DOI:
10.1039/D2NR01479C
(Paper)
Nanoscale, 2022,
14, 8833-8841
Cracking enabled unclonability in colloidal crystal patterns authenticated with computer vision†
Received
17th March 2022
, Accepted 17th May 2022
First published on 17th May 2022
Abstract
Colloidal crystals with iridescent structural coloration have appealing applications in the fields of sensors, displays, anti-counterfeiting, etc. A serious issue accompanying the facile chemical self-assembly approach to colloidal crystals is the formation of uncontrolled and irregular cracks. In contrast to the previous efforts to avoid cracking, the unfavorable and random micro-cracks in colloidal crystals were utilized here as unclonable codes for tamper-proof anti-counterfeiting. The special structural and optical characteristics of the colloidal crystal patterns assembled with monodisperse poly(styrene-methyl methacrylate-acrylic acid) core–shell nanospheres enabled multi-anti-counterfeiting modes, including angle-dependent structural colors and polarization anisotropy, besides the physically unclonable functions (PUFs) of random micro-cracks. Moreover, by using the random cracks in the colloidal crystals as templates to guide fluorescent silica nanoparticle deposition, an fluorescent anti-counterfeiting mode with PUFs was introduced. To validate the PUFs of the fluorescent micro-cracks in the colloidal crystals, a novel edge-sensitive template matching approach based on a computer vision algorithm with an accuracy of ∼100% was developed, enabling ultimate security immune to forgery. The computer-vision verifiable physically unclonable colloidal crystals with multi-anti-counterfeiting modes are superior to conventional photonic crystal anti-counterfeiting materials that rely on angle-dependent or tunable structural colors, and the conventional PUF labels in the aspect of decorative functions, which will open a new avenue for advanced security materials with multi-functionality.
1. Introduction
Chemical self-assembly is a facile and convenient approach to colloidal crystals that have wide-spread applications in sensors,1–3 displays,4,5 waveguides,6 anti-counterfeiting7–11 and high-performance optical devices.12 Uncontrolled and irregular micro-cracks would occur in the final drying stage of the common evaporation-induced self-assembly process, which is usually regarded as an unfavorable and challenging issue in the advancement of high-performance optical devices.13,14 For decades, researchers have been trying to find methods to avoid cracking in colloidal crystals, mainly through the optimization of evaporation conditions,15,16 assembly of soft nanoparticles,17 the co-assembly approach,18 crystallization on the liquid,19 superhydrophobic20 or lithographically patterned substrates,21etc.22,23 In contrast to the effort of trying to avoid cracking, our group directly “sewed” the cracks through in situ sequential chemical reactions with nanostructure transformations of iron-based species, after crack formation.24 On the other hand, several research groups have managed to regulate the cracks by flow-induced self-assembly25 or through the use of crack initiators with well-designed microscopic geometries14 and utilized the regular cracks as templates to produce 1D nanostructures or flexible conductors.25 However, the random irregular cracks normally occurring in the chemical self-assembly process are challenging to find any applications due to their randomness and uncontrolled nature.
Physical objects with inherent disordered structures could enable physically unclonable functions (PUFs).26–28 For instance, the randomness in the outputs (currents or voltages) of modern electronics originating from materials growth and device fabrication, including field-effect transistors,28–30 metal–oxide transistors,31 and memristors,32 could guarantee information security during communication by generating unclonable digital keys. However, electronic devices are usually costly and inconvenient for authentication of common goods. On the other hand, optical materials, based on the random arrangement of fluorescent nanowires,33 carbon dots,34 liquid crystals,35 plasmonic nano-islands,36 and light-scattering nanoparticles,37 provide a variety of low-cost platforms for PUFs. But the current electrical/optical PUFs usually lack attractive decorative functions when used in packaging for anti-counterfeiting purposes, or automatic and accurate validation.26–37 It is highly desirable to develop decorative coatings with PUFs and convenient validation for tamper-proof anti-counterfeiting applications.
In this work, the uncontrolled and irregular micro-cracks naturally occurring in the self-assembled decorative colloidal crystal patterns of poly(styrene-methyl methacrylate-acrylic acid) [P(St-MMA-AA)] arrays with iridescent structural colors were utilized as PUF codes for ultimate security. Angle-dependent structural colors under white-light illumination and the polarization anisotropy of the crystal structure under cross-polarized light enabled multi-anti-counterfeiting modes. Moreover, by using the random micro-cracks in colloidal crystals as templates to guide fluorescent silica nanoparticle deposition, fluorescent anti-counterfeiting mode was further introduced. To validate the PUFs of the fluorescent irregular micro-cracks, a novel edge sensitive template matching approach based on a computer vision algorithm with accuracy of ∼100% was developed. The first computer-vision verifiable anti-counterfeiting colloidal-crystals with PUFs developed herein are advantageous to conventional anti-counterfeiting photonic crystal materials depending on clonable iridescent and tunable structural colors,7,10,38–41 and conventional PUF labels in an aspect of decorative functions,26–37 which opens a new avenue for multi-functional materials with ultimate security.
2. Materials and methods
2.1. Fabrication of fluorescent colloidal crystals with diverse patterns
Monodisperse P(St-MMA-AA) particles with diameters of ∼166 nm, ∼186 nm, ∼204 nm, ∼228 nm, ∼243 nm, and ∼268 nm were synthesized by the one-pot emulsion polymerization method.42 Hydrophobic substrates with hydrophilic patterns were obtained according to the reported method.43 SiO2 nanoparticles with sizes of ∼194 nm were synthesized by a modified Stöber method and grafted with fluorescein isothiocyanate via a silane coupling reaction to obtain fluorescent SiO2 nanoparticles.44 The P(St-MMA-AA) nanoparticle dispersions (0.12 wt% in water) self-assembled on the substrates with designed wettability through vertical deposition at a constant temperature of 60 °C and a relative humidity of 60% to obtain the colloidal crystal patterns. Then, fluorescent SiO2 nanoparticle dispersions (0.15 wt% in water) were infiltrated into micro-crack templates of the colloidal crystals with the help of capillary forces to enable fluorescent anti-counterfeiting mode with PUFs.
2.2. Characterization
The photographs of the colloidal crystal films with diverse patterns were taken using a digital camera (Canon EOS 80D). The angle-resolved reflectance spectra of the colloidal crystals with different structural colors were recorded using a UV–vis optical fiber spectrometer (Ocean Optics Inc., HR 4000) via a 600 μm broadband optical fiber (Ocean Optics Inc., QP600-1-UV–vis). A xenon lamp was used as the light source with wavelengths from 250 to 800 nm. A standard high reflectivity specular mirror (Ocean Optics Inc., STAN-SSH) was used as the reference. Optical microscopy images of the colloidal crystal films were taken using a motorized optical microscope (Leica DM6M) under white light, UV light (400–450 nm), and cross-polarized light, which could be replaced by a more convenient portable microscope in practical situations. The high-resolution structures of the colloidal crystals were characterized by FESEM (Hitachi S-4800). The static water contact angles of the glass substrates were measured on an OCA20 contact-angle system (Data Physics, Germany) in an ambient environment.
2.3. Decoding of the random fluorescent micro-cracks with PUFs of the colloidal crystals via a computer vision algorithm
The automatic decoding process with computer vision was composed of 2 steps:45 crack edge extraction and similarity comparison. Since edge extraction was susceptible to noises in the image, we used a Gaussian filter to remove the noises. Then, a Sobel kernel is applied to the smoothed image to obtain the intensity and direction of each pixel. The resulting image consisted of both thick and thin edges, and the non-maximum suppression could help in mitigating the thick edges and retaining the thin ones. Finally, two threshold values, namely minVal and maxVal, were used to further filter out edges: any edges with an intensity gradient below minVal were discarded and edges with an intensity gradient above maxVal were considered as true edges, and those in between were classified based on their connectivity. All these steps were put together in one function termed cv2.Canny (in OpenCV). The choice of the threshold values was important in edge extraction as the images were captured under different lighting conditions, which could result in different outputs of detected edges. To solve this, we used adaptive thresholding instead of using a fixed global value for all the images. Specifically, Otsu thresholding was used to determine the minVal and maxVal mentioned above. This can be achieved by simply adding cv2.THRESH_OTSU as an extra flag in cv2. threshold.
After preprocessing by Canny, the resulting images were only represented by edges without any color information. Here, Canny edge extraction was applied to process all the images, including all the test images (for validation) and template images (for establishment of the database). Furthermore, the extracted edges of the input test image were compared with all the templates in the database by counting the number of matched pixels. If the rate of matched pixels is high, the input image is more likely to match certain templates and be classified as a real sample. In order to evaluate the result quantitatively and make it understandable by the users, we transfer the matching score to a matching probability through normalizing the matching score in the range of 0–1, by applying the softmax function on all the template matching scores to obtain a matching probability for each template. The equation of softmax normalization is:
where
Zi is the matching score;
Zc is the number of the matched pixels of the extracted edges; and
C is the number of templates.
If the highest probability is above a certain value (determined by validation results), the test image is clarified to be a real sample; otherwise, when none of the templates is matched, the test image is recognized to be a fake sample. The program can automatically wire the feedback to the users in practical applications upon receiving data from the users, which is convenient and user-friendly. Also, on the manufacture end, a server of this kind does not add much cost per label.
2.4. Encoding capacity of the patterned PCs with PUFs
Judging from Fig. 1d and Fig. S4,† quadrangles were with random sizes of ∼16.51 μm–40.54 μm. Their average area was ∼272.58 μm2–1643.49 μm2. The image area, 309.71 μm in length and 232.29 μm in width, was calculated to be 71
942.54 μm2. Because the quadrangles fully covered the image area, the average number of quadrangles in the observed area was ∼44–264. Since these quadrangles were different in sizes and shapes from one another, their permutations 44! to 264! in the image area were as high as (2.658 × 1054–1.819 × 10526). Thus, the encoding capacity of the patterned PC in this area was calculated to be 3.695 × 1049 μm−2–2.527 × 10521 μm−2.
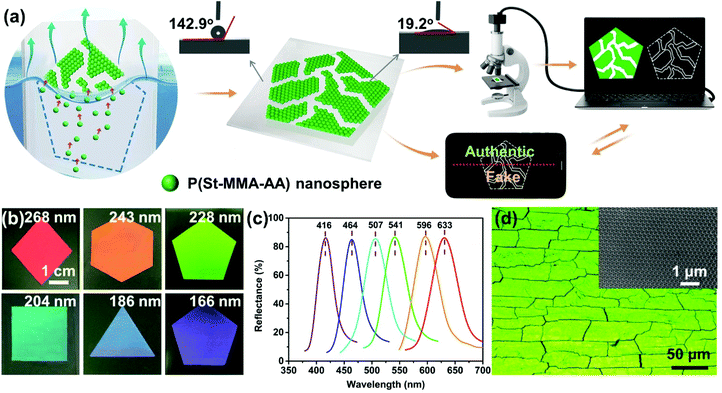 |
| Fig. 1 Fabrication of patterned colloidal crystal films with random micro-cracks and PUFs by evaporation-induced self-assembly. (a) Schematic illustration of the self-assembly process on the hydrophilic patterns of the hydrophobic substrate and the authentication process with computer vision. The random arrangements of the micro-cracks in the colloidal crystals enabled PUFs, and a novel edge sensitive template matching approach based on a computer vision algorithm was developed for accurate decoding. (b) Colloidal crystal films with diverse patterns and rich color hues assembled with P(St-MMA-AA) nanospheres of different sizes. The inset numbers indicate the diameters of the used particles. (c) The reflectance spectra of the colloidal crystal films with the wavelength covering the entire visible region. (d) Optical microscopy image of the colloidal crystals with random cracks under reflective mode. The inset shows a typical SEM image of the colloidal crystals with well-ordered FCC arrangements of P(St-MMA-AA) nanospheres. | |
3. Results and discussion
3.1. Fabrication and characterization of patterned colloidal crystal films with PUFs enabled by random micro-cracks
Patterned colloidal crystal films with irregular micro-cracks and the corresponding PUFs were fabricated by a facile evaporation-induced self-assembly approach on hydrophobic substrates (water contact angle of ∼142.6°) with designed hydrophilic regions (water contact angle of ∼19.2°) (Fig. 1a). The monodisperse P(St-MMA-AA) core–shell nanospheres selectively self-assembled in the hydrophilic pattern area due to the attractive capillary forces under the menisci,46,47 while the hydrophobic region expels water and the nanospheres to avoid particle deposition (Fig. 1a).43,46 The high monodispersity of the P(St-MMA-AA) nanospheres and dynamic hydrogen bonding of PAA on the nanoparticle surface could facilitate their self-assembly into high quality colloidal crystals.20,42 In this way, decorative iridescent structural-color films with rich color hues and various patterns (pentagon, rhombus, square, hexagon, and triangle for instance) could be conveniently obtained in the designed hydrophilic regions on common flat or curved surfaces (Fig. 1b and Fig. S1†). The red to blue structural colors of the patterned colloidal crystal films with the wavelength covering the entire visible spectra were uniform, vivid and brilliant, which could be evidenced by the intense and narrow reflection peaks centered at ∼633 nm, ∼596 nm, ∼541 nm, ∼507 nm, ∼464 nm, and ∼416 nm, respectively (Fig. 1c). The observed color was controlled by the sizes of the P(St-MMA-AA) nanospheres at ∼268 nm, ∼243 nm, ∼228 nm, ∼204 nm, ∼166 nm, and ∼186 nm (inset in Fig. 1d and Fig. S2†). These brilliant colors, with no chemical pigment/dye involvement, originated from the Bragg's diffraction from the well-ordered periodic face-centered cubic (FCC) arrangements of the monodisperse nanoparticles. The soft PAA shell of the P(St-MMA-AA) nanospheres enabled the area contact modes in the FCC structures (Fig. 1d and Fig. S2†) and the strong hydrogen bonding could enhance the mechanical stability of the colloidal crystals.42 Furthermore, transparent tape or thermoset polymers could be used to cover the colloidal crystal patterns to protect against mechanical or water-induced damage (Fig. S3†).
Normally, random microscale cracks would occur in colloidal crystal films obtained by chemical self-assembly,13,14,18,47 as was also evidenced here in the optical microscopy and SEM images (Fig. 1d and Fig. S2, 4†). These uncontrollable cracks were formed due to the tensile stress generated in the final drying stage of the chemical self-assembly, because of the mismatch between the shrinkage of the colloidal spheres and the constraint from the rigid substrate.13,23,47 Cracking in colloidal crystals is usually considered a serious unfavorable issue for PCs’ practical applications,14,20 due to the uncontrolled manner and harmful optical effects. Here, the Y-shaped micro-cracks actually divided the colloidal crystal patterns into pseudo-quadrangles with random sizes of approximately 16.51–40.54 um and irregular shapes, which enabled PUF with a coding capacity of 3.695 × 1049–2.527 × 10521 μm−2 in the image area of ∼0.7 mm2 in Fig. 1d (see the Experimental section). The first colloidal-crystal-based anti-counterfeiting materials with PUFs of extremely large encoding capacity enabled by the irregular micro-crack “fingerprints” could open a new avenue for ultimate security applications in diverse fields, including high-value goods, confidential documents, bank notes, medicine, etc.
The random micro-cracks in the patterned colloidal crystal films, acting as PUF codes, are complex with significantly similar characteristics, making their accurate decoding extremely difficult. Initial experiments on decoding of the PUFs enabled by irregular micro-cracks with artificial intelligence gave high false feedbacks, although deep learning has demonstrated success in anti-counterfeiting technology based on angle-independent structural colors.48,49 The high false rate, probably due to interference from the color variations at the microscale (Fig. 1d and Fig. S4†), made artificial intelligence impractical for real-world authentication in this case. Thus, a novel edge-sensitive template matching decoding approach based on a computer vision algorithm was developed in this work (see the Experimental section), as illustrated in Fig. 1a.35 Briefly, a multi-stage algorithm called Canny was used to extract and detect various edges in each image to avoid interference from the colors during decoding. The extracted edges of the micro-cracks in the database were used as templates and the edges of the input images taken by users were compared with all the templates by counting the number of matched pixels and a similarity threshold for classification was set. If the rate of matched pixels is higher than the threshold, the input image is more likely to match certain templates and thus is classified as an authentic sample; otherwise, if the match score is lower than the threshold, the sample is considered a fake one (Fig. 1a). The users could take images with a portable microscope mounted on cellphones, wire them to the database and receive feedbacks through messages sent by the server automatically in real-world applications. The whole validation process is facile and convenient. The computer-vision verifiable decorative colloidal crystal patterns with PUFs enabled by random micro-cracks would have enormous potential in advanced anti-counterfeiting applications.
3.2. Anti-counterfeiting modes enabled by angle-dependent structural colors and anisotropic polarization of the patterned colloidal crystal films with PUFs
The periodic arrangement of the monodisperse P(St-MMA-AA) nanospheres with FCC structures across the whole film of ∼4 μm in thickness (Fig. S2†) led to decorative iridescent colors depending on the viewing angles under directional white-light illumination (Fig. 2a and Fig. S5†), based on Bragg's diffraction,8,50,51 The angle-dependent colors enabled the hiding of the red pentagon patterns on plastic substrates with a similar color. Upon changing the observation angles, the covert pattern was revealed due to its blue-shift of the structural colors from red at a viewing angle of 0° to green at 40° (Fig. 2b). The photographs were taken by fixing the light on the top and moving the camera around the film (inset in Fig. 2b). The result was consistent with the shift of the peak wavelength of the reflectance spectra of the colloidal crystal films from 630 nm to 510 nm when measured from 0° to 60° (Fig. 2c). Furthermore, we calculated the theoretical reflectance peaks of the colloidal crystal films with red structural color assembled with P(St-MMA-AA) nanospheres with a diameter of 268 nm, according to the modified Bragg's diffraction equation,8
where D is the center-to-center distance between the nearest spheres, which is 268 nm; ni and Vi are the refractive index and volume fraction of the P(St-MMA-AA) nanospheres and air, which are 1.57 and 0.74 for FCC P(St-MMA-AA) nanosphere arrays, and 1.0 and 0.26 for the air in the void; and Φ is the angle between the incident light and the sample normal. We can see that the experimental results are highly consistent with the theoretical values. The iridescent structural colors of the decorative colloidal crystals accounted for the first-level angle-dependent anti-counterfeiting mode observable with the naked eye.
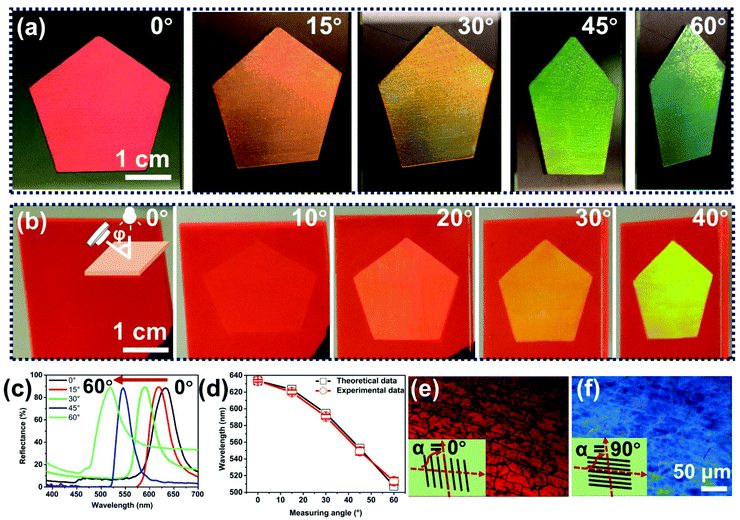 |
| Fig. 2 Optical properties of colloidal crystal films with PUFs. (a) A typical colloidal crystal film with the pentagon pattern changed its structural color from red to cyan at viewing angles from 0° to 60°. (b) The angle-dependent structural-colors of the colloidal crystal film enabled the hiding and revealing of the pentagon patterns on a red plastic substrate. The inset indicates the relative positions of the light source, sample and camera. (c) The reflectance spectra of the red pentagon pattern colloidal crystal film at testing angles from 0° to 60°. (d) The dependence of the reflectance peaks on the testing angles (black line, theoretical data and red line, experimental data). (e) and (f) Cross-polarized optical microscopy images of the colloidal crystal films at 0° and 90°. The color of the film changed to blue due to the polarization anisotropy of the crystal structure. | |
Moreover, the polarization anisotropy of the FCC colloidal crystal structure resulted in the change of the observed colors between crossed polarizers in Fig. 2e and f (Fig. S6†), which is due to the resonant and non-resonant light reflection of the defects (micro-cracks for instance) in the colloidal crystals.52,53 Interestingly, horizontal rotation of the colloidal crystal film between the crossed-polarizers led to a color change from the original red structural color to blue color, which enabled the second-level anti-counterfeiting mode decodable under polarized light. These two levels of anti-counterfeiting modes also have attractive decoration functions, which are appealing for packaging applications.
3.3. Fluorescence anti-counterfeiting mode by deposition of fluorescent nanospheres into the crack template
The irregular and random micro-cracks were further utilized as templates to guide the fluorescent silica nanosphere deposition with the help of capillary forces. Under UV light, the silica nanospheres in the physically unclonable micro-cracks could show bright fluorescence (Fig. 3a), opening the third-level fluorescence anti-counterfeiting mode. Fig. 3b shows the SEM image of a typical crack of ∼0.64 μm in width incorporated with 3 and 4 rows of fluorescent silica nanospheres with a diameter of ∼194 nm, while a 0.95 μm wide tortuous crack embedded with 5 and 6 rows of silica nanospheres of the same size (Fig. 3c). Actually, the number of the rows of the silica microspheres filled in the micro-crack templates was determined by their relative size ratio with the crack width, based on the space confinement effect.54 Under UV light excitation, the random black micro-cracks under white light showed bright yellow fluorescence (Fig. 3d and e), due to the conjugated fluorescent molecules on the surface of the incorporated silica nanospheres. The irregular pattern of the random micro-cracks after fluorescent nanosphere deposition was similar to the microscope image in Fig. 1d, indicating the robustness of the structural and optical performance of the decorative colloidal crystals. These microscopic stochastic fluorescent ‘fingerprints’ of cracks could act as fluorescent PUF codes, which is advantageous to the conventional anti-counterfeiting technique based on the easily clonable macroscopic fluorescent patterns.55,56 The colloidal crystal films with unclonable fluorescence ‘fingerprints’ of micro-cracks would have enormous potential for ultimate security applications when they could be decoded conveniently and accurately.
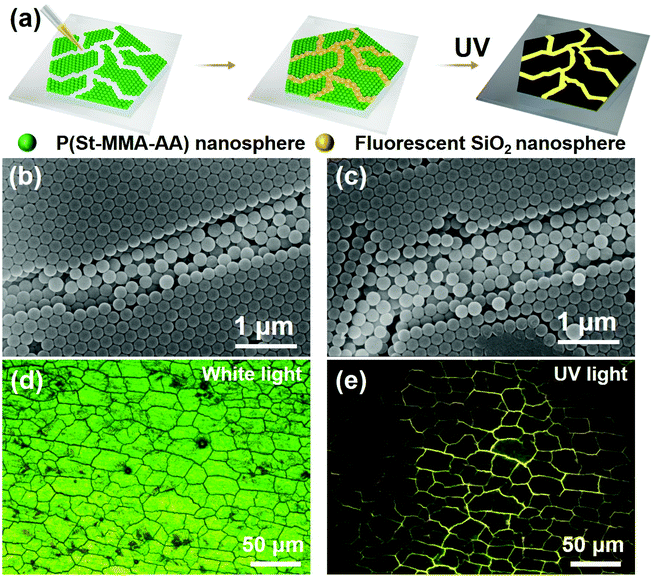 |
| Fig. 3 Fluorescence anti-counterfeiting modes with PUFs using random micro-cracks as templates to direct fluorescent nanosphere deposition. (a) Schematic illustration of deposition of the fluorescent silica nanospheres into the micro-crack templates by capillary forces. (b) and (c) The SEM images of the cracks with widths of 0.64 μm and 0.95 μm incorporated with different rows of fluorescent silica nanospheres with a diameter of ∼194 nm. (d) and (e) Optical microscopy images of the colloidal crystals with random micro-cracks under white or UV light excitation. | |
3.4. Authentication of the fluorescent random micro-cracks via a computer vision algorithm
To validate the fluorescent random micro-cracks used as PUF codes here, we developed a computer-vision based edge-sensitive template matching approach with a multi-stage algorithm called Canny to extract and detect various crack edges of the fluorescence microscopy images of the colloidal crystals (Fig. 4a1–6 and Fig. S7 and 8†).45 The extraction of the edges of the fluorescence microscopy images by the computer vision algorithm could avoid the color interference and improve validation accuracy. The whole decoding processes comprising 2 steps, micro-crack edge extraction and similarity comparison, are described in detail in the Experimental section.
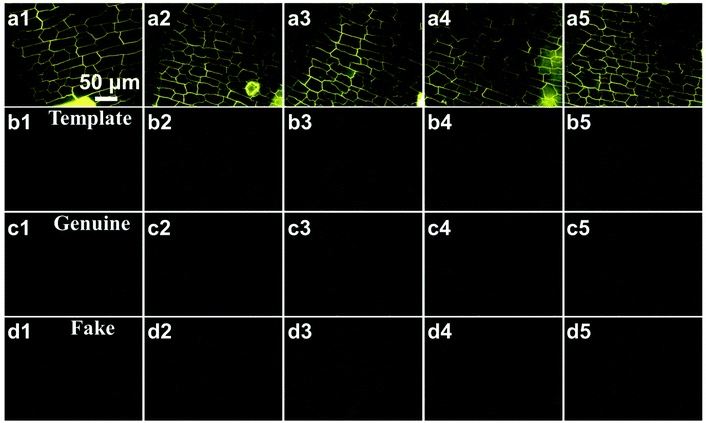 |
| Fig. 4 Database of the colloidal crystal films with PUF codes for authentication via a novel edge-sensitive template matching approach based on a computervision algorithm. (a1–5) Five typical fluorescence microscopy images with random micro-cracks, which were processed by a computer vision algorithm to extract the crack edges to form the templates (b1–5). (c1–5) Extracted edges of the genuine samples (a1) with varying exposure, brightness, contrast, and a mixture of the above-mentioned factors for validation of the genuine labels. (d1–5) Extracted edges of the fluorescence microscopy images that were not in the template database of a1–5, which stand for fake products. | |
After pre-processed by Canny edge extraction based on a computer vision algorithm, all the resulting images were only represented by edges without any color information (Fig. 4b–d and Fig. S7 and S8†), including all the test images (genuine samples taken under different photographing conditions to simulate actual environments, including brightness, contract, and rotations in Fig. 4c and Fig. S8,† as well as the fake ones not in the database in Fig. 4d for validation), and template images (Fig. 4b, for establishment of the database). Furthermore, we compared the input test image (Fig. 4c and d and Fig. S8†) with all the templates in the database by counting the number of matched pixels. Based on the results shown in Fig. 5, the matching probability thresholds of 0.7 and 0.02 were set to distinguish the authentic samples from the fake ones. If the matching probability for an image is above 0.7, the test image is recognized as a real sample (Fig. 5a); otherwise, when none of the templates is matched or the matching probability is low (below 0.02), the test image is recognized to be a fake sample (Fig. 5b). The wide gap in the distributions of the identical rates over the matched and unmatched samples in Fig. 5c guarantees the decoding accuracy of 100% of the novel edge-sensitive template matching approach, fulfilling the requirements for practical anti-counterfeiting applications of the PUFs of colloidal crystals. Under real-world applications, Users just need to take a photograph with a portable microscope (used by many children as a toy) mounted on their cellphones, and send the images to our database; the database will give feedbacks based on matching probability in seconds to the user via messages automatically. The authentication process was convenient and user-friendly. For the manufacture, a server automatically carrying out the authentication also does not add much cost, considering the vast number of products manufactured by the corporation.
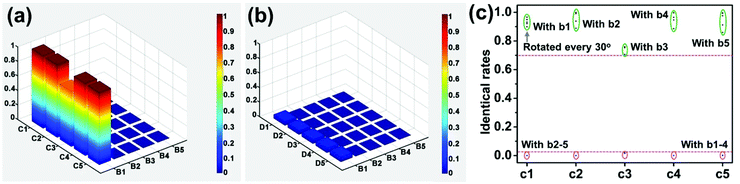 |
| Fig. 5 (a) and (b) Identical rates of labels in Fig. 4 (c1–5, for genuine ones) and (d1–5, for fake ones) authenticated by a computer vision algorithm. The color scales from blue to red stand for the matching score (ranging from 0 to 1) of the input images of the sample. (c) The distributions of the identical rates between the edges of Fig. 4c taken under varying rotations and all the templates in Fig. 4b1–5. The wide gap in the distributions of the identical rates for the matched and unmatched samples could guarantee high validation accuracy. | |
4. Conclusions
Decorative anti-counterfeiting colloidal crystal films with PUFs were fabricated by a facile self-assembly of P(St-MMA-AA) nanospheres on hydrophobic substrates with designed hydrophilic patterns. The unique structural and optical properties of the colloidal crystals accounted for multi-anti-counterfeiting modes, including angle-dependent structural colors and polarization anisotropy. Moreover, the unfavorable random and irregular micro-cracks naturally occurring in the colloidal crystal films were used as PUF codes for ultimate security applications. As a proof of concept, the irregular micro-scale cracks were used as templates to guide the fluorescent microsphere deposition, which enabled advanced fluorescent anti-counterfeiting mode with PUFs. To validate the random and irregular micro-cracks and realize accurate decoding of the fluorescent PUFs, a novel edge-sensitive template matching authentication approach based on a computer vision algorithm was developed, which demonstrated decoding accuracy of ∼100%. The physically unclonable colloidal crystals with facile fabrication and convenient and accurate validation by computer vision are superior to conventional photonic crystal materials that rely on clonable angle-dependent or tunable structural colors for anti-counterfeiting,7,10,38–41 and the conventional PUF labels in an aspect of decorative functions,26–37 which opens a new avenue for advanced security materials with multi-functionality.
Author contributions
Li HY, Wang JH, Wu N, and Jia P fabricated and characterized the samples; Mao YX, Liu ZW and Wang JQ carried out validation by computer vision; and Zhou JM wrote the paper with support from Yu HT and Song YL. All authors contributed to the general discussion. Yuhuan Li and Yexin Mao contributed equally to this work.
Conflicts of interest
There are no conflicts to declare.
Acknowledgements
This work was supported by the National Natural Science Foundation of China, Grant No. 21975063, the top 100 innovative talents program in higher institutions of Hebei Province, Grant No. SLRC2019034, and the Hebei 333 Talent Project, Grant No. A202001007.
References
- S. A. Asher, V. L. Alexeev, A. V. Goponenko, A. C. Sharma, I. K. Lednev, C. S. Wilcox and D. N. Finegold, J. Am. Chem. Soc., 2003, 125, 3322–3329 CrossRef CAS PubMed.
- Y. J. Zhao, X. W. Zhao, J. Hu, M. Xu, W. J. Zhao, L. G. Sun, C. Zhu, H. Xu and Z. Z. Gu, Adv. Mater., 2009, 21, 569–572 CrossRef CAS PubMed.
- Q. Q. Fu, B. T. Zhu and J. P. Ge, Nanoscale, 2017, 9, 2457–2463 RSC.
- A. C. Arsenault, D. P. Puzzo, I. Manners and G. A. Ozin, Nat. Photonics, 2007, 1, 468–472 CrossRef CAS.
- Z. W. Li, X. J. Wang, L. L. Han, C. H. Zhu, H. L. Xin and Y. D. Yin, Adv. Mater., 2021, 34, 2107398 CrossRef PubMed.
- Y. A. Vlasov, M. O'boyle, H. F. Hamann and S. J. McNab, Nature, 2005, 438, 65–69 CrossRef CAS PubMed.
- Y. Heo, H. Kang, J. S. Lee, Y. K. Oh and S. H. Kim, Small, 2016, 12, 3819–3826 CrossRef CAS PubMed.
- J. P. Ge and Y. D. Yin, Angew. Chem., Int. Ed., 2011, 50, 1492–1522 CrossRef CAS PubMed.
- Z. P. Meng, S. L. Wu, B. T. Tang, W. Ma and S. F. Zhang, Nanoscale, 2018, 10, 14755–14762 RSC.
- X. T. Lai, J. S. Peng, Q. F. Cheng, A. P. Tomsia, G. L. Zhao, L. Liu, G. S. Zou, Y. L. Song, L. Jiang and M. Z. Li, Angew. Chem., Int. Ed., 2021, 60, 14307–14312 CrossRef CAS PubMed.
- G. Li, W. Luo, Z. Y. Che, Y. Y. Pu, P. Deng, L. Shi, H. R. Ma and J. G. Guan, Small, 2022, 2200662, DOI:10.1002/smll.202200662.
- R. Morita, T. Inoue, M. De Zoysa, K. Ishizaki and S. Noda, Nat. Photonics, 2021, 15, 311–318 CrossRef CAS.
- K. B. Singh and M. S. Tirumkudulu, Phys. Rev. Lett., 2007, 98, 218302 CrossRef PubMed.
- K. R. Phillips, C. T. Zhang, T. Yang, T. Kay, C. Gao, S. Brandt, L. Liu, H. Yang, Y. Li, J. Aizenberg and L. Li, Adv. Funct. Mater., 2019, 30, 1908242 CrossRef.
- Z. Y. Zheng, K. Y. Gao, Y. H. Luo, D. M. Li, Q. B. Meng, Y. R. Wang and D. Z. Zhang, J. Am. Chem. Soc., 2008, 130, 9785–9789 CrossRef CAS PubMed.
- Z. M. Jiang and J. H. Pikul, Nat. Mater., 2021, 20, 1512–1518 CrossRef CAS PubMed.
- X. J. Wu, R. Hong, J. K. Meng, R. Cheng, Z. J. Zhu, G. Wu, Q. Li, C. F. Wang and S. Chen, Angew. Chem., Int. Ed., 2019, 58, 13556–13564 CrossRef CAS PubMed.
- B. Hatton, L. Mishchenko, S. Davis, K. H. Sandhage and J. Aizenberg, Proc. Natl. Acad. Sci. U. S. A., 2010, 107, 10354–10359 CrossRef CAS PubMed.
- B. Griesebock, M. Egen and R. Zentel, Chem. Mater., 2002, 14, 4023–4025 CrossRef CAS.
- Y. Huang, J. M. Zhou, B. Su, L. Shi, J. X. Wang, S. R. Chen, L. B. Wang, J. Zi, Y. L. Song and L. Jiang, J. Am. Chem. Soc., 2012, 134, 17053–17058 CrossRef CAS PubMed.
- C. Jin, M. A. McLachlan, D. W. McComb, R. M. De la Rue and N. P. Johnson, Nano Lett., 2005, 5, 2646–2650 CrossRef CAS PubMed.
- J. Zhang, Z. J. Zhu, Z. Y. Yu, L. T. Ling, C.-F. Wang and S. Chen, Mater. Horiz., 2019, 6, 90–96 RSC.
- J. M. Zhou, J. X. Wang, Y. Huang, G. M. Liu, L. B. Wang, S. R. Chen, X. H. Li, D. J. Wang, Y. L. Song and L. Jiang, NPG Asia Mater., 2012, 4, e21 CrossRef.
- H. L. Zhu, Y. J. Zhang, J. Q. Zhu, Y. H. Li, S. H. Jiang, N. Wu, Y. Wei, J. M. Zhou and Y. L. Song, J. Mater. Chem. A, 2020, 8, 22929–22937 RSC.
- B. Li, B. B. Jiang, W. Han, M. He, X. Li, W. Wang, S. W. Hong, M. Byun, S. L. Lin and Z. Q. Lin, Angew. Chem., Int. Ed., 2017, 56, 4554–4559 CrossRef CAS PubMed.
- J. D. Buchanan, R. P. Cowburn, A. V. Jausovec, D. Petit, P. Seem, G. Xiong, D. Atkinson, K. Fenton, D. A. Allwood and M. T. Bryan, Nature, 2005, 436, 475 CrossRef CAS PubMed.
- R. Arppe and T. J. Sørensen, Nat. Rev. Chem., 2017, 1, 0031 CrossRef CAS.
- A. Dodda, S. S. Radhakrishnan, T. F. Schranghamer, D. Buzzell, P. Sengupta and S. Das, Nat. Electron., 2021, 4, 364–374 CrossRef CAS.
- B. Shao, T. H. Choy, F. Zhou, J. Chen, C. Wang, Y. J. Park, J.-H. Ahn and Y. Chai, Nano Res., 2021, 14, 1784–1788 CrossRef CAS.
- A. T. Erozan, G. C. Marques, M. S. Golanbari, R. Bishnoi, S. Dehm, J. Aghassi-Hagmann and M. B. Tahoori, IEEE Trans. Very Large Scale Integr. Syst., 2018, 26, 2935–2946 Search PubMed.
- A. Scholz, L. Zimmermann, U. Gengenbach, L. Koker, Z. Chen, H. Hahn, A. Sikora, M. B. Tahoori and J. Aghassi-Hagmann, Nat. Commun., 2020, 11, 5543 CrossRef CAS PubMed.
- J. Rajendran, R. Karri, J. B. Wendt, M. Potkonjak, N. McDonald, G. S. Rose and B. Wysocki, Proc. IEEE, 2015, 103, 829–849 Search PubMed.
- J. Kim, J. M. Yun, J. Jung, H. Song, J.-B. Kim and H. Ihee, Nanotechnology, 2014, 25, 155303 CrossRef PubMed.
- V. Caligiuri, A. Patra, M. P. De Santo, A. Forestiero, G. Papuzzo, D. M. Aceti, G. E. Lio, R. Barberi and A. De Luca, ACS Appl. Mater. Interfaces, 2021, 13, 49172–49183 CrossRef CAS PubMed.
- K. Nakayama and J. Ohtsubo, Opt. Eng., 2012, 51, 040506 CrossRef.
- V. Caligiuri, A. Patra, M. P. De Santo, A. Forestiero, G. Papuzzo, D. M. Aceti, G. E. Lio, R. Barberi and A. De Luca, ACS Appl. Mater. Interfaces, 2021, 13, 49172–49183 CrossRef CAS PubMed.
- B. R. Anderson, R. Gunawidjaja and H. Eilers, Appl. Opt., 2017, 56, 2863 CrossRef CAS PubMed.
- S. L. Wu, B. Q. Liu, X. Su and S. F. Zhang, J. Phys. Chem. Lett., 2017, 8, 2835–2841 CrossRef CAS PubMed.
- R. Y. Xuan and J. P. Ge, J. Mater. Chem., 2012, 22, 367–372 RSC.
- K. Zhong, J. Li, L. Liu, S. Van Cleuvenbergen, K. Song and K. Clays, Adv. Mater., 2018, 30, e1707246 CrossRef PubMed.
- W. Hong, Z. K. Yuan and X. D. Chen, Small, 2020, 16, 1907626 CrossRef CAS PubMed.
- J. X. Wang, Y. Q. Wen, H. L. Ge, Z. W. Sun, Y. M. Zheng, Y. L. Song and L. Jiang, Macromol. Chem. Phys., 2006, 207, 596–604 CrossRef CAS.
- Z. Z. Gu, A. Fujishima and O. Sato, Angew. Chem., 2002, 114, 2068–2070 Search PubMed.
- A. van Blaaderen and A. Vrij, Langmuir, 1992, 8, 2921–2931 CrossRef CAS.
- J. Canny, IEEE Trans. Pattern Anal. Mach. Intell., 1986, 8, 679–698 CAS.
- C. A. Fustin, G. Glasser, H. W. Spiess and U. Jonas, Adv. Mater., 2003, 15, 1025–1028 CrossRef CAS.
- L. Li, C. Goodrich, H. Yang, K. R. Phillips, Z. Jia, H. Chen, L. Wang, J. Zhong, A. Liu and J. Lu, Proc. Natl. Acad. Sci. U. S. A., 2021, 118, e2107588118 CrossRef CAS PubMed.
- X. Y. He, Y. N. Gu, B. R. Yu, Z. W. Liu, K. Zhu, N. Wu, X. Zhao, Y. Wei, J. M. Zhou and Y. L. Song, J. Mater. Chem. C, 2019, 7, 14069–14074 RSC.
- Y. H. Li, Z. W. Liu, K. Zhu, L. Q. Ai, P. Jia, N. Wu, H. T. Yu, J. Q. Wang, X. Yao, J. M. Zhou and Y. L. Song, Adv. Mater. Interfaces, 2021, 8, 2101281 CrossRef CAS.
- J. B. Kim, C. Chae, S. H. Han, S. Y. Lee and S.-H. Kim, Sci. Adv., 2021, 7, eabj8780 CrossRef CAS PubMed.
- M. Qin, Y. Huang, Y. N. Li, M. Su, B. D. Chen, H. Sun, P. Y. Yong, C. Q. Ye, F. Y. Li and Y. L. Song, Angew. Chem., Int. Ed., 2016, 55, 6911–6914 CrossRef CAS PubMed.
- S. G. Romanov, Phys. Rev. B, 2017, 95, 155441 CrossRef.
- Y. Monovoukas, G. G. Fuller and A. P. Gast, J. Chem. Phys., 1990, 93, 8294 CrossRef CAS.
- Y. D. Yin, Y. Lu, B. Gates and Y. N. Xia, J. Am. Chem. Soc., 2001, 123, 8718–8729 CrossRef CAS PubMed.
- B. Yoon, D.-Y. Ham, O. Yarimaga, H. An, C. W. Lee and J.-M. Kim, Adv. Mater., 2011, 23, 5492–5497 CrossRef CAS PubMed.
- Z. Gao, Y. F. Han and F. Wang, Nat. Commun., 2018, 9, 3977 CrossRef PubMed.
|
This journal is © The Royal Society of Chemistry 2022 |
Click here to see how this site uses Cookies. View our privacy policy here.