Fungal diversity and key functional gene abundance in Iowa bioretention cells: implications for stormwater remediation potential†
Received
14th May 2024
, Accepted 17th August 2024
First published on 20th August 2024
Abstract
Stormwater bioretention cells are green stormwater infrastructure systems that can help mitigate flooding and remove contaminants. Plants and bacteria improve nutrient removal and degrade organic contaminants; however, the roles of fungi in bioretention cells are less known. Although mycorrhizal fungi aid in plant growth/improve nutrient uptake, there is a notable lack of research investigating fungal diversity in bioretention cells. Other types of fungi could benefit bioretention cells (e.g., white rot fungi degrade recalcitrant contaminants). This study addresses the knowledge gap of fungal function and diversity within stormwater bioretention cells. We collected multiple soil samples from 27 different bioretention cells in temperate-climate eastern Iowa USA, characterized soil physicochemical parameters, sequenced the internal transcribed spacer (ITS) amplicon to identify fungal taxa from extracted DNA, and measured functional gene abundances for two fungal laccases (Cu1, Cu1A) and a fungal nitrite reductase gene (nirKf). Fungal biodegradation functional genes were present in bioretention soils (mean copies per g: 7.4 × 105nirKf, 3.2 × 106Cu1, 4.0 × 108Cu1A), with abundance of fungal laccase and fungal nitrite reductase genes significantly positively correlated with soil pH and organic matter (Pearson's R: >0.39; rho < 0.05). PERMANOVA analysis determined soil characteristics were not significant explanatory variables for community composition (beta diversity). In contrast, planting specifications significantly impacted fungal diversity; the presence/absence of a few planting types and predominant vegetation type in the cell explained 89% of variation in fungal diversity. These findings further emphasize the importance of plants and media as key design parameters for bioretention cells, with implications for fungal bioremediation of captured stormwater contaminants.
Environmental significance
Bioretention cells capture and treat urban runoff laden with organic contaminants, and fungi may be a useful bioremediation approach. We characterized fungal communities in eastern Iowa USA, quantified biodegradation function genes, and correlated plant/soil types with fungal diversity. The taxa that include white/brown rot fungi capable of biodegrading recalcitrant contaminants were highly represented, with plant type a significant driver of fungal diversity. Fungal laccase/nitrite reductase functional genes were significantly correlated with soil pH and organic matter. We provide new evidence that diverse fungi are present within bioretention cells and elucidate plantings as key influences. Implications include optimizing planting/media types in green stormwater infrastructure design, and potential for fungal bioaugmentation/inoculation of bioretention to improve stormwater contaminant removal.
|
1. Introduction
It is well-established that stormwater is a highly complex mixture of contaminants.1 Outside of “traditional” stormwater contaminants (e.g., heavy metals, nutrients, polycyclic aromatic hydrocarbons), evidence is growing for the presence of trace mobile/hydrophilic organic contaminants within stormwater.2–4 To prevent mobile organic contaminants from entering ground and surface waters, there is a need to re-examine methods of stormwater management. Green stormwater infrastructure is one approach to stormwater management and includes practices such as green roofs, permeable pavements, and bioretention cells (the system of focus for this work; Fig. S.1 and S.2†).5 Bioretention cells are primarily designed to emulate the pre-development hydrologic regime (peak flow and volume reduction, rapid infiltration) and additionally abate many non-point stormwater contaminants.1,6 The contaminant abatement can occur via abiotic means such as particle filtration or sorption to soil media. Although bioretention cells can filter particle-associated pollutants,7 dissolved pollutants present an additional challenge to treatment. Dissolved pollutants can adsorb to the bioretention soils, but compounds that are hydrophilic/polar (e.g., benzotriazole) can pass through the cell with minimal retention.8–10 This phenomenon could be remedied via geomedia amendments such as biochar or black carbon;11–13 for example, biochar-amended mesocosms were able to maintain 99% of trace organic contaminant removal relative to only 50% retention in un-amended columns.14 Nevertheless, even with geomedia amendments, dissolved pollutants may not be retained well in bioretention cells once sorption capacity of the soil media/amendments is reached. Additionally, hydrophobic compounds such as high molecular weight polycyclic aromatic hydrocarbons (PAHs) found in stormwater could contribute to exhausting sorption capacity within bioretention geomedia. For example, a recent study of a field bioretention site reported recalcitrant PAHs most accumulated at medium depth where bacterial biodegradation may be more limited.15
To mitigate limitations from abiotic removal mechanisms, maximized contaminant sorption/filtration can be coupled with biotic uptake and transformation processes in microbes and plants. Plant selection is important for functioning bioretention cells; plants with deeper rooting zones can prevent clogging and improve infiltration.16 Phosphorus and nitrogen removal can also improve in planted systems, though efficacy may depend on plant type.17 Increasing evidence demonstrates plants can impact trace organic contaminant removal in bioretention. For example, plants can remove and transform benzotriazole hydroponically,18 and benzotriazole phytotransformation products were detected in bench-scale bioretention mesocosms19 as well as a field-scale tracer study.20 In addition to plants, organisms such as bacteria and fungi also affect contaminant fate within bioretention cells. Bacteria have mostly been studied in context of nutrients within bioretention cells but have also demonstrated contributions to organic contaminant removal. One recent study suggests biochar-amended bioretention cells, with the contributions of active microbial biofilms, could extend the lifetime of the system by 1.8–2.3 times and enhance removal of trace contaminants like atrazine and neonicotinoids.21
Although there is growing evidence on the roles of plants and bacteria within bioretention cells, fungi have received very limited consideration. Within these limited studies, mycorrhizal fungi have garnered the most attention, as they associate with and often provide benefits to plants such as nutrients and protection against pathogens.22,23 Mycorrhizal fungi have been observed in bioretention cells,24 and initial studies indicate functional improvements such as increased plant growth and stress resistance, improved nutrient uptake and heavy metal removal.25–27 Beyond mycorrhizal fungi, fungal enzymes such as lignin peroxidases or laccases could enhance biotransformation of contaminants; indeed, many of these enzymes are not substrate-specific and have widely demonstrated the break-down of recalcitrant contaminants such as polychlorinated biphenyls,28,29 carbamazepine,30,31 and azo dyes.32 Despite the potential of fungi to enhance bioretention function, we found very few studies reporting on fungal diversity within green stormwater infrastructure bioswales. These initial findings indicated fungal communities in bioswales (bioswales serve similar functions to bioretention cells) were distinct from park/tree pit soils, likely influenced by differences in micro-environment/habitat,33 and that community differences were correlated with plant species differences.34
Though emerging studies on microbial communities in green stormwater infrastructure provide important initial insights on fungal diversity, more work is needed to understand the role of fungi in green stormwater infrastructure, particularly bioretention cells. The single study probing the connection between plant species/physiology and microbial community differences focused on plant transpiration rates as the relevant physiological trait. Other traits, such as rooting depth, or non-plant variables such as runoff type (e.g., parking lot vs. road), remain unexplored. Abundance of relevant bacterial biotransformation genes such as denitrification genes,35,36bphA,37 and naphthalene degradation genes38,39 have been quantified within bioretention cells or mesocosms, but no studies have specifically investigated relevant fungal biotransformation genes such as laccases and peroxidases in bioretention cells. Thus, there is a paucity of information on fungal communities and function within bioretention cells and how fungi (native or bioaugmented) could enhance removal of dissolved organic contaminants. To address these knowledge gaps, we measured fungal diversity and key fungal functional gene abundance in 27 different bioretention cells in eastern Iowa (in the temperate Midwestern United States). The objective of this study was to determine driving factors of fungal diversity and functional gene abundance in representative Iowa bioretention cells. We hypothesized that bioretention plant rooting depth and soil characteristics would significantly influence fungal diversity and functional gene abundance.
2. Methods
2.1 Bioretention cell soil sampling
2.1.1 Sampling location selection.
Local officials helped identify bioretention cells in Iowa City, Coralville, North Liberty, and Cedar Rapids, Iowa (Johnson and Linn Counties). Sampling permission as well as any available site information (e.g., plantings, plans, etc.) for each site was obtained from these officials (see Table S.1† for site information). Prior to sampling, all sites were visited to confirm feasibility of sampling and to record initial site information. Bioretention cells were selected to encompass representative geographic variability, vegetation variety, and runoff source (e.g., building, parking lot, or major road). A total of 27 bioretention cells were sampled. All bioretention cell soil sampling occurred during the week of September 20, 2021. At each bioretention site, soils were collected from 4–6 sampling locations distributed throughout the cell. A composite sample was created from an equal mixture of each sampling location within the cell to better encompass within-cell heterogeneity. Vegetation types within the cell and at each sampling location within the cell were recorded and pictures were taken of each cell and individual sampling point.
2.1.2 Sampling methodology and storage.
Two types of soil samples were taken: soil for DNA extraction and soil for physicochemical characterization. To collect soil samples for DNA extraction, we cleared away the mulch layer (if present) and used 2.2 × 53 cm (7/8′′ × 21′′) plated soil probes (AMS) inserted vertically to obtain soil cores at 15 cm maximum depth. Metal scoops were used to deposit soil between 2 and 10 cm depth from the corer into labeled sterile Whirl-Pak bags (one bag per sampling location). At one cell (cv14), soil was collected for both the top 10 cm and bottom 10 cm of the core. For physicochemical characterization of soil samples, a metal trowel was used to scoop soil into a virgin plastic (gallon Ziploc) bag at each sampling point within the cell, using the same bag for all sampling points and mixed on-site. Between each sampling location within a cell (and in between bioretention cells), all sampling equipment was cleaned and wiped down with 70% ethanol. All samples were transported on ice to the lab. To create composite soil samples for DNA extraction, a nominal 2 g (1.7 ± 0.04 g) of soil was measured from each individual soil sample within a site and combined using a 2.0 mm sieve. Soil samples for DNA extraction were stored at −80 °C until DNA extraction. Soil samples for physicochemical analysis were stored at 4 °C.
2.1.3 Physicochemical analysis.
Composite soil samples (n = 27; approximately 500 g per sample) collected for physicochemical analysis were submitted to the Minnesota Valley Testing Laboratories, Inc. (New Ulm, MN). The following physicochemical analyses were acquired: pH, phosphorus (Bray I/Olsen), potassium, zinc, manganese, copper, percent organic matter (OM), nitrate, and ammonium nitrogen. Raw soil data are available in Table S.5.†
2.2 Soil DNA extraction and sequencing
Soil DNA was extracted from the composite samples for each bioretention cell with a DNeasy PowerSoil Pro Kit (QIAGEN) according to manufacturer instructions. Sub-aliquots of extracted DNA were diluted with TE buffer (QIAGEN) and sent overnight on ice to MR (Molecular Research) DNA Laboratories (an end-to-end sequencing and bioinformatics service). Concentrations of DNA samples were recorded prior to shipment using a Qubit fluorometer. Due to some samples exceeding the range of detection of the instrument, DNA normalization services were requested. One no-template negative control (nuclease free water) was sent along with the DNA samples for sequencing. We requested fungal internal transcribed spacer (ITS) amplicon sequencing with specifications detailed in Table S.2.† The ITS amplicon primers were based on prior literature (forward40 [fITS7], 5′-3′: GTGARTCATCGAATCTTG; reverse41 [ITS4], 5′-3′: TCCTCCGCTTATTGATATGC). After DNA extraction, all sequencing-related steps were conducted at MR DNA laboratories; methodology described here for sequencing steps were adapted from protocols provided by the laboratory. To amplify the ITS region, the HotStarTaq Plus Master Mix Kit (QIAGEN) was used with the following thermocycling parameters: 94 °C for 3 minutes, 30–35 cycles of 94 °C for 30 seconds, 53 °C for 40 seconds, 72 °C for 1 minute, and a final elongation step at 72 °C for 5 minutes. Amplified products were run through gel electrophoresis on a 2% agarose gel to confirm amplification and band intensity. Gel images were not provided with the sequencing data. Samples were pooled in equimolar amounts and purified with Ampure XP beads. Library preparation followed manufacturer's guidelines for the Illumina MiSeq platform.
2.3 Bioinformatics pipeline
The bioinformatics pipeline to process DNA sequences was conducted using PipeCraft2 (1.0.0). PipeCraft2 is an open-source software with a graphical user interface and is designed to process sequencing data with many customizable options.42 We employed the operational taxonomic unit (OTU) workflow with some additions/modifications (Fig. S.3† for a workflow visualization). Briefly, the steps with PipeCraft names, in order, are: Cut Primers, Merge Reads, Quality Filtering, Chimera Filtering, ITSX, Clustering, Post-Clustering (LULU), Filter Tag-Jumps (UNCROSS2), and Assign Taxonomy. The OTU workflow was chosen over the ASV workflow, as exact sequence variants (ESV) approaches have been noted to overestimate common fungal species and underestimate rare species.43,44 Raw multiplexed files were provided in FASTQ format with a mapping text file. Raw files were demultiplexed into individual sample files using the free FASTQ Splitter software provided by MR DNA (http://www.mrdnafreesoftware.com/fastq-splitter.html). Primers were removed in PipeCraft2. Reads were assembled and filtered by quality and chimeras using the VSEARCH algorithm with default settings.45 The ITS2 region was extracted using ITSx.46 Extracted ITS sequences were clustered into OTUs using VSEARCH and the default settings (97% similarity threshold). LULU was used as a post-clustering step to improve biodiversity metrics.47 Putative tag-jumps were filtered using UNCROSS2.48 We performed taxonomic assignment using BLASTN with the UNITE database (UNITE version 9.0).49 UNITE is an open access, curated, and annotated database for the ITS region. UNITE uses the fungal classification from Tedersoo et al. 2018.50 BLAST first-hit matches were filtered to include hits with greater than 80% coverage. The bioinformatics pipeline outputs include taxonomic assignments for each OTU as well as a counts table which contains the number of each OTU detected for each sample.
2.4 Statistical analysis for sequencing data
Counts tables of OTU data are typically normalized or transformed to account for sequencing depth and data sparsity. For this work, exploratory analysis on processed sequencing data was conducted on an OTU table that was transformed using the Aitchison distance metric and a robust centered log ratio transform (rCLR) using the DEICODE implementation within PipeCraft.51 An rCLR transformation with the Aitchison distance metric accounts for data compositionality, data sparsity, and sequencing depth.43 DEICODE output includes the rCLR-transformed OTU table, the ordination from the robust principal components analysis (PCA), and the distance matrix between samples. Transformed data were analyzed and visualized using R scripts (RStudio version 4.2.0 “Vigorous Calisthenics” release for Windows).
To test relationships between site characteristic variables and the beta diversity distance matrix, permutational multivariate analysis of variance (PERMANOVA) was used. PERMANOVA is a non-parametric method of hypothesis testing for multivariate variation that can be applied to distance matrices such as the rCLR-transformed counts table with the Aitchison distance.52 The PERMANOVA test assumes sample “exchangeability” (data can be rearranged without changing the overall distribution), and random permutations of the data are compared to determine if the centroid of the random permutations are equivalent. The null hypothesis of the test states that there are no differences in the positioning of the centroids when comparing the random permutations of the data to the distance matrix.46 PERMANOVA is considered a powerful statistical tool for microbiome and ecology data, as data do not need to be normally distributed. However, PERMANOVA can be affected by uneven/unbalanced sampling for groups (which applied for many of our site characteristics). To account for this, we conducted tests for homogeneity of multivariate dispersions (PERMDISP).52 Soil characteristics, planting variables, and physical location were analyzed using PERMANOVA (adonis2 function in the R ‘vegan’ package).53 Soil characteristics (continuous variables) and planting/site categorical variables were analyzed in separate PERMANOVA analyses.
2.5 Fungal functional gene analysis
To investigate fungal functional characteristics relevant to biotransformation, we performed quantitative polymerase chain reaction (qPCR) with select functional genes (primers summarized in Table S.3†). Two laccase gene targets (Cu1, basidiomycete laccase;54Cu1A, ascomycete laccase55) and one nitrite reductase gene (nirKf, fungal nitrite reductase56) were selected to probe involvement in biotransformation of relevant stormwater contaminants (trace organics and nutrients, respectively). Primers were selected from previously published work and are degenerate to encompass a broader diversity of fungi for functional genes.48–50 All primers/oligos were ordered from Integrated DNA Technologies (IDT, Coralville, IA), delivered dry, and re-suspended within one day of receipt in sterile low-EDTA TE Buffer (10 mM Tris, 0.1 mM EDTA, pH 8.0; Ambion-Life Technologies stocks). After re-suspension, primers were stored at −20 °C per manufacturer recommendations. For qPCR standards, synthetic gene fragments (gBlocks) were ordered from IDT. The sequence for each standard was determined via a GenBank search, starting with accession numbers for each functional gene found in the literature. As available, full gene sequences were used; otherwise, partial coding sequences were used. We used the Geneious Prime software to run in silico PCR on the sequences. To confirm the appropriate amplicon, we extracted sequences based on matches with expected amplicon size from the literature and fewest number of mismatches with the primer. When possible, we included five additional base pairs outside of the primer binding sites on both ends of the sequence (see Table S.6† for the full gBlock sequences). The gBlocks (IDT) were delivered dry, re-suspended in low-EDTA TE Buffer, and incubated at 50 °C for 20 minutes prior to use per manufacturer instructions. Sub-aliquots were created to avoid more than three freeze–thaw cycles and stored at −20 °C. Proper amplification of gBlocks standards was confirmed using conventional PCR (Tables S.7, S.8 and Fig. S.4–S.6† for gel electrophoresis).
2.5.1 qPCR conditions.
To quantify the fungal functional genes for which amplification was observed for gBlocks standards, we conducted qPCR with the primer sets in Table S.3.† Thermocycling and primer optimization were deemed necessary, as gBlocks failed to produce amplicons under the manufacturer recommendations for the qPCR master mix and instrument. The final qPCR reaction conditions are summarized in Table S.9.† The thermal cycling program was adapted from the conventional PCR step for each gene target (see Fig. S.7 and S.8†), with an added melt curve from 95 °C to 60 °C to check for primer-dimers and non-specific amplification. Reactions were prepared for 96-well plates, with extracted DNA samples in triplicate, no-template controls in duplicate, and a five-point standard curve in duplicate ranging from 3 × 102 to 3 × 108 gene copies. The standards were prepared by 1
:
10 serial dilutions with 10× diluted EB Buffer (QIAGEN). Samples were run at the University of Iowa Institute of Human Genetics on a QuantStudio 7 Flex Real-Time PCR System (Applied Biosystems). Template DNA concentrations were measured prior to plate setup using a Qubit fluorometer and ranged from 4 to 23 ng μL−1. When not run immediately, the plates were stored at 4 °C in the dark for no greater than 24 hours. All qPCR quality control information (melt curves, standard curves, and no-template control information) can be found in Fig. S.9–S.14 and Table S.10.†
2.5.2 Statistical analysis for functional gene abundance.
Raw data processing, including the calculation of standard curves and melt curve visualization, was conducted in Design & Analysis Software (version 2.6.0, Thermo Fisher Scientific). All statistical analyses and graphing for functional gene data were conducted in GraphPad Prism 9.0.0. The relationship between functional gene abundance and site characteristics such as soil properties was examined via a Pearson's correlation matrix and unpaired two-tailed t tests (α = 0.05). A Spearman's correlation matrix was calculated for categorical variables such as rooting depth by coding each category numerically.
3. Results and discussion
3.1 Fungal diversity in bioretention cells
We characterized fungal genetic diversity in 27 representative bioretention cells in eastern Iowa in the temperate Midwestern US. Our results indicate similar sequencing depth, or the number of times a “read” is aligned to a reference base on the sequencing instrument, compared to Gill et al.'s study on microbial diversity in green stormwater infrastructure.33 For our study, 1
593
552 reads were used in the quality filtering step, from which 1
531
879 reads passed quality filtering. After chimera filtering, there were 1
459
034 reads that corresponded to 13
138 OTU's. We filtered out 1584 tag jumps from the reads. In Gill et al.'s study (which included non-engineered soils from parks and tree pits), 2
933
063 ITS reads were obtained from 102 samples that clustered into 7448 ASVs.33 We note that the amplicon targets between this study and Gill et al. are different, along with the bioinformatics pipeline and post-processing analysis. Gill et al. used the ITS1-F/ITS2 primer pairs targeting the first internal transcribed spacer region and the DADA2 pipeline. Despite differences, given the number of samples processed (29 including the non-template control compared to 102 from Gill et al.'s study), the similar number of final unique OTU's provides an initial indication of comparable sequencing depth between studies.
Results from PERMANOVA analysis (Table 1) provide insight into the variables that influence differences in community composition (beta diversity). Soil characteristics were not significant explanatory variables for beta diversity. Rather, a few key planting variables explained 89% of the variability in the distance matrix: the presence of native grasses (R2 = 0.43), the presence of forbs (R2 = 0.12), and the type of plant determined to be predominant in the cell (R2 = 0.24) based on sampling field notes and site images. Presence/absence of different plants were coded as Boolean variables. For example, if native grasses such as switchgrass (Panicum virgatum) were recorded as present within the cell and confirmed in pictures, presence of native grasses was coded as TRUE (otherwise coded as FALSE.) Presence/absence variables do not necessarily indicate the plant is dominant, but simply that it was growing within the cell. In contrast, the type of plant determined to be “dominant” in a cell was based on the abundance of the plant within a cell; for example, if the cell consisted of mostly Black-eyed Susan (Rudbeckia fulgida) and other forbs, the dominant planting type was classified as “forb”. Some cells had multiple planting types that could be considered dominant (Fig. S.2†) and were classified as “mixed”. PERMDISP analyses of each of the statistically significant PERMANOVA variables indicate homogeneous dispersions within groups for the presence of forbs variable and the dominant planting variable, but not for the native grasses nor legumes variables (Table S.11 and Fig. S.15–S.18† for PERMDISP results). For the native grasses/legumes variables, the significant results from the PERMDISP indicate that effects from within group dispersion (variances) could be confounding the results from the PERMANOVA; in essence, although the PERMANOVA is not ‘invalid’ per se, caution must be taken when interpreting results. The statistically significant PERMDISP implicates that within-group variance is also driving some of the differences seen in beta diversity and not solely the effect from presence of native grasses. These within-group variances are further explored using other visualizations (Fig. S.19 and S.20†). For the other two variables, the results of the PERMDISP further confirm the appropriateness of the PERMANOVA test, and within-group variances do not need to be considered when evaluating effects on beta diversity from the variables.
Table 1 Results from the PERMANOVA analyses. The presence of different plant types in the “Plantings Model” were treated as separate variables for each planting type, with presence indicated in Boolean “TRUE” or “FALSE”. Statistically significant variables are marked with an asterisks (* means p value between 0.01 and 0.05, ** means p value < 0.01). Variables lacking asterisks had p values > 0.05
Model variables |
Total R2 explained by the model |
p-Value |
“Soils model” soil pH, OM (%), NO3, ammonium, Zn, Mn, Cu, P |
0.14 |
0.927 |
|
“Plantings model” rooting depth, presence of different plant types (forbs**, weeds, turf grass, trees, legumes, native grass**, decorative cultivars, weeds), dominant planting*, city |
0.89 |
0.003 |
|
“Geography model” city**, sampling location, runoff type (parking lot, roof, minor road, major road) |
0.75 |
0.008 |
In the visualization of the PCA for the distance matrix, we colored points by the variables explaining the most variation: type of dominant planting, presence of native grasses, and presence of forbs (Fig. 1). Legumes, while a statistically significant variable from the PERMANOVA model, were excluded from the PCA analysis due to the low R2 (0.04). The PCA ordination explained 79% of the variance in PC1, and 21% of the variance in PC2. The high values of variance explained is not uncommon for this type of ordination in microbiome datasets.43,51 When examining the PCA for the native grasses, we can consider the results from both the PERMANOVA and PERMDISP tests (i.e., visually check for differences in within-group variation). The cells lacking native grasses visually appear to have heterogenous dispersion (spread unevenly within the group); given the significance of other planting variables, we posit that other planting types within the cells with no native grasses could be influencing results, in addition to the effect of not having native grasses. Within the six sites with no native grasses present, there were three within the group that are turf grass cells, two that were dominated by legumes, and one that had mostly weeds but legumes present. These planting types are physiologically different, with turf grass having short rooting depths and less transpiration than legumes. These differences could explain the significant result of the PERMDISP for the presence of native grasses.
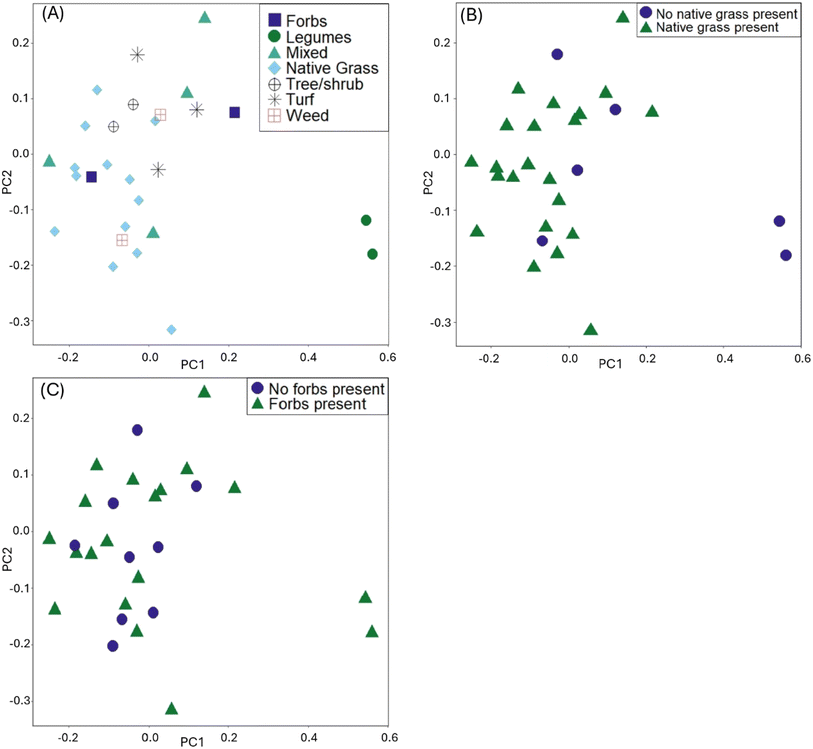 |
| Fig. 1 Principal component analysis plots using the rCLR-transformed data from DEICODE, colored by: (A) dominant planting type, (B) presence of native grasses, (C) presence of native forbs. | |
The cluster dendrogram (Fig. 2) based on the beta diversity rCLR-transformed Aitchison distance matrix is another visualization method for relatedness between sampling locations. The two North Liberty cells appear to be distinct, exhibiting a high branch height and clustering separately from all other samples. These two cells were the only cells with legumes as the dominant planting. Given the small sample size for this dominant planting group, it is challenging to attribute the difference to just one variable and should be considered as an area for future research. In contrast to the legume-dominant cells, the cells sampled from Cedar Rapids do not all cluster together, despite having some similarities in plantings within those sampling locations. Another interesting separation on the dendrogram is the “cvcell14top” vs. “cvcell14bottom” sample. At this cell, we sampled at two different depths in the soil cores – one at the top 10 cm and one at the bottom 10 cm of the corer. Though the sample size for depth is limited, this preliminary indication of difference with depth suggests that soil depth could be another variable of interest.
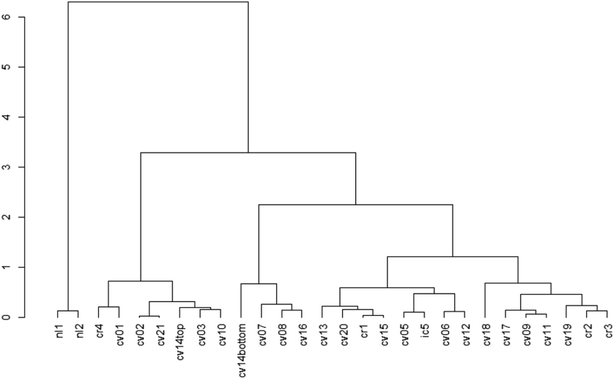 |
| Fig. 2 Dendrogram showing beta diversity differences based on the rCLR-transformed data with Aitchison distances. Labels are each sampling site, with the first two letter abbreviations standing for city names (‘nl’ = North Liberty, ‘cv’ = Coralville, ‘cr’ = Cedar Rapids, ‘ic’ = Iowa City). The height and branching of the dendrogram indicate differences, where samples clustered on the same branch at “shorter” heights are more similar, and samples that have “high” branch heights are less similar. | |
Altogether, the strong contributions from the planting categorical variables strengthens the evidence from Gill et al.33 who reported that differences in micro-environment drove community differences. In a separate study on influence of plant transpiration rates on fungal/bacterial diversity in bioswales, Brodsky et al.34 found that plants with higher transpiration rates were associated with higher fungal and bacterial diversity. Though planting categorical variables appear to explain much of the variation in the data for our study, we cannot rule out the possibility that further variability could be explained by site-specific characteristics not considered here, such as redox conditions, presence of a saturated zone, size of the cell/runoff volume, specific pollutant loading, cell age, cell maintenance strategies/frequency, etc. We also note that certain fungi are likely excluded from this study based on the sampling design. Examples of excluded fungi are endomycorrhizal fungi and plant pathogens that would inhabit within the vegetation of the bioretention cells, because only soil samples were analyzed. Additionally, fungal community variation with depth was not studied, as soil samples were collected near the subsurface of the cell, excepting cell 14. This limitation could exclude fungi that can survive under low oxygen conditions. Thus, while subsurface soil diversity was studied, more studies may be needed to further understand fungal diversity in bioretention cells.
Plots of mean relative abundance data (Fig. 3, S.19 and 20†) provide another way to compare the fungal communities between our study and Gill et al.'s and insight into taxonomic information. One key difference is the abundance of Ascomycota (a diverse phylum with the largest number of fungal species); in Gill et al.'s study, Ascomycota represent over 50% of the fungal sequences in all sample types.33 A different study on microbiomes of urban greenspaces (non-engineered soils) had 40–50% abundance for Ascomycota.57 Surprisingly, Ascomycota abundance did not fall within the 40–50% range for all samples. Only forb- and turf-dominant cells exhibit close to 50% abundance of Ascomycota, with abundance at or below 30% in legume-, trees/shrubs-, and weed-dominant cells. Another unexpected result is the high percentage of Basidiomycota relative to Ascomycota within our samples, especially in the weed-dominant cells. Basidiomycota include a multitude of fungal taxa that fall on the “white/brown rot continuum”.58 These fungal taxa could aid in contaminant removal within bioretention cells, as demonstrated in our previous work.59
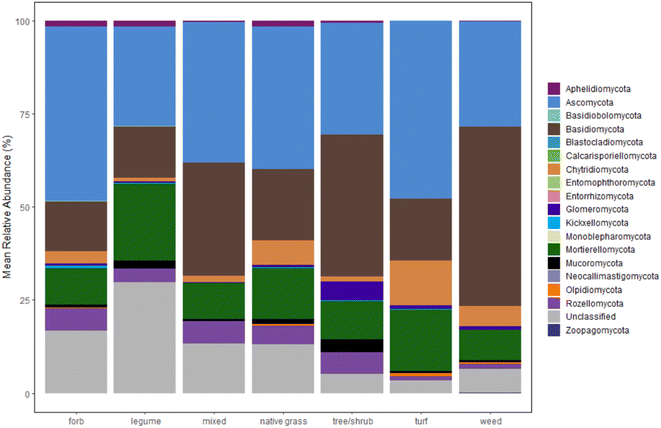 |
| Fig. 3 Relative abundance of fungal phyla for bioretention cells, grouped by the dominant planting type in each cell. Number of cells for each category is as follows: forb (n = 2), legume (n = 2), mixed (n = 4), native grass (n = 13), tree/shrub (n = 2), turf (n = 3), weed (n = 2). | |
The distribution of other phyla is also notable. Interestingly, the early-diverging phylum, Aphelidiomycota, was detected with a relative abundance around 2% in forb-, legume-, and native grass-dominant samples. Aphelidiomycota, once considered protists and/or a sister group to fungi, parasitize algae and diatoms and may have a role in leaf litter decomposition.60,61 The majority of fungi with an arbuscular mycorrhizal lifestyle are found in the Glomeromycota phylum. Within our samples, Glomeromycota were scarce in most samples, except in cells with trees/shrubs (5% abundance), and were only detected at or above 1% in samples with dominant plantings of trees/shrubs, turf, and weeds. Surprisingly, the two cells with dominant legumes (the North Liberty cells that also grouped separately in the dendrogram) had negligible Glomeromycota relative abundance, despite evidence that legumes host higher arbuscular mycorrhizal diversity relative to some native grass species.62 Indeed, the opposite was true within our results. Thus, there is a possible need for mycorrhizal inoculation within bioretention cells to enhance benefits from mycorrhizal fungi, as well as a need to further investigate the presence of mycorrhizal fungi in legume-dominant bioretention cells.
3.2 Functional gene quantification
All target functional genes were successfully amplified in DNA extracted from each soil sample (Fig. 4 and Table S12;† results for each sampling location presented in Fig. S.21–S.23†). We note that the use of degenerate primers required non-standard thermocycling conditions, which could be further optimized to increase amplification efficiency (quality control information described in Methods and ESI†). Gene copies normalized by gram of soil varied by orders of magnitude relative to each functional gene (Fig. 4(A); each significantly different p < 0.0001), with nirKf (fungal nitrite reductase) having the least overall abundance (mean copies per g dry soil: 7.4 × 105, range: 8.7 × 104–1.6 × 106) and Cu1A laccase having the highest abundance (mean copies per g dry soil: 4.0 × 108, range: 3.2 × 107–1.1 × 109; Fig. 4 and Table S.12†). In contrast to our results above wherein planting explained significant variability in the community diversity, we observed no significant differences in the functional genes between planting types (p > 0.8; Fig. 4(B)). Studies measuring fungal nirKf abundance report 107–109 copies g−1 for a variety of arable soils with histories of fertilizer application.63,64 The lack of heavy fertilization and the difference between engineered and arable soil properties could explain the lower abundance of nirKf. Nonetheless, these results warrant further investigation into the role of fungi in denitrification within bioretention cells, particularly because nirKf is involved in nitrous oxide emissions. We also note that existing nirKf primers do not amplify the full diversity of fungal nirK genes.65 Thus, our results could be missing other fungal nitrite reductase gene abundances. Nonetheless, the successful measurement of fungal nitrite reductase and laccases functional gene abundance in bioretention cell soils indicates the presence of fungi relevant to biotransformation of stormwater contaminants.
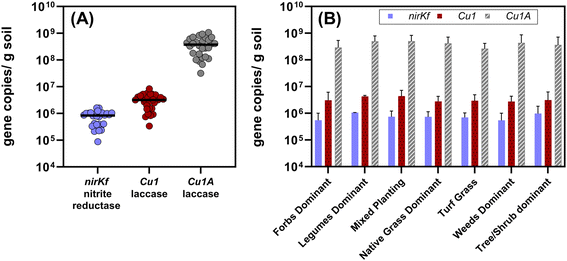 |
| Fig. 4 (A) Aggregated quantified functional gene copies per gram dry soil quantified from all of the bioretention sites (n = 28, including the composite sample from the bottom 10 cm of the core). Each dot represents the mean of triplicate extractions from composited soil samples collected from each site. The black bars represent the median value for each functional gene for all sites. Each gene set was significantly different from the others (p < 0.0001; Cu1A > Cu1 > nirKf). (B) Functional gene copies separated by dominant planting type. Plot bars represent mean with standard error. The functional gene quantities were not different between dominant plant type (p > 0.8) for a given functional gene. Additional information on the sites and planting type designations is in the ESI.† | |
Soil characteristics correlated with functional gene abundance in surprising ways (Fig. 5). Despite the involvement of fungal nirK in the nitrogen cycle, neither soil nitrate nor soil ammonium was significantly or strongly correlated with nirKf gene abundance. Somewhat similarly, none of the functional gene abundances were correlated with soil copper content, despite both laccase and nirKf being copper-containing enzymes. All functional genes were significantly positively correlated (p < 0.05) with soil pH and percent organic matter. Additionally, we found that percent organic matter (OM) and pH result in statistically significant differences in gene abundance for each functional gene (Fig. S.24;† note that OM/pH graphs would be equivalent and only one is depicted, as the samples group in the same manner for both variables). When we compared functional gene abundance for samples with percent OM less than the median to samples with percent OM greater than the median, we found statistically significant differences between the means, as indicated by the 95% confidence intervals excluding zero for all functional genes. We posit that the correlation between functional genes and percent organic matter as well as the differences in gene abundances with percent OM are likely because higher organic matter could result in a higher overall fungal richness.66 Indeed, based on the correlation matrix, it appears that percent OM and pH are driving soil characteristics for fungal species carrying these functional genes. These findings in stormwater bioretention cells are consistent with related literature from the fields of mycology/soil science. For example, soil fungal communities in the natural environment are known to vary with depth and organic matter content,67,68 with greater richness and diversity in the organic horizon69 that decreases with depth.70,71 Additionally, soil pH has been shown to affect fungal abundance and composition, e.g., resulting in changes in nutrient cycling through stimulated heterotrophic nitrification.72 Nevertheless, we caveat the interpretation of pH with the results in that pH values were generally circumneutral (mean of 7.4 ± 0.06); thus large deviations in soil pH are not expected, whereas percent organic matter can vary depending on the proportion of compost added to the geomedia. In the context of Spearman's correlations between functional gene abundance and site characteristic categorical variables were generally not statistically significant (Fig. S.25†); Cu1 was weakly negatively correlated with the presence of forbs (p = 0.044, rho = −0.383) and nirKf was weakly positively correlated with smaller-scale local geography (p = 0.042, rho = 0.388). Local geography was categorized based on within-city areas (e.g., “Iowa River Landing”) whereas geography was categorized based on city. It is possible that the fungi represented by the selected functional genes are widespread within bioretention cells, which could explain the weak correlation with planting variables and significant correlation with soil organic matter and pH.
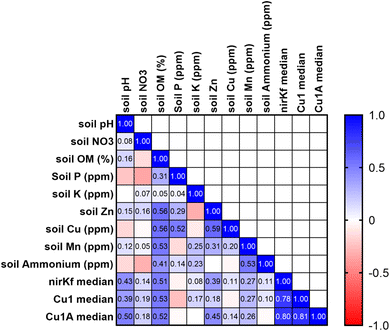 |
| Fig. 5 Pearson's correlation matrix for soil physicochemical characteristics and functional gene abundance. Median functional gene abundances were calculated for each site and used in the analysis. Numerical values are correlation coefficients for statistically significant values (rho < 0.05). | |
Our work demonstrated the utility of measuring fungal functional genes in bioretention cells. Pairing functional gene abundance measurements with fungal sequencing allows for a quantitative metric of key processes along with knowledge of the fungal community. One challenge of using DNA extracts from soil is that there is a possibility for detection of “relic” DNA, or DNA from organisms no longer alive.73 This could be potentially problematic if a study's goal is to capture a fully representative picture of bioretention function. An alternative could be RNA extraction, although this process has substantial methodological challenges. Nonetheless, qPCR is a useful method to understand how bioretention design parameters (e.g., amount of compost addition) enhances or reduces desirable biotic functions such as contaminant transformation. Future studies could investigate impacts from bioretention saturated zones, further optimize qPCR primer specificity, and target a wider range of fungal functional genes. Altogether, our study increases understanding of factors influencing fungal diversity and functional gene abundance in bioretention cells and revealed fruitful areas of further research.
In a broader context, there is only limited existing work investigating the role of microbial (and even less on fungal) diversity or functional genes and their role in green stormwater infrastructure (GSI). Several papers studying GSI practices in New York City have characterized microbial community diversity in vegetated swales, right-of-way bioswales, tree pits, and other practices. In one investigation,37 bacterial communities in engineered soils were compositionally distinct from non-engineered soils/not-engineered site. Specifically, bioswales exhibited distinct, phylogenetically diverse communities, including taxa associated with nutrient cycling and contaminants biodegradation. Bioswale soils also had a significantly greater diversity of genes involved in several functional pathways, including carbon and nitrogen cycling, as well as for degradation of a diverse suite of contaminants (e.g., noxZ and amoA for N cycling; bphA and monooxygenases for contaminant biodegradation). GSI design had significant impacts on measured microbial variables.74 Nevertheless, a subsequent NYC study indicated bacterial communities in GSI had levels of diversity similar to nonurban soils.75 A study of microbial (bacteria, archaea) and fungal diversity of urban greenspaces in France76 reported fungal richness increased in urban agriculture soils while bacterial richness was lower in public leisure areas. Similar to our findings, trace metals nor PAH contents in their study explained variations in microbial communities; organic carbon and C/N best predicted overall biomass. A study in the southwest US studying divergence of microbial communities in GSI soils concluded that diversifying vegetation can lead to positive feedback cycles between plants and microbes.77 These positive feedback cycles can result in more resilient systems and higher carbon storage, strengthening evidence for the importance of vegetation–microbe relationships in GSI. Indeed, a recent study on greenroofs78 in the Midwestern US reported greenroof fungal community composition was distinct between their nearby ground areas and between cities, positively correlated with plant cover. The overall paucity of work characterizing fungal (and even bacterial) communities in GSI practices represents a critical need—especially in the context of potential to bioremediation captured stormwater contaminants37–39,59—and warrants further investigation.
4. Conclusions
This work is the first to measure fungal diversity of bioretention cells in the Midwest US and to use qPCR to quantify fungal functional gene abundances in bioretention soils. Our results further emphasize the importance of plant selection in bioretention cells, as well as reveal soil characteristics that drive differences in fungal functional gene abundance. Plant selection already has a myriad of effects on bioretention cell function, including affecting infiltration rates of stormwater, contaminant removal by plants, bacterial functional genes for contaminant degradation, and longevity of the cell (i.e., preventing or causing clogging).79 This study observed differences in fungal communities and was able to attribute some of the differences to plantings. We hypothesize that plant selection and dominance could also impact fungal diversity in bioretention cells; thus, consideration of plants in bioretention cell design to improve biodegradation potential is warranted for further investigation. One example of benefits that may overlap between specific plant selection and fungal diversity is demonstrated by native grasses. Native grasses have extensive rooting systems that allow for increased evapotranspiration,79 and from our results, the presence of native grasses increased the relative abundance of Basidiomycota, the phylum with numerous brown/white rot fungi. Glomeromycota, the phylum comprising most arbuscular mycorrhizal fungi, were not found to be highly relatively abundant in our samples, even in the two cells dominated by legumes, implicating a possible need for fungal inoculation or bioaugmentation. Overall, our study strengthens evidence for connections between plants and fungal diversity in bioretention. There is a broader need to understand the intricacies of interactions between plants, fungi, and bacteria within bioretention cells, including potential for antagonistic or positive interactions between organisms.
Our work also demonstrates potential for in situ fungal biotransformation within GSI practices. We noted the unexpectedly high representation of Basidiomycota within our samples, a fungal taxa that fall on the “white/brown rot continuum”.58 These fungal taxa could aid in contaminant removal within bioretention cells, as demonstrated in our previous work59 that white-rot fungi biodegrade toxic tire-wear compounds. White-rot fungi are able to biodegrade a wide suite of other recalcitrant organic contaminants that may be present in stormwater (e.g., some pesticides80–82) and may otherwise accumulate in bioretention cells. Indeed, a recent study revealed that more recalcitrant, higher molecular weight PAHs were accumulating at the mid-depth below the surface of a bioretention cell where biodegradation may be limited, despite presence of PAH-ring cleaving dioxygenase bacterial genes at the surface.15 This suggests potential utility for in situ fungal bioremediation in GSI, and indicates value in investigating fungal bioaugmentation/inoculation opportunities of native/beneficial bioremediating fungi.83 Another area for further exploration would be the impact of bioretention geomedia amendments to fungal diversity in bioretention cells, as the addition of biochar can impact beta diversity of fungi due to alterations in soil characteristics.84 With growing interest in synergizing coupled contaminant sorption to geomedia with subsequent biological degradation in stormwater systems,21,83 probing the relationships between fungi and bioretention geomedia will be critical. Our novel reporting of fungal denitrification genes in bioretention systems also points towards a possible role of fungi for enhanced nutrient cycling in GSI; there is growing interest in using passive treatment systems for non-point nitrogen pollution control85–88 (e.g., bioretention, woodchip bioreactors). Overall, our work points to great value in further understanding the fungal communities of GSI and potential for improved contaminant degradation.
Data availability
The data supporting this article have been included as part of the ESI.†
Conflicts of interest
There are no conflicts of interest to declare.
Acknowledgements
This material is based upon work supported by the National Science Foundation (NSF) Graduate Research Fellowship under Grant No. 1546595. Additional support for this work was provided by the NSF Division of Graduate Education under Grant No. 1633098 and by the NSF CBET CAREER under Grant No. 1844720. Any opinion, findings, and conclusions or recommendations expressed in this material are those of the authors and do not necessarily reflect the views of the National Science Foundation. Additional financial support was provided by the University of Iowa Graduate College Post-Comprehensive Research Fellowship. We thank Jim Greene, Amy Foster, Kasey Hutchinson, Cara Matteson, and Mike Wolfe for assisting with locating and acquiring permission to sample at bioretention cells as well as their enthusiastic engagement with our work. We thank Grant Hemphill and Rachel Smoak for assistance with bioretention cell soil sampling. We also thank Qin Dong and Dr Tim Mattes for their guidance in laboratory and data analysis procedures. Our gratitude goes out to Dr Sten Anslan (University of Tartu) for responding to inquiries about DEICODE implementation in PipeCraft. We express our appreciation for the University of Iowa Institute of Human Genetics Genomics Division for qPCR sample processing.
References
- G. LeFevre, K. Paus, P. Natarajan, J. Gulliver, P. Novak and R. Hozalski, Review of Dissolved Pollutants in Urban Storm Water and Their Removal and Fate in Bioretention Cells, J. Environ. Eng., 2015, 141(1), 4014050, DOI:10.1061/(ASCE)EE.1943-7870.0000876
.
- S. Spahr, M. Teixidó, D. L. Sedlak and R. G. Luthy, Hydrophilic Trace Organic Contaminants in Urban Stormwater: Occurrence, Toxicological Relevance, and the Need to Enhance Green Stormwater Infrastructure, Environ. Sci.: Water Res. Technol., 2020, 6(1), 15–44, 10.1039/C9EW00674E
.
- J. R. Masoner, D. W. Kolpin, I. M. Cozzarelli, L. B. Barber, D. S. Burden, W. T. Foreman, K. J. Forshay, E. T. Furlong, J. F. Groves, M. L. Hladik, M. E. Hopton, J. B. Jaeschke, S. H. Keefe, D. P. Krabbenhoft, R. Lowrance, K. M. Romanok, D. L. Rus, W. R. Selbig, B. H. Williams and P. M. Bradley, Urban Stormwater: An Overlooked Pathway of Extensive Mixed Contaminants to Surface and Groundwaters in the United States, Environ. Sci. Technol., 2019, 53(17), 10070–10081, DOI:10.1021/acs.est.9b02867
.
- B. Seiwert, P. Klöckner, S. Wagner and T. Reemtsma, Source-Related Smart Suspect Screening in the Aqueous Environment: Search for Tire-Derived Persistent and Mobile Trace Organic Contaminants in Surface Waters, Anal. Bioanal. Chem., 2020, 412(20), 4909–4919, DOI:10.1007/s00216-020-02653-1
.
-
W. F. Hunt, R. G. Traver, A. P. Davis, C. H. Emerson, K. A. Collins and J. H. Stagge, Low Impact Development Practices: Designing to Infiltrate in Urban Environments, in Effects of Urbanization on Groundwater, American Society of Civil Engineers, Reston, VA, 2010, pp. 308–343 Search PubMed
.
- A. P. Davis, W. F. Hunt, R. G. Traver and M. Clar, Bioretention Technology: Overview of Current Practice and Future Needs, J. Environ. Eng., 2009, 135(3), 109–117, DOI:10.1061/(ASCE)0733-9372(2009)135:3(109)
.
- J. E. Grebel, S. K. Mohanty, A. A. Torkelson, A. B. Boehm, C. P. Higgins, R. M. Maxwell, K. L. Nelson and D. L. Sedlak, Engineered Infiltration Systems for Urban Stormwater Reclamation, Environ. Eng. Sci., 2013, 30(8), 437–454, DOI:10.1089/ees.2012.0312
.
- T. F. M. Rodgers, L. Wu, X. Gu, S. Spraakman, E. Passeport and M. L. Diamond, Stormwater Bioretention Cells Are Not an Effective Treatment for Persistent and Mobile Organic Compounds (PMOCs), Environ. Sci. Technol., 2022, 56(10), 6349–6359, DOI:10.1021/acs.est.1c07555
.
- K. Zhang, A. Randelovic, D. Page, D. T. McCarthy and A. Deletic, The Validation of Stormwater Biofilters for Micropollutant Removal Using in Situ Challenge Tests, Ecol. Eng., 2014, 67, 1–10, DOI:10.1016/j.ecoleng.2014.03.004
.
- T. F. M. Rodgers, S. Spraakman, Y. Wang, C. Johannessen, R. C. Scholes and A. Giang, Bioretention Design Modifications Increase the Simulated Capture of Hydrophobic and Hydrophilic Trace Organic Compounds, Environ. Sci. Technol., 2024, 58(12), 5500–5511, DOI:10.1021/acs.est.3c10375
.
- S. K. Mohanty, R. Valenca, A. W. Berger, I. K. M. Yu, X. Xiong, T. M. Saunders and D. C. W. Tsang, Plenty of Room for Carbon on the Ground: Potential Applications of Biochar for Stormwater Treatment, Sci. Total Environ., 2018, 625, 1644–1658, DOI:10.1016/j.scitotenv.2018.01.037
.
- R. A. Tirpak, A. N. Afrooz, R. J. Winston, R. Valenca, K. Schiff and S. K. Mohanty, Conventional and Amended Bioretention Soil Media for Targeted Pollutant Treatment: A Critical Review to Guide the State of the Practice, Water Res., 2021, 189, 116648, DOI:10.1016/j.watres.2020.116648
.
- S. Spahr, M. Teixidó, S. S. Gall, J. C. Pritchard, N. Hagemann, B. Helmreich and R. G. Luthy, Performance of Biochars for the Elimination of Trace Organic Contaminants and Metals from Urban Stormwater, Environ. Sci.: Water Res. Technol., 2022, 8(6), 1287–1299, 10.1039/D1EW00857A
.
- B. A. Ulrich, M. Loehnert and C. P. Higgins, Improved Contaminant Removal in Vegetated Stormwater Biofilters Amended with Biochar, Environ. Sci.: Water Res. Technol., 2017, 3(4), 726–734, 10.1039/C7EW00070G
.
- C. Yuan, A. P. Davis, D. Kaya and B. V. Kjellerup, Distribution and Biodegradation Potential of Polycyclic Aromatic Hydrocarbons (PAHs) Accumulated in Media of a Stormwater Bioretention, Chemosphere, 2023, 336, 139188, DOI:10.1016/j.chemosphere.2023.139188
.
- A. Skorobogatov, J. He, A. Chu, C. Valeo and B. van Duin, The Impact of Media, Plants and Their Interactions on Bioretention Performance: A Review, Sci. Total Environ., 2020, 715, 136918, DOI:10.1016/j.scitotenv.2020.136918
.
- H. S. Fowdar, B. E. Hatt, T. Cresswell, J. J. Harrison, P. L. M. Cook and A. Deletic, Phosphorus Fate and Dynamics in Greywater Biofiltration Systems, Environ. Sci. Technol., 2017, 51(4), 2280–2287, DOI:10.1021/acs.est.6b04181
.
- G. H. LeFevre, C. E. Müller, R. J. Li, R. G. Luthy and E. S. Sattely, Rapid Phytotransformation of Benzotriazole Generates Synthetic Tryptophan and Auxin Analogs in Arabidopsis, Environ. Sci. Technol., 2015, 49(18), 10959–10968, DOI:10.1021/acs.est.5b02749
.
- J. Pritchard, Y.-M. Cho, N. Ashoori, J. Wolfand, J. Sutton, M. Carolan, E. Gamez, K. Doan, J. Wiley and R. Luthy, Benzotriazole Uptake and Removal in Vegetated Biofilter Mesocosms Planted with Carex Praegracilis, Water, 2018, 10(11), 1605, DOI:10.3390/w10111605
.
- X. Gu, T. F. M. Rodgers, S. Spraakman, T. Van Seters, R. Flick, M. L. Diamond, J. Drake and E. Passeport, Trace Organic Contaminant Transfer and Transformation in Bioretention Cells: A Field Tracer Test with Benzotriazole, Environ. Sci. Technol., 2021, 55(18), 12281–12290, DOI:10.1021/acs.est.1c01062
.
- A. C. Portmann, G. H. LeFevre, R. Hankawa, D. Werner and C. P. Higgins, The Regenerative Role of Biofilm in the Removal of Pesticides from Stormwater in Biochar-Amended Biofilters, Environ. Sci.: Water Res. Technol., 2022, 8(5), 1092–1110, 10.1039/D1EW00870F
.
- L. Tedersoo, M. Bahram and M. Zobel, How Mycorrhizal Associations Drive Plant Population and Community Biology, Science, 2020, 367(6480) DOI:10.1126/science.aba1223
.
- A. Berruti, E. Lumini, R. Balestrini and V. Bianciotto, Arbuscular Mycorrhizal Fungi as Natural Biofertilizers: Let's Benefit from Past Successes, Front. Microbiol., 2016, 6(JAN), 1559, DOI:10.3389/fmicb.2015.01559
.
- B. K. Winfrey, B. E. Hatt and R. F. Ambrose, Arbuscular Mycorrhizal Fungi in Australian Stormwater Biofilters, Ecol. Eng., 2017, 102, 483–489, DOI:10.1016/j.ecoleng.2017.02.041
.
- C. Poor, C. Balmes, M. Freudenthaler and A. Martinez, Role of Mycelium in Bioretention Systems: Evaluation of Nutrient and Metal Retention in Mycorrhizae-Inoculated Mesocosms, J. Environ. Eng., 2018, 144(6), 04018034, DOI:10.1061/(ASCE)EE.1943-7870.0001373
.
- Y. M. Palacios, R. Gleadow, C. Davidson, W. Gan and B. Winfrey, Do Mycorrhizae Increase Plant Growth and Pollutant Removal in Stormwater Biofilters?, Water Res., 2021, 202, 117381, DOI:10.1016/j.watres.2021.117381
.
- Y. M. Palacios and B. K. Winfrey, Three Mechanisms of Mycorrhizae That May Improve Stormwater Biofilter Performance, Ecol. Eng., 2021, 159, 106085, DOI:10.1016/j.ecoleng.2020.106085
.
- I. Kamei, S. Sonoki, K. Haraguchi and R. Kondo, Fungal Bioconversion
of Toxic Polychlorinated Biphenyls by White-Rot Fungus, Phlebia Brevispora, Appl. Microbiol. Biotechnol., 2006, 73(4), 932–940, DOI:10.1007/s00253-006-0529-9
.
- Č. Novotný, B. R. M. Vyas, P. Erbanová, A. Kubátová and V. Šašek, Removal of PCBs by Various White Rot Fungi in Liquid Cultures, Folia Microbiol., 1997, 42(2), 136–140, DOI:10.1007/BF02898723
.
- N. Golan-Rozen, B. Chefetz, J. Ben-Ari, J. Geva and Y. Hadar, Transformation of the Recalcitrant Pharmaceutical Compound Carbamazepine by Pleurotus Ostreatus: Role of Cytochrome P450 Monooxygenase and Manganese Peroxidase, Environ. Sci. Technol., 2011, 45(16), 6800–6805, DOI:10.1021/es200298t
.
- N. Golan-Rozen, B. Seiwert, C. Riemenschneider, T. Reemtsma, B. Chefetz and Y. Hadar, Transformation Pathways of the Recalcitrant Pharmaceutical Compound Carbamazepine by the White-Rot Fungus Pleurotus Ostreatus: Effects of Growth Conditions, Environ. Sci. Technol., 2015, 49(20), 12351–12362, DOI:10.1021/acs.est.5b02222
.
- Y.-C. Toh, J. J. L. Yen, J. P. Obbard and Y.-P. Ting, Decolourisation of Azo Dyes by White-Rot Fungi (WRF) Isolated in Singapore, Enzyme Microb. Technol., 2003, 33(5), 569–575, DOI:10.1016/S0141-0229(03)00177-7
.
- A. S. Gill, K. Purnell, M. I. Palmer, J. Stein and K. L. McGuire, Microbial Composition and Functional Diversity Differ Across Urban Green Infrastructure Types, Front. Microbiol., 2020, 11, 912, DOI:10.3389/fmicb.2020.00912
.
- O. L. Brodsky, K. L. Shek, D. Dinwiddie, S. G. Bruner, A. S. Gill, J. M. Hoch, M. I. Palmer and K. L. McGuire, Microbial Communities in Bioswale Soils and Their Relationships to Soil Properties, Plant Species, and Plant Physiology, Front. Microbiol., 2019, 10, 2368, DOI:10.3389/fmicb.2019.02368
.
- L. J. Waller, G. K. Evanylo, L.-A. H. Krometis, M. S. Strickland, T. Wynn-Thompson and B. D. Badgley, Engineered and Environmental Controls of Microbial Denitrification in Established Bioretention Cells, Environ. Sci. Technol., 2018, 52(9), 5358–5366, DOI:10.1021/acs.est.7b06704
.
- X. Chen, E. Peltier, B. S. M. M. Sturm and C. B. Young, Nitrogen Removal and Nitrifying and Denitrifying Bacteria Quantification in a Stormwater Bioretention System, Water Res., 2013, 47(4), 1691–1700, DOI:10.1016/j.watres.2012.12.033
.
- A. S. Gill, A. Lee and K. L. McGuire, Phylogenetic and Functional Diversity of Total (DNA) and Expressed (RNA) Bacterial Communities in Urban Green Infrastructure Bioswale Soils, Appl. Environ. Microbiol., 2017, 83(16) DOI:10.1128/AEM.00287-17
.
- G. H. LeFevre, R. M. Hozalski and P. J. Novak, The Role of Biodegradation in Limiting the Accumulation of Petroleum Hydrocarbons in Raingarden Soils, Water Res., 2012, 46(20), 6753–6762, DOI:10.1016/j.watres.2011.12.040
.
- G. H. LeFevre, P. J. Novak and R. M. Hozalski, Fate of Naphthalene in Laboratory-Scale Bioretention Cells: Implications for Sustainable Stormwater Management, Environ. Sci. Technol., 2012, 46(2), 995–1002, DOI:10.1021/es202266z
.
- K. Ihrmark, I. T. M. Bödeker, K. Cruz-Martinez, H. Friberg, A. Kubartova, J. Schenck, Y. Strid, J. Stenlid, M. Brandström-Durling, K. E. Clemmensen and B. D. Lindahl, New Primers to Amplify the Fungal ITS2 Region - Evaluation by 454-Sequencing of Artificial and Natural Communities, FEMS Microbiol. Ecol., 2012, 82(3), 666–677, DOI:10.1111/j.1574-6941.2012.01437.x
.
-
T. J. White, T. Bruns, S. Lee and J. Taylor, Amplification and Direct Sequencing of Fungal Ribosomal RNA Genes for Phylogenetics, in PCR Protocols, Elsevier, 1990, pp. 315–322 Search PubMed
.
- S. Anslan, M. Bahram, I. Hiiesalu and L. Tedersoo, PipeCraft: Flexible Open-Source Toolkit for Bioinformatics Analysis of Custom High-Throughput Amplicon Sequencing Data, Mol. Ecol. Resour., 2017, 17(6), e234–e240, DOI:10.1111/1755-0998.12692
.
- L. Tedersoo, M. Bahram, L. Zinger, R. H. Nilsson, P. G. Kennedy, T. Yang, S. Anslan and V. Mikryukov, Best Practices in Metabarcoding of Fungi: From Experimental Design to Results, Mol. Ecol., 2022, 31(10), 2769–2795, DOI:10.1111/mec.16460
.
- L. Joos, S. Beirinckx, A. Haegeman, J. Debode, B. Vandecasteele, S. Baeyen, S. Goormachtig, L. Clement and C. De Tender, Daring to Be Differential: Metabarcoding Analysis of Soil and Plant-Related Microbial Communities Using Amplicon Sequence Variants and Operational Taxonomical Units, BMC Genomics, 2020, 21(1), 733, DOI:10.1186/s12864-020-07126-4
.
- T. Rognes, T. Flouri, B. Nichols, C. Quince and F. Mahé, VSEARCH: A Versatile Open Source Tool for Metagenomics, PeerJ, 2016, 4(10), e2584, DOI:10.7717/peerj.2584
.
- J. Bengtsson-Palme, M. Ryberg, M. Hartmann, S. Branco, Z. Wang, A. Godhe, P. De Wit, M. Sánchez-García, I. Ebersberger, F. de Sousa, A. S. Amend, A. Jumpponen, M. Unterseher, E. Kristiansson, K. Abarenkov, Y. J. K. Bertrand, K. Sanli, K. M. Eriksson, U. Vik, V. Veldre and R. H. Nilsson, Improved Software Detection and Extraction of ITS1 and ITS2 from Ribosomal ITS Sequences of Fungi and Other Eukaryotes for Analysis of Environmental Sequencing Data, Methods Ecol. Evol., 2013, 4(10), 914–919, DOI:10.1111/2041-210X.12073
.
- T. G. Frøslev, R. Kjøller, H. H. Bruun, R. Ejrnæs, A. K. Brunbjerg, C. Pietroni and A. J. Hansen, Algorithm for Post-Clustering Curation of DNA Amplicon Data Yields Reliable Biodiversity Estimates, Nat. Commun., 2017, 8(1), 1188, DOI:10.1038/s41467-017-01312-x
.
-
R. C. Edgar, UNCROSS2: Identification of Cross-Talk in 16S RRNA OTU Tables, bioRxiv, 2018, p. 400762, DOI:10.1101/400762.
-
K. Abarenkov, A. Zirk, T. Piirmann, R. Pöhönen, F. Ivanov, R. H. Nilsson and U. Kõljalg, UNITE General FASTA Release for Fungi, UNITE community, 2023, DOI:10.15156/BIO/2938067
.
- L. Tedersoo, S. Sánchez-Ramírez, U. Kõljalg, M. Bahram, M. Döring, D. Schigel, T. May, M. Ryberg and K. Abarenkov, High-Level Classification of the Fungi and a Tool for Evolutionary Ecological Analyses, Fungal Diversity, 2018, 90(1), 135–159, DOI:10.1007/s13225-018-0401-0
.
- C. Martino, J. T. Morton, C. A. Marotz, L. R. Thompson, A. Tripathi, R. Knight and K. Zengler, A Novel Sparse Compositional Technique Reveals Microbial Perturbations, mSystems, 2019, 4(1), e00016–e00019, DOI:10.1128/mSystems.00016-19
.
-
M. J. Anderson, Permutational Multivariate Analysis of Variance (PERMANOVA), in Wiley StatsRef: Statistics Reference Online, Wiley, 2017, pp. 1–15 Search PubMed
.
-
J. Oksanen, G. L. Simpson, F. G. Blanchet, R. Kindt, P. Legendre, P. R. Minchin, R. B. O'Hara, P. Solymos, M. H. H. Stevens, E. Szoecs, H. Wagner, M. Barbour, M. Bedward, B. Bolker, D. Borcard, G. Carvalho, M. Chirico, M. De Caceres, S. Durand, H. B. A. Evangelista, R. FitzJohn, M. Friendly, B. Furneaux, G. Hannigan, M. O. Hill, L. Lahti, D. McGlinn, M.-H. Ouellette, E. Ribeiro Cunha, T. Smith, A. Stier, C. J. F. Ter Braak and J. Weedon, Vegan: Community Ecology Package, 2022 Search PubMed
.
- P. Luis, G. Walther, H. Kellner, F. Martin and F. Buscot, Diversity of Laccase Genes from Basidiomycetes in a Forest Soil, Soil Biol. Biochem., 2004, 36(7), 1025–1036, DOI:10.1016/j.soilbio.2004.02.017
.
- H. Kellner, P. Luis and F. Buscot, Diversity of Laccase-like Multicopper Oxidase Genes in Morchellaceae: Identification of Genes Potentially Involved in Extracellular Activities Related to Plant Litter Decay, FEMS Microbiol. Ecol., 2007, 61(1), 153–163, DOI:10.1111/j.1574-6941.2007.00322.x
.
- W. Wei, K. Isobe, Y. Shiratori, T. Nishizawa, N. Ohte, Y. Ise, S. Otsuka and K. Senoo, Development of PCR Primers Targeting Fungal NirK to Study Fungal Denitrification in the Environment, Soil Biol. Biochem., 2015, 81, 282–286, DOI:10.1016/j.soilbio.2014.11.026
.
- M. Delgado-Baquerizo, D. J. Eldridge, Y.-R. Liu, B. Sokoya, J.-T. Wang, H.-W. Hu, J.-Z. He, F. Bastida, J. L. Moreno, A. R. Bamigboye, J. L. Blanco-Pastor, C. Cano-Díaz, J. G. Illán, T. P. Makhalanyane, C. Siebe, P. Trivedi, E. Zaady, J. P. Verma, L. Wang, J. Wang, T. Grebenc, G. F. Peñaloza-Bojacá, T. U. Nahberger, A. L. Teixido, X.-Q. Zhou, M. Berdugo, J. Duran, A. Rodríguez, X. Zhou, F. Alfaro, S. Abades, C. Plaza, A. Rey, B. K. Singh, L. Tedersoo and N. Fierer, Global Homogenization of the Structure and Function in the Soil Microbiome of Urban Greenspaces, Sci. Adv., 2021, 7(28), eabg5809, DOI:10.1126/sciadv.abg5809
.
- R. Riley, A. A. Salamov, D. W. Brown, L. G. Nagy, D. Floudas, B. W. Held, A. Levasseur, V. Lombard, E. Morin, R. Otillar, E. A. Lindquist, H. Sun, K. M. LaButti, J. Schmutz, D. Jabbour, H. Luo, S. E. Baker, A. G. Pisabarro, J. D. Walton, R. A. Blanchette, B. Henrissat, F. Martin, D. Cullen, D. S. Hibbett and I. V. Grigoriev, Extensive Sampling of Basidiomycete Genomes Demonstrates Inadequacy of the White-Rot/Brown-Rot Paradigm for Wood Decay Fungi, Proc. Natl. Acad. Sci. U. S. A., 2014, 111(27), 9923–9928, DOI:10.1073/pnas.1400592111
.
- E. A. Wiener and G. H. LeFevre, White Rot Fungi Produce Novel Tire Wear Compound Metabolites and Reveal Underappreciated Amino Acid Conjugation Pathways, Environ. Sci. Technol. Lett., 2022, 9(5), 391–399, DOI:10.1021/acs.estlett.2c00114
.
- P. M. Letcher and M. J. Powell, A Taxonomic Summary of Aphelidiaceae, IMA Fungus, 2019, 10(1), 4, DOI:10.1186/s43008-019-0005-7
.
- J. Li and C. Yang, Inconsistent Response of Soil Bacterial and Fungal Communities in Aggregates to Litter Decomposition during Short-Term Incubation, PeerJ, 2019, 2019(11), e8078, DOI:10.7717/peerj.8078
.
- A. H. Eom, D. C. Hartnett and G. W. T. Wilson, Host Plant Species Effects on Arbuscular Mycorrhizal Fungal Communities in Tallgrass Prairie, Oecologia, 2000, 122(3), 435–444, DOI:10.1007/s004420050050
.
- H. Xu, R. Sheng, X. Xing, W. Zhang, H. Hou, Y. Liu, H. Qin, C. Chen and W. Wei, Characterization of Fungal NirK-Containing Communities and N2O Emission From Fungal Denitrification in Arable Soils, Front. Microbiol., 2019, 10(FEB), 117, DOI:10.3389/fmicb.2019.00117
.
- Y. Lin, G. Ye, J. Luo, H. J. Di, S. Lindsey, J. Fan, D. Liu and W. Ding, Long-Term Organic Fertilization Regulates the Abundance of Major Nitrogen-Cycling-Related Genes in Aggregates from an Acidic Ultisol, Appl. Soil Ecol., 2021, 165, 104014, DOI:10.1016/j.apsoil.2021.104014
.
- Y. Ma, J. L. Zilles and A. D. Kent, An Evaluation of Primers for Detecting Denitrifiers via Their Functional Genes, Environ. Microbiol., 2019, 21(4), 1196–1210, DOI:10.1111/1462-2920.14555
.
- M. del M. Montiel-Rozas, Á. López-García, P. Madejón and E. Madejón, Native Soil Organic Matter as a Decisive Factor to Determine the Arbuscular Mycorrhizal Fungal Community Structure in Contaminated Soils, Biol. Fertil. Soils, 2017, 53(3), 327–338, DOI:10.1007/s00374-017-1181-5
.
- J. P. van Leeuwen, I. Djukic, J. Bloem, T. Lehtinen, L. Hemerik, P. C. de Ruiter and G. J. Lair, Effects of Land Use on Soil Microbial Biomass, Activity and Community Structure at Different Soil Depths in the Danube Floodplain, Eur. J. Soil Biol., 2017, 79, 14–20, DOI:10.1016/j.ejsobi.2017.02.001
.
- F. Ekelund, R. Rønn and S. Christensen, Distribution with Depth of Protozoa, Bacteria and Fungi in Soil Profiles from Three Danish Forest Sites, Soil Biol. Biochem., 2001, 33(4), 475–481, DOI:10.1016/S0038-0717(00)00188-7
.
- X. Luo, K. Liu, Y. Shen, G. Yao, W. Yang, P. E. Mortimer and H. Gui, Fungal Community Composition and Diversity Vary With Soil Horizons in a Subtropical Forest, Front. Microbiol., 2021, 12 DOI:10.3389/fmicb.2021.650440
.
- C. Du, Z. Geng, Q. Wang, T. Zhang, W. He, L. Hou and Y. Wang, Variations in Bacterial and Fungal Communities through Soil Depth Profiles in a Betula Albosinensis Forest, J. Microbiol., 2017, 55(9), 684–693, DOI:10.1007/s12275-017-6466-8
.
- T. Yang, J. M. Adams, Y. Shi, H. Sun, L. Cheng, Y. Zhang and H. Chu, Fungal Community Assemblages in a High Elevation Desert Environment: Absence of Dispersal Limitation and Edaphic Effects in Surface Soil, Soil Biol. Biochem., 2017, 115, 393–402, DOI:10.1016/j.soilbio.2017.09.013
.
- Y. Zhang, S. Dai, X. Huang, Y. Zhao, J. Zhao, Y. Cheng, Z. Cai and J. Zhang, PH-Induced Changes in Fungal Abundance and Composition Affects Soil Heterotrophic Nitrification after 30 Days of Artificial PH Manipulation, Geoderma, 2020, 366, 114255, DOI:10.1016/j.geoderma.2020.114255
.
- P. Carini, P. J. Marsden, J. W. Leff, E. E. Morgan, M. S. Strickland and N. Fierer, Relic DNA Is Abundant in Soil and Obscures Estimates of Soil Microbial Diversity, Nat. Microbiol., 2017, 2(3), 16242, DOI:10.1038/nmicrobiol.2016.242
.
- M. Deeb, P. M. Groffman, J. L. Joyner, G. Lozefski, A. Paltseva, B. Lin, K. Mania, D. L. Cao, J. McLaughlin, T. Muth, B. Prithiviraj, J. Kerwin and Z. Cheng, Soil and Microbial Properties of Green Infrastructure Stormwater Management Systems, Ecol. Eng., 2018, 125, 68–75, DOI:10.1016/j.ecoleng.2018.10.017
.
- J. L. Joyner, J. Kerwin, M. Deeb, G. Lozefski, B. Prithiviraj, A. Paltseva, J. McLaughlin, P. Groffman, Z. Cheng and T. R. Muth, Green Infrastructure Design Influences Communities of Urban Soil Bacteria, Front. Microbiol., 2019 DOI:10.3389/fmicb.2019.00982
.
- A. Christel, S. Dequiedt, N. Chemidlin-Prevost-Bouré, F. Mercier, J. Tripied, G. Comment, C. Djemiel, L. Bargeot, E. Matagne, A. Fougeron, J.-B. Mina Passi, L. Ranjard and P.-A. Maron, Urban Land Uses Shape Soil Microbial Abundance and Diversity, Sci. Total Environ., 2023, 883, 163455, DOI:10.1016/j.scitotenv.2023.163455
.
- V. Buzzard, J. Gil-Loaiza, N. Graf Grachet, H. Talkington, C. Youngerman, M. M. Tfaily and L. K. Meredith, Green Infrastructure Influences Soil Health: Biological Divergence One Year after Installation, Sci. Total Environ., 2021, 801, 149644, DOI:10.1016/j.scitotenv.2021.149644
.
- A. G. Droz, R. R. Coffman, A. C. Eagar and C. B. Blackwood, Drivers of Fungal Diversity and Community Biogeography Differ between Green Roofs and Adjacent Ground-Level Green Space, Environ. Microbiol., 2022, 24(12), 5809–5824, DOI:10.1111/1462-2920.16190
.
- C. P. Muerdter, C. K. Wong and G. H. LeFevre, Emerging Investigator Series: The Role of Vegetation in Bioretention for Stormwater Treatment in the Built Environment: Pollutant Removal, Hydrologic Function, and Ancillary Benefits, Environ. Sci.: Water Res. Technol., 2018, 4(5), 592–612, 10.1039/c7ew00511c
.
- J. M. Wolfand, C. Seller, C. D. Bell, Y.-M. Cho, K. Oetjen, T. S. Hogue and R. G. Luthy, Occurrence of Urban-Use Pesticides and Management with Enhanced Stormwater Control Measures at the Watershed Scale, Environ. Sci. Technol., 2019, 53(7), 3634–3644, DOI:10.1021/acs.est.8b05833
.
- J. M. Wolfand, G. H. LeFevre and R. G. Luthy, Metabolization and Degradation Kinetics of the Urban-Use Pesticide Fipronil by White Rot Fungus Trametes Versicolor, Environ. Sci.: Processes Impacts, 2016, 18(10), 1256–1265, 10.1039/C6EM00344C
.
- L. M. M. Weaver, N. L. Alexander, M. A. Cubeta, D. R. U. Knappe and T. N. Aziz, Degradation of Imidacloprid by Phanerodontia Chrysosporium on Wood Chips for Stormwater Treatment, Environ. Sci.: Water Res. Technol., 2023, 9(12), 3333–3343, 10.1039/D3EW00545C
.
- D. S. Tanmoy and G. H. LeFevre, Development of Composite Alginate Bead Media with Encapsulated Sorptive Materials and Microorganisms to Bioaugment Green Stormwater Infrastructure, Environ. Sci.: Water Res. Technol., 2024, 10(8), 1890–1907, 10.1039/D4EW00289J
.
- Q. Yao, J. Liu, Z. Yu, Y. Li, J. Jin, X. Liu and G. Wang, Three Years of Biochar Amendment Alters Soil Physiochemical Properties and Fungal Community Composition in a Black Soil of Northeast China, Soil Biol. Biochem., 2017, 110, 56–67, DOI:10.1016/j.soilbio.2017.03.005
.
- B. J. Halaburka, G. H. LeFevre and R. G. Luthy, Quantifying the Temperature Dependence of Nitrate Reduction in Woodchip Bioreactors: Experimental and Modeled Results with Applied Case-Study, Environ. Sci.: Water Res. Technol., 2019, 5(4), 782–797, 10.1039/C8EW00848E
.
- B. J. Halaburka, G. H. LeFevre and R. G. Luthy, Evaluation of Mechanistic Models for Nitrate Removal in Woodchip Bioreactors, Environ. Sci. Technol., 2017, 51(9), 5156–5164, DOI:10.1021/acs.est.7b01025
.
- J. Li, T. B. Culver, P. P. Persaud and J. M. Hathaway, Developing Nitrogen Removal Models for Stormwater Bioretention Systems, Water Res., 2023, 120381, DOI:10.1016/j.watres.2023.120381
.
- E. V. Lopez-Ponnada, T. J. Lynn, S. J. Ergas and J. R. Mihelcic, Long-Term Field Performance of a Conventional and Modified Bioretention System for Removing Dissolved Nitrogen Species in Stormwater Runoff, Water Res., 2020, 170, 115336, DOI:10.1016/j.watres.2019.115336
.
|
This journal is © The Royal Society of Chemistry 2024 |
Click here to see how this site uses Cookies. View our privacy policy here.