Seasonal and spatial patterns differ between intracellular and extracellular antibiotic resistance genes in urban stormwater runoff†
Received
7th April 2022
, Accepted 17th June 2022
First published on 17th June 2022
Abstract
Antibiotic resistance is a public health threat that is exacerbated by the dispersion of antibiotic resistance genes (ARGs) into aquatic environments. Urban stormwater runoff has been recognized as a source of and a mechanism by which intracellular ARGs (iARGs) can be transported into receiving environments. The prevalence and behavior of extracellular ARGs (eARGs) in stormwater, however, has yet to be considered. This study quantified four iARGs and eARGs under baseflow and stormflow conditions. Urban stormwater runoff was found to be a source of all the ARGs examined, with the absolute concentration of all iARGs and two eARGs (ermF and tetC) increasing significantly (p < 0.05) between baseflow and stormflow. The occurrence of iARGs and eARGs in stormwater runoff was also investigated across three seasons to differentiate temporal trends. All eARGs were found to be most abundant in the fall, while the iARGs did not display a consistent seasonal trend. Following, spatial patterns of the ARGs were elucidated by targeting four sampling locations, including direct runoff from a curbside storm inlet and a stormwater outfall, and two receiving environments, the Menomonee River and Lake Michigan. Stormwater was found to have the largest impact, in terms of the percent increase in ARG concentrations between baseflow and stormflow, on the outfall location where on average the iARGs and eARGs increased 16% and 12.3%, respectively. The variability in seasonal and spatial trends between the iARGs and eARGs suggests a difference in sources and transport mechanisms of the ARGs into the environment. Moreover, the results of this study revealed that eARGs are relevant contaminants to consider when determining the threat of antibiotic resistance originating from stormwater runoff.
Environmental significance
Research aiming to characterize the spread of antibiotic resistance in the environment has largely overlooked the role of extracellular antibiotic resistance genes (eARGs). eARGs are especially relevant in the propagation of resistance because they can be acquired by living cells via transformation; however, the prevalence of eARGs in many aquatic environments has yet to be evaluated, particularly in urban stormwater. Pollution transported via stormwater runoff is a major concern for urban environments as receiving water bodies are plagued with increases in solids, nutrients, and other unfavorable contaminants. To investigate the presence and behavior of eARGs in stormwater runoff, we assessed the abundance of eARG comparatively to intracellular ARGs across three seasons at four stormwater infrastructure locations in an urban watershed.
|
Introduction
Antibiotics are life-saving medicines that are being rendered ineffective by antibiotic resistant bacteria (ARB). ARB can acquire resistance through ARGs, i.e., naturally occurring genetic elements that, when integrated into a bacterial cell, confer resistance to one or more antibiotics.1 ARB and ARGs, and other resistance genetic elements, such as mobile genetic elements (MGEs) and plasmids, can be transferred on a global scale.2 In response, there is a need to identify routes of ARG dissemination that facilitate resistance development and threaten human health. Input sources known to contain elevated levels of ARGs include municipal, industrial, hospital, and agricultural point and nonpoint waste streams.3 Fecal contamination from domestic and agrarian animals and land applied wastewater derived biosolids have also been shown to contain high levels of ARGs.3 More recently, stormwater has been identified as a source of ARGs, with urban runoff specifically found to be abundant in ARGs at levels comparable to wastewater effluent.4,5
Stormwater could potentially transport ARB and ARGs from diverse environmental niches across an entire watershed. Urban watersheds can transport pollutants in stormwater runoff, and thus, urban stormwater runoff is likely enabling the large-scale mixing of resistance elements from environments that are otherwise disconnected.6,50 Urban stormwater is also a vector of many other contaminants, including heavy metals, pesticides, nutrients, and sediments.7,8 Such pollutants are known to select for or correlate with the occurrence of ARGs and ARB.9–11 The incidence of environmental stressors with indigenous and exogenous bacteria, including human commensal bacteria and pathogens, presents the possibility of an increased prevalence of ARGs and ARB in stormwater runoff and receiving environments throughout an urban watershed.
Previous research on the occurrence of ARGs in stormwater found elevated concentrations of iARGs in direct runoff and in surface waters impacted by urban stormwater runoff when compared with baseflow or dry weather conditions.12–19 iARGs are a concern due to their persistence and stability in nutrient-rich environments with complex microbial community compositions that enable their transfer via conjugation.20 eARGs are also of concern because they are highly transferable and in environments with a great abundance of eARGs, transfer via transformation directly increases the number ARB.13–16,19 While eARGs have been reported as an insignificant fraction of the total ARGs of a microbial community within aquatic waters,21,22 they are more prevalent in soils, sediments, and wastewater sludge environments due to their affinity to bind to particles, colloids, and other organic molecules.20,21,23,24 Consequently, as stormwater transports sediments and soil from the urban environment, it is hypothesized that it also conveys eARGs. However, to date no other studies have quantified the concentrations of eARGs in stormwater runoff.
In order to design stormwater infrastructure that mitigates ARGs in urban runoff, fluctuations on a seasonal basis must be identified and incorporated into the proposed strategies. This is because stormwater exhibits seasonal trends for different water constituents, including water temperature, pH, conductivity, dissolved oxygen, metals, and turbidity,25,26 that are known to affect microbial concentrations and processes.27,28 Accordingly, the abundances of microorganisms have shown seasonal variations in stormwater. For example, the concentrations of Enterococci, E. coli, Pseudomonas aeruginosa, and Staphylococcus aureus were found to be decreased in winter stormwater runoff when compared to fall, spring, and summer.29 The bacteria quantified by Selvakumar and Borst commonly developed resistance and have been identified in stormwater; however, seasonal differences of the ARGs present in urban stormwater runoff have yet to be elucidated.29,30
The objectives of this study were to (1) determine the extent of eARG pollution originating from urban stormwater runoff, (2) define seasonal change in the prevalence of iARGs and eARGs in stormwater, and (3) establish the impact of stormwater infrastructure on the abundance of iARGs and eARGs in stormwater. To achieve these objectives, stormflow samples were taken at four locations (storm drain inlet, stormwater outfall, receiving river, and receiving lake), during a storm event in the fall, winter, and spring with baseflow conditions characterized at the outfall, river, and lake during a dry period preceding the storm event. The intracellular and extracellular fraction of the 16S rRNA gene, the MGE intI1, and ARGs ermF, blaTEMsul1, and tetC were quantified in each sample via droplet digital polymerase chain reaction (ddPCR) to assess the risk of such contaminants by defining changes in abundance during a year and across locations. In doing so, this was the first study to present the concentration of eARGs in stormwater and the first time that i-tetC has been quantified in stormwater. It was hypothesized that (1) across the four sampling locations there would be a decreasing trend in the absolute abundance of the iARGs and eARGs along the hydraulic flow path from the storm drain to the receiving lake due to dilution, and (2) seasonally the iARGs and eARGs will be the most abundant in the spring and the least abundant in the winter, based upon the trends exhibited by bacteria.
Methods
Study area and sampling events
Sampling targeted four key stormwater infrastructure locations, shown in Fig. S1.† The first, a curbside grated stormwater inlet, drained a 4500 m2 area of a street. Runoff to the stormwater inlet is conveyed to an underground stormwater pipe network that discharges to a stormwater outfall, the second sampling location. This outfall location collects runoff from a roughly 4.4 km2 drainage area and discharges to the Menomonee river.31 The third sampling location was in the river, downstream of the point that the water from the outfall reaches the river. The drainage area of the Menomonee Watershed is approximately 352 km2 and the land uses include urban (42%), grasslands (22%), and agriculture (17%).32 The final sample was taken downstream of the convergence of the Menomonee, Milwaukee, and Kinnikinic rivers in Lake Michigan. This location drains a basin of approximately 2260 km2 that contains about 800 km of perennial stream and over 640 km of intermittent streams.32
Three sampling events in different seasons were undertaken for this study. During each event, samples were captured under baseflow and stormflow conditions. Streamflow data was gathered from U.S. Geological Survey (USGS) stream gage 04087120 Menomonee River at Wauwatosa, WI (Fig. S4†). The fall baseflow and storm sampling occurred on November 5th, 2020, and November 10th, 2020, respectively. The average discharge in the river on the baseflow sampling day was 29.4 ± 0.47 cfs, while the peak storm discharge was 320 cfs. The winter baseflow and stormflow samples were taken on February 19th, 2021, and March 10th, 2021, respectively. Streamflow was not recorded by the USGS stream gage on the day the winter baseflow was captured due to ice conditions. The peak stream flow of the winter storm event was 504 cfs. The spring baseflow conditions were sampled on May 13th, 2021; the average daily stream discharge on this day was 59.04 ± 0.75 cfs. The spring storm event was sampled on May 23rd, 2021, and the storm's peak discharge was 225 cfs.
Sample collection and DNA extraction
Grab samples were collected at the stormwater inlet (only during stormflow sampling), the stormwater outfall, in the Menomonee River, and in Lake Michigan at a surface water depth of 0–2 feet. Six one-liter samples were taken at each location for molecular (n = 3) and water quality analysis (n = 3). All samples were transported back to the lab and stored at 4 °C until processing. Intracellular DNA (iDNA) and extracellular DNA (eDNA) were extracted according to a previously published method, and the details can be found in the ESI.†5 Following. iDNA and eDNA extracts were stored at −20 °C until further analysis.
Antibiotic resistance gene quantification
Four ARGs, blaTEM, tetC, sul1, and ermF, the integrase gene of the class 1 integrons, intI1, and the 16S rRNA gene were quantified in the iDNA and eDNA extracts via ddPCR. ddPCR was conducted as previously described and the steps are detailed in the ESI.†33 The 16S rRNA gene was analyzed to quantify the total bacterial biomass of a sample. The MGE intI1 was quantified in this study to evaluate the potential for ARG dissemination by horizontal gene transfer. intI1 has also been suggested to be a marker of anthropogenic pollution.34 The four ARGs quantified confer resistance to four different classes of antibiotics: beta-lactams (blaTEM), tetracyclines (tetC), sulfonamides (sul1), and macrolides (ermF). Moreover, these four ARGs have been well documented in various environmental compartments35,36 and in different waste streams entering the environment37 with blaTEM, sul1, and ermF being classified as high priority target ARGs for furthering an understanding of environmental matrices.4
Water quality analysis
Following the transport of the water quality samples back to the lab, the temperature, pH, and conductivity of each sample was measured with Thermo Scientific Orion probes (Thermo Fisher Scientific, Waltham, MA). Total nitrogen (TN) was quantified with the Hach Total Nitrogen Reagent Set (0.5–25 mg N L−1) and total phosphorous (TP) was quantified with the Hach Phosphorous TNTplus Vial Tests, LR (0.15–4.50 mg L−1) according to manufacturer instructions. The concentration of total suspended solids (TSS) was determined according to Standard Method 2540D. US EPA Method 415.3 was utilized to quantify the dissolved organic carbon (DOC) with a TOC-VCSN analyzer (Shimadzu, Kyoto, JP) and ultraviolet-visible spectrophotometry (UV-Vis) with a GENESYS™ 50 UV-Vis spectrophotometer (Thermo Fisher Scientific, Waltham, MA). Lastly, metals were quantified via inductively coupled plasma mass spectrometry. Results of the water quality analysis can be found in Fig. S5–S12.†
Statistical analysis
QuantaSoft™ Analysis Pro Software (version 1.0.596) was used to analyze the ddPCR results in which the concentration (gene copies (GC) per μL) of the target genes was estimated by modeling the Poisson distribution based on the fraction of positive amplifications. Following, gene concentrations were log-transformed after the Shapiro–Wilk test (α = 0.05) confirmed non-normal distributions. Variances of the log transformed gene abundances within and between flow conditions, locations, and seasons were determined using one-way analysis of variance with the post hoc Tukey's multiple comparisons test. Significant relationships were assessed at a p-value ≤0.05. Unpaired t-tests of the average gene abundances between baseflow and stormflow were also conducted at a significance level of p ≤ 0.05. Correlation analysis was conducted to generate Pearson correlation coefficients and p-values between gene abundance and water quality data and produce heat map figures. All statistical analyses were performed, and figures were produced using GraphPad Prism 7® (GraphPad Software, La Jolla, CA).
Results
Impact of storm events on abundance of extracellular and intracellular genes assessed against baseflow conditions
The absolute abundance of the extracellular 16S rRNA, intI1, blaTEM, ermF, sul1, and tetC genes quantified during baseflow and stormflow conditions is shown in Fig. 1A. All extracellular genes were quantifiable in the stormwater samples with concentrations ranging from 2.69 × 105 to 7.59 × 109 GC per L. When the abundance of the extracellular genes in stormflow was compared to baseflow conditions, it was found that the concentration of two of the four eARGs in stormwater, e-ermF and e-tetC, was statistically greater than the concentration measured in the baseflow samples. The e-16S rRNA gene and the e-intI1 MGE also increased significantly. Among the extracellular genes quantified, the abundance of e-intI1 was the most impacted in stormwater, with a 1.18-fold change between baseflow and stormflow.
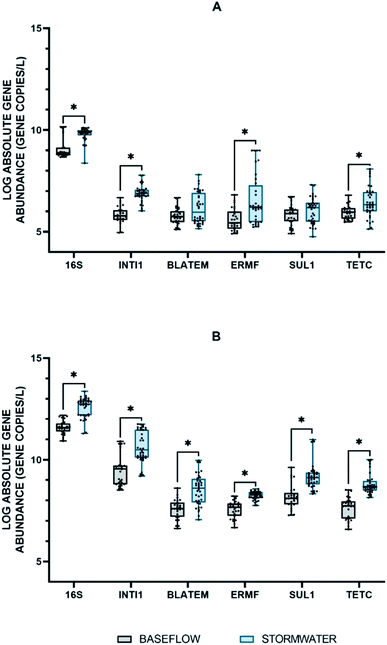 |
| Fig. 1 Box plots displaying the distribution of the absolute abundance of extracellular (A), and intracellular (B) genes quantified in samples taken during baseflow (n = 27) and stormflow (n = 36) conditions as measured with ddPCR. The asterisk indicates a statistically significant relationship, p-value <0.05, between the baseflow and stormwater abundances and was determine by a Student's t-test. | |
The intracellular fraction of the 16S rRNA, intI1, blaTEM, ermF, sul1, and tetC genes were also quantified in the baseflow and stormflow samples (Fig. 1B). A statistically significant increase in the average absolute concentration of all intracellular genes was observed between the baseflow and the stormwater samples with an average fold change of 1.12. i-tetC displayed the greatest increase under stormflow conditions with the average abundance increasing from 4.2 × 107 GC per L during baseflow to 6.3 × 108 GC per L; this is the first time that i-tetC has been quantified in stormwater.
Seasonal variability of iARGs and eARGs in stormwater
When the abundances of the extracellular and intracellular genes were evaluated across the three sampling events, corresponding to three seasons, no one season was found to have a statistically greater or lesser average for every intracellular or extracellular gene quantified under stormflow conditions, but there were differences in specific genes between seasons (Fig. 2). For the concentrations of the extracellular 16S rRNA gene and intI1 in stormwater no change was observed across the seasons. Variations in concentration of the eARGs (blaTEM, ermF, sul1, and tetC) were found across the season, but each gene was the most abundant in the fall. When each season is evaluated separately, e-ermF was found to be the most prevalent eARG in the fall storm samples, while e-sul1 was the most abundant in the winter and spring. For the intracellular genes, no consistent relationship was discovered across the three seasons in stormwater. For the fall and winter stormwater events, the most abundant iARG was i-sul1 and in the spring it was i-tetC.
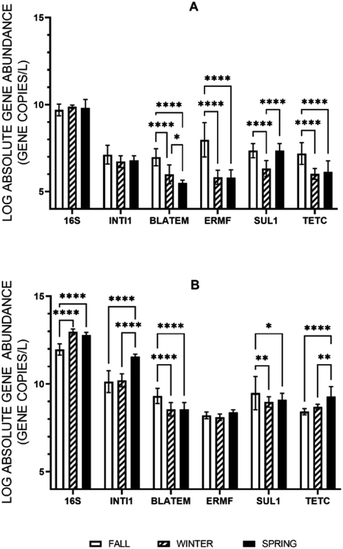 |
| Fig. 2 Absolute abundance of the extracellular (A) and intracellular (B) genes 16S rRNA, intI1, blaTEM, ermF, sul1, and tetC quantified in stormflow across three storm events as measured with ddPCR. The asterisk indicates a statistically significant relationship between seasons (one-way ANOVA with Tukey's posthoc test; *(p < 0.05), **(p < 0.01), ***(p < 0.001), and ****(p < 0.0001)) and the error bars represent the standard deviation of the mean (n = 12). | |
The average absolute concentration of all intracellular genes in stormwater across all seasons was statistically greater than the extracellular genes. Subsequently, the relative abundance of the intracellular and extracellular genes was determined through normalization of the gene concentrations to that of the i-16S rRNA and e-16S rRNA genes, respectively (Fig. 3). Significantly, the relative abundance of e-ermF and e-tetC were not statistically different than i-ermF and i-tetC, respectively, in each stormwater sample. The intracellular fraction of blaTEM and sul1 were only statistically greater than the extracellular fraction in the fall samples, while the relative abundance of the intI1 MGE was the only gene in which the intracellular fraction was statistically greater than the extracellular fraction in each storm event.
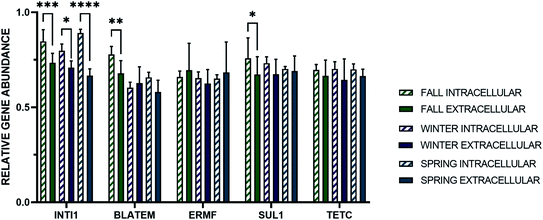 |
| Fig. 3 Relative gene abundance of the intracellular and extracellular fraction of intI1, blaTEM, ermF, sul1, and tetC in stormwater samples normalized to the i-16S rRNA and e-16S RNA genes, respectively. The asterisk indicates a statistically significant relationship between the intracellular and extracellular relative concentrations (Student's t-test, p-value <0.05) and the error bars represent the standard deviation of the mean (n = 12). | |
Following, the percent difference in the average absolute ARG concentrations between baseflow and stormflow was calculated to determine in which season stormwater had the greatest deviation from baseline conditions. In this case, the iARGs and eARGs exhibited the same trend as on average the genes in stormflow were found to increase 19% and 18% in the fall, 13% and 12% in the spring, and 6% and 9% in the winter, respectively, across all locations. This analysis was then extended to differentiate between the different iARGs and eARGs. Every iARG and eARG was found to follow the same trend with the percent increase being greatest in the fall followed by the spring and then the winter, except for the e-blaTEM gene (fall > winter > spring).
Seasonality of the intracellular and extracellular genes in baseflow and stormflow was then comparatively analyzed across the sampling locations. For the intracellular genes specifically, more variance across the seasons was observed in the stormflow samples than baseflow (Fig. S2†). Under stormflow conditions, the concentrations of i-16S rRNA, i-intI1, i-blaTEM, and i-tetC displayed several seasonal changes while concentrations of i-ermF and i-sul1 were less prone to seasonal variability. The four intracellular genes that were seasonally variable in stormwater, however, did not exhibit consistent shifts between the genes or among the sampling locations. i-blaTEM, for example, at all locations was most abundant in the fall and least abundant in the winter. The i-16S rRNA, i-intI1 i-ermF, and i-tetC genes, on the other hand, were most abundant in the spring at either three or four of the four locations. i-sul1 was not consistently elevated at one location, with the greatest concentrations at the inlet and river found in the fall and at the outlet and lake samples in the spring.
The e-16S rRNA gene was the only gene to be more seasonally variable under baseflow conditions than in stormflow (Fig. S3†). For the other extracellular genes quantified, similar to the intracellular genes, baseflow concentrations were not subject to seasonal fluctuations. The seasonality of eARGs in baseflow, or lack thereof, greatly differs from their occurrence in stormflow. The abundances of e-blaTEM, e-ermF, e-sul1 and e-tetC were all subject to seasonal shifts across all locations in stormflow. The e-intI1 MGE, did not show great variability over the seasons but was primarily most abundant in the fall (five of seven locations). This trend is carried throughout as all eARG in stormflow in the fall at all locations were statistically greater than in the winter and spring, except in two cases, e-tetC and e-sul1 in the lake stormwater sample. No clear trend, however, is observed for eARGs at the sampling locations between the winter and spring stormwater samples.
Spatial variability of iARGs and eARGs in stormwater
Four hydrologically connected locations were sampled at in this study, a stormwater inlet, outfall, a river, and lake. Among those locations, three were sampled under both baseflow and stormflow, the exception being the inlet which could not be sampled for baseflow. The average concentrations of the intracellular and extracellular genes quantified and their statistical relationships across the sampling locations can be found in Fig. 4 and 5. Statistically, many different relationships over the three baseflow locations and four stormflow locations existed that were specific to the individual genes in each storm event. In general, the iARGs and eARGs were less variable, showing no statistical difference across the locations, in baseflow (e-sul1 and i-tetC in the fall, i-blaTEM, i/e-ermF, e-sul1, and e-tetC in the winter, as well as e-blaTEM, e-ermF, i-sul1, and e-tetC in the spring) when compared to stormflow (e-sul1 in the fall, i-ermF and i/e-tetC in the winter, and i-ermF and e-blaTEM in the spring). It was hypothesized that the ARG concentrations would follow a decreasing trend on account of dilution caused by the increasing size of the water bodies being sampled. However, a decreasing trend was only exhibited by i-sul1 in the fall under baseflow and stormflow conditions. An increasing trend across the locations was more common and was observed in baseflow (i-blaTEM, i-ermF, and i-sul1 in the fall, i-ermF i-tetC e-sul1 and e-tetC in spring) and stormflow (i-blaTEM and e-tetC in the spring and e-sul1 in the winter). In stormflow specifically, the abundance of intracellular genes at the stormwater inlet was the outlying location, with nine instances of a statistical difference between the inlet and outlet and seven instances of a statistical difference between the inlet and both the river and lake. The extracellular genes in stormflow were variable across the four locations, with the exception of the inlet and outlet, which only had three cases (e-ermF in the fall and spring and e-sul1 in the winter) of a statistical difference between the average gene concentrations.
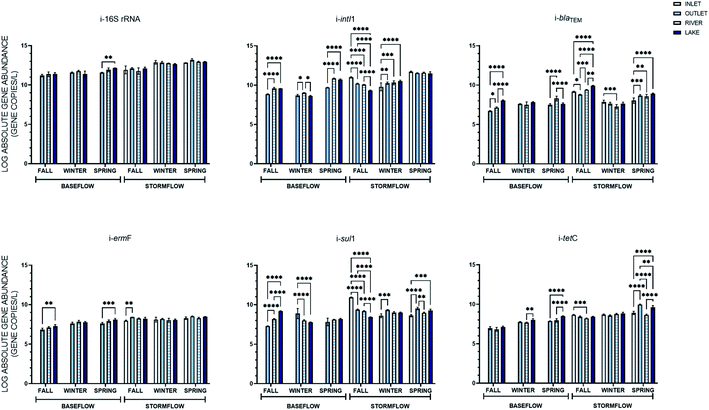 |
| Fig. 4 Absolute gene abundance of intracellular genes at the inlet, outfall, river, and lake sampling location under baseflow and stormflow conditions across three sampling events. Error bars represent the standard deviation of the mean (n = 3) and statistically significant relationships between the average absolute abundance of the extracellular and intracellular genes were evaluated by Tukey's posthoc test and shown with *(p < 0.05), **(p < 0.01), ***(p < 0.001), and ****(p < 0.0001). | |
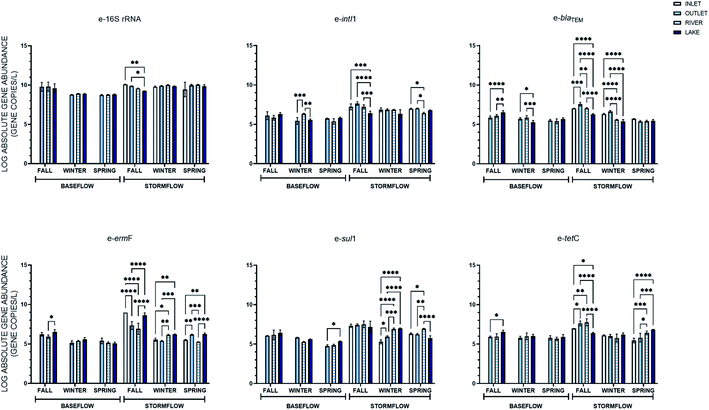 |
| Fig. 5 Absolute gene abundance of extracellular genes at the inlet, outfall, river, and lake sampling location under baseflow and stormflow conditions across three sampling events. Error bars represent the standard deviation of the mean (n = 3) and statistically significant relationships between the average absolute abundance of the extracellular and intracellular genes were evaluated by Tukey's posthoc test and shown with *(p < 0.05), **(p < 0.01), ***(p < 0.001), and ****(p < 0.0001). | |
The percent difference in the average ARG concentrations between baseflow and stormflow was again calculated across the different sampling locations from which a baseflow and stormflow sample was taken. Each intracellular gene had the greatest percent increase between baseflow and the storm events at the outfall location, where the average abundance increased between 11.0–19.2%. This increase was also statistically greater than that found at the river (6.8–14.4%) and lake (6.8–14.4%) locations. The extracellular genes displayed the same pattern as the intracellular genes in this case, as on average the extracellular genes increase 12.3% at the outfall, followed by 11.2% and 7.1% at the river and lake locations, respectively. Thus, while there was not a decrease in the absolute concentration of ARGs from the outlet to the lake, there was a decreasing trend in the percent difference between baseflow and stormflow, indicating that stormwater has a greater impact on the abundance of ARGs at stormwater locations of a lesser volume.
eARGs did not display the same relationships as iARGs over space. Notably, two genes were only significantly increased during the storm events at one location, e-blaTEM at the outfall and e-tetC in the river. This result suggests that the source of the extracellular genes in stormwater varies. More to this point, the location at which the extracellular genes saw the greatest increase compared to baseflow was not the same; e-16S rRNA, e-intI1, and e-blaTEM at the outfall, sul1 and tetC in the river, and ermF in the lake.
intI1 was quantified in this study because it is a mobile genetic element that is regularly found to carry ARGs, facilitating their transport in the environment. Correlation analysis was conducted between the ARGs and intI1, to determine if mobile genetic elements were a mechanism by which iARGs and eARGs are transported in stormwater; the results can be found in Tables S1 and S2.† Seasonally, iARG and eARG occurrence was most significantly correlated with intI1 during the fall storm event. The Pearson correlation coefficients (r) for the i-blaTEM, i-sul1, e-blaTEM, and e-tetC genes during this sampling event were −0.68, 0.98, 0.85, and 0.70, respectively. By location, the ARG concentrations at the outfall were strongly correlated (r ≥ 0.60) for seven of the eight genes under storm conditions. i-tetC and e-tetC, specifically, correlated to i-intI1 and e-intI1, respectively, strongly at four of the eight locations and moderately (0.40 ≤ r < 0.60) at three locations. None of the ARGs, though, were found to correlate significantly with intI1 across all storm events and locations.
Correlation between ARGs and water quality parameters
Correlation analysis was also conducted between all intracellular and extracellular genes and the water quality parameters quantified (Fig. S13–S14 and Tables S3–S4†). Under baseflow conditions iARGs and eARGs were found to be positively correlated to TSS with Pearson's r values ranging between 0.42–0.77 for 8 of the 12 genes and negatively correlated with chromium (−0.44 to −0.75, for 8/12 genes), iron (−0.45 to −0.86, for 7/12 genes), copper (−0.54 to −0.92, for 7/12 genes), and lead (−0.4 to −0.93, for 8/12 genes). Of the 192 correlations made between iARG and eARGs with water quality parameters under stormwater conditions, only 6 were found to have Pearson's r values ≥0.60. This included i-16S rRNA with pH, DOC, chromium, and lead, as well as e-ermF, and e-tetC with copper.
Discussion
eARGs are essential components of an environment's resistome that are available for the development of bacterial resistance. Yet their quantification is regularly not included in studies of aquatic environments because they are difficult to extract and assumed to be found at low concentrations. As a result, there is a large gap in knowledge about the abundance of eARG in different aquatic matrices. The quantification of eARGs in urban runoff was the focus of this study, and it was hypothesized that the eARGs would be highly abundant due to their affinity to adhere to sediments which can be picked up and transported via stormwater runoff. This is the first time eARGs have been quantified in urban stormwater runoff and water bodies impacted by stormwater. The sampling campaign undertaken targeted eARGs during three seasons at four locations across an urban watershed. This study also evaluated the seasonality of ARGs in stormwater, which is currently understudied but is necessary for providing perspective for stormwater management efforts that incorporates ARG variability on a yearly scale. Subsequently, the fraction of eARGs was comparatively analyzed to iARGs which were extracted and quantified from the stormwater runoff.
Stormwater runoff contributes to an increase in extracellular resistance elements in urban water bodies
The absolute abundances of the eARGs blaTEM, ermF, sul1, and tetC in stormwater were found to range between 1.29 × 105 to 9.77 × 108 GC per L across all locations. In comparison to eARG abundances reported in other aquatic environments, concentrations were generally greater than those quantified in surface water samples21,33 and more comparable to wastewater effluent.22,38 The inflow of eARGs via stormwater at such levels presents many potential impacts to receiving aquatic water bodies due to an increase in the abundance of resistance genetic material. One potential impact is the development of environmental hotspots, or an area heavily polluted with ARGs that acts as a reservoir for gene acquisition by horizontal gene transfer (HGT). HGT can impact the evolution of microorganisms, including pathogenic bacteria, as ARGs, virulence factors, and diverse functions can be encoded on horizontally transferred genes.39,40 Thus, as the rate of HGT increases due to an increase in the abundance of ARGs, so does the probability of transfer of ARGs to pathogenic bacteria that can lead to human health consequences.39
Exasperating this issue is the presence of selective pressures, such as heavy metals, and MGEs in stormwater. Selective pressures are known to increase mutation rates, recombination, horizontal gene transfer, and expression of antibiotic resistance mechanisms thereby diversifying the overall resistome and increasing the risk of ARG dissemination to human pathogens.41,42 The mobility of ARGs is also known to impact its rate of transfer into bacterial cells.43 ARGs that are not associated with MGEs are not easily transferred between bacteria in the environment; consequently, they are less likely to be acquired horizontally.44 MGEs, such as integrons, can carry a large number of ARGs and are able to move themselves within a cell and transfer to a recipient cell freely.2 Thus, the transfer of ARGs via MGEs is found to be much more frequent and can enrich host cells with multidrug resistance.43–45
The urban stormwater runoff analyzed in this study was abundant in iARGs, eARGs, intI1, a MGE, and heavy metals. This confluence of factors indicates that stormwater is likely a hotspot for HGT of iARGs and eARGs via conjugation and transformation, respectively. Further research will be needed to confirm this conclusion and determine the rate of HGT in urban stormwater runoff. The stormflow concentrations of both iARGs and eARGs reported in this study were also elevated above baseline conditions. Of the iARGs and eARGs quantified, i-tetC and e-ermF were found to have the greatest percent increase (15.4 and 16.7%, respectively) in their average concentration analyzed between baseflow and stormflow. This is the first time that both genes have been reported in stormwater and the significant increase in abundance indicates that stormwater runoff is altering the resistomes of receiving environments.
eARGs and iARGs in stormwater vary over time
The absolute concentrations of ARGs in stormwater were found to be inconsistently influenced by seasonal dynamics. All eARGs were discovered to be statistically elevated in the fall. The relationships observed for the iARGs on the other hand, were specific to the individual gene. Seasonality of ARGs in stormwater is underreported but three studies have investigated iARGs over time in environments that are impacted by stormwater runoff, including a karst spring in Germany,26 urban beaches in Australia,16 and Canadian streams.46 Monitoring of these environments indicated that the concentrations of ARGs were not significantly elevated in one season versus another, but rather were positively correlated with turbidity concentrations and rainfall depths and negatively correlated with conductivity. In this study, the winter sampling event had the greatest streamflow at the time of sampling as well as the greatest concentration of solids, but no ARG was found to be the most abundant in the winter. It was hypothesized that ARG concentrations would fluctuate due to the impact varying seasonal water quality parameters can have microbial activity. In addition to TSS and conductivity, water temperature, nutrients, metals, and DOC were water quality parameters that displayed seasonal variability. The presence of numerous pollutants in stormwater that are known to influence bacterial activity is likely facilitating complex interactions with iARGs and eARGs that cannot be captured in one sampling event per season. This conclusion is evident by the lack of significant correlations found between ARG concentrations and the water quality parameters measured under stormwater conditions. Further research is needed to elucidate the specific role different stormwater parameters play on the abundance on iARGs and eARGs.
Across seasons, the absolute concentration of all iARGs was significantly greater than eARGs. This result was expected as it has regularly been reported that iARGs are more prevalent than eARGs in aquatic environments.20 However, when the relative abundances were compared there was minimal differences between the iARG and eARG concentrations across all sampling events. This result not only supports the understanding that eARGs are abundant in stormwater runoff, but also confirms that they are a significant and relevant portion of the resistance elements entering the environment through stormwater.
The fall sampling event was found to produce the greatest increase in iARG and eARGs when the seasonal concentrations of iARGs and eARGs in stormwater were compared to baseflow. Water quality parameters that also increased most significantly in the fall were DOC, TP, and the heavy metals chromium, copper, zinc, and lead. The propagation of ARGs as well as water quality pollutants in the fall storm event could be the result of hydrological factors. An increased antecedent dry weather period, for instance, has been correlated with higher concentrations of pollutants in stormwater runoff due to the buildup and subsequent transport of chemicals and solids from urban surfaces.47 The antecedent dry weather period that preceded the fall sampling event was 17 days, while only 7 and 3 days of dry weather came before the winter and spring sampling, respectively. The time of sampling could have also impacted the abundance of iARGs and eARGs quantified as ARGs have been found to present intra-storm variability.48
eARGs and iARGs vary across stormwater locations
It was hypothesized that across the four sampling locations there would be a decreasing trend in the absolute abundance of the iARGs and eARGs, as the stormflow volume of the sampling locations increased. Statistically, a decreasing trend in the absolute concentration across the locations was only observed for one gene, during one storm event; it was largely found that the receiving waterbody locations, the river and lake, were not distinctly different from the direct runoff sampled at the storm drain inlet and stormwater outfall. Consequently, it can be concluded that the input of ARGs at elevated concentrations across the watershed is widespread and is leading to the accumulation and persistence of ARGs in stormwater receiving environments.
A decreasing trend was observed from the outlet to the lake in the percent increase of iARGs and eARGs between baseflow and stormflow conditions. This result implies that stormwater is having the largest impact on the abundance of resistance elements in environments where it is a larger fraction of the overall flow volume. Management of ARGs in stormwater would thus require implementing stormwater control measures that treat stormwater where it falls to eliminate large volumes of runoff, thus controlling the flow of ARGs and other pollutants to a central location.4,35,49 The results of this study indicate, moreover, that there are many sources and transport mechanisms of ARGs into the environment as transport across the four locations was found to be variable for iARGs and eARGs for each seasonal storm event. Therefore, when considering engineering controls for ARG pollution into the environment via urban stormwater runoff, identification of key sources of ARGs for optimized implementation will be needed.51 Green infrastructure is a potential stormwater engineering control measure that can limit heavy runoff and with a small footprint, can be presented as a localized treatment of direct urban runoff. Further research will be needed though to determine if different green infrastructure systems are able to capture ARGs from urban runoff and the fate of ARGs in these systems.
Conclusion
Antibiotic resistance is a major public health concern, and ARGs are the emerging contaminants that drive the concern. In this study, the seasonal and spatial dynamics of iARGs and eARGs were investigated in urban stormwater runoff. Crucially, eARGs were identified as an emerging contaminant in urban stormwater and the aquatic environments impacted by runoff. In addition, the greatest increases in the average concentrations of both iARGs and eARGs between baseflow and stormflow were found in the fall season and at the outfall location. These results indicated that stormwater has the largest impact on the prevalence of iARG and eARGs in direct runoff, presenting the opportunity for engineering controls that capture and treat runoff near the source. The increase specific to the fall season is attributable, in part, to the antecedent dry period and the potential for buildup of ARG contaminants. This research highlights the significance of eARGs for its contribution to the resistome of stormwater and the need for further investigations into the potential of eARG acquisition via horizontal gene transformation in aquatic environments impacted by stormwater runoff.
Conflicts of interest
There are no conflicts to declare.
Acknowledgements
This material is based upon work supported by the National Science Foundation Graduate Research Fellowship under Grant no. 2041851. Any opinion, findings, and conclusions or recommendations expressed in this material are those of the authors and do not necessarily reflect the views of the National Science Foundation. This research was also supported by an Opus College of Engineering Seed Grant funded by the GHR Foundation.
References
- H. W. Stokes and M. R. Gillings, Gene flow, mobile genetic elements and the recruitment of antibiotic resistance genes into Gram-negative pathogens, FEMS Microbiol. Rev., 2011, 35, 790–819 CrossRef CAS PubMed.
- S. R. Partridge, S. M. Kwong, N. Firth and S. O. Jensen, Mobile genetic elements associated with antimicrobial resistance, Clin. Microbiol. Rev., 2018, 31, 1–61 CrossRef PubMed.
- P. J. Vikesland, A. Pruden, P. J. J. Alvarez, D. Aga, H. Bürgmann, X. D. Li, C. M. Manaia, I. Nambi, K. Wigginton, T. Zhang and Y. G. Zhu, Toward a Comprehensive Strategy to Mitigate Dissemination of Environmental Sources of Antibiotic Resistance, Environ. Sci. Technol., 2017, 51, 13061–13069 CrossRef CAS PubMed.
- K. A. Hamilton, E. Garner, S. Joshi, W. Ahmed, N. Ashbolt, G. Medema and A. Pruden, Antimicrobial-resistant microorganisms and their genetic determinants in stormwater: a systematic review, Curr. Opin. Environ. Sci. Heal., 2020, 16, 101–112 CrossRef.
- K. O'Malley, W. McDonald and P. McNamara, An extraction method to quantify the fraction of extracellular and intracellular antibiotic resistance genes in aquatic environments, J. Environ. Eng., 2022, 148, 04022017 CrossRef.
- S. Saifur and C. M. Gardner, Loading, transport, and treatment of emerging chemical and biological contaminants of concern in stormwater, Water Sci. Technol., 2021, 83, 2863–2885 CrossRef CAS PubMed.
- J. Bengtsson-Palme, E. Kristiansson and D. G. J. Larsson, Environmental factors influencing the development and spread of antibiotic resistance, FEMS Microbiol. Rev., 2018, 42, 68–80 CrossRef CAS PubMed.
- S. S. Kaushal, W. H. McDowell, W. M. Wollheim, T. A. N. Johnson, P. M. Mayer, K. T. Belt and M. J. Penninov, Urban evolution: the role of water, Water, 2015, 7, 4063–4087 CrossRef CAS.
- Z. Zhao, J. Wang, Y. Han, J. Chen, G. Liu, H. Lu, B. Yan and S. Chen, Nutrients, heavy metals and microbial communities co-driven distribution of antibiotic resistance genes in adjacent environment of mariculture, Environ. Pollut., 2017, 220, 909–918 CrossRef CAS PubMed.
- K. Rangasamy, A. Murugan, N. Devarajan and J. A. Parray, Emergence of multi drug resistance among soil bacteria exposing to insecticides, Microb. Pathog., 2017, 105, 153–165 CrossRef CAS PubMed.
- X. Zuo, P. Suo, Y. Li and Q. Xu, Diversity and distribution of antibiotic resistance genes associated with road sediments transported in urban stormwater runoff, Environ. Pollut., 2022, 292, 118470 CrossRef CAS PubMed.
- D. Baral, B. I. Dvorak, D. Admiraal, S. Jia, C. Zhang and X. Li, Tracking the Sources of Antibiotic Resistance Genes in an Urban Stream during Wet Weather using Shotgun Metagenomic Analyses, Environ. Sci. Technol., 2018, 52, 9033–9044 CrossRef CAS PubMed.
- W. Ahmed, Q. Zhang, A. Lobos, J. Senkbeil, M. J. Sadowsky, V. J. Harwood, N. Saeidi, O. Marinoni and S. Ishii, Precipitation influences pathogenic bacteria and antibiotic resistance gene abundance in storm drain outfalls in coastal sub-tropical waters, Environ. Int., 2018, 116, 308–318 CrossRef CAS PubMed.
- S. Zhang, S. Pang, P. F. Wang, C. Wang, N. Han, B. Liu, B. Han, Y. Li and K. Anim-Larbi, Antibiotic concentration and antibiotic-resistant bacteria in two shallow urban lakes after stormwater event, Environ. Sci. Pollut. Res., 2016, 23, 9984–9992 CrossRef CAS PubMed.
- E. Garner, R. Benitez, E. von Wagoner, R. Sawyer, E. Schaberg, W. C. Hession, L. A. H. Krometis, B. D. Badgley and A. Pruden, Stormwater loadings of antibiotic resistance genes in an urban stream, Water Res., 2017, 123, 144–152 CrossRef CAS PubMed.
- R. L. Carney, M. Labbate, N. Siboni, K. A. Tagg, S. M. Mitrovic and J. R. Seymour, Urban beaches are environmental hotspots for antibiotic resistance following rainfall, Water Res., 2019, 167, 115081, DOI:10.1016/j.watres.2019.115081.
- A. Chaudhary, I. Kauser and A. Ray, Taxon-Driven Functional Shifts Associated with Storm Flow in an Urban Stream Microbial Community, Appl. Environ. Sci., 2018, 3, e00194 CAS.
- A. Eramo, H. D. Reyes and N. L. Fahrenfeld, Partitioning of antibiotic resistance genes and fecal indicators varies intra and inter-storm during combined sewer overflows, Front. Microbiol., 2017, 8, 1–13 Search PubMed.
- A. Di Cesare, E. M. Eckert, M. Rogora and G. Corno, Rainfall increases the abundance of antibiotic resistance genes within a riverine microbial community, Environ. Pollut., 2017, 226, 473–478 CrossRef CAS PubMed.
- A. Zarei-Baygi and A. L. Smith, Intracellular versus extracellular antibiotic resistance genes in the environment: prevalence, horizontal transfer, and mitigation strategies, Bioresour. Technol., 2021, 319, 124181 CrossRef CAS PubMed.
- D. Mao, Y. Luo, J. Mathieu, Q. Wang, L. Feng, Q. Mu, C. Feng and P. J. J. Alvarez, Persistence of extracellular DNA in river sediment facilitates antibiotic resistance gene propagation, Environ. Sci. Technol., 2014, 48, 71–78 CrossRef CAS PubMed.
- Y. Zhang, A. Li, T. Dai, F. Li, H. Xie, L. Chen and D. Wen, Cell-free DNA: A Neglected Source for Antibiotic Resistance Genes Spreading from WWTPs, Environ. Sci. Technol., 2018, 52, 248–257 CrossRef CAS PubMed.
- P. Dong, H. Wang, T. Fang, Y. Wang and Q. Ye, Assessment of extracellular antibiotic resistance genes (eARGs) in typical environmental samples and the transforming ability of eARG, Environ. Int., 2019, 125, 90–96 CrossRef CAS PubMed.
- Q. Sui, Y. Chen, D. Yu, T. Wang, Y. Hai, J. Zhang, M. Chen and Y. Wei, Fates of intracellular and extracellular antibiotic resistance genes and microbial community structures in typical swine wastewater treatment processes, Environ. Int., 2019, 133, 105183, DOI:10.1016/j.envint.2019.105183.
- M. Walaszek, P. Bois, J. Laurent, E. Lenormand and A. Wanko, Urban stormwater treatment by a constructed wetland: seasonality impacts on hydraulic efficiency, physico-chemical behavior and heavy metal occurrence, Sci. Total Environ., 2018, 637–638, 443–454 CrossRef CAS PubMed.
- C. Stange and A. Tiehm, Occurrence of antibiotic resistance genes and microbial source tracking markers in the water of a karst spring in Germany, Sci. Total Environ., 2020, 742, 140529, DOI:10.1016/j.scitotenv.2020.140529.
- D. N. Rietz and R. J. Haynes, Effects of irrigation-induced salinity and sodicity on soil microbial activity, Soil Biol. Biochem., 2003, 35, 845–854 CrossRef CAS.
- C. Zhang, S. Nie, J. Liang, G. Zeng, H. Wu, S. Hua, J. Liu, Y. Yuan, H. Xiao, L. Deng and H. Xiang, Effects of heavy metals and soil physicochemical properties on wetland soil microbial biomass and bacterial community structure, Sci. Total Environ., 2016, 557–558, 785–790 CrossRef CAS PubMed.
- A. Selvakumar and M. Borst, Variation of microorganism concentration in urban stormwater runoff with land use and seasons, J. Water Health, 2006, 4, 109–124 CrossRef PubMed.
- W. C Reygaert, An overview of the antimicrobial resistance mechanisms of bacteria, AIMS Microbiol., 2018, 4, 482–501 Search PubMed.
-
S. W. R. P. Commission, A Watercourse System Plan for Schoonmaker Creek, https://www.sewrpc.org/SEWRPCFiles/Publications/mr/mr-241-schoonmaker-creek-watercourse-report.pdf, (accessed June 2022) Search PubMed.
-
W. D. of N. Resources, Milwaukee River Basin, https://dnr.wisconsin.gov/topic/Watersheds/basins/milw, (accessed June 2022) Search PubMed.
- M. Liu, A. Hata, H. Katayama and I. Kasuga, Consecutive ultrafiltration and silica adsorption for recovery of extracellular antibiotic resistance genes from an urban river, Environ. Pollut., 2020, 260, 114062 CrossRef CAS PubMed.
- M. R. Gillings, W. H. Gaze, A. Pruden, K. Smalla, J. M. Tiedje and Y. G. Zhu, Using the class 1 integron-integrase gene as a proxy for anthropogenic pollution, ISME J., 2015, 9, 1269–1279 CrossRef CAS PubMed.
- R. Singh, A. P. Singh, S. Kumar, B. S. Giri and K. H. Kim, Antibiotic resistance in major rivers in the world: a systematic review on occurrence, emergence, and management strategies, J. Cleaner Prod., 2019, 234, 1484–1505 CrossRef CAS.
- P. Sivalingam, J. Pote and K. Prabakar, Extracellular DNA (eDNA): Neglected and Potential Sources of Antibiotic Resistant Genes (ARGs) in the Aquatic Environments, Pathogens, 2020, 9, 874 CrossRef CAS PubMed.
- K. Qin, L. Wei, J. Li, B. Lai, F. Zhu, H. Yu, Q. Zhao and K. Wang, A review of ARGs in WWTPs: sources, stressors and elimination, Chin. Chem. Lett., 2020, 31, 2603–2613 CrossRef CAS.
- Q. Bin Yuan, Y. M. Huang, W. Bin Wu, P. Zuo, N. Hu, Y. Z. Zhou and P. J. J. Alvarez, Redistribution of intracellular and extracellular free & adsorbed antibiotic resistance genes through a wastewater treatment plant by an enhanced extracellular DNA extraction method with magnetic beads, Environ. Int., 2019, 131, 104986, DOI:10.1016/j.envint.2019.104986.
- M. Juhas, Horizontal gene transfer in human pathogens, Crit. Rev. Microbiol., 2015, 41, 101–108 CrossRef CAS PubMed.
- A. J. Lopatkin, T. A. Sysoeva and L. You, Dissecting the effects of antibiotics on horizontal gene transfer: analysis suggests a critical role of selection dynamics, Bioessays, 2016, 38, 1283 CrossRef CAS PubMed.
- C. Baker-Austin, M. S. Wright, R. Stepanauskas and J. V. McArthur, Co-selection of antibiotic and metal resistance, Trends Microbiol., 2006, 14, 176–182 CrossRef CAS PubMed.
- G. W. Sundin and C. L. Bender, Dissemination of the strA–strB streptomycin-resistance genes among commensal and pathogenic bacteria from humans, animals, and plants, Mol. Ecol., 1996, 5, 133–143 CrossRef CAS.
- J. L. Martinez, T. M. Coque and F. Baquero, What is a resistance gene? Ranking risk in resistomes, Nat. Rev. Microbiol., 2015, 13, 116–123 CrossRef CAS PubMed.
- K. Pärnänen, A. Karkman, M. Tamminen, C. Lyra, J. Hultman, L. Paulin and M. Virta, Evaluating the mobility potential of antibiotic resistance genes in environmental resistomes without metagenomics, Sci. Rep., 2016, 6, 35790 CrossRef PubMed.
- D. J. Rankin, E. P. C. Rocha and S. P. Brown, What traits are carried on mobile genetic elements, and why?, Heredity, 2011, 106, 1–10 CrossRef CAS PubMed.
- P. L. Keen, C. W. Knapp, K. J. Hall and D. W. Graham, Seasonal dynamics of tetracycline resistance gene transport in the Sumas River agricultural watershed of British Columbia, Canada, Sci. Total Environ., 2018, 628–629, 490–498 CrossRef CAS PubMed.
- J. Zhang, P. Hua and P. Krebs, Influences of land use and antecedent dry-weather period on pollution level and ecological risk of heavy metals in road-deposited sediment, Environ. Pollut., 2017, 228, 158–168 CrossRef CAS PubMed.
- E. Garner, J. S. Wallace, G. A. Argoty, C. Wilkinson, N. Fahrenfeld, L. S. Heath, L. Zhang, M. Arabi, D. S. Aga and A. Pruden, Metagenomic profiling of historic Colorado Front Range flood impact on distribution of riverine antibiotic resistance genes, Sci. Rep., 2016, 6, 38432, DOI:10.1038/srep38432.
- A. D. Steinman, E. S. Isely and K. Thompson, Stormwater runoff to an impaired lake: impacts and solutions, Environ. Monit. Assess., 2015, 187, 549, DOI:10.1007/s10661-015-4776-z.
- K. O'Malley, P. McNamara and W. McDonald, Antibiotic resistance genes in an urban stream before and after a state fair, J. Water Health, 2021, 19(6), 885–894, DOI:10.2166/wh.2021.151.
- K. Liguori, I. Keenum, B. C. Davis, J. Calarco, E. Milligan, V. J. Harwood and A. Pruden, Antimicrobial Resistance Monitoring of Water Environments: A Framework for Standardized Methods and Quality Control, Environ. Sci. Technol., 2022 DOI:10.1021/acs.est.1c08918.
|
This journal is © The Royal Society of Chemistry 2022 |
Click here to see how this site uses Cookies. View our privacy policy here.