DOI:
10.1039/D3LC00829K
(Tutorial Review)
Lab Chip, 2024,
24, 1494-1528
Heart-on-a-chip systems: disease modeling and drug screening applications
Received
1st October 2023
, Accepted 8th January 2024
First published on 6th February 2024
Abstract
Cardiovascular disease (CVD) is the leading cause of death worldwide, casting a substantial economic footprint and burdening the global healthcare system. Historically, pre-clinical CVD modeling and therapeutic screening have been performed using animal models. Unfortunately, animal models oftentimes fail to adequately mimic human physiology, leading to a poor translation of therapeutics from pre-clinical trials to consumers. Even those that make it to market can be removed due to unforeseen side effects. As such, there exists a clinical, technological, and economical need for systems that faithfully capture human (patho)physiology for modeling CVD, assessing cardiotoxicity, and evaluating drug efficacy. Heart-on-a-chip (HoC) systems are a part of the broader organ-on-a-chip paradigm that leverages microfluidics, tissue engineering, microfabrication, electronics, and gene editing to create human-relevant models for studying disease, drug-induced side effects, and therapeutic efficacy. These compact systems can be capable of real-time measurements and on-demand characterization of tissue behavior and could revolutionize the drug development process. In this review, we highlight the key components that comprise a HoC system followed by a review of contemporary reports of their use in disease modeling, drug toxicity and efficacy assessment, and as part of multi-organ-on-a-chip platforms. We also discuss future perspectives and challenges facing the field, including a discussion on the role that standardization is expected to play in accelerating the widespread adoption of these platforms.
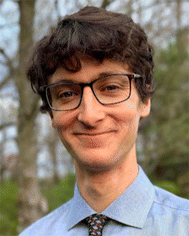 Derrick Butler | Derrick Butler is currently a National Research Council/NIST Postdoctoral Fellow at the National Institute of Standards and Technology. He received a B.S. in Physics from the University of Vermont, USA in 2016 followed by a M.S.E. in Materials Science and Engineering from the University of Pennsylvania, USA in 2017. In 2022, he completed his Ph.D. in Electrical Engineering from Penn State University, USA where his research focused on developing electrochemical and electronic point-of-care biosensors for neurochemicals and bacteria based on two-dimensional materials. His current research is focused on the development of novel bioelectronics for integration in heart-on-a-chip systems. |
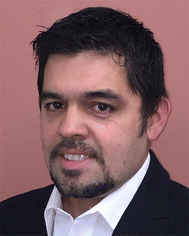 Darwin Reyes | Dr. Darwin Reyes is a project leader at the National Institute of Standards and Technology. He received a B.S. and Ph.D. in chemistry from the University of Puerto Rico (USA), and an M.S. in Applied Biomedical Engineering from The Johns Hopkins University (USA). He worked with Prof. Andreas Manz at Imperial College (London, UK) as an NSF Postdoctoral Fellow. Dr. Reyes is currently working on the development of microfluidic systems with electronic manipulation and measurement elements for microphysiological systems as well as developing acoustofluidic sensors and protocols for localized flow and for the assessment of fluid properties within micro systems. |
1 Introduction
Cardiovascular disease (CVD) is the leading cause of death worldwide, with an estimated 17.9 million attributable deaths each year,1 and accounted for 12% of total US health expenditures from 2017 to 2018 ($378 billion US Dollars (USD)).2 As such, modeling, preventing, and treating the various subsets of CVD is a highly active area of research.3 While therapeutic intervention has improved patient outcome and quality of life, numerous challenges remain unaddressed. For instance, the hospitalization rate in the US for acute myocardial infarction (AMI), which is caused by an unstable ischemic syndrome,4 has declined significantly over the last decade and beyond.2 Despite these improvements, post-ischemia reperfusive injury leaves patients especially susceptible to future heart dysfunction/failure,5 and, despite promising pre-clinical studies, very few, if any, clinical treatments are available to improve disease prognosis.6
The most likely culprit for the poor translation of therapies from pre-clinical studies to widespread clinical usage is the disconnect between the animal models used in pre-clinical studies and human physiology. For example, in the context of the heart, murine models have provided valuable mechanistic insight into heart aging and injury response.7 However, the typical heart rate for a mouse is 400 to 650 beats per minute (BPM),7 nearly 7 to 10 times that of a human. Furthermore, the predominant isoform of myosin heavy chain (MHC) is α-MHC (encoded by MYH6) in fetal humans and β-MHC (encoded by MYH7) in adults, although this is reversed in mice.8 While these are just a selection of physiological differences between animals and humans, they raise the question regarding the relevance of animal models in pre-clinical assessments. In fact, of the novel drugs that do make it passed pre-clinical animal testing, approximately 89% fail in human clinical trials, oftentimes due to unforeseen toxicity.9–11 Even after making it to market, many drugs are removed or recalled due to adverse side effects, with cardiovascular toxicity being the second most common cause behind liver toxicity.12 Not only do these unanticipated side effects have a tremendous impact on human health and well-being, they come at significant cost to pharmaceutical companies as well. Drug development already takes over 10 years from initial synthesis to completion of clinical trials and costs over $1 billion USD for a single drug. Recalls can add to this, with a high-profile example being the $8.5 billion USD spent by a pharmaceutical company for legal settlements after the removal of one of their products from the market.10
Given the extensive resource requirement for drug development and limited translatability of current animal models, clinicians, pharmaceutical companies, and policymakers are actively searching for a human-relevant pre-clinical alternative to animal models. To address the setbacks of animal models, in vitro microphysiological systems, often referred to as “organ-on-a-chip (OoC)”, that have the potential to replicate human physiology and pharmacology have emerged as a potentially viable alternative.13 An organ-on-a-chip is, by definition, “a subset of microphysiological systems that replicates one or more aspects of an organ's in vivo dynamics, functionality, structure, and/or (patho)physiological response(s) of multiple cell types integrated within a non-biological platform”.14 The synergistic convergence of microfabrication, microfluidics, and tissue engineering has accelerated the development of OoCs in recent times. Microfluidic chips allow for the transport and manipulation of small volumes of liquid, benefiting tremendously from microfabrication processes, rigorously developed in the semiconductor industry. Microfabrication enables the precise spatial arrangement of cell culture chambers, fluidic channels, and analytical components, such as electrodes or cantilevers for real-time monitoring of tissue behavior. To augment these benefits, improvements in tissue engineering have enabled the generation of more complex microtissues comprised of multiple cell types. Furthermore, the advent of human-induced pluripotent stem cells (hiPSCs) has opened up the possibility of tailoring these platforms to individual patients with unique genetic profiles. hiPSCs are especially crucial for the development of heart-on-a-chip (HoC) due to the difficulty of acquiring human heart samples and the limited ability of cardiomyocytes to divide. Overall, these systems have demonstrated the ability to model key aspects of cardiopathologies,15,16 tissue regeneration,17 and pharmaceutical response,18 oftentimes agreeing well with clinical results.
In this review, we discuss recent reports and advances in HoC systems, engineered cardiac tissue, and cardiac microphysiological systems. We first present a brief overview of cardiac physiology followed by a more in-depth discussion of the major components that make up an organ-on-a-chip. We then focus on two key applications that could benefit from the use of HoCs in addition to or in place of animal testing, namely disease modeling and drug discovery-which includes drug efficacy testing and cardiotoxicity assessment. Finally, a discussion of standardization within the OoC community follows and transitions into the conclusion and future outlook.
2 The heart: a biological pump
The heart is the pump and main organ of the cardiovascular system (Fig. 1). Circulating blood provides tissues and organs with nutrients and oxygen.44 The heart is comprised of four chambers which act as reservoirs for blood: the left and right atria in the upper portion of the heart, and the left and right ventricles in the lower portion, separated by the atrial or ventricular septum, respectively.45 Oxygen-deficient blood from tissue and other organs enters the right atrium through the vena cava.45 The oxygen-deficient blood is pumped through the pulmonary artery to the lungs, where it releases waste products (e.g. carbon dioxide) and gets re-oxygenated. The oxygenated blood flows from the lungs back to the heart, where it is circulated to the rest of the body.45
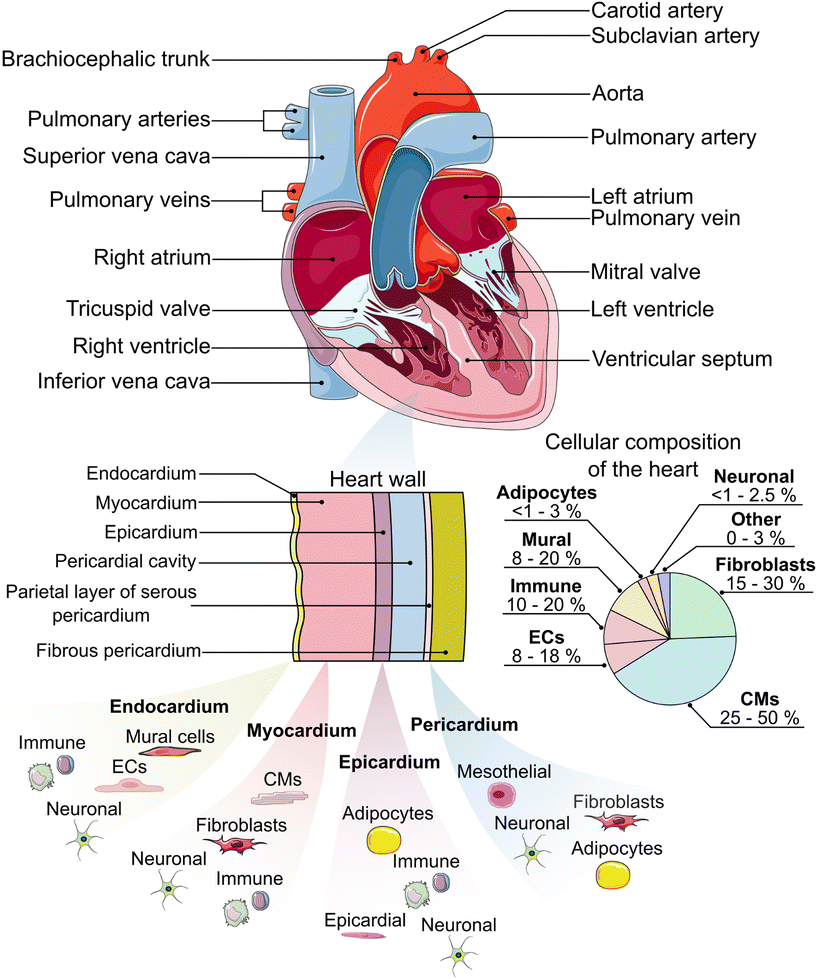 |
| Fig. 1 An anatomical schematic of the heart and heart wall with the main constituent cell types in each layer.19–37 Cellular composition ranges are estimated from the literature.38–40 ECs = endothelial cells, CMs = cardiomyocytes. Some components of this figure are used under a Creative Commons license.41–43 | |
To facilitate the circulation of blood throughout the body, the heart wall is comprised of three specialized layers: the endocardium (innermost layer), the myocardium (middle layer), and the epicardium (outermost layer). The endocardium is primarily comprised of endothelial cells, which line the inner walls of the heart's valves and chambers.46 The endocardium is a thin layer and serves as the barrier between the blood and the remainder of the heart wall. The myocardium is primarily made up of cardiac fibroblasts, which support the three-dimensional structure of the heart, and cardiomyocytes, which are responsible for the mechanical contraction of the heart. Cardiomyocytes make up the largest percentage of the heart by volume (≈75%), while they are similar to fibroblasts in percentage by number (≈30–35%),39 although other reports have put CM percentages near 50%.38 It is worth noting that the distribution of cell types, especially cardiomyocytes and fibroblasts, varies between heart chambers and between males and females.38–40 The epicardium, which includes a layer of mesothelial cells,47 plays an important role in paracrine signaling and contributing cardiac progenitor cells during cardiogenesis and post-injury repair.48–50 Lastly, the heart is enclosed within the pericardium,19,46 which provides lubrication, support for the heart, and protection from blunt force injury and infection.51
In addition to the major cell types indicated previously, the heart also contains a number of other important cell types that are critical for its function. Specialized cardiomyocytes in the His-Purkinje system, sinoatrial node, and atrioventricular node make up the electrical system of the heart, which is responsible for initiating and transmitting the electrical impulses that enable synchronized contractions.46,52,53 Mural cells, including pericytes and vascular smooth muscle cells, help to provide support for the vasculature.54 Adipocytes are present in the epicardium and pericardium and assist in protecting the underlying myocardium.55 Neuronal cells help to form the cardiac nervous system.56 Finally, a variety of immune cells, including macrophages, monocytes, neutrophils, B cells, natural killer (NK) cells, mast cells, and T cells, can also be found in the heart.38,39 These cells respond to, for example, infection, myocardial infarction, non-ischemic heart failure, and the presence of toxic compounds.57 When designing a HoC model, it is crucial that the appropriate cell type(s) are included to ensure the model captures the native physiology and/or pathology expected in the human body.
The human heart undergoes extensive changes throughout its course of development.58–64 When establishing a HoC model, it is important to consider the maturity of the cells being used. This is especially relevant for cardiomyocytes, which show significant differences in metabolic, structural, and electrical properties between fetal-like and adult cells.65,66 Importantly, the proliferative ability of cardiomyocytes declines as cells mature, which can make generating enough cells for in vitro experiments a challenge. Structurally, fetal-like cardiomyocytes are typically round and small (5 to 10 μm in diameter) and mono-nucleated, whereas adult cardiomyocytes are larger and elongated (100+ μm long) and most are multi-nucleated.65,67–69 The organization and length of sarcomeres also increases as cardiomyocytes mature,70 which contributes to an increase in the contractile force generated per cell.70–72 Fetal cardiomyocytes also show differences in myofibrillar isoform expression compared to their more mature counterparts, including titin (N2BA to N2B),73,74 troponin (slow skeleton troponin I (ssTnI) to cardiac troponin I (cTnI)),75,76 and less α-MHC.77 Moreover, the metabolic pathway switches from glycolysis in fetal cardiomyocytes to oxidation of fatty acids in adult cardiomyocytes,58 which could impact the response to drugs and cardiotoxic compounds.70 From an electrophysiological standpoint, fetal cardiomyocytes demonstrate a lower membrane potential magnitude (−60 mV vs. −90 mV)78 and capacitance,79 along with a slower conduction velocity.70 Altogether, the degree of cell maturation must be considered when translating results and conclusions from heart-on-a-chip models to human beings.
3 Heart-on-a-chip: components and assembly
HoC systems are comprised of four major sub-systems: the microfluidic architecture (Fig. 2a–c), the cell tissue(s) and associated matrices, environmental controls (e.g. O2 gradient, drug delivery, mechanical actuation/stimulation) (Fig. 2d–f), and analytical components (e.g. in-line biochemical sensors, electrodes) (Fig. 2g–i).80–82 The conceptual design and construction of a HoC begins with a clear understanding of the target objective(s) and question(s) to be answered,83 which could preclude certain sub-systems. For instance, a key characteristic of a system intended to model myocardial infarction would be a stable and controllable oxygen gradient, whereas a system designed to quantify contractile forces would perhaps emphasize recapitulating the mechanical properties of the cellular microenvironment. As such, the design and engineering of each component within a HoC system is very much interdependent on the others. In this section, each of the four components are discussed in more detail with the goal of providing the reader with a basic understanding of the materials, fabrication techniques, and auxiliary features that are needed when constructing a HoC system.
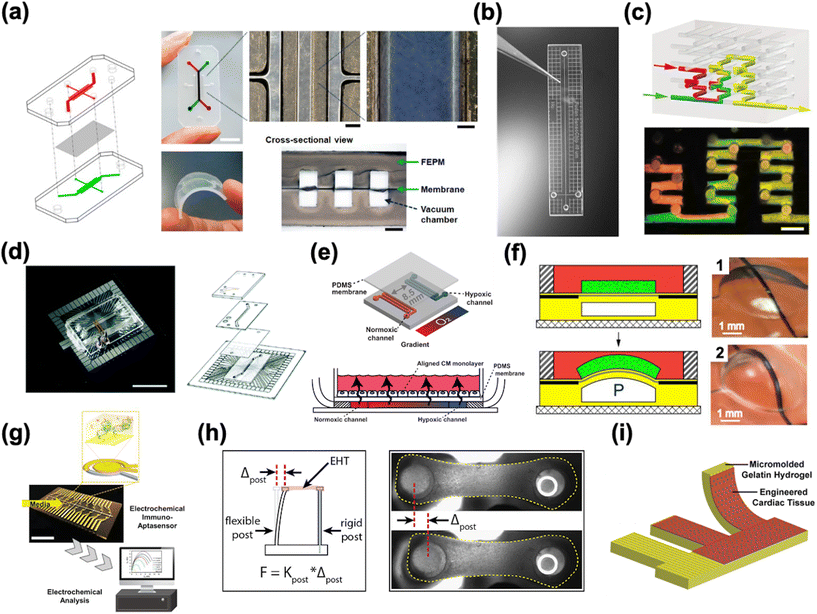 |
| Fig. 2 Exemplary microfluidic chips fabricated using a) a tetrafluoroethylene-propylene elastomer to prevent small molecule absorption (reproduced from ref. 90 under Creative Commons License CC BY 4.0), b) poly(methylmetachrylate) (PMMA) (reproduced from ref. 101 with permission from John Wiley and Sons), and c) a 3D printer (reproduced from ref. 110 with permission from Springer Nature). d) A microfluidic chip combined with a microelectrode array (MEA) (reproduced from ref. 160 with permission from the Royal Society of Chemistry). e) A microfluidic chip with two adjacent channels (one normoxic and one hypoxic) beneath a polydimethylsiloxane (PDMS) membrane for establishment of an oxygen gradient to mimic the infarct border zone (reprinted from ref. 146 under Creative Commons NonCommercial License 4.0 (CC BY-NC)). f) A pressure-actuated membrane with an integrated carbon nanotube (CNT)-based strain sensor for on-chip mechanical conditioning of cardiac cells (1 = resting, 2 = actuated) (reproduced from ref. 161 with permission from Elsevier). g) An electrochemical immuno-aptasensor array for biomarker quantification (reproduced from ref. 162 with permission from John Wiley and Sons). h) A two-pillar construct for monitoring the mechanical behavior of cardiac tissue based on the deflection of the flexible post (reproduced from ref. 163 with permission from Elsevier). i) An example of a muscular thin film array (MTF), which quantifies mechanical behavior of cardiac tissue by measuring the curvature of the flexible cantilever as the tissue contracts and relaxes (reproduced from ref. 164 with permission from Elsevier). | |
3.1 Microfluidics
The field of microfluidics deals with the manipulation of small volumes of fluid in microscale (i.e. 1–1000 μm in height or width) channels.84,85 Microfluidics is applicable across a range of fields, from chemical synthesis to bioanalysis. Microfluidics offers a number of key advantages that make it appealing for organ-on-a-chip systems in particular. Microfluidic systems are inherently dynamic whereas traditional 2D cell culture is largely a static process. The small fluid volume (on the order of nL to μL) minimizes the use of expensive reagents and human samples, and fewer cells are needed for experiments.86 The compatibility with many microfabrication methods also allows for the creation of complex chip architectures that can house one or more organ chambers and are capable of cell sorting, fluid mixing, and downstream analysis, all in a device with a small footprint.87 Furthermore, microfluidic chips are also capable of mimicking the cellular microenvironment, including physiological shear stress, periodic mechanical strain, and chemical gradients.
While the use of microfluidics in organ-on-a-chip systems has come a long way, one key challenge hindering its widespread adoption is the choice of material(s).87 Common materials utilized in the microfluidic structure of HoC systems include polydimethylsiloxane (PDMS), poly(methyl methacrylate) (PMMA), polyurethane (PU), polystyrene (PS), and other elastomers.88–91 Pattern transfer to these materials can be done using a variety of techniques,92 including soft lithography,93 photolithography,94 hot embossing,95,96 and 3D printing,97,98 to name a selection. PDMS is widely used for prototyping and lab-scale experiments due to the ease with which it can be cast and molded. Furthermore, its optical transparency and gas permeability make it well-suited for prolonged cell culture and monitoring.88 PDMS chips can also be integrated with auxiliary components, such as porous membranes for cell migration studies for example, via a plethora of bonding techniques. Nonetheless, PDMS is relatively expensive and readily absorbs many small molecules, making applications such as drug discovery particularly challenging.13 As a result, other materials are being explored as alternatives to PDMS. PMMA, PS, and polycarbonate (PC) are all biocompatible, inexpensive, ubiquitous materials used in cell culture system and microfluidics.99–103 The challenge with these materials is they are relatively difficult to microfabricate in an academic lab, autofluoresce, and can be difficult to interface with peripheral equipment, such as pumps.104–106 PU is a less-explored material that addresses many of the drawbacks associated with PDMS, all while maintaining the advantageous properties, including optical transparency for microscopy.107,108
It is worth briefly highlighting recent efforts to 3D print microfluidic components, which is widely seen as a new paradigm in manufacturing organ-on-a-chip systems.109 3D printing is an additive process that involves the layer-by-layer deposition of a material in a precisely programmed fashion. 3D printing is commonly used to quickly prototype and fabricate molds for casting PDMS, which are otherwise prepared using traditional microfabrication processes, such as photolithography. However, due to the limited scalability of PDMS, researchers are exploring the direct printing of microfluidic components rather than printing of soft lithography templates. Direct 3D printing of microfluidic components enables truly three-dimensional, multi-planar fluidic constructs (e.g. a helix),110 rather than two-dimensional patterns that are projected into the third dimension. Furthermore, 3D printing is largely an automated process that involves significantly less manual labor when assembling organ-on-a-chip devices. Despite the advantages 3D printing offers, critical technological challenges remain, including the resolution limit of the printer, the cytocompatibility of the resin materials, low throughput, low gas permeability, and ensuring water-tight sealing of the channels. Nonetheless, 3D printing offers tremendous prospect for bringing organ-on-a-chip products to market.84,111
3.2 Cell sources and matrices
Before the HoC system can be engineered and constructed, careful consideration of the type(s) of cell(s) to be incorporated is necessary. Animal-derived cardiac cells, typically from the hearts of rats or pigs, are one potential source of cells. The handling, isolation, and cultivation of animal-derived cells is well-established and reproducible. While animal models and animal-derived cells have provided insight into heart development, they can be less effective at modeling cardiac diseases and adverse drug effects due to differences between human and animal physiology and pharmacodynamics.112,113 The advent of human embryonic stem cells (hESCs) and, more recently, human-induced pluripotent stem cells (hiPSCs) has enabled in vitro heart models with cells of human origin.112,114 hiPSCs, in particular, alleviate many of the ethical and legal issues surrounding the use of hESCs,114 and therefore, it is worth emphasizing the advantages hiPSCs offer. The isolation of human cardiac samples is difficult, if not impossible, and the collected cardiomyocytes do not easily divide, making culture maintenance and expansion challenging. On the contrary, hiPSCs can be derived from somatic cells obtained through trivial means, such as a skin or blood sample. hiPSCs readily proliferate, making them amenable to conventional cell culture practices. Additionally, hiPSCs possess the genetic profile of their source, opening up the potential for patient-specific drug screening and personalized disease modeling.
hiPSCs are oftentimes differentiated into cardiomyocytes (hiPSC-CM), which is now a well-established procedure, given the large volume of the heart they comprise (≈75% by volume, ≈30% by number).115,116 The use of hiPSC-CMs has enabled the evaluation of various cardiotoxic compounds and pathological environments on contraction dynamics in vitro. To create a more holistic in vitro model with multiple cell types, methods for differentiating hiPSCs into other cell types, such as endothelial cells (EC) and cardiac fibroblasts (CF), which are responsible for producing the extracellular matrix (ECM) in vivo, are being developed.117–119 Strategically incorporating different cell types to more accurately represent the heart in vivo could enable more comprehensive studies of cell–cell communication within the heart. However, the controllable placement and selective differentiation of multiple cell types for in vitro modeling has proven to be challenging.120,121 Perhaps the most pressing challenge facing the widespread adoption of hiPSC-based in vitro models is the immaturity of hiPSC-derived cardiac cells.8,59,122,123 It is known that mature CMs, for instance, show distinctly different mechanical, morphological, and electrophysiological characteristics compared to immature CMs. Such differences can directly impact how the cells respond to certain stimuli and stressors, leading to an incomplete understanding of the model.8 Guided by the mechanisms of cardiac development in vivo, methods for cardiac maturation in HoC systems are being explored. Perhaps the most simple method for maturation is to culture the CMs for a long period of time (>6 months), which leads to cells that more closely resemble the phenotype and of mature CMs.8 While easily implemented, such long culture duration is not practical for the applications targeted by HoC systems. Other maturation methods include co-culturing with other cardiac cells, patterned cell seeding, biochemical means, and mechanical or electrical stimulation/conditioning, which are discussed further in section 4.1.1.
Depending on the type(s) of cell(s) to be incorporated in the HoC system, careful selection of the ECM is paramount.124–126 For example, seeding undifferentiated hiPSCs or hESCs requires an ECM that can facilitate the subsequent differentiation. Likewise, seeding hiPSC-CMs requires an ECM suitable for tissue formation. Most commonly, ECMs incorporate proteins, hydrogels, polycations and polyanions, growth factors, and various small molecules.127–130 Common proteins incorporated in ECMs include various isoforms of laminin, collagen, fibronectin, and vitronectin.129,131 Matrigel,132 a common commercially available ECM from Corning that is comprised of 60% laminin-111 and 30% collagen-IV, is often used for hESC and hiPSC culture. However, because Matrigel is derived from animals (Engelbreth–Holm–Swarm mouse sarcoma cells), it lacks chemical definition, which can lead to varying concentration of proteins and a lack of reproducibility between studies.133 These discrepancies have motivated investigations into whether particular protein isoforms can support hESC and hiPSC growth and differentiation. Laminin-111, -511, and -521 have had the most success in supporting the growth and efficient differentiation of pluripotent hiPSCs and hESCs, especially laminin-521 combined with laminin-211 or -221.115,134,135 However, on their own, laminin-211 and -221 were unable to support pluripotent stem cell growth and differentiation.135 Collagen I has also been shown to be a poor ECM for pluripotent stem cell differentiation into CMs.134 Fibronectin and vitronectin are both glycoproteins expressed within the ECM of the heart. Nonetheless, hiPSCs have shown poor growth on fibronectin,115 making subsequent differentiation into cardiomyocytes a challenge. hiPSC-CMs re-seeded on fibronectin coated substrates were able to attach but showed inferior contractile behavior compared to those seeded on laminin.136 While vitronectin has been shown to support hiPSC differentiation to CMs,137 it does not appear to be the optimal ECM material. CM yield was lower on vitronectin when compared to Matrigel and laminin.115 Moreover, Sung et al. reported a slower beating frequency, lower cTnT expression, and shorter sarcomere length for hESCs differentiated on vitronectin compared to Matrigel and other common ECM materials.134
3.3 Environmental controls
The purpose of introducing environmental control into the HoC system is to better recapitulate the conditions experienced by the cells in vivo. HoC models can be studied using a standard cell culture incubator, with a temperature of 37 °C, atmospheric oxygen level of 21%, and high relative humidity (>90%). The bicarbonate buffer of many common culture media requires a CO2 concentration of 5% to maintain a physiologically relevant pH value at or near 7.4.91 If the HoC system will be maintained outside of a standard culture incubator, the media can be pre-conditioned in an incubator before adding to the chip to ensure appropriate pH. HEPES buffer may also be added to maintain physiologically relevant pH values.91,138 Inside the chip, environmental controls can be used to promote cardiomyocyte maturation, dictate cell structure, location, and orientation, and replicate pathological conditions. Environmental controls can be either static or dynamic and include electrical/mechanical/biochemical stimulation, spatial and temporal chemical gradients, and templated culture regions, among others.
Electrical stimulation, or pacemaking, can be incorporated into a HoC system through the use of microfabricated electrodes.139,140 Applying a periodic voltage pulse to the electrodes can regulate cardiomyocyte contractions. Mechanical stimulation, on the other hand, can be accomplished through a variety of methods.141 Typically, it accomplished by growing cardiomyocytes on a flexible material that can be actuated periodically, which mimics the mechanical contractions experienced in vivo.142 To realize this actuation, specific vacuum channels are oftentimes incorporated within the HoC, causing the flexible growth substrate to bend when vacuum is applied, and flatten when vacuum is relaxed. Biochemical stimulation involves introducing a stimulant into the culture chamber to increase or decrease beating rate, such as isoproterenol.143–145
One key advantage of incorporating microfluidics into HoC systems is having control over the spatial distribution of (bio)chemicals of interest. Chemical gradients are often encountered in the body, for example, in the context of myocardial infarction, where a portion of the heart is deprived of the necessary oxygen, and pharmacokinetics and pharmacodynamics, which are important for drug efficacy evaluation. These gradients can be established through the use of two or more independent channels with differing levels of the chemical of interest.146,147 Porous and/or permeable membranes may also incorporated into the HoC system to help establish the necessary gradients and model chemical transport through a barrier.146,148 For instance, PET membranes can be sandwiched between two PDMS chips to allow for cross-talk between upper and lower channels.149 PDMS can also serve as a membrane to control oxygen levels given its excellent gas permeability. Incorporating polymer membranes requires the surface to be functionalized to promote bonding with the microfluidic layer. Plasma treatment and arc discharge are useful for generating reactive oxygen groups on the surface of both the polymer membrane and microfluidic layer. Silane chemistry is another common functionalization strategy that can be used to introduce irreversible amine-epoxy or O–Si–O bonds between the membrane and microfluidic layer.150–152
Cell patterning is a technique for controlling the spatial position and orientation of cells on a substrate.153 This technique often involves using topographical cues to promote a particular cell alignment or morphology,60 which is especially relevant when culturing cardiomyocytes to promote tissue anisotropy and uniaxial contraction as seen in vivo.154–157 Orienting cells can be accomplished by patterning the extracellular matrix in such a manner that promotes cell growth along a particular direction.146 Engineering a cell “template” through the use of physical barriers, guides, and pillars can also be used to direct cell growth and orientation.139,158,159 Ensuring biologically relevant orientation of the tissue with a HoC helps to better replicate the in vivo mechanical properties and subsequent response to stimuli and stressors.
3.4 Analytical components
One of the major advantages of organ-on-a-chip systems is the ability to integrate in-line analytical tools within the chip. Generally, researchers are interested in characterizing electrophysiology, contractile force, secreted biomarker dynamics, or mechanical properties of the cells/tissue.165 Examples of in-line analytical tools include microelectrodes, electrochemical biosensors, field-effect transistor (FET) biosensors, elastic cantilevers, optical microscopy, optical sensors (e.g. surface plasmon resonance (SPR)), magnetic sensors, and piezoelectric biosensors, which are chosen based on the desired properties to be measured.166,167 Some examples have the added benefit of acting as both an analytical component and environmental control. For instance, a microelectrode could be used to electrically stimulate the tissue, thereby expediting maturation, while also allowing for electrophysiological measurements to be made in tandem.
The preparation and integration of an analytical component begins by determining the characteristic(s) to be measured. Microelectrodes, including electrical and FET biosensors, are often used for probing the electrophysiology of entire tissue down to individual cells by recording changes in impedance, potential, etc.168–173 These measurements require cells to be grown or seeded directly in contact with the electrode. Planar microelectrodes and microelectrode arrays are typically prepared via semiconductor fabrication methods, enabling highly controllable electrode size, geometry, and density. The most common substrate for microelectrode fabrication is glass given its low cost, biocompatibility, optical transparency, and ease with which it bonds to PDMS. Probing individual intracellular electrophysiology generally requires nano- or micro-pillars that can penetrate the cell membrane without damage.174 Again, semiconductor fabrication methods allow for precise control over pillar location and size. The pillars are made from an inert metal (e.g. Pt) to ensure adequate conductivity and minimal chance of cytotoxicity. By fabricating arrays of microelectrodes or micropillars, tissue properties can be sampled at multiple locations and time points, which improves data robustness and allows for real- or near real-time measurements. Moreover, these arrays can be easily integrated with peripheral data processing and readout equipment as well. Electrochemical and FET biosensors can also used to for biomarker quantification. To ensure sufficient selectivity towards the target biomarker, the sensor surface is functionalized with a bioreceptor, such as an antibody, aptamer, nucleic acid, etc. Common electrode materials include metals, conductive polymers, and nanomaterials. These sensors are typically placed downstream from the cell culture chamber to capture secreted biomarkers, such as proteins and small molecules.162,166,175
Mechanical properties, such as contractile force, can be probed using two-pillar constructs,176–178 flexible cantilevers,164,179,180 and piezoelectric materials.181 The two-pillar structure typically serves more as a guide for tissue formation, rather than a sensor per se, and it often requires a microscope to visualize contraction dynamics. Likewise, the flexible cantilever often requires optical visualization to quantify cantilever displacement, which is then used to extract mechanical information. However, some recent examples have integrated electrical strain sensors with the flexible cantilever to monitor contraction mechanics.180,182,183 Similarly, permanent magnets can be incorporated into one of the two pillars to generate an electromagnetic signal to characterize mechanical contractions.184 Piezoelectric materials inherently convert mechanical energy into an electrical output, making these materials well-suited for recording contraction profiles from cardiomyocytes.181
4 Applications of heart-on-a-chip technologies
Once the HoC system has been assembled, the target cell type(s) are cultured in the region of interest. The cells are allowed to grow for the desired duration to ensure the appropriate degree of maturation. Perhaps the three most common and apposite applications for HoC devices are disease modeling, cardiogenesis, and drug/therapeutic screening. The particular application and physiology will dictate the complexity of the system, which in the simplest case contains one cell type. More advanced models could require two or more cell types, for example to study the cardiotoxicity of chemotherapeutic drug metabolism in another organ. In the following section, examples of HoC systems for disease modeling and drug screening are explored in more detail.
4.1 Disease modeling and cardiac maturation
Control over the cellular microenvironment and spatiotemporal (bio)chemical distribution makes HoC platforms well-suited for in vitro disease modeling and studying cardiac maturation. Pathological conditions can be modulated in a precise manner while on-board analytical tools enable real-time measurements of tissue functionality, which is difficult to achieve with endpoint measurements commonly used in static 2D cultures. Furthermore, the advent of hiPSC-derived cardiomyocytes has provided an opportunity to model diseases with cells containing an individual's unique genetic profile, thereby providing a more personalized understanding of disease progression. To that end, HoC systems have been leveraged to study cardiac maturation as well as model a range of cardiomyopathies, myocardial infarction, ischemia/reperfusion injury (IRI), and fibrosis, discussed in more detail in the following section.
4.1.1 Cardiomyocyte maturation.
The importance of hiPSC-derived cardiac cells in HoC platforms cannot be overstated. While animal models and animal-derived cell lines have played a crucial role in many of these applications, they do not adequately recapitulate the structural and physiological properties of the human heart.141 The ability to easily obtain hiPSCs and readily generate a large number of patient-specific cardiac cells, including cardiomyocytes, fibroblasts, and endothelial cells, has been a game changer for in vitro applications. However, despite these advances, hiPSC-derived cells oftentimes display an immature/fetal-like phenotype that fails to fully mimic the human adult heart.59,185 For instance, hiPSC-CMs typically rely on glycolysis for energy production, which resembles embryonic CMs, rather than lipid oxidation commonly seen in adult CMs.186 To improve the relevance and translatability of in vitro models, various techniques for promoting maturation, including mechanical, electrical, (bio)chemical, and cell co-culture, have been investigated.8,123,141
4.1.1.1 Long-term culture.
Perhaps the most straightforward method to promote CM maturation is long-term culturing. Lundy et al. cultured hiPSC- and hESC-derived CMs for over 100 days and noted significant increases in sarcomere length, myofibril organization, cell size, and percentage of multi-nuclear cells.72 Subsequently, the calcium handling and contractile function were improved, explained in part by a shift from the α- to β-isoform of myosin heavy chain as evidenced by changes in gene expression. Similar morphological shifts were seen by Snir et al. with hESC-CMs after 36 days in culture.68 This observation aligns with genomic data reported by Piccini et al. that showed stark differences in the expression of structural markers, such as MYH6, MYL7, and MYL4, between 1 week and 8 week old hPSC-CMs.187 The differences between 4 week and 8 week old hPSC-CMs, however, was minimal, indicating much of the maturation takes place in the initial 4 weeks of culture.
4.1.1.2 Biochemical stimulation.
While long-term culture is a seemingly straightforward means to promote CM maturation, the long time requirements may not be suitable in all settings. Biochemical cues appear to be a suitable alternative method to promote CM maturation. Tailoring the level of fatty acids, glucose, and galactose in the culture media can have substantial impacts on CM maturation.188–192 This promotion of maturity is due to a shift in the energy metabolic pathway from glycolytic to oxidative, which more closely captures that seen in the adult heart. However, media supplemented entirely with fatty acids and no carbon source appeared cytotoxic.190 This effect could be reversed upon incorporation of galactose to improve the oxidative capacity of the cells, although others have reported galactose incorporation alone was not sufficient for improving cell viability.188 Hormones have also demonstrated the ability to promote cardiac maturation. Yang et al. investigated the effect of tri-iodo-L-thyronine (T3), a thyroid hormone, on cardiac maturation.193 The incorporation of T3 into the culture medium promoted physiological hypertrophy, contractile force, cell size and anisotropy, and increased mitochondrial respiratory function, all of which are signs of a more mature state. Parikh et al. also investigated T3 combined with the glucocorticoid, dexamethasone, on cardiac maturation, which increased the density of T-tubules and improved Ca2+ synchronization.194 Supplementing culture media with growth factors has been implicated as a way to improve maturity. Rupert and Coulombe studied two growth factors crucial to cardiac development, insulin-like growth factor 1 (IGF-1) and neuregulin-1β (NRG1).195 IGF-1 was found to drastically increase CM proliferation on its own, while NRG1 promoted a more mature metabolic phenotype. However, timing appears to be crucial given that simultaneous administration of both growth factors negated some of the positive effects, such as improved CM proliferation.
4.1.1.3 Mechanical conditioning.
Mechanical conditioning has been reported as a means to promote cardiac maturation.197–203 The goal is to mimic the various forces that cardiac cells and tissue experience during development.124,141,204 These include shear stress from blood flow, strain arising from cyclic contraction, stretching as a result of blood pressure changes, and forces caused by changes to the elastic modulus of the tissue. Passive mechanical stimulation was demonstrated by Rao et al. who developed a culture substrate with parallel PDMS microscopic grooves coated with fibronectin.205 The grooves helped guide the alignment of hiPSC-CMs as seen by more organized sarcomere assembly. Subsequent analysis of Ca2+ dynamics generally showed more rapid kinetics for the cells grown on structured substrates as opposed to non-structured. To study the effect of shear force on cardiac maturation, Cruz-Moreira et al. created a HoC platform with peristaltic pump capabilities.206 CMs subjected to the highest flow rate (48 μL min−1) were structurally and functionally more mature, demonstrating an upregulation of many genes involved in structure (e.g. ACTC1 and MYH6), intercellular communication (e.g. GJA1 (encodes connexin 43)), calcium cycling (e.g. SERCA2) and metabolism (e.g. PGC-1A) and a downregulation of hypoxia-inducible factor 1 alpha (HIF-1α) which suggests improved oxygen and nutrient delivery at higher flow rates. The effect of afterload, which is the systolic load experienced during a contraction, was studied using a two-post system with tunable stiffness (Fig. 3a).163 Low to moderate levels (0.1 to 1 μN μm−1) of afterload were found to promote cardiac maturation, as indicated by improved contractile function, improvements in Ca2+ handling, and genetic markers of maturation.
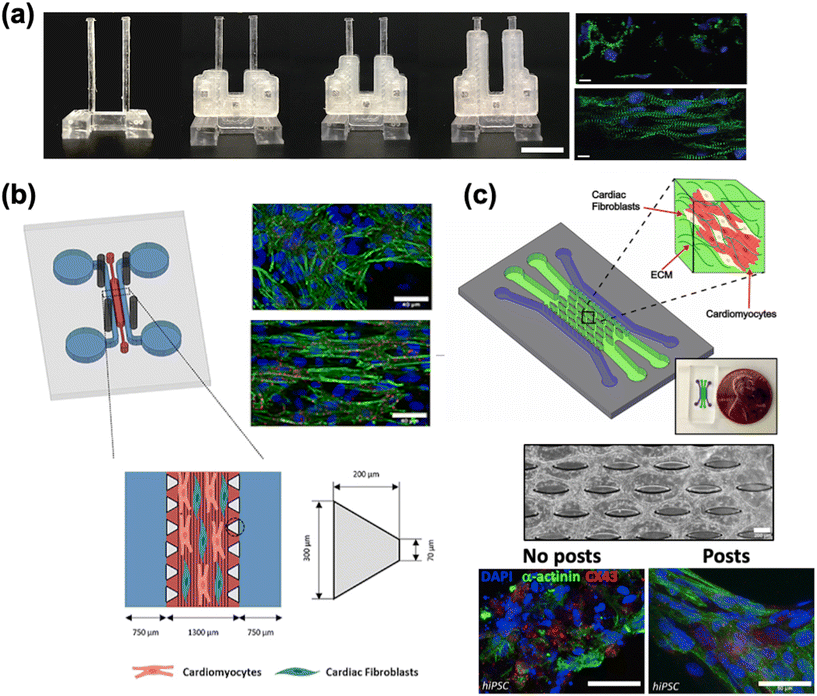 |
| Fig. 3 Cardiac maturation methods. a) A two-pillar construct with braces of varying length to modify the stiffness of the post and resulting afterload. The fluorescence images show more aligned cells grown with afterload (bottom) compared to without afterload (top) (scale bar = 10 μm) (reproduced from ref. 163 with permission from Elsevier). b) A multi-channel PDMS chip with steel electrodes to generate an electric field perpendicular to the channel, leading to improved tissue alignment (reproduced from ref. 196 with perrmission from IOP Publishing). c) A multi-channel chip with an elliptical micropost array to provide support for tissue growth (scale bar = 200 μm). Immunostaining of cardiac markers shows improved tissue alignment (scale bar = 50 μm) (reproduced from ref. 159 with permission from Elsevier). | |
4.1.1.4 Electrical pacing.
Electrical pacing attempts to replicate the constant electrical impulses experienced by CMs in vivo.122 These impulses are typically applied at frequencies in the range of 0.5 Hz to 5 Hz. Tissue subjected to electrical stimulation has shown evidence of hypertrophy, improved contractile function and calcium handling, a more organized distribution of sarcomeres, and upregulation of genes associated with maturation (Fig. 3b).139,140,154,196,207–211 Ronaldson-Bouchard et al. compared hiPSC-CMs harvested immediately after first spontaneous contractions (early-stage) and those harvested after 28 days in culture.211 Each cohort of cells was subjected to either no electrical stimulation, constant stimulation at 2 Hz, or gradual ramping from 2 Hz to 6 Hz at 0.33 Hz per day. Constant stimulation did have some effect on the early-stage cells in terms of gene expression, the gradual frequency ramping of early-stage tissue showed an upregulation of many genetic markers associated with maturation, including MYH7, GJA1, NPPA, and MAPK1. Additionally, increases to cell area, sarcomere organization, area of mitochondria, and contractile force were reported as well. However, these changes were not nearly as apparent in late-stage tissue subjected to similar conditioning.
4.1.1.5 Cellular co-culture.
Given that CMs comprise only 30% by number of total cells in the heart, co-culture with other cell types, such as fibroblasts, neural cells, endothelial cells, and immune cells has been shown to promote maturation through intercellular interaction and paracrine signaling.8,212–226 Co-culture of hESC-CMs with human cardiac fibroblasts (at an optimized 4
:
1 ratio) in a microfluidic chip containing an array of microposts was found to improve tissue maturity and anisotropy after just 2 weeks (Fig. 3c).159 Closer inspection revealed that the fibroblasts were capable of proliferating into the surrounding region, perhaps due to the mechanical cues arising from the microposts. Numerous genes associated with maturation were also upregulated for cardiac tissue culture with microposts, including conduction genes (e.g. HCN1, HCN2), calcium handling genes (e.g. CAV2.1, CAV3.1, PLN), and structural genes (e.g. GJA5, TNNT2). Co-culture of CMs with endothelial cells has also been shown to improve expression of cardiac maturation markers, including Cx43, TNNI3, Kir2.1, and CD36, with a 3
:
1 CM
:
EC ratio being most favorable.226 Moreover, this ratio showed improved electrophysiological characteristics and sarcomere organization. While 4 genetically distinct subtypes of CM were identified when hiPSC-CMs were cultured alone, hiPSC-CMs cultured with ECs showed an additional subtype that upregulated genes associated with metabolism and cardiac muscle contraction. Furthermore, the authors examined ligand–receptor complexes which unveiled many intercellular signaling pathways between CMs and ECs, including endothelin-1, ephrin, and vascular endothelial growth factor (VEGF), that are responsible for promoting CM organization, maturation of the vasculature, and the development of the conduction system. Taken together, these results suggest robust intercellular communication between CMs and other cell types and present a means to improve cardiac maturation and biological relevance.
4.1.2 Myocardial infarction and ischemia–reperfusion injury.
Myocardial infarction is caused by an occlusion in the coronary artery that results in the oxygen-deprivation of downstream cardiac tissue. Within 20 minutes to 40 minutes, sarcolemmal disruption can occur, followed by mitochondrial changes. Widespread cell death and necrosis occur within hours, even in as little as 20 minutes. Similar phenomena were demonstrated in H9c2 cells using a HoC platform designed by Ren et al.147 Here, the authors developed a system with 4 parallel culture chambers, each capable of sustaining an oxygen gradient across a central channel through the use of carbonyl cyanide-p-trifluoromethoxyphenylhydrazone (FCCP)-infused culture media. The presence of an oxygen gradient mimics the “border-zone” established between infarct and normal tissue in vivo, which has been implicated as a source of arrhythmia. The authors noted alterations to the mitochondrial membrane potential, along with a decrease in average cell size and an increase in the activation of caspase-3 (an indicator of apoptosis), were confirmed using fluorescence microscopy and found to be most drastic at the hypoxic boundary of the channel and largely unchanged at the normoxic boundary. Rexius-Hall et al. also developed a HoC device to study the border zone resulting from myocardial infarction.146 Using an elastic muscular thin film assay (MTF), the authors noted a dysfunction in contraction mechanics, namely a decrease in peak systolic and diastolic stress at the normoxic boundary compared to a uniformly normoxic environment, in agreement with previous reports.227,228 Furthermore, changes in the transcriptional profile and a decrease in Ca2+ wavefront propagation velocity were found as well, in agreement with previous reports.229 Similarly, Liu et al. reported changes in the contraction dynamics, wavefront propagation, and action potential of HL-1 cells under hypoxic conditions.174 While their system did not establish an oxygen gradient, it did incorporate both extra- and intracellular electrical sensors. The authors recorded an initial period of tachycardia after removing oxygen from the medium, followed by a decrease in the beating rate to below normoxic levels and the development of an arrhythmic beating pattern.
Restoration of blood flow and oxygen supply after the ischemic episode results in the generation of reactive oxygen species, rapid changes in intracellular pH, and an overload of Ca2+, which can result in further cell death.230–237 Additionally, due to the poor regenerative ability of the myocardium, post-ischemia tissue reparation results in increased scarring and, subsequently, increased stiffening of the myocardium. However, pre-clinical animal trials have failed to successfully recapitulate the human physiology and, as a result, no clinical treatment for IRI exists despite its ubiquity. To help address the lack of clinically relevant treatments, Chen and Vunjak-Novakovic developed an on-chip IRI model which utilized flexible pillars for hiPSC-CMs to attach and align to.238 The authors explored four therapeutic strategies using the developed system: ischemic preconditioning, normalization of intracellular pH, minimization of mitochondrial permeability transition pore (MPTP) opening, and reduction of oxidative stress levels. Ischemic preconditioning, whereby the tissue is exposed to brief cycle(s) of controlled ischemia and reperfusion before the primary ischemic or reperfusive episode,5,239 was found to improve cell viability after reperfusion, possibly due to the activation of pro-survival kinases during preconditioning.240,241 Intracellular pH normalization and minimization of MPTP opening were also found to improve outcomes after reperfusion to varying degrees. On the contrary, treatment with an antioxidant during reperfusion to mitigate oxidative stress levels did not lead to an improved outcome. Clinical trials studying the effect of antioxidants on patients undergoing MI or coronary artery bypass surgery have shown varying results,242–247 which further emphasizes the need for robust pre-clinical in vitro models. While most studies focus on the effect of MI and IRI on cardiomyocytes given their role in the degradation of contractile force in vivo, they comprise only 30% of the cell population in the heart. Endothelial cells, fibroblasts, and immune cells make up a substantial portion of the cell population within the heart, and their incorporation in an in vitro model can improve physiological relevance. Fibroblasts, for instance, have demonstrated cardio-protective behavior through intercellular signaling.248–250 Endothelial251–253 and immune254–256 cells have also demonstrated cardio-protective and cardio-reparative capabilities through nitric oxide generation via endothelial nitric oxide synthase (eNOS) and other anti-inflammatory behavior. In contrast, others have reported pro-inflammatory effects from fibroblasts257 and vascular damage from endothelial and immune cells.258–260 Furthermore, the heterogeneity in spatiotemporal and phenotypic distribution of immune cells is extremely complex during and after ischemia but largely dictates the degree of inflammatory and reparative response.255,261,262 While it is impossible to completely replicate the complex orchestrated response to MI in a HoC system, the addition of other cell types may lead to more physiologically relevant results and eventually improved clinical outcomes.
To this end, Veldhuizen et al. developed a HoC platform with embedded microposts to improve tissue formation using hiPSC-CMs and CFs as model cell lines.263 The authors examined three environments, namely hypoxic (1% O2), physioxic (5% O2), and hyperoxic (21% O2), and demonstrated some of the hallmarks of an ischemic episode, including altered expression of the key hypoxia-responsive genes, smooth muscle alpha (α)-2 actin (ACTA2) and vascular endothelial growth factor A (VEGFA). However, no changes in transforming growth factor beta-1 (TGFB1) gene expression were found. It is speculated that the addition of macrophages into the platform could change this given they release the proteins TGFβ-1 and angiotensin II (ANGII). Signs of contractile dysfunction, reduced cell viability after reperfusion, and increased markers of fibrosis, including α-smooth muscle actin (αSMA) and F-actin were also reported. Interestingly, (αSMA) and F-actin levels did not change when hiPSC-CMs and CFs were co-cultured in 2D on a coverslip, further highlighting the importance of 3D culture models to more accurately replicate human physiology.
Yadid et al. also noted a reduction in cell viability and contractile function in human stem cell-derived CMs subjected to hypoxia for 3 h.264 Here, the authors set out to better elucidate the role of endothelial cell-derived extracellular vesicles (EEVs) in mitigating the deleterious effects of IRI. EVs are small (typically <300 nm) cell-secreted particles that play an important role in intercellular communication by transporting proteins, lipids, microRNAs, and other genetic material between cells.265,266 While the content of an EV is highly dependent on the source and receptor cell type, in general, EVs appear to be cardio-protective or reparative.267–277 Yadid and co-workers found that treatment with EEVs (ECs are not in co-culture) after IR led to improved viability and contractility with the treated CMs displaying a protein profile that more closely resembled uninjured CMs. A key finding here is that the cardio-protective effects of EEVs require their uptake by CMs, indicating an intracellular mechanism (Fig. 4a and b). Ellis et al. followed up this work with a new HoC system that places hiPSC-CMs and hiPSC-ECs in co-culture to better mimic the vasculature of the human heart. By analyzing three characteristic EV markers (CD9, CD63, and TSG101) at different points of the IR episode, the authors found that specific EV subpopulations are released at various stages of IR. Additionally, their system integrated on-board biosensors and EV lysis chamber based on standing acoustic waves (SAW), which enabled the analysis of the EV internal contents, particularly miR-1, miR-208b, and miR-499. Comparison of miRNA levels between the HoC system and clinical samples showed very good agreement at both ischemic and reperfusive stages, indicating the in vitro system could replicate some in vivo behavior.
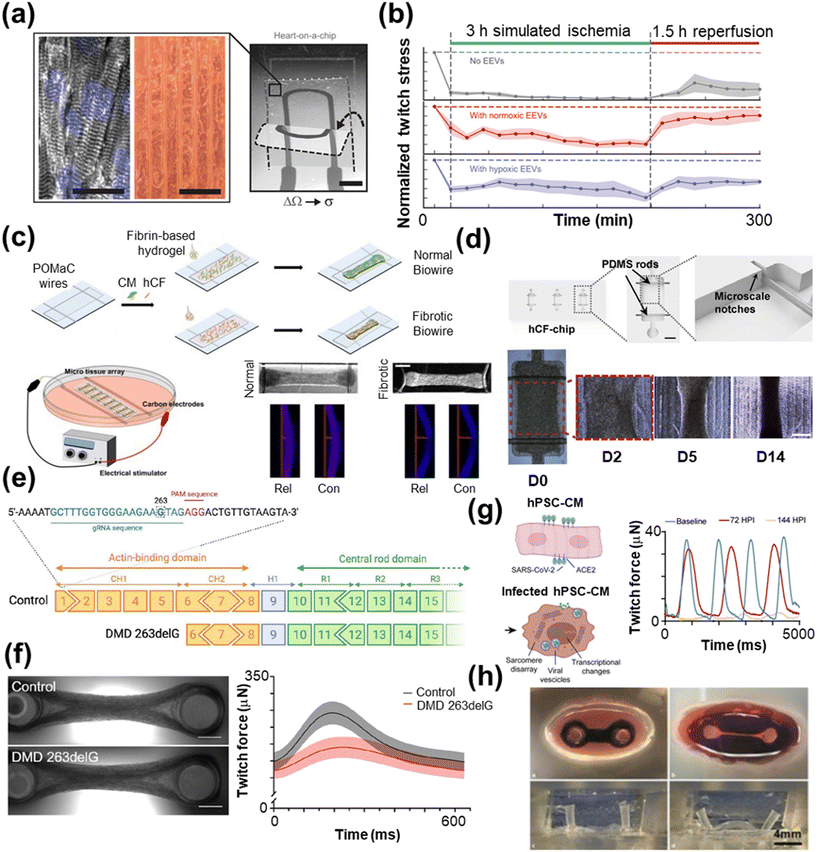 |
| Fig. 4 Disease modeling with HoC systems. a) A MTF with an integrated strain sensor (scale bar = 2 mm) and microgroove template for cardiac tissue alignment (scale bars = 100 μm) to monitor contractile behavior during ischemia–reperfusion. b) The resulting twitch force measurements post-extracellular vesicle treatment (reproduced from ref. 264 with permission from the American Association for the Advancement of Science). c) A Biowire model of cardiac fibrosis comprised of poly(octamethylene maleate (anhydride) citrate) (POMaC) wires are carbon electrodes for electrical conditioning (scale bar = 500 μm) (reproduced from ref. 285 with permission from the American Chemical Society). d) A human cardiac fibroblast (hCF) chip with PDMS rods (scale bar = 1 mm) to support the tissue over the course of 14 days (scale bar = 500 μm) (reproduced from ref. 286 with permission from Elsevier). e) An example gene editing process using the CRISPR/Cas9 technique, which is particularly useful for modeling genetic diseases. f) A two-pillar assay of control and tissue with Duchenne muscular dystrophy (DMD), which shows lower twitch force compared with control tissue (scale bar = 1 mm) (reprinted from ref. 288 under Creative Commons NonCommercial License 4.0 (CC BY-NC)). g) hPSC-CMs infected with SARS-CoV-2 show lower twitch force compared to the control 72 and 144 hours past infection (HPI) (reproduced from ref. 289 under Creative Commons License CC BY 4.0). h) Optical images of a biomimetic cardiac tissue model (BCTM) before loading cells (top left) and after establishing the engineered heart tissue (EHT) (top right). The system can mimic the end of systole (bottom left) and diastole (bottom right), as shown without EHT present (reproduced from ref. 290 with permission from Karver Publishers). | |
As demonstrated by these reports, incorporating multiple cell types is crucial for recapitulating the native human physiology. Crucially, intercellular communication via EV release and other paracrine signaling processes has garnered tremendous interest for both improving the fundamental understanding of IRI and MI, as well as a potential target for early diagnosis and therapeutic intervention.265,278
4.1.3 Cardiac fibrosis and remodeling.
Closely related to MI, cardiac fibrosis describes the accumulation of ECM proteins within the cardiac interstitium, which results in increased stiffening of the heart muscle and reduced contractile function.279,280 Cardiac fibrosis is a reparative process associated with or a result of IRI, aging, diabetes, genetic cardiomyopathies, or metabolic syndrome. Fibrotic remodeling is often caused by a substantial loss of cardiomyocytes, for example during IRI. Because the heart has limited regenerative ability, the damaged tissue is replaced with collagen-rich scar tissue by myofibroblasts in an effort to maintain the structural integrity of the heart and prevent rupture. The alteration of the mechanical properties of the myocardium can lead to poor contractile force, poor electrical connection between myofibroblasts and CMs, arrhythmia, and reduced ejection fraction, which, as a result, can further lead to cardiac hypertrophy and accelerated decline in heart function.
Ugolini et al. developed a HoC system to study mechanical stimulation on CFs in vitro.142 Mechanical strain did have an effect on CF morphology, including cell area and preferential alignment of the cellular main axis orthogonal to the strain direction. An increase in CF proliferation was also reported for cells subject to 2% and 8% mechanical strain. Occhetta et al. leveraged a HoC platform with mechanical actuation to study the formation of cardiac scar tissue in vitro.281 Using cardiac fibroblasts suspended within a fibrin hydrogel, the authors examined the effects of mechanical stimulation and exposure to the pro-fibrotic growth factor TGFβ-1 on scar tissue evolution. Hallmarks of cardiac wound-healing, include fibroblast proliferation, fibroblast to myofibroblast transition, matrix deposition, and tissue stiffening were seen. TGFβ-1 was found to have a profound impact on fibroblast proliferation while mechanical stimulation (both with and without TGFβ-1) led to more pronounced transition from fibroblast to myofibroblast phenotype, which was also seen by Kong et al.282 Interestingly, the increased level of myofibroblasts as a result of mechanical stimulation also coincided with a more homogeneous and stiffer tissue, likely attributable to the central role myofibroblasts play in ECM generation and deposition.
Given that cardiac fibrosis is intrinsically a multi-cellular process between CMs and (myo)fibroblasts, examples of HoC platforms typically contain multiple cell types to recapitulate this behavior. Lee et al. developed an HoC platform with cardiac microtissues that combined hESC-CMs and mesenchymal stem cells (MSCs) as a source of fibroblasts.283 Subjecting the microtissue to TGFβ-1 for two weeks resulted in irregular contraction patterns and myofibroblast differentiation, a result also seen by Sadeghi et al.284 Moreover, a thicker collagen layer was observed despite an overall decrease in microtissue diameter caused by CM apoptosis. Wang et al. also reported increased collagen density, impaired contractile function, increased tissue stiffness and passive tension, reduced active force, and increased excitation threshold in a HoC system that incorporated electrical stimulation and parallel rods to monitor contraction mechanics (Fig. 4c).285 Mastikhina et al. observed similar behavior after exposing cardiac microtissue to TGFβ-1 for three weeks (Fig. 4d).286 By incorporating parallel PDMS rods for mechanical characterization within the culture chamber, the authors were better able to quantify the degradation of contractile function. For instance, after 21 days of TGFβ-1 exposure, contraction force was approximately 7 times lower compared to the control. The authors also treated the tissue with the anti-fibrotic drug pirfenidone and measured a reduction in tissue stiffness. However, pirfenidone was not able to fully reverse tissue fibrosis. In a follow up report using a similar system, Mourad et al. showed fibrotic tissue possessed a genetic profile indicative of senescence.287 Treatment with a combination of the anti-senescence drugs dasatinib and quercetin led to improvements in active force and a downregulation of many senescence markers, such as tumor-suppressors CDKN1A and CDKN2A and cytokines such as interleukin-17A (IL-17A), IL-1α, and macrophage inflammatory protein 1 α/β. However, no difference in collagen density or cell proliferation was seen after drug treatment.
4.1.4 Genetic and inherited cardiomyopathies.
Cardiomyopathies can manifest from a variety of genetic mutations which cause dysfunctional energy metabolism, structural irregularities, or poor ionic homeostasis. Recent advances in gene editing along with the ability to obtain cardiac cells from patient-derived iPSCs have enabled researchers to study genetic and inherited cardiomyopathies in human relevant models.291 While in vitro models, HoCs, and engineered cardiac tissue cannot fully recapitulate all of the intricacies of the human heart, they have led to improvements in disease modeling and evaluation of potential treatments. Furthermore, the rarity of many genetic disorders make it difficult, if not impossible, to establish a large enough cohort for a clinical trial. This challenge could be overcome through the use of stem cell-derived cardiac cells and new gene editing methods. In this section, we highlight and discuss examples of various inherited and genetic cardiomyopathies that have been modeled with HoC and engineered heart tissue platforms.
4.1.4.1 Barth syndrome.
Barth syndrome (BTHS) is a rare, but under-diagnosed, multi-system disorder that occurs as a result of mutations or deletions of the tafazzin gene (TAZ), which is responsible for the acylation of cardiolipin, a major phospholipid of the inner mitochondrial membrane.292–296 BTHS can cause a variety of cardiomyopathies, including prolonged QTc interval, hypertrophy, and arrhythmia, among others. Wang et al. developed a HoC model of mitochondrial cardiomyopathy using CMs derived from hiPSCs collected from two patients with BTHS.297 BTHS-hiPSC-CMs demonstrated impaired sarcomere assembly and lower twitch and peak systolic stress when grown on a thin film cantilever assay, both of which contributed to increased production of ROS. The authors also measured increased basal oxygen consumption rates in diseased tissue. Contractile function of the BTHS-hiPSC-CMs tissue could be restored, however, through transfection with TAZ-modified RNA or treatment with linoleic acid, a precursor of mature cardiolipin,298 thereby demonstrating the potential of in vitro HoC systems for combined disease modeling and therapeutic evaluation.
4.1.4.2 Hypertrophic cardiomyopathy (HCM).
Hypertrophic cardiomyopathy is a common (≈1 in 500)299 genetic heart disease that can lead to arrhythmic sudden death, heart failure, and atrial fibrillation.300 The disease is often, but not exclusively, characterized by a remodeling of the left ventricle, particularly changes in wall thickness.301,302 A majority (70%) of the genetic mutations responsible for HCM occur in two sarcomeric genes, MYH7 and myosin-binding protein C (MYBPC3).300 To better understand the mechanisms that link these genetic modifications with particular HCM phenotypes, Cohn et al. leveraged the CRISPR/Cas9 gene editing technique to introduce mutations in the MYH7 and MYBPC3 genes. Using a two-pillar assay, they found the modified cardiac tissue exhibited altered Ca2+ transients, prolonged relaxation times, and increased twitch force and resting tension.303 The observed hypercontractile behavior was reversible to some degree through treatment with verapamil, a Ca2+ channel blocker, or blebbistatin, a direct myosin inhibitor, although only blebbistatin lowered both twitch force and resting tension. In the same year, Zhao et al. built upon their previously reported “Biowire” platform304 to study phenotypic differences between hypertensive patients with and without left ventricular hypertrophy (LVH).305 The Biowire II platform enabled electrical conditioning and culturing of hiPSC-CMs obtained from patients for up to 8 months. Engineered tissue from patients affected with hypertension and LVH consistently showed an upregulation of genes associated with cardiac hypertrophy and heart failure, as well as an inability to generate a contractile active force after 8 months. In another report, Cashman et al. sought to better understand the role of the BRAF gene in patients with cardio-facio-cutaneous syndrome (CFCS) that show evidence of HCM.306 Using patient-derived hiPSC-CMs and cardiac stromal cells cultured on PDMS pillars, the authors reported a larger tissue cross-section, accelerated twitch dynamics, and higher levels of the hypertrophic marker atrial natriuretic peptide (ANP) in BRAF-mutant tissue, which is in agreement with other reports of hypertrophic tissue.307,308 The E99K mutation in the ACTC1 gene (encodes α-cardiac actin) was studied using patient-derived hiPSC-CMs from a patient displaying HCM and left ventricular non-compaction (LVNC) along with the patient's two sons (one non-carrier and one carrier with a normal ECG).309 Interestingly, while the hiPSC-CMs derived from the father showed numerous pathogenic phenotypes, most were not observed in hiPSC-CMs derived from the two sons, even those with the E99K mutation. The common pathogenic phenotype was arrhythmia as determined with a two-pillar assay. The distinct differences between diseased tissues highlights the complex interplay between a particular mutation, background genetics, and age-dependence.
4.1.4.3 Duchenne muscular dystrophy (DMD).
Duchenne muscular dystrophy is an X-chromosome linked, muscle-wasting disorder that is prevalent in less than 100 in 1
000
000 males and less than 1 in 1
000
000 females.310–312 Mutations to the DMD gene alter the encoding of muscular dystrophin, making the muscles more susceptible to damage and subsequent loss of functionality. As a result, cardiomyopathy can arise and eventually lead to heart failure.310 Bremner et al. leveraged CRISPR/Cas9 to introduce a mutation in the DMD gene (DMD 263delG) of hiPSCs derived from a healthy patient to delineate genotype–phenotype correlations (Fig. 4e and f).288 After differentiation to hiPSC-CMs, a two pillar HoC platform was used to study the contractile behavior of the engineered microtissue. The modified tissue showed a dysregulation of biological processes related to cardiac muscle development, contraction, membrane potential regulation, Ca2+ handling, and ECM organization. Measurements of contractile function confirmed lower twitch force, beating irregularities, and slower kinetics in modified tissue, while fluorescence imaging revealed elevated cytosolic Ca2+ levels and prolonged Ca2+ transients. Macadangdang et al. also saw similar behavior that was more pronounced in hiPSC-CMs subjected to a combinatorial maturation (ComboMat) procedure compared to immature hiPSC-CMs, emphasizing the importance of cardiac maturation in in vitro HoC systems.313
4.1.4.4 Dilated cardiomyopathy (DCM).
Dilated cardiomyopathy is one of the most common cardiomyopathies in the world.314 This disorder is typically characterized by the dilation of the left or both ventricle(s) and systolic dysfunction that cannot be attributed to pressure–volume overload or coronary artery disease.314,315 Alterations of genes that encode cytoskeletal and sarcomeric proteins are a common cause of inherited DCM.314 Examples of causative genes are TTN (encodes titin), DMD (encodes dystrophin), LMNA (encodes lamin A/C), MYH7 (encodes β-myosin heavy chain), RBM20 (encodes RNA binding motif protein 20), and TNNI1 (encodes cardiac troponin 1), among others, especially those implicated in HCM.177,314–316 The effect of a missense mutation S635A in the RBM20 gene was examined by Streckfuss-Bomeke et al. using DCM patient-derived hiPSC-CMs.317 Although no differences in cell surface area were seen, the modified tissue showed a markedly irregular distribution of the sarcomeric protein α-actinin along with dysfunctional Ca2+ cycling. As a result, the modified tissue demonstrated lower contractile force and decreased passive stress compared to control samples. The RBM20 mutation also led to preferential retention of the larger and more elastic TTN protein isoform N2BA as opposed to smaller and stiffer N2B isoform, which is predominant in a healthy heart318 while higher N2BA content has been reported in failing hearts.319,320
Mutations of the TNNT2 gene also caused sarcomeric irregularities and contractile dysfunction.321 Dai et al. investigated a particular mutation, R173W, and confirmed poor sarcomeric organization and contractile dysfunction using a two-pillar assay.322 The mutated troponin T (TnT) showed reduced binding with tropomyosin (Tm), which could be the cause for poor sarcomeric organization. Activation of AMP-activated protein kinase (AMPK), which interacts with myosin heavy chain 7, using A-769662 led to improved contractile function and sarcomeric alignment, suggesting a potential therapeutic pathway for improving DCM recovery.
In addition to sarcomeric and cytoskeletal genes, Wauchop et al. recently reported on the impact of mutations to the sodium voltage-gated channel alpha subunit 5 (SCN5A) gene, which is responsible for encoding the cardiac sodium channel (Nav1.5).323 Patient-derived hiPSC-CMs with the R222Q missense mutation to SCN5A were first seeded as isolated single cells or cardiac sheets, although both failed to display the electrophysiological characteristics associated with the R222Q mutation. This is most likely due to tissue immaturity as the R222Q mutation is only expressed in adult isoforms of SCN5A.324 To promote hiPSC-CM maturation, the tissue was seeded in the previously reported Biowire platform285 for long-term culture. Expression of the adult variant of SCN5A was ≈90% in mature tissue, and as a result, the disease phenotype (i.e. contractile dysfunction, dilation, sarcomeric irregularity) was seen, which again highlights the importance of tissue maturity in in vitro disease modeling.
4.1.4.5 Pompe disease.
Pompe disease is a severe autosomal recessive disorder that typically, although not exclusively, presents itself during infancy and is caused by the loss of acid α-glucosidase (GAA).325 GAA is the enzyme responsible for converting glycogen into free glucose within lysosomes. Accumulation of glycogen can result in muscle weakness, respiratory issues, and cardiac hypertrophy. To study the effect of Pompe disease on cardiac function, Raval et al. used two patient-derived hiPSC-CM cell lines, each possessing different mutations in the GAA gene (del ex18/del ex18: deletion of exon 18 and 1441delT/2237G>A: deletion of a T nucleotide at GAA cDNA position 1441 and G to A transition at GAA cDNA position 2237).326 Accumulation of glycogen within the lysosome was confirmed by electron microscopy. Contractile function was evaluated using a two-pillar assay, and the del ex18/del ex18 hiPSC-CMs demonstrated accelerated kinetics and reduced paced peak contractile force. Nonetheless, contractile function was largely similar between diseased and control tissues.
4.1.4.6 Friedreich's ataxia.
Friedreich's ataxia is an autosomal recessive disease that is caused by a mutation in the FXN gene, which suppresses the expression of frataxin, a small mitochondrial protein.327 Cardiac dysfunction is the most attributed cause of death in patients with Friedreich's ataxia.328 Using two patient-derived hiPSC-CM lines and human fibroblasts, Wong et al. utilized the two-pillar PDMS chip to study contractile function in engineered cardiac tissues.329 Expression of FXN was confirmed to be about 70% lower at the transcriptional level and 40% to 60% lower at the protein level in diseased tissue compared to the control. Force generation was less than half in diseased tissue and contraction kinetic were significantly slower in diseased tissue compared to control samples. Contractile dysfunction was largely reversed, however, after transduction with lentiviral-FXN.
4.1.4.7 Hypoplastic right heart syndrome (HRHS).
Hypoplastic right heart syndrome is characterized by an underdeveloped right ventricle and can be accompanied by a defect in the atrial septum.330 Using a two-pillar PDMS chip, Lam et al. seeded patient-derived hiPSC-CMs with human fibroblasts to study contractile function.331 The tissue was subjected to 1 Hz, 1.5 Hz, and 2 Hz electrical pacing and showed significantly reduced contractile force compared to the control samples. The contraction kinetics also remained constant in diseased tissue over the course of 2 weeks, whereas control samples showed a gradual acceleration as the cells matured. Furthermore, Ca2+ dynamics and electrophysiology were comparable between normal and diseased tissue, suggesting the calcium handling remained intact. Diseased tissue was found to downregulate gene transcripts involved in cardiac maturation and development (natriuretic peptide B (NPPB), four and a half LIM domains 2 (FHL2), PDZ and LIM domain 3 (PDLIM3)) and cardiac contraction (MYH7, MYL2, etc.), suggesting abnormal and immature cardiac development.
4.1.4.8 Catecholaminergic polymorphic ventricular tachycardia (CPVT).
Catecholaminergic polymorphic ventricular tachycardia is an exercise- or emotional-induced polymorphic ventricular tachycardia that occurs in the absence of structural abnormalities.332,333 Many incidences of CPVT are caused by mutations to the RyR2 or CASQ2 genes, which encode proteins that are responsible for Ca2+ handling and transport. Using engineered heart tissue on a thin film cantilever array and Ca2+ imaging, Park et al. demonstrated hallmarks of CPVT with patient-derived iPSC-CMs, including re-entrant arrhythmia after electrical pacing and catecholamine (isoproterenol) administration.334 Furthermore, the authors were able to show the phosphorylation of ryanodine receptor type 2 (RYR2) at the serine-2814 position by Ca2+/calmodulin-dependent protein kinase II (CaMKII) is needed to realize the arrhythmic potential in CPVT patient-derived cardiac tissue.
4.1.5 Severe acute respiratory coronavirus-2 (SARS-CoV-2) and cardiac dysfunction.
Severe acute respiratory coronavirus-2 is the virus responsible for causing coronavirus disease 2019 (COVID-19), which was first reported in Wuhan, China in late 2019.181 Since then, the World Health Organization has reported almost 770 million confirmed cases and nearly 7 million deaths worldwide as of August 2023, although the actual count could be much higher.335 Although COVID-19 is predominantly a respiratory disease, it has also been implicated in various cardiovascular complications, such as arrhythmia, myocardial infarction, and myocarditis.16,336,337 SARS-CoV-2 viral particles were even found in cardiac tissue obtained from a child who died following COVID-19 early on in the pandemic.338 Nonetheless, the mechanism(s) by which COVID-19 causes cardiovascular dysfunction, be it directly through myocardial infection or indirectly through virus-associated systemic inflammation, is not entirely understood. To improve upon this, Bailey et al. developed an engineered heart tissue model with hiPSC-derived cardiomyocytes, fibroblasts, and/or macrophages to study COVID-19 myocarditis.339 The two-pillar PDMS system showed infected cardiomyocytes primarily at the periphery of the tissue along with macrophage accumulation in the infected regions. Using a two-pillar assay, the infected tissue also demonstrated reduced contractile function and slower kinetics, which resulted from sarcomere loss and reduced troponin T expression. The authors tested two therapeutic agents, a TANK-binding kinase 1 (TBK1) inhibitor, which blocks viral nucleic acid sensing pathways, and remdesivir, which inhibits viral replication. While both remdesivir and TBK1 inhibition prevented reductions in TNNT2 and MYH7 mRNA expression, only remdesivir could prevent sarcomere loss and cell death suggesting cardiomyocyte infection as a root cause of disease rather than inflammation. Marchiano et al. also generated engineered cardiac tissue from hPSCs to study SARS-CoV-2 infection (Fig. 4g).289 CMs were found to express ACE2, making them susceptible to viral entry and infection, whereas smooth muscle cells did not. Two multiplicities of infection (MOI) were studied, 0.1 and 5. Both MOIs led to viral infection and replication within the CMs, even causing cessation of beating and cell death after 48 h at MOI = 5. Using a multi-electrode array (MEA), the authors noted a decline in the electrophysiological properties of the infected cells, including a reduced beating rate, lower depolarization spike amplitude, and decreased electrical conduction velocity. Furthermore, using a two-pillar PDMS chip with an embedded magnetic sensor, a degradation in the contractile properties could be seen beginning at 48 h post-infection with the twitch force falling to near 0 after 6 days, which could be attributed to decreased CM density and a loss of sarcomeric organization. These results again suggest that SARS-CoV-2 can directly cause heart damage independent of inflammation. A similar decline in contractile function was reported in a pre-print paper from Xing et al. who developed a HoC platform that mimicked the myocardium and vasculature based on their previously reported InVADE platform.340,341 However, effects to contractile function could only be seen after incorporating peripheral blood mononuclear cells (PBMC) into the chip, which resulted in increased production of cytokines and chemokines and higher levels of circulating cell-free mitochondrial DNA. Interestingly, these effects were largely reversible upon treatment with EEVs, indicating a potential therapeutic pathway to minimize inflammation in patients with SARS-CoV-2 induced myocarditis.
4.1.6 Mechanical stress.
A distinct advantage of in vitro HoC systems over conventional 2D static cell cultures is the ability to incorporate mechanical stimulation within the cellular microenvironment. In the case of stem cell-derived-CMs, mechanical stimulation has been demonstrated as a means to advance maturation,163,342 which has remained a fundamental challenge in their usage for disease modeling and drug testing. On-chip conditioning of CMs with mechanical stimulation aims to mimic the various mechanical forces experienced in vivo, including shear stress from blood flow, cyclic strain during beating, stretching due to blood pressure changes, and forces due to changes in elastic modulus over time.124,141,204 While mechanical stimulation promotes cardiac maturation, it has also been implicated in various cardiopathologies.343 For instance, Rogers et al. utilized a two pillar design capable of mimicking the rhythmic pressure and volume changes of the heart to study the effects of mechanical stimuli (Fig. 4h).178,344 Both volume and pressure overload resulted in structural changes to the tissue, altered gene expression, and oxidative stress. Pressure overload conditions in particular were associated with concentric hypertrophy and increased fibrotic signatures, while tissue subjected to volume overload showed myocyte thinning and matrix degradation. Leonard et al. also measured changes to gene expression in hiPSC-derived cardiac microtissues subject to varying degrees of afterload cultured in an adjustable two post system.163 While low levels of afterload (i.e., ≈0.1 μN μm−1) improved maturation, higher levels of afterload (i.e., ≈10 μN μm−1) resulted in upregulation of hypertrophic markers, including ANP, brain natriuretic peptide (BNP), and α-skeletal actin (ACTA1), metabolic changes from fatty acid utilization (mature-like) to glycolysis (fetal-like), and increased expression of fibrosis-associated growth factors, similar to other reports.176,345 Despite changes in gene expression, the authors noted the tissue stiffness remained unchanged despite the increase in fibrosis markers.
4.2 Heart-on-a-chip for drug screening and cardiotoxicity assessment
HoC systems have tremendous potential in drug development and cardiotoxicity applications, which are currently dominated by animal studies. While animal models have been instrumental for evaluating therapeutics, there remains significant disagreement between results of animal studies and clinical trials346–349 prompting some to call into question their use.350 Moreover, animal models contribute millions of US dollars and years of time to the already significant cost and time associated with drug development,10,351 which takes around 1 billion USD and 12 years per drug.352,353 Nonetheless, animal studies oftentimes fail to adequately capture human (patho)physiology and pharmacology, leading to poor translation to clinical trials349 or removal from the market due to safety concerns.354 Because of this poor translation, policies surrounding animal testing are beginning to change, as evidenced, for example, by the US Food and Drug Administration (FDA) recently lifting the requirement of animal testing for new drugs.355 Traditional 2D cell culture, while inexpensive and well-established, is also largely limited in its capacity to recreate the disease phenotypes seen in vivo, likely due to the mechanical properties of culture plates and altered pharmacokinetics and pharmacodynamics. The use of in vitro human microphysiological models is a viable alternative to conventional 2D cell culture due to their potential for automation, human relevance, and improved control over experimental conditions. Furthermore, the ability to obtain patient-derived PSCs has opened the door to increasingly personalized disease treatment, while advances in microfabrication and automation have improved throughput tremendously, potentially leading to rapid screening and characterization of drug–drug interactions. In the following section, we discuss in more detail reports of HoC systems for drug/therapeutic screening and cardiotoxicity assessment.
4.2.1 Evaluation of drug and therapeutic efficacy.
The high costs and long timeline (10+ years)356 currently required to bring a drug to market has prompted the search for alternatives to animal testing. Furthermore, many drugs fail to make it through clinical trials despite the large resource requirements, with only a ≈10% success rate. To reduce time-to-market and improve success rate, HoC systems could provide a valuable link between pre-clinical animal studies and clinical trials or even serve as a standalone replacement to animal studies all together. One of the most popular drugs tested with HoC systems is the non-selective β-adrenergic receptor agonist isoproterenol,143–145,155,160,168,172,181,224,225,357–368 which can help treat bradycardia. Isoproterenol is similar in structure and function to epinephrine, which has also been evaluated using HoC systems.369,370 The response to either drug in vitro has been similar to clinical data, which show responses in the range of 5 nM to 800 nM.371–375
Using the Biowire platform, Xiao et al. studied the effect of sodium nitroprusside (SNP) perfusion on cardiac tissue (Fig. 5a).304 The system was designed such that the cardiac tissue grows circumferentially around a suspended lumen, which mimics the capillaries found in the native myocardium and helped to align the CMs. SNP, a nitric oxide (NO) donor, was perfused through the lumen and NO levels in the surrounding medium reached 100 μM, which is higher than physiological levels in vivo. NO is a vasodilator released by endothelial cells in the native myocardium and plays a crucial role cardiovascular disease progression and prevention.376 In the Biowire, NO was found to decrease beating frequency of the cardiac tissue due to a degradation of the myofibrillar cytoskeleton as determined by α-actinin fluorescent imaging. In another iteration of the Biowire platform (Biowire II), this time with the tissue grown perpendicular to and anchored to two suspended wires, Wang et al. evaluated the efficacy of three drugs used to treat angiotensin II (Ang II)-induced cardiomyopathy, losartan, relaxin, and saracatinib.377 Ang II treatment of the microtissue showed various hallmarks of disease, including increased passive tension, an acute positive inotropic response followed by a chronic negative inotropic response, and an increase of the excitation threshold. After 2 weeks of treatment, all 3 of the drugs reduced passive tension, although saracatinib also reduced the active force, indicating tissue degradation. Relaxin appeared to be the most effective at reducing passive tension, increasing active force, and rescuing the electrophysiological properties of the tissue, all at a dose determined to be safe from toxicity tests conducted on the same system.
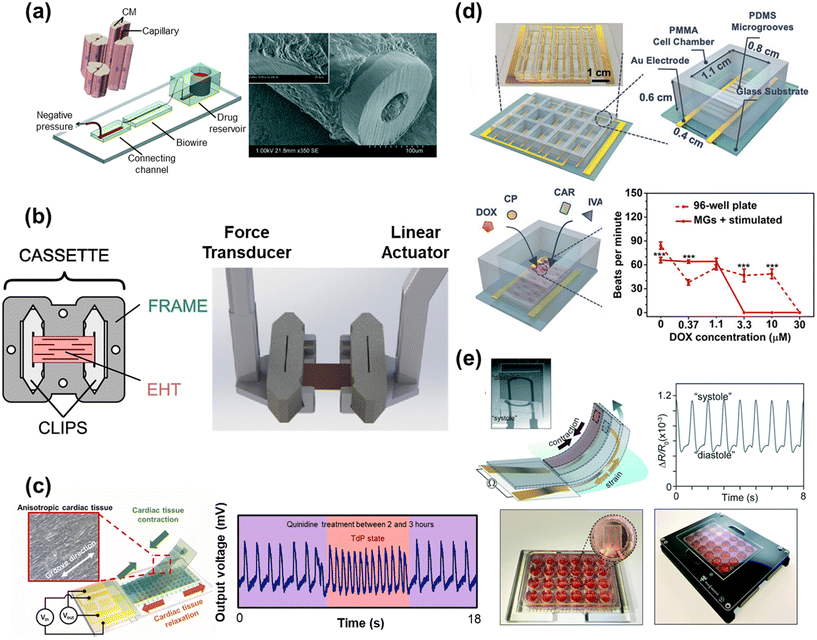 |
| Fig. 5 Drug efficacy and toxicity assessment with HoC systems. a) A perfusable Biowire platform to mimic drug diffusion through the capillaries and muscle tissue, used here for studying the effect of nitric oxide on muscle tissue (reproduced from ref. 304 with permission from the Royal Society of Chemistry). b) A custom cardiac tissue holder capable of mechanical characterization and adjustable loading (reproduced from ref. 344 with permission from Elsevier). c) A flexible cantilever with an integrated strain sensor and patterned microgrooves to promote tissue alignment. The resulting signal output after treatment with quinidine shows a torsades de pointes (TdP) state (reproduced from ref. 182 with permission from Elsevier). d) A HoC platform with patterned microgrooves (MGs) and electrical stimulation capabilities for evaluating the cardiotoxicity of antineoplastics (doxorubicin (DOX) and cyclophosphamide (CP)) and potential drugs to ameliorate cardiotoxicity (ivabradine (IVA) and carbachol (CAR)) (reproduced from ref. 378 with permission from John Wiley and Sons). e) A flexible cantilever with an integrated strain sensor for monitoring contractile behavior as part of a larger array for high-throughput drug toxicity screening (reproduced from ref. 379 with permission from the Royal Society of Chemistry). | |
For the treatment of HCM, Sewanan et al. and Prondzynski et al. examined two potential therapeutic candidates, mavacamten (a β-blocker) and diltiazem (a Ca2+-channel blocker), respectively.380,381 The Campbell group developed a custom tissue holder that was capable of on-board mechanical characterization and adjustable loading (Fig. 5b).376,344 Using this platform, mavacamten treatment was shown to improve diastolic tissue stiffness and relaxation time, as well as reduce the myocardial workload and power output, which would help lower the energetic cost of contraction caused by HCM.382,383 Prondzynski et al. also observed a reduction in absolute force, relaxation time, and APD after treatment with 3 μM diltiazem using a two-pillar HoC system. Here, iPSC-CMs were derived from a patient with HCM that exhibited a rare ACTN2 mutation. Treatment with diltiazem resulted in more drastic changes in the HCM patient-derived iPSC-CMs compared to the isogenic control prepared using CRISPR/Cas9. Motivated by these improvements, diltiazem was prescribed to the son and sister of the original patient, both of whom carried the same mutation. Diltiazem was shown to reduce the QT interval from 460 ms to 387 ms in the son and from 477 ms to 439 ms in the sister, implicating diltiazem as a candidate for future clinical treatment of HCM.
4.2.2 Cardiotoxicity assessment.
The most well-known families of drugs to induce cardiotoxicity are antineoplastics (e.g., anthracyclines, VEGF- and kinase inhibitors, taxanes),384 antibiotics (e.g., chloroquine), antiarrhythmics (e.g., quinidine), opiates (e.g., methadone), Ca2+ channel blockers (e.g., verapamil), and antihistamines (e.g., astemizole).356 Even after a drug makes it to market, recalls are not uncommon. Adverse cardiovascular effects are the primary cause for nearly 20% of the drugs that are recalled from the market in the US.385 Some of the most common cardiovascular complications include arrhythmia, myocyte toxicity, myocardial infarction, thrombosis, low blood pressure, and coronary artery disorders.356,384–387 Oftentimes, these detrimental effects are not seen in preclinical animal testing.10 Perhaps one of the most high-profile recent examples of this is the 2004 recall of rofecoxib, which is a non-steroidal anti-inflammatory drug (NSAID).10,388,389 A study by Graham et al. estimated that rofecoxib caused between 88
000 and 140
000 cases of serious coronary heart disease, including acute myocardial infarction requiring admission and sudden cardiac death, before being voluntarily pulled from the market in late 2004.390
4.2.2.1 Ion-channel blockers.
Numerous HoC platforms with different analytical capabilities have been developed to study drug-induced cardiotoxic side effects.126 One of the most commonly observed side effects is arrhythmia, including bradycardia, atrial fibrillation, long QT syndrome, and torsades de pointes (TdP). Many of the proarrhythmic drugs block one or more of the ion channels found in the heart (K+, Na+, Ca2+), making HoC systems well-suited to study their effects. Examples include verapamil (a Ca2+ channel blocker),168,180,182,183,360,365,366,369,391–394 quinidine (Na+-channel blocker),182,359,392,395 E-4031 (hERG K+-channel blocker),180,359,360,363,396–398 sotalol (K+-channel blocker),391 nifedipine (a Ca2+-channel blocker),305,361,396,397 ranolazine (Na+-channel blocker),396 flecainide (Na+-channel blocker),362,397,398 tetrodotoxin (Na+-channel blocker),398 ouabain (Na+/K+-ATPase blocker),359 ATX-II (Na+-channel blocker),359 and dofetilide (K+-channel blocker).359,395 The Lee group has developed various iterations of cantilever-based sensors to monitor real-time changes in contractile function after exposing cardiac tissue to different concentrations of drugs known to be cardiotoxic, including quinidine, E-4031, and verapamil.180,182,183,391,392 The cell culture surface is patterned with a series of microgrooves to promote tissue anisotropy and maturation (as discussed in section 4.1.1) and contractile function is characterized by measuring cantilever displacement (Fig. 5c). Using this technology, the group measured various forms of arrhythmia, including prolonged beating duration caused by E-4031,180,359,360,363,396–398 decreased contraction force and bradycardia after verapamil and lidocaine exposure,180,182,183,391,392 early after depolarization (EAD) and TdP at high quinidine levels.182,392 Given that arrhythmia is typically detected using electrocardiogram (ECG) and arises due to a dysfunctional conduction system, electrical measurements are well-suited to record drug-induced changes in field potential duration (FPD), which is analogous to the QT interval used in the clinic.399,400 Visone et al. developed a microelectrode chip that was benchmarked with three drugs, aspirin, sotalol, and verapamil.393 While aspirin showed no significant changes in FPD, both sotalol (a K+ channel blocker) and verapamil showed substantial changes, in agreement with other reports.394 Interestingly, after verapamil treatment, the human tissue showed a dose-dependent decrease in FPD while the rat model showed a dose-dependent increase, reiterating the importance of using human-relevant in vitro models for assessing drug toxicity.
4.2.2.2 Antineoplastics.
Certain antineoplastic therapies, while effective for cancer treatment, have also been implicated as cardiotoxic.384 Various chemotherapeutics have been studied using HoC systems, including linsitinib,358 oxaliplatin,401 and cyclophosphamide (CP).402 One of the most widely used, clinically-approved, anti-cancer drugs is the anthracycline, doxorubicin (DOX).384 Anthracycline-induced cardiotoxicity is likely caused by an increase in the generation of reactive oxygen and nitrogen species (ROS/RNS)403 Given its well-established cardiotoxicity, many HoC reports demonstrate doxorubicin as a proof-of-concept.145,162,166,236,378,394,401,404 An example from Ren et al. reported the design of a HoC platform that combined electrical stimulation with patterned PDMS microgrooves to promote tissue maturation (Fig. 5d).378 The device was used to study the effects of DOX and CP, in addition to two potentially cardioprotective supplements, ivabradine (IVA) and carbachol (CAR). Both DOX and CP appeared to be chronotropic and cytotoxic, although only DOX caused a dose-dependent decrease in cell viability. When tested with DOX, both CAR and IVA fully restored cell viability and appeared to reduce the chronotropic effects of DOX. HoC systems have also been used to evaluate the cardiotoxicity of phytochemicals with potential anti-cancer properties, such as sulforophane405,406 and thapsigargin,306,407,408 as well as kinase inhibitors,408 which have been widely explored as chemotherapeutics.409 The cardiotoxic effects of a range of kinase-inhibitors, specifically microtubule affinity regulating kinase (MARK) and checkpoint kinase (Chk), were characterized using an impedimetric assay.410 Two MARK-inhibitors synthesized by the authors caused dose-dependent decrease in beat amplitude also seen in tests with well-established microtubule targeting drugs, paclitaxel, vinblastine, nocodazole, and colchicine. The decrease in beat amplitude was determined not to be a result of cytotoxicity. Similar effects were also seen in all but two of the nine Chk-inhibitors tested with the impedimetric assay, although the half-maximal inhibitory concentration (IC50) varied more than two orders of magnitude across samples.
4.2.2.3 Antimicrobials.
Antimicrobial drugs, including antibiotics, antivirals, and antifungals, have saved countless lives since their introduction in the 20th century, perhaps even earlier.411 The cardiotoxicity of a selection of antimicrobial drugs, including ketoconazole,412 and azithromycin,413 has been evaluated using HoC systems. Of particular relevance currently are the cardiotoxic effects of various treatments for COVID-19. Xu et al. investigated 4 repurposed antiviral drugs, namely remdesivir, apilimod, ritonavir, and lopinavir, using a human EHT model.414 All 4 were found to affect Ca2+ transients and contractile function while causing broad transcriptional changes in the EHTs, although apilimod and remdesivir showed a far more altered transcriptome compared to ritonavir and lopinavir. Over 2000 potential protective drugs were also screened to reduce the remdesivir-induced cardiotoxicity. Astaxanthin was found to be an excellent protectant independent of its antioxidant activity, largely restoring cardiac function when used in tandem with remdesivir. The use of azithromycin (AZM), a microlide antibiotic, and hydroxychloroquine (HCQ), an antimalarial drug, separately or combined, was also a widely used treatment for COVID-19 (ref. 415) despite a lack of evidence supporting any clinical benefit416 and evidence suggesting they are cardiotoxic.417,418 Both Wong et al.419 and Charrez et al.413 validated the cardiotoxic properties of HCQ and AZM separately and jointly in human EHT systems. Each concluded HCQ and AZM have proarrhythmic effects and increase the action potential duration (APD), which is analogous to an increase in QT (long QT syndrome). In the context of a worldwide pandemic, like COVID-19, where time is crucial, HoC systems could be especially useful for rapid cardiotoxicity assessment of drugs under consideration for emergency use authorization.
4.2.2.4 Nanomaterials and other drugs.
The cardiotoxicity of various other classes of drugs and nanomaterials has been studied using HoC systems, including antihistamines (e.g. terfenidine,172,378 astemizole,393 and fexodenadine172), anesthetics361,371,393,397) steroids (e.g. dexamethasone144 and hydrocortisone412), anti-depressants420) pain relievers (e.g. acetaminophen166,412 and diclofenac412), myosin modulators (e.g. blebbistatin363,364 and omecamtiv mecarbil364), anti-fibrotics286) beta blockers,181,362 prokinetics,398 phosphodiesterase inhibitors,421 hormones,144 and nanomaterials.379,422 Although this is a small sampling of the many therapeutics in pre-clinical studies, clinical trials, and on the market, it highlights the broad range of drug types that could potentially be studied using HoC systems.
4.2.2.5 Medium- and high-throughput screening.
Perhaps one of the most advantageous properties of in vitro HoC platforms is the potential for higher throughput, (semi-)automated, evaluation of large libraries of therapeutics. The result is highly robust datasets with redundant measurements taken in nearly identical environmental conditions. Pointon et al. developed an improved throughput workflow combining timelapses with automated image analysis to evaluate 29 inotropic and 13 non-inotropic compounds using 3D engineered cardiac microtissues.423 The system was capable of distinguishing between positive and negative inotropes and detecting contraction changes with 80% sensitivity and 91% specificity. Similar results were seen in a separate study form Ando et al., where they evaluated a total of 60 drugs, 57 of which are known to be torsadogenic, from 4 distinct drug families using a microelectrode array (MEA) to measure changes in field potential duration.424 The developed assay was able to categorize drugs based on torsadogenic risk and when compared to the CredibleMeds database, yielded a sensitivity of 81%, specificity of 87%, and accuracy of 83%. A two-tiered assay was developed by Keung et al. to examine inotropic behavior in human ventricular-like cardiac tissue strips (hvCTS) and human ventricular-like organoid chambers (hvCOC).425 25 drugs were screened, first with hvCTS to determine any negative inotropic response, then with hvCOC to determine any positive inotropic response. The assay demonstrated accuracies of 100%, 86%, and 80% for negative, positive, and null inotropic responses, respectively. A 24-well plate-style HoC platform was developed by Lind et al., which contained microgrooved PDMS cantilevers with integrated electronic strain gauges for on-board quantification of twitch stress and beat rate (Fig. 5e).426 The system was benchmarked by measuring the dose-dependent response to 12 distinct cardiac drugs. Furthermore, the open-well format allowed for the use of Transwell® inserts to model the pharmacological response to drug diffusing through an endothelial barrier, which was found to prolong the time to total cardiac failure compared to a direct exposure.
5 Heart-on-a-chip as part of multi-organ-on-a-chip platforms
Up to this point, we have discussed the heart and HoC systems as an isolated organ. However, in reality the human body exists as a complex interplay between its various organs. An advantage of organ-on-a-chip platforms is the ability to integrate two or more organs to study a particular organ–organ coupling or create a “body-on-a-chip”.427 This is especially relevant for drug and therapeutic testing, where a detailed understanding of the pharmacokinetics and pharmacodynamics could lead to significant improvements in drug development and clinical trials.13,81
Two of the most common organs affected by drug-induced adverse side effects are the heart and the liver.387 An ingested drug is first absorbed by the small intestine and reaches the liver, where it is metabolized.428 To reach a more comprehensive understanding the pharmacokinetics and pharmacodynamics of a drug and its metabolites, heart–liver-on-a-chip (HLC) systems are being developed (Fig. 6a).378,406,414,430–432 Soltantabar et al. found doxorubicin treatment to be more damaging to the heart tissue as a result of exposure to not only doxorubicin, but also its metabolite doxorubicinol, which was generated by the hepatocellular carcinoma cells (HepG2) in the chip.404,431 One advantage of this HLC platform is the lack of external pumps necessary for fluid perfusion, which reduces the overall device complexity and footprint. However, the use of murine cardiomyocytes (H9c2) and cancerous hepatocytes (HepG2) could lead to different metabolic and pharmacological behavior than that seen with human-derived CMs and normal hepatocytes. To address this discrepancy, Lee-Montiel et al. developed an isogenic HLC that utilized hiPSC-CMs and hiPSC-hepatocytes derived from a healthy adult.429 One challenge associated with HLCs is the need for a common culture medium that supports both organ chips. The authors confirmed the liver culture medium to be compatible with the cardiac chip. The multi-organ platform was then used to study drug–drug interaction between cisapride, a gastroprokinetic metabolized by the cytochrome P450 enzyme CYP3A4, and ketoconazole, an antifungal that inhibits CYP3A4. The inherent cardiotoxic effects of cisapride were minimized if cisapride was first passed through the liver tissue before reaching the heart, due to the metabolism of cisapride into non-arrhythmogenic norcisapride. However, the addition of ketoconazole, which inhibits the metabolism of cisapride, resulted in a prolongation of the APD, analogous to clinically observed long QT syndrome. Pires de Mello et al. developed a HLC with an added skin surrogate to study common topical pharmaceuticals, including ketoconazole, diclofenac, acetaminophen, and hydrocortisone (Fig. 6b).412
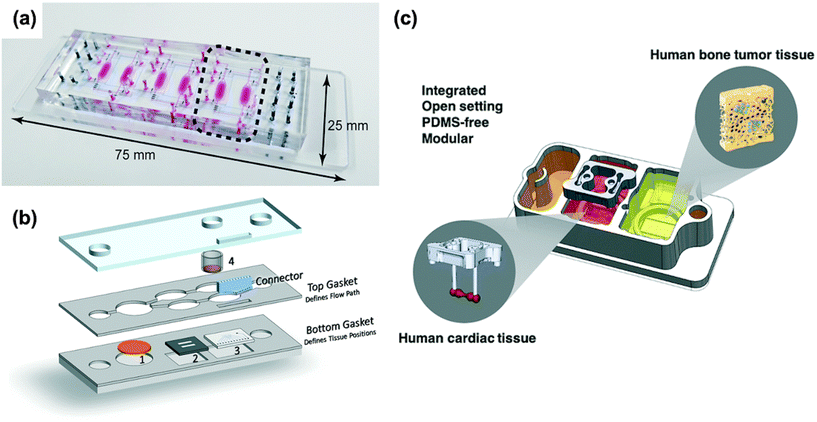 |
| Fig. 6 HoCs as part of multi-OoCs. a) An image of a heart-liver-on-a-chip (HLC) system with three independent co-culture regions on the same chip (reproduced from ref. 431 under Creative Commons 3.0 (CC BY 3.0)). b) A multi-layer HLC comprised of a bottom layer containing the tissues (1 = liver, 2 = cardiomyocytes on a cantilever array, 3 = cardiomyocytes on a microelectrode array, and 4 = skin), middle layer for fluid manipulation, and top layer with access ports (reproduced from ref. 411 with permission from the Royal Society of Chemistry). c) A modular heart–cancer-on-a-chip system with separate chambers for cardiac tissue and Ewing sarcoma (ES) tissue, which can be connected for perfusion studies or kept isolated (reproduced from ref. 360 with permission from the Royal Society of Chem. | |
The system involved a liver module (containing primary human hepatocytes) and two CM modules (containing hiPSC-CMs), one cantilever array and one microelectrode array for mechanical and electrical characterization, respectively. The skin surrogate was crucial for slowing the delivery of the drug to the main organ chip, which better replicates clinical pharmacokinetics.
Given the well-established cardiotoxicity of many antineoplastics, heart–tumor-on-a-chip systems have been employed to study drug efficacy and cardiotoxicity in one platform. Chramiec et al. developed a PDMS-free multi-organ chip to study linsitinib, an experimental anti-cancer drug, for the treatment of Ewing sarcoma (ES), a rare form of bone cancer (Fig. 6c).360 The chip also contained an engineered cardiac tissue chamber to study drug-induced cardiotoxicity in parallel. When tested in the integrated heart–tumor chip, linsitinib (12 μmol L−1) was found to have an insignificant effect on cell viability in non-metastatic ES tissue when introduced via perfusion, in agreement with observations in clinical trials. However, direct introduction of the entire volume of linsitinib to the tumor resulted in significant reduction in cell viability, in disagreement with clinical results, which emphasizes the importance of drug perfusion in in vitro systems to better replicate clinical scenarios. Furthermore, the perfused experiment showed a slight reduction (≈11%) in beat frequency of the cardiac tissue after treatment, which is similar to clinical observations. In another report, Weng et al. developed a heart–colon cancer chip with SW620 cells to study doxorubicin and oxaliplatin efficacy and cardiotoxicity.403 Here, the tissues (tumor, endothelial cell, and cardiac) were grown in parallel channels with perpendicular capillaries connecting each channel together, rather than growing each tissue in isolated chambers. One advantage of this configuration is that all three channels can be monitored in the same microscope field of view. Furthermore, the iPSC-EC tissue served as a physiological barrier between the tumor and cardiac tissues. Both DOX and oxaliplatin demonstrated a dose-dependent inhibition of tumor growth in the chip, as well as varying degrees of cardiotoxicity. The IC50 of the spontaneous beating rate was measured to be ≈0.041 μmol L−1 for DOX and 33.9 μmol L−1 for oxaliplatin. Lee et al. also tested the efficacy and cardiotoxicity of DOX in a breast cancer (SK-BR-3)-HoC with in-line electrochemical immunosensors to quantify secreted biomarkers.162 The cardiac tissue was modelled at various stages of fibrosis to replicate breast cancer patients with pre-existing cardiac dysfunctions. DOX treatment was found to decrease beating frequency in both healthy and fibrotic cardiac tissue. Using the electrochemical immunosensors, the healthy cardiac tissue demonstrated a significant increase in troponin T (a marker of myocardial damage) production between 1 and 5 days in culture without DOX, whereas the fibrotic tissue showed no significant change. After treatment with DOX, no significant change in TnT levels were seen between days 1 and 5, while a significant decrease in (human epidermal growth factor receptor 2) HER-2 levels were seen with fibrotic tissue over the same timeline. The authors also compared DOX delivery using magnetic nanoparticles (MNP) with regular free delivery and found MNP delivery to induce less troponin T secretion while maintaining a similar reduction in breast cancer proliferation. Taken together, these examples demonstrate the potential of multi-organ chips to sufficiently model drug metabolism and explore novel therapeutic strategies under controlled and potentially isogenic conditions.
In a paper by McAleer et al., a pumpless liver–heart–tumor-on-a-chip was developed to test both cardiotoxicity and drug efficacy.432 Here, the authors included two cardiac chambers (containing hiPSC-CMs), one with thin film cantilevers and one with a microelectrode array, and two cancer chambers, all downstream of the liver chamber prepared with primary human hepatocytes. The organization of the chambers allows chemotherapeutic drugs to be introduced in a common serum-free medium and metabolized by the liver before reaching the cardiac and cancer chambers, which included breast cancer and multi-drug-resistant vulva cancer cell lines (MCF-7 and SW-962, respectively). The system showed a reduction in viability of the breast cancer cells after tamoxifen treatment with the liver chamber present, demonstrating the importance of metabolism on drug efficacy. The drug-resistant cancer cells showed a reduction in viability only after treatment with tamoxifen co-administered with verapamil. Off-target effects were observed in the cardiac modules as well, with a reduction in contractile force, conduction velocity, and beat frequency all seen despite without a significant change in cardiomyocyte viability.
6 Standardization in organ-on-a-chip systems
The microphysiological systems (MPS) community have expressed great interest in the development of standards for these platforms. Stemming from the promise of MPS to greatly improve in vitro results for toxicity and efficacy testing in drug development, the need for documentary standards (to start with) is of upmost importance in the mind of a number of (bio)pharmaceuticals, and biotechnology companies as well as suppliers of microfluidics and other device components.433,434
The push for standards is illustrated by the efforts of organizations like the Standards Coordinating Body (SCB) in the USA,14 the North American 3Rs Collaborative,435 the Centre for Alternative to Animal Testing or CAAT (Johns Hopkins University, USA and University of Konstanz, Germany),436,437 the Organ-on-a-Chip/Tissue-on-a-Chip Engineering and Efficacy Standardization Working Group (O/TEES WG, USA with collaboration from European Commission),438 CEN-CENELEC (Europe),439 European Organ-on-a-Chip Society (EurOoCS),440 and the Microfluidics Association (MFA, International),441 who have been working to get consensus for specific standard processes, measurements and methods. All these organizations are working with stakeholders from the academia, industry, government research labs and regulatory agencies in the development of guidelines and standards for MPS as well as conferences and publications to further disseminate the work that has been carried out by this community. Although all these efforts are underway, there has always been a concern in certain sectors of the scientific/technology community regarding the possibility of innovation being hindered by the development of standards. However, the development of standards provides the basis for common language and measurement tools that ultimately allow for further advancements to be possible. The areas for standardization discussed below provide a framework for all MPS, but when deciding what specific standards to develop, some of the areas can apply to all MPS and some are more specific. For example, the engineering aspects, combined with protocols, for some of those future standards will likely be applicable to all or almost all MPS. Aspects like flow conditions/control is one that can apply to many MPS uniformly since what could really change from organ to organ is the range at which flow should be validated. The same way other standards could be applied uniformly, and others will need to be more specific. To make these efforts worthwhile, the community is taking this into consideration and whenever possible standards will be developed in a way that could be applicable as broadly as possible.
The first efforts to develop standards for MPS and related microfluidic devices have so far produced the first three standards in the form of the ISO 22916:2022, “Microfluidic devices – interoperability requirements for dimensions, connections and initial device classification”,442 the ISO 10991:2023, “Microfluidics – Vocabulary”,443 and the ASTM International standard titled “Standard Terminology Relating to Microphysiological Systems”, designation F3570-22.14 However, debates about the next aspects to be standardized are part of the current conversations between and within the different standard developing organizations. Heart MPS is currently being discussed at least by the SCB and O/TEES WG. For each organ model there are numerous areas for the development of standards, and the heart model is no exception. In general, we can think that for all organ models, including Heart MPS, there are three major areas where the development of (documentary) standards will be needed. Those areas are: 1) engineering aspects of microdevices and their components (i.e., the hardware's capabilities and performance); 2) the microenvironment (i.e., the cell adhesive extracellular materials surrounding the cells to provide signals for cell growth, proliferation and more); and 3) protocols to generate functional cells (e.g., mature cardiomyocytes) and their characterization outside and in the microdevice. These are basic areas to establish a baseline of normal cell behavior in the MPS as well as under different perturbations during the assessment of toxicity effects and efficacy of drugs.
In terms of standardization of engineering aspects or hardware capabilities there are several items that apply to Heart MPS as well as to a few other models. For example, heart MPS will require standardized methods to assess the capabilities of integrated components for sensing and actuation. Specifically, it should be considered the development of protocols to reliably measure the mechanical stretching of such components. There should also be considered the development of reliable methods to measure the heart cells beating behavior (e.g., amplitude and frequency). Also, standardization of engineering aspects of the MPS that can modulate the cell state while in the microsystem. One example of engineering aspects to consider is flow control within the system. This parameter is critical since flow affects shear stress, which can exert differences in gene expression in cardiomyocytes, thus producing impaired function and cardiomyopathies.444 The microenvironment is an area under a lot of active work to define the optimum ECM and other conditions for cell differentiation and maturation. Components like laminins (e.g., 111, 521 and 511), collagen (e.g., collagen IV), fibronectin and a few other ECM components will need to be part of a combination of standard materials needed to produce a reliable and reproducible Heart MPS microenvironment.444–446 In addition, scaffolds for 3D cultures would need to be considered as part of the microenvironment.447 Lastly, protocols to generate functional cells will need to be developed. Those protocols will need to consider the two previous areas discussed above (i.e., engineering aspects and microenvironment) to provide an informed set of procedures for consistently obtaining healthy and mature cardiomyocytes and other cells associated to the organ. Among some of the parameters to be considered are oxygen partial pressure and pH.448 Currently, the O/TEES WG is considering cell maturity, beat frequency, conduction velocity, force, and ejection fraction as markers for heart cells behavior and functionality within the MPS. These parameters refer to protocols to assess functional cells, mainly physical measurements of cell processes. However, other efforts would be expected to take a different route and investigate other parameters such as ATP content, cardiac troponin and chemical markers for cardiac cells function (i.e., protocols focusing on biochemical assessment).
7 Conclusions and outlook
The heart is responsible for efficiently delivering blood, nutrients, and oxygen to tissue and organs throughout the body. Given its outsize role in regulating physiological homeostasis, there is great interest in holistically understanding cardiovascular physiology and pathophysiology. Historically, improving this understanding has been challenging because of a lack of sufficiently relevant human models. HoC systems have advanced tremendously over the past decade, coinciding with improvements to tissue engineering, gene editing, and especially the advent of human-induced pluripotent stem cells. These advancements have brought about new applications for HoCs as discussed in this review, and with that, new companies working to commercialize this technology. Nonetheless, significant work remains to be done before HoCs, and OoCs more broadly, become commonly used in pre-clinical testing.
One substantial hurdle that needs to be addressed is the challenge of tissue immaturity in these systems. This is especially a problem for stem-cell derived cardiac cells, which typically express a fetal-like phenotype. As discussed in section 4.1.1, numerous methods for improving maturity have been reported, including mechanical conditions, electrical stimulation, biochemical treatment, and cell co-culture. As a result, more human-relevant assessments of disease progression and drug toxicity have been made. We expect to see continued work in the area of tissue maturation as HoCs advance along the technological development pathway. Despite advances in tissue maturation, the ability to holistically model the comorbidities that often accompany cardiovascular dysfunction in adults is still in its infancy. Another area that remains a bottleneck to widespread commercialization is chip material. PDMS is the most widely used material for fabricating microfluidic chips for OoCs. Although PDMS has advantages in terms of gas permeability, biocompatibility, optical transparency, and ease of patterning, it suffers from a number of key drawbacks that could ultimately limit its use as OoC technology progresses. Without any form of automation, the assembly of PDMS OoCs can be tedious and artisanal. Another key issue particularly relevant for drug development is the absorption of many small molecules in PDMS, which can skew toxicity and efficacy results if not addressed and/or accounted for.88 Furthermore, PDMS is relatively expensive when compared to other materials currently used in cell biology, such as polystyrene, poly(methyl methacrylate), and other polymers. These materials are more conducive to industrial-scale manufacturing processes, such as 3D printing and injection molding. Until OoCs can be quickly and inexpensively prepared using large-scale manufacturing methods, commercialization of the technology will likely be limited.
We believe that standardization will play a critical role in many of the areas associated with OoCs, making widespread adoption of OoCs more appealing. From a production and design perspective, standardized materials, geometries, and treatments will enable easier cross-platform comparison. Cell biology has benefited tremendously from the geometric standardization of tissue culture vessels, allowing for more universal protocols and viable high-throughput instrumentation. Reporting of a common set of metrics will also accelerate progress and make commercialization easier. By establishing a common set of metrics, not only can researchers compare their work to that of others, but also approval from regulatory agencies should become less cumbersome as a result. Rigorous methods for benchmarking results from OoCs with animal studies will most likely lead to faster and broader acceptance of a given OoC model as a comparable, if not better, alternative to the equivalent animal model.
Regulatory agencies, such as the US Food and Drug Administration (FDA), have already lifted the requirement of animal testing when seeking approval of a new drug, which opens the door for new and novel alternatives, including OoCs, to replace them. As a result, one application in which OoCs could find widespread acceptance is in the re-purposing or accelerated approval of drugs and therapeutics during public health emergencies, where animal studies and clinical trials may be too time-consuming. The COVID-19 pandemic is a recent example where numerous existing drugs, such as hydroxychloroquine and azithromycin, were claimed to treat or manage COVID-19. However, these claims were ultimately nullified after users experienced a number of adverse side effects, including kidney injury and liver damage.449 While these instances are not entirely avoidable, OoCs could help improve and accelerate drug re-purposing and dissemination of information during rapidly changing situations, such as a global pandemic or other public health emergency.
Finally, we expect to see the incorporation of on-board analytical tools increase as OoCs become more sophisticated. Biosensors, including electrochemical and optical, will become more heavily integrated in OoC systems as real-time understanding of tissue behavior is needed and traditional end-point assays lack the ability to provide the necessary information. Real-time measurements are especially important for dynamic samples, such as cardiac tissue, which can change behavior (e.g., beating frequency) in a matter of seconds. Moreover, integration of auxiliary sensors, such as temperature and pH, among others, will assist in standardization and improve data robustness. As costs decrease, and sensor integration and on-board analysis improves, OoC systems could see more decentralized usage outside of a clinical environment in more resource-limited settings.
We envision marked advancements to OoCs in the near future, driven by increased human relevance, decreased costs, and robust standardization. As tissue engineering, scalable manufacturing, gene editing and analytical methods improve, more complex models can be realized leading to a deeper and more personalized mechanistic insight of cardiophysiology, disease, and toxicity. As models improve, we expect better agreement between results obtained from OoCs and clinical observations, leading to more widespread adoption of OoC models as a suitable replacement for animal models. While widespread adoption and commercialization will take time, it is likely that OoCs will find use in certain applications sooner than others. For example, rapid drug screening during public health emergencies or rare disease treatment where there may otherwise be difficult in establishing a trial cohort are just some of the applications that could benefit from prompt adoption of OoCs. Overall, OoCs are only in their infancy and are expected to cause a paradigm shift in the way pre-clinical and clinical assessments of disease treatment and drug efficacy and toxicity are carried out.
Author contributions
D. B. and D. R. R. both contributed to literature review, writing, and editing.
Conflicts of interest
There are no conflicts to declare.
Acknowledgements
D. B. would like to acknowledge support from the NIST-NRC (National Research Council) Postdoctoral Fellowship. D. R. R. acknowledges support from NIST-on-a-Chip Initiative funding.
Notes and references
-
World Health Organization, Cardiovascular diseases, 2023, https://www.who.int/health-topics/cardiovascular-diseases#tab=tab_1.
- C. W. Tsao, A. W. Aday, Z. I. Almarzooq, A. Alonso, A. Z. Beaton, M. S. Bittencourt, A. K. Boehme, A. E. Buxton, A. P. Carson, Y. Commodore-Mensah, M. S. Elkind, K. R. Evenson, C. Eze-Nliam, J. F. Ferguson, G. Generoso, J. E. Ho, R. Kalani, S. S. Khan, B. M. Kissela, K. L. Knutson, D. A. Levine, T. T. Lewis, J. Liu, M. S. Loop, J. Ma, M. E. Mussolino, S. D. Navaneethan, A. M. Perak, R. Poudel, M. Rezk-Hanna, G. A. Roth, E. B. Schroeder, S. H. Shah, E. L. Thacker, L. B. Vanwagner, S. S. Virani, J. H. Voecks, N. Y. Wang, K. Yaffe and S. S. Martin, Circulation, 2022, 145, E153–E639 CrossRef PubMed.
-
T. Gaziano, K. S. Reddy, F. Paccaud, S. Horton and V. Chaturvedi, Disease Control Priorities in Developing Countries, The International Bank for Reconstruction and Development/The World Bank, Washington D.C., 2nd edn, 2006, ch. 33, vol. 2, pp. 645–662 Search PubMed.
- J. L. Anderson and D. A. Morrow, N. Engl. J. Med., 2017, 376, 2053–2064 CrossRef CAS PubMed.
- G. Heusch, Nat. Rev. Cardiol., 2020, 17, 773–789 CrossRef PubMed.
- G. Heusch, Circ. Res., 2017, 120, 1477–1486 CrossRef CAS PubMed.
- M. L. Lindsey, Z. Kassiri, J. A. Virag, L. E. De Castro Brás and M. Scherrer-Crosbie, Am. J. Physiol., 2018, 314, H733–H752 Search PubMed.
- E. Karbassi, A. Fenix, S. Marchiano, N. Muraoka, K. Nakamura, X. Yang and C. E. Murry, Nat. Rev. Cardiol., 2020, 17, 341–359 CrossRef PubMed.
- G. A. Van Norman, JACC Basic Transl. Sci., 2016, 1, 170–179 CrossRef PubMed.
- G. A. Van Norman, JACC Basic Transl. Sci., 2019, 4, 845–854 CrossRef PubMed.
- G. A. Van Norman, JACC Basic Transl. Sci., 2019, 4, 428–437 CrossRef PubMed.
- V. B. Siramshetty, J. Nickel, C. Omieczynski, B. O. Gohlke, M. N. Drwal and R. Preissner, Nucleic Acids Res., 2016, 44, D1080–D1086 CrossRef CAS PubMed.
- D. E. Ingber, Nat. Rev. Genet., 2022, 23, 467–491 CrossRef CAS PubMed.
-
American National Standards Institute, Standard Terminology Relating to Microphysiological Systems, American national standards institute technical report, 2022 Search PubMed.
- N. N. Khalil and M. L. McCain, Front. Cardiovasc. Med., 2021, 8, 709871 CrossRef CAS PubMed.
- R. X. Z. Lu, Y. Zhao and M. Radisic, Bioeng. Transl. Med., 2023, 1–18 Search PubMed.
- D. N. Tavakol, S. Fleischer and G. Vunjak-Novakovic, Cell Stem Cell, 2021, 28, 993–1015 CrossRef CAS PubMed.
- A. Polini, L. Prodanov, N. S. Bhise, V. Manoharan, M. R. Dokmeci and A. Khademhosseini, Expert Opin. Drug Discovery, 2014, 9, 335–352 CrossRef CAS PubMed.
- E. R. Rodriguez and C. D. Tan, Prog. Cardiovasc. Dis., 2017, 59, 327–340 CrossRef PubMed.
- W. Hasan, Organogenesis, 2013, 9, 176–193 CrossRef PubMed.
- L. Tsun-cheung Chow, S. Sau-ming Chow, R. H. Anderson and J. A. Gosling, J. Anat., 1995, 187, 107–114 Search PubMed.
- S. J. Crick, R. H. Anderson, S. Y. Ho and M. N. Sheppard, J. Anat., 1999, 195, 359–373 CrossRef CAS PubMed.
- K. Marron, J. Wharton, M. N. Sheppard, S. Gulbenkian, D. Royston, M. H. Yacoub, R. H. Anderson and J. M. Polak, Cardiovasc. Res., 1994, 28, 1490–1499 CrossRef CAS PubMed.
- L. Gordon, J. M. Polak, G. J. Moscoso, A. Smith, D. M. Kuhn and J. Wharton, J. Anat., 1993, 183, 131–140 CAS.
- J. Wharton, J. M. Polak, L. Gordon, N. R. Banner, D. R. Springall, M. Rose, A. Khagani, J. Wallwork, M. H. Yacoub, H. Hospital and M. Harefield, Circ. Res., 1990, 66, 900–912 CrossRef CAS PubMed.
- M. D. Tallquist, Cold Spring Harbor Perspect. Biol., 2020, 12, a037184 CrossRef CAS PubMed.
- C. A. Isidoro and J. F. Deniset, Can. J. Cardiol., 2023, 39, 1078–1089 CrossRef PubMed.
- M. J. Ivey and M. D. Tallquist, Circ. J., 2016, 80, 2269–2276 CrossRef CAS PubMed.
- P. Snider, K. N. Standley, J. Wang, M. Azhar, T. Doetschman and S. J. Conway, Circ. Res., 2009, 105, 934–947 CrossRef CAS PubMed.
- J. Jin, Y. Jiang, S. Chakrabarti and Z. Su, Front. Immunol., 2022, 13, 963444 CrossRef CAS PubMed.
- W. P. Lafuse, D. J. Wozniak and M. V. Rajaram, Cell, 2021, 10, 1–27 Search PubMed.
- M. Hulsmans, F. Sam and M. Nahrendorf, J. Mol. Cell. Cardiol., 2016, 93, 149–155 CrossRef CAS PubMed.
- V. Talman and R. Kivelä, Front. Cardiovasc. Med., 2018, 5, 101 CrossRef PubMed.
- H. Zhang, K. O. Lui and B. Zhou, Circ. Res., 2018, 122, 774–789 CrossRef CAS PubMed.
- E. Warren and S. Gerecht, Vasc. Biol., 2023, 5, e220021 CAS.
- G. Iacobellis and A. C. Bianco, Trends Endocrinol. Metab., 2011, 22, 450–457 CrossRef CAS PubMed.
- J. Liu, C. S. Fox, D. M. Hickson, D. Sarpong, L. Ekunwe, W. D. May, G. W. Hundley, J. J. Carr and H. A. Taylor, Diabetes Care, 2010, 33, 1635–1639 CrossRef CAS PubMed.
- M. Litviňuková, C. Talavera-López, H. Maatz, D. Reichart, C. L. Worth, E. L. Lindberg, M. Kanda, K. Polanski, M. Heinig, M. Lee, E. R. Nadelmann, K. Roberts, L. Tuck, E. S. Fasouli, D. M. DeLaughter, B. McDonough, H. Wakimoto, J. M. Gorham, S. Samari, K. T. Mahbubani, K. Saeb-Parsy, G. Patone, J. J. Boyle, H. Zhang, H. Zhang, A. Viveiros, G. Y. Oudit, O. A. Bayraktar, J. G. Seidman, C. E. Seidman, M. Noseda, N. Hubner and S. A. Teichmann, Nature, 2020, 588, 466–472 CrossRef PubMed.
- N. R. Tucker, M. Chaffin, S. J. Fleming, A. W. Hall, V. A. Parsons, K. C. Bedi, A. D. Akkad, C. N. Herndon, A. Arduini, I. Papangeli, C. Roselli, F. Aguet, S. H. Choi, K. G. Ardlie, M. Babadi, K. B. Margulies, C. M. Stegmann and P. T. Ellinor, Circulation, 2020, 142, 466–482 CrossRef CAS PubMed.
- A. L. Koenig, I. Shchukina, J. Amrute, P. S. Andhey, K. Zaitsev, L. Lai, G. Bajpai, A. Bredemeyer, G. Smith, C. Jones, E. Terrebonne, S. L. Rentschler, M. N. Artyomov and K. J. Lavine, Nature Cardiovascular Research, 2022, 1, 263–280 CrossRef PubMed.
- Heart icon by Servier, https://smart.servier.com/, is licensed under CC-BY 3.0 Unported, A copy of the license can be found at the following link: https://creativecommons.org/licenses/by/3.0/.
- A copy of the license can be found at the following link: https://creativecommons.org/licenses/by/4.0/.
- Credit to Servier Medical Art and DBCLS. Use does not suggest or imply endorsement.
-
J. R. Levick, An Introduction to Cardiovascular Physiology, Butterworths, 1st edn, 1991 Search PubMed.
-
A. M. Katz, Physiology of the Heart, Wolters Kluwer, 5th edn, 2011 Search PubMed.
-
A. Arackal and K. Alsayouri, Histology, Heart, 2023, https://www.ncbi.nlm.nih.gov/books/NBK545143/ Search PubMed.
- T. J. Streef and A. M. Smits, Front. Cardiovasc. Med., 2021, 8, 750243 CrossRef CAS PubMed.
- A. Wessels and J. M. Pérez-Pomares, Anat. Rec., Part A, 2004, 276, 43–57 CrossRef CAS PubMed.
- A. M. Smits, E. Dronkers and M. J. Goumans, Pharmacol. Res., 2018, 127, 129–140 CrossRef CAS PubMed.
- P. Quijada, M. A. Trembley and E. M. Small, Circ. Res., 2020, 126, 377–394 CrossRef CAS PubMed.
- R. H. Whitaker, Medicine, 2010, 38, 333–335 CrossRef.
- M. Misfeld and H. H. Sievers, Philos. Trans. R. Soc., B, 2007, 362, 1421–1436 CrossRef PubMed.
- L. M. Monteiro, F. Vasques-Nóvoa, L. Ferreira, P. Pinto-Do-ó and D. S. Nascimento, npj Regener. Med., 2017, 2, 1–13 CrossRef PubMed.
- K. Gaengel, G. Genové, A. Armulik and C. Betsholtz, Arterioscler., Thromb., Vasc. Biol., 2009, 29, 630–638 CrossRef CAS PubMed.
- G. Iacobellis, Nat. Rev. Cardiol., 2022, 19, 593–606 CrossRef CAS PubMed.
- J. A. Armour, Am. J. Physiol., 2004, 287, R262–R271 CAS.
- F. J. Carrillo-Salinas, N. Ngwenyama, M. Anastasiou, K. Kaur and P. Alcaide, Am. J. Pathol., 2019, 189, 1482–1494 CrossRef PubMed.
- J. Piquereau and R. Ventura-Clapier, Front. Physiol., 2018, 9, 959 CrossRef PubMed.
- R. E. Ahmed, T. Anzai, N. Chanthra and H. Uosaki, Front. Cell Dev. Biol., 2020, 8, 178 CrossRef PubMed.
- Y. Guo and W. T. Pu, Circ. Res., 2020, 126, 1086–1106 CrossRef CAS PubMed.
- S. E. Lindsey, J. T. Butcher and H. C. Yalcin, Front. Physiol., 2014, 5, 318 Search PubMed.
- A. C. Gittenberger-De Groot, M. M. Bartelings, M. C. Deruiter and R. E. Poelmann, Pediatr. Res., 2005, 57, 169–176 CrossRef PubMed.
- F. Li, X. Wang, J. M. Capasso and A. M. Gerdes, J. Mol. Cell. Cardiol., 1996, 28, 1737–1746 CrossRef CAS PubMed.
- M. Maillet, J. H. Van Berlo and J. D. Molkentin, Nat. Rev. Mol. Cell Biol., 2013, 14, 38–48 CrossRef CAS PubMed.
- C. Robertson, D. D. Tran and S. C. George, Stem Cells, 2013, 31, 829–837 CrossRef CAS PubMed.
- G. J. Scuderi and J. Butcher, Front. Cell Dev. Biol., 2017, 5, 50 CrossRef PubMed.
- M. Gherghiceanu, L. Barad, A. Novak, I. Reiter, J. Itskovitz-Eldor, O. Binah and L. M. Popescu, J. Cell. Mol. Med., 2011, 15, 2539–2551 CrossRef CAS PubMed.
- M. Snir, I. Kehat, A. Gepstein, R. Coleman, J. Itskovitz-Eldor, E. Livne and L. Gepstein, Am. J. Physiol., 2003, 285, 2355–2363 Search PubMed.
- J. J. Smolich, Reprod., Fertil. Dev., 1995, 7, 451–461 CrossRef CAS PubMed.
- X. Yang, L. Pabon and C. E. Murry, Circ. Res., 2014, 114, 511–523 CrossRef CAS PubMed.
- S. D. Bird, P. A. Doevendans, M. A. Van Rooijen, A. Brutel De La Riviere, R. J. Hassink, R. Passier and C. L. Mummery, Cardiovasc. Res., 2003, 58, 423–434 CrossRef CAS PubMed.
- S. D. Lundy, W. Z. Zhu, M. Regnier and M. A. Laflamme, Stem Cells Dev., 2013, 22, 1991–2002 CrossRef CAS PubMed.
- C. A. Opitz, M. C. Leake, I. Makarenko, V. Benes and W. A. Linke, Circ. Res., 2004, 94, 967–975 CrossRef CAS PubMed.
- S. Lahmers, Y. Wu, D. R. Call, S. Labeit and H. Granzier, Circ. Res., 2004, 94, 505–513 CrossRef CAS PubMed.
- L. Saggin, L. Gorza, S. Ausoni and S. Schiaffino, J. Biol. Chem., 1989, 264, 16299–16302 CrossRef CAS PubMed.
- N. M. Hunkeler, J. Kullman and A. M. Murphy, Circ. Res., 1991, 69, 1409–1414 CrossRef CAS PubMed.
- Q. X. Xiu, Y. S. Set, W. Sun and R. Zweigerdt, Stem Cells, 2009, 27, 2163–2174 CrossRef PubMed.
- E. Drouin, F. Charpentier, C. Gauthier, K. Laurent and H. L. Marec, J. Am. Coll. Cardiol., 1995, 26, 185–192 CrossRef CAS PubMed.
- T. J. Kolanowski, C. L. Antos and K. Guan, Int. J. Cardiol., 2017, 241, 379–386 CrossRef PubMed.
- Q. Wu, J. Liu, X. Wang, L. Feng, J. Wu, X. Zhu, W. Wen and X. Gong, J. Geophys. Res. Planets, 2020, 19, 1–19 Search PubMed.
- B. Zhang, A. Korolj, B. F. L. Lai and M. Radisic, Nat. Rev. Mater., 2018, 3, 257–278 CrossRef.
- Q. Yang, Z. Xiao, X. Lv, T. Zhang and H. Liu, Int. J. Bioprint., 2021, 7, 54–70 CAS.
- C. F. Mandenius, Bioengineering, 2018, 5, 56 CrossRef CAS PubMed.
- A. V. Nielsen, M. J. Beauchamp, G. P. Nordin and A. T. Woolley, Annu. Rev. Anal. Chem., 2020, 13, 45–65 CrossRef PubMed.
- G. M. Whitesides, Nature, 2006, 442, 368–373 CrossRef CAS PubMed.
- B. Xiong, K. Ren, Y. Shu, Y. Chen, B. Shen and H. Wu, Adv. Mater., 2014, 26, 5525–5532 CrossRef CAS PubMed.
- S. N. Bhatia and D. E. Ingber, Nat. Biotechnol., 2014, 32, 760–772 CrossRef CAS PubMed.
- M. Radisic and P. Loskill, ACS Biomater. Sci. Eng., 2021, 7, 2861–2863 CrossRef CAS PubMed.
- S. B. Campbell, Q. Wu, J. Yazbeck, C. Liu, S. Okhovatian and M. Radisic, ACS Biomater. Sci. Eng., 2021, 7, 2880–2899 CrossRef CAS PubMed.
- E. Sano, C. Mori, N. Matsuoka, Y. Ozaki, K. Yagi, A. Wada, K. Tashima, S. Yamasaki, K. Tanabe, K. Yano and Y. S. Torisawa, Micromachines, 2019, 10, 793 CrossRef PubMed.
- C. M. Leung, P. de Haan, K. Ronaldson-Bouchard, G. A. Kim, J. Ko, H. S. Rho, Z. Chen, P. Habibovic, N. L. Jeon, S. Takayama, M. L. Shuler, G. Vunjak-Novakovic, O. Frey, E. Verpoorte and Y. C. Toh, Nat. Rev. Methods Primers, 2022, 2, 33 CrossRef CAS.
- S. M. Scott and Z. Ali, Micromachines, 2021, 12, 319 CrossRef PubMed.
- D. Qin, Y. Xia and G. M. Whitesides, Nat. Protoc., 2010, 5, 491–502 CrossRef CAS PubMed.
- D. G. Kasi, M. N. de Graaf, P. A. Motreuil-Ragot, J. P. M. Frimat, M. D. Ferrari, P. M. Sarro, M. Mastrangeli, A. M. van den Maagdenberg, C. L. Mummery and V. V. Orlova, Micromachines, 2022, 13, 49 CrossRef PubMed.
- H. Shadpour and S. A. Soper, Anal. Chem., 2006, 78, 3519–3527 CrossRef CAS PubMed.
- Q. Mei, Z. Xia, F. Xu, S. A. Soper and Z. H. Fan, Anal. Chem., 2008, 80, 6045–6050 CrossRef CAS PubMed.
- R. Amin, S. Knowlton, A. Hart, B. Yenilmez, F. Ghaderinezhad, S. Katebifar, M. Messina, A. Khademhosseini and S. Tasoglu, Biofabrication, 2016, 8, 022001 CrossRef PubMed.
- F. Mayer, S. Richter, J. Westhauser, E. Blasco, C. Barner-Kowollik and M. Wegener, Sci. Adv., 2019, 5, eaau9160 CrossRef CAS PubMed.
- C. R. Reedy, C. W. Price, J. Sniegowski, J. P. Ferrance, M. Begley and J. P. Landers, Lab Chip, 2011, 11, 1603–1611 RSC.
- P. Jankowski, D. Ogonczyk, A. Kosinski, W. Lisowski and P. Garstecki, Lab Chip, 2011, 11, 748–752 RSC.
- X. Yao, Z. Chen and G. Chen, Electrophoresis, 2009, 30, 4225–4229 CrossRef CAS PubMed.
- F. Darain, K. L. Gan and S. C. Tjin, Biomed. Microdevices, 2009, 11, 653–661 CrossRef CAS PubMed.
- D. Ogonczyk, J. Wgrzyn, P. Jankowski, B. Dabrowski and P. Garstecki, Lab Chip, 2010, 10, 1324–1327 RSC.
- E. W. Young, E. Berthier and D. J. Beebe, Anal. Chem., 2013, 85, 44–49 CrossRef CAS PubMed.
- E. Berthier, E. W. Young and D. Beebe, Lab Chip, 2012, 12, 1224–1237 RSC.
- E. K. Sackmann, A. L. Fulton and D. J. Beebe, Nature, 2014, 507, 181–189 CrossRef CAS PubMed.
- K. Domansky, D. C. Leslie, J. McKinney, J. P. Fraser, J. D. Sliz, T. Hamkins-Indik, G. A. Hamilton, A. Bahinski and D. E. Ingber, Lab Chip, 2013, 13, 3956–3964 RSC.
- P. Gu, T. Nishida and Z. H. Fan, Electrophoresis, 2014, 35, 289–297 CrossRef CAS PubMed.
- N. Bhattacharjee, A. Urrios, S. Kang and A. Folch, Lab Chip, 2016, 16, 1720–1742 RSC.
- D. Therriault, S. R. White and J. A. Lewis, Nat. Mater., 2003, 2, 265–271 CrossRef CAS PubMed.
- A. K. Au, W. Huynh, L. F. Horowitz and A. Folch, Am. Ethnol., 2016, 128, 3926–3946 Search PubMed.
- J. Huang, Q. Feng, L. Wang and B. Zhou, Front. Cell Dev. Biol., 2021, 9, 655161 CrossRef PubMed.
- H. Savoji, M. H. Mohammadi, N. Rafatian, M. K. Toroghi, E. Y. Wang, Y. Zhao, A. Korolj, S. Ahadian and M. Radisic, Biomaterials, 2019, 198, 3–26 CrossRef CAS PubMed.
- S.-i. Nishikawa, R. A. Goldstein and C. R. Nierras, Nat. Rev. Mol. Cell Biol., 2008, 9, 725–729 CrossRef CAS PubMed.
- P. W. Burridge, E. Matsa, P. Shukla, Z. C. Lin, J. M. Churko, A. D. Ebert, F. Lan, S. Diecke, B. Huber, N. M. Mordwinkin, J. R. Plews, O. J. Abilez, B. Cui, J. D. Gold and J. C. Wu, Nat. Methods, 2014, 11, 855–860 CrossRef CAS PubMed.
- D. M. Lyra-Leite, O. Gutierrez-Gutierrez, M. Wang, Y. Zhou, L. Cyganek and P. W. Burridge, STAR Protoc., 2022, 3, 101560 CrossRef CAS PubMed.
- A. Kahn-Krell, D. Pretorius, B. Guragain, X. Lou, Y. Wei, J. Zhang, A. Qiao, Y. Nakada, T. J. Kamp, L. Ye and J. Zhang, Front. Bioeng. Biotechnol., 2022, 10, 908848 CrossRef PubMed.
- A. J. Rufaihah, N. F. Huang, J. Kim, J. Herold, K. S. Volz, T. S. Park, J. C. Lee, E. T. Zambidis, R. Reijo-Pera and J. P. Cooke, Am. J. Transl. Res., 2013, 5, 21–35 CAS.
- S. Soussi, L. Savchenko, D. Rovina, J. S. Iacovoni, A. Gottinger, M. Viallettes, J. M. Pioner, A. Farini, S. Mallia, M. Rabino, G. Pompilio, A. Parini, O. Lairez, A. Gowran and N. Pizzinat, Biol. Direct, 2023, 18, 41 CrossRef CAS PubMed.
- Z. Wang, S. J. Lee, H. J. Cheng, J. J. Yoo and A. Atala, Acta Biomater., 2018, 70, 48–56 CrossRef CAS PubMed.
- S. Rahmani Dabbagh, M. Rezapour Sarabi, M. T. Birtek, N. Mustafaoglu, Y. S. Zhang and S. Tasoglu, Aggregate, 2023, 4, e197 CrossRef CAS.
- Y. Jiang, P. Park, S. M. Hong and K. Ban, Mol. Cells, 2018, 41, 613–621 CAS.
- Y. Wang, M. Yu, K. Hao, W. Lei, M. Tang and S. Hu, Stem Cell Rev. Rep., 2022, 18, 2966–2981 CrossRef CAS PubMed.
- R. Gaetani, E. A. Zizzi, M. A. Deriu, U. Morbiducci, M. Pesce and E. Messina, Front. Cell Dev. Biol., 2020, 8, 334 CrossRef PubMed.
- S. F. Badylak, Biomaterials, 2007, 28, 3587–3593 CrossRef CAS PubMed.
- Y. K. Kurokawa and S. C. George, Adv. Drug Delivery Rev., 2016, 96, 225–233 CrossRef CAS PubMed.
- E. Moradi, S. Jalili-Firoozinezhad and M. Solati-Hashjin, Acta Biomater., 2020, 116, 67–83 CrossRef CAS PubMed.
- L. A. Osório, E. Silva and R. E. Mackay, Bioengineering, 2021, 8, 113 CrossRef PubMed.
- H. Kutluk, E. E. Bastounis and I. Constantinou, Adv. Healthcare Mater., 2023, 12, 2203256 CrossRef CAS PubMed.
- S. Deir, Y. Mozhdehbakhsh Mofrad, S. Mashayekhan, A. Shamloo and A. Mansoori-Kermani, Talanta, 2024, 266, 124901 CrossRef CAS PubMed.
- M. T. Lam and M. T. Longaker, J. Tissue Eng. Regener. Med., 2012, 6, s80–s86 CrossRef PubMed.
- Certain commercial equipment, instruments, or materials are identified in this paper to foster understanding. Such identification does not imply recommendation or endorsement by the National Institute of Standards and Technology, nor does it imply that the materials or equipment is necessarily the best available for the purpose.
- A. M. Barnes, T. B. Holmstoen, A. J. Bonham and T. J. Rowland, Bioengineering, 2022, 9, 1–17 CrossRef PubMed.
- T. C. Sung, C. H. Liu, W. L. Huang, Y. C. Lee, S. S. Kumar, Y. Chang, Q. D. Ling, S. T. Hsu and A. Higuchi, Biomater. Sci., 2019, 7, 5467–5481 RSC.
- L. Yap, J. W. Wang, A. Moreno-Moral, L. Y. Chong, Y. Sun, N. Harmston, X. Wang, S. Y. Chong, M. K. Öhman, H. Wei, R. Bunte, S. Gosh, S. Cook, O. Hovatta, D. P. de Kleijn, E. Petretto and K. Tryggvason, Cell Rep., 2019, 26, 3231–3245 CrossRef CAS PubMed.
- M. L. Rodriguez, B. T. Graham, L. M. Pabon, S. J. Han, C. E. Murry and N. J. Sniadecki, J. Biomech. Eng., 2014, 136, 051005 CrossRef PubMed.
- M. R. Poorna, S. Pravallika, A. Ashok, S. S, M. V. Thampi, P. K. Varma and U. Mony, Biochem. Eng. J., 2022, 187, 108521 CrossRef CAS.
- Y. C. Toh, C. Zhang, J. Zhang, Y. M. Khong, S. Chang, V. D. Samper, D. Van Noort, D. W. Hutmacher and H. Yu, Lab Chip, 2007, 7, 302–309 RSC.
- S. S. Nunes, J. W. Miklas, J. Liu, R. Aschar-Sobbi, Y. Xiao, B. Zhang, J. Jiang, S. Massé, M. Gagliardi, A. Hsieh, N. Thavandiran, M. A. Laflamme, K. Nanthakumar, G. J. Gross, P. H. Backx, G. Keller and M. Radisic, Nat. Methods, 2013, 10, 781–787 CrossRef CAS PubMed.
- G. Eng, B. W. Lee, L. Protas, M. Gagliardi, K. Brown, R. S. Kass, G. Keller, R. B. Robinson and G. Vunjak-Novakovic, Nat. Commun., 2016, 7, 10312 CrossRef CAS PubMed.
- M. Carlos-Oliveira, F. Lozano-Juan, P. Occhetta, R. Visone and M. Rasponi, Biophys. Rev., 2021, 13, 717–727 CrossRef PubMed.
- G. S. Ugolini, M. Rasponi, A. Pavesi, R. Santoro, R. Kamm, G. B. Fiore, M. Pesce and M. Soncini, Biotechnol. Bioeng., 2016, 113, 859–869 CrossRef CAS PubMed.
- L. Sun, Z. Chen, D. Xu and Y. Zhao, Adv. Sci., 2022, 9, 2105777 CrossRef CAS PubMed.
- L. Sun, Y. Wang, F. Bian, D. Xu and Y. Zhao, Sci. Bull., 2023, 68, 938–945 CrossRef CAS PubMed.
- M. Sakamiya, Y. Fang, X. Mo, J. Shen and T. Zhang, Med. Eng. Phys., 2020, 75, 36–44 CrossRef PubMed.
- M. L. Rexius-Hall, N. N. Khalil, S. S. Escopete, X. Li, J. Hu, H. Yuan, S. J. Parker and M. L. McCain, Sci. Adv., 2022, 8, eabn7097 CrossRef CAS PubMed.
- L. Ren, W. Liu, Y. Wang, J. C. Wang, Q. Tu, J. Xu, R. Liu, S. F. Shen and J. Wang, Anal. Chem., 2013, 85, 235–244 CrossRef CAS PubMed.
- K. Corral-Nájera, G. Chauhan, S. O. Serna-Saldívar, S. O. Martínez-Chapa and M. M. Aeinehvand, Microsyst. Nanoeng., 2023, 9, 107 CrossRef PubMed.
- T. E. Park, N. Mustafaoglu, A. Herland, R. Hasselkus, R. Mannix, E. A. FitzGerald, R. Prantil-Baun, A. Watters, O. Henry, M. Benz, H. Sanchez, H. J. McCrea, L. C. Goumnerova, H. W. Song, S. P. Palecek, E. Shusta and D. E. Ingber, Nat. Commun., 2019, 10, 1–12 CrossRef PubMed.
- C. G. Sip and A. Folch, Biomicrofluidics, 2014, 8, 036504 CrossRef PubMed.
- N. Y. Lee and B. H. Chung, Langmuir, 2009, 25, 3861–3866 CrossRef CAS PubMed.
- M. E. Vlachopoulou, A. Tserepi, P. Pavli, P. Argitis, M. Sanopoulou and K. Misiakos, J. Micromech. Microeng., 2009, 19, 015007 CrossRef.
- W. Yang, Y. Qin, Z. Wang, T. Yu, Y. Chen and Z. Ge, Sens. Actuators, A, 2022, 333, 113229 CrossRef CAS.
- X. Sun and S. S. Nunes, J. Visualized Exp., 2017, 2017, e55373 Search PubMed.
- N. Huebsch, P. Loskill, N. Deveshwar, C. I. Spencer, L. M. Judge, M. A. Mandegar, C. B. Fox, T. M. Mohamed, Z. Ma, A. Mathur, A. M. Sheehan, A. Truong, M. Saxton, J. Yoo, D. Srivastava, T. A. Desai, P. L. So, K. E. Healy and B. R. Conklin, Sci. Rep., 2016, 6, 24726 CrossRef CAS PubMed.
- A. Agarwal, J. A. Goss, A. Cho, M. L. McCain and K. K. Parker, Lab Chip, 2013, 13, 3599–3608 RSC.
- F. Navaee, N. Khornian, D. Longet, S. Heub, S. Boder-Pasche, G. Weder, A. Kleger, P. Renaud and T. Braschler, Cells, 2023, 12, 576 CrossRef CAS PubMed.
- N. Annabi, K. Tsang, S. M. Mithieux, M. Nikkhah, A. Ameri, A. Khademhosseini and A. S. Weiss, Adv. Funct. Mater., 2013, 23, 4950–4959 CrossRef CAS PubMed.
- J. Veldhuizen, J. Cutts, D. A. Brafman, R. Q. Migrino and M. Nikkhah, Biomaterials, 2020, 256, 120195 CrossRef CAS PubMed.
- B. M. Maoz, A. Herland, O. Y. Henry, W. D. Leineweber, M. Yadid, J. Doyle, R. Mannix, V. J. Kujala, E. A. Fitzgerald, K. K. Parker and D. E. Ingber, Lab Chip, 2017, 17, 2294–2302 RSC.
- H. Liu, L. A. MacQueen, J. F. Usprech, H. Maleki, K. L. Sider, M. G. Doyle, Y. Sun and C. A. Simmons, Biomaterials, 2018, 172, 30–40 CrossRef CAS PubMed.
- J. Lee, S. Mehrotra, E. Zare-Eelanjegh, R. O. Rodrigues, A. Akbarinejad, D. Ge, L. Amato, K. Kiaee, Y. C. Fang, A. Rosenkranz, W. Keung, B. B. Mandal, R. A. Li, T. Zhang, H. Y. Lee, M. R. Dokmeci, Y. S. Zhang, A. Khademhosseini and S. R. Shin, Small, 2021, 17, 2004258 CrossRef CAS PubMed.
- A. Leonard, A. Bertero, J. D. Powers, K. M. Beussman, S. Bhandari, M. Regnier, C. E. Murry and N. J. Sniadecki, J. Mol. Cell. Cardiol., 2018, 118, 147–158 CrossRef CAS PubMed.
- M. L. McCain, A. Agarwal, H. W. Nesmith, A. P. Nesmith and K. K. Parker, Biomaterials, 2014, 35, 5462–5471 CrossRef CAS PubMed.
- J. Criscione, Z. Rezaei, C. M. Hernandez Cantu, S. Murphy, S. R. Shin and D. H. Kim, Biosens. Bioelectron., 2023, 220, 114840 CrossRef CAS PubMed.
- J. Aleman, T. Kilic, L. S. Mille, S. R. Shin and Y. S. Zhang, Nat. Protoc., 2021, 16, 2564–2593 CrossRef CAS PubMed.
- A. Shinde, K. Illath, U. Kasiviswanathan, S. Nagabooshanam, P. Gupta, K. Dey, P. Chakrabarty, M. Nagai, S. Rao, S. Kar and T. S. Santra, Anal. Chem., 2023, 95, 3121–3146 CrossRef CAS PubMed.
- F. Zhang, K. Y. Qu, B. Zhou, Y. Luo, Z. Zhu, D. J. Pan, C. Cui, Y. Zhu, M. L. Chen and N. P. Huang, Biosens. Bioelectron., 2021, 179, 113080 CrossRef CAS PubMed.
- X. Wang, L. Wang, W. Dou, Z. Huang, Q. Zhao, M. Malhi, J. T. Maynes and Y. Sun, Biosens. Bioelectron., 2020, 166, 112399 CrossRef CAS PubMed.
- M. Yang, C. C. Lim, R. Liao and X. Zhang, J. Microelectromech. Syst., 2006, 15, 1483–1491 CAS.
- W. Cheng, N. Klauke, H. Sedgwick, G. L. Smith and J. M. Cooper, Lab Chip, 2006, 6, 1424–1431 RSC.
- V. J. Kujala, F. S. Pasqualini, J. A. Goss, J. C. Nawroth and K. K. Parker, J. Mater. Chem. B, 2016, 4, 3534–3543 RSC.
- J. K. Yip, D. Sarkar, A. P. Petersen, J. N. Gipson, J. Tao, S. Kale, M. L. Rexius-Hall, N. Cho, N.
N. Khalil, R. Kapadia and M. L. McCain, Lab Chip, 2021, 21, 674–687 RSC.
- H. Liu, O. A. Bolonduro, N. Hu, J. Ju, A. A. Rao, B. M. Duffy, Z. Huang, L. D. Black and B. P. Timko, Nano Lett., 2020, 20, 2585–2593 CrossRef CAS PubMed.
- Y. Liang, M. Ernst, F. Brings, D. Kireev, V. Maybeck, A. Offenhäusser and D. Mayer, Adv. Healthcare Mater., 2018, 7, 1800304 CrossRef PubMed.
- M. N. Hirt, N. A. Sörensen, L. M. Bartholdt, J. Boeddinghaus, S. Schaaf, A. Eder, I. Vollert, A. Stöhr, T. Schulze, A. Witten, M. Stoll, A. Hansen and T. Eschenhagen, Basic Res. Cardiol., 2012, 107, 307 CrossRef PubMed.
- J. T. Hinson, A. Chopra, N. Nafissi, W. J. Polacheck, C. C. Benson, S. Swist, J. Gorham, L. Yang, S. Schafer, C. C. Sheng, A. Haghighi, J. Homsy, N. Hubner, G. Church, S. A. Cook, W. A. Linke, C. S. Chen, J. G. Seidman, C. E. Seidman and S. O. Science, Science, 2015, 349, 982–986 CrossRef CAS PubMed.
- A. J. Rogers, J. M. Miller, R. Kannappan and P. Sethu, IEEE Trans. Biomed. Eng., 2019, 66, 3436–3443 Search PubMed.
- A. Agarwal, Y. Farouz, A. P. Nesmith, L. F. Deravi, M. L. Mc-Cain and K. K. Parker, Adv. Funct. Mater., 2013, 23, 3738–3746 CrossRef CAS PubMed.
- M. Dong, N. E. Oyunbaatar, P. P. Kanade, D. S. Kim and D. W. Lee, ACS Sens., 2021, 6, 3556–3563 CrossRef CAS PubMed.
- Y. H. Huang, C. F. Yang and Y. H. Hsu, Lab Chip, 2020, 20, 3423–3434 RSC.
- D. S. Kim, Y. J. Jeong, A. Shanmugasundaram, N. E. Oyunbaatar, J. Park, E. S. Kim, B. K. Lee and D. W. Lee, Biosens. Bioelectron., 2021, 190, 113380 CrossRef CAS PubMed.
- D. S. Kim, Y. J. Jeong, J. Park, A. Shanmugasundaram and D. W. Lee, Analyst, 2021, 146, 7160–7167 RSC.
- J. Javor, S. Sundaram, C. S. Chen and D. J. Bishop, J. Microelectromech. Syst., 2021, 30, 96–104 Search PubMed.
- T. Kamakura, T. Makiyama, K. Sasaki, Y. Yoshida, Y. Wuriyanghai, J. Chen, T. Hattori, S. Ohno, T. Kita, M. Horie, S. Yamanaka and T. Kimura, Circ. J., 2013, 77, 1307–1314 CrossRef CAS PubMed.
- I. Karakikes, M. Ameen, V. Termglinchan and J. C. Wu, Circ. Res., 2015, 117, 80–88 CrossRef CAS PubMed.
- I. Piccini, J. Rao, G. Seebohm and B. Greber, Genomics Data, 2015, 4, 69–72 CrossRef PubMed.
- N. Huebsch, B. Charrez, G. Neiman, B. Siemons, S. C. Boggess, S. Wall, V. Charwat, K. H. Jaeger, D. Cleres, A. Telle, F. T. Lee-Montiel, N. C. Jeffreys, N. Deveshwar, A. G. Edwards, J. Serrano, M. Snuderl, A. Stahl, A. Tveito, E. W. Miller, K. E. Healy, N. Biomed and E. Author, Nat. Biomed. Eng., 2022, 6, 372–388 CrossRef CAS PubMed.
- X. Yang, M. L. Rodriguez, A. Leonard, L. Sun, K. A. Fischer, Y. Wang, J. Ritterhoff, L. Zhao, S. C. Kolwicz, L. Pabon, H. Reinecke, N. J. Sniadecki, R. Tian, H. Ruohola-Baker, H. Xu and C. E. Murry, Stem Cell Rep., 2019, 13, 657–668 CrossRef CAS PubMed.
- C. Correia, A. Koshkin, P. Duarte, D. Hu, A. Teixeira, I. Domian, M. Serra and P. M. Alves, Sci. Rep., 2017, 7, 8590 CrossRef PubMed.
- D. Hu, A. Linders, A. Yamak, C. Correia, J. D. Kijlstra, A. Garakani, L. Xiao, D. J. Milan, P. Van Der Meer, M. Serra, P. M. Alves and I. J. Domian, Circ. Res., 2018, 123, 1066–1079 CrossRef CAS PubMed.
- R. J. Mills, D. M. Titmarsh, X. Koenig, B. L. Parker, J. G. Ryall, G. A. Quaife-Ryan, H. K. Voges, M. P. Hodson, C. Ferguson, L. Drowley, A. T. Plowright, E. J. Needham, Q. D. Wang, P. Gregorevic, M. Xin, W. G. Thomas, R. G. Parton, L. K. Nielsen, B. S. Launikonis, D. E. James, D. A. Elliott, E. R. Porrello and J. E. Hudson, Proc. Natl. Acad. Sci. U. S. A., 2017, 114, E8372–E8381 CrossRef CAS PubMed.
- X. Yang, M. Rodriguez, L. Pabon, K. A. Fischer, H. Reinecke, M. Regnier, N. J. Sniadecki, H. Ruohola-Baker and C. E. Murry, J. Mol. Cell. Cardiol., 2014, 72, 296–304 CrossRef CAS PubMed.
- S. S. Parikh, D. J. Blackwell, N. Gomez-Hurtado, M. Frisk, L. Wang, K. Kim, C. P. Dahl, A. Fiane, T. Tønnessen, D. O. Kryshtal, W. E. Louch and B. C. Knollmann, Circ. Res., 2017, 121, 1323–1330 CrossRef CAS PubMed.
- C. E. Rupert and K. L. Coulombe, Stem Cells Int., 2017, 2017, 7648409 Search PubMed.
- A. López-Canosa, S. Perez-Amodio, E. Yanac-Huertas, J. Ordoño, R. Rodriguez-Trujillo, J. Samitier, O. Castaño and E. Engel, Biofabrication, 2021, 13, 035047 CrossRef PubMed.
- N. L. Tulloch, V. Muskheli, M. V. Razumova, F. S. Korte, M. Regnier, K. D. Hauch, L. Pabon, H. Reinecke and C. E. Murry, Circ. Res., 2011, 109, 47–59 CrossRef CAS PubMed.
- M. Tiburcy, J. E. Hudson, P. Balfanz, S. Schlick, T. Meyer, M. L. C. Liao, E. Levent, F. Raad, S. Zeidler, E. Wingender, J. Riegler, M. Wang, J. D. Gold, I. Kehat, E. Wettwer, U. Ravens, P. Dierickx, L. W. Van Laake, M. J. Goumans, S. Khadjeh, K. Toischer, G. Hasenfuss, L. A. Couture, A. Unger, W. A. Linke, T. Araki, B. Neel, G. Keller, L. Gepstein, J. C. Wu and W. H. Zimmermann, Circulation, 2017, 135, 1832–1847 CrossRef CAS PubMed.
- A. Mihic, J. Li, Y. Miyagi, M. Gagliardi, S. H. Li, J. Zu, R. D. Weisel, G. Keller and R. K. Li, Biomaterials, 2014, 35, 2798–2808 CrossRef CAS PubMed.
- V. F. Shimko and W. C. Claycomb, Tissue Eng., 2008, 14, 49–58 CrossRef CAS PubMed.
- G. Kensah, I. Gruh, J. Viering, H. Schumann, J. Dahlmann, H. Meyer, D. Skvorc, A. Bär, P. Akhyari, A. Heisterkamp, A. Haverich and U. Martin, Tissue Eng., Part C, 2011, 17, 463–473 CrossRef PubMed.
- J. L. Ruan, N. L. Tulloch, M. Saiget, S. L. Paige, M. V. Razumova, M. Regnier, K. C. Tung, G. Keller, L. Pabon, H. Reinecke and C. E. Murry, Stem Cells, 2015, 33, 2148–2157 CrossRef CAS PubMed.
- W. H. Zimmermann, K. Schneiderbanger, P. Schubert, M. Didié, F. Münzel, J. F. Heubach, S. Kostin, W. L. Neuhuber and T. Eschenhagen, Circ. Res., 2002, 90, 223–230 CrossRef CAS PubMed.
- T. Hendrickson, C. Mancino, L. Whitney, C. Tsao, M. Rahimi and F. Taraballi, Nanomed.: Nanotechnol., Biol. Med., 2021, 33, 102367 CrossRef CAS PubMed.
- C. Rao, T. Prodromakis, L. Kolker, U. A. Chaudhry, T. Trantidou, A. Sridhar, C. Weekes, P. Camelliti, S. E. Harding, A. Darzi, M. H. Yacoub, T. Athanasiou and C. M. Terracciano, Biomaterials, 2013, 34, 2399–2411 CrossRef CAS PubMed.
- D. Cruz-Moreira, R. Visone, F. Vasques-Nóvoa, A. S. Barros, A. Leite-Moreira, A. Redaelli, M. Moretti and M. Rasponi, Biotechnol. Bioeng., 2021, 118, 3128–3137 CrossRef CAS PubMed.
- M. Radisic, H. Park, H. Shing, T. Consi, F. J. Schoen, R. Langer, L. E. Freed and G. Vunjak-Novakovic, Proc. Natl. Acad. Sci. U. S. A., 2004, 101, 18129–18134 CrossRef CAS PubMed.
- W. LaBarge, S. Mattappally, R. Kannappan, V. G. Fast, D. Pretorius, J. L. Berry and J. Zhang, PLoS One, 2019, 14, e0219442 CrossRef CAS PubMed.
- S. Shen, L. R. Sewanan, S. Shao, S. S. Halder, P. Stankey, X. Li and S. G. Campbell, Stem Cell Rep., 2022, 17, 2037–2049 CrossRef CAS PubMed.
- Y. C. Chan, S. Ting, Y. K. Lee, K. M. Ng, J. Zhang, Z. Chen, C. W. Siu, S. K. Oh and H. F. Tse, J. Cardiovasc. Transl. Res., 2013, 6, 989–999 CrossRef PubMed.
- K. Ronaldson-Bouchard, S. P. Ma, K. Yeager, T. Chen, L. J. Song, D. Sirabella, K. Morikawa, D. Teles, M. Yazawa and G. Vunjak-Novakovic, Nature, 2018, 556, 239–243 CrossRef CAS PubMed.
- D. Tirziu, F. J. Giordano and M. Simons, Circulation, 2010, 122, 928–937 CrossRef PubMed.
- O. Bergmann, S. Zdunek, A. Felker, M. Salehpour, K. Alkass, S. Bernard, S. L. Sjostrom, M. Szewczykowska, T. Jackowska, C. Dos Remedios, T. Malm, M. Andrä, R. Jashari, J. R. Nyengaard, G. Possnert, S. Jovinge, H. Druid and J. Frisén, Cell, 2015, 161, 1566–1575 CrossRef CAS PubMed.
- A. R. Pinto, A. Ilinykh, M. J. Ivey, J. T. Kuwabara, M. L. D'antoni, R. Debuque, A. Chandran, L. Wang, K. Arora, N. A. Rosenthal and M. D. Tallquist, Circ. Res., 2016, 118, 400–409 CrossRef CAS PubMed.
- D. L. Brutsaert, Physiol. Rev., 2003, 83, 59–115 CrossRef CAS PubMed.
- W. J. Kowalski, I. H. Garcia-Pak, W. Li, H. Uosaki, E. Tampakakis, J. Zou, Y. Lin, K. Patterson, C. Kwon and Y. S. Mukouyama, Front. Cell Dev. Biol., 2022, 10, 1–13 Search PubMed.
- H. Vuorenpää, K. Penttinen, T. Heinonen, M. Pekkanen-Mattila, J. R. Sarkanen, T. Ylikomi and K. Aalto-Setälä, Cytotechnology, 2017, 69, 785–800 CrossRef PubMed.
- P. Beauchamp, C. B. Jackson, L. C. Ozhathil, I. Agarkova, C. L. Galindo, D. B. Sawyer, T. M. Suter and C. Zuppinger, Front. Mol. Biosci., 2020, 7, 1–17 CrossRef PubMed.
- C. Kim, M. Majdi, P. Xia, K. A. Wei, M. Talantova, S. Spiering, B. Nelson, M. Mercola and H. S. V. Chen, Stem Cells Dev., 2010, 19, 783–795 CrossRef CAS PubMed.
- Y. Li, H. Asfour and N. Bursac, Acta Biomater., 2017, 55, 120–130 CrossRef CAS PubMed.
- X. Qu, C. Harmelink and H. S. Baldwin, Front. Cardiovasc. Med., 2022, 9, 857581 CrossRef CAS PubMed.
- W. Z. Zhu, Y. Xie, K. W. Moyes, J. D. Gold, B. Askari and M. A. Laflamme, Circ. Res., 2010, 107, 776–786 CrossRef CAS PubMed.
- P. C. Hsieh, M. E. Davis, L. K. Lisowski and R. T. Lee, Annu. Rev. Physiol., 2006, 68, 51–66 CrossRef CAS PubMed.
- K. K. Dunn, I. M. Reichardt, A. D. Simmons, G. Jin, M. E. Floy, K. M. Hoon and S. P. Palecek, Biotechnol. J., 2019, 14, e1800725 CrossRef PubMed.
- A. Vivas, C. Ijspeert, J. Y. Pan, K. Vermeul, A. van den Berg, R. Passier, S. S. Keller and A. D. van der Meer, Adv. Mater. Technol., 2022, 7, 2101355 CrossRef CAS.
- Z. Lin, J. C. Garbern, R. Liu, Q. Li, E. Mancheño Juncosa, H. L. Elwell, M. Sokol, J. Aoyama, U.-S. Deumer, E. Hsiao, H. Sheng, R. T. Lee and J. Liu, Sci. Adv., 2023, 9, eade8513 CrossRef CAS PubMed.
- F. H. Epstein, Z. Yang, W. D. Gilson, S. S. Berr, C. M. Kramer and B. A. French, Magn. Reson. Med., 2002, 48, 399–403 CrossRef PubMed.
- J. F. Wenk, D. Klepach, L. C. Lee, Z. Zhang, L. Ge, E. E. Tseng, A. Martin, S. Kozerke, J. H. Gorman, R. C. Gorman and J. M. Guccione, Ann. Thorac. Surg., 2012, 93, 1188–1193 CrossRef PubMed.
- A. G. Kleber, M. J. Janse, F. J. G. Wilms-Schopmann, A. A. M. Wilde, R. Coronel and A. G. Kleber, Circulation, 1986, 73, 189–198 CrossRef CAS PubMed.
-
T. Kalogeris, C. P. Baines, M. Krenz and R. J. Korthuis, International Review of Cell and Molecular Biology, Elsevier Inc., 2012, vol. 298, pp. 229–317 Search PubMed.
- T. Qian, A.-L. Nieminen, B. Herman and J. J. Lemasters, Am. J. Physiol., 1997, 273, C1783–C1792 CrossRef CAS PubMed.
- S. Toyokuni, Pathol. Int., 1999, 49, 91–102 CrossRef CAS PubMed.
- J.-S. Kim, Y. Jin and J. J. Lemasters, Am. J. Physiol., 2006, 290, 2024–2034 Search PubMed.
- H. K. Eltzschig and T. Eckle, Nat. Med., 2011, 17, 1391–1401 CrossRef CAS PubMed.
- G. Khanal, K. Chung, X. Solis-Wever, B. Johnson and D. Pappas, Analyst, 2011, 136, 3519–3526 RSC.
- D. J. Richards, Y. Li, C. M. Kerr, J. Yao, G. C. Beeson, R. C. Coyle, X. Chen, J. Jia, B. Damon, R. Wilson, E. Starr Hazard, G. Hardiman, D. R. Menick, C. C. Beeson, H. Yao, T. Ye and Y. Mei, Nat. Biomed. Eng., 2020, 4, 446–462 CrossRef CAS PubMed.
- Y. Yamasaki, K. Matsuura, D. Sasaki and T. Shimizu, Regener. Ther., 2021, 18, 66–75 CrossRef CAS PubMed.
- T. Chen and G. Vunjak-Novakovic, Tissue Eng., Part A, 2019, 25, 711–724 CrossRef PubMed.
- H. E. Bøtker, R. Kharbanda, M. R. Schmidt, M. Bøttcher, A. K. Kaltoft, C. J. Terkelsen, K. Munk, N. H. Andersen, T. M. Hansen, S. Trautner, J. F. Lassen, E. H. Christiansen, L. R. Krusell, S. D. Kristensen, L. Thuesen, S. S. Nielsen, M. Rehling, H. T. Sørensen, A. N. Redington and T. T. Nielsen, Lancet, 2010, 375, 727–734 CrossRef PubMed.
- D. Schulman, D. S. Latchman and D. M. Yellon, Am. J. Physiol., 2002, 283, H1481–H1488 CAS.
- T. Uchiyama, R. M. Engelman, N. Maulik and D. K. Das, Circulation, 2004, 109, 3042–3049 CrossRef CAS PubMed.
- S. Haghjooy Javanmard, A. Ziaei, S. Ziaei, E. Ziaei and M. Mirmohammad-Sadeghi, Oxid. Med. Cell. Longevity, 2013, 2013, 676829 Search PubMed.
- A. Dominguez-Rodriguez, P. Abreu-Gonzalez, J. M. de la Torre-Hernandez, L. Consuegra-Sanchez, R. Piccolo, J. Gonzalez-Gonzalez, T. Garcia-Camarero, M. del Mar Garcia-Saiz, A. Aldea-Perona, R. J. Reiter, N. Caballero-Estevez, A. de la Rosa, T. Virgos-Aller, J. Nazco-Casariego, I. Laynez-Cerdeña, F. Bosa-Ojeda, A. Sanchez-Grande, G. Yanes-Bowden, M. Vargas-Torres, A. Lara-Padrón, P. Perez-Jorge, L. Diaz-Flores, J. Lopez, J. Lacalzada-Almeida, A. Duque, M. Bethencourt, M. Izquierdo, R. Juarez-Prera, G. Blanco-Palacios, A. Barragan-Acea, J. Ferrer-Hita, B. Marí-Lopez, M. Padilla, E. Gonzalez, M. Martin-Cabeza, C. Mendez-Vargas, P. Barrios, C. Belleyo-Belkasem, M. Leiva, I. Betancor, J. Miranda, T. Giménez Poderós, F. Soria-Arcos and L. Martinez, Am. J. Cardiol., 2017, 120, 522–526 CrossRef CAS PubMed.
- K. H. Dwaich, F. G. Al-Amran, B. I. Al-Sheibani and H. A. Al-Aubaidy, Int. J. Cardiol., 2016, 221, 977–986 CrossRef PubMed.
- P. Ghaeli, S. Vejdani, A. Ariamanesh and A. Hajhossein Talasaz, Iran. J. Pharm. Res., 2015, 14, 851–855 CAS.
- J. Šochman, J. Vrbská, B. Musilová and M. Roček, Clin. Cardiol., 1996, 19, 94–100 CrossRef PubMed.
- J. T. Flaherty, B. Pitt, J. W. Gruber, R. R. Heuser, D. A. Rothbaum, L. R. Burwell, B. S. George, D. J. Kereiakes, D. Deitchman, N. Gustafson, J. A. Brinker, L. C. Becker, G. B. J. Mancini, E. Topol and S. W. Werns, Circulation, 1994, 89, 1982–1991 CrossRef CAS PubMed.
- N. Liu, L. Xie, P. Xiao, X. Chen, W. Kong, Q. Lou, F. Chen and X. Lu, Mol. Cell. Biochem., 2022, 477, 1249–1260 CrossRef CAS PubMed.
- M. Abrial, C. C. Da Silva, B. Pillot, L. Augeul, F. Ivanes, G. Teixeira, R. Cartier, D. Angoulvant, M. Ovize and R. Ferrera, J. Mol. Cell. Cardiol., 2014, 68, 56–65 CrossRef CAS PubMed.
- C. Humeres and N. G. Frangogiannis, JACC Basic Transl. Sci., 2019, 4, 449–467 CrossRef PubMed.
- T. M. Leucker, Z. D. Ge, J. Procknow, Y. Liu, Y. Shi, M. Bienengraeber, D. C. Warltier and J. R. Kersten, PLoS One, 2013, 8, e70088 CrossRef CAS PubMed.
- R. Pabla and M. J. Curtis, J. Mol. Cell. Cardiol., 1996, 28, 2097–2110 CrossRef CAS PubMed.
- G. Chen, C. Xu, T. G. Gillette, T. Huang, P. Huang, Q. Li, X. Li, Q. Li, Y. Ning, R. Tang, C. Huang, Y. Xiong, X. Tian, J. Xu, J. Xu, L. Chang, C. Wei, C. Jin, J. A. Hill and Y. Yang, Theranostics, 2020, 10, 11754–11774 CrossRef CAS PubMed.
- M. Horckmans, L. Ring, J. Duchene, D. Santovito, M. J. Schloss, M. Drechsler, C. Weber, O. Soehnlein and S. Steffens, Eur. Heart J., 2017, 38, 187–197 CAS.
- I. Andreadou, H. A. Cabrera-Fuentes, Y. Devaux, N. G. Frangogiannis, S. Frantz, T. Guzik, E. A. Liehn, C. P. Gomes, R. Schulz and D. J. Hausenloy, Cardiovasc. Res., 2019, 115, 1117–1130 CrossRef CAS PubMed.
- V. Ryabov, A. Gombozhapova, Y. Rogovskaya, J. Kzhyshkowska, M. Rebenkova and R. Karpov, Immunobiology, 2018, 223, 413–421 CrossRef CAS PubMed.
- M. Kawaguchi, M. Takahashi, T. Hata, Y. Kashima, F. Usui, H. Morimoto, A. Izawa, Y. Takahashi, J. Masumoto, J. Koyama, M. Hongo, T. Noda, J. Nakayama, J. Sagara, S. Taniguchi and U. Ikeda, Circulation, 2011, 123, 594–604 CrossRef CAS PubMed.
- G. Niccoli, F. Burzotta, L. Galiuto and F. Crea, J. Am. Coll. Cardiol., 2009, 54, 281–292 CrossRef PubMed.
- B. R. Ito, G. Schmid-Schönbein and R. L. Engler, Blood Cells, 1990, 16, 145–166 CAS.
- R. L. Engler, G. W. Schmid-Schonbein and R. S. Pavelec, Am. J. Pathol., 1983, 111, 98–111 CAS.
- M. Nahrendorf and F. K. Swirski, Circ. Res., 2013, 112, 1624–1633 CrossRef CAS PubMed.
- A. M. Van Der Laan, A. Hirsch, L. F. Robbers, R. Nijveldt, I. Lommerse, R. Delewi, P. A. Van Der Vleuten, B. J. Biemond, J. J. Zwaginga, W. J. Van Der Giessen, F. Zijlstra, A. C. Van Rossum, C. Voermans, C. E. Van Der Schoot and J. J. Piek, Am. Heart J, 2012, 163, 57–65 CrossRef CAS PubMed.
- J. Veldhuizen, R. Chavan, B. Moghadas, J. G. Park, V. D. Kodibagkar, R. Q. Migrino and M. Nikkhah, Biomaterials, 2022, 281, 121336 CrossRef CAS PubMed.
- M. Yadid, J. U. Lind, A. M. Ardoña, S. P. Sheehy, L. E. Dickinson, F. Eweje, M. M. C. Bastings, B. Pope, B. B. O'connor, J. R. Straubhaar, B. Budnik, A. G. Kleber and K. K. Parker, Sci. Transl. Med., 2020, 12, 8005 CrossRef PubMed.
- K. T. Wagner, T. R. Nash, B. Liu, G. Vunjak-Novakovic and M. Radisic, Trends Biotechnol., 2021, 39, 755–773 CrossRef CAS PubMed.
- D. A. Chistiakov, A. N. Orekhov and Y. V. Bobryshevy, Int. J. Mol. Sci., 2016, 17, 1–18 Search PubMed.
- Y. Wang, L. Zhang, Y. Li, L. Chen, X. Wang, W. Guo, X. Zhang, G. Qin, S.-h. He, A. Zimmerman, Y. Liu, I.-m. Kim, N. L. Weintraub and Y. Tang, Int. J. Cardiol., 2015, 192, 61–69 CrossRef PubMed.
- T. M. Ribeiro-Rodrigues, T. L. Laundos, R. Pereira-Carvalho, D. Batista-Almeida, R. Pereira, V. Coelho-Santos, A. P. Silva, R. Fernandes, M. Zuzarte, F. J. Enguita, M. C. Costa, P. Pinto-Do-O, M. T. Pinto, P. Gouveia, L. Ferreira, J. C. Mason, P. Pereira, B. R. Kwak, D. S. Nascimento and H. Girao, Cardiovasc. Res., 2017, 113, 1338–1350 CrossRef CAS PubMed.
- H. Li, Y. Liao, L. Gao, T. Zhuang, Z. Huang, H. Zhu and J. Ge, Theranostics, 2018, 8, 2079–2093 CrossRef CAS PubMed.
- B. He, J. Xiao, A. J. Ren, Y. F. Zhang, H. Zhang, M. Chen, B. Xie, X. G. Gao and Y. W. Wang, J. Biomed. Sci., 2011, 18, 22 CrossRef CAS PubMed.
- J. Wang, Z. Jia, C. Zhang, M. Sun, W. Wang, P. Chen, K. Ma, Y. Zhang, X. Li and C. Zhou, RNA Biol., 2014, 11, 339–350 CrossRef PubMed.
- S. J. Matkovich, W. Wang, Y. Tu, W. H. Eschenbacher, L. E. Dorn, G. Condorelli, A. Diwan, J. M. Nerbonne and G. W. Dorn, Circ. Res., 2010, 106, 166–175 CrossRef CAS PubMed.
- H. Luo, X. Li, T. Li, L. Zhao, J. He, L. Zha, Q. Qi and Z. Yu, Cardiovasc. Res., 2019, 115, 1189–1204 CrossRef CAS PubMed.
- B. W. Balkom, O. G. Jong, M. Smits, J. Brummelman, K. d. Ouden, P. M. Bree, M. A. Eijndhoven, D. M. Pegtel, W. Stoorvogel, T. Würdinger and M. C. Verhaar, Blood, 2013, 121, 3997–4006 CrossRef PubMed.
- M. S. Njock, H. S. Cheng, L. T. Dang, M. Nazari-Jahantigh, A. C. Lau, E. Boudreau, M. Roufaiel, M. I. Cybulsky, A. Schober and J. E. Fish, Blood, 2015, 125, 3202–3212 CrossRef CAS PubMed.
- M. Adamiak, G. Cheng, S. Bobis-Wozowicz, L. Zhao, S. Kedracka-Krok, A. Samanta, E. Karnas, Y. T. Xuan, B. Skupien-Rabian, X. Chen, U. Jankowska, M. Girgis, M. Sekula, A. Davani, S. Lasota, R. J. Vincent, M. Sarna, K. L. Newell, O. L. Wang, N. Dudley, Z. Madeja, B. Dawn and E. K. Zuba-Surma, Circ. Res., 2018, 122, 296–309 CrossRef CAS PubMed.
- M. Khan, E. Nickoloff, T. Abramova, J. Johnson, S. K. Verma, P. Krishnamurthy, A. R. Mackie, E. Vaughan, V. N. S. Garikipati, C. Benedict, V. Ramirez, E. Lambers, A. Ito, E. Gao, S. Misener, T. Luongo, J. Elrod, G. Qin, S. R. Houser, W. J. Koch and R. Kishore, Circ. Res., 2015, 117, 52–64 CrossRef CAS PubMed.
- Y. Zhang, Y.-W. Hu, L. Zheng and Q. Wang, DNA Cell Biol., 2017, 36, 202–211 CrossRef CAS PubMed.
- N. G. Frangogiannis, Cardiovasc. Res., 2021, 117, 1450–1488 CrossRef CAS PubMed.
- N. G. Frangogiannis, Mol. Aspects Med., 2019, 65, 70–99 CrossRef CAS PubMed.
- P. Occhetta, G. Isu, M. Lemme, C. Conficconi, P. Oertle, C. Räz, R. Visone, G. Cerino, M. Plodinec, M. Rasponi and A. Marsano, Integr. Biol., 2018, 10, 174–183 CrossRef CAS PubMed.
- M. Kong, J. Lee, I. K. Yazdi, A. K. Miri, Y.-D. Lin, J. Seo, Y. S. Zhang, A. Khademhosseini and S. R. Shin, Adv. Healthcare Mater., 2019, 8, 1801146 CrossRef PubMed.
- M. O. Lee, K. B. Jung, S. J. Jo, S. A. Hyun, K. S. Moon, J. W. Seo, S. H. Kim and M. Y. Son, J. Biol. Eng., 2019, 13, 15 CrossRef PubMed.
- A. H. Sadeghi, S. R. Shin, J. C. Deddens, G. Fratta, S. Mandla, I. K. Yazdi, G. Prakash, S. Antona, D. Demarchi, M. P. Buijsrogge, J. P. G. Sluijter, J. Hjortnaes and A. Khademhosseini, Adv. Healthcare Mater., 2017, 6, 1601434 CrossRef PubMed.
- E. Y. Wang, N. Rafatian, Y. Zhao, A. Lee, B. F. L. Lai, R. X. Lu, D. Jekic, L. Davenport Huyer, E. J. Knee-Walden, S. Bhattacharya, P. H. Backx and M. Radisic, ACS Cent. Sci., 2019, 5, 1146–1158 CrossRef CAS PubMed.
- O. Mastikhina, B. U. Moon, K. Williams, R. Hatkar, D. Gustafson, O. Mourad, X. Sun, M. Koo, A. Y. Lam, Y. Sun, J. E. Fish, E. W. Young and S. S. Nunes, Biomaterials, 2020, 233, 119741 CrossRef CAS PubMed.
- O. Mourad, O. Mastikhina, S. Khan, R. Hatkar, K. Williams and S. S. Nunes, ACS Mater. Au, 2023, 3, 360370 Search PubMed.
- S. B. Bremner, C. J. Mandrycky, A. Leonard, R. M. Padgett, A. R. Levinson, E. S. Rehn, J. M. Pioner, N. J. Sniadecki and D. L. Mack, J. Tissue Eng., 2022, 13, 1–16 CAS.
- S. Marchiano, T. Y. Hsiang, A. Khanna, T. Higashi, L. S. Whitmore, J. Bargehr, H. Davaapil, J. Chang, E. Smith, L. P. Ong, M. Colzani, H. Reinecke, X. Yang, L. Pabon, S. Sinha, B. Najafian, N. J. Sniadecki, A. Bertero, M. Gale and C. E. Murry, Stem Cell Rep., 2021, 16, 478–492 CrossRef CAS PubMed.
- A. J. Rogers, R. Kannappan, H. Abukhalifeh, M. Ghazal, J. M. Miller, A. El-Baz, V. Fast and P. Sethu, Hemodynamic Stimulation Using the Biomimetic Cardiac Tissue Model (BCTM) Enhances Maturation of Human Induced Pluripotent Stem Cell-Derived Cardiomyocytes, Cells Tissues Organs, 2019, 206, 82–94 CrossRef PubMed.
- A. Brodehl, H. Ebbinghaus, M. A. Deutsch, J. Gummert, A. Gärtner, S. Ratnavadivel and H. Milting, Int. J. Mol. Sci., 2019, 20, 4381 CrossRef CAS PubMed.
- S. L. Clarke, A. Bowron, I. L. Gonzalez, S. J. Groves, R. Newbury-Ecob, N. Clayton, R. P. Martin, B. Tsai-Goodman, V. Garratt, M. Ashworth, V. M. Bowen, K. R. Mccurdy, M. K. Damin, C. T. Spencer, M. J. Toth, R. I. Kelley and C. G. Steward, Orphanet J. Rare Dis., 2013, 8, 23 CrossRef PubMed.
- R. H. Houtkooper, M. Turkenburg, B. T. Poll-The, D. Karall, C. Pérez-Cerdá, A. Morrone, S. Malvagia, R. J. Wanders, W. Kulik and F. M. Vaz, Biochim. Biophys. Acta, 2009, 1788, 2003–2014 CrossRef CAS PubMed.
- S. Bione, P. D'adamo, E. Maestrini, A. K. Gedeon, P. A. Bolhuis and D. Toniolo, Nat. Genet., 1996, 12, 385–389 CrossRef CAS PubMed.
- R. Zweigerdt, I. Gruh and U. Martin, Cell Stem Cell, 2014, 15, 9–11 CrossRef CAS PubMed.
- B. M. Ulmer and T. Eschenhagen, Biochim. Biophys. Acta, Mol. Cell Res., 2020, 1867, 118471 CrossRef CAS PubMed.
- G. Wang, M. L. McCain, L. Yang, A. He, F. S. Pasqualini, A. Agarwal, H. Yuan, D. Jiang, D. Zhang, L. Zangi, J. Geva, A. E. Roberts, Q. Ma, J. Ding, J. Chen, D. Z. Wang, K. Li, J. Wang, R. J. Wanders, W. Kulik, F. M. Vaz, M. A. Laflamme, C. E. Murry, K. R. Chien, R. I. Kelley, G. M. Church, K. K. Parker and W. T. Pu, Nat. Med., 2014, 20, 616–623 CrossRef CAS PubMed.
- F. Valianpour, R. J. Wanders, H. Overmars, F. M. Vaz, P. G. Barth and A. H. Van Gennip, J. Lipid Res., 2003, 44, 560–566 CrossRef CAS PubMed.
- B. J. Maron, J. M. Gardin, J. M. Flack, S. S. Gidding, T. T. Kurosaki and D. E. Bild, Circulation, 1995, 92, 785–789 CrossRef CAS PubMed.
- B. J. Maron and M. S. Maron, Lancet, 2013, 381, 242–255 CrossRef PubMed.
- K. M. Harris, P. Spirito, M. S. Maron, A. G. Zenovich, F. Formisano, J. R. Lesser, S. Mackey-Bojack, W. J. Manning, J. E. Udelson and B. J. Maron, Circulation, 2006, 114, 216–225 CrossRef PubMed.
- B. J. Maron, P. Spirito, Y. Wesley and J. Arce, N. Engl. J. Med., 1986, 315, 610–614 CrossRef CAS PubMed.
- R. Cohn, K. Thakar, A. Lowe, F. A. Ladha, A. M. Pettinato, R. Romano, E. Meredith, Y. S. Chen, K. Atamanuk, B. D. Huey and J. T. Hinson, Stem Cell Rep., 2019, 12, 71–83 CrossRef CAS PubMed.
- Y. Xiao, B. Zhang, H. Liu, J. W. Miklas, M. Gagliardi, A. Pahnke, N. Thavandiran, Y. Sun, C. Simmons, G. Keller and M. Radisic, Lab Chip, 2014, 14, 869–882 RSC.
- Y. Zhao, N. Rafatian, N. T. Feric, B. J. Cox, R. Aschar-Sobbi, E. Y. Wang, P. Aggarwal, B. Zhang, G. Conant, K. Ronaldson-Bouchard, A. Pahnke, S. Protze, J. H. Lee, L. Davenport Huyer, D. Jekic, A. Wickeler, H. E. Naguib, G. M. Keller, G. Vunjak-Novakovic, U. Broeckel, P. H. Backx and M. Radisic, Cell, 2019, 176, 913–927 CrossRef CAS PubMed.
- T. J. Cashman, R. Josowitz, B. V. Johnson, B. D. Gelb and K. D. Costa, PLoS One, 2016, 11, e0146697 CrossRef PubMed.
- R. Josowitz, S. Mulero-Navarro, N. A. Rodriguez, C. Falce, N. Cohen, E. M. Ullian, L. A. Weiss, K. A. Rauen, E. A. Sobie and B. D. Gelb, Stem Cell Rep., 2016, 7, 355–369 CrossRef CAS PubMed.
- H. Cai, B. Li, A. Bai, J. Huang, Y. Zhan, N. Sun, Q. Liang and C. Xu, Exp. Cell Res., 2020, 387, 111736 CrossRef CAS PubMed.
- J. G. Smith, T. Owen, J. R. Bhagwan, D. Mosqueira, E. Scott, I. Mannhardt, A. Patel, R. Barriales-Villa, L. Monserrat, A. Hansen, T. Eschenhagen, S. E. Harding, S. Marston and C. Denning, Stem Cell Rep., 2018, 11, 1226–1243 CrossRef CAS PubMed.
- E. Mercuri, C. G. Bönnemann and F. Muntoni, Lancet, 2019, 394, 2025–2038 CrossRef PubMed.
- D. Duan, N. Goemans, S. Takeda, E. Mercuri and A. Aartsma-Rus, Nat. Rev. Dis. Primers, 2021, 7, 13 CrossRef PubMed.
- P. Ferriert, F. Bamatter and D. Klein, J. Med. Genet., 1965, 965, 38 CrossRef PubMed.
-
J. R. Macadangdang, J. W. Miklas, A. S. T. Smith, E. Choi, W. Leung, Y. Wang, X. Guan, S. Lee, M. R. Salick, M. Regnier, D. Mack, M. K. Childers, H. Ruohola-Baker and D.-H. Kim, bioRxiv, 2018, preprint, DOI:10.1101/456301.
- J. L. Jefferies and J. A. Towbin, Lancet, 2010, 375, 752–762 CrossRef PubMed.
- M. Merlo, A. Cannatà, M. Gobbo, D. Stolfo, P. M. Elliott and G. Sinagra, Eur. J. Heart Failure, 2018, 20, 228–239 CrossRef PubMed.
- D. S. Herman, L. Lam, M. R. G. Taylor, L. Wang, D. Christodoulou, L. Conner, S. R. Depalma, B. Mcdonough, E. Sparks, D. Lin Teodorescu, A. L. Cirino, N. R. Banner, D. J. Pennell, S. Graw, M. Merlo, A. D. Lenarda, G. Sinagra, J. Martijn Bos, M. J. Ackerman, R. N. Mitchell, C. E. Murry, N. K. Lakdawala, C. Y. Ho, P. J. R. Barton, S. A. Cook, L. Mestroni, J. G. Seidman, C. E. Seidman, D. J. G. Seidman and C. E. Seidman, N. Engl. J. Med., 2012, 366, 619–628 CrossRef CAS PubMed.
- K. Streckfuss-Bomeke, M. Tiburcy, A. Fomin, X. Luo, W. Li, C. Fischer, C. Ozcelik, A. Perrot, S. Sossalla, J. Haas, R. O. Vidal, S. Rebs, S. Khadjeh, B. Meder, S. Bonn, W. A. Linke, W. H. Zimmermann, G. Hasenfuss and K. Guan, J. Mol. Cell. Cardiol., 2017, 113, 9–21 CrossRef PubMed.
- C. A. Tharp, M. E. Haywood, O. Sbaizero, M. R. Taylor and L. Mestroni, Front. Physiol., 2019, 10, 1436 CrossRef PubMed.
- S. F. Nagueh, G. Shah, Y. Wu, G. Torre-Amione, N. M. King, S. Lahmers, C. C. Witt, K. Becker, S. Labeit and H. L. Granzier, Circulation, 2004, 110, 155–162 CrossRef CAS PubMed.
- W. Guo, S. Schafer, M. L. Greaser, M. H. Radke, M. Liss, T. Govindarajan, H. Maatz, H. Schulz, S. Li, A. M. Parrish, V. Dauksaite, P. Vakeel, S. Klaassen, B. Gerull, L. Thierfelder, V. Regitz-Zagrosek, T. A. Hacker, K. W. Saupe, G. W. Dec, P. T. Ellinor, C. A. MacRae, B. Spallek, R. Fischer, A. Perrot, C. Ozcelik, K. Saar, N. Hubner and M. Gotthardt, Nat. Med., 2012, 18, 766–773 CrossRef CAS PubMed.
- A. M. Pettinato, F. A. Ladha, D. J. Mellert, N. Legere, R. Cohn, R. Romano, K. Thakar, Y. S. Chen and J. T. Hinson, Circulation, 2020, 142, 2262–2275 CrossRef CAS PubMed.
- Y. Dai, A. Amenov, N. Ignatyeva, A. Koschinski, H. Xu, P. L. Soong, M. Tiburcy, W. A. Linke, M. Zaccolo, G. Hasenfuss, W. H. Zimmermann and A. Ebert, Sci. Rep., 2020, 10, 209 CrossRef CAS PubMed.
- M. Wauchop, N. Rafatian, Y. Zhao, M. Gagliardi, S. Massé, B. J. Cox, P. Lai, T. Liang, S. S. Landau, S. Protze, X. D. Gao, E. Y. Wang, K. C. Tung, Z. Laksman, R. Lu, G. Keller, K. Nanthakumar, M. Radisic and P. H. Backx, Biomaterials, 2023, 122255 CrossRef CAS PubMed.
- R. Onkal, J. H. Mattis, S. P. Fraser, J. K. Diss, D. Shao, K. Okuse and M. B. Djamgoz, J. Cell. Physiol., 2008, 216, 716–726 CrossRef CAS PubMed.
- P. S. Kishnani, R. D. Steiner, D. Bali, K. Berger, B. J. Byrne, L. Case, J. F. Crowley, S. Downs, R. R. Howell, R. M. Kravitz, J. Mackey, D. Marsden, A. M. Martins, D. S. Millington, M. Nicolino, G. O'Grady, M. C. Patterson, D. M. Rapoport, A. Slonim, C. T. Spencer, C. J. Tifft and M. S. Watson, Genet. Med., 2006, 8, 267–288 CrossRef PubMed.
- K. K. Raval, R. Tao, B. E. White, W. J. De Lange, C. H. Koonce, J. Yu, P. S. Kishnani, J. A. Thomson, D. F. Mosher, J. C. Ralphe and T. J. Kamp, J. Biol. Chem., 2015, 290, 3121–3136 CrossRef CAS PubMed.
- A. H. Koeppen, J. Neurol. Sci., 2011, 303, 1–12 CrossRef CAS PubMed.
- A. Y. Tsou, E. K. Paulsen, S. J. Lagedrost, S. L. Perlman, K. D. Mathews, G. R. Wilmot, B. Ravina, A. H. Koeppen and D. R. Lynch, J. Neurol. Sci., 2011, 307, 46–49 CrossRef PubMed.
- A. O. T. Wong, G. Wong, M. Shen, M. Z. Y. Chow, W. W. Tse, B. Gurung, S. Y. Mak, D. K. Lieu, K. D. Costa, C. W. Chan, A. Martelli, J. F. Nabhan and R. A. Li, Stem Cell Res. Ther., 2019, 10, 203 CrossRef PubMed.
- A. Dimopoulos, R. J. Sicko, D. M. Kay, S. L. Rigler, C. M. Druschel, M. Caggana, M. L. Browne, R. Fan, P. A. Romitti, L. C. Brody and J. L. Mills, Birth Defects Res., 2017, 109, 8–15 CrossRef CAS PubMed.
- Y. Y. Lam, W. Keung, C. H. Chan, L. Geng, N. Wong, D. Brenière-Letuffe, R. A. Li and Y. F. Cheung, J. Am. Heart Assoc., 2020, 9, e016528 CrossRef CAS PubMed.
- N. Liu, Y. Ruan and S. G. Priori, Prog. Cardiovasc. Dis., 2008, 51, 23–30 CrossRef PubMed.
-
C. Napolitano, A. Mazzanti, R. Bloise and S. G. Priori, Catecholaminergic Polymorphic Ventricular Tachycardia, 1993 Search PubMed.
- S. J. Park, D. Zhang, Y. Qi, Y. Li, K. Y. Lee, V. J. Bezzerides, P. Yang, S. Xia, S. L. Kim, X. Liu, F. Lu, F. S. Pasqualini, P. H. Campbell, J. Geva, A. E. Roberts, A. G. Kleber, D. J. Abrams, W. T. Pu and K. K. Parker, Circulation, 2019, 140, 390–404 CrossRef CAS PubMed.
- H. Lau, T. Khosrawipour, P. Kocbach, H. Ichii, J. Bania and V. Khosrawipour, Pulmonology, 2021, 27, 110–115 CrossRef CAS PubMed.
-
N. Rezaei, Coronavirus Disease - COVID-19, Springer, 2021 Search PubMed.
- F. Bader, Y. Manla, B. Atallah and R. C. Starling, Heart Failure Rev., 2021, 26, 1–10 CrossRef CAS PubMed.
- M. Dolhnikoff, J. Ferreira Ferranti, R. A. de Almeida Monteiro, A. N. Duarte-Neto, M. Soares Gomes-Gouvêa, N. Viu Degaspare, A. Figueiredo Delgado, C. Montanari Fiorita, G. Nunes Leal, R. M. Rodrigues, K. Taverna Chaim, J. R. Rebello Pinho, M. Carneiro-Sampaio, T. Mauad, L. F. Ferraz da Silva, W. Brunow de Carvalho, P. H. N. Saldiva and E. Garcia Caldini, Lancet Child Adolesc. Health, 2020, 4, 790–794 CrossRef PubMed.
- A. L. Bailey, O. Dmytrenko, L. Greenberg, A. L. Bredemeyer, P. Ma, J. Liu, V. Penna, E. S. Winkler, S. Sviben, E. Brooks, A. P. Nair, K. A. Heck, A. S. Rali, L. Simpson, M. Saririan, D. Hobohm, W. T. Stump, J. A. Fitzpatrick, X. Xie, X. Zhang, P. Y. Shi, J. T. Hinson, W. T. Gi, C. Schmidt, F. Leuschner, C. Y. Lin, M. S. Diamond, M. J. Greenberg and K. J. Lavine, JACC Basic Transl. Sci., 2021, 6, 331–345 CrossRef PubMed.
-
R. Xing, Z. Lu, N. Rafatian, Y. Zhao, K. T. Wagner and E. L. Beroncal, bioRxiv, 2023, preprint, DOI:10.1101/2023.08.09.552495.
- B. F. L. Lai, L. D. Huyer, R. X. Z. Lu, S. Drecun, M. Radisic and B. Zhang, Adv. Funct. Mater., 2017, 27, 1703524 CrossRef.
- A. Marsano, C. Conficconi, M. Lemme, P. Occhetta, E. Gaudiello, E. Votta, G. Cerino, A. Redaelli and M. Rasponi, Lab Chip, 2016, 16, 599–610 RSC.
- R. J. Mills, H. K. Voges, E. R. Porrello and J. E. Hudson, Curr. Opin. Physiol., 2018, 1, 80–88 CrossRef.
- S. Shen, L. R. Sewanan and S. G. Campbell, Evidence for synergy between sarcomeres and fibroblasts in an in vitro model of myocardial reverse remodeling, J. Mol. Cell. Cardiol., 2021, 158, 11–25 CrossRef CAS PubMed.
- H. Parsa, B. Z. Wang and G. Vunjak-Novakovic, Lab Chip, 2017, 17, 3264–3271 RSC.
- P. Perel, I. Roberts, E. Sena, P. Wheble, C. Briscoe, P. Sandercock, M. Macleod, L. E. Mignini, P. Jayaram and K. S. Khan, Br. Med. J., 2007, 334, 197–200 CrossRef CAS PubMed.
- F. Fluri, M. K. Schuhmann and C. Kleinschnitz, Drug Des., Dev. Ther., 2015, 9, 3445–3454 CAS.
- J. C. Reynolds, J. C. Rittenberger and J. J. Menegazzi, Resuscitation, 2007, 74, 13–26 CrossRef CAS PubMed.
- I. W. Mak, N. Evaniew and M. Ghert, Am. J. Transl. Res., 2014, 6, 114–118 Search PubMed.
- P. Pound, S. Ebrahim, P. Sandercock, M. B. Bracken and I. Roberts, BMJ, 2004, 328, 514–517 CrossRef PubMed.
- L. Meigs, L. Smirnova, C. Rovida, M. Leist and T. Hartung, ALTEX, 2018, 35, 275–305 CrossRef PubMed.
- O. J. Wouters, M. McKee and J. Luyten, JAMA, J. Am. Med. Assoc., 2020, 323, 844–853 CrossRef PubMed.
- D. van Berlo, E. van de Steeg, H. E. Amirabadi and R. Masereeuw, Curr. Opin. Toxicol., 2021, 27, 8–17 CrossRef CAS.
- D. K. Wysowski and L. Swartz, Arch. Intern. Med., 2005, 165, 1363–1369 CrossRef PubMed.
- M. Wadman, Science, 2023, 379, 127–128 CrossRef CAS PubMed.
- P. Ovics, D. Regev, P. Baskin, M. Davidor, Y. Shemer, S. Neeman, Y. Ben-Haim and O. Binah, Int. J. Mol. Sci., 2020, 21, 1–42 Search PubMed.
- M. S. Ma, S. Sundaram, L. Lou, A. Agarwal, C. S. Chen and T. G. Bifano, Front. Bioeng. Biotechnol., 2023, 11, 1177688 CrossRef PubMed.
- A. Chramiec, D. Teles, K. Yeager, A. Marturano-Kruik, J. Pak, T. Chen, L. Hao, M. Wang, R. Lock, D. N. Tavakol, M. B. Lee, J. Kim, K. Ronaldson-Bouchard and G. Vunjak-Novakovic, Lab Chip, 2020, 20, 4357–4372 RSC.
- I. Goldfracht, Y. Efraim, R. Shinnawi, E. Kovalev, I. Huber, A. Gepstein, G. Arbel, N. Shaheen, M. Tiburcy, W. H. Zimmermann, M. Machluf and L. Gepstein, Acta Biomater., 2019, 92, 145–159 CrossRef CAS PubMed.
- A. Mathur, P. Loskill, K. Shao, N. Huebsch, S. G. Hong, S. G. Marcus, N. Marks, M. Mandegar, B. R. Conklin, L. P. Lee and K. E. Healy, Sci. Rep., 2015, 5, 8883 CrossRef CAS PubMed.
- N. Thavandiran, C. Hale, P. Blit, M. L. Sandberg, M. E. McElvain, M. Gagliardi, B. Sun, A. Witty, G. Graham, V. T. Do, M. A. Bakooshli, H. Le, J. Ostblom, S. McEwen, E. Chau, A. Prowse, I. Fernandes, A. Norman, P. M. Gilbert, G. Keller, P. Tagari, H. Xu, M. Radisic and P. W. Zandstra, Sci. Rep., 2020, 10, 6919 CrossRef CAS PubMed.
- W. Dou, A. Daoud, X. Chen, T. Wang, M. Malhi, Z. Gong, F. Mirshafiei, M. Zhu, G. Shan, X. Huang, J. T. Maynes and Y. Sun, Nano Lett., 2023, 23, 2321–2331 CrossRef CAS PubMed.
- Y. Tang, F. Tian, X. Miao, D. Wu, Y. Wang, H. Wang, K. You, Q. Li, S. Zhao and W. Wang, Biofabrication, 2023, 15, 015010 CrossRef PubMed.
- M. Abulaiti, Y. Yalikun, K. Murata, A. Sato, M. M. Sami, Y. Sasaki, Y. Fujiwara, K. Minatoya, Y. Shiba, Y. Tanaka and H. Masumoto, Sci. Rep., 2020, 10, 19201 CrossRef CAS PubMed.
- K. S. Bielawski, A. Leonard, S. Bhandari, C. E. Murry and N. J. Sniadecki, Tissue Eng., Part C, 2016, 22, 932–940 CrossRef CAS PubMed.
- M. E. Kupfer, W. H. Lin, V. Ravikumar, K. Qiu, L. Wang, L. Gao, D. B. Bhuiyan, M. Lenz, J. Ai, R. R. Mahutga, D. Townsend, J. Zhang, M. C. McAlpine, E. G. Tolkacheva and B. M. Ogle, Circ. Res., 2020, 127, 207–224 CrossRef CAS PubMed.
- S. Chikae, A. Kubota, H. Nakamura, A. Oda, A. Yamanaka, T. Akagi and M. Akashi, Biomed. Mater., 2021, 16, 025017 CrossRef CAS PubMed.
- O. Schneider, L. Zeifang, S. Fuchs, C. Sailer and P. Loskill, Tissue Eng., Part A, 2019, 25, 786–798 CrossRef CAS PubMed.
- N. Thavandiran, N. Dubois, A. Mikryukov, S. Massé, B. Beca, C. A. Simmons, V. S. Deshpande, J. P. McGarry, C. S. Chen, K. Nanthakumar, G. M. Keller, M. Radisic and P. W. Zandstra, Proc. Natl. Acad. Sci. U. S. A., 2013, 110, E4701 CrossRef PubMed.
- A. Grosberg, P. W. Alford, M. L. McCain and K. K. Parker, Lab Chip, 2011, 11, 4165–4173 RSC.
- I. Mannhardt, A. Eder, B. Dumotier, M. Prondzynski, E. Kr-amer, M. Traebert, F. Flenner, K. Stathopoulou, M. D. Lemoine, L. Carrier, T. Christ, T. Eschenhagen and A. Hansen, Toxicol. Sci., 2017, 158, 164–175 CrossRef CAS PubMed.
- G. Hasenfuss, L. A. Mulieri, B. J. Leavitt and N. R. Alpert, J. Mol. Cell. Cardiol., 1994, 26, 1461–1469 CrossRef CAS PubMed.
- A. Mügge, D. Posselt, U. Reimer, W. Schmitz and H. Scholz, Klin. Wochenschr., 1985, 63, 26–31 CrossRef.
- M. Bohm, I. Morano, B. Pieske, J. C. Ruegg, M. Wankerl, R. Zimmermann and E. Erdmann, Circ. Res., 1991, 68, 689–701 CrossRef CAS PubMed.
- F. H. H. Leenen, Y. K. Chan, D. L. Smith and R. A. Reeves, Clin. Pharmacol. Ther., 1988, 43, 519–528 CrossRef CAS PubMed.
- P. B. Massion, O. Feron, C. Dessy and J. L. Balligand, Circ. Res., 2003, 93, 388–398 CrossRef CAS PubMed.
- E. Y. Wang, U. Kuzmanov, J. B. Smith, W. Dou, N. Rafatian, B. F. L. Lai, R. X. Z. Lu, Q. Wu, J. Yazbeck, X. O. Zhang, Y. Sun, A. Gramolini and M. Radisic, J. Mol. Cell. Cardiol., 2021, 160, 97–110 CrossRef CAS PubMed.
- L. Ren, X. Zhou, R. Nasiri, J. Fang, X. Jiang, C. Wang, M. Qu, H. Ling, Y. Chen, Y. Xue, M. C. Hartel, P. Tebon, S. Zhang, H. J. Kim, X. Yuan, A. Shamloo, M. R. Dokmeci, S. Li, A. Khademhosseini, S. Ahadian and W. Sun, Small Methods, 2020, 4, 2000438 CrossRef CAS PubMed.
- K. L. Miller, I. Sit, Y. Xiang, J. Wu, J. Pustelnik, M. Tang, W. Kiratitanaporn, V. Grassian and S. Chen, Bioprinting, 2023, 32, e00284 CrossRef.
- L. R. Sewanan, S. Shen and S. G. Campbell, Am. J. Physiol., 2021, 320, H1112–H1123 CrossRef CAS PubMed.
- M. Prondzynski, M. D. Lemoine, A. T. Zech, A. Horváth, V. Di Mauro, J. T. Koivumäki, N. Kresin, J. Busch, T. Krause, E. Krämer, S. Schlossarek, M. Spohn, F. W. Friedrich, J. Münch, S. D. Laufer, C. Redwood, A. E. Volk, A. Hansen, G. Mearini, D. Catalucci, C. Meyer, T. Christ, M. Patten, T. Eschenhagen and L. Carrier, EMBO Mol. Med., 2019, 11, e11115 CrossRef CAS PubMed.
- J. Van Der Velden, E. R. Witjas-Paalberends, G. J. M. Stienen, C. Dos Remedios, F. J. Ten Cate, C. Y. Ho, M. Michels and C. Poggesi, Eur. Heart J., 2013, 34, P4192 CrossRef.
- E. R. Witjas-Paalberends, A. Güclü, T. Germans, P. Knaapen, H. J. Harms, A. M. Vermeer, I. Christiaans, A. A. Wilde, C. DosRemedios, A. A. Lammertsma, A. C. Van Rossum, G. J. Stienen, M. Van Slegtenhorst, A. F. Schinkel, M. Michels, C. Y. Ho, C. Poggesi and J. Van Der Velden, Cardiovasc. Res., 2014, 103, 248–257 CrossRef CAS PubMed.
- C. G. Tocchetti, C. Cadeddu, D. Di Lisi, S. Femminò, R. Madonna, D. Mele, I. Monte, G. Novo, C. Penna, A. Pepe, P. Spallarossa, G. Varricchi, C. Zito, P. Pagliaro and G. Mercuro, Antioxid. Redox Signaling, 2019, 30, 2110–2153 CrossRef CAS PubMed.
- J. P. Piccini, D. J. Whellan, B. R. Berridge, J. K. Finkle, S. D. Pettit, N. Stockbridge, J. P. Valentin, H. M. Vargas and M. W. Krucoff, Am. Heart J, 2009, 158, 317–326 CrossRef PubMed.
- H. G. Laverty, C. Benson, E. J. Cartwright, M. J. Cross, C. Garland, T. Hammond, C. Holloway, N. McMahon, J. Milligan, B. K. Park, M. Pirmohamed, C. Pollard, J. Radford, N. Roome, P. Sager, S. Singh, T. Suter, W. Suter, A. Trafford, P. G. Volders, R. Wallis, R. Weaver, M. York and J. P. Valentin, Br. J. Pharmacol., 2011, 163, 675–693 CrossRef CAS PubMed.
- P. Mladěnka, L. Applová, J. Patočka, V. M. Costa, F. Remiao, J. Pourová, A. Mladěnka, J. Karlíčková, L. Jahodář, M. Vopršalová, K. J. Varner and M. Štěrba, Med. Res. Rev., 2018, 38, 1332–1403 CrossRef PubMed.
- P. Jüni, L. Nartey, S. Reichenbach, R. Sterchi, P. A. Dieppe and P. M. Egger, Lancet, 2004, 364, 2021–2029 CrossRef PubMed.
- S. M. London, BMJ, 2005, 330, 212 CrossRef.
- D. J. Graham, D. Campen, R. Hui, M. Spence, C. Cheetham, G. Levy, S. Shoor and W. A. Ray, Lancet, 2005, 365, 475–481 CrossRef CAS PubMed.
- N. E. Oyunbaatar, Y. Dai, A. Shanmugasundaram, B. K. Lee, E. S. Kim and D. W. Lee, ACS Sens., 2019, 4, 2623–2630 CrossRef CAS PubMed.
- D. S. Kim, Y. W. Choi, A. Shanmugasundaram, Y. J. Jeong, J. Park, N. E. Oyunbaatar, E. S. Kim, M. Choi and D. W. Lee, Nat. Commun., 2020, 11, 535 CrossRef CAS PubMed.
- R. Visone, G. S. Ugolini, D. Cruz-Moreira, S. Marzorati, S. Piazza, E. Pesenti, A. Redaelli, M. Moretti, P. Occhetta and M. Rasponi, Biofabrication, 2021, 13, 035026 CrossRef PubMed.
- X. Zhang, T. Wang, P. Wang and N. Hu, Micromachines, 2016, 7, 122 CrossRef PubMed.
- N. Shaheen, A. Shiti, I. Huber, R. Shinnawi, G. Arbel, A. Gepstein, N. Setter, I. Goldfracht, A. Gruber, S. V. Chorna and L. Gepstein, Stem Cell Rep., 2018, 10, 1879–1894 CrossRef CAS PubMed.
- I. Itzhaki, L. Maizels, I. Huber, L. Zwi-Dantsis, O. Caspi, A. Winterstern, O. Feldman, A. Gepstein, G. Arbel, H. Hammerman, M. Boulos and L. Gepstein, Nature, 2011, 471, 225–230 CrossRef CAS PubMed.
- A. Faulkner-Jones, V. Zamora, M. P. Hortigon-Vinagre, W. Wang, M. Ardron, G. L. Smith and W. Shu, Bioengineering, 2022, 9, 32 CrossRef CAS PubMed.
- A. H. Soepriatna, A. Navarrete-Welton, T. Y. Kim, M. C. Daley, P. Bronk, C. M. Kofron, U. Mende, K. L. Coulombe and B. R. Choi, PLoS One, 2023, 18, e0280406 CrossRef CAS PubMed.
- O. Caspi, I. Itzhaki, I. Kehat, A. Gepstein, G. Arbel, I. Huber, J. Satin and L. Gepstein, Stem Cells Dev., 2009, 18, 161–172 CrossRef CAS PubMed.
- S. R. Braam, L. Tertoolen, A. van de Stolpe, T. Meyer, R. Passier and C. L. Mummery, Stem Cell Res., 2010, 4, 107–116 CrossRef CAS PubMed.
- K. C. Weng, Y. K. Kurokawa, B. S. Hajek, J. A. Paladin, V. S. Shirure and S. C. George, Tissue Eng., Part C, 2020, 26, 44–55 CrossRef CAS PubMed.
- C. Oleaga, A. Riu, S. Rothemund, A. Lavado, C. W. McAleer, C. J. Long, K. Persaud, N. S. Narasimhan, M. Tran, J. Roles, C. A. Carmona-Moran, T. Sasserath, D. H. Elbrecht, L. Kumanchik, L. R. Bridges, C. Martin, M. T. Schnepper, G. Ekman, M. Jackson, Y. I. Wang, R. Note, J. Langer, S. Teissier and J. J. Hickman, Biomaterials, 2018, 182, 176–190 CrossRef CAS PubMed.
- D. Cardinale, F. Iacopo and C. M. Cipolla, Front. Cardiovasc. Med., 2020, 7, 26 CrossRef CAS PubMed.
- P. Soltantabar, E. L. Calubaquib, E. Mostafavi, A. Ghazavi and M. C. Stefan, Organs-on-a-Chip, 2021, 3, 100008 CrossRef CAS.
- A. Rhoden, F. W. Friedrich, T. Brandt, J. Raabe, M. Schweizer, J. Meisterknecht, I. Wittig, B. M. Ulmer, B. Klampe, J. Uebeler, A. Piasecki, K. Lorenz, T. Eschenhagen, A. Hansen and F. Cuello, Redox Biol., 2021, 41, 101951 CrossRef CAS PubMed.
- C. Fimognari and P. Hrelia, Mutat. Res., Rev. Mutat. Res., 2007, 635, 90–104 CrossRef CAS PubMed.
- A. Jaskulska, A. E. Janecka and K. Gach-Janczak, Int. J. Mol. Sci., 2021, 22, 1–12 Search PubMed.
- G. Conant, S. Ahadian, Y. Zhao and M. Radisic, Sci. Rep., 2017, 7, 11807 CrossRef PubMed.
- P. Cohen, D. Cross and P. A. Jänne, Nat. Rev. Drug Discovery, 2021, 20, 551–569 CrossRef CAS PubMed.
- S. D. Lamore, H. W. Kamendi, C. W. Scott, Y. P. Dragan and M. F. Peters, Toxicol. Sci., 2013, 135, 402–413 CrossRef CAS PubMed.
- R. I. Aminov, Front. Microbiol., 2010, 1, 134 Search PubMed.
- C. P. Pires De Mello, C. Carmona-Moran, C. W. McAleer, J. Perez, E. A. Coln, C. J. Long, C. Oleaga, A. Riu, R. Note, S. Teissier, J. Langer and J. J. Hickman, Lab Chip, 2020, 20, 749–759 RSC.
- B. Charrez, V. Charwat, B. Siemons, H. Finsberg, E. W. Miller, A. G. Edwards and K. E. Healy, Clin. Transl. Sci., 2021, 14, 1155–1165 CrossRef CAS PubMed.
- H. Xu, G. Liu, J. Gong, Y. Zhang, S. Gu, Z. Wan, P. Yang, Y. Nie, Y. Wang, Z. p. Huang, G. Luo, Z. Chen, D. Zhang and N. Cao, Adv. Sci., 2022, 9, 2203388 CrossRef CAS PubMed.
- L. Bull-Otterson, E. B. Gray, D. S. Budnitz, H. M. Strosnider, L. Z. Schieber, J. Courtney, M. C. García, J. T. Brooks, W. R. Mac Kenzie and A. V. Gundlapalli, Morb. Mortal. Wkly. Rep., 2020, 69, 1210–1215 CrossRef CAS PubMed.
- J. M. Molina, C. Delaugerre, J. Le Goff, B. Mela-Lima, D. Ponscarme, L. Goldwirt and N. de Castro, Med. Mal. Infect., 2020, 50, 384 CrossRef CAS PubMed.
- O. Atli, S. Ilgin, H. Altuntas and D. Burukoglu, Int. J. Clin. Exp. Med., 2015, 8, 3681–3690 Search PubMed.
- E. Joyce, A. Fabre and N. Mahon, Eur. Heart J. Acute Cardiovasc. Care, 2013, 2, 77–83 CrossRef PubMed.
- A. O. T. Wong, B. Gurung, W. S. Wong, S. Y. Mak, W. W. Tse, C. M. Li, D. K. Lieu, K. D. Costa, R. A. Li and R. J. Hajjar, J. Mol. Cell. Cardiol., 2021, 153, 106–110 CrossRef CAS PubMed.
- F. Yin, X. Zhang, L. Wang, Y. Wang, Y. Zhu, Z. Li, T. Tao, W. Chen, H. Yu and J. Qin, Lab Chip, 2021, 21, 571–581 RSC.
- B. Z. Wang, T. R. Nash, X. Zhang, J. Rao, L. Abriola, Y. Kim, S. Zakharov, M. Kim, L. J. Luo, M. Morsink, B. Liu, R. I. Lock, S. Fleischer, M. A. Tamargo, M. Bohnen, C. L. Welch, W. K. Chung, S. O. Marx, Y. V. Surovtseva, G. Vunjak-Novakovic and B. M. Fine, Cell Rep. Med., 2023, 100976 CrossRef CAS PubMed.
- S. Ahn, H. A. M. Ardoña, J. U. Lind, F. Eweje, S. L. Kim, G. M. Gonzalez, Q. Liu, J. F. Zimmerman, G. Pyrgiotakis, Z. Zhang, J. Beltran-Huarac, P. Carpinone, B. M. Moudgil, P. Demokritou and K. K. Parker, Anal. Bioanal. Chem., 2018, 410, 6141–6154 CrossRef CAS PubMed.
- A. Pointon, J. Pilling, T. Dorval, Y. Wang, C. Archer and C. Pollard, Toxicol. Sci., 2017, 155, 444–457 CrossRef CAS PubMed.
- H. Ando, T. Yoshinaga, W. Yamamoto, K. Asakura, T. Uda, T. Taniguchi, A. Ojima, T. Osada, S. Hayashi, C. Kasai, N. Miyamoto, H. Tashibu, D. Yamazaki, A. Sugiyama, Y. Kanda, K. Sawada, Y. Sekino, H. Ando, T. Yoshinaga, K. Asakura, T. Osada, S. Hayashi, C. Kasai, H. Tashibu, A. Sugiyama, K. Sawada, Y. Sekino, H. Ando, T. Uda, T. Yoshinaga, T. Taniguchi, A. Ojima, R. Shinkyo, K. Kikuchi, N. Miyamoto, K. Sawada, W. Yamamoto, K. Asakura, S. Hayashi, T. Osada, C. Kasai, H. Tashibu, D. Yamazaki, Y. Kanda, Y. Sekino and A. Sugiyama, J. Pharmacol. Toxicol. Methods, 2017, 84, 111–127 CrossRef CAS PubMed.
- W. Keung, P. K. Chan, P. C. Backeris, E. K. Lee, N. Wong, A. O. Wong, G. K. Wong, C. W. Chan, B. Fermini, K. D. Costa and R. A. Li, Clin. Pharmacol. Ther., 2019, 106, 402–414 CrossRef CAS PubMed.
- J. U. Lind, M. Yadid, I. Perkins, B. B. O'Connor, F. Eweje, C. O. Chantre, M. A. Hemphill, H. Yuan, P. H. Campbell, J. J. Vlassak and K. K. Parker, Lab Chip, 2017, 17, 3692–3703 RSC.
- P. Loskill, S. G. Marcus, A. Mathur, W. M. Reese and K. E. Healy, PLoS One, 2015, 10, e0139587 CrossRef PubMed.
- E. Ferrari and M. Rasponi, APL Bioeng., 2021, 5, 031505 CrossRef CAS PubMed.
- F. T. Lee-Montiel, A. Laemmle, V. Charwat, L. Dumont, C. S. Lee, N. Huebsch, H. Okochi, M. J. Hancock, B. Siemons, S. C. Boggess, I. Goswami, E. W. Miller, H. Willenbring and K. E. Healy, Front. Pharmacol., 2021, 12, 667010 CrossRef CAS PubMed.
- C. W. McAleer, A. Pointon, C. J. Long, R. L. Brighton, B. D. Wilkin, L. R. Bridges, N. Narasimhan Sriram, K. Fabre, R. McDougall, V. P. Muse, J. T. Mettetal, A. Srivastava, D. Williams, M. T. Schnepper, J. L. Roles, M. L. Shuler, J. J. Hickman and L. Ewart, Sci. Rep., 2019, 9, 9619 CrossRef PubMed.
- K. I. Kamei, Y. Kato, Y. Hirai, S. Ito, J. Satoh, A. Oka, T. Tsuchiya, Y. Chen and O. Tabata, RSC Adv., 2017, 7, 36777–36786 RSC.
- C. W. McAleer, C. J. Long, D. Elbrecht, T. Sasserath, L. R. Bridges, J. W. Rumsey, C. Martin, M. Schnepper, Y. Wang, F. Schuler, A. B. Roth, C. Funk, M. L. Shuler and J. J. Hickman, Sci. Transl. Med., 2019, 11, eaav1386 CrossRef CAS PubMed.
- D. R. Reyes, H. van Heeren, S. Guha, L. Herbertson, A. P. Tzannis, J. Ducree, H. Bissig and H. Becker, Lab Chip, 2021, 21, 9–21 RSC.
- M. Piergiovanni, S. B. Leite, R. Corvi and M. Whelan, Lab Chip, 2021, 21, 2857–2868 RSC.
-
North American 3Rs Collaborative, Microphysiological Systems Initiative, 2023, https://www.na3rsc.org/mps/, (accessed January 2024) Search PubMed.
- D. Pamies, M. Leist, S. Coecke, G. Bowe, D. G. Allen, G. Gstraunthaler, A. Bal-Price, F. Pistollato, R. B. M. de Vries, H. T. Hogberg, T. Hartung and G. Stacey, ALTEX, 2022, 39, 30–70 Search PubMed.
- T. Hartung, ALTEX, 2017, 34, 193–200 CrossRef PubMed.
- Organ-on-a-Chip/Tissue-on-a-Chip Engineering and Efficacy Standardization Working Group, https://www.nist.gov/pml/microsystems-and-nanotechnology-division/biophysical-and-biomedical-measurement-group/organ, (accessed January 2024).
- CEN-CENELEC, Organ on Chip, https://www.cencenelec.eu/areas-of-work/cen-cenelec-topics/organ-on-chip/, (accessed January 2024).
- European Organ-on-Chip Society (EUROoCS), https://euroocs.eu, (accessed January 2024).
- D. R. Reyes and H. van Heeren, J. Res. Natl. Inst. Stand. Technol., 2019, 124, 124001 CrossRef CAS PubMed.
- ISO 22916:2022 Microfluidic devices — Interoperability requirements for dimensions, connections and initial device classification, https://www.iso.org/standard/74157.html (accessed September 2023).
- ISO 10991:2023 Microfluidics – Vocabulary, https://www.iso.org/standard/82146.html (accessed September 2023).
- K. Breckwoldt, D. Letuffe-Breniere, I. Mannhardt, T. Schulze, B. Ulmer, T. Werner, A. Benzin, B. Klampe, M. C. Reinsch, S. Laufer, A. Shibamiya, M. Prondzynski, G. Mearini, D. Schade, S. Fuchs, C. Neuber, E. Kraemer, U. Saleem, M. L. Schulze, M. L. Rodriguez, T. Eschen-hagen and A. Hansen, Nat. Protoc., 2017, 12, 1177–1197 CrossRef CAS PubMed.
- H. Tani, E. Kobayashi, S. Yagi, K. Tanaka, K. Kameda-Haga, S. Shibata, N. Moritoki, K. Takatsuna, T. Moriwaki, O. Sekine, T. C. Umei, Y. Morita, Y. Soma, Y. Kishino, H. Kanazawa, J. Fujita, S. Hattori and S. Tohyama, Biomaterials, 2023, 299, 122174 CrossRef CAS PubMed.
- O. Pagliarosi, V. Picchio, I. Chimenti, E. Messina and R. Gaetani, Front. Cell Dev. Biol., 2020, 8, 559032 CrossRef PubMed.
- M. D. Lemoine, I. Mannhardt, K. Breckwoldt, M. Prondzynski, F. Flenner, B. Ulmer, M. N. Hirt, C. Neuber, A. Horvath, B. Kloth, H. Reichenspurner, S. Willems, A. Hansen, T. Eschenhagen and T. Christ, Sci. Rep., 2017, 7, 5464 CrossRef PubMed.
- H. Vuorenpää, M. Bjöerninen, H. Välimäki, A. Ahola, M. Kroon, L. Honkamäki, J. T. Koivumäki and M. Pekkanen-Mattila, Front. Physiol., 2023, 14, 1213959 CrossRef PubMed.
-
World Health Organization, Coronavirus disease (COVID-19): Hydroxychloroquine, https://www.who.int/news-room/questions-and-answers/item/coronavirus-disease-(covid-19)444-hydroxychloroquine, (accessed September 2023) Search PubMed.
|
This journal is © The Royal Society of Chemistry 2024 |