DOI:
10.1039/D3LC01027A
(Critical Review)
Lab Chip, 2024,
24, 3199-3225
Bridging barriers: advances and challenges in modeling biological barriers and measuring barrier integrity in organ-on-chip systems
Received
1st December 2023
, Accepted 23rd April 2024
First published on 1st May 2024
Abstract
Biological barriers such as the blood–brain barrier, skin, and intestinal mucosal barrier play key roles in homeostasis, disease physiology, and drug delivery – as such, it is important to create representative in vitro models to improve understanding of barrier biology and serve as tools for therapeutic development. Microfluidic cell culture and organ-on-a-chip (OOC) systems enable barrier modelling with greater physiological fidelity than conventional platforms by mimicking key environmental aspects such as fluid shear, accurate microscale dimensions, mechanical cues, extracellular matrix, and geometrically defined co-culture. As the prevalence of barrier-on-chip models increases, so does the importance of tools that can accurately assess barrier integrity and function without disturbing the carefully engineered microenvironment. In this review, we first provide a background on biological barriers and the physiological features that are emulated through in vitro barrier models. Then, we outline molecular permeability and electrical sensing barrier integrity assessment methods, and the related challenges specific to barrier-on-chip implementation. Finally, we discuss future directions in the field, as well important priorities to consider such as fabrication costs, standardization, and bridging gaps between disciplines and stakeholders.
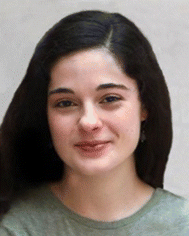 Alisa Ugodnikov | Alisa Ugodnikov received her B.Sc. in Pharmacology & Biomedical Toxicology and Economics from the University of Toronto in 2017 and her PhD from the Institute of Biomedical Engineering at the University of Toronto in 2023. In 2015–2016 she completed a research training year in biomedical engineering at Harvard Medical School, with a focus on vascular models of inflammation and organ-on-chip. Her current research interests include biosensors, biological barrier modeling, microfluidics, and organ-on-chip. |
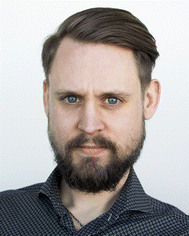 Henrik Persson | Henrik Persson received his M.Sc. in Engineering Nanoscience from Lund University, Sweden, where he was later awarded a PhD in physics. After a 2 year postdoc stay at the Medical Faculty in Lund, he joined Craig Simmons' biomedical engineering group at University of Toronto as a postdoctoral fellow. He now works at Aspect Biosystems, a bioprinting company in Vancouver, BC, Canada. His research interests include microfluidics, organ on a chip and advanced in vitro models for drug development. |
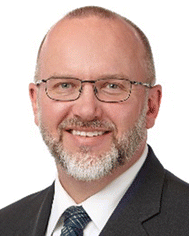 Craig Simmons | Craig Simmons is the University of Toronto Distinguished Professor of Mechanobiology in the Department of Mechanical & Industrial Engineering and the Institute of Biomedical Engineering and the Scientific Director of the Translational Biology and Engineering Program in the Ted Rogers Centre for Heart Research. His research interests include mechanobiology of cardiovascular diseases and regeneration, aided by microfluidic platforms. |
1. Introduction
Biological barriers – such as the blood–brain endothelial and intestinal epithelial barriers – are fundamental for homeostasis and protection against infection, controlling the movement and concentration of endogenous and exogenous substances between body compartments, and between the body and the environment.1 Various components – tissue-specific combinations of junctional proteins, transporters, supporting cells, and extracellular matrices2 – come together to form these barriers, which beyond maintaining concentration gradients and keeping out pathogens, are also critical in the distribution and absorption of pharmaceutical agents, affecting their efficacy and toxicity.1,3
Microfluidic cell culture systems, often referred to as organ-on-a-chip (OOC) systems or microphysiological systems (MPS), allow researchers to introduce key physiological aspects such as fluid shear, accurate microscale dimensions, mechanical cues, concentration gradients, extracellular matrix and geometrically defined co-culture to in vitro cell culture systems, helping bring in vitro closer to in vivo with the advantages of both. As such, OOC systems are well-suited for modeling biological barriers, and hold great promise overall for understanding fundamental biology, developing novel pharmaceuticals, and evaluating efficacy and safety prior to animal trials.4 As applied to biological barriers, OOC systems can facilitate the study of barrier formation and disruption, as well as the interaction between barrier function and physiological cues. Understanding these cellular responses requires integrated sensing that can monitor real-time changes in the barrier without disrupting the carefully engineered microenvironment.
Here, we first review biological barriers and the approaches for modeling barriers in vitro. An overview is given on the methods for barrier integrity assessment, broadly categorized into molecular permeability and electrical sensing, as well as the challenges of their implementation in barrier-on-chip platforms. Finally, we discuss important future directions for the field. For reviews of biosensing in OOC platforms more broadly, the interested reader is referred other recent articles.5–9
2. Biological barriers in vivo
2.1 Biological barrier structure and function
The key function common to all biological barriers is their ability to selectively prevent or allow transport of substances, which is enabled by various structural features summarized in Fig. 1. Within endothelial or epithelial barriers, cell–cell contact plays a key role in establishing barrier function, and is primarily accomplished through two classes of junctional complexes – adherens junctions and tight junctions.10 Adherens junctions (AJ), composed of cadherin and nexin adhesive units, mediate mechanical adhesion between neighboring cells.11 Tight junctions (TJ), on the other hand, regulate paracellular transport of ions, water and macromolecules, as well as serving a “fence” function, which enables the formation of distinct apical and basolateral domains by restricting the distribution of lipids within the membrane to maintain cell polarity.12 Of the proteins that comprise TJ – occludins, claudins, junctional adhesion molecules, and scaffolding proteins – claudins are considered essential for regulation of transport, as well as formation of the interconnecting strand networks featured in TJ nanoarchitecture.13,14 Both junction complexes associate with the actin cytoskeleton, and appear to be highly interdependent, with AJ being essential for assembly of TJ, and scaffolding protein zonula occludens-1 (ZO-1) co-localizing with AJ and TJ at different stages of junction formation.11,12 These junctions modulate the barrier through regulating paracellular and intramembrane flow of solutes,15 and their expression is important for maintaining physiological function. Indeed, disruption TJ in endothelial and epithelial barriers is correlated with negative clinical outcomes in patients (e.g., reduced expression of occludin and ZO-1 in the intestinal tract of patients with necrotizing enterocolitis, and disrupted TJ in autopsy specimens of patients with cerebral edema16) and is associated with various disease states including Crohn's disease, encephalitis, and diabetes.17 Moreover, measures of TJ expression are being explored for their potential utility as biomarkers; for example, serum levels of claudin-5 as a prognosticator for hemorrhaging after ischemic strokes and serum levels of ZO-1 as a marker to stratify sepsis severity and organ failure.16
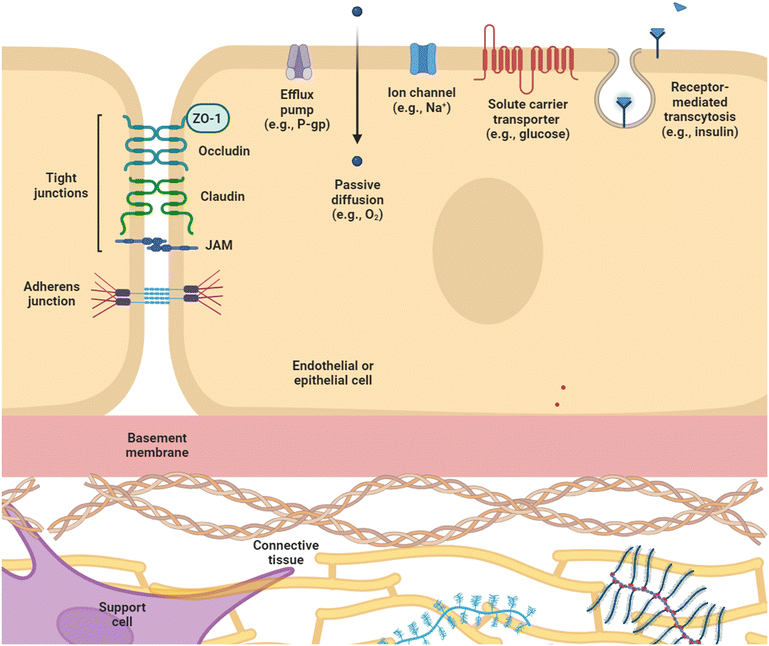 |
| Fig. 1 General biological barrier structure. Biological barriers are comprised of a variety of components that regulate paracellular and transcellular transport. Cell–cell contact between endothelial or epithelial cells is enabled through junctional complexes including tight junctions, e.g., occludin, claudin, and JAMs; adherens junctions, e.g., VE-cadherin; and scaffolding proteins, e.g., ZO-1. Barrier function is also maintained through passive diffusion, ion channels, solute carrier transporters, receptor-mediated transcytosis, and efflux pumps. Additional components contributing to barrier function include the basement membrane, connective tissue, and support cells. Created using https://www.BioRender.com. ZO-1 = zonula occludens-1; P-gp = P-glycoprotein; JAM = junctional adhesion molecule. | |
Beside paracellular transport, which is determined by cell–cell junctions, biological barriers also regulate transcellular transport. Passive diffusion across the plasma membrane is limited to lipophilic molecules smaller than 400 Da, e.g., O2, CO2.18,19 Charged species and larger molecules require carrier-mediated transport via ion channels and solute carrier transporters (SLC), which selectively allow passage of ions and molecules such as amino acids, glucose, and hormones,13,20,21 and receptor-mediated transcytosis for ligands like insulin and transferrin.22,23 Efflux transporters also contribute to barrier function by pumping molecules out of body compartments. One example is P-glycoprotein (P-gp), which is expressed in a range of tissues from the gastrointestinal tract24 and the blood–brain barrier (BBB),13 to liver, kidney, testes, and placenta.25 The dysregulated expression of P-gp, as well as multi-drug resistance proteins (MRP) and brain cancer resistance protein (BCRP) have been correlated with diseases such as the epileptic brain24 and drug resistance in cancer.20,26
However, barrier function does not only depend on proteins contained in endothelial or epithelial cell layers. Other cell types support barrier function through physical and biochemical means. The brain microvascular system, for example, is coordinated by highly structured neurovascular units (NVU), which contain multiple cell types including vascular cells (endothelial cells (ECs), pericytes, smooth muscle cells), glia (astrocytes, oligodendrocytes, microglia), and neurons.13 Within the NVU, the unique morphology of brain microvascular ECs as well as underlying basement membrane, tightly associated pericytes, and surrounding astrocytes come together to form the BBB, the structural and functional barrier between the central nervous system (CNS) and the blood stream.14 Astrocyte end-feet span and pericytes wrap around the ECs of the BBB13 to physically modulate barrier resistance, while other supporting cells exert their effect through signaling molecules, such as enterochromaffin cells in the intestine secreting serotonin, a molecule suggested to reinforce the intestinal epithelial barrier through modulating ZO-1 distribution.27 Even non-human cells, e.g., commensal bacteria, contribute to barrier formation, with a number of probiotics being implicated in improving intestinal barrier function in pathological conditions.28 Extracellular matrix (ECM)13,29 and mucus layers28,30 provide additional contributions to barrier function.
Many disease states are correlated with enhancement of barrier permeability by dysregulated tissue structure, e.g., the BBB in Azheimer's and Parkinson's diseases,13 the tympanic membrane in otitis media,31 and both affected and unaffected areas of skin in atopic dermatitis.32 Notably, while many pathologies are related to disrupted barrier integrity, not all disease states result in increased permeability – for example P-gp is upregulated in the epileptic brain.24 The development of therapeutics for such diseases must therefore consider barrier function in the design of both molecular structures and delivery mechanisms. The BBB, for example, prevents up to 98% of small molecule drugs from penetrating into and being active in the brain,33 creating a significant challenge to drug development for disorders of the central nervous system. Other important examples include the skin, the most commonly studied barrier for drug delivery,3 and the intestinal barrier, which impedes the bioavailability of orally administered medications.34 Various approaches to disrupt barrier integrity have been shown to improve movement of therapeutic agents across biological barriers. Such techniques include the use of microbubbles and ultrasound,35 lasers,36 microneedles,37 and nanoparticles.38
2.2 Microenvironmental cues in biological barriers
Various physiological cues come together in vivo to support the function of biological barriers. Here we discuss a few key microenvironmental cues of particular relevance to barrier modeling, namely: cell–cell interactions, 3D architecture, fluid shear, and mechanical stretch.
2.2.1 Cell–cell interactions.
In biological barriers, endothelial and/or epithelial cells exist in a multicellular environment where interactions between different cell types influence barrier function. In the gut, commensal bacteria can positively regulate epithelial barrier integrity as well as decrease translocation of pathogenic bacteria across the barrier in vivo.39 Short-chain fatty acids, a by-product of dietary fiber fermentation, are a mediator in this phenomenon – for example, butyrate modulates TJ expression to increase barrier integrity, and is linked with reduced inflammatory response.30 Gut microbiota influence other aspects of barrier function as well, regulating luminal pH, mucus secretion, and lipid absorption and export across the epithelium.30,40 In turn, epithelial cells also regulate microbiota to maintain healthy barrier function. For example, Paneth cell production of antimicrobial peptide Reg3 promotes spatial segregation of microbiota from epithelial cells, which limits bacterial colonization and activation of inflammatory responses.41 Cells on the other side of the epithelium can also impact the barrier – for example, mouse intestinal fibroblasts can exert mechanical forces on the ECM to influence gut epithelial folding.42 In the placenta, crosstalk between trophoblasts and ECs is essential for proper development of the barrier.43 Although trophoblasts play a dominant role in determining placental barrier function,44,45 the fetal endothelium has distinct contributions to placental transport, tightly regulating the movement of metabolic substances like glucose and cholesterol across the placental barrier.46,47 In the BBB, the effect of NVU support cells is well-established, with a number of studies in the last 30 years incorporating astrocyte-conditioned medium or primary astrocytes into brain EC cell culture to increase barrier integrity.48–52 Pericyte co-culture has a similar effect, although barrier strengthening is most effective when combining multiple cell types, e.g., tri-culture systems of ECs, pericytes, and astrocytes.53–56 Appelt-Menzel et al. investigated a quad-culture of human induced pluripotent stem cell (hiPSC)-derived ECs, primary astrocytes, primary pericytes, and hiPSC-derived neural stem cells; the authors found that this co-culture setup not only resulted in significantly increased barrier integrity, but also in upregulation of the glucose transporter gene SLC2A1 compared to hiPSC-EC monoculture.57
2.2.2 3D architecture.
The various cell types in biological barriers, together with basement membranes and connective tissue, are intricately organized, and this 3D architecture plays a key role in barrier function. A well-known example of this is the skin, a highly-stratified barrier with layers that have distinct functions associated with their spatial arrangement in relation to each other and the external environment. The stiff, outermost layer of the epidermis – the stratum corneum – is composed of cornified, dead keratinocytes that protect the underlying layers from dehydration, exogenous substances, and physical damage.58 The position of the epidermis in relation to the external environment exposes it to mechanical forces; however, the transduction of these forces is layer-specific. For example, only the last viable granular layer directly underneath the stratum corneum experiences sufficiently high tension for the formation of TJs.59 The mechanical forces experienced by keratinocytes and subsequent effects on cell phenotype not only depend on the position of the cell layer, but also the position of the cells within the layer. Undulations in the basement membrane are associated with distinct patterning of epidermal stem cells in the basal layer, with higher cell density and degree of differentiation at the “bases” (closer to the basement membrane) compared to cells at the “tips” (closer to the skin surface); this is determined in part by the slope of the undulations, which influence forces exerted at intercellular junctions.60 Similarly, the gut barrier exhibits topographically-dependent cell patterning at the crypt and villus structures,30,42,61 and in the placenta, basement membrane thickness and composition play an important role in trophoblast differentiation and organization.45 In the BBB, the structural arrangement of the basement membrane contributes to barrier stability,29 providing scaffolding that facilitates close contact and communication between brain ECs and astrocyte end-feet, which physically surround brain microvessels and contribute to the regulation of blood flow in the CNS.62
2.2.3 Fluid flow.
Biological barriers are subjected to various mechanical forces from their microenvironment,63 including shear stress induced by fluid flow. This is of particular importance in blood vessels, where ECs have a variety of mechanotransductive responses to shear, including modulation of gene expression,64 secretion of vasoactive molecules,65,66 and cell alignment in the direction of flow, as well as increased junctional protein expression and barrier function.67 In the BBB, physiological fluid shear falls within 1–40 dyn cm−2 (0.1–4 Pa), ranging from 1–4 dyn cm−2 in venous blood vessels to 5–40 dyn cm−2 in the brain capillaries.68–70 In a dynamic in vitro model of the BBB using a capillary culture system, laminar fluid shear ramped from 1 dyn cm−2 to 6.2 dyn cm−2 over the course of two weeks was shown to increase barrier function, induce significant upregulation of various BBB junctional genes, and improve correlation to in vivo data for permeability of various compounds.71 Additionally, in this study, shear stress increased the gene expression of a wide panel of genes related to BBB drug transport function, including ion channels, ABC transporters, and SLC transporters. More recently, Vatine et al. demonstrated in an hiPSC-derived BBB model that even low shear fluid flow can lead to upregulated expression of BBB-relevant markers like occludin and PECAM-1, with perfusion condition (0.01 dyn cm−2) and low level fluid shear (0.5 dyn cm−2) having distinct expression profiles of endothelial genes compared to each other and to static culture, as determined by principal component analysis.72 Fluid flow is also a critical microenvironmental feature of the kidney, which filters approximately 180 litres of blood per day;73,74 the filtration unit of the kidney, the glomerulus, is thus unsurprisingly subjected to substantial levels of shear stress, up to 20 dyn cm−2.75 In contrast, the intestinal barrier sees lower levels of fluid shear in vivo, with estimates in the range of 0.002–0.4 dyn cm−2 (0.0002–0.04 Pa).76,77 Variations in the magnitude of fluid shear stress can happen in physiologically healthy conditions; for instance, in the placental barrier, maternal blood flow exerts shear stress ranging from 0.001 to 30 dyn cm−2 on trophoblasts during different stages of pregnancy, with lower shear values occurring in the first trimester due to extravillous trophoblastic plugs impeding blood flow to the intervillous space.78 On the other hand, changes in fluid flow can also be linked with barrier dysfunction. For example, hypoperfusion of the gut is associated with intestinal inflammation, impaired barrier function, and disruption of microbiome diversity.30,79 Similarly, various brain pathologies involve changes in fluid mechanics of blood flow,80e.g., vascular dementia is associated with a decrease in cerebral blood flow81 and an increase in BBB permeability.82
2.2.4 Mechanical stretch.
The gut barrier is subjected to repetitive mechanical strain through the rhythmic movement of villi, and on a larger scale, peristalsis in the intestinal tract.83 Stretching of the intestinal epithelium drives cell division, proliferation, and extrusion.30,83 In particular, there is evidence indicating that cyclic strain can induce proliferation and migration.84 This dynamic cue also appears to play a role in cell patterning, with a recent study by Tallapragada et al. finding that the stretching of intestinal organoids through inflation and their subsequent collapse resulted in the fission of crypt-like stem cell zones.85 Further, stem cells at the base of intestinal crypts are maintained by Wnt and Notch signaling, which are activated by compression and stretching forces, respectively.42 The mechanical forces relevant to in vivo peristalsis have been mimicked in OOC systems, using conditions of 10–15% strain at 0.15 Hz.77,86 Likewise, lung tissue experiences cyclical mechanical loading through respiration at a rate of 12–20 breaths per min.87 Stretch is not only critical for lung development and growth,88 but also promotes lung endothelial barrier function, with alveolar stretch-induced Piezo1 activation preventing the phosphorylation of VE-cadherin to stabilize adherens junction expression at the plasma membrane.89 Estimated physiological strain ranges from 0–5% for normal tidal breathing and 15–40% going from functional residual capacity to total lung capacity,90 with the seminal studies establishing the alveolus-on-chip using a similar value of 10% strain at 0.2 Hz.91,92
3. Modeling biological barriers in vitro
In vitro models of the permeability and coupled selective transport that constitute barrier function are usually assessed by measuring permeability to a compound or measuring the electrical resistance across the barrier. To assess cross-barrier transport, access to both sides of the barrier is typically necessary – to introduce the compound of interest on one side, and sample accumulated compound from the other side – and important to consider when designing an in vitro barrier model. The main approaches to in vitro barrier modelling are summarized in Fig. 2.
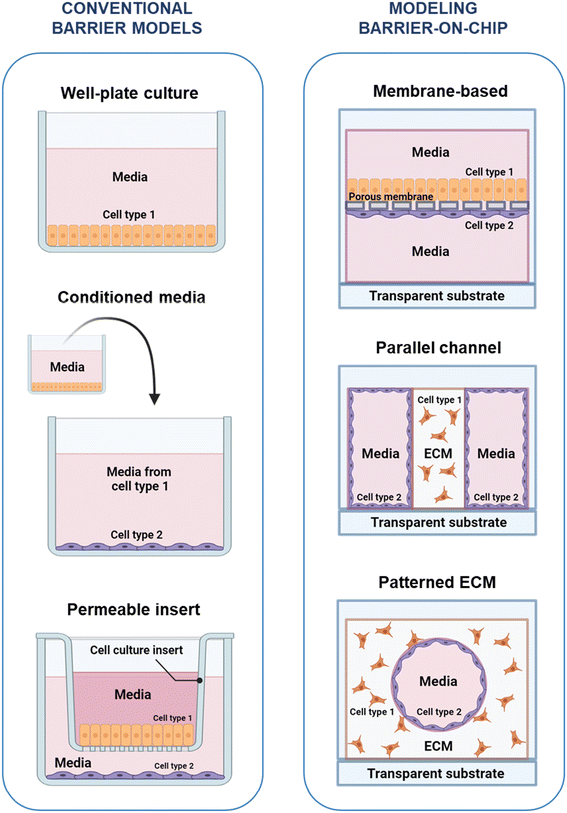 |
| Fig. 2
In vitro barrier models. Left: Conventional barrier models – well-plate monoculture; treatment of one cell type with conditioned media from another cell type; co-culture using a permeable cell culture insert. Right: OOC barrier models – membrane-based, with top and bottom channels formed by a permeable membrane; parallel channel, where one or more channels contained an ECM analogue; patterned ECM, where a fluidic channel is created within an ECM analogue. ECM = extracellular matrix. Created using https://www.BioRender.com. | |
3.1 Conventional barrier modeling
The interactions between cells in biological barriers can be modelled through the co-culture of different cell types in various formats. This can involve the separate or combined use of direct co-culture – where cells are grown in direct contact with each other, enabling juxtracrine signaling – and indirect co-culture – where cells types may only interact through secreted factors in the culture medium, i.e., paracrine signaling. The use of conditioned medium, in which medium collected from culture of one cell type is added to the culture environment of another cell type, enables the isolation of paracrine signaling effects. This method, however, has the disadvantage of not fully representing dynamic signaling at the in vivo barrier, as it only allows for one-way interactions and secreted factors may degrade in conditioned medium.93 Using a permeable membrane, as in the Transwell system, enables both real-time multi-way interactions, as well as access to both sides of the barrier, making the Transwell system a widely-used tool in in barrier research.94 The Transwell system is a commercialization of the original Boyden chamber and consists of a plastic, basket-like insert with a porous membrane as a floor that hangs from the edges of a tissue culture well plate95,96 (Fig. 2A). Cells can be seeded on the porous membrane inside the insert, on the membrane outside the insert, or on the bottom of the well, allowing for various co-contact and non-contact culture scenarios. The ease-of-use, compatibility with standard plates and workflow, and the ready access to both sides of the barrier have ensured widespread adoption of the Transwell insert. However, despite its utility as a robust tool for in vitro barrier research, the Transwell insert is a limited model, missing key aspects of the in vivo barrier microenvironment, which has motivated barrier models with greater physiological fidelity.
3.2 Modeling barriers in OOC platforms
The in vivo microenvironment comprises various features that are not captured in simpler wellplate and Transwell setups. These include ECM, 3D microarchitecture and co-culture of cells in close proximity, which combined with the high surface-to-volume ratio at the microscale play important roles in paracrine signaling and biochemical reaction rates.8,97 Microphysiological cell culture systems, often referred to as OOC, aim to address the drawbacks of traditional culture systems by implementing physiological cues such as fluid shear, accurate microscale dimensions, mechanical cues, ECM and geometrically defined co-culture.4,97,98
There are three dominating approaches to modelling biological barriers at the microscale, as illustrated in Fig. 2B. The most commonly used approach is the membrane-based configuration, where vertically stacked microfluidic channels are separated by porous membranes, on which cells can be cultured to create a horizontal barrier. Commonly the membranes are similar to those found in Transwells (e.g., polyethylene terephthalate (PETE)99 and polycarbonate100), but a variety of materials, porosities, and membrane thicknesses have been used, including polydimethylsiloxane (PDMS) to allow for dynamic stretching,101 as well as substitution of synthetic material by thin suspended hydrogels to allow for closer interactions between cell types.102 This device design has been used to study a broad range of endothelial and epithelial barriers, including the vasculature,99,103–106 liver,107–110 lung,91,92,111,112 gut,76,101,113–117 BBB,100,118–126 retina127,128 and placenta.129 A number of commercial OOC systems are available with this configuration, including single chips from Emulate,72,115,130 and high-throughput plates from Draper.131–133 Another approach uses parallel channels in a single layer, with one or more channels containing a constrained ECM analogue, where a hydrogel is confined using pillars or phase guides.134–138 Platforms with this configuration have been commercialized by companies like AIM Biotech139,140 and Mimetas.141,142 Finally, in patterned ECM devices, vascular channels are created within an ECM analogue using a variety of methods including 3D printing;143–145 removal of scaffolds such as needles,80,146,147 PDMS,148 or gelatin;149 soft lithography;150 or the removal of hydrogel prior to curing, using flow of air or media.151,152 Nortis is an example of a company that has developed chips incorporating patterned ECM channels to create microphysiological models of the epithelial barrier in the kidney.153–155
Due to the size of microfluidic channels, OOC platforms are well-suited for modeling microvascular phenomena,105 and the dimensions of the channels enables well-controlled laminar flow to induce predictable shear stress.63 This allows for the probing of cellular behaviours in the context of physiologically relevant levels of shear. Generally, all three of the microfluidic barrier model approaches support controlled flow for shear exposure as well as good microscopy conditions. However, the different device designs vary in their physiological resemblance, ease of integration with on-chip readout, compatibility with barrier integrity assessment methods, as well as sampling constraints and shear stress profile.
The membrane-based design provides structural integrity that allows for a wide range of shear stresses, as well as a simpler, more reproducible device geometry. Many devices use traditional synthetic porous membranes, which physically separate cell types and limit cell–cell communication primarily to paracrine signaling. A number of studies have designed thinner membranes to facilitate closer association between co-cultured cells, including PDMS membranes in the range of 1–10 μm,156–159 and nanofibrous scaffolds in the sub-micron to 5 μm range.160–162 However, the main advantage of this design is the ease of access to device compartments on either side of the barrier, enabling off-chip measurements of analytes (e.g., drug permeability via mass spectrometry). If channels on either side of the barrier are accessible to flow, it is possible to create stable chemical gradients,163,164 which are important to extravasation events such as cancer metastasis and bacterial dissemination. This design also allows for consistent application of fluid flow on one or both sides of the barrier; for example, van der Helm et al. made use of both top and bottom channels to apply perfusion-level fluid shear (4 × 10−4 dyn cm−2) to an intestinal epithelial monolayer to induce the formation of villi-like structures within 7 days of culture.113 Both the membrane-based and parallel channel designs, however, suffer from varying shear stress profiles along channel walls due to their rectangular cross section.165 Nonetheless, the defined geometry and structural stability of membrane-based designs can be leveraged in certain cases to implement controlled cycles of mechanical strain, another important microenvironmental cue. Through the use of flexible membranes and chip-integrated vacuum chambers, the barrier culture can be stretched to simulate the mechanical strain that occurs at the microscale during breathing and peristalsis. Huh et al. demonstrated this in a complex OOC model of the alveolar-capillary interface, showing that a physiological strain (10% cyclic strain at 0.2 Hz) amplified inflammatory response to silica nanoparticles, and played a key role in interleukin-2 (IL-2)-induced vascular leakage associated with pulmonary edema in cancer patients.92,166 This model not only recapitulated in vivo-like conditions, but also highlighted the potential of OOC systems to further our understanding of the roles played by microenvironmental cues in disease physiology and tissue-level drug response. Similar regimens of cyclic strain have been employed in gut OOC models to mimic peristaltic motions, supporting intestinal epithelial polar polarization and formation of villi.76,101,115
In contrast to membrane-based designs, parallel channel and patterned ECM designs, through their incorporation of ECM compartments, more closely mimic in vivo substrate stiffness167 and enable the direct association of the vascular component with support cells.152 The porous membranes used in membrane-based designs, aside from creating a physical separation between co-cultured cell types, can also be an obstacle for imaging; parallel channel designs provide an alternative, facilitating the imaging of cells in direct contact while still retaining some benefits of defined channel geometry.
Pattern ECM designs, on the other hand, allow for the formation of in vivo-like cylindrical blood vessels with uniform shear stress along the entire perimeter of the channel,165 and provide the best setup for studying cell migration and invasion.98 The hydrogel substrate, however, often does not provide enough structural integrity to perform shear stress studies, and assessment of permeability into the ECM compartment requires live imaging or digestion of the matrix, which limits sample collection.98,165 This creates a limitation for barrier function measurement by traditional molecular permeability assays, largely restricting their application to fluorescence image-based detection, which requires bulky and costly instrumentation.138 Moreover, the ECM component also presents an obstacle to barrier assessment by trans-epithelial/endothelial electrical resistance (TEER) due to difficulties with electrode placement and a non-uniform electrical field;152 this topic is further discussed in section 4.2.1. Similar barrier assessment challenges are applicable to ECM-containing parallel channel devices.
More details on considerations for microfluidic device configurations have been reviewed elsewhere.63,165,168,169
4. Methods for barrier integrity assessment
The two key characteristics shared by all biological barriers is that they create a boundary between two different compartments or environments and control the exchange between the two. Hence, measurements of the barrier tightness and selective passage of cells and molecules are important regardless of what barrier is being modelled and are common in biological barrier modeling. The classical method for evaluating endothelial barrier integrity is to use molecular tracer dyes – e.g., fluorescein isothiocyanate-conjugated dextran (FITC-dextran) – and monitor their diffusion across the monolayer over time. Electrical sensing provides a non-invasive alternative to molecular tracer dye assays, which can interfere with cell physiology, e.g., molecular transport and barrier integrity.170 While biological barriers have various additional functions – including metabolic activity, cell–cell signaling, molecular transport, and immune response modulation171 – a more in-depth discussion of biosensing for these applications can be found in other publications,8,171,172 and the scope of this review is specific to barrier integrity. The measurement methods discussed in this section are illustrated in Fig. 3 and summarized in Table 1.
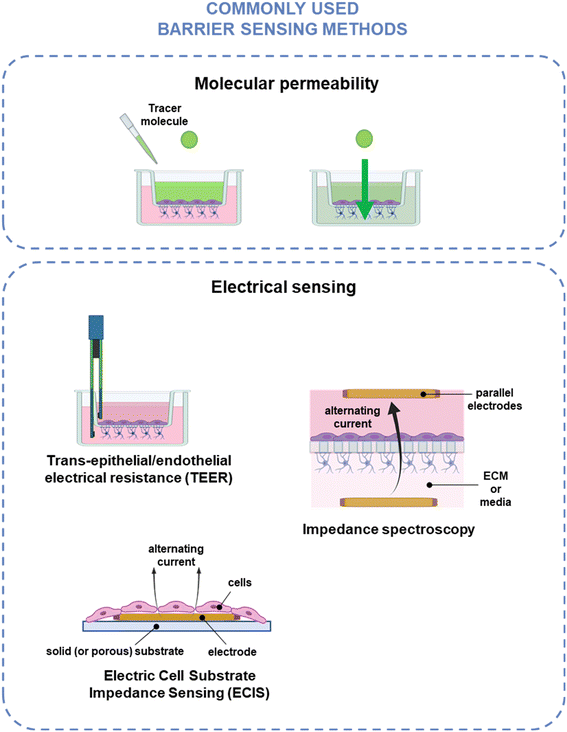 |
| Fig. 3 Common barrier integrity assessment techniques. Top: Molecular permeability techniques, where the movement of a tracer molecule across the barrier is monitored using fluid sampling, optical detection, or electrochemical detection. Bottom: Electrical sensing techniques, where barrier integrity is measured by applying an electrical current across the barrier using chopstick TEER, impedance spectroscopy, or ECIS. Created using https://www.BioRender.com. | |
Table 1 Summary of commonly used techniques for assessing biological barriers
Category |
Method |
Measured output |
Common measurement method |
Advantages |
Disadvantages |
Molecular permeability |
Fluid sampling |
Fluorescence; |
Plate reader; |
Compatible with most platforms |
Low time resolution |
Radiolabeled molecules; |
Scintillation counter; |
Variety of readouts possible |
Invasive |
Protein concentration; |
ELISA; |
Drug concentration |
Mass spectrometry |
|
Optical detection |
Fluorescence |
Microscopy |
Suitable for variety of platforms |
End-point assay |
|
Electrochemical tracers |
Change in current |
Electrochemical sensor |
High temporal resolution |
Electrode fouling |
|
Electrical sensing |
Trans-epithelial/endothelial electrical resistance (TEER) |
Resistance at single frequency |
Handheld chopstick electrodes; |
Gold standard |
Non-homogenous current distribution; |
Parallel electrodes |
Indirect measurement of cell layer |
|
Impedance spectroscopy |
Impedance at multiple frequencies |
Parallel electrodes |
Can optimize frequency for cell type, assay |
Indirect measurement of cell layer |
|
Electrical cell substrate impedance sensing (ECIS) |
Impedance at multiple frequencies |
Planar electrodes |
Can optimize frequency for cell type, assay |
Not compatible with patterned ECM platforms |
Direct measurement of cell layer |
4.1 Molecular permeability
Barrier tightness can be quantified by measuring cross-barrier transport of a compound using either on-chip or off-chip detection and calculating a device-independent permeability coefficient; this method yields information about both passive and active transport across the barrier.173,174
4.1.1 Fluid sampling.
Measurements of permeability of fluorescent tracers is common in barrier modelling and any microfluidic device compatible with live cell imaging can accommodate these measurements. The typical experimental approach is to add a fluorescent compound to the luminal side of a cell layer cultured on a permeable membrane and either measure intensity in situ or collect media for off-chip concentration determination (e.g., by fluorescence plate reader). The change in concentration of the compound over time can be used to calculate the apparent permeability constant for the barrier.174,175 The permeability to a tracer molecule is given by:101,119,142
where Papp is the apparent permeability, Creceiver is the concentration of tracer molecule in the receiving compartment, Vreceiver is the volume of sample taken from the receiving compartment, Abarrier is the cell seeding area, Cdonor is the concentration of tracer in the donor compartment, and Δt the assay time. Accounting for the permeability corresponding to cell-free membrane, Pm, the permeability specific to the cell layer is given by99,119
where Pmc is the permeability obtained from membranes seeded with cells, and Pc is the permeability of the cell layer.
Commonly used fluorescent tracers include various sizes of FITC-dextran126 (typically 4–70 kDa) and smaller molecules like Cascade blue115,176 (Fig. 4A) and sodium fluorescein,177 which are in the <1 kDa size range. Different sizes of tracers can be used to distinguish between two categories of transport mechanism – paracellular transport for ions and small hydrophylic molecules under 3 nm in diameter, and transcellular transport for larger molecules (including most proteins),178 with the former being particularly suitable for detecting subtle changes in barrier integrity.179 Mass spectrometry can be used to detect drugs like cimetidine,126 citalopram,176 verapamil,180 and nicotine,110 while scintillation counting enables detection of radiolabeled small molecules (e.g., sucrose) and proteins (e.g., albumin).68,179 The passage of proteins like transferrin, albumin and IgG across the barrier can also be measured through ELISA assays, and their ratios (e.g., transferrin/albumin, IgG/albumin) can be used as an indicator of barrier health72 (Fig. 4B). Beyond barrier integrity, fluid sampling can be used to obtain information about other aspects of barrier function, such as the function of transporters. Specifically, a bidirectional permeability assay – where both apical-to-basal and basal-to-apical transport are assessed – can be used to determine whether active transport is occurring using the efflux ratio. An efflux ratio greater than 2 indicates drug efflux, and a ratio smaller than 0.5, drug uptake; selective inhibitors can then be used to identify the relevant transporter.52,69,181 The molecular permeability assay can additionally be applied to evaluating transcytosis of target molecules in the presence or absence of a target receptor inhibitor,182 or in comparison to a fluorescently-labeled dextran of similar size to the molecule of interest to determine background paracellular flux and efficiency of transcytosis for the target molecule.183
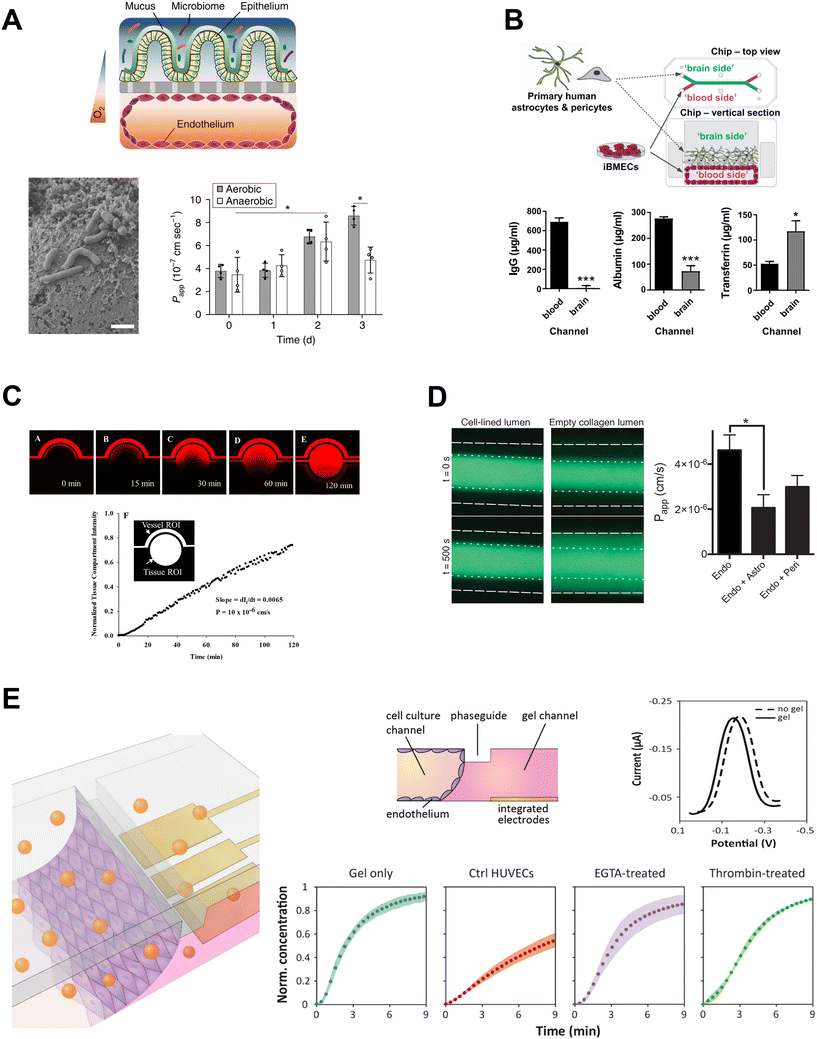 |
| Fig. 4 Molecular permeability assays in OOC. A: Permeability of cascade blue across intestinal barrier co-cultured with B. fragilis in aerobic and anaerobic conditions. Adapted from Jalili-Firoozinezhad et al.,115 with permission from Springer Nature. B: Permeability of proteins from blood to brain compartments quantified by ELISA. Adapted from Vatine et al.,72 with permission from Elsevier. C and D: Fluorescence microscopy used to assess permeability to C: Texas Red 40 kDa dextran, reproduced from Deosarkar et al.189 and licensed under CC BY 4.0; and D: 3 kDa dextran-Alexa488, reproduced from Herland et al.,152 and licensed under CC BY 4.0. E: Permeability assessed by detection of electrochemical tracer hexaammineruthenium. Adapted from Wong et al.138 with permission from Elsevier. | |
This method is highly versatile, allowing for a variety of readouts based on the tracer compound used, as well as broad compatibility across platforms, including traditional transwell inserts and membrane-based OOC devices. There are some key limitations, however, including: low time resolution due to the need for sampling,178 bleaching of fluorescent probes,184 damage to the cell barrier,170 and sensitivity to device materials. For example, it is well-established that PDMS, the most commonly used material in OOC microfluidic devices, has a capacity for absorbing small molecules.185,186 Although models have been developed to account for this phenomenon,187,188 permeability coefficients obtained in PDMS devices should be interpreted with caution.
4.1.2 Optical detection.
Optical detection is often used as a supplemental or primary method of permeability assessment in OOC systems. This is particularly true for patterned ECM device designs where sampling from the ECM compartment is challenging. Typically, a fluorescently-labeled molecule is allowed to perfuse across the barrier and its accumulation over time is observed using fluorescence microscopy. The rate at which the intensity changes correlates with barrier permeability (Fig. 4C and D).142,152,189–195 This technique measures permeability on a global level, but local heterogeneities in the barrier may have outsized effect on molecular permeability values.196 To address this limitation, Dubrovskyi et al. developed an avidin–biotin localized permeability assay that detects the binding of fluorescently-labeled avidin to the exposed ECM-bound biotin on the culture substrate.197 The high resolution of this assay has been effectively leveraged to directly compare to colocalization of different cell–cell junction phenotypes in brain ECs,198 and more recently adapted to a range of biotin–avidin compounds by Gao et al. to assess permeability in non-permeable microfluidic models of microvessels.199 Optical detection of fluorescent tracers can also enable detection of other barrier functions, e.g., efflux pump activity using a non-selective P-gp/BCRP/MRP substrate such as daunorubicin, or a P-gp transporter-specific substrate like rhodamine 123.52
4.1.3 Electrochemical tracers.
Due to the small volumes and relatively large surface area in microchannels, diffusion distances are shorter and mixing more efficient than in standard culture conditions, enabling sensitive and rapid detection of analytes.8 A broad range of OOC-integrated biosensor applications have been explored – such as detecting metabolic factors (e.g., glucose, lactate), inflammatory cytokines (e.g., IL-6, TNF-α), and key parameters for cell survival (e.g., oxygen, pH levels)8 – and this includes molecular permeability. Wong et al. implemented electrochemical sensing of hexaammineruthenium in both a membrane-based platform and a parallel channel device with constrained hydrogel, to monitor the barrier integrity of endothelial monolayers on chip138,200 (Fig. 4E). The method monitored the diffusion of the tracer amperometrically, with current signal being correlated to barrier permeability, providing a sensitive detection method that avoids the manual sampling typically used in molecular permeability assays. Ino et al. used a similar principle to design a tool for the purpose of assessing endothelial OOC permeability using the diffusion of [Fe(CN6)4−].201
4.2 Electrical sensing
4.2.1
Trans-epithelial/endothelial electrical resistance (TEER).
TEER measures the paracellular transport of small ions across the barrier. Whereas tracer-based methods typically provide an end-point assessment of permeability at a specific time point, TEER can be less invasive and can be measured continuously over time in culture.
4.2.1.1. Measuring TEER in conventional barrier models.
For conventional in vitro barrier modelling in transwell inserts, TEER is measured by temporarily placing electrodes on either side of the cell barrier. This is often done using a commercially available ‘chopstick’ setup, where one electrode pair is immersed inside the Transwell and another electrode pair is immersed in the opposite chamber. One set of electrodes applies a current across the cell layer – most commonly at a frequency of at 12.5 Hz and the other measures voltage. The resulting resistance is then normalized through multiplying by cell culture area to enable comparison between different culture platforms. This technique allows for benchmarking barrier integrity for different cell types and co-culture models in vitro. For example, the immortalized hCMEC/d3 human brain EC line, despite having an overall tight junction expression pattern characteristic of the BBB, has a typical TEER range of 30–50 Ω cm2,23 compared to estimated in vivo values in the range of 5000–8000 Ω cm2.170,202 Studies using human induced pluripotent stem cell (hiPSC)-derived brain ECs, on the other hand, indicate that this cell type is well-suited for modelling barrier integrity in the BBB,176,203 yielding monolayers with TEER values as high as 8000 Ω cm2, and TEER in excess of 9000 Ω cm2 when co-cultured with hiPSC-derived astrocytes.203 In contrast, Caco-2 cells, which are a standard cell line used for modelling the intestinal epithelium, tend to form tighter barriers in vitro – i.e., 150–2500 Ω cm2 (ref. 204–206) – compared to ex vivo measurements of the small intestine (30–100 Ω cm2).170,207
While simple to implement, this chopstick implementation is particularly susceptible to variation in measurements because of the manual handling involved. Additionally, the electrical field produced by chopstick electrodes is uneven, which causes the cell area close to the electrodes to contribute a disproportional amount to the resistance measurement.170,208–210 This can cause large discrepancies in TEER values obtained for the same cells with seemingly the same culture conditions.210 For example, a head-to-head comparison by Vigh et al. found a ∼1000 Ω cm2 difference in chopstick TEER values for Caco-2 cells cultured in 6.5 mm vs. 24 mm inserts.204 Thus, when using the conventional chopstick electrodes, smaller Transwell inserts are preferred over larger ones as the non-uniform flow field across larger membranes results in an overestimation of TEER values.170,209 To help standardize measurements by removing the manual electrode handling and creating a more uniform electric field, products using concentric, circular electrodes in the top/bottom compartments have been developed (EndOhm, World Precision Instruments).211 Other commercial platforms (TEER 24 from Applied Biophysics, cellZscope 2) further address these challenges by incorporating electrodes into multiwell plates and allowing for continuous monitoring.212,213 Despite these modifications, the parallel configuration of TEER electrodes (Fig. 3) results in measurements that assess impedance of the bulk model – that is, all the materials between the electrodes – rather than the cell layer directly.
4.2.1.2. TEER in OOC systems.
As the field of in vitro organ modelling is moving toward microfluidic implementations in OOC systems, researchers have explored methods to implement TEER measurements on-chip. As a simple approach to add TEER to a microfluidic cell culture system, different research groups have temporarily immersed electrodes in chip reservoirs connecting to channels on either side of the cell layer. This solution is simple but the measurements are often unreliable due to the geometry of the system; the voltage drop is measured over a long distance and does not fall evenly across the cell culture area.125,208,210,214 Nonetheless, commercial chopstick electrodes189,215 or custom made immersed electrodes76,125,195,216 are often used in OOC systems. The precision of TEER measurements made with electrodes that are immersed temporarily can suffer from variations in electrode placement, though the limited size of reservoirs compared to Transwell inserts can help reduce this discrepancy.215 Variability due to electrode placement can be addressed by permanently immobilizing electrodes in the device reservoirs.216 However, this solution still does not address the long distance between electrodes and uneven electric field. Instead, TEER electrodes can be integrated directly into the channels of OOC systems, with the electrodes optimally positioned in close proximity and parallel to the cell layer. This configuration is most readily implemented in membrane-based microfluidic devices,170 and has been applied to measure TEER of BBB,118,119,122,217–219 lung219,220 and gut214 barrier models.
While incorporating on-chip electrodes greatly improves TEER measurements compared to electrodes immersed in reservoirs, electrode size and placement, device geometry, reporting practices, and use of supportive biomaterials vary widely between OOC platforms, making it challenging to produce TEER values that are directly comparable among different studies. For example, in the hiPSC-derived BBB-on-chip literature, there is a wide range of TEER values reported using various measurement and validation approaches, often confounded by whether they are reported as raw impedance values or normalized to culture area. To address this, Wang et al. used a Transwell-like calibration device with the same dimensions as a 24-well plate insert to obtain TEER values of up to 4000 Ω cm2 in their BBB co-culture devices.126 On the other hand, Park et al. performed a chip to transwell comparison, but reported TEER as impedance values that are not normalized to culture area (e.g., >20
000 Ω in BBB chips).176 From a fabrication standpoint, the integration of parallel facing TEER electrodes – that is, electrodes incorporated into both the top and bottom channels – creates an additional challenge of electrode alignment when bonding top and bottom layers.221 For those researchers seeking ready-made, commercially-available OOC barrier measurement systems, both Mimetas141,142 and Draper131–133 have developed high-throughput platforms with integrated TEER measurement. While some studies connect the electrodes to standard TEER measurement systems (e.g., EVOM2 from WPI Instruments),131,132,219 other platforms incorporate impedance spectroscopy, a method that makes use of measurements taken over a range of alternating current frequencies, to derive TEER values.122,141,142,214,217,220
4.2.2 Impedance spectroscopy.
An additional method for electrically evaluating biological barriers is through measuring the impedance, Z. Impedance is the ratio of the voltage–time function, V(t), and current–time function, I(t), in an alternating current circuit:170,222–224
where Vm is the maximal voltage value measured, Im is maximal value of the alternating current, t is time, ω is angular frequency for a given AC frequency, f = ω/2π, and θ is the phase shift between voltage and current sine waves. The complex impedance, Z, is comprised of a frequency-independent resistance component, ZR, and a frequency-dependent reactance component, ZX, which includes the capacitive contributions of the system – the magnitude of the impedance vector can be expressed as
. Impedance sensing techniques generate a low amplitude frequency-dependent AC signal, which is applied across the cell layer, and the response in electrical current; impedance is calculated using the resulting values.225 Whereas classical TEER provides monolayer resistance across a single frequency, impedance spectroscopy measures impedance over multiple AC frequencies, providing additional information on the biological barrier. Current across the barrier can flow around the cell bodies, taking the more inherently resistive paracellular pathway and reflecting the state of cell–cell contacts (i.e., RTEER), but also can couple capacitively across the cells' membranes, taking the transcellular route that reflects cell layer capacitance (i.e., CC).178,226 These pathways can be described using equivalent circuits (Fig. 5A, left and middle panels). The contribution to overall impedance by the cell culture system components can be plotted against frequency, and broadly divided into three frequency regions, where electrode capacitance (CE), cell layer resistance (RTEER) and capacitance (CC), and medium resistance (Rmedium) dominate, respectively (Fig. 5A, right panel).170,226 While the specific frequencies will vary with electrode orientation and cell type, generally the low frequencies (<102 Hz) are dominated by CE, the mid-range frequencies (103–105 Hz) by cell layer properties RTEER and CC, and the higher frequencies (>105 Hz) by Rmedium.178,226–228
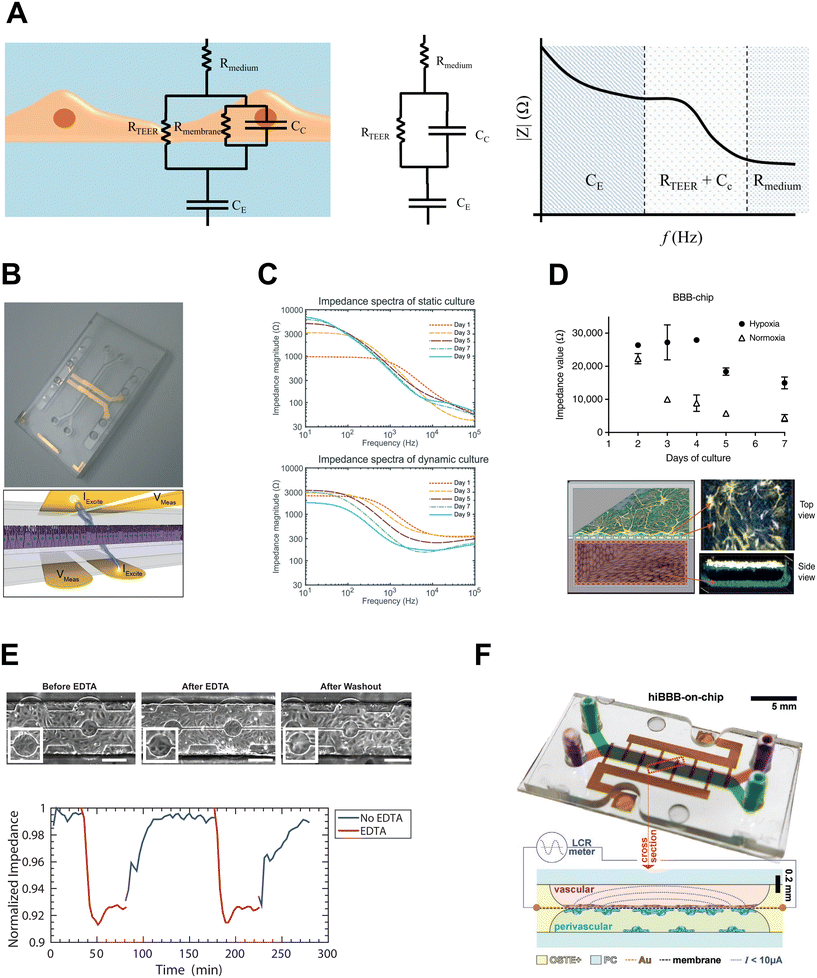 |
| Fig. 5 Impedance sensing integrated on chip. A: Left: Typical equivalent circuit for cell layer. Middle: Simplified equivalent circuit. Right: Impedance vs. frequency plot – contributions of cell culture system to overall impedance. Adapted from Srinivasan et al.,170 and licensed under CC BY 4.0. B: Impedance electrodes integrated on chip. Adapted from Henry et al.,220 with permission pending from the Royal Society of Chemistry. C: Impedance spectra for gut OOC in static and flow conditions. Reproduced from van der Helm et al.,113 with permission from the Royal Society of Chemistry, licensed under CC BY NC-3.0. D: Impedance measurements in OOC model of BBB in hypoxia and normoxia. Adapted from Park et al.,176 and licensed under CC BY 4.0. E: HUVEC barrier disruption and recovery in microfluidic device with transparent indium tin oxide ECIS electrodes. Adapted from Lewis et al.,232 with permission from AIP Publishing. F: Microfluidic BBB model with gold ECIS electrodes integrated onto porous membrane using e-beam deposition. Reproduced from Matthiesen et al.,100 licensed under CC BY 4.0. | |
Commercial platforms for impedance spectroscopy include Real-Time Cell Analysis (RTCA) from xCELLigence, which allows culture of cells directly on electrodes, but only measures high frequency responses (10 kHz, 25 kHz, 50 kHz). CellZScope, on the other hand, enables measurement over a wide range of frequencies (0–105 Hz), although the electrodes are external and thus, like TEER, measure impedance of the bulk model.
4.2.2.1. Impedance spectroscopy on chip.
A number of studies have incorporated impedance spectroscopy into membrane microfluidic devices, using electrodes oriented as in conventional TEER, with electrodes on either side of the membrane. The equivalent circuits can be used to generate a model of the cell culture system, and measurements taken over a spectrum of frequencies can be fitted to the model, minimizing the difference between actual and theoretical impedance values to determine the contributions of cell culture system components to overall impedance.214,217,220 Douville et al. took advantage of the multi-frequency feature of impedance spectroscopy to resolve RTEER values for well-characterized cell lines bEND.3 and MDCK-2, being able to both distinguish between the cell types and obtain resistance values comparable to those in literature.217 Since then, various organ chip models have employed integrated impedance spectroscopy measurements, including lung and intestinal barrier113,220 (Fig. 5B and C), kidney,229 BBB176 (Fig. 5D and F) and blood-retinal barrier.194 The utility of combining impedance electrodes with circuit modelling extends beyond determining RTEER – indeed, Henry et al. were able to use such an approach to isolate changes in cell layer capacitance as intestinal epithelium differentiated and formed villi-like structures in response to fluid flow.220
4.2.3 Electrical cell substrate impedance sensing (ECIS).
Electrical cell substrate impedance sensing is a technique developed by Keese and Giaever230,231 to measure cell behaviours in vitro by measuring impedance across a range of frequencies. ECIS contrasts to traditional impedance spectroscopy in that it measures impedance of a cell layer grown directly on an electrode (Fig. 3). In the conventional setup, cells are cultured on a large counter electrode and small working electrode (as small as ∼250 μm diameter) embedded on a solid substrate; this contrast in size enables sensitive measurement of rapid, transient changes in cells grown on the working electrode.178
In contrast to the parallel facing electrodes featured in many OOC systems, which require the alignment of electrodes in top and bottom channels, the coplanar electrode configuration used in ECIS makes electrode patterning and device assembly more streamlined and reproducible.221 Furthermore, the small electrode size lends itself particularly well to microfluidic applications, as well as models where limited number of cells are available.
An ECIS measurement setup includes an oscillator which generates an AC signal (e.g., 1 V rms) at a specified frequency, connected to a large resistor (e.g., 1 MΩ) to provide a small current (e.g., 1 μA). The small magnitude of the current ensures the measurements are not destructive to the cell monolayer.233 The voltage drop across the device containing cells grown on electrodes is measured by a phase-sensitive lock-in amplifier (LIA), which allows for small AC signals to be resolved in high noise systems. The LIA increases signal-to-noise ratio using a low-pass filter with a narrow frequency bandwidth around the target signal frequency to exclude noise signals that are outside the signal reference frequency.234 Using the phase-sensitive lock-in amplifier and the ECIS model described by Giaever and Keese,230 the overall voltage drop is broken down into its resistive and capacitive component voltages, through which the cell layer resistance and capacitance can be determined. Lower frequency measurements (e.g., 4 kHz) provide information on changes in cell–cell contacts affecting barrier integrity due to the current taking a primarily paracellular route, and higher frequency measurements (e.g., 40 kHz) enable assessment of processes related to electrode coverage – e.g., cell attachment, spreading, and proliferation – corresponding to a predominantly transcellular current path;178,226,231,235 at the intermediate frequencies, the current takes a combination of those pathways.178
4.2.3.1. Impedance sensing vs. molecular permeability.
Electrical impedance sensing offers an advantage over the conventional molecular tracer dye assay for assessing barriers. In a head-to-head comparison between ECIS, xCELLigence, and tracer molecule permeability assay, both impedance methods were more temporally sensitive to cell response after addition of barrier modulating agents compared to the molecular permeability assay; moreover, unlike the impedance methods, 40 kDa FITC-dextran permeability did not show significant changes in response to barrier disruptors histamine and blebbistatin.236 Importantly, the impedance methods were better able to detect rapid and transient changes to permeability induced by histamine. This mirrors the increased sensitivity in TEER systems to changes in EC barrier compared to molecular permeability assays;126 the small working electrode size in ECIS setups has also been shown to provide high sensitivity detection of nanometer-scale changes in cell junctions.230 Nevertheless, Bischoff et al. cautioned against directly comparing functional data from cells grown on permeable inserts vs. structural data of cells grown on solid substrates, as in conventional ECIS.236
Yet, barrier function assessment can benefit from the synergy of impedance-based techniques and molecular permeability assays. Indeed, this gap has been addressed in one aspect through efforts to integrate both impedimetric sensing of barrier integrity and electrochemical permeability into the same permeable insert device228,237 to enable more precise assessment of the barrier modulation mechanism of a cell layer by a target molecule. Possible applications for this include the study of pharmacological agents that could treat barrier disruption-induced diseases such as diabetic retinopathy. For example, Eltanani et al. recently used ECIS to assess the impact of mitochondrial inhibitors targeting oxidative phosphorylation in retinal ECs, finding that inhibition of complex I by rotenone had the greatest influence on barrier integrity.238 In this context, simultaneous assessment by electrical sensing and molecular permeability assays could provide insight into mechanism of action and possible side effects for pharmacological agents of interest. Also, electrical sensing techniques can be adapted to monitor barrier functions that are typically assessed with molecular permeability – for example, capacitance-base arrays that can detect receptor-mediated endocytosis.239
4.2.3.2. ECIS in OOC.
Efforts have been made to apply ECIS technology to the microscale, with a recent study by Stolwijk and Wegener adapting Applied Biophysics ECIS well plates with silicone inserts to allow for microlitre volume cultures.240 Various customized microfluidic ECIS devices have been developed in the last several years,241,242 though the use of ECIS to measure flow-induced changes in endothelial barriers stretches back over the last two decades.243,244 The small scale of ECIS electrodes lends itself well to microfluidic configurations, with various ECIS flow array products available commercially from Applied Biophysics.245 Implementation of platforms developed in-house includes work by Lewis et al. (Fig. 5E), where HUVEC barrier disruption and recovery were evaluated on transparent indium tin oxide electrodes,232 and Velasco et al., who recently demonstrated fluid shear effect on endothelial barrier resistance with three different working electrode sizes (d = 50, 100, 200 μm).246 These platforms, however, use electrodes embedded on solid substrates, which do not allow for direct measurement of barrier changes in co-culture setups.
Implementation of ECIS electrodes on porous membrane cell culture inserts has recently been performed by Rajasekaran et al.237 and by Chebotarev et al.247,248 The latter studies introduced a novel, cost-effective fabrication method for integrating gold electrodes onto porous membrane in Transwell-like inserts, enabling sensitive detection of barrier formation and disruption, as well as simultaneous validation against conventional TEER and molecular permeability in the same devices.247,248 This electrode configuration lends itself well to OOC barrier modes, facilitating direct measurements of monolayers in complex OOC systems that contain multiple cell types, as demonstrated in models of the placenta74 and BBB73 (Fig. 5F). Ugodnikov et al. also found that in a membrane-based OOC setup, porous membrane ECIS electrodes were robust to other common OOC microenvironmental features.249 For example, they demonstrated that fluid shear stress of up to 5 dyn cm−2 had no significant effect on cell-free electrode measurements, and in contrast to TEER, the presence of collagen hydrogel in the bottom channel did not influence ECIS measurements.
5. Challenges for barrier integrity assessment in OOC
Despite significant progress in assessment of OOC barriers, several challenges remain and must be considered for specific contexts of use. Here we summarize three classes of challenges: (1) taking measurements that are sensitive as well as representative of the dynamic and heterogeneous nature of biological barriers; (2) considerations that must be made when integrating sensors into devices; and (3) obstacles to comparing biosensor readouts to gold standard methods.
5.1 Representative measurements
5.1.1 Time-sensitivity.
Efforts to automate fluid handling – e.g. the interrogator instrument, which allows for media changes, sampling, and imaging without uncoupling devices or removing them from the incubator110,250 – help minimize the disturbance to cell culture conditions associated with manual handling when assessing molecular permeability of the barrier. However, constraints based on experimental conditions, like device volumes and assay limits of detection, dictate the use of discrete sampling timepoints.110 Conversely, chip-integrated biosensors allow for real-time detection of analytes, with Wong et al. applying the use of hexaammineruthenium as an electrochemical tracer to obtain sub-1 minute resolution measurements on endothelial barrier permeability.138,200 This is particularly relevant in bioassays that assess rapid, transient changes in the barrier, as occurs with G-protein-coupled receptor agonists.178 A well-known example of this is histamine treatment, which can elicit barrier-modulating effects as quickly as within 30 seconds – a phenomenon that is readily detected by impedimetric measurement systems, in contrast to traditional molecular permeability assays that rely on discrete sampling.236
5.1.2 Barrier heterogeneity.
Recent findings from Richter et al. indicate that there is an edge effect for in vitro cultures of biological barriers in terms of permeability and tight junction expression, where variation in occludin expression localized to the periphery of cell culture insert membranes significantly contributes to global molecular permeability assessment.196 This is of particular relevance in OOC systems where due to channel size and geometry, a greater proportion of the cells in the monolayer are in contact with edges than those cultured in standard well plates or inserts. Methods that evaluate local barrier integrity (e.g., avidin–biotin,197 ECIS) can be used in conjunction with standard permeability assays to help distinguish between distinct areas of the monolayer and provide more accurate understanding of changes in the barrier.
5.2 Integrating sensors
The development of barrier on chip devices with in-line biosensing requires balancing a number of competing priorities, including fabrication methods, compatibility with imaging, and measurement sensitivity. This section will discuss the resulting considerations and challenges for integrating sensors in barrier OOC.
5.2.1 Fabrication.
The history of OOC is heavily intertwined with that of the microelectronics industry, with the introduction of soft lithography251 – an application of semiconductor fabrication technology – being widely considered as a key enabler for the explosive growth of the OOC field. This original OOC fabrication technique using PDMS bonded to glass was compatible with existing thin-film electrode deposition techniques for the integration of biosensors on glass substrates.252 Currently, there is growing preference toward using thermoplastics to fabricate OOC that are more amenable to scalable manufacturing184 and are more inert than PDMS;97 integrating electrodes onto substrates such as polycarbonate also allows for greater ease in machining compared to glass,220 and for simplified composition, e.g., creating OOC platforms composed primarily of thermoplastic layers.208 However, the increasing complexity of OOC devices often requires the integration of various modular components, whether that be multiple sensors171,253 (e.g., optical and electrical) or multiple materials to enable biomimetic compartmentalization, e.g., combinations of glass, PDMS and thermoplastics for BBB devices.117 This creates the need to consider the compatibility of various manufacturing processes in the production of a single platform – for example, whether the fabrication techniques for different sensors,171 or device layer bonding and sterilization methods184 may be damaging to one or more components in the platform. One proposed approach for addressing this issue is the use of additive manufacturing processes (e.g., 3D printing), which can simplify integration and assembly.254
As the demand for in-line monitoring precision and variety increases, so does the number and type of sensors integrated into OOC devices. This creates a challenge in chip-to-world interface complexity. For example, arrays of electrodes can increase throughput and capacity for data acquisition, but also introduce large numbers of electrical connections that make operation of devices more complicated for the end-user.171 There have been solutions proposed to simplify the electrical and fluidic connection interfaces, for example using matrix configurations of electrodes and flow channels, where columns and rows of electrodes can be selectively activated, and bands of reference and working electrodes are positioned in parallel and orthogonally to flow channels, respectively.255
With specific regard to barrier on chip sensing, integrating TEER electrodes can pose a challenge for chip fabrication processes,8 due to the additional layers required to position electrodes above and below the cell layer. An advantage of ECIS integration onto porous membranes systems is the coplanar electrode configuration, which simplifies the fabrication process by decreasing the number of device layers, as well as facilitating chip-to-world connectivity.100 On the other hand, there are cases where having a bilayer configuration with measurement electrodes separated from the cells may be beneficial not only from a biosensing perspective but also from the fabrication standpoint. For example, Wong et al. propose that this type of design can provide better control of analyte transport for electrochemical barrier measurement by shielding the biosensor from high flow rates, as well as enabling modular fabrication by having separate compartments for biosensing and culture of cells.256
5.2.2 Optical transparency.
Compatibility with microscopy is typically a necessity for the operation and monitoring of OOC devices, and thus consideration must be given to ensuring optical access in devices that integrate electrodes, both for the electrode substrate and for the electrodes themselves.
From the standpoint of optical transparency, glass is the optimal substrate material, being well-established as a substrate for thin-film electrode fabrication.184 Beyond its optical properties, glass is biocompatible, chemically inert, and non-conducting, but is fragile and expensive to incorporate in OOC devices.97,257 Silicon, another standard substrate for electrodes, is not optically clear and therefore OOC devices that employ silicon electrode substrates need to be design to include regions of the device that replace silicon with an optically transparent layer for imaging.257 On the other hand, many thermoplastics are transparent, cost-effective, and compatible with mass-production manufacturing processes like injection-moulding97 and therefore provide a suitable alternative as substrates for electrode deposition. Various approaches have been used in or have potential for barrier-on-chip biosensing applications; examples include e-beam evaporated gold electrodes patterned onto polycarbonate;220 inkjet printing gold, silver and platinum electrodes onto polyimide, polyethylene, and polyethylene terephthalate (PET);258 and gold leaf laminated onto PET using pressure sensitive adhesive.259
Electrodes themselves are often fabricated from optically non-transparent materials, and therefore their positioning on chip and the subsequent impact on microscopy must be considered, especially for TEER electrodes in a two-layer microfluidic membrane device. Importantly, a trade-off must be considered between creating a uniform electric field – which is enabled by larger electrode size260 – and being able to visualize the cells of interest – which motivates the confinement of the electrodes to a smaller and/or less central location along the microchannel, affecting the platform's capacity for taking measurements that are accurate and representative of the overall barrier. Alternatively, transparent conductive materials, such as indium tin oxide (ITO) have been used to fabricate electrodes that allow for improved cell visualization;219,232,261 the use of a semi-transparent thin gold layer offers some compatibility with microscopy as well.113,220
5.2.3 Electrode configuration.
Initial work on TEER electrodes on chip relied on very simple geometries, often implemented as simple metal wires threaded into a dedicated side channel in a PDMS slab.118,217 Recent efforts have sought electrode configurations that increase the accuracy of TEER readings. Yeste et al. recommended interdigitated electrodes to create a uniform electric field across the cell layer.208,262 Other groups have instead used a traditional four-point measurement approach where the voltage drop is provided by one electrode pair while the current is measured through another, reducing the impact of parasitic resistances.113,220,263,264 van der Helm et al. used a measurement scheme based on four electrodes, measuring the resistance across all six electrode pair combinations to isolate the barrier resistance in their model.264,265 The authors note that this approach, as compared to measuring the resistance in a cell-less control device, has the advantage of eliminating fluctuations in temperature, media conductivity and other non-biological factors that might vary between measurements (in addition to the advantages of eliminating device-to-device variations and the reduced number of devices required).
For TEER systems, which provide global barrier integrity assessment, accuracy is affected by the geometry of the measured compartment relative to the electrodes; for example, it is especially difficult to ensure a uniform current distribution in barrier-on-chip devices due to low conductivity within microfluidic channels.210,260 Additionally, the small device dimensions and requirements for cell imaging create constraints on possible electrode size and placement,210,266 key parameters that determine electric field uniformity. Conversely, ECIS systems typically use smaller sized electrodes for increased signal sensitivity,267 with the tradeoff of sampling a smaller cell population and local barrier properties. If a small decrease in sensitivity is tolerable for an application, then using multiple small electrodes measuring in parallel can provide a more representative picture of the overall barrier.178
OOC systems that integrate parallel electrodes (e.g., positioned on either side of the barrier) may be limited in their sensitivity to detect subtle barrier changes, as the electrodes are at a distance from the cell layer, and are measuring a series of impedances related to the bottom compartment contents, membrane thickness and porosity, cell barrier, and top compartment media.129,204,268 A head-to-head comparison of commercial impedance sensing platforms by Hucklesby et al. showed that the coplanar ECIS electrodes had a greater sensitivity to endothelial monolayer changes than the parallel CellZScope electrodes.268
5.2.4 Electrode materials and deposition methods.
Electrodes can be functionalized with coatings to prevent fouling, promote binding of specific molecules, or increase biocompatibility. For instance, many electrodes are fabricated using toxic silver wires that are subsequently transformed into biocompatible AgCl using ferric chloride.118,119,217,218 Platinum electrodes can be electroplated with platinum black, which increases the surface area of the electrodes, decreases intrinsic impedance and susceptibility to noise, and produced impedance values that were three orders of magnitude lower than those from planar platinum.214 The choice of base metal for the electrode also has implications on measurement sensitivity. Schmiedinger et al. compared electrodes fabricated using various metals, finding that aluminum electrodes had similar impedance outputs across frequencies compared to gold, while titanium had higher impedance values, likely because of higher inherent resistance within the material.269 A similar trend was observed by Walter et al. in a head-to-head comparison of ITO and thin layer gold electrodes, where the gold electrodes were ultimately chosen for subsequent experiments due to their higher conductivity.219
The method of depositing metal onto a substrate also plays a key role in the inherent resistivity of electrodes and electrical traces. For example, printed circuit board (PCB)-based techniques like electroplating and electroless plating create thicker metal traces with lower resistance compared to thin-film deposition.270 Similarly, heat-bonded gold leaf provides a flat, continuous electrode surface with lower resistivity compared to sputtered gold.247 Interestingly, inkjet-printed gold electrodes can have as much as 10-fold greater sensitivity compared to sputtered gold.258 Meanwhile, the surface morphology – and therefore sensitivity – of nanoporous gold may vary depending on fabrication method (e.g., electrochemical etching vs. dealloying), and on parameters like annealing temperature and printing speed for ink deposition.271
5.3 Comparison across in vitro platforms
Immersing electrodes in inlets to measure TEER values has received considerable criticism.125,208,210,214 Odijk et al. derived an equivalent circuit model based on serial and parallel resistances to show how this electrode placement overestimates TEER values in microfluidic systems by a factor of ∼1.5–5 (higher overestimation for low TEER values).210 This discrepancy is due to the majority of the voltage drop across the cell layer occurring across the cell area close to the inlet and outlet. Subsequent normalization by area multiplication usually assumes that the entire cell area contributes to the resistance and hence leads to an overestimation of TEER. Their model also showed that small imperfections in confluency, such as the cells being 99.6% confluent, can result in a reduction of 80% of the measured TEER. Yeste et al. used a finite element model to investigate effects of electrode placement on final TEER values and found similar results for inlet based electrodes,208 with two-fold overestimation of TEER values often observed in vitro (10–100 Ω cm2). Both groups suggested that correction factors based on modelling of the specific system can be used to correct previously obtained data but solutions without reservoir-based electrodes are preferable. As an alternative to applying a correction factor to TEER values in microfluidic platforms, they advocate an array of interdigitated electrodes positioned along the top and bottom surfaces of the top and bottom channels, respectively, to create a more uniform electric field and minimize these errors in the measurements.208
Beyond channel geometry, however, any variations in electrode location or membrane thickness, porosity and composition can affect TEER values and create discrepancies between measurements for the same cell types.129,204 Moreover, conditions specific to OOC culture may introduce additional error.272 Many OOC setups employ the use of biomaterials like hydrogels and co-culture with support cells, and simply subtracting hydrogel-only TEER values from cell culture devices does not take into account the effect of cell distribution and behaviours, nor the inherent stability of the matrix on the hydrogel's electrical properties.273,274 Finally, common practices of reporting TEER, impedance spectroscopy and ECIS outputs as normalized values can mask flaws in the raw data, as well as making it difficult to compare results across publications.178
6. Future directions
The development of biosensing in OOC barriers will be shaped by innovations in fabrication as well as the priorities considered in sensor design, testing and contextualization.
6.1 Lab-on-PCB
Over the last three decades, OOC device microfabrication techniques that were originally based in manufacturing methods from the semiconductor industry have evolved to better meet the needs of organ-on-chip modelling, including biocompatibility, biosensing, and the complexity of tissue-level microarchitecture. These innovations, however, have largely been led by academic research groups and have focused on proof-of-concept. The resulting drawbacks in the field – lack of standardized production, design guidelines or benchmarks270 – present an obstacle to the commercialization and adoption of OOC systems. As such, there has been a recent swing of the pendulum back toward PCB manufacturing principles and techniques, in the form of lab-on-PCB platforms. The incorporation of PCBs into biosensing platforms could, for example, replace handmade electrodes with custom patterns ordered from PCB manufacturers, covering the standard copper traces with more biocompatible gold,184 or make use of existing standard boards, e.g. Arduino, as a base for microfluidic channels.275 Channels can be 3D-printed directly onto the board,275 or devices assembled in a modular fashion, as in the platform designed by Wu et al., where a glass microfluidic component was attached with PDMS to a flexible PCB substrate featuring flip-chip bonded integrated circuit sensors.276 A modular approach to design and assembly could conceivably be applied to measuring barriers on chip, for example in a bilayer device similar to Wong et al.200,256 for detecting the movement of an electrochemical tracer. Using Lab-on-PCB for barrier chip applications would take advantage of the well-established infrastructure of the PCB industry to improve scalability, standardization and system-level integration277 in OOC devices.
6.2 Flexible electrodes
Various biological barriers, like the lung and gut epithelium, are subject to stretch in vivo, which has motivated the development of OOC models incorporating stretchable barriers.76,91 Innovations like flexible electrodes could enable more direct measurements of barrier models under strain. For example, Zhou et al. developed a stretchable ECIS platform to monitor cell monolayers under strain, with interdigitated gold electrodes integrated onto PDMS membrane.278 As chips with stretchable PDMS membrane for modeling gut and lung barriers are well-established,76,91 one could extrapolate an integration of the stretchable ECIS electrodes into models of the intestinal barrier and lung, monitoring the integrity of the stretched monolayer in real time. Flexible electrodes can also be fabricated on substrates that emulate other microenvironmental features like the topography and mechanical properties of the extracellular matrix. For example, Krysiak et al. incorporated inkjet-printed electrodes into a variety of electrospun micro- and nano-fibrous membranes,279 and Tringides et al. developed a platform that combines graphene flakes and carbon nanotubes with an alginate-based hydrogel to create viscoelastic electrodes that mimic the biomechanical properties of soft tissues like the brain.280
6.3 Non-invasive, real-time monitoring
Understanding the dynamic nature of biological barriers requires integrated sensing that can monitor cell responses in real-time without affecting barrier function.281 The need for non-invasive monitoring is thus particularly pronounced in OOC systems, which are designed with the purpose of providing a controlled microenvironment to recapitulate tissue-level functions. To this end, device designs should prioritize features that reduce disturbance to cell culture and enable sensitive detection of rapid, transient changes, including automated fluid handling, streamlined chip-to-world interfaces, and integrated circuits to combine transducing and signal processing. A greater emphasis on collaborations between disciplines and with pharmaceutical companies can help with designing sensors that fit these goals.171
6.4 Cost of fabrication
The methods commonly used to produced high-resolution features in OOC devices (e.g., soft lithography, electron-beam and X-ray lithography techniques117,252) require the use of expensive equipment, cleanroom facilities and time-consuming steps. This creates obstacles to translation both in terms of resources and expertise. The success of biosensor implementation in OOC will include prioritizing device design and assembly that reflects the need for and recent movement toward developing simplified, lower-cost microfabrication processes.117,271 Low-cost materials like thermoplastics and double-sided adhesives can be combined with techniques like laser cutting, hot embossing, inkjet printing and micromilling to streamline device prototyping as well as electrode fabrication.117,129,282–285 For example, Winkler et al. used laser cut double-sided tape to create a bilayer microfluidic platform for intestinal barrier modelling that cost €0.50 per device.187 Similarly, McIntyre et al. employed micromilling and carbon-based conductive ink to create microfluidic polycarbonate devices with integrated electrodes for a cost of less than $10 USD per device.282 A thorough discussion of low-resource setting techniques for gold electrodes, including screen printing, inkjet printing, and gold leaf fabrication can be found in a recent review from Zamani et al.271 The implementation of these techniques will not only help in democratizing the field for researchers, but may also help boost the pipeline from academia to commercialization through reducing the time and cost for barrier OOC prototyping.
6.5 Standardization
As the variety of microfluidic barrier models increases, so does the importance of easy and direct comparison between different models of the same tissue. Unfortunately, different studies use different methods to benchmark their models. To accelerate the forward movement of the microfluidic barrier modeling field, a more uniform set of evaluation parameters needs to be established.
Permeability is frequently measured to assess the health of a biological barrier but large methodological variations exist in literature. For instance, permeability might be measured for 10 kDa dextran in one paper and for luciferase in another, making comparisons impossible. In addition, permeability can be reported in different ways. The most useful way is to calculate a permeability coefficient that is independent of device geometry and the presence of any membrane or ECM and report it in units of length/time.174 However, results are frequently reported as percent of control or the intensity value at a certain distance from the barrier. Such metrics can be useful within a paper to assess effects of different treatments but is not useful for comparing results between different publications.
Similarly, the analyses used to obtain the permeability constant varies between publications. When calculating the permeability coefficient, the slope of the transported compound as a function of time is used. This slope can be estimated in different ways, such as simple subtraction of two concentration values or as a linear regression in a time series. Alternately, an analytical function can be fitted to the concentration measurements instead of using the derivative. Often, the permeability coefficient obtained from the intensity slope consists of the permeability coefficients for both the cell layer and the support they have been cultured on (if any). To compare to other publications and in vivo data, the barrier contribution to the overall permeability needs to be determined by measuring permeability of a control device.
The same issue found for permeability measurements exists in the case of electrical sensing outputs. TEER measurements too are often given as percent of control or similar rather than being converted to a geometry-independent unit of Ω cm2, making comparisons between systems impossible. While the goal of many studies is to compare the effects of some perturbation (drug, hypoxia, cytokine, etc.) in which case normalization to a control is useful, in order for the reader to be able to ascertain that the barrier model is of high quality and to compare it to other systems, a correctly standardized TEER value should be reported as well. In the same vein, normalized values for in impedance and/or resistance obtained from impedance spectroscopy and ECIS are helpful metrics within the experiment, but do not give an indication of whether this change is meaningful and how to contextualize with existing data. Raw baseline values should be provided as well as electrode areas, and where possible, comparison to existing values in the literature or validation against other established barrier assessment techniques is necessary.
Another area that would benefit from standardization is the design, fabrication and testing of electrodes within an OOC context. Although many barrier-on-chip platforms use similar components (microfluidic channels, culture chambers and integrated sensors), they are for the most part unique proof-of-concept devices developed by academic groups, and lack a systematic approach for classification, functional requirements, and compatibility. This not only creates a barrier to the comparison of measurements between platforms, but also impairs the path to commercialization. Currently there is no standardized testing for electrode failure modes within the OOC context, e.g., the effect of bubbles, particle precipitation, temperature fluctuations, delamination, surface-to-volume effects, etc.253 There is also a lack of guidelines for barrier-on-chip electrical sensing functionalities, including dynamic range, electrode size, sensor readout systems, etc. A number of standards exist in related fields – including microtechnology, medical devices and in vitro cell culture286 – that can help with determining guidelines for barrier-on-chip devices and their integrated biosensors; ISO 22916:2022 for example defines requirements for microfluidic device dimensions and connections. Electronic packaging standards from associations like the Institute of Printed Circuits (IPC) can also be leveraged for this application.253
6.6 Bridging gaps between disciplines and stakeholders
The OOC field by nature is incredibly interdisciplinary, combining both biological and engineering features, as well as a variety of stakeholders in academia, industry and healthcare. The further development of OOC barrier biosensing is therefore highly dependent on bridging gaps between disciplines and stakeholders.
Although barrier-on-chip modelling has seen many exciting innovations in the last decade, widescale adoption requires a consideration of the interests of biologists. Firstly, there is a need for literature that is written not only for engineers, but a broad, non-specialized audience; examples of publications that address this need include reviews written by Simitian et al. and Leung et al.97,98 From the biologist's perspective, development of standardized and user-friendly platforms, as well as an emphasis on contextualizing on-chip biosensing findings to existing in vitro and in vivo methods, will increase the quantity of reliable barrier-on-chip data that is more easily comparable between studies. Conversely, from the engineer's perspective, guidelines published on the specific biological implications of modelling barriers on chip – e.g. limited volumes, gas permeability, thermal stress from physical manipulation of cells287 – as well as the biologically-relevant assays for assessing these effects will provide a better context for how OOC assays relate to standard in vitro assays, and inform decisions about whether a given biological barrier study may benefit from the inclusion of OOC experiments.
Similarly, the incorporation of barrier-on-chip into the pharmaceutical industry's tools for drug development depends on the ability to validate OOC models against standard preclinical and clinical research methods. To this end, various stakeholders in the industry have defined criteria for the qualification of OOC models. Notable examples include GlaxoSmithKline's organ-specific checklists of biological questions and key endpoint assay parameters, as well as a collection of white papers published by members of the International Consortium for Innovation and Quality in Pharmaceutical Development,288 including recommendations for drug safety and ADME experiments in lung,289 skin,290 gut,291 and kidney292 OOC models. Academic researchers should consider these priorities for OOC barriers and use them to guide decisions about device design, cell sources, biosensing techniques, and experimental outputs.
Overall, incorporating principles from product development and mature manufacturing processes at the early stages of platform development can facilitate the pathway to commercialization, and the standardization of OOC barrier biosensing will enable closer and more meaningful collaboration between biologists, engineers, academia, and industry.
Author contributions
AU: conceptualization, visualization, writing – original draft, funding acquisition; HP: conceptualization, writing – original draft, funding acquisition; CAS: conceptualization, writing – review & editing, supervision, project administration, funding acquisition.
Conflicts of interest
The authors declare no conflicts of interest.
Acknowledgements
We are grateful for fellowship support to AU from the Natural Sciences and Engineering Research Council of Canada CREATE Training Program in Organ-on-a-Chip Engineering and Entrepreneurship (TOeP), and to HP from the Swedish Research Council (2016-06646).
References
- L. Cacopardo, J. Costa, S. Giusti, L. Buoncompagni, S. Meucci, A. Corti, G. Mattei and A. Ahluwalia, Biosens. Bioelectron., 2019, 140, 1–17 CrossRef PubMed.
- J. Yeste, X. Illa, M. Alvarez and R. Villa, J. Biol. Eng., 2018, 12, 1–19 CrossRef PubMed.
- R. Yang, T. Wei, H. Goldberg, W. Wang, K. Cullion and D. S. Kohane, Adv. Mater., 2017, 29, 1–25 Search PubMed.
- K. Ronaldson-Bouchard and G. Vunjak-Novakovic, Cell Stem Cell, 2018, 22, 310–324 CrossRef CAS PubMed.
- J. Kim, J. Kim, Y. Jin and S. W. Cho, Biofabrication, 2023, 15, 042002 CrossRef PubMed.
- P. Tawade and M. Mastrangeli, ChemBioChem, 2023, 25, e202300560 CrossRef PubMed.
- H. Nazari, J. Shrestha, V. Y. Naei, S. R. Bazaz, M. Sabbagh, J. P. Thiery and M. E. Warkiani, Biosens. Bioelectron., 2023, 234, 115355 CrossRef CAS PubMed.
- L. Mou, K. Mandal, M. M. Mecwan, A. L. Hernandez, S. Maity, S. Sharma, R. D. Herculano, S. Kawakita, V. Jucaud, M. R. Dokmeci and A. Khademhosseini, Lab Chip, 2022, 22, 3801–3816 RSC.
- M. Mir, S. Palma-Florez, A. Lagunas, M. J. López-Martínez and J. Samitier, ACS Sens., 2022, 7, 1237–1247 CrossRef CAS PubMed.
- C. M. Niessen, J. Invest. Dermatol., 2007, 127, 2525–2532 CrossRef CAS PubMed.
- H. Gonschior, V. Haucke and M. Lehmann, Int. J. Mol. Sci., 2020, 21, 1–15 Search PubMed.
- H. K. Campbell, J. L. Maiers and K. A. DeMali, Exp. Cell Res., 2017, 358, 39–44 CrossRef CAS PubMed.
- Z. Zhao, A. R. Nelson, C. Betsholtz and B. V. Zlokovic, Cell, 2015, 163, 1064–1078 CrossRef CAS PubMed.
- B. T. Hawkins and T. P. Davis, Pharmacol. Rev., 2005, 57, 173–185 CrossRef CAS PubMed.
- C. Zihni, C. Mills, K. Matter and M. S. Balda, Nat. Rev. Mol. Cell Biol., 2016, 17, 564–580 CrossRef CAS PubMed.
- D. Vermette, P. Hu, M. F. Canarie, M. Funaro, J. Glover and R. W. Pierce, Intensive Care Med. Exp., 2018, 6, 1–18 Search PubMed.
- J. M. Mullin, N. Agostino, E. Rendon-Huerta and J. J. Thornton, Drug Discovery Today, 2005, 10, 395–408 CrossRef CAS PubMed.
-
M. E. O'Donnell, in Advances in Pharmacology, 2014, vol. 71, pp. 113–146 Search PubMed.
- E. Belykh, K. V. Shaffer, C. Lin, V. A. Byvaltsev, M. C. Preul and L. Chen, Front. Oncol., 2020, 10, 1–27 CrossRef PubMed.
-
T. Ashraf, A. Kao and R. Bendayan, in Advances in Pharmacology, Elsevier Inc., 1st edn, 2014, vol. 71, pp. 45–111 Search PubMed.
- K. Brune, J. Frank, A. Schwingshackl, J. Finigan and V. K. Sidhaye, Am. J. Physiol., 2015, 308, L731–L745 CAS.
- A. R. Jones and E. V. Shusta, Pharm. Res., 2007, 24, 1759–1771 CrossRef CAS PubMed.
- H. C. Helms, N. J. Abbott, M. Burek, R. Cecchelli, P. O. Couraud, M. A. Deli, C. Förster, H. J. Galla, I. A. Romero, E. V. Shusta, M. J. Stebbins, E. Vandenhaute, B. Weksler, B. Brodin and J. Cereb, Blood Flow Metab., 2016, 36, 862–890 CrossRef CAS PubMed.
- N. Marchi, K. L. Hallene, K. M. Kight, L. Cucullo, G. Moddel, W. Bingaman, G. Dini, A. Vezzani and D. Janigro, BMC Med., 2004, 2, 1–10 CrossRef PubMed.
- R. Kim, Drug Metab. Rev., 2002, 34, 47–54 CrossRef CAS PubMed.
- R. Daneman and A. Prat, Cold Spring Harbor Perspect. Biol., 2015, 7, a020412 CrossRef PubMed.
- D. Keszthelyi, F. J. Troost, D. M. Jonkers, H. M. Van Eijk, P. J. Lindsey, J. Dekker and W. A. Buurman, Aliment. Pharmacol. Ther., 2014, 392–402 CrossRef CAS PubMed.
- J. R. Kelly, P. J. Kennedy, J. F. Cryan and T. G. Dinan, Front. Cell. Neurosci., 2015, 9, 392 Search PubMed.
-
M. Hannocks, J. Huppert, X. Zhang, E. Korpos and L. Sorokin, in The Blood Brain Barrier and Inflammation, 2017, pp. 49–60 Search PubMed.
- S. C. Bischoff, G. Barbara, W. Buurman, T. Ockhuizen, J. Schulzke, M. Serino, H. Tilg, A. Watson and J. M. Wells, BMC Gastroenterol., 2014, 14, 189 CrossRef PubMed.
- J. Zhang, S. Chen, Z. Hou, J. Cai, M. Dong and X. Shi, PLoS One, 2015, 10, e0122572 CrossRef PubMed.
- J. Sugarman, J. Fluhr, A. Fowler, T. Bruckner, T. L. Diepgen and M. L. Williams, Arch. Dermatol., 2003, 139, 1417–1422 Search PubMed.
- W. M. Pardridge, Expert Opin. Ther. Targets, 2015, 19, 1059–1072 CrossRef CAS PubMed.
- Y. Xu, N. Shrestha, V. Préat and A. Beloqui, J. Controlled Release, 2020, 322, 486–508 CrossRef CAS PubMed.
- O. Vince, S. Peeters, V. A. Johanssen, M. Gray, S. Smart, N. R. Sibson and E. Stride, Adv. Healthcare Mater., 2021, 10, 1–11 Search PubMed.
- E. H. Taudorf, C. M. Lerche, A. M. Erlendsson, P. A. Philipsen, S. H. Hansen, C. Janfelt, U. Paasch, Ã. R. R. Anderson and M. Haedersdal, Lasers Surg. Med., 2016, 529, 519–529 CrossRef PubMed.
- A. H. Sabri, Y. Kim, M. Marlow, D. J. Scurr, J. Segal, A. K. Banga, L. Kagan and J. Bong, Adv. Drug Delivery Rev., 2019, 153, 195–215 CrossRef PubMed.
- S. Ding, A. I. Khan, X. Cai, Y. Song, Z. Lyu, D. Du, P. Dutta and Y. Lin, Mater. Today, 2020, 37, 112–125 CrossRef CAS PubMed.
- V. A. Pedicord, A. A. K. Lockhart, K. J. Rangan, J. W. Craig, J. Loschko, A. Rogoz, H. C. Hang and D. Mucida, Sci. Immunol., 2016, 1, eaai7732 Search PubMed.
- A. Zhou, Y. Yuan, M. Yang, Y. Huang, X. Li, S. Li, S. Yang and B. Tang, Front. Cell. Infect. Microbiol., 2022, 12, 1–11 Search PubMed.
- S. Vaishnava, M. Yamamoto, K. M. Severson, K. A. Ruhn, X. Yu, O. Koren, R. Ley, E. K. Wakeland and L. V. Hooper, Science, 2011, 334, 255–258 CrossRef CAS PubMed.
- C. Pérez-González, G. Ceada, M. Matejčić and X. Trepat, Curr. Opin. Genet. Dev., 2022, 72, 82–90 CrossRef PubMed.
- A. Nishiguchi, C. Gilmore, A. Sood, M. Matsusaki, G. Collett, D. Tannetta, I. L. Sargent, J. McGarvey, N. D. Halemani, J. Hanley, F. Day, S. Grant, C. Murdoch-Davis, H. Kemp, P. Verkade, J. D. Aplin, M. Akashi and C. P. Case, Biomaterials, 2019, 192, 140–148 CrossRef CAS PubMed.
- L. Aengenheister, K. Keevend, C. Muoth, R. Schönenberger, L. Diener, P. Wick and T. Buerki-Thurnherr, Sci. Rep., 2018, 8, 1–12 CAS.
- N. Arumugasaamy, K. D. Rock, C. Y. Kuo, T. L. Bale and J. P. Fisher, Adv. Drug Delivery Rev., 2020, 161–162, 161–175 CrossRef CAS PubMed.
- R. Levkovitz, U. Zaretzky, A. Jaffa, M. Hod and D. Elad, Placenta, 2013, 34, 708–715 CrossRef CAS PubMed.
- J. Stefulj, U. Panzenboeck, T. Becker, B. Hirschmugl, C. Schweinzer, I. Lang, G. Marsche, A. Sadjak, U. Lang, G. Desoye and C. Wadsack, Circ. Res., 2009, 104, 600–608 CrossRef CAS PubMed.
- L. L. Rubin, D. E. Hall, S. Porter, K. Barbu, C. Cannon, H. C. Homer, M. Janatpour, C. W. Liaw, K. Manning, J. Morales, L. Tanner, K. J. Tbmaselli and F. Bard, J. Cell Biol., 1991, 115, 1725–1735 CrossRef CAS PubMed.
- R. Cecchelli, B. Dehouck, L. Descamps, L. Fenart, V. Buée-Scherrer, C. Duhem, S. Lundquist, M. Rentfel, G. Torpier and M. P. Dehouck, Adv. Drug Delivery Rev., 1999, 36, 165–178 CrossRef CAS PubMed.
- P. J. Gaillard, L. H. Voorwinden, J. L. Nielsen, A. Ivanov, R. Atsumi, H. Engman, C. Ringbom, A. G. De Boer and D. D. Breimer, Eur. J. Pharm. Sci., 2001, 12, 215–222 CrossRef CAS PubMed.
- M. Boveri, V. Berezowski, A. Price, S. Slupek, A. M. Lenfant, C. Benaud, T. Hartung, R. Cecchelli, P. Prieto and M. P. Dehouck, Glia, 2005, 51, 187–198 CrossRef PubMed.
- N. Perrière, S. Yousif, S. Cazaubon, N. Chaverot, F. Bourasset, S. Cisternino, X. Declèves, S. Hori, T. Terasaki, M. Deli, J. M. Scherrmann, J. Temsamani, F. Roux and P. O. Couraud, Brain Res., 2007, 1150, 1–13 CrossRef PubMed.
- S. Nakagawa, M. A. Deli, S. Nakao, M. Honda, K. Hayashi, R. Nakaoke, Y. Kataoka and M. Niwa, Cell. Mol. Neurobiol., 2007, 27, 687–694 CrossRef CAS PubMed.
- S. Nakagawa, M. A. Deli, H. Kawaguchi, T. Shimizudani, T. Shimono, Á. Kittel, K. Tanaka and M. Niwa, Neurochem. Int., 2009, 54, 253–263 CrossRef CAS PubMed.
- S. Dohgu, F. Takata, A. Yamauchi, S. Nakagawa, T. Egawa, M. Naito, T. Tsuruo, Y. Sawada, M. Niwa and Y. Kataoka, Brain Res., 2005, 1038, 208–215 CrossRef CAS PubMed.
- K. Hatherell, P. O. Couraud, I. A. Romero, B. Weksler and G. J. Pilkington, J. Neurosci. Methods, 2011, 199, 223–229 CrossRef PubMed.
- A. Appelt-Menzel, A. Cubukova, K. Günther, F. Edenhofer, J. Piontek, G. Krause, T. Stüber, H. Walles, W. Neuhaus and M. Metzger, Stem Cell Rep., 2017, 8, 894–906 CrossRef CAS PubMed.
- L. Norlén, M. Lundborg, C. Wennberg, A. Narangifard and B. Daneholt, J. Invest. Dermatol., 2022, 142, 285–292 CrossRef PubMed.
- L. C. Biggs, C. S. Kim, Y. A. Miroshnikova and S. A. Wickström, J. Invest. Dermatol., 2020, 140, 284–290 CrossRef CAS PubMed.
- S. A. Mobasseri, S. Zijl, V. Salameti, G. Walko, A. Stannard, S. Garcia-Manyes and F. M. Watt, Acta Biomater., 2019, 87, 256–264 CrossRef CAS PubMed.
- D. J. Huels and J. P. Medema, Cell Stem Cell, 2018, 22, 7–9 CrossRef CAS PubMed.
- J. I. Alvarez, T. Katayama and A. Prat, Glia, 2013, 61, 1939–1958 CrossRef PubMed.
- E. W. K. Young and C. A. Simmons, Lab Chip, 2010, 10, 143–160 RSC.
- N. Baeyens, S. Yun, M. A. Schwartz, N. Baeyens, C. Bandyopadhyay, B. G. Coon, S. Yun and M. A. Schwartz, J. Clin. Invest., 2016, 126, 821–828 CrossRef PubMed.
- F. Vozzi, F. Bianchi, A. Ahluwalia and C. Domenici, Biotechnol. J., 2014, 9, 146–154 CrossRef CAS PubMed.
- M. Bongrazio, C. Baumann, A. Zakrzewicz, A. R. Pries and P. Gaehtgens, Cardiovasc. Res., 2000, 47, 384–393 CrossRef CAS PubMed.
- K. M. Gray and K. M. Stroka, Semin. Cell Dev. Biol., 2017, 71, 106–117 CrossRef CAS PubMed.
- L. Yan, R. A. Moriarty and K. M. Stroka, Theranostics, 2021, 11, 10148–10170 CrossRef CAS PubMed.
- J. G. Destefano, J. J. Jamieson, R. M. Linville and P. C. Searson, Fluids Barriers CNS, 2018, 15, 1–15 CrossRef PubMed.
- S. Jeong, S. Kim, J. Buonocore, J. Park, C. J. Welsh, J. Li and A. Han, IEEE Trans. Biomed. Eng., 2018, 65, 431–439 Search PubMed.
- L. Cucullo, M. Hossain, V. Puvenna, N. Marchi and D. Janigro, BMC Neurosci., 2011, 12, 1–15 CrossRef PubMed.
- G. D. Vatine, R. Barrile, M. J. Workman, S. Sances, B. K. Barriga, M. Rahnama, S. Barthakur, M. Kasendra, C. Lucchesi, J. Kerns, N. Wen, W. R. Spivia, Z. Chen, J. Van Eyk and C. N. Svendsen, Cell Stem Cell, 2019, 24, 995–1005.e6 CrossRef CAS PubMed.
- K. Kanasaki, Y. Kanda, K. Palmsten, H. Tanjore, S. B. Lee, V. S. Lebleu, V. H. Gattone and R. Kalluri, Dev. Biol., 2008, 313, 584–593 CrossRef CAS PubMed.
- K. M. DiKun and L. J. Gudas, Pharmacol. Ther., 2023, 248, 108481 CrossRef CAS PubMed.
- H. S. Bevan, S. C. Slater, H. Clarke, P. A. Cahill, P. W. Mathieson, G. I. Welsh and S. C. Satchell, Am. J. Physiol., 2011, 301, F733–F742 Search PubMed.
- H. J. Kim, D. Huh, G. Hamilton and D. E. Ingber, Lab Chip, 2012, 12, 2165–2174 RSC.
- A. J. Clevenger, M. K. McFarlin, C. A. Collier, V. S. Sheshadri, A. K. Madyastha, J. P. M. Gorley, S. C. Solberg, A. N. Stratman and S. A. Raghavan, Cell. Mol. Bioeng., 2023, 16, 261–281 CrossRef CAS PubMed.
- R. Cao, Y. Wang, J. Liu, L. Rong and J. Qin, Cell Proliferation, 2023, 56, 1–15 Search PubMed.
- J. Yracheta, W. Muraoka, X. Wu, D. Burmeister, D. Darlington, D. Zhao, Z. Lai, S. Sayyadioskoie, A. P. Cap, J. Bynum and S. E. Nicholson, J. Trauma Acute Care Surg., 2021, 91, 1002–1009 CrossRef CAS PubMed.
- P. P. Partyka, G. A. Godsey, J. R. Galie, M. C. Kosciuk, N. K. Acharya, R. G. Nagele and P. A. Galie, Biomaterials, 2017, 115, 30–39 CrossRef CAS PubMed.
- N. Schuff, S. Matsumoto, J. Kmiecik, C. Studholme, A. Du, F. Ezekiel, B. L. Miller, J. H. Kramer, W. J. Jagust, H. C. Chui and M. W. Weiner, Alzheimer's Dementia, 2009, 5, 454–462 CrossRef PubMed.
- A. Wallin, K. Blennow, P. Fredman, C. G. Gottfries, I. Karlsson and L. Svenner-holm, Acta Neurol. Scand., 1990, 81, 318–322 CrossRef CAS PubMed.
- F. Meng, C. Shen, L. Yang, C. Ni, J. Huang, K. Lin, Z. Cao, S. Xu, W. Cui, X. Wang, B. Zhou, C. Xiong, J. Wang and B. Zhao, Cell Regener., 2022, 11, 1–14 CrossRef PubMed.
- C. P. Gayer and M. D. Basson, Cell. Signalling, 2009, 21, 1237–1244 CrossRef CAS PubMed.
- N. P. Tallapragada, H. M. Cambra, T. Wald, S. Keough Jalbert, D. M. Abraham, O. D. Klein and A. M. Klein, Cell Stem Cell, 2021, 28, 1516–1532.e14 CrossRef CAS PubMed.
- A. Grassart, V. Malardé, S. Gobba, A. Sartori-Rupp, J. Kerns, K. Karalis, B. Marteyn, P. Sansonetti and N. Sauvonnet, Cell Host Microbe, 2019, 26, 435–444.e4 CrossRef CAS PubMed.
- A. Marchioni, R. Tonelli, S. Cerri, I. Castaniere, D. Andrisani, F. Gozzi, G. Bruzzi, L. Manicardi, A. Moretti, J. Demurtas, S. Baroncini, A. Andreani, G. F. Cappiello, S. Busani, R. Fantini, L. Tabbì, A. V. Samarelli and E. Clini, Int. J. Mol. Sci., 2021, 22, 1–14 Search PubMed.
- C. D. Foster, L. S. Varghese, L. W. Gonzales, S. S. Margulies and S. H. Guttentag, Pediatr. Res., 2010, 67, 585–590 CrossRef CAS PubMed.
- M. Zhong, W. Wu, H. Kang, Z. Hong, S. Xiong, X. Gao, J. Rehman, Y. A. Komarova and A. B. Malik, Am. J. Respir. Cell Mol. Biol., 2020, 62, 168–177 CrossRef CAS PubMed.
- E. Roan and C. M. Waters, Am. J. Physiol., 2011, 301, 625–635 Search PubMed.
- D. Huh, B. D. Matthews, A. Mammoto, M. Montoya-Zavala, H. Yuan Hsin and D. E. Ingber, Science, 2010, 328, 1662–1668 CrossRef CAS PubMed.
- D. Huh, D. C. Leslie, B. D. Matthews, J. P. Fraser, S. Jurek, G. A. Hamilton, K. S. Thorneloe, M. A. McAlexander and D. E. Ingber, Sci. Transl. Med., 2012, 4, 159ra147 Search PubMed.
- M. J. Carroll, L. E. Stopfer and P. K. Kreeger, Chem. Commun., 2014, 50, 5279–5281 RSC.
- A. Wolff, M. Antfolk, B. Brodin and M. Tenje, J. Pharm. Sci., 2015, 104, 2727–2746 CrossRef CAS PubMed.
- S. Boyden, J. Exp. Med., 1962, 115, 453–466 CrossRef CAS PubMed.
-
J. Marshall, in Cell Migration: Developmental Methods and Protocols, ed. C. M. Wells and M. Parsons, Humana Press, Totowa, NJ, 2011, pp. 97–110 Search PubMed.
- C. M. Leung, P. de Haan, K. Ronaldson-Bouchard, G. A. Kim, J. Ko, H. S. Rho, Z. Chen, P. Habibovic, N. L. Jeon, S. Takayama, M. L. Shuler, G. Vunjak-Novakovic, O. Frey, E. Verpoorte and Y. C. Toh, Nat. Rev. Methods Primers, 2022, 2, 33 CrossRef CAS.
- G. Simitian, M. Virumbrales-Muñoz, C. Sánchez-De-Diego, D. J. Beebe and D. Kosoff, Lab Chip, 2022, 22, 3618–3636 RSC.
- H. Persson, S. Park, M. Mohan, K. K. Cheung, C. A. Simmons and E. W. K. Young, Sens. Actuators, B, 2022, 356, 131342 CrossRef CAS.
- I. Matthiesen, D. Voulgaris, P. Nikolakopoulou, T. E. Winkler and A. Herland, Small, 2021, 17, 2101785 CrossRef CAS PubMed.
- M. Kasendra, A. Tovaglieri, A. Sontheimer-Phelps, S. Jalili-Firoozinezhad, A. Bein, A. Chalkiadaki, W. Scholl, C. Zhang, H. Rickner, C. A. Richmond, H. Li, D. T. Breault and D. E. Ingber, Sci. Rep., 2018, 8, 1–14 CAS.
- S. Park and E. W. K. Young, Adv. Mater. Technol., 2021, 6, 1–12 Search PubMed.
- J. W. Song, S. P. Cavnar, A. C. Walker, K. E. Luker, M. Gupta, Y. C. Tung, G. D. Luker and S. Takayama, PLoS One, 2009, 4, e5756 CrossRef PubMed.
- S. Srigunapalan, C. Lam, A. R. Wheeler and C. A. Simmons, Biomicrofluidics, 2011, 5, 013409 CrossRef PubMed.
- M. B. Chen, S. Srigunapalan, A. R. Wheeler and C. A. Simmons, Lab Chip, 2013, 13, 2591 RSC.
- R. Molteni, E. Bianchi, P. Patete, M. Fabbri, G. Baroni, G. Dubini and R. Pardi, Lab Chip, 2015, 15, 195–207 RSC.
- M. J. Powers, D. M. Janigian, K. E. Wack, C. S. Baker, D. B. Stolz and L. G. Griffith, Tissue Eng., 2002, 8, 499–513 CrossRef PubMed.
- M. J. Powers, K. Domansky, M. R. Kaazempur-Mofrad, A. Kalezi, A. Capitano, A. Upadhyaya, P. Kurzawski, K. E. Wack, D. B. Stolz, R. Kamm, L. G. Griffith and L. G. Grif, Biotechnol. Bioeng., 2002, 78, 257–269 CrossRef CAS PubMed.
- A. Carraro, W. M. Hsu, K. M. Kulig, W. S. Cheung, M. L. Miller, E. J. Weinberg, E. F. Swart, M. Kaazempur-Mofrad, J. T. Borenstein, J. P. Vacanti and C. Neville, Biomed. Microdevices, 2008, 10, 795–805 CrossRef PubMed.
- A. Herland, B. M. Maoz, D. Das, M. R. Somayaji, R. Prantil-Baun, R. Novak, M. Cronce, T. Huffstater, S. S. F. Jeanty, M. Ingram, A. Chalkiadaki, D. Benson Chou, S. Marquez, A. Delahanty, S. Jalili-Firoozinezhad, Y. Milton, A. Sontheimer-Phelps, B. Swenor, O. Levy, K. K. Parker, A. Przekwas and D. E. Ingber, Nat. Biomed. Eng., 2020, 4, 421–436 CrossRef CAS PubMed.
- K. H. Benam, R. Novak, J. Nawroth, M. Hirano-Kobayashi, T. C. Ferrante, Y. Choe, R. Prantil-Baun, J. C. Weaver, A. Bahinski, K. K. Parker and D. E. Ingber, Cell Syst., 2016, 3, 456–466.e4 CrossRef CAS PubMed.
- K. H. Benam, R. Villenave, C. Lucchesi, A. Varone, C. Hubeau, H. H. Lee, S. E. Alves, M. Salmon, T. C. Ferrante, J. C. Weaver, A. Bahinski, G. A. Hamilton and D. E. Ingber, Nat. Methods, 2016, 13, 151–157 CrossRef CAS PubMed.
- M. W. van der Helm, O. Y. F. Henry, A. Bein, T. Hamkins-Indik, M. J. Cronce, W. D. Leineweber, M. Odijk, A. D. van der Meer, L. I. Segerink and D. E. Ingber, Lab Chip, 2019, 19, 452–463 RSC.
- A. Tovaglieri, A. Sontheimer-Phelps, A. Geirnaert, R. Prantil-Baun, D. M. Camacho, D. B. Chou, S. Jalili-Firoozinezhad, T. De Wouters, M. Kasendra, M. Super, M. J. Cartwright, C. A. Richmond, D. T. Breault, C. Lacroix and D. E. Ingber, Microbiome, 2019, 7, 43 CrossRef PubMed.
- S. Jalili-Firoozinezhad, F. S. Gazzaniga, E. L. Calamari, D. M. Camacho, C. W. Fadel, A. Bein, B. Swenor, B. Nestor, M. J. Cronce, A. Tovaglieri, O. Levy, K. E. Gregory, D. T. Breault, J. M. S. Cabral, D. L. Kasper, R. Novak and D. E. Ingber, Nat. Biomed. Eng., 2019, 3, 520–531 CrossRef CAS PubMed.
- A. Sontheimer-Phelps, D. B. Chou, A. Tovaglieri, T. C. Ferrante, T. Duckworth, C. Fadel, V. Frismantas, A. D. Sutherland, S. Jalili-Firoozinezhad, M. Kasendra, E. Stas, J. C. Weaver, C. A. Richmond, O. Levy, R. Prantil-Baun, D. T. Breault and D. E. Ingber, Cell. Mol. Gastroenterol. Hepatol., 2020, 9, 507–526 CrossRef PubMed.
- T. E. Winkler, M. Feil, E. F. G. J. Stronkman, I. Matthiesen and A. Herland, Lab Chip, 2020, 20, 1212–1226 RSC.
- R. Booth and H. Kim, Lab Chip, 2012, 12, 1784–1792 RSC.
- R. Booth and H. Kim, Ann. Biomed. Eng., 2014, 42, 2379–2391 CrossRef CAS PubMed.
- D. J. Alcendor, F. E. Block III, D. E. Cliffel, J. S. Daniels, K. L. Ellacott, C. R. Goodwin, L. H. Hofmeister, D. Li, D. A. Markov, J. C. May, L. J. McCawley, B. McLaughlin, J. A. McLean, K. D. Niswender, V. Pensabene, K. T. Seale, S. D. Sherrod, H.-J. Sung, D. L. Tabb, D. J. Webb and J. P. Wikswo, Stem Cell Res. Ther., 2013, 4, 1–5 CrossRef PubMed.
- B. Prabhakarpandian, M.-C. Shen, J. B. Nichols, I. R. Mills, M. Sidoryk-Wegrzynowicz, M. Aschner and K. Pant, Lab Chip, 2013, 13, 1093 RSC.
- L. M. Griep, F. Wolbers, B. De Wagenaar, P. M. Ter Braak, B. B. Weksler, I. A. Romero, P. O. Couraud, I. Vermes, A. D. Van Der Meer and A. Van Den Berg, Biomed. Microdevices, 2013, 15, 145–150 CrossRef CAS PubMed.
- A. K. H. Achyuta, A. J. Conway, R. B. Crouse, E. C. Bannister, R. N. Lee, C. P. Katnik, A. A. Behensky, J. Cuevas and S. S. Sundaram, Lab Chip, 2013, 13, 542–553 RSC.
- K. L. Sellgren, B. T. Hawkins and S. Grego, Biomicrofluidics, 2015, 9, 6–10 CrossRef PubMed.
- J. A. Brown, V. Pensabene, D. A. Markov, V. Allwardt, M. Diana Neely, M. Shi, C. M. Britt, O. S. Hoilett, Q. Yang, B. M. Brewer, P. C. Samson, L. J. McCawley, J. M. May, D. J. Webb, D. Li, A. B. Bowman, R. S. Reiserer and J. P. Wikswo, Biomicrofluidics, 2015, 9, 1–15 CrossRef PubMed.
- Y. I. Wang, H. E. Abaci and M. L. Shuler, Biotechnol. Bioeng., 2017, 114, 184–194 CrossRef CAS PubMed.
- K. Achberger, C. Probst, J. C. Haderspeck, S. Bolz, J. Rogal, J. Chuchuy, M. Nikolova, V. Cora, L. Antkowiak, W. Haq, N. Shen, K. Schenke-Layland, M. Ueffing, S. Liebau and P. Loskill, eLife, 2019, 8, 1–26 CrossRef PubMed.
- K. Achberger, M. Cipriano, M. J. Düchs, C. Schön, S. Michelfelder, B. Stierstorfer, T. Lamla, S. G. Kauschke, J. Chuchuy, J. Roosz, L. Mesch, V. Cora, S. Pars, N. Pashkovskaia, S. Corti, S. M. Hartmann, A. Kleger, S. Kreuz, U. Maier, S. Liebau and P. Loskill, Stem Cell Rep., 2021, 16, 2242–2256 CrossRef CAS PubMed.
- P. Schuller, M. Rothbauer, S. R. A. Kratz, G. Höll, P. Taus, M. Schinnerl, J. Genser, N. Bastus, O. H. Moriones, V. Puntes, B. Huppertz, M. Siwetz, H. Wanzenböck and P. Ertl, Sens. Actuators, B, 2020, 312, 127946 CrossRef CAS.
- A. Apostolou, R. A. Panchakshari, A. Banerjee, D. V. Manatakis, M. D. Paraskevopoulou, R. Luc, G. Abu-Ali, A. Dimitriou, C. Lucchesi, G. Kulkarni, T. I. Maulana, M. Kasendra, J. S. Kerns, B. Bleck, L. Ewart, E. S. Manolakos, G. A. Hamilton, C. Giallourakis and K. Karalis, Cell. Mol. Gastroenterol. Hepatol., 2021, 12, 1719–1741 CrossRef CAS PubMed.
- E. M. Shaughnessey, S. H. Kann, H. Azizgolshani, L. D. Black, J. L. Charest and E. M. Vedula, Sci. Rep., 2022, 12, 13182 CrossRef CAS PubMed.
- A. L. Gard, R. J. Luu, R. Maloney, M. H. Cooper, B. P. Cain, H. Azizgolshani, B. C. Isenberg, J. T. Borenstein, J. Ong, J. L. Charest and E. M. Vedula, Commun. Biol., 2023, 6, 1–12 CrossRef PubMed.
- H. Azizgolshani, J. R. Coppeta, E. M. Vedula, E. E. Marr, B. P. Cain, R. J. Luu, M. P. Lech, S. H. Kann, T. J. Mulhern, V. Tandon, K. Tan, N. J. Haroutunian, P. Keegan, M. Rogers, A. L. Gard, K. B. Baldwin, J. C. de Souza, B. C. Hoefler, S. S. Bale, L. B. Kratchman, A. Zorn, A. Patterson, E. S. Kim, T. A. Petrie, E. L. Wiellette, C. Williams, B. C. Isenberg and J. L. Charest, Lab Chip, 2021, 21, 1454–1474 RSC.
- P. Q. H. Nguyen, D. D. Duong, J. D. Kwun and N. Y. Lee, Biomed. Microdevices, 2019, 21, 1–11 Search PubMed.
- A. R. Aref, R. Y. J. Huang, W. Yu, K. N. Chua, W. Sun, T. Y. Tu, J. Bai, W. J. Sim, I. K. Zervantonakis, J. P. Thiery and R. D. Kamm, Integr. Biol., 2013, 5, 381–389 CrossRef CAS PubMed.
- M. B. Chen, J. A. Whisler, J. S. Jeon and R. D. Kamm, Integr. Biol., 2013, 5, 1262–1271 CrossRef CAS PubMed.
- G. Adriani, D. Ma, A. Pavesi, R. D. Kamm and E. L. K. Goh, Lab Chip, 2017, 17, 448 RSC.
- J. F. Wong, M. D. Mohan, E. W. K. Young and C. A. Simmons, Biosens. Bioelectron., 2020, 147, 111757 CrossRef CAS PubMed.
- M. Campisi, Y. Shin, T. Osaki, C. Hajal, V. Chiono and R. D. Kamm, Biomaterials, 2018, 180, 117–129 CrossRef CAS PubMed.
- Y. Xiao, D. Kim, B. Dura, K. Zhang, R. Yan, H. Li, E. Han, J. Ip, P. Zou, J. Liu, A. T. Chen, A. O. Vortmeyer, J. Zhou and R. Fan, Adv. Sci., 2019, 6, 1801531 CrossRef PubMed.
- H. Ehlers, A. Nicolas, F. Schavemaker, J. P. M. Heijmans, M. Bulst, S. J. Trietsch and L. J. van den Broek, Front. Immunol., 2023, 14, 1–11 Search PubMed.
- A. Nicolas, F. Schavemaker, K. Kosim, D. Kurek, M. Haarmans, M. Bulst, K. Lee, S. Wegner, T. Hankemeier, J. Joore, K. Domansky, H. L. Lanz, P. Vulto and S. J. Trietsch, Lab Chip, 2021, 21, 1676–1685 RSC.
- V. K. Lee, D. Y. Kim, H. Ngo, Y. Lee, L. Seo, S. S. Yoo, P. A. Vincent and G. Dai, Biomaterials, 2014, 35, 8092–8102 CrossRef CAS PubMed.
- Y. Zhang, Y. Yu and I. T. Ozbolat, J. Nanotechnol. Eng. Med., 2013, 4, 1–7 CAS.
- K. A. Homan, D. B. Kolesky, M. A. Skylar-Scott, J. Herrmann, H. Obuobi, A. Moisan and J. A. Lewis, Sci. Rep., 2016, 6, 1–13 CrossRef PubMed.
- G. M. Price, K. H. K. Wong, J. G. Truslow, A. D. Leung and J. Tien, Biomaterials, 2010, 31, 6182–6189 CrossRef CAS PubMed.
- J. A. Kim, H. N. Kim, S. K. Im, S. Chung, J. Y. Kang, N. Choi, N. Kim, S. K. Im and S. Chung, Biomicrofluidics, 2015, 9, 1–15 Search PubMed.
- J. A. Jiménez-Torres, S. L. Peery, K. E. Sung and D. J. Beebe, Adv. Healthcare Mater., 2016, 5, 198–204 CrossRef PubMed.
- A. P. Golden and J. Tien, Lab Chip, 2007, 7, 720–725 RSC.
- Y. Zheng, J. Chen, M. Craven, N. W. Choi, S. Totorica, A. Diaz-Santana, P. Kermani, B. Hempstead, C. Fischbach-Teschl, J. A. López and A. D. Stroock, Proc. Natl. Acad. Sci. U. S. A., 2012, 109, 9342–9347 CrossRef CAS PubMed.
- L. L. Bischel, E. W. K. Young, B. R. Mader and D. J. Beebe, Biomaterials, 2013, 34, 1471–1477 CrossRef CAS PubMed.
- A. Herland, A. D. Van Der Meer, E. A. FitzGerald, T. E. Park, J. J. F. Sleeboom and D. E. Ingber, PLoS One, 2016, 11, 1–21 CrossRef PubMed.
- T. T. G. Nieskens, M. Persson, E. J. Kelly and A. K. Sjögren, Drug Metab. Dispos., 2020, 48, 1303–1311 CrossRef CAS PubMed.
- C. Maass, N. B. Sorensen, J. Himmelfarb, E. J. Kelly, C. L. Stokes and M. Cirit, CPT: Pharmacometrics Syst. Pharmacol., 2019, 8, 316–325 CAS.
- C. Sakolish, Z. Chen, C. Dalaijamts, K. Mitra, Y. Liu, T. Fulton, T. L. Wade, E. J. Kelly, I. Rusyn and W. A. Chiu, Toxicol. In Vitro, 2020, 63, 104752 CrossRef CAS PubMed.
- M. Zakharova, M. P. Tibbe, L. S. Koch, H. Le-The, A. M. Leferink, A. van den Berg, A. D. van der Meer, K. Broersen and L. I. Segerink, Adv. Mater. Technol., 2021, 6, 2100138 CrossRef CAS.
- W. F. Quirós-Solano, N. Gaio, O. M. J. A. Stassen, Y. B. Arik, C. Silvestri, N. C. A. Van Engeland, A. Van der Meer, R. Passier, C. M. Sahlgren, C. V. C. Bouten, A. van den Berg, R. Dekker and P. M. Sarro, Sci. Rep., 2018, 8, 1–11 Search PubMed.
-
F. Zheng, Q. Li, P. Yang, S. Qiu, H. Mao and J. Zhao, 21st Int. Conf. Solid-State Sensors, Actuators Microsystems, TRANSDUCERS 2021, 2021, pp. 279–282 Search PubMed.
- H. Iwadate, M. Yamada, N. Kimua, R. Hashimoto, Y. Yajim, R. Utoh and M. Seki, Sens. Actuators, B, 2019, 486–495 CrossRef CAS.
- J. Youn, H. Hong, W. Shin, D. Kim, H. J. Kim and D. S. Kim, Biofabrication, 2022, 14, 025010 CrossRef PubMed.
- C. P. Tasiopoulos, L. Gustafsson, W. Van Der Wijngaart and M. Hedhammar, ACS Biomater. Sci. Eng., 2021, 7, 3332–3339 CrossRef CAS PubMed.
- X. Yang, K. Li, X. Zhang, C. Liu, B. Guo, W. Wen and X. Gao, Lab Chip, 2018, 18, 486–495 RSC.
- S. Kim, H. J. Kim and N. L. Jeon, Integr. Biol., 2010, 2, 584–603 CrossRef CAS PubMed.
- R. F. Taylor, T. H. Price, S. M. Schwartz and D. C. Dale, J. Clin. Invest., 1981, 67, 584–587 CrossRef CAS PubMed.
- M. W. van der Helm, A. D. van der Meer, J. C. T. Eijkel, A. van den Berg and L. I. Segerink, Tissue Barriers, 2016, 4, e1142493 CrossRef PubMed.
- D. Huh, B. D. Matthews, A. Mammoto, M. Montoya-Zavala, H. Y. Hsin and D. E. Ingber, Science, 2010, 328, 1662–1668 CrossRef CAS PubMed.
- R. G. Wells, Hepatology, 2008, 47, 1394–1400 CrossRef CAS PubMed.
- C. M. Hovell, Y. J. Sei and Y. Kim, J. Lab. Autom., 2015, 20, 251–258 CrossRef CAS PubMed.
- M. I. Bogorad, J. DeStefano, J. Karlsson, A. D. Wong, S. Gerecht and P. C. Searson, Lab Chip, 2015, 15, 4242–4255 RSC.
- B. Srinivasan, A. R. Kolli, M. B. Esch, H. E. Abaci, M. L. Shuler and J. J. Hickman, J. Lab. Autom., 2015, 20, 107–126 CrossRef CAS PubMed.
- E. Ferrari, C. Palma, S. Vesentini, P. Occhetta and M. Rasponi, Biosensors, 2020, 10, 110 CrossRef CAS PubMed.
- Y. Zhu, K. Mandal, A. L. Hernandez, S. Kawakita, W. Huang, P. Bandaru, S. Ahadian, H. J. Kim, V. Jucaud, M. R. Dokmeci and A. Khademhosseini, Curr. Opin. Biomed. Eng., 2021, 19, 100309 CrossRef PubMed.
- I. Hubatsch, E. G. E. Ragnarsson and P. Artursson, Nat. Protoc., 2007, 2, 2111–2119 CrossRef CAS PubMed.
- V. H. Huxley, F. E. Curry and R. H. Adamson, Am. J. Physiol., 1987, 252, H188–H197 CAS.
-
S. Tavelin, J. Gråsjö, J. Taipalensuu, G. Ocklind and P. Artursson, in Epithelial Cell Culture Protocols, ed. C. Wise, Humana Press, New Jersey, 2002, vol. 188, pp. 233–272 Search PubMed.
- T. E. Park, N. Mustafaoglu, A. Herland, R. Hasselkus, R. Mannix, E. A. FitzGerald, R. Prantil-Baun, A. Watters, O. Henry, M. Benz, H. Sanchez, H. J. McCrea, L. C. Goumnerova, H. W. Song, S. P. Palecek, E. Shusta and D. E. Ingber, Nat. Commun., 2019, 10, 1–12 CrossRef PubMed.
- C. Kwok, A. Marshall, J. Rasko and J. Wong, J. Hematol. Oncol., 2017, 10, 1–6 CrossRef PubMed.
- J. A. Stolwijk, K. Matrougui, C. W. Renken and M. Trebak, Pflugers Arch., 2015, 467, 2193–2218 CrossRef CAS PubMed.
- A. Oddo, B. Peng, Z. Tong, Y. Wei, W. Y. Tong, H. Thissen and N. H. Voelcker, Trends Biotechnol., 2019, 37, 1295–1314 CrossRef CAS PubMed.
- P. de Haan, M. J. C. Santbergen, M. van der Zande, H. Bouwmeester, M. W. F. Nielen and E. Verpoorte, Sci. Rep., 2021, 11, 1–13 CrossRef PubMed.
- I. Wilhelm and I. A. Krizbai, Mol. Pharmaceutics, 2014, 11, 1949–1963 CrossRef CAS PubMed.
- S. H. Hansen, A. Olsson and J. E. Casanova, J. Biol. Chem., 1995, 270, 28425–28432 CrossRef CAS PubMed.
- R. Beard, D. C. A. Gaboriau, A. D. Gee and E. W. Tate, Chem. Sci., 2019, 10, 10772–10778 RSC.
- S. Fuchs, S. Johansson, A. Tjell, G. Werr, T. Mayr and M. Tenje, ACS Biomater. Sci. Eng., 2021, 7, 2926–2948 CrossRef CAS PubMed.
- M. W. Toepke and D. J. Beebe, Lab Chip, 2006, 6, 1484–1486 RSC.
- J. D. Wang, N. J. Douville, S. Takayama and M. Elsayed, Ann. Biomed. Eng., 2012, 40, 1862–1873 CrossRef PubMed.
- T. E. Winkler and A. Herland, ACS Appl. Mater. Interfaces, 2021, 13, 45161–45174 CrossRef CAS PubMed.
- J. Grant, A. Özkan, C. Oh, G. Mahajan, R. Prantil-Baun and D. E. Ingber, Lab Chip, 2021, 21, 3509–3519 RSC.
- S. P. Deosarkar, B. Prabhakarpandian, B. Wang, J. B. Sheffield, B. Krynska and M. F. Kiani, PLoS One, 2015, 10, 1–21 CrossRef PubMed.
- E. W. K. Young, M. W. L. Watson, S. Srigunapalan, A. R. Wheeler and C. A. Simmons, Anal. Chem., 2010, 82, 808–816 CrossRef CAS PubMed.
- S. Bergmann, S. E. Lawler, Y. Qu, C. M. Fadzen, J. M. Wolfe, M. S. Regan, B. L. Pentelute, N. Y. R. Agar and C. F. Cho, Nat. Protoc., 2018, 13, 2827–2843 CrossRef CAS PubMed.
- G. Nzou, R. T. Wicks, E. E. Wicks, S. A. Seale, C. H. Sane, A. Chen, S. V. Murphy, J. D. Jackson and A. J. Atala, Sci. Rep., 2018, 8, 1–10 CAS.
- D. R. Hill, S. Huang, Y. H. Tsai, J. R. Spence and V. B. Young, J. Visualized Exp., 2017, 2017, 1–10 Search PubMed.
- J. Yeste, M. García-Ramírez, X. Illa, A. Guimerà, C. Hernández, R. Simó and R. Villa, Lab Chip, 2017, 18, 95–105 RSC.
- H. Xu, Z. Li, Y. Yu, S. Sizdahkhani, W. S. Ho, F. Yin, L. Wang, G. Zhu, M. Zhang, L. Jiang, Z. Zhuang and J. Qin, Sci. Rep., 2016, 6, 36670 CrossRef CAS PubMed.
- J. F. Richter, M. Hildner, R. Schmauder, J. R. Turner, M. Schumann and J. Reiche, Tissue Barriers, 2019, 7, 1–14 CrossRef PubMed.
- O. Dubrovskyi, A. A. Birukova and K. G. Birukov, Lab. Invest., 2013, 93, 254–263 CrossRef CAS PubMed.
- K. M. Gray, J. W. Jung, C. T. Inglut, H. C. Huang and K. M. Stroka, Fluids Barriers CNS, 2020, 17, 1–20 CrossRef PubMed.
- F. Gao, H. Sun, X. Li and P. He, Am. J. Physiol., 2022, 322, H71–H86 CAS.
- J. F. Wong and C. A. Simmons, Lab Chip, 2019, 19, 1060–1070 RSC.
- K. Ino, H. J. Pai, K. Hiramoto, Y. Utagawa, Y. Nashimoto and H. Shiku, ACS Omega, 2021, 6, 35476–35483 CrossRef CAS PubMed.
- M. A. Erickson, M. L. Wilson and W. A. Banks, Fluids Barriers CNS, 2020, 17, 1–16 CrossRef PubMed.
- E. H. Neal, N. A. Marinelli, Y. Shi, P. M. McClatchey, K. M. Balotin, D. R. Gullett, K. A. Hagerla, A. B. Bowman, K. C. Ess, J. P. Wikswo and E. S. Lippmann, Stem Cell Rep., 2019, 12, 1380–1388 CrossRef CAS PubMed.
- J. P. Vigh, A. Kincses, B. Ozgür, F. R. Walter, A. R. Santa-Maria, S. Valkai, M. Vastag, W. Neuhaus, B. Brodin, A. Dér and M. A. Deli, Micromachines, 2021, 12, 1–18 CrossRef PubMed.
-
B. Srinivasan and A. R. Kolli, in Blood-Brain Barrier. Neuromethods, Humana Press, New York, NY, 2019, vol. 142, pp. 99–114 Search PubMed.
- N. J. Darling, C. L. Mobbs, A. L. González-Hau, M. Freer and S. Przyborski, Front. Bioeng. Biotechnol., 2020, 8, 1–15 Search PubMed.
- S. Lopez-Escalera and A. Wellejus, Biochem. Biophys. Rep., 2022, 31, 101314 CAS.
- J. Yeste, X. Illa, C. Gutiérrez, M. Solé, A. Guimerà and R. Villa, J. Phys. D: Appl. Phys., 2016, 49, 375401 CrossRef.
- B. Jovov, N. K. Wills and S. A. Lewis, Am. J. Physiol., 1991, 261, C1196–C1203 CrossRef CAS PubMed.
- M. Odijk, A. D. Van Der Meer, D. Levner, H. J. Kim, M. W. Van Der Helm, L. I. Segerink, J. P. Frimat, G. A. Hamilton, D. E. Ingber and A. Van Den Berg, Lab Chip, 2015, 15, 745–752 RSC.
- WPI, EndOhm Instruction Manual, https://www.wpiinc.com/media/wysiwyg/pdf/EndOhm_IMs.pdf, (accessed 6 March 2021).
- TEER24 – Barrier Function Measurements – Applied Biophysics, https://www.biophysics.com/teer24.php, (accessed 6 March 2021).
- TER measurements automated – cellZscope – nanoAnalytics EN, https://www.nanoanalytics.com/en/products/cellzscope.html, (accessed 6 March 2021).
- B. M. Maoz, A. Herland, O. Y. F. Henry, W. D. Leineweber, M. Yadid, J. Doyle, R. Mannix, V. J. Kujala, E. A. Fitzgerald, K. K. Parker and D. E. Ingber, Lab Chip, 2017, 17, 2294–2302 RSC.
- J. D. Stucki, N. Hobi, A. Galimov, A. O. Stucki, N. Schneider-Daum, C. M. Lehr, H. Huwer, M. Frick, M. Funke-Chambour, T. Geiser and O. T. Guenat, Sci. Rep., 2018, 8, 1–13 CAS.
- N. Ferrell, R. R. Desai, A. J. Fleischman, S. Roy, H. D. Humes and W. H. Fissell, Biotechnol. Bioeng., 2010, 107, 707–716 CrossRef CAS PubMed.
- N. J. Douville, Y.-C. C. Tung, R. Li, J. D. Wang, M. E. H. El-Sayed and S. S. Takayama, Anal. Chem., 2010, 82, 2505–2511 CrossRef CAS PubMed.
- R. Booth, S. Noh and H. Kim, Lab Chip, 2014, 14, 1880–1890 RSC.
- F. R. Walter, S. Valkai, A. Kincses, A. Petneházi, T. Czeller, S. Veszelka, P. Ormos, M. A. Deli and A. Dér, Sens. Actuators, B, 2016, 222, 1209–1219 CrossRef CAS.
- O. Y. F. Henry, R. Villenave, M. J. Cronce, W. D. Leineweber, M. A. Benz and D. E. Ingber, Lab Chip, 2017, 17, 2264–2271 RSC.
- V. Errico, A. De Ninno, F. R. Bertani, L. Businaro, P. Bisegna and F. Caselli, Sens. Actuators, B, 2017, 247, 580–586 CrossRef CAS.
-
J. R. Macdonald and W. B. Johnson, in Impedance Spectroscopy: Theory, Experiment, and Applications, 2nd edn, 2005, pp. 1–7 Search PubMed.
-
S. C. Pillai and Y. Lang, Toxicity of Nanomaterials, 2019 Search PubMed.
-
H. Semat and R. Katz, in Physics, 1958, pp. 629–647 Search PubMed.
- Y. Xu, X. Xie, Y. Duan, L. Wang, Z. Cheng and J. Cheng, Biosens. Bioelectron., 2016, 77, 824–836 CrossRef CAS PubMed.
- K. Benson, S. Cramer and H. J. Galla, Fluids Barriers CNS, 2013, 10, 1–11 CrossRef PubMed.
- F. A. Alexander, M. Celestin, D. T. Price, M. Nanjundan and S. Bhansali, Analyst, 2013, 138, 3728–3734 RSC.
- F. Urban, K. Hajek, T. Naber, B. Anczykowski, M. Schäfer and J. Wegener, Sci. Rep., 2020, 10, 1–12 CrossRef PubMed.
- J. Yeste, L. Martínez-Gimeno, X. Illa, P. Laborda, A. Guimerà, J. P. Sánchez-Marín, R. Villa and I. Giménez, Biotechnol. Bioeng., 2018, 115, 1604–1613 CrossRef CAS PubMed.
- I. Giaever and C. R. Keese, Cell Biol., 1991, 88, 78–7900 Search PubMed.
- J. Wegener, C. R. Keese and I. Giaever, Exp. Cell Res., 2000, 259, 158–166 CrossRef CAS PubMed.
- D. M. Lewis, N. Mavrogiannis, Z. Gagnon and S. Gerecht, Biomicrofluidics, 2018, 12, 1–10 CrossRef PubMed.
- R. Szulcek, H. J. Bogaard and G. P. van Nieuw Amerongen, J. Visualized Exp., 2014, 1–12 Search PubMed.
-
Stanford Research Systems, About Lock-In Amplifiers - Application Note #3, 2005, pp. 1–9 Search PubMed.
- W. Gamal, H. Wu, I. Underwood, J. Jia, S. Smith and P. O. Bagnaninchi, Philos. Trans. R. Soc., B, 2018, 373, 20170226 CrossRef PubMed.
- I. Bischoff, M. C. Hornburger, B. A. Mayer, A. Beyerle, J. Wegener and R. Fürst, Sci. Rep., 2016, 6, 1–11 CrossRef PubMed.
- P. Ramiah Rajasekaran, A. A. Chapin, D. N. Quan, J. Herberholz, W. E. Bentley and R. Ghodssi, Microsyst. Nanoeng., 2020, 6, 100 CrossRef CAS PubMed.
- S. Eltanani, T. Yumnamcha, A. Gregory, M. Elshal, M. Shawky and A. S. Ibrahim, Cells, 2022, 11, 4128 CrossRef CAS PubMed.
- R. Lee, P. H. Kim, J. W. Choi, K. Oh-Joon, K. Kim, D. Kim, C. O. Yun and K. H. Yoo, Biosens. Bioelectron., 2010, 25, 1325–1332 CrossRef CAS PubMed.
- J. A. Stolwijk and J. Wegener, Sci. Rep., 2020, 10, 1–14 CrossRef PubMed.
- T. A. Nguyen, T. I. Yin, D. Reyes and G. A. Urban, Anal. Chem., 2013, 85, 11068–11076 CrossRef CAS PubMed.
- T. B. Tran, S. B. Cho and J. Min, Biosens. Bioelectron., 2013, 50, 453–459 CrossRef CAS PubMed.
- J. Seebach, P. Dieterich, F. Luo, H. Schillers, D. Vestweber, H. Oberleithner, H. Galla and H. Schnittler, Lab. Invest., 2000, 80, 1819–1831 CrossRef CAS PubMed.
- N. DePaola, J. E. Phelps, L. Florez, C. R. Keese, F. L. Minnear, I. Giaever and P. Vincent, Ann. Biomed. Eng., 2001, 29, 648–656 CrossRef CAS PubMed.
- ECIS Cultureware – Applied Biophysics, https://www.biophysics.com/cultureware.php, (accessed 6 September 2023).
- V. Velasco, P. Soucy, R. Keynton and S. J. Williams, Lab Chip, 2022, 22, 4705–4716 RSC.
- O. Chebotarev, A. Ugodnikov and C. A. Simmons, ACS Appl. Bio Mater., 2024, 7, 2000–2011 CrossRef CAS PubMed.
-
A. Ugodnikov, O. Chebotarev, H. Persson and C. A. Simmons, bioRxiv, 2023, preprint, DOI:10.1101/2023.07.11.548631.
-
A. Ugodnikov, J. Lu, O. Chebotarev and C. A. Simmons, bioRxiv, 2023, preprint, DOI:10.1101/2023.11.25.568615.
- R. Novak, M. Ingram, S. Marquez, D. Das, A. Delahanty, A. Herland, B. M. Maoz, S. S. F. Jeanty, M. R. Somayaji, M. Burt, E. Calamari, A. Chalkiadaki, A. Cho, Y. Choe, D. B. Chou, M. Cronce, S. Dauth, T. Divic, J. Fernandez-Alcon, T. Ferrante, J. Ferrier, E. A. FitzGerald, R. Fleming, S. Jalili-Firoozinezhad, T. Grevesse, J. A. Goss, T. Hamkins-Indik, O. Henry, C. Hinojosa, T. Huffstater, K. J. Jang, V. Kujala, L. Leng, R. Mannix, Y. Milton, J. Nawroth, B. A. Nestor, C. F. Ng, B. O'Connor, T. E. Park, H. Sanchez, J. Sliz, A. Sontheimer-Phelps, B. Swenor, G. Thompson, G. J. Touloumes, Z. Tranchemontagne, N. Wen, M. Yadid, A. Bahinski, G. A. Hamilton, D. Levner, O. Levy, A. Przekwas, R. Prantil-Baun, K. K. Parker and D. E. Ingber, Nat. Biomed. Eng., 2020, 4, 407–420 CrossRef PubMed.
- Y. Xia and G. M. Whitesides, Angew. Chem., Int. Ed., 1998, 37, 550–575 CrossRef CAS PubMed.
- V. Faustino, S. O. Catarino, R. Lima and G. Minas, J. Biomech., 2016, 49, 2280–2292 CrossRef PubMed.
- D. R. Reyes, H. Van Heeren, S. Guha, L. Herbertson, A. P. Tzannis, J. Ducree, H. Bissig and H. Becker, Lab Chip, 2021, 21, 9–21 RSC.
- Q. Ramadan and M. Zourob, Biomicrofluidics, 2020, 14, 041501 CrossRef CAS PubMed.
- F. Sassa, G. C. Biswas and H. Suzuki, Lab Chip, 2020, 20, 1358–1389 RSC.
- J. F. Wong, E. W. K. Young and C. A. Simmons, AIP Adv., 2017, 7, 115116 CrossRef.
- S. B. Campbell, Q. Wu, J. Yazbeck, C. Liu, S. Okhovatian and M. Radisic, ACS Biomater. Sci. Eng., 2021, 7, 2880–2899 CrossRef CAS PubMed.
- Y. Sui, Y. Dai, C. C. Liu, R. M. Sankaran and C. A. Zorman, Adv. Mater. Technol., 2019, 4, 1–10 Search PubMed.
- B. L. Thompson, C. Birch, D. A. Nelson, J. Li, J. A. DuVall, D. Le Roux, A. C. Tsuei, D. L. Mills, B. E. Root and J. P. Landers, Lab Chip, 2016, 16, 4569–4580 RSC.
- EndOhm Chamber, https://www.wpi-europe.com/products/cell-and-tissue/teer-measurement/endohm-24snap.aspx, (accessed 3 September 2019).
- N. Meghani, K. H. Kim, S. H. Kim, S. H. Lee and K. H. Choi, Arch. Pharmacal Res., 2020, 43, 503–513 CrossRef CAS PubMed.
- J. Yeste, X. Illa, A. Guimerà and R. Villa, Bio-MEMS Med. Microdevices II, 2015, 9518, 95180N Search PubMed.
- E. Sarró, M. Lecina, A. Fontova, C. Solà, F. Gòdia, J. J. Cairó and R. Bragós, Biosens. Bioelectron., 2012, 31, 257–263 CrossRef PubMed.
- M. W. van der Helm, M. Odijk, J. P. Frimat, A. D. van der Meer, J. C. T. Eijkel, A. van den Berg and L. I. Segerink, Biosens. Bioelectron., 2016, 85, 924–929 CrossRef CAS PubMed.
- Y. B. Arık, M. W. Van Der Helm, M. Odijk, L. I. Segerink, R. Passier, A. Van Den Berg and A. D. Van Der Meer, Biomicrofluidics, 2018, 12, 1–13 CrossRef PubMed.
- T. Gerasimenko, S. Nikulin, G. Zakharova, A. Poloznikov, V. Petrov, A. Baranova and A. Tonevitsky, Front. Bioeng. Biotechnol., 2020, 7, 474 CrossRef PubMed.
- Y. H. Hung, W. C. Chiu, S. R. Fuh, Y.
T. Lai, T. H. Tung, C. C. Huang and C. M. Lo, Biosensors, 2022, 12, 293 CrossRef CAS PubMed.
- J. J. W. Hucklesby, A. Anchan, S. J. O'carroll, C. P. Unsworth, E. S. Graham and C. E. Angel, Biosensors, 2021, 11, 1–13 CrossRef PubMed.
- T. Schmiedinger, S. Partel, T. Lechleitner, O. Eiter, D. Hekl, S. Kaseman, P. Lukas, J. Edlinger, J. Lechner and T. Seppi, Biomed. Microdevices, 2020, 22, 30 CrossRef CAS PubMed.
- H. Y. Tseng, J. H. Lizama, N. A. S. Alvarado and H. H. Hou, Biomicrofluidics, 2022, 16, 031302 CrossRef CAS PubMed.
- M. Zamani, C. M. Klapperich and A. L. Furst, Lab Chip, 2023, 1410–1419 RSC.
- D. H. Elbrecht, C. J. Long and J. J. Hickman, J. Rare Dis. Res. Treat., 2016, 1, 46–52 Search PubMed.
- S. E. De Leon, L. Cleuren, Z. Y. Oo, P. R. Stoddart and S. L. McArthur, Bioengineering, 2021, 8, 1–15 CrossRef PubMed.
- S. E. De León, A. Pupovac and S. L. McArthur, Biotechnol. Bioeng., 2020, 117, 1230–1240 CrossRef PubMed.
- T. Ching, Y. Li, R. Karyappa, A. Ohno, Y. C. Toh and M. Hashimoto, Sens. Actuators, B, 2019, 297, 126609 CrossRef CAS.
- A. Wu, L. Wang, E. Jensen, R. Mathies and B. Boser, Lab Chip, 2010, 10, 519–521 RSC.
- D. Moschou and A. Tserepi, Lab Chip, 2017, 17, 1388–1405 RSC.
- C. Zhou, S. Bette, A. Babendreyer, C. Hoffmann, S. Gerlach, T. Kremers, A. Ludwig, B. Hoffmann, R. Merkel, S. Uhlig and U. Schnakenberg, Sens. Actuators, B, 2021, 336, 129656 CrossRef CAS.
- Z. J. Krysiak, H. Abdolmaleki, S. Agarwala and U. Stachewicz, Polymers, 2022, 14, 5043 CrossRef CAS PubMed.
- C. M. Tringides, N. Vachicouras, I. de Lázaro, H. Wang, A. Trouillet, B. R. Seo, A. Elosegui-Artola, F. Fallegger, Y. Shin, C. Casiraghi, K. Kostarelos, S. P. Lacour and D. J. Mooney, Nat. Nanotechnol., 2021, 16, 1019–1029 CrossRef CAS PubMed.
- N. Yi, H. Cui, L. G. Zhang and H. Cheng, Acta Biomater., 2019, 95, 91–111 CrossRef CAS PubMed.
- D. McIntyre, A. Lashkaripour and D. Densmore, Lab Chip, 2020, 20, 3690–3695 RSC.
- H. L. Ma, A. C. Urbaczek, F. Zeferino Ribeiro de Souza, P. Augusto Gomes Garrido Carneiro Leão, J. Rodrigues Perussi and E. Carrilho, Micromachines, 2021, 12, 1–13 Search PubMed.
- S. R. A. Kratz, C. Eilenberger, P. Schuller, B. Bachmann, S. Spitz, P. Ertl and M. Rothbauer, Sci. Rep., 2019, 9, 1–12 CrossRef CAS PubMed.
- J. F. Hernández-Rodríguez, M. Á. López, D. Rojas and A. Escarpa, Lab Chip, 2022, 22, 4805–4821 RSC.
- M. Piergiovanni, S. B. Leite, R. Corvi and M. Whelan, Lab Chip, 2021, 21, 2857–2868 RSC.
- S. Varma and J. Voldman, Lab Chip, 2018, 18, 3333–3352 RSC.
- P. Hargrove-Grimes, L. A. Low and D. A. Tagle, Cells Tissues Organs, 2022, 211, 269–281 CrossRef CAS PubMed.
- G. R. Ainslie, M. Davis, L. Ewart, L. A. Lieberman, D. J. Rowlands, A. J. Thorley, G. Yoder and A. M. Ryan, Lab Chip, 2019, 19, 3152–3161 RSC.
- R. N. Hardwick, C. J. Betts, J. Whritenour, R. Sura, M. Thamsen, E. H. Kaufman and K. Fabre, Lab Chip, 2020, 20, 199–214 RSC.
- M. F. Peters, A. L. Choy, C. Pin, D. J. Leishman, A. Moisan, L. Ewart, P. J. Guzzie-Peck, R. Sura, D. A. Keller, C. W. Scott and K. L. Kolaja, Lab Chip, 2020, 20, 1177–1190 RSC.
- J. A. Phillips, T. S. P. Grandhi, M. Davis, J. C. Gautier, N. Hariparsad, D. Keller, R. Sura and T. R. Van Vleet, Lab Chip, 2020, 20, 468–476 RSC.
|
This journal is © The Royal Society of Chemistry 2024 |