
Acknowledgements
We thank the Luo lab in University of Macau for providing the SK-N-SH cells, the Saggio lab in Sapienza University of Rome for providing the lamin B1 antibody, the Chen lab in Nanyang Technological university (NTU) for the access to the plasma cleaner, the Miao lab in the School of Biological Science and the central facility of the School of Chemical and Biomedical Engineering in NTU for the confocal microscope access, and Nanyang NanoFabrication Center (N2FC) and the Centre of Disruptive Photonic Technologies (CDPT) in NTU for supporting the nanopillar chip fabrication and characterization. Fig. 1d was created with BioRender.com. This work is supported by the Singapore Ministry of Education (MOE) (W. Zhao, RG145/18 and RG112/20), the Singapore National Research Foundation (W. Zhao, NRF2019-NRF-ISF003-3292), the NTU Start-up Grant (W. Zhao) and NTU-NNI Neurotechnology Fellowship (W. Zhao).
References
- W. B. London, V. Castel, T. Monclair, P. F. Ambros, A. D. J. Pearson, S. L. Cohn, F. Berthold, A. Nakagawara, R. L. Ladenstein, T. Iehara and K. K. Matthay, J. Clin. Oncol., 2011, 29, 3286–3292 CrossRef PubMed.
- J. M. Maris, M. D. Hogarty, R. Bagatell and S. L. Cohn, Lancet, 2007, 369, 2106–2120 CrossRef CAS PubMed.
- G. M. Brodeur, J. Pritchard, F. Berthold, N. L. Carlsen, V. Castel, R. P. Castelberry, B. De Bernardi, A. E. Evans, M. Favrot and F. Hedborg, J. Clin. Oncol., 1993, 11, 1466–1477 CrossRef CAS.
- H. Shimada, I. M. Ambros, L. P. Dehner, J. Hata, V. V. Joshi, B. Roald, D. O. Stram, R. B. Gerbing, J. N. Lukens, K. K. Matthay and R. P. Castleberry, Cancer, 1999, 86, 364–372 CrossRef CAS.
- G. M. Brodeur, R. C. Seeger, M. Schwab, H. E. Varmus and J. M. Bishop, Science, 1984, 224, 1121–1124 CrossRef CAS.
- R. C. Seeger, G. M. Brodeur, H. Sather, A. Dalton, S. E. Siegel, K. Y. Wong and D. Hammond, N. Engl. J. Med., 1985, 313, 1111–1116 CrossRef CAS PubMed.
- A. T. Look, F. A. Hayes, R. Nitschke, N. B. McWilliams and A. A. Green, N. Engl. J. Med., 1984, 311, 231–235 CrossRef CAS.
- L. J. Valentijn, J. Koster, F. Haneveld, R. A. Aissa, P. van Sluis, M. E. C. Broekmans, J. J. Molenaar, J. van Nes and R. Versteeg, Proc. Natl. Acad. Sci. U. S. A., 2012, 109, 19190–19195 CrossRef CAS.
- T. J. Pugh, O. Morozova, E. F. Attiyeh, S. Asgharzadeh, J. S. Wei, D. Auclair, S. L. Carter, K. Cibulskis, M. Hanna, A. Kiezun, J. Kim, M. S. Lawrence, L. Lichenstein, A. McKenna, C. S. Pedamallu, A. H. Ramos, E. Shefler, A. Sivachenko, C. Sougnez, C. Stewart, A. Ally, I. Birol, R. Chiu, R. D. Corbett, M. Hirst, S. D. Jackman, B. Kamoh, A. H. Khodabakshi, M. Krzywinski, A. Lo, R. A. Moore, K. L. Mungall, J. Qian, A. Tam, N. Thiessen, Y. Zhao, K. A. Cole, M. Diamond, S. J. Diskin, Y. P. Mosse, A. C. Wood, L. Ji, R. Sposto, T. Badgett, W. B. London, Y. Moyer, J. M. Gastier-Foster, M. A. Smith, J. M. Guidry Auvil, D. S. Gerhard, M. D. Hogarty, S. J. M. Jones, E. S. Lander, S. B. Gabriel, G. Getz, R. C. Seeger, J. Khan, M. A. Marra, M. Meyerson and J. M. Maris, Nat. Genet., 2013, 45, 279–284 CrossRef CAS PubMed.
- I. M. Ambros, J. Hata, V. V. Joshi, B. Roald, L. P. Dehner, H. Tüchler, U. Pötschger and H. Shimada, Cancer, 2002, 94, 1574–1583 CrossRef PubMed.
- D. Zink, A. H. Fischer and J. A. Nickerson, Nat. Rev. Cancer, 2004, 4, 677–687 CrossRef CAS PubMed.
- G. Bussolati, C. Marchiò, L. Gaetano, R. Lupo and A. Sapino, J. Cell. Mol. Med., 2008, 12, 209–218 CrossRef.
- K.-H. Chow, R. E. Factor and K. S. Ullman, Nat. Rev. Cancer, 2012, 12, 196–209 CrossRef CAS.
- A. Batistatou and C. D. Scopa, Int. J. Surg. Pathol., 2009, 17, 107–110 CrossRef.
- J. I. de Las Heras and E. C. Schirmer, Adv. Exp. Med. Biol., 2014, 773, 5–26 CrossRef.
- D. Gisselsson, J. Björk, M. Höglund, F. Mertens, P. Dal Cin, M. Akerman and N. Mandahl, Am. J. Pathol., 2001, 158, 199–206 CrossRef CAS.
- C. Xie, Z. Lin, L. Hanson, Y. Cui and B. Cui, Nat. Nanotechnol., 2012, 7, 185–190 CrossRef CAS PubMed.
- J. L. Tan, J. Tien, D. M. Pirone, D. S. Gray, K. Bhadriraju and C. S. Chen, Proc. Natl. Acad. Sci. U. S. A., 2003, 100, 1484–1489 CrossRef CAS PubMed.
- J.-Y. Shiu, L. Aires, Z. Lin and V. Vogel, Nat. Cell Biol., 2018, 20, 262–271 CrossRef CAS PubMed.
- X. Li, L. H. Klausen, W. Zhang, Z. Jahed, C.-T. Tsai, T. L. Li and B. Cui, Nano Lett., 2021, 21, 8518–8526 CrossRef CAS.
- Y.-R. Na, S. Y. Kim, J. T. Gaublomme, A. K. Shalek, M. Jorgolli, H. Park and E. G. Yang, Nano Lett., 2013, 13, 153–158 CrossRef CAS.
- C. Chiappini, J. O. Martinez, E. De Rosa, C. S. Almeida, E. Tasciotti and M. M. Stevens, ACS Nano, 2015, 9, 5500–5509 CrossRef CAS.
- B. K. K. Teo, S.-H. Goh, T. S. Kustandi, W. W. Loh, H. Y. Low and E. K. F. Yim, Biomaterials, 2011, 32, 9866–9875 CrossRef CAS PubMed.
- A. R. K. Kumar, Y. Shou, B. Chan, K. L. and A. Tay, Adv. Mater., 2021, 33, e2007421 CrossRef PubMed.
- B. Yin, L. W. C. Ho, S. Liu, H. Hong, X. Y. Tian, H. Li and C. H. J. Choi, Nano Lett., 2021, 21, 1839–1847 CrossRef CAS PubMed.
- V. Bhingardive, A. Kossover, M. Iraqi, B. Khand, G. Le Saux, A. Porgador and M. Schvartzman, Nano Lett., 2021, 21, 4241–4248 CrossRef CAS.
- W. Zhao, L. Hanson, H.-Y. Lou, M. Akamatsu, P. D. Chowdary, F. Santoro, J. R. Marks, A. Grassart, D. G. Drubin, Y. Cui and B. Cui, Nat. Nanotechnol., 2017, 12, 750–756 CrossRef CAS PubMed.
- H. Yurugi, Y. Zhuang, F. A. Siddiqui, H. Liang, S. Rosigkeit, Y. Zeng, H. Abou-Hamdan, E. Bockamp, Y. Zhou, D. Abankwa, W. Zhao, L. Désaubry and K. Rajalingam, J. Cell Sci., 2020, 133, jcs244111 CrossRef CAS PubMed.
- M. Su, Y. Zhuang, X. Miao, Y. Zeng, W. Gao, W. Zhao and M. Wu, iScience, 2020, 23, 101712 CrossRef CAS PubMed.
- H.-Y. Lou, W. Zhao, Y. Zeng and B. Cui, Acc. Chem. Res., 2018, 51, 1046–1053 CrossRef CAS.
- H. Liang, H. Mu, F. Jean-Francois, B. Lakshman, S. Sarkar-Banerjee, Y. Zhuang, Y. Zeng, W. Gao, A. M. Zaske, D. V. Nissley, A. A. Gorfe, W. Zhao and Y. Zhou, Life Sci. Alliance, 2019, 2, e201900343 CrossRef PubMed.
- H.-Y. Lou, W. Zhao, X. Li, L. Duan, A. Powers, M. Akamatsu, F. Santoro, A. F. McGuire, Y. Cui, D. G. Drubin and B. Cui, Proc. Natl. Acad. Sci. U. S. A., 2019, 116, 23143–23151 CrossRef CAS PubMed.
- L. Hanson, W. Zhao, H.-Y. Lou, Z. C. Lin, S. W. Lee, P. Chowdary, Y. Cui and B. Cui, Nat. Nanotechnol., 2015, 10, 554–562 CrossRef CAS PubMed.
- C. S. Hansel, S. W. Crowder, S. Cooper, S. Gopal, M. João Pardelha da Cruz, L. de Oliveira Martins, D. Keller, S. Rothery, M. Becce, A. E. G. Cass, C. Bakal, C. Chiappini and M. M. Stevens, ACS Nano, 2019, 13, 2913–2926 CrossRef CAS PubMed.
-
Y. Zeng, Y. Zhuang, A. Mitra, P. Chen, I. Saggio, G. V. Shivashankar and W. Gao, 2022, bioRxiv, DOI:10.1101/2022.01.30.478168.
- G. Maresca, M. Natoli, M. Nardella, I. Arisi, D. Trisciuoglio, M. Desideri, R. Brandi, S. D'Aguanno, M. R. Nicotra, M. D'Onofrio, A. Urbani, P. G. Natali, D. Del Bufalo, A. Felsani and I. D'Agnano, PLoS One, 2012, 7, e45513 CrossRef CAS.
- J. Lammerding, P. C. Schulze, T. Takahashi, S. Kozlov, T. Sullivan, R. D. Kamm, C. L. Stewart and R. T. Lee, J. Clin. Invest., 2004, 113, 370–378 CrossRef CAS.
- P. M. Davidson and J. Lammerding, Trends Cell Biol., 2014, 24, 247–256 CrossRef CAS.
- J. Lammerding, L. G. Fong, J. Y. Ji, K. Reue, C. L. Stewart, S. G. Young and R. T. Lee, J. Biol. Chem., 2006, 281, 25768–25780 CrossRef CAS PubMed.
- J. D. Pajerowski, K. N. Dahl, F. L. Zhong, P. J. Sammak and D. E. Discher, Proc. Natl. Acad. Sci. U. S. A., 2007, 104, 15619–15624 CrossRef CAS PubMed.
- D. Constantinescu, H. L. Gray, P. J. Sammak, G. P. Schatten and A. B. Csoka, Stem Cells, 2006, 24, 177–185 CrossRef CAS PubMed.
- L. Vergnes, M. Péterfy, M. O. Bergo, S. G. Young and K. Reue, Proc. Natl. Acad. Sci. U. S. A., 2004, 101, 10428–10433 CrossRef CAS PubMed.
- E. Fonck, G. G. Feigl, J. Fasel, D. Sage, M. Unser, D. A. Rüfenacht and N. Stergiopulos, Stroke, 2009, 40, 2552–2556 CrossRef CAS PubMed.
- J. Schindelin, I. Arganda-Carreras, E. Frise, V. Kaynig, M. Longair, T. Pietzsch, S. Preibisch, C. Rueden, S. Saalfeld, B. Schmid, J.-Y. Tinevez, D. J. White, V. Hartenstein, K. Eliceiri, P. Tomancak and A. Cardona, Nat. Methods, 2012, 9, 676–682 CrossRef CAS PubMed.
- I. Rauschert, F. Aldunate, J. Preussner, M. Arocena-Sutz, V. Peraza, M. Looso, J. C. Benech and R. Agrelo, PLoS One, 2017, 12, e0175953 CrossRef PubMed.
- T. Brabletz, R. Kalluri, M. A. Nieto and R. A. Weinberg, Nat. Rev. Cancer, 2018, 18, 128–134 CrossRef PubMed.
- A. E. Patteson, A. Vahabikashi, K. Pogoda, S. A. Adam, K. Mandal, M. Kittisopikul, S. Sivagurunathan, A. Goldman, R. D. Goldman and P. A. Janmey, J. Cell Biol., 2019, 218, 4079–4092 CrossRef CAS PubMed.
- R. Ladenstein, U. Pötschger, A. D. J. Pearson, P. Brock, R. Luksch, V. Castel, I. Yaniv, V. Papadakis, G. Laureys, J. Malis, W. Balwierz, E. Ruud, P. Kogner, H. Schroeder, A. F. de Lacerda, M. Beck-Popovic, P. Bician, M. Garami, T. Trahair, A. Canete, P. F. Ambros, K. Holmes, M. Gaze, G. Schreier, A. Garaventa, G. Vassal, J. Michon, D. Valteau-Couanet and SIOP Europe Neuroblastoma Group (SIOPEN), Lancet Oncol., 2017, 18, 500–514 CrossRef CAS PubMed.
- X. Bian, L. M. McAllister-Lucas, F. Shao, K. R. Schumacher, Z. Feng, A. G. Porter, V. P. Castle and A. W. Opipari, J. Biol. Chem., 2001, 276, 48921–48929 CrossRef CAS PubMed.
Footnote |
† Electronic supplementary information (ESI) available. See DOI: 10.1039/d1nr04996h |
|
This journal is © The Royal Society of Chemistry 2022 |
Click here to see how this site uses Cookies. View our privacy policy here.