A prospective ecological risk assessment of high-efficiency III–V/silicon tandem solar cells†
Received
7th November 2023
, Accepted 11th January 2024
First published on 1st February 2024
Abstract
III–V/Silicon tandem solar cells offer one of the most promising avenues for high-efficiency, high-stability photovoltaics. However, a key concern is the potential environmental release of group III–V elements, especially arsenic. To inform long-term policies on the energy transition and energy security, we develop and implement a framework that fully integrates future PV demand scenarios with dynamic stock, emission, and fate models in a probabilistic ecological risk assessment. We examine three geographical scales: local (including a floating utility-scale PV and waste treatment), regional (city-wide), and continental (Europe). Our probabilistic assessment considers a wide range of possible values for over one hundred uncertain technical, environmental, and regulatory parameters. We find that III–V/silicon PV integration in energy grids at all scales presents low-to-negligible risks to soil and freshwater organisms. Risks are further abated if recycling of III–V materials is considered at the panels' end-of-life.
Environmental significance
Solar PV continues to spearhead the energy transition with its exponential growth in installed capacity. Of the 50 components tracked by the International Energy Agency, solar PV is one of only three that appears on track with the Net Zero by 2050 Scenario trajectory. This continued trend is likely to open markets for high-efficiency photovoltaics that can compensate for restricted space with higher conversion efficiencies. For several years now, multijunction III–V cells have been a promising candidate in this front. A previous Life Cycle Assessment (LCA) of III–V cells on silicon substrates (III–V/Si) revealed important potential for environmental benefits vs. commercial silicon PERC cells when compared per kW h of electricity generated. LCA cannot, however, quantify actual chemical risks, an aspect that should be observed if the quantities of panels installed (and disposed at end-of-life) is projected to grow exponentially. We introduce here an integrated model that allows a prospective estimation of potential risks to soil and freshwater organisms by coupling future PV demand and emission scenarios with dynamic environmental fate models. Our model allows probabilistic predictions for a robust and conservative analysis. Global sensitivity analysis is used to identify and address elements of large uncertainty in the model and to suggest roadmaps for further research and improvement of III–V/Si cells. We conclude that the potential risks of large-scale III–V/Si PV deployment are low to negligible. The modelling framework we developed is made available open-source and can be readily adapted to similar technologies in support of safer and more sustainable innovation.
|
Introduction
Recent decades have seen a dramatic increase in the deployment of photovoltaic (PV) electricity installations across energy markets worldwide.1 Next to lower manufacturing costs, a key driver has been the environmental benefits when compared to fossil or nuclear alternatives.2,3 The crystalline silicon (c-Si) panels which dominate today's PV market are especially appealing due to silicon's abundance and low toxicity.4 The lower toxicity especially sets an important benchmark against which emerging PV technologies such as III–V/silicon tandem cells (III–V/Si) would be judged. III–V/Si tandem cells stack thin light-absorbing layers of Group III and V elements (gallium, indium, arsenide, phosphide) on top of a c-Si wafer to achieve record-breaking conversion efficiencies for non-concentrating systems that can exceed 35%.5 Manufacturing III–V/Si with current technology is expensive and efforts are underway to make them more economically attractive.6–9 However, concerns regarding potential toxic releases of III–V metals and metalloids to the environment could hinder investment and stall commercialization of the technology.
Investigating the potential environmental impacts of innovative PV designs such as III–V/Si during early research and development stages is therefore important to guide research towards more environmentally compelling solutions.10–12 The impacts of emerging PV technologies have often been assessed in a prospective way using life cycle assessment (LCA) with forward-looking projections.13 While previous LCA work has shown that III–V/Si could perform similar or better than silicon PV across most environmental impact categories,14 LCA only allows a comparison of impact indicators in a relative sense and in a hypothetical situation where environmental emissions are aggregated across space and time.15 To determine whether the emissions pose actual risks, they must be evaluated in a specified temporal and spatial context using tools like ecological risk assessment.16 Ecological risk assessments are challenging for both modelling and data requirements and have not been conducted to date for III–V/Si PV systems. In this paper we address this important knowledge gap.
Recent studies of toxicity aspects of emerging PV technologies focus narrowly on subsets of specific PV components, life-cycle stages, release mechanisms and/or toxicity endpoints.17–19 To avoid these shortcomings, we adopt a comprehensive approach by screening for relevant emissions in all life-cycle stages of III–V/Si panels and estimating the risks posed by these emissions in plausible and well-defined PV demand scenarios at three geographical scales: local, regional and continental. We also take into account foreseeable but relevant technological developments in the PV cell design (currently at Technology Readiness Level 5), namely cell performance improvements (conversion efficiency) and manufacturing optimization (MOVPE reactor deposition rates and required amounts of III–V materials). Such developments were investigated by a recently completed European project that focused on bringing the III–V/Si design closer to commercial readiness.14,20
This holistic and forward-looking approach introduces numerous and large uncertainties and variabilities21 so we use a probabilistic risk assessment to explicitly consider them in an integrated PV demand-emission-fate model. We quantify this uncertainty in the outcomes of the assessment in the risk indicators22 and conduct a global sensitivity analysis23 to reveal which factors contribute most to uncertainty in the calculated risk indicators. While the III–V/Si technology is still in development, the identification of influential factors is equally or more important than the magnitude of the risk indicators, as it can help prioritize further research and development of the technology as well as simplify the assessment by disregarding trivial uncertainties and variabilities. The stochastic models developed in this work are unprecedented for PV technology assessment and account for temporal variations, multiple nested geographical scales, and integration of demand scenarios with environmental fate and effect modelling. The framework can easily be adapted and extended to other PV technologies for robust ecological risk assessment and decision-making during early stages of PV innovation.
Results and discussion
III–V/Si panel demand and stock flows
We developed future demand and III–V/Si market-penetration scenarios and used a dynamic stock model to calculate the projected stocks of III–V/Si panels installed and reaching their end-of-life in three geographical scales:
• EUR, a continental scale where we based future PV demand on the Shell Sky Scenario24 for Europe, which is the most ambitious with regards to electrification and future participation of PV from the Shell family of scenarios. We combined the Sky projections for total PV demand with the IEA's “High GaAs” scenario, in which III–V cells comprise 5% of the distributed and 15% of the utility-scale PV demand.25
• AMS, a regional scale representing the city of Amsterdam and based on the municipality's stated ambitions in their Regional Energy Strategy (RES v1.0).26 Here we also applied III–V/Si market shares from the IEA “High GaAs” scenario.
• LOC, a local scale reflecting a utility PV plant consisting of 50 MW of floating III–V/Si panels installed on a lake area of 0.9 km2 in addition to 50
000 distributed panels (14 MW) installed on rooftops in the surrounding area and draining towards the lake. End-of-life (EOL) PV treatment is also assumed to take place within this area. As such the local scale is meant to represent an unlikely worst-case scenario for the local water compartment.‡.
Assuming demand follows an S-shaped sigmoid (logistic growth) curve27 (see Methods) carrying capacity in Europe is reached after the year 2110 while for Amsterdam it is reached at around the year 2080 (Fig. 1). In the local scale we assumed an initial installation in the year 2031 which is kept stable until the end of the modelling period. The steep ramp-up followed by stabilization in the demand growth curves (left) produces a ripple in the amount of PV materials that are available for recycling or final disposal at end-of-life (right). These oscillations are smoothened by uncertainty in the lifetime of each cohort, which is assumed to be normally distributed around a mean of 30 years.
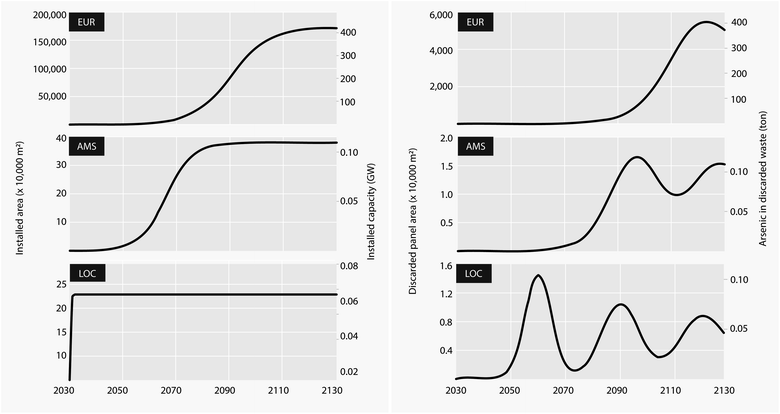 |
| Fig. 1 Projected III–V/Si PV demand (left) and discarded materials (right) for the three scales. | |
III–V metals and metalloid emissions
We calculated the expected annual emissions from PV stocks during operation (USE) and end-of-life (EOL) in a “recycling” and a “no recycling” scenario for each scale, where recycling specifically refers to recovery of the III–V materials from the cells for reuse (Fig. 2). The emissions shown in Fig. 2 reflect base-case values for each parameter in the emissions model (see ESI† for parameter distributions). Emissions during the use phase, which could only come from a small fraction of potentially cracked panels (see Experimental procedure), are several orders of magnitude lower than the emissions from the EOL phase in all cases. At the largest scale (EUR), the quantity of arsenic emitted during the use phase starts stabilizing towards the end of the modelling period at around 30 kg per year. In the regional (AMS) and local (LOC) scales with larger concentration of PV panels per unit area, total emissions amount to grams. This indicates that in all scenarios, the more relevant emissions are expected to occur at EOL.
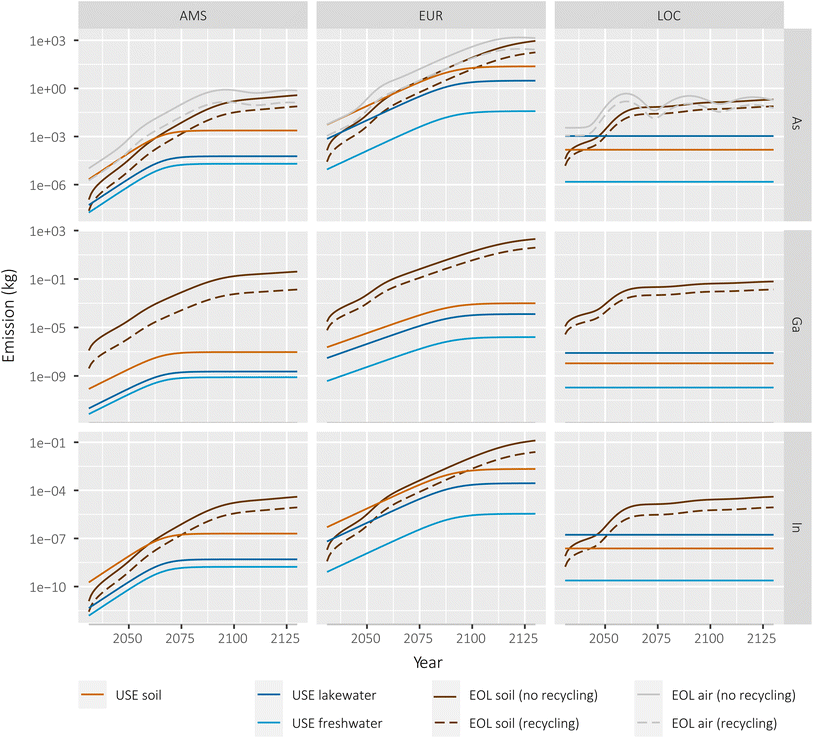 |
| Fig. 2 Life cycle emissions of III–V materials from III–V/Si PV installations in three different scenarios. (EOL: end-of-life phase; USE: use phase). | |
In the EOL phase, total life-cycle emissions approach 1 ton per year in the EUR continental scale for the soil and air compartments. For reference, a study of EU-wide inventories of arsenic and arsenic-related compound emissions estimated these at ca. 195 tonnes (air), 100 tonnes (water) and 135 tonnes (soil, mostly from manure application) in the year 2014.28 The quantities emitted to the air compartment are larger than the quantities emitted to soil at the beginning of the modelling period. This can be explained by the immediacy of the emissions during incineration: emissions that are not captured by the electrostatic precipitator during/after incineration will be immediately released into the air compartment. On the other hand, landfill emissions are subject to an important retardation factor represented by the large waste/leachate partitioning coefficients (Kw) (see Methods). Towards the end of the modelling period, the emissions from landfill to the soil compartment are of similar magnitude than those to air in all scales. No emissions to air are foreseen for gallium and indium due to their negligible volatilities.
Environmental fate of III–V metals and metalloids
The predicted environmental concentration (PEC) in soil and freshwater compartments are shown in Fig. 3. At the end of the 100 year modelling period, the 75th percentile PEC of arsenic in freshwater (including lakewater) in the local scale remains almost two orders of magnitude below the drinking water limits established by the World Health Organisation (without considering background emissions). At regional and continental scales, the PEC is even lower. In soil, the 75th percentile PEC of arsenic is several orders of magnitude lower than average concentrations reported in natural soils (1–40 mg kg−1).61 The geometric means are closer to the lower boundaries, suggesting skewed distributions with a long tail extending to the higher PEC values. The expected environmental concentrations of gallium and indium are in the nanogram range and lower, resulting in a very low exposure and suggesting a negligible potential of these substances to pose an ecological risk.
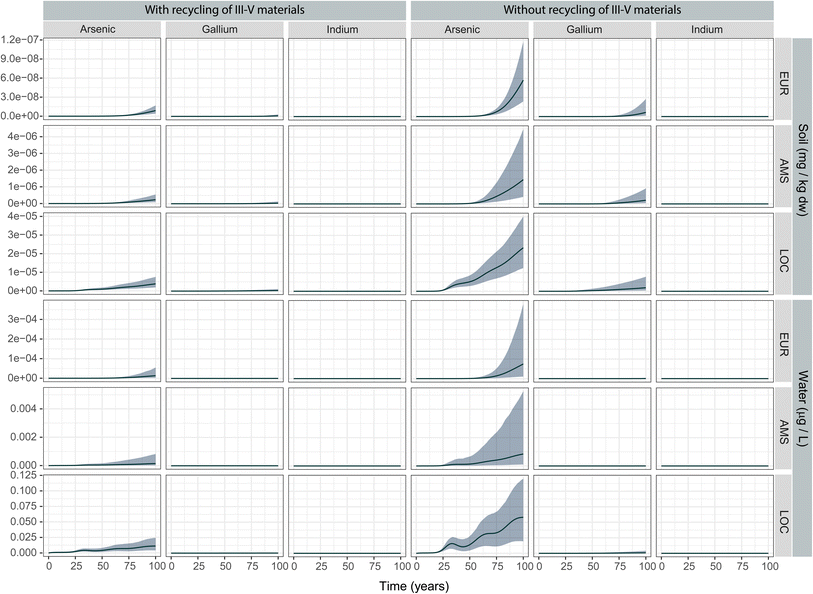 |
| Fig. 3 Predicted environmental concentrations of arsenic in soil and freshwater (including lake) compartments in all scales, with and without recovery of III–V materials during recycling. The shaded area encloses the 25th and 75th percentiles and the solid line shows the geometric mean. | |
Ecological risks to freshwater and soil organisms
We calculated risk quotients (RQ) as a ratio of predicted exposure concentrations (PEC) relative to predicted no-effect concentrations (PNEC). As per European REACH regulations and guidelines, risk quotients greater than one require action and new chemicals can only be marketed when the RQs fall below 1.29 The RQ's observed for III–V/Si deployment in the 100th year of simulation for all scales and scenarios are very low to negligible (Fig. 4). As the volumes of the environmental compartments decrease in size (from continental to regional to local scale), the PECs and risk quotients (RQs) increase. The local freshwater compartment presents the highest RQ for arsenic at ca. 0.03 for the higher end of the interquartile range. The risk quotients for all other scales, compartments and metals are below 0.001. In all cases, recovery of the III–V content during recycling of the cells would reduce risks by one order of magnitude, as a large fraction of EOL emissions would be avoided by reintroducing these materials in the economy.
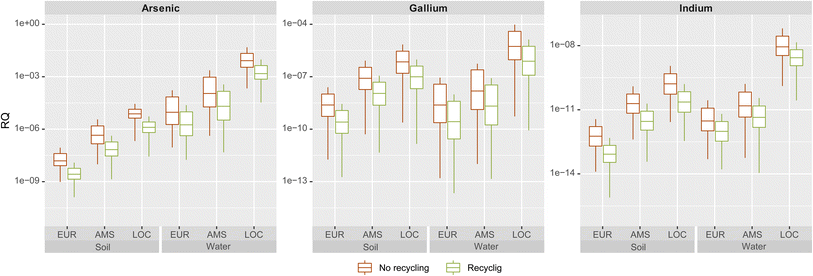 |
| Fig. 4 Risk quotients for arsenic, gallium and indium in soil and freshwater compartments in all scales, with and without recycling. | |
For the extreme local scenario conditions in which RQ approaches 0.03 for arsenic, some of the underlying assumptions merit further inspection with the aim of identifying potential risk-attenuating mechanisms. A key starting assumption was that all emitted arsenic dissolves to its most toxic ion, arsenite (As(III)), which is assumed to persist as such. However, arsenic undergoes several transformation processes which result in arsenate ions (As(V)) or even less toxic methylated organic forms.30,31 The PNEC for As(III) is approximately 5 times lower than for As(V) in plant species.32 A study of landfill leachate in Nordic countries33 found that arsenic in leachate is typically 80% arsenate, 10% arsenite and the rest is methylated. Even lower percentages of As(III) (<5%) and higher amounts of methylated forms were reported by Pinel-Raffaitin and colleagues31 in landfill leachates sampled in France.
In situ mechanisms to address As(III) mobilization in leakage from cracked panels during operation may be implemented as an additional precaution, especially in floating PV plants. Shumlas et al.,34 for example, reported accelerated oxidation of As(III) to As(V) when exposed to sunlight on layered manganese oxide. While such applications were developed for wastewater treatment in the case of arsenic, in situ mitigation concepts have already been proposed for perovskite PV cells where accidental lead leakage is immediately sequestered by lead-absorbing coatings.35
Long term implications
Modelling technology demand and behaviour of energy systems beyond a 100 year time horizon would be highly speculative and subject to very large uncertainties. However, predicted environmental concentrations are still rising at the 100th year (see Fig. 3) so it is worthwhile exploring hypothetically what could happen over longer timeframes. To investigate this longer-term outlook, we calculated long-term steady-state conditions for arsenic in the recycling scenario (more realistic given policy directions). In steady-state conditions, the demand curves stabilize at carrying capacity and the emissions also stabilized, even though this could happen hundreds or even thousands of years in the future. In the steady-state model, we took a conservative approach by fixing the model's underlying parameters in a way to match the upper quartile for risk, and the fixing the emissions at the upper quartile observed in the year 100 of the dynamic and probabilistic model. Under such conditions, long-term steady state emissions (and therefore III–V/Si PV deployment) would result in a PEC for arsenic in the local freshwater compartment of 5 × 10−7 g L−1 and a RQ of 0.09 (given the PNEC is 5.6 × 10−6 g L−1).
Sensitivity ranking of variable and uncertain parameters
We conducted a global sensitivity analysis to determine sensitivity rankings for over one-hundred uncertain and variable model inputs in the integrated model and for all scales and compartments (Fig. 5). The most sensitive parameters are the waste/leachate partitioning coefficient in the landfill, the landfill cell depth, the fraction of vapourised arsenic captured in the incinerator's ESP, and the fraction of PV collected for recycling. For the landfill partitioning coefficient, the range of possible values spans several orders of magnitude.36 It is likely that a large part of this dispersion is irreducible due to very different landfill chemistries and environmental conditions. Further studying the specific behaviour of arsenic in waste when exposed to leachate could help reduce the uncertainty to a large extent. This has already been strongly advocated by Söderberg et al.37 who reviewed 245 articles on soil/solution partitioning of metals in different media and found that no studies prior to a 2005 report by the U.S. Environmental Protection Agency36 investigated this parameter in waste disposal systems.
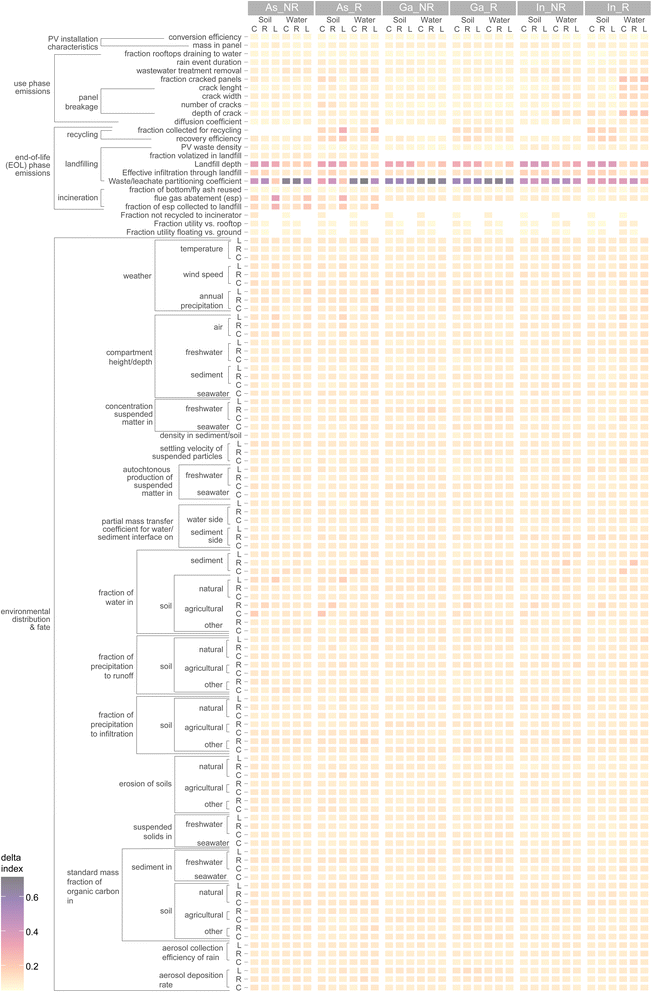 |
| Fig. 5 Sensitivity ranking of model parameters. C: continental, R: regional, L: local scale, R: recycling, NR: no recycling. | |
It is also noteworthy that despite the complexity and spatial dependency of the fate model, most of the fate-related parameters ranked low in terms of their contribution to uncertainty in the risk quotient. The uncertainty/variability hotspots are thus found in the emissions model for the EOL phase. An important takeaway from this result is that more efforts must be placed on agreed or standardized approaches for proposing future emission scenarios. This is especially important for EOL emissions which are expected far in the future and for which technological concepts (e.g. recycling, disposal methods) are usually not available to researchers. Detailed investigation of EOL behaviour of emerging technologies such as III–V/Si is thus a pivotal complement to R&D projects which could be stimulated as a mandatory component in funding programs.
Effective strategies for further risk mitigation
The most influential factors identified in the global sensitivity analysis can offer the most effective opportunities to improve the design, not only of the photovoltaic cell, but of the configuration of large-scale deployments and the ancillary/complementary supporting services such as waste treatment. We provide analyses of each of these influential factors in turn.
Waste/leachate partitioning coefficient.
Despite its large variability, this highly influential factor can be partially addressed by controlling landfill chemistry, especially the pH of the leachate. It is likely that a construction and demolition (CDW) waste landfill with low organic waste content will produce leachate in higher pH ranges than a municipal solid waste (MSW) landfill where organic matter is being degraded and more acidic conditions emerge. Reaction of the ethyl vinyl acetate (EVA) encapsulation in PV panels with infiltrated water in the landfill may also produce acidic conditions, even in CDW landfills, by formation of acetic acid on the surface of discarded PV waste. Thus, delamination prior to disposal and/or replacement of the EVA encapsulation for alternative materials38 in the panel's design may further reduce risks. This measure could also reduce leakage during operation of cracked panels, however the contribution of this release mechanism to the overall risk is already negligible. A more effective alternative would be to use underground landfills rather than above-ground landfills which would make the freshwater/soil impact pathway negligible.
Landfill depth.
Stacking discarded PV waste in landfills vertically rather than horizontally can considerably increase the retardation (thus time dilution) factor. Fig. 6 shows the shift in the distribution curve of arsenic emissions to soil after the landfill depth is fixed at its higher range (10 m). The distribution is shifted significantly to the left and its tail size reduced.
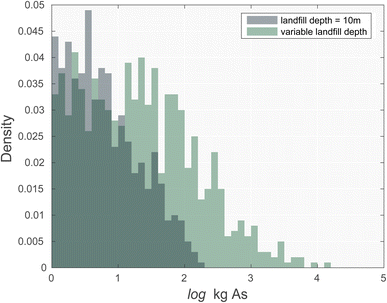 |
| Fig. 6 Change in probability distribution of arsenic emissions to soil as a result of fixing landfill depth at 10 m. | |
Incinerator abatement efficiency (electrostatic precipitator).
The fraction of vapourised arsenic that gets captured in the incinerator's electrostatic precipitator (thus prevented from direct release to the air compartment), has an important influence. Even though the abatement efficiency range we modelled (98–99.9%) leaves little room for improvement, the results suggest that efforts to implement best available practice and marginal further improvements in abatement efficiency can result in noticeable risk reductions.
PV collection for recycling rate.
By reintroducing III–V materials in PV waste into new economic products, they are effectively prevented from being released into the environment. The analysis not only showed an order of magnitude difference between the recycling and no-recycling scenario, but within the recycling scenario any efforts to increase collection above 85% will also result in important risk reductions.
The global sensitivity analysis also reveals where mitigation may not be as effective in relation to the effort/cost required to implement them. For example, reducing the arsenic content of cells in design and manufacturing will not have a noticeable effect on the risk profile. The same applies to measures to further reduce the cracking of panels – the use phase emissions are already too low to offer noticeable risk reductions.
Limitations and directions for future research
The integrated model we developed is complex in that it incorporates numerous interconnected cause-effect mechanisms to ensure as many relevant factors as possible are given consideration. Producing the data for such a model can be very time consuming if the data is available at all and some important assumptions were made. To partially ameliorate this we adopted a conservative (worst-case) approach in the scenarios and estimates we use. First, while the underlying landfill model is a good approximation for a monofill, the waste/leachate partitioning values (Kw) from EPA we used were taken from municipal solid waste (MSW) landfills, which will have phases where leachate has a lower pH. This may significantly accelerate the release of arsenic from PV waste to the leachate. Second, we opted not to include a detailed speciation model for the dissolution of III–V species during the use phase when exposed to acid rain or acetic acid attack. These models can increase the complexity of the assessment significantly, and they depend on a very large variability of water and waste chemistries which are difficult to determine. Given that use phase emissions were considerably lower than the EOL emissions we decided to make conservative assumptions, although this may be an important aspect to incorporate if more detailed risk assessments are needed. Third, the dynamic emissions we calculated are largely dependent on the demand scenarios, more specifically the growth rates assumed for PV deployment (and the assumption of logistic growth curves). The market dynamics for emerging PV are difficult to predict, and many forecasts of PV deployment have proved overly pessimistic in recent years.39 Further coupling and updating of expected PV growth rates (specifically for III–V/Si markets) may shift the time-dependent results in a way that has important implications for this assessment.
Integration with LCA and SSbD frameworks
Our modelling framework takes an urgent step towards incorporating a Safe and Sustainable-by-design (SSbD) approach to technology development and innovation. Recent efforts such as the SSbD framework promoted by the EU40 call for an integration of LCA with chemical safety assessments (as well as other social and economic assessments). The SSbD framework centres on materials and chemicals; however, a comprehensive evaluation of their SSbD characteristics can only be achieved by taking into account the functional performance of these materials when incorporated into a product or service. This convolutes the linking of LCA (product-focused) with chemical risk assessment (materials focused) in such frameworks, as the life-cycles of products are very different to the life-cycles of the materials they are made of.41 The solution we propose is to raise the chemical safety assessment to the level of product/service -as done in this study-so that it can be integrated with LCA-based approaches, including environmental and social LCA as well as Life Cycle Costing (LCC). In our framework, this achieved by introducing technology-specific demand and emission scenarios which serve as a necessary bridge between the chemical risk assessment and the LCA type of framing.
This integration can happen in several ways, the most important one being that all life cycles are compatibilized across assessment types. Thus the underlying model representations of the technology and the social, economic and environmental context in which it is embedded can be shared across the chemical risk and LCA, S-LCA and LCC assessments. In our view, an even more promising benefit of such integration is in the identification and treatment of shared uncertainties and variabilities which span different modelling domains. Global sensitivity analysis can then be applied as we did here to prioritize and reduce the high-dimensionality problem of SSbD approaches. As a result, the robustness of SSbD decision-making processes during early innovation stages can be greatly enhanced.
Another aspect that can now be readily integrated is the use-phase and EOL emissions from the risk assessment model, which can be used to calculate environmental outflows in the corresponding unit processes of the LCA model. This is highly relevant, particularly for EOL, given that LCA disposal processes (e.g. incineration, landfilling) are typically taken from generic databases such as ecoinvent42 and do not represent technology-specific processes and emissions. If overlooked, this simplification could potentially introduce a large bias in the LCA model and mislead the choice of one technology over another, or of one particular design configuration over another. The risk assessment model, on the other hand, offers a sound estimation of technology-specific use-phase and EOL emissions that can be expected.
Conclusions
We conclude that the ecological risks from III–V materials emissions throughout the lifetime of III–V/Si PV panels are unlikely to pose a cause for concern, even under worst-case extreme situations such as those modelled in the local scale. The main source of potentially toxic releases would be the disposal of III–V/Si cells in above-ground landfills, a waste destination which countries are likely to continue to move away from in the coming years. In worst-case scenarios, we found that the relevant increases in concentrations would occur mostly in freshwater compartments. In soil, the mobility of III–V materials is very low, and releases will be diluted on the order of hundreds or thousands of years. Adhering to strict regulations for landfill containment and monitoring systems will dilute these processes further. In the case of gallium and indium, these elements have much lower reactivity, so the emissions that do occur will have negligible effects. Nevertheless, at smaller scales with the co-occurrence of intense PV utilization and disposal, the risks may increase so that careful monitoring of the efficacy of control measures is required, particularly around landfill and incineration abatement, collection of used PV panels and increased recycling of arsenic. These factors will become increasingly important considering potential future expansion of markets for other arsenic containing electronic waste, such as that from discarded integrated circuits and LED diodes.
It is also important to consider that current social and regulatory trends in Europe have a clear direction away from landfilling but also to considerable reducing waste while increasing the circularity of the economy. As an example, Germany sends less than 1% of its construction and demolition waste to landfills as of 2021.43 European regulations have set demanding thresholds for electronic waste recycling, and numerous studies have demonstrated technologies for recovering materials from LEDs, integrated circuits, and photovoltaic devices with III–V materials grown via MOVPE.44–47 These recycling techniques can only be expected to become more efficient and cost-effective in time. Furthermore, the growing concerns over resource availability and supply risks of III–V materials like indium and gallium will provide further incentives. Considering these factors, a low-emission and low-risk scenario for the life cycle of future III–V/Si PV technologies is likely.
Apart from the valuable insights gained regarding III–V/Si technology, the significance of the integrated model established in this study is equally crucial for conducting early-stage evaluations of chemical risks associated with emerging technologies. The model can be readily extended to other technologies beyond PV. In the past, such complex integrated models have seldom been applied at early R&D stages because of the effort and resources (time, knowledge) required to construct and set up the models and the numerous uncertainties faced. But the framework and calculation algorithms we have made available make the rapid screening of different scenarios possible, while preserving the complexity and wide spectrum of variable and uncertain factors found in real life. Furthermore, the framework offers an ideal tool to prioritize research and data collection on the most influential factors during subsequent R&D stages. It is vital to understand and address potential adverse impacts before widespread environmental dispersion of materials, especially for emerging technologies using new synthetic materials.
Methods
Overview of modelling framework
To assess the ecological risks from III–V/Si panels in future PV demand scenarios we developed an integrated model that consists of five steps. First, we determined demand for installed PV capacity (in MW or GW) over a one-hundred-year modelling period (2031–2130) for three geographical scales (continental, regional, local), based on relevant PV demand scenarios and stated policies in Europe and Amsterdam (see “Demand projections”). Second, we used a dynamic stock model to calculate the amount of PV panels that would be manufactured, installed, operated, recycled and discarded each year in order to satisfy the demand required in the previous step, while accounting for accidental panel breakage and panels reaching the end of their useful life (see “Dynamic stock flows”). Third, we calculated potential releases of arsenic, gallium and indium from PV panels to the environment at each life-cycle stage with a specific emission model developed for each release mechanism (see “Emissions of III–V metals and metalloids”). Fourth, we modelled the environmental distribution and fate of the emitted masses across different environmental compartments (soil, freshwater, air) in each year using a dynamic fate model, in order to obtain predicted environmental concentrations (PEC) in each compartment (see “Predicted Environmental Concentrations”). Finally, we calculated a risk quotient (RQ) as the ratio of PEC to the predicted no-effect concentration (PNEC) that has been reported in literature for each substance in each compartment (see “Predicted No-effect Concentration and Risk Quotients”).
All components of the model allow for the consideration of probability distributions for input parameters. The model's input parameter descriptions and the corresponding distributions used in this case study are reported in the ESI,† along with further details on calculations and assumptions. The model was built on the statistical software R supported by macro-enabled Microsoft Excel spreadsheets.
Demand projections
The growth in installed PV capacity over the period 2031–2130 in both the EUR and the AMS scenarios were modelled using logistic-growth curves. In EUR, we assumed an initial capacity addition of 100 MWp and stabilizing at 430 GWp. We took an annual growth rate of 14.1% from the 75th percentile of 1100 different PV deployment scenarios in Europe that were reviewed and harmonized by Jaxa-Rozen et al.48 In the AMS scenario we assumed an initial capacity addition of 0.1 MWp in the year 2031 and stabilizing at 110 MWp following a higher growth rate of 20%. For the LOC scenario the amounts of PV panels installed were kept constant throughout the modelling period, with replacement of broken panels and those that reach their EOL.
With an expected 28% panel conversion efficiency, III–V/Si panels will have a rating of 280 Wp per m2 of panel. Thus, every 1 MWp of planned installed capacity would require a PV installation with an effective area of 3571 m2.
Dynamic stock flows
Yearly stock flows of III–V/Si panels (quantified as m2 of PV panel) were calculated using a dynamic stock model49,50 for a one-hundred year modelling period. In the stock model, additional panels are manufactured each year to meet the increasing demand, to replace broken panels, and to replace panels that reached the end of their useful life (due to long-term degradation). In lieu of specific panel lifetime data, we assumed a normal distribution for III–V/Si panel lifetime of each yearly cohort centred at 30 years and with a standard deviation of 5. Accidental panel breakage rates of 0.06–0.12% per year were taken based on panel crack statistics reported by the International Energy Agency.51
Emissions of III–V metals and metalloids
Based on III–V/Si cell design specifications proposed by a European project,20 each m2 of panel would contain 8.81 g of arsenic (As), 15.06 g of gallium (Ga) and 0.1 g of indium (In). The As, Ga and In content in each panel is subject to environmental release depending on the specific conditions and dissolution processes that can take place during manufacturing, operation (use phase), end-of-life (EOL) phase (Fig. 7).
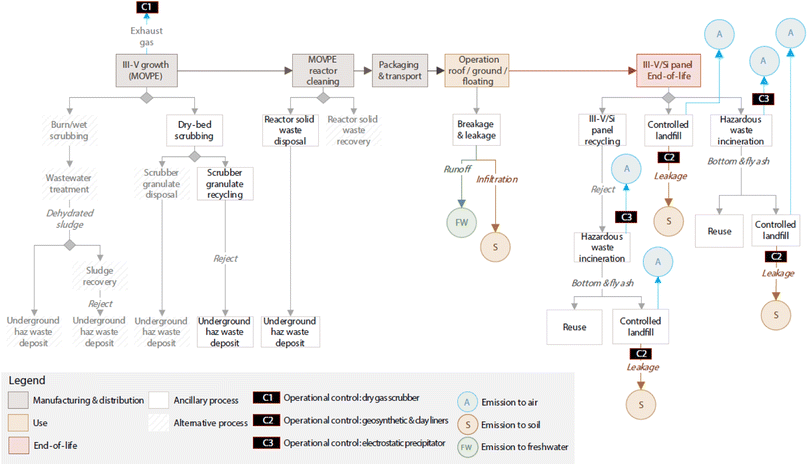 |
| Fig. 7 Identification of potential sources of III–V emissions in the life cycle of III–V/Si PV panels. | |
Manufacturing.
III–V substances enter the supply chain of III–V/Si cells in the metalorganic vapour phase epitaxy process (MOVPE) which is used to grow the absorber III–V layers on top of the silicon wafer. These substances are supplied from hydride gases and metalorganic precursors (arsine, trimethylgallium and trimethylindium). The fraction not deposited on the solar cells is distributed in two waste streams: a gas stream that is captured by a scrubber, and a solid stream composed of materials that deposit on the different elements of the reactor and on filters which are cleaned periodically. In the scrubber, a dry zeolite/copper-based granulate adsorbs the toxic substances.
The current best practice in the industry is to reintroduce the used scrubber granulate into the smelting process for copper, in which case the III–V content is captured as an acceptable impurity in the metal. It is likely that the valuable metals (indium, gallium) will be eventually separated and recovered. For arsenic there is no economic case at present, however there is technical feasibility for arsenic recovery from the used adsorbent granulates. Such recoveries may become economically viable when the arsenic content in waste is sufficient (e.g., ∼100 ton per year). Recovery may also be driven by resource scarcity of critical materials like indium and gallium,52 Recovery processes will have an associated efficiency, typically between 95–99%, and the remaining fraction (rejects) would be disposed in an underground hazardous waste storage facility.
The solid waste stream from MOVPE that deposits in the reactor is periodically removed as a standard cleaning procedure. This waste is also discarded in an underground hazardous waste storage facility. These types of facilities in Europe are typically installed on sealed and carefully monitored abandoned mine shafts, where potential migration of contaminants to relevant environmental compartments is deemed implausible.
Use phase (operation).
Two processes were modelled to estimate potential releases during operation: dissolution at the cracked surface of III–V materials directly exposed to rain, and transport of III–V materials on non-exposed parts that get dissolved by water ingress and are transported to the crack where it is then released. We modelled the former process following the method proposed by Celik et al.,53 which is based on an application of the Noyes–Whitney equation.54 The latter process was modelled using eqn (1) and (2), where transcrack is the transport of dissolved metal to the crack (g s−1), Jcrack is the flux of dissolved metal to the crack (g m−2 s−1), D is the diffusion coefficient of metal (m2 s−1), Cs is the saturated mass concentration of metal in water in g m−3, Cb is the concentration of metal in bulk solvent (rainwater) in g m−3, and distancecr is the average travel distance of the metal from any point in the panel to the crack (m), calculated using the method of Mathai et al.55 Cracked panels were assumed to leach for one year after which they would be replaced. | transcrack = Jcrack × Acr_side | (1) |
| 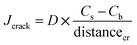 | (2) |
End-of-life phase: recycling.
The European Waste Management Directive for electronic products including photovoltaic panels-requires that 85% is collected for treatment and preparation for reuse/recycling.56 It is likely, however, that the panels are disassembled to recover the easily recyclable materials such as aluminium and glass. We modelled two recycling scenarios for each scale: with and without recovery of III–V materials. In the former case we assumed recovery efficiencies for these processes based on existing patents and published recycling methods for similar technologies.45,47,57
End-of-life phase: incineration.
In incineration facilities, it has been found that 20–80% of arsenic in waste may remain in the bottom ash while the rest is volatized.58 The volatized fraction is directed to emission control mechanisms at the stack such as electrostatic precipitators (ESP) with removal efficiencies that typically range between 99.5–99.9%.59 Arsenic that is not vaporised in the incinerated panels is emitted to air and the remaining fraction is collected as secondary waste with bottom ash, fly ash and filters. Gallium and indium do not form volatile organic compounds, so we assumed 100% remains in the bottom ash. In Europe, secondary waste from incineration facilities is typically either sent to a controlled landfill or reused in construction material.60
End-of-life phase: landfill.
Two main processes drive emissions from landfilled PV waste: leaching from the waste to the leachate within the landfill, and leakage of the leachate from the landfill to the surrounding soil. The former will be largely regulated by a waste/leachate partitioning coefficient (kW) which can be determined empirically from leaching tests or field measurements. Leaching and subsequent leakage from the landfill will also be largely regulated by the effective infiltration (I), the amount of rainfall that infiltrates and passes through the landfill's containment structures such as clay or geosynthetic liners. We use a simplified version of EPA's Composite Model for Leachate Migration with Transformation Products (EPACMTP),61 where the mass balance for a landfill cell is given by eqn (3) and (4). |  | (3) |
When modelling emissions we took a conservative approach and assumed that all III–V elements in the PV cells are fully soluble. This is a common starting point for metals risk assessment within the EU.62 Another important consideration is that, once released, metals and metalloids can exist in different forms like organic complexes with dissolved organic matter, inorganic complexes with dissolved anions, or free hydrated metal ions. This applies especially to arsenic, which can exist in four oxidation states with different toxicities: −3, 0, +3, and +5. In this study, we assumed that arsenic dissolves entirely to its most toxic form (arsenite, +3). Indium and gallium may also exist in different oxidation states, but once released to the environment tend to revert to their +3 oxidation state.63
Predicted environmental concentrations
We then modelled the distribution of the emitted III–V substances in the environment using SimpleBox v4, a widely used tool for fate modelling developed by the Netherlands Institute for Public Health and the Environment (RIVM).64,65 For the EUR continental scale we used the landscape settings for the European continent that were established for the European Union System for the Evaluation of Substances (EUSES),66 In the SimpleBox model, the continental scale contained the regional AMS scale embedded, which in turn contained the embedded local LOC scale (SimpleBox calculates exchanges between embedded scales). To model the regional AMS and LOC landscape we derived surface water and soil coverage data from GIS data made available by the Amsterdam municipality,67 and weather data provided by the Royal Netherlands Meteorological Institute (KNMI).68 In our fate model, the emissions to lakewater in the local scale are added to the freshwater compartment.
To conduct dynamic PEC calculations we coupled a probabilistic implementation of the SimpleBox model using the @Risk add-in (Palisade, v8.1.0) with the deSolve69 package in R. SimpleBox is based on the original implementation as described in Schoorl et al.64,65 with the addition of a local scale with an air, soil, water and sediment compartment based on van de Meent et al.70 In this implementation, the model matrix of all rate constants is read from the SimpleBox Excel spreadsheets and combined with the annual III–V emissions using the event function in deSolve.
Predicted no-effect concentrations and risk quotients
We took the PNEC values recommended by the European Chemicals Agency (ECHA) in the registration dossiers for each substance.71–73 Depending on each case, these were derived by ECHA from EC10 or EC50 (concentration at which 10% or 50% of the target organism presents the observed effect), LC50 (lethal concentration for 50% of the observed organisms) and LOEC (lowest observed effect concentration) values reported in literature. An assessment factor is applied to account for uncertainty in extrapolation from lab to field results, from single-species to ecosystem level, and/or for the limited availability of datapoints.74,75
Arsenic.
The PNEC value recommended by ECHA for freshwater organisms is 5.6 μg L−1, after application of an assessment factor of 3. For soil, the recommended PNEC is 2.9 mg kg−1 soil (dry weight) after an assessment factor of 2 is applied.
Gallium.
One NOEC for freshwater organisms was reported in the ECHA database of 10
300 μg L−1.72 Following ECHA guidelines,74 an assessment factor of 100 should be applied for a single NOEC value, resulting in a PNEC of 103 μg L−1. There was only one datum for soil organisms reported in literature, an EC50 of 0.271 g kg−1 soil (dw) for rice plants in acidic soil (no effects were observed in neutral soils).76 Applying an assessment factor of 100 gives a PNEC of 2.7 × 10−3 g kg−1 soil (dw). For soil, ECHA recommends using the Equilibrium Partitioning Method as an alternative calculation method when only one datum is available and choosing the lowest PNEC obtained from both methods. The Equilibrium Partitioning Method uses the PNEC in water to estimate PNEC in soil according to eqn (5): | 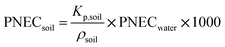 | (5) |
In eqn (5), Kp,soil is the soil water partition coefficient for gallium (see ESI Table S2†) and ρsoil is the density of soil phase, 2500 kg m−3. This would result in a PNEC of 4 × 10−2 g kg−1 soil (dw). We therefore take the lower PNEC of 2.7 × 10−3 g kg−1 soil (dw).
Indium.
The toxicity data for indium (In3+) were taken from the ECHA database, which recommends a PNEC of 40.6 μg L−1 after applying an assessment factor of 3. For terrestrial organisms, the recommended value is 7.3 × 10−3 g kg−1 soil (dw), after applying an assessment factor of 10.73
RQs for each compartment were calculated as the PEC/PNEC ratio, where RQ values approaching or exceeding 1 indicate a potential situation of concern.
Uncertainty and global sensitivity analysis
We used the Monte Carlo uncertainty propagation method77 to determine uncertainty in the PECs and RQs as a result of uncertainties and variabilities in the model's input parameters. For the Monte Carlo simulation we sampled 1000 sets of random values for these parameters from their underlying distributions, and recalculated PECs and RQs for each set of values throughout the period 2031–2130. This produced a probability distribution for each PEC and RQ in each year, from which summary statistics (geometric mean, 25th and 75th percentiles and interquartile ranges) were derived.
Finally, we conducted a global sensitivity analysis using the moment-independent sensitivity importance measure proposed by Borgonovo78,79 to rank all uncertain parameters in terms of their contribution to uncertainty in the resulting RQs in freshwater and natural soil compartments for all scales. We calculated these sensitivity measures using the sensiFdiv function in the sensitivity package for R developed by Iooss et al.80
Data availability
The data and source code for the analysis can be found in: https://doi.org/10.5281/zenodo.7032992.
Author contributions
C. F. B., J. T. Q., M. H., W. J. G. M. P, M. G. V. and P. B. conceived the research. C. F. B., J. T. Q., M. H. and A. F. designed the model. C. F. B., J. T. Q., M. H. and A. F. developed the code. C. F. B., M. H. and F. D collected and/or provided data. Supervision was provided by M. G. V, W. J. G. M. P., and S. C. W. J. G. M. P., M. G. V., J. T. Q. and F. D. secured the funding. All authors discussed the results, wrote, and commented on the manuscript.
Conflicts of interest
There are no conflicts of interest to declare.
Acknowledgements
This work was supported by the European Union's ERC-consolidator grant agreement no. 101002123 granted to MGV, the European Union's Horizon 2020 research and innovation programme under the SiTaSol project grant agreement no. 727497; the Dutch National Institute for Public Health and the Environment (RIVM) Strategic Programme (SPR) under the DIRECT project (S/030003). The authors would also like to thank Niall Hodgins and Dr Mengya Tao for their valuable insights which guided part of this work.
References
-
IEA PVPS, Snapshot of Global PV Markets 2022, 2022 Search PubMed.
- W. Jager, Stimulating the diffusion of photovoltaic systems: A behavioural perspective, Energy Policy, 2006, 34(14), 1935–1943 CrossRef.
- J. Leenheer, M. de Nooij and O. Sheikh, Own power: Motives of having electricity without the energy company, Energy Policy, 2011, 39(9), 5621–5629 CrossRef.
-
European Chemicals Agency, Silicon – Registration Dossier, 2020, available from: https://echa.europa.eu/registration-dossier/-/registered-dossier/16144/7/1 Search PubMed.
- S. Essig, C. Allebé, T. Remo, J. F. Geisz, M. A. Steiner and K. Horowitz,
et al., Raising the one-sun conversion efficiency of III–V/Si solar cells to 32.8% for two junctions and 35.9% for three junctions, Nat. Energy, 2017, 2(9), 17144 CrossRef CAS.
- M. Feifel, J. Ohlmann, J. Benick, M. Hermle, J. Belz and A. Beyer,
et al., Direct growth of III–V/Silicon triple-junction solar cells with 19.7% efficiency, IEEE J. Photovolt., 2018, 8(6), 1590–1595 Search PubMed . available from: https://ieeexplore.ieee.org/document/8467307/.
- R. Cariou, J. Benick, F. Feldmann, O. Höhn, H. Hauser and P. Beutel,
et al., III–V-on-silicon solar cells reaching 33% photoconversion efficiency in two-terminal configuration, Nat. Energy, 2018, 3(4), 326–333 CrossRef CAS . available from: https://www.nature.com/articles/s41560-018-0125-0.
-
F. Dimroth, III–V Solar Cells – Materials, Multi-Junction Cells – Cell Design and Performance, in Photovoltaic Solar Energy, John Wiley & Sons, Ltd, Chichester, UK, 2017, pp. 371–82, available from: http://doi.wiley.com/10.1002/9781118927496.ch34 Search PubMed.
-
U. Heitmann, S. Kluska, J. Bartsch, H. Hauser, A. Ivanov and S. Janz, Novel Approach for the Bonding of III–V on Silicon Tandem Solar Cells with a Transparent Conductive Adhesive, in 2018 IEEE 7th World Conference on Photovoltaic Energy Conversion, WCPEC 2018 – A Joint Conference of 45th IEEE PVSC, 28th PVSEC and 34th EU PVSEC, IEEE, 2018, pp. 201–205 Search PubMed.
- C. van der Giesen, S. Cucurachi, J. Guinée, G. J. Kramer and A. Tukker, A critical view on the current application of LCA for new technologies and recommendations for improved practice, J. Cleaner Prod., 2020, 259, 120904 CrossRef.
- M. Villares, A. Işıldar, C. van der Giesen and J. Guinée, Does ex ante application enhance the usefulness of LCA? A case study on an emerging technology for metal recovery from e-waste, Int. J. Life Cycle Assess., 2017, 1–16 Search PubMed . available from: http://link.springer.com/10.1007/s11367-017-1270-6.
-
S. Cucurachi and C. F. Blanco, Practical solutions for ex-ante LCA illustrated by emerging PV technologies, in Ensuring the Environmental Sustainability of Emerging Technologies, ed. Florin M. V., EPFL International Risk Governance Center, Lausanne, 2023, pp. 149–67 Search PubMed.
- C. F. Blanco, S. Cucurachi, W. J. G. M. Peijnenburg, A. Beames and M. G. Vijver, Are Technological Developments Improving the Environmental Sustainability of Photovoltaic Electricity?, Energy Technol., 2020, 1901064 CrossRef CAS . available from: https://onlinelibrary.wiley.com/doi/abs/10.1002/ente.201901064.
- C. F. Blanco, S. Cucurachi, F. Dimroth, J. B. Guinée, W. J. G. M. Peijnenburg and M. G. Vijver, Environmental impacts of III–V/silicon photovoltaics: life cycle assessment and guidance for sustainable manufacturing, Energy Environ. Sci., 2020, 13(11), 4280–4290 RSC . available from: http://xlink.rsc.org/?DOI=D0EE01039A.
- A. W. Sleeswijk, R. Heijungs and S. T. Erler, Risk Assessment and Life-cycle Assessment: Fundamentally different yet reconcilable, Greener Manag. Int., 2003, 77–87 CrossRef.
- H. A. Udo de Haes, A. W. Sleeswijk and R. Heijungs, Similarities, Differences and Synergisms Between HERA and LCA—An Analysis at Three Levels, Hum. Ecol. Risk Assess., 2006, 12(3), 431–449 CrossRef . available from: http://www.tandfonline.com/doi/abs/10.1080/10807030600561659.
- Y. S. Zimmermann, A. Schäffer, P. F. X. Corvini and M. Lenz, Thin-film photovoltaic cells: Long-term metal(loid) leaching at their end-of-life, Environ. Sci. Technol., 2013, 47(22), 13151–13159 CrossRef CAS PubMed.
- A. Babayigit, A. Ethirajan, M. Muller and B. Conings, Toxicity of organometal halide perovskite solar cells, Nat. Mater., 2016, 15(3), 247–251 CrossRef CAS PubMed . available from: http://www.nature.com/articles/nmat4572.
-
I. Celik, Z. Song, M. J. Heben, Y. Yan and D. S. Apul, Life cycle toxicity analysis of emerging PV cells, in Conference Record of the IEEE Photovoltaic Specialists Conference, IEEE, 2016, pp. 3598–601 Search PubMed.
-
Fraunhofer ISE, SiTaSol: Application Relevant Validation of C-Si Based Tandem Solar Cell Processes, 2021, available from: https://sitasol.com/ Search PubMed.
-
D. Collingridge, The Social Control of Technology, Frances Pinter, New York, 1980, p. 200 Search PubMed.
-
U.S. Environmental Protection Agency, Risk Assessment Forum White Paper: Probabilistic Risk Assessment Methods and Case Studies, Washington D.C., 2014, Report no.: EPA/100/R-09/001A, available from: http://epa.gov/raf/prawhitepaper/index.htm Search PubMed.
-
A. Saltelli, M. Ratto, T. Andres, F. Campolongo, J. Cariboni and D. Gatelli, et al., Global Sensitivity Analysis. The Primer. Global Sensitivity Analysis. The Primer, John Wiley and Sons, 2008, pp. 1–292 Search PubMed.
-
Shell International B.V., Shell Scenarios SKY Meeting the Goals of the Paris Agreement, 2018, Sky Scenario, available from: https://www.shell.com/energy-and-innovation/the-energy-future/scenarios/shell-scenario-sky.html Search PubMed.
-
IEA, The Role of Critical Minerals in Clean Energy Transitions, Paris, 2021 Search PubMed.
-
City of Amsterdam. Policy: Sustainability and energy. Policy: Renewable energy, available from: https://www.amsterdam.nl/en/policy/sustainability/renewable-energy/.
- D. Kucharavy and R. De Guio, Logistic substitution model and technological forecasting, Procedia Eng., 2011, 402–416 CrossRef.
- A. Leclerc, S. Sala, M. Secchi and A. Laurent, Building national emission inventories of toxic pollutants in Europe, Environ. Int., 2019, 130, 104785 CrossRef CAS PubMed.
- D. van de Meent, D. de Zwart, J. Struijs, J. L. M. Hermens, N. M. van Straalen and K. H. den Haan,
et al., Expected Risk as basis for assessment of safe use of chemicals, Environ. Sci. Eur., 2023, 35(1), 16 CrossRef CAS.
- C. K. Jain and I. Ali, Arsenic: occurrence, toxicity and speciation techniques, Water Res., 2000, 34(17), 4304–4312 CrossRef CAS . available from: http://linkinghub.elsevier.com/retrieve/pii/S0043135400001822.
- P. Pinel-Raffaitin, I. Le Hecho, D. Amouroux and M. Potin-Gautier, Distribution and Fate of Inorganic and Organic Arsenic Species in Landfill Leachates and Biogases, Environ. Sci. Technol., 2007, 41(13), 4536–4541 CrossRef CAS PubMed.
-
World Health Organization, Environmental Health Criteria 224 Arsenic and Arsenic Compounds, Geneva, 2nd edn, 2001 Search PubMed.
-
K. Harstad, Handling and Assessment of Leachates from Municipal Solid Waste Landfills in the Nordic Countries, 2007, p. 175 Search PubMed.
- S. L. Shumlas, S. Singireddy, A. C. Thenuwara, N. H. Attanayake, R. J. Reeder and D. R. Strongin, Oxidation of arsenite to arsenate on birnessite in the presence of light, Geochem. Trans., 2016, 17(1), 5 CrossRef PubMed . available from: https://geochemicaltransactions.biomedcentral.com/articles/10.1186/s12932-016-0037-5.
- X. Li, F. Zhang, H. He, J. J. Berry, K. Zhu and T. Xu, On-device lead sequestration for perovskite solar cells, Nature, 2020, 578(7796), 555–558 CrossRef CAS PubMed . available from: https://www.nature.com/articles/s41586-020-2001-x.
-
J. D. Allison and T. L. Allison, Partitioning Coefficients for Metals in Surface Water, Soil and Waste. Washington, D.C., 2005, available from: https://cfpub.epa.gov/si/si_public_record_report.cfm?Lab=NERL%26dirEntryId=135783 Search PubMed.
- T. Uddh Söderberg, D. Berggren Kleja, M. Åström, J. Jarsjö, M. Fröberg and A. Svensson,
et al., Metal solubility and transport at a contaminated landfill site – From the source zone into the groundwater, Sci. Total Environ., 2019, 668, 1064–1076 CrossRef PubMed , available from: https://linkinghub.elsevier.com/retrieve/pii/S0048969719309921.
- B. Adothu, P. Bhatt, S. Chattopadhyay, S. Zele, J. Oderkerk and H. P. Sagar,
et al., Newly developed thermoplastic polyolefin encapsulant – A potential candidate for crystalline silicon photovoltaic modules encapsulation, Sol. Energy, 2019, 194, 581–588 CrossRef CAS.
- F. Creutzig, P. Agoston, J. C. Goldschmidt, G. Luderer, G. Nemet and R. C. Pietzcker, The underestimated potential of solar energy to mitigate climate change, Nat. Energy, 2017, 2(9), 17140 CrossRef . available from: http://www.nature.com/articles/nenergy2017140.
-
European Commission, Recommendation 2022/2510 of 8 December 2022 Establishing a European Assessment Framework for ‘Safe and Sustainable by Design’ Chemicals and Materials, Official Journal of the European Union, 2022, p. 2531 Search PubMed.
- J. B. Guinée, R. Heijungs, M. G. Vijver, W. J. G. M. Peijnenburg and G. Villalba Mendez, The meaning of life … cycles: lessons from and for safe by design studies, Green Chem., 2022, 24, 7787–7800 RSC.
- G. Wernet, C. Bauer, B. Steubing, J. Reinhard, E. Moreno-Ruiz and B. Weidema, The ecoinvent database version 3 (part I): overview and methodology, Int. J. Life Cycle Assess., 2016, 21(9), 1218–1230 CrossRef . available from: http://link.springer.com/10.1007/s11367-016-1087-8.
- W. Ferdous, A. Manalo, R. Siddique, P. Mendis, Y. Zhuge and H. S. Wong,
et al., Recycling of landfill wastes (tyres, plastics and glass) in construction – A review on global waste generation, performance, application and future opportunities, Resour., Conserv. Recycl., 2021, 173, 105745 CrossRef CAS . available from: https://linkinghub.elsevier.com/retrieve/pii/S0921344921003542.
- L. Zhan, J. Li, B. Xie and Z. Xu, Recycling Arsenic from Gallium Arsenide Scraps through Sulfurizing Thermal Treatment, ACS Sustain. Chem. Eng., 2017, 5(4), 3179–3185 CrossRef CAS . available from: http://pubs.acs.org/doi/pdf/10.1021/acssuschemeng.6b02962.
- A. Van Den Bossche, W. Vereycken, T. Vander Hoogerstraete, W. Dehaen and K. Binnemans, Recovery of Gallium, Indium, and Arsenic from Semiconductors Using Tribromide Ionic Liquids, ACS Sustain. Chem. Eng., 2019, 7(17), 14451–14459 CrossRef CAS.
-
Nanjing Jinmei Gallium Co. Ltd, Vacuum Decomposing Apparatus for Separating Gallium Arsenide as Metal Gallium and Metal Arsenic, CN101413065A, 2009, available from: https://www.google.com/patents/CN101413065A?cl=en.
- L. Zhan, F. Xia, Y. Xia and B. Xie, Recycle Gallium and Arsenic from GaAs-Based E-Wastes via Pyrolysis-Vacuum Metallurgy Separation: Theory and Feasibility, ACS Sustain. Chem. Eng., 2018, 6(1), 1336–1342 CrossRef CAS.
- M. Jaxa-Rozen and E. Trutnevyte, Sources of uncertainty in long-term global scenarios of solar photovoltaic technology, Nat. Clim. Change, 2021, 11(3), 266–273 CrossRef . available from: https://doi.org/10.1038/s41558-021-00998-8.
- S. Deetman, S. Pauliuk, D. P. van Vuuren, E. van der Voet and A. Tukker, Scenarios for Demand Growth of Metals in Electricity Generation Technologies, Cars, and Electronic Appliances, Environ. Sci. Technol., 2018, 52(8), 4950–4959 CrossRef CAS PubMed . available from: https://pubs.acs.org/doi/full/10.1021/acs.est.7b05549.
- S. Pauliuk and N. Heeren, ODYM—An open software framework for studying dynamic material systems: Principles, implementation, and data structures, J. Ind. Ecol., 2020, 24(3), 446–458 CrossRef . available from: https://onlinelibrary.wiley.com/doi/full/10.1111/jiec.12952.
-
M. Köntges, S. Kurtz, U. Jahn, K. Berger, K. Kato and T. Friesen, et al., Review of Failures of Photovoltaic Modules. 2014, available from: https://iea-pvps.org/wp-content/uploads/2020/01/IEA-PVPS_T13-01_2014_Review_of_Failures_of_Photovoltaic_Modules_Final.pdf Search PubMed.
- C. Licht, L. T. Peiró and G. Villalba, Global Substance Flow Analysis of Gallium, Germanium, and Indium: Quantification of Extraction, Uses, and Dissipative Losses within their Anthropogenic Cycles, J. Ind. Ecol., 2015, 19(5), 890–903 CrossRef.
- I. Celik, Z. Song, A. B. Phillips, M. J. Heben and D. Apul, Life cycle analysis of metals in emerging photovoltaic (PV) technologies: A modeling approach to estimate use phase leaching, J. Cleaner Prod., 2018, 186, 632–639 CrossRef CAS . available from: https://www.sciencedirect.com/science/article/pii/S0959652618307200.
- A. A. Noyes and W. R. Whitney, The rate of solution of solid substances in their own solutions, J. Am. Chem. Soc., 1897, 19(12), 930–934 CrossRef . available from: https://pubs.acs.org/doi/abs/10.1021/ja02086a003.
- A. M. Mathai, P. Moschopoulos and G. Pederzoli, Random points associated with rectangles, Rend. Circ. Mat. Palermo, 1999, 48(1), 163–190 CrossRef . available from: https://link.springer.com/article/10.1007/BF02844387.
-
European Parliament; Council of the European Union, Directive 2012/19/EU of the European Parliament and of the Council of 4 July 2012 on Waste Electrical and Electronic Equipment (WEEE), 2012 Search PubMed.
- M. Maarefvand, S. Sheibani and F. Rashchi, Recovery of gallium from waste LEDs by oxidation and subsequent leaching, Hydrometallurgy, 2020, 191 Search PubMed.
- C. H. Jung, T. Matsuto, N. Tanaka and T. Okada, Metal distribution in incineration residues of municipal solid waste (MSW) in Japan, Waste Manage., 2004, 24(4), 381–391 CrossRef CAS PubMed . available from: https://linkinghub.elsevier.com/retrieve/pii/S0956053X03001375.
- F. Hasselriis and A. Licata, Analysis of heavy metal emission data from municipal waste combustion, J. Hazard. Mater., 1996, 47(1–3), 77–102 CrossRef CAS.
- D. Blasenbauer, F. Huber, J. Lederer, M. J. Quina, D. Blanc-Biscarat and A. Bogush,
et al., Legal situation and current practice of waste incineration bottom ash utilisation in Europe, Waste Manage., 2020, 102, 868–883 CrossRef PubMed.
-
U.S. Environmental Protection Agency, Office of Solid Waste. EPA’s Composite Model for Leachate Migration with Transformation Products (EPACMTP) Technical Background Document, Washington, D.C., 2003 Search PubMed.
-
European Chemicals Agency, Guidance on Information Requirements and Chemical Safety Assessment – ECHA, Helsinki, 2011, available from: https://echa.europa.eu/guidance-documents/guidance-on-information-requirements-and-chemical-safety-assessment Search PubMed.
- H. Jensen, S. Gaw, N. J. Lehto, L. Hassall and B. H. Robinson, The mobility and plant uptake of gallium and indium, two emerging contaminants associated with electronic waste and other sources, Chemosphere, 2018, 209, 675–684 CrossRef CAS PubMed.
-
M. Schoorl, A. Hollander and D. van de Meent, SimpleBox 4.0 A Multimedia Mass Balance Model for Evaluating the Fate of Chemical Substances, Bilthoven, The Netherlands, 2015, available from: https://www.rivm.nl/bibliotheek/rapporten/2015-0161.pdf Search PubMed.
- A. Hollander, M. Schoorl and D. van de Meent, SimpleBox 4.0: Improving the model while keeping it simple…, Chemosphere, 2016, 148, 99–107 CrossRef CAS PubMed . available from: https://linkinghub.elsevier.com/retrieve/pii/S0045653516300066.
- T. G. Vermeire, D. T. Jager, B. Bussian, J. Devillers, K. Den Haan and B. Hansen,
et al., European Union System for the Evaluation of Substances (EUSES). Principles and structure, Chemosphere, 1997, 34(8), 1823–1836 CrossRef CAS PubMed.
-
City of Amsterdam. 2019. Maps Data, available from: https://maps.amsterdam.nl/open_geodata/?LANG=en.
-
The Royal Netherlands Meteorological Institute (KNMI), Klimatologie – Metingen en waarnemingen, 2021, available from: https://www.knmi.nl/nederland-nu/klimatologie-metingen-en-waarnemingen Search PubMed.
- K. Soetaert, T. Petzoldt and R. W. Setzer, Solving Differential
Equations in R : Package deSolve, J. Stat. Softw., 2010, 33(9), 1–25 Search PubMed . available from: https://www.jstatsoft.org/index.php/jss/article/view/v033i09/v33i09.pdf.
- D. van de Meent, D. Zwart and L. Posthuma, Screening-Level Estimates of Environmental Release Rates, Predicted Exposures, and Toxic Pressures of Currently Used Chemicals, Environ. Toxicol. Chem., 2020, 39(9), 1839–1851 CrossRef CAS PubMed . available from: https://onlinelibrary.wiley.com/doi/10.1002/etc.4801.
-
European Chemicals Agency, Arsenic. Arsenic – Registration Dossier – ECHA, 2022, available from: https://echa.europa.eu/registration-dossier/-/registered-dossier/22366 Search PubMed.
-
European Chemicals Agency, Gallium. Gallium – Registration Dossier – ECHA, 2022, available from: https://echa.europa.eu/registration-dossier/-/registered-dossier/23228/6/2/4 Search PubMed.
-
European Chemicals Agency, Indium. Indium – Registration Dossier – ECHA, 2021, available from: https://echa.europa.eu/registration-dossier/-/registered-dossier/22264 Search PubMed.
-
ChemSafetyPro, How to Calculate Predicted No-Effect Concentration (PNEC), 2021 Search PubMed.
-
European Chemicals Agency, Guidance on Information Requirements and Chemical Safety Assessment Chapter R.10: Characterisation of Dose [concentration]-Response for Environment, 2008 Search PubMed.
- J. Y. Su, C. H. Syu and D. Y. Lee, Growth inhibition of rice (Oryza sativa L.) seedlings in Ga- and In-contaminated acidic soils is respectively caused by Al and Al + In toxicity, J. Hazard. Mater., 2018, 344, 274–282 CrossRef CAS PubMed.
-
M. Firestone, P. Fenner-Crisp, T. Barry, D. Bennett, S. Chang and M. Callahan, et al.Guiding Principles for Monte Carlo Analysis – Technical Panel, Washington, D.C., 1997, available from: https://www.epa.gov/risk/guiding-principles-monte-carlo-analysis Search PubMed.
- E. Borgonovo, A new uncertainty importance measure, Reliab. Eng. Syst. Saf., 2007, 92(6), 771–784 CrossRef . available from: https://www.sciencedirect.com/science/article/pii/S0951832006000883.
- E. Plischke and E. Borgonovo, Fighting the Curse of Sparsity: Probabilistic Sensitivity Measures From Cumulative Distribution Functions, Risk Anal., 2020, 2639–2660 CrossRef PubMed , available from: https://onlinelibrary.wiley.com/doi/abs/10.1111/risa.13571.
-
B. Iooss, S. Da Veiga, A. Janon and G. Pujol, Global Sensitivity Analysis of Model Outputs, 2021, available from: https://cran.r-project.org/web/packages/sensitivity/sensitivity.pdf Search PubMed.
Footnotes |
† Electronic supplementary information (ESI) available. See DOI: https://doi.org/10.1039/d3em00492a |
‡ In their Regional Energy Strategy, the Amsterdam municipality has marked floating PV as a last resort, only to take place if the regional and national goals cannot be satisfied with installation on rooftops and other public infrastructure. |
|
This journal is © The Royal Society of Chemistry 2024 |