Estimation of hourly black carbon aerosol concentrations from glass fiber filter tapes using image reflectance-based method†
Received
29th November 2022
, Accepted 5th March 2023
First published on 6th March 2023
Abstract
Black carbon (BC) is a carbonaceous component of fine particulate matter (PM2.5) that is quantified via light absorption. We demonstrate a low-cost method for quantifying BC using cell phone camera images. BC concentration is correlated with red light absorbance that is measured from photographs of particle filter samples following image processing steps to account for distortion (geometric calibration) and lighting conditions (color calibration). We trained multiple Red channel to BC models using ambient air filter samples and found that the exponential model best explains the correlation (R2 ∼ 0.94). Our approach has an effective minimum detection limit of 0.15 μg m−3 of ambient BC for an hourly sample collected at 1 m3 h−1. This detection limit should be sufficient to quantify BC concentrations at high time resolution at locations worldwide. We demonstrate the performance of our optical BC method using a combination of filter samples and filter tapes from beta attenuation monitors (BAMs) operating at two sites in an urban setting in Pittsburgh, Pennsylvania, USA. Our approach compares favorably to reference filter-based methods, suggesting that post-analysis of BAM tapes collected worldwide can be a valuable source for PM composition data, especially in countries in the Global South where there is insufficient air quality data to make informed policy decisions.
Environmental significance
PM2.5 exposure is responsible for around 3.3 million premature deaths every year, and yet, there is insufficient air quality data in many developing nations. PM2.5 composition data is critical to identify sources and help in evidence-based policymaking. This work developed a low-cost method to quantify atmospheric black carbon (BC), a component of PM2.5. The method estimates hourly BC concentrations using photographs of particulate deposits on filter tapes from existing PM2.5 monitors. We will be able to measure the BC concentrations above 0.15 μg m−3 in places across the globe with instruments that have fixed-interval particulate deposit spots on filters. The BC concentration data generated from this work will be publicly shared, and we plan to reach out to local collaborators in developing nations who can use the data to build air quality control policies.
|
1 Introduction
Exposure to air pollution poses a severe risk to global public health.1–3 It is the fifth leading cause of death worldwide and is attributed to around 4.9 million premature deaths every year.4 The risk is more critical in low- and middle-income countries due to a lack of effective public health policies. Among the air pollutants, PM2.5 (particulate matter with an aerodynamic diameter of 2.5 μm or smaller) alone is responsible for nearly 3.3 million premature deaths annually.2
Air quality in the US is regulated by the Environmental Protection Agency (EPA) under the Clean Air Act, and there is a robust infrastructure to measure pollutant concentrations nationwide. Yet, air quality information is not adequate in many densely populated lands around the world to make informed policy decisions, especially in the Global South (Africa, Latin America, developing parts of Asia and the Middle East).5–7 These developing countries are still industrializing and continue to emit large amounts of air pollutants in the process, but do not always have enough resources to monitor the emissions and ambient concentrations. There is a critical need for better quantification of both PM2.5 concentration and composition in the Global South.5–12 PM2.5 concentration tells about exposure; composition data can help us investigate emission sources and define future policies.13 A major barrier is the high capital and operational costs of research-grade air pollutant monitors. Thus, there is incentive to develop low-cost methods for improving air quality monitoring in the Global South.5
Black carbon (BC), commonly referred to as soot, is a component of PM2.5. It is a refractory form of carbon and is primarily formed by incomplete combustion of fossil fuels, biofuel, or biomass.14 BC is emitted from gasoline and diesel engines, coal-fired power plants, and other sources burning fossil fuels. In addition, it does not participate in chemical reactions in the atmosphere which makes it an important tracer for combustion emissions.15–17 BC is important to quantify and control as it adds to the health burden due to PM2.5 and has shown higher health impacts compared to PM2.5 for similar mass concentrations.18,19
Particulate pollutants in the atmosphere interact with solar radiation by absorbing and scattering it at different wavelengths.20 BC is defined as optically absorbing carbon in particles and is generally measured optically by quantifying the absorbance of infrared or red light through particle-laden filters.21,22 EC (elemental carbon) is another measure of carbon soot in the air and is quantified operationally as carbonaceous aerosols measured via thermal-optical methods. Contini et al. reported anthropogenic and natural combustion sources to be the main contributors to BC or EC in the atmosphere.23 The correlation between BC and EC as well as the BC-to-EC mass ratio can vary with sampling environments due to differences in sources.24 PM mass concentrations can also be optically quantified by the transmission of beta rays through a filter tape laden with PM. This study builds on these optical attenuation methods.
Aethalometers are widely used for continuous measurement of ambient BC concentrations.22 An aethalometer collects particulate matter on a quartz fiber filter tape at a fixed flow rate. Then, it uses Beer–Lambert law to estimate BC by measuring attenuation of a near-infrared light beam (880 nm) through the particle sample. Most aethalometers also measure light absorption by particles at 370 nm. Organic aerosol components of wood smoke exhibit an enhanced light absorption at 370 nm compared to 880 nm.25 The difference in PM concentrations measured by aethalometers at the two wavelengths (Delta-C = UVPM − BC) is strongly correlated with wood smoke markers in heating seasons and hence has been used as a tracer for wood smoke particles.26,27 However, applications of aethalometer are limited due to its high installation and operational cost as well as a need for high technical expertise.
A few studies have tried to find cost-effective ways of measuring ambient BC using photographs of PM on filter surfaces. A digital image is composed of multiple pixels and each pixel in a colored image is a combination of three color channels (R – Red, G – Green and B – Blue). Every color channel ranges between 0 to 255 for a 24 bit image (8 bits per channel), where 0 indicates no light (zero intensity) and 255 means maximum light (maximum intensity). Cheng et al.28 found a high correlation of EC loading on a filter sample with the average of R, G and B channels in the sample image. Ramanathan et al.29 used reflectance of red light (R channel) from photos of BC captured on quartz fiber filters to measure its concentration. Jeronimo et al.30 evaluated the method for polytetrafluoroethylene (PTFE) filters. These studies used BC reflectance on discrete filter samples with long sampling periods which gives a low temporal resolution dataset. However, high time resolution (daily or preferably hourly) is needed to get a better understanding of temporally changing PM sources.
The US Department of State collects air pollutant data at US Embassies around the world to inform US personnel and citizens of air quality overseas. These measurements use Beta Attenuation Monitors (BAMs) to measure hourly ambient PM2.5 concentrations.31 BAMs collect PM2.5 onto a glass-fiber filter tape and estimate particle concentrations by measuring absorption of beta radiation across the sample using the Beer–Lambert law.
This study investigates a cost-effective method to leverage these existing PM2.5 monitors to expand the limited air quality dataset in the Global South. The mean ambient BC concentrations at these locations tend to be very high (up to daily average of 80 μg m−3) compared to that in developed nations (<1 μg m−3).32–37 Our long-term goal is to use these BAM tapes to extract BC concentrations. To achieve this goal, we collected PM2.5 on 51 glass-fiber filters, designed a custom color-coded reference card, captured photos of sampled filters on the reference card, developed an image-processing algorithm to extract Red channel values (R) from the filter images and trained an R to BC model with the measured R values and BC concentrations for the 51 filter samples. Particulate loadings for each filter sample varied and BC concentrations were measured with an aethalometer (BCAeth). We evaluated the trained model at two EPA Chemical Speciation Network (CSN) urban sites in Pittsburgh by comparing daily EC measurements with daily averaged BAM-based BCs estimated with our model. We empirically defined a minimum detection limit for the method to assess its applicability in various sampling environments. This work also investigates the possibility to quantify wood smoke BC by utilizing color channels of filter samples from varied sources, mainly wood smoke, diesel and ambient.
2 Methods
2.1 Particle sampling setup
We collected ambient aerosol samples to test the image reflectance-based method. Samples were collected at the Carnegie Mellon University (CMU) campus (Fig. S1†). This is an urban background location that is far from major roadways or other local BC sources. The nearest arterial road from the sampling point is ∼225 m away.
Fig. 1 shows the sampling setup. A wire mesh enveloped the inlet to avoid macro contaminants or insects from entering the inlet. The ambient air passed through a stainless-steel PM2.5 cyclone (2.5 μm at 92 LPM, URG-2000-30EP, URG Corporation) to allow only PM2.5. Most of the freshly emitted combustion-based BC falls in this size range.38 The air through the cyclone branched into four lines; an aethalometer (AE-31, Magee Scientific) measured BC concentration (μg m−3) as a reference monitor, and two lines parallelly collected PM2.5 on a 47 mm glass-fiber filter (A/E Glass Fiber Filter, Pall Corporation) and 47 mm quartz-fiber filters (2500 QAO-UP, Pall Corporation), both at a flow rate of 16.67 LPM (1 m3 h−1). The remaining line was to draw excess air, making cumulative flow through the cyclone to 92 LPM for a cutoff diameter of 2.5 μm. The parallel sampling on the two filter types was done to compare the effect of compositional differences between glass filters used in BAMs and conventionally used quartz filters on image-processing performance (Fig. S3†).
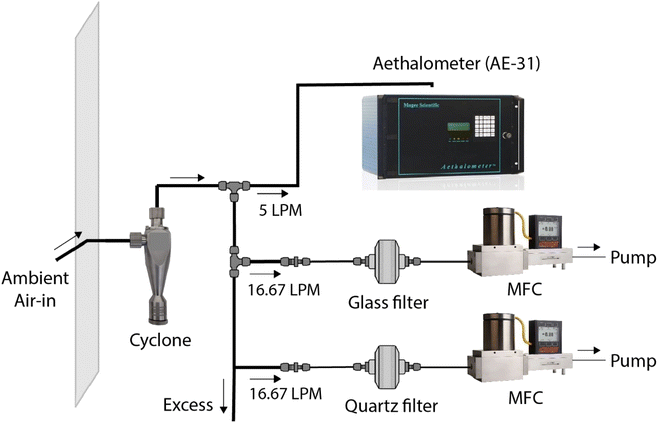 |
| Fig. 1 Sampling set-up used for collecting ambient particulates on glass and quartz-fiber filters. An aethalometer (AE-31) parallelly measured BC for training filter R to BC model and to validate image-based BC. A cyclone was used at the collective inlet to allow only PM2.5 during sampling. | |
The flows for filter samples were maintained with mass flow controllers (MCR-50SLPM-D, Alicat Scientific, Inc.), each attached to a diaphragm pump for a pressure source. Filter holders (Gelman Sciences 2220 47 mm Stainless Steel Filter Holder, Gelman Sciences, Inc.) were used to support the filters and ensure a uniform PM loading during sampling. The setup used copper tubing (3/8′′) to avoid particle loss by sticking to the tubing wall surface. We collected 7 sets of quartz- and glass-fiber filters parallelly and 44 additional glass-fiber filters. The hourly ambient BC level varied between 0.03–3.77 μg m−3 for our sampling location. Since the image reflectance method measures area concentration (μg cm−2) on filters, we maintained the flow rate of 1 m3 h−1 and changed sampling durations to achieve BC filter loadings between 0–16 μg cm−2. This range of surface concentrations corresponds to ambient concentrations of 0–15.2 μg m−3 for one-hour samples collected by BAMs with a spot size of 0.95 cm2. The 51 glass-fiber filter samples were used for training the R to BC model, where R is red scale values for a filter sample that takes integer values between 0 to 255, and BC represents area loading of black carbon (μg cm−2) in the filter sample.
Filter-based light attenuation techniques face challenges due to continuous particle loading on the same spot and multiple scattering of light rays. The AE-31 tends to underestimate BC concentrations as the filter tape gradually becomes loaded with particles, a phenomenon referred to as the “shadowing effect”, that is prominently observed in experiments with high concentrations of freshly emitted soot.39 We applied appropriate loading corrections to the raw BC concentrations from AE-31 and the corrected BC is referred to as BCAeth in this article.40
Subramanian et al. reported that filter-based optical BC measurements can experience errors due to aerosol emissions from smoldering biomass burning or other sources of liquid organic matter.41 Therefore, we compared BCAeth with EC for 7 quartz fiber filter samples to evaluate the performance of the aethalometer (Fig. S2†). EC was quantified using the Interagency Monitoring of PROtected Visual Environments (IMPROVE)-A protocol. Fig. S2† shows a high correlation between BC and EC (R2 > 0.99), as expected, and EC concentrations were ∼13% higher than BC for these filters sampled at CMU.
BCAeth was used as reference BC concentration for all 51 training filters to ensure readily available data at high time resolution and to avoid always relying on time consuming thermo-optical methods for reference measurements. The aethalometer is used as ground truth for our models due to its wide applicability in continuous monitoring of BC in ambient environments.
This study measures area concentration (μg cm−2) of BC on filter surfaces using a reflectance-based method, whereas particle concentrations are typically reported as volume concentration (μg m−3). We used CA = CVQTS/AF for converting one to another, where CA is mass of BC per unit area of the filter surface and CV is the concentration of BC in the ambient air. The sampling was done at a flow rate of Q (1 m3 h−1) for TS hours on filters with collection area AF (9.6 cm2 for 47 mm filters).
2.2 Image processing
The image reflectance method relies on cell phone camera photos of each filter to determine BC concentrations. The filters are placed on a reference card (described in section 2.2.1) for each photo. A camera captures the reflected light from an object in the form of a photograph. A photo is composed of pixels and each pixel is a combination of RGB channels. We aim to determine BC concentration of a filter sample by extracting the averaged Red channel of all pixels of the sampled area in the photo. This approach requires that we first perform geometric and color calibration of the original image.
The raw images of filter samples undergo geometric calibration to correct for image distortion from the nature of the lens in the camera during photo capture. Cameras generally exhibit some form of lens distortion, especially radial distortion.42 Geometric calibration is followed by color calibration to correct for the effects of lighting conditions and scale the RGB channels of the resulting image as close as possible to a common reference.
2.2.1 Reference card.
We prepared a reference card with twelve grayscale values, each with Red–Green–Blue (RGB) color channel values between [0, 0, 0] and [255, 255, 255] printed on a matte finish photo paper (Fig. 2a). These grayscales are present on the card in duplicates (a total of 24) for a better-averaged extraction of RGB scales from each grayscale box. A black-fill square encompassing a white circular zone at the center of the card is for placing filter samples. There are two sets of three colored boxes to aid in color calibration; each box consists of different RGB channel combinations. All the grayscale and colored squares were given black ([0, 0, 0]) boundaries for added advantage in contour detection (Fig. S6a†).
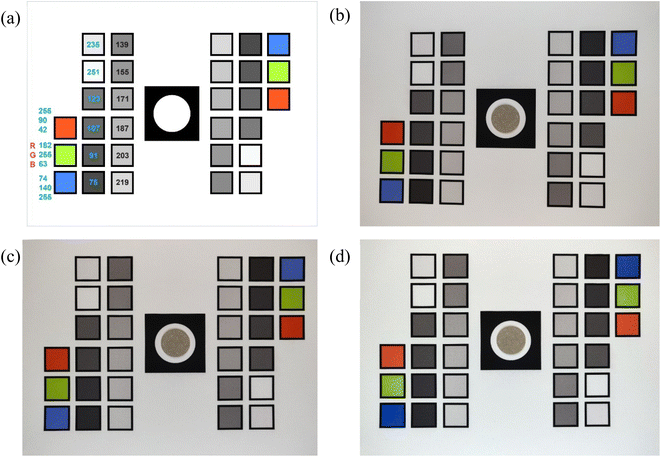 |
| Fig. 2 The reference card used in capturing filter sample images is shown here. Particulate deposit spots are placed at the center circle. (a) Red, green and blue (RGB) color channels are shown for all the square boxes. The 3 colored boxes in the reference card are used for color calibration, whereas the Red channel of each grayscale box (R values for the gray boxes are 75, 91, 107, 123, 139, 155, 171, 187, 203, 219, 235, 251) corresponds to a unique BC concentration (μg cm−2). The card contains a duplicate set of the colored and grayscale boxes for an improved RGB estimation through averaging. (b) A raw image with one of the calibration filters placed at the center of the calibration card. (c) The same image after geometric correction (d) The image after geometric correction and color calibration, which is used for extraction of RGB channels for the squares and filter samples. | |
In this study, we used the abovementioned reference card to capture images of filters (Section S1, ESI†). The filters were placed on the reference card one at a time and a photo was captured using a cell phone camera (OnePlus 6, OnePlus Technology Co., Ltd., China), fixed at ∼9′′ above the card. Images were taken in a dark room under uniform diffused lighting established with two ring lights with a fixed light intensity (Fig. S5†).
2.2.2 Geometric and color calibrations.
The extraction of RGB channels of a raw image was executed with the use of a custom python script. The full code is available here (https://github.com/eloyjaws/filter_extraction). The pipeline for filter sample RGB extraction used traditional computer vision algorithms from OpenCV and can be broken down into two stages: geometric calibration of the input image and color calibration of the geometrically calibrated input image. The pipeline was designed to use the reference card (Fig. 2a) as a template in both stages.
2.2.2.1 Geometric calibration.
Camera images of a horizontally aligned filter and reference card assembly usually have image distortion. We ran a series of transformations on the raw input images to correct for distortion, translation, or rotation errors. To geometrically calibrate the image, we resized the input image to a fixed resolution and aspect ratio. We then ran a computer vision algorithm called SIFT (Scale-Invariant Feature Transform) on both the resized input image and the reference card image (a digital template designed in Adobe Illustrator) to extract points of interest that are not only invariant to the image scale and rotation but are also robust to changes in illumination, noise and minor changes in viewpoint.43 We call these extracted points SIFT features. We used a feature matcher algorithm from OpenCV to find and match the SIFT features extracted from our resized input image with the reference image.44 After extracting these matches, we selected the optimum matches by applying Lowe's ratio test.45 We computed a homography matrix (a 3 × 3 matrix that maps the transformation between the two image planes) through a robust estimation technique called Random Sample Consensus (RANSAC) using key points from the images that were highlighted in our optimum matches.46 With the resulting homography matrix, a transformation was applied to all pixels in our resized input image to map it to the reference. Any distortion, scale, or rotation differences in the input image were corrected by warping the perspective of the resized input image to the perspective of the reference image by using the homography matrix. The geometrically calibrated image of Fig. 2b, which shows a slight distortion, is shown in Fig. 2c.
2.2.2.2 Color calibration.
Once the input image was geometrically aligned with the reference image, the locations of the colored boxes were used to extract their RGB channels for color calibrations. Color calibration helps to minimize effects on extracted RGB values due to variations in lighting conditions in photo-capturing environments. Fig. 2a shows predesignated set of RGB values of colored boxes in the reference card. The color calibration uses known RGB values from these squares in the reference image to scale RGB for the entire input image. To accomplish this, we extracted the RGB values of all 30 boxes from the geometrically calibrated input image (Fig. 2c) and the reference image (Fig. 2a). We then normalize the RGB values from the geometrically calibrated input image (target colors) to have a value between 0 and 1. We use a piece-wise function “eotf_sRGB” (sRGB electro-optical transfer function) to decode the normalized values that can represent the image in a linear color space.47 ‘Finlayson 2015’ method was used for color correction to learn a function f that maps target colors to reference colors.48 We used the function f to color-correct the target image. The average RGB of the extracted pixels in the filter sample area (Fig. S6b†) represents the geometrically and color corrected color channels of the sample.
3 Results and discussion
3.1 Red scale to BC algorithm
The red scale value of a sample is an indicator of the attenuation of visible red light through the sample. Light attenuation through the atmosphere is exponential and can be explained by the Beer–Lambert law. Existing filter-based BC and PM instruments follow this exponential optical extinction principle to measure mass concentrations.22,49 Therefore, we expect an exponential relationship between red light reflectance and BC area concentration on a filter sample in the form:
BC(μg cm−2) = a × e(b×R) + c |
where R is the averaged Red scale value of a particle spot on a filter, a (μg cm−2), b (unitless) and c (μg cm−2) are coefficients of the exponential curve.
Nonetheless, we tested a set of R to BC (μg cm−2) calibration models to investigate for the best-performing model. Linear, polynomial and logarithmic models are commonly used regression models. Additionally, gradient boosting, random forest, ridge and support vector machines are machine learning models widely used in air quality research recently. Ensemble methods try to improve accuracy by combining predictive performances of different machine learning models. We also explored a hybrid model to exploit the fairly linear correlation of BC with R on lower BC concentrations. This hybrid model consisted of a linear part for low BC values and an exponential curve to explain the dependence of high BC range on R.
We used R2, mean absolute error (MAE) and root mean square error (RMSE) to assess model performance. Table 1 summarizes the performance of all trained models, and the training data are shown in Fig. 3 and Table S2.† All models in Table 1 were evaluated with random 4-fold cross-validation. While many of the models showed similar performance, the exponential model seems to be the simplest model with the best performance. Thus, we used this model; the model fit and parameters are shown in Fig. 3.
Table 1 Red scale to BC concentration model performance. R2, MAE and RMSE are used as metrics for assessment. All metrics represent an average of 4-fold cross-validation. For models tested over subsets of concentration, “low BC” corresponds to BC concentrations below a threshold BC (BCth, μg cm2), and “high BC” corresponds to concentrations ≥ BCth μg cm2. BCth for each model is calculated separately to maximize model performance. The BCth calculation is discussed in Section S3 of the ESI
Models |
R
2
|
MAE |
RMSE |
Linear regression |
Low BC |
0.95 |
0.09 |
0.11 |
High BC |
0.89 |
0.82 |
1.05 |
All |
0.79 |
0.76 |
1.08 |
Polynomial regression |
Low BC |
0.93 |
0.10 |
0.11 |
High BC |
0.88 |
0.81 |
0.99 |
All |
0.90 |
0.55 |
0.79 |
Exponential |
Low BC |
0.93 |
0.11 |
0.13 |
High BC |
0.91 |
0.77 |
1.00 |
All |
0.94 |
0.59 |
0.86 |
Hybrid |
Low BC–linear |
0.95 |
0.09 |
0.11 |
High BC – exponential (using all 51 data points) |
0.91 |
0.74 |
0.98 |
All |
0.94 |
0.59 |
0.85 |
Logarithmic |
0.93 |
0.70 |
0.95 |
Gradient boosting |
0.82 |
0.73 |
0.99 |
Random forests |
0.81 |
0.83 |
1.09 |
Support vector machines (SVMs) |
0.64 |
1.04 |
1.78 |
Ensemble |
0.79 |
0.76 |
1.16 |
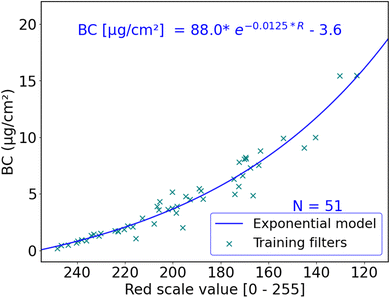 |
| Fig. 3 The solid blue line represents a fitted exponential model for sample Red scale to BC concentration. The crosses are calibration filters (51 glass-fiber filter samples) used for fitting the model. BC values for calibration are measured with an aethalometer. | |
A cell phone is used in our experiments due to its easy availability. A lower resolution camera such as a webcam is equally suited for the task as this method requires an average of only a few pixels of evenly deposited particles on a filter to quantify BC concentrations.
We investigated the effects of lighting conditions on estimation of BC concentration with the image reflectance method for a filter sample (Section S1†). BC concentrations for two filters, with BC loadings of 1.725 μg cm−2 and 8.089 μg cm−2, were calculated with the R to BC model at five light intensities from very dim (level 6) to very bright (level 10) lighting during image capture (Table S1†). We observed a maximum increase of only 1.2% in R and a 3.8% decline in BC compared to those in the reference light settings. Thus, the same model can work for quantifying BC concentrations for an unknown sample in a wide range of lighting conditions.
The method relies on Red channel values hence the detection limit is controlled by the maximum value of the Red channel, which is 255 (no red light intensity). Red channel values are reported with integer resolution. Thus, we can use the exponential model for a Red channel value of 254 to determine an effective detection limit (EDL) of 0.08 μg cm2 for the image reflectance method.
Converting this surface concentration to the corresponding hourly BC volume concentration depends on the model of BAM. Different models will have varying sampling flow rates and filter loading areas. The two most commonly used research-grade BAMs are BAM-1020 (Continuous Particulate Monitor BAM 1020, Met One Instruments Inc.) and BAM-5014i (Beta Continuous Ambient Particulate Monitor, Thermo Fisher Scientific Inc.) with particulate loading filter spot area of 0.95 cm2 and 2.01 cm2, respectively. Both instruments sample at a flow rate of 1 m3 h−1. The image-based approach can therefore detect BC concentrations of 0.07 μg m−3 for the Met One BAM and 0.15 μg m−3 for the Thermo Fisher BAM at an hourly resolution.
Our method relies on red light reflected from the sample spot. It is possible that under very high filter loadings, BC could accumulate into such a dark spot that R = 0 and additional material does not change the absorbance. Such a situation is unlikely under ambient conditions but could be encountered if this method is used to quantify BC for direct emissions testing from combustion sources. We illustrate our performance up to an hourly BC concentration of 15 μg m−3 (and a filter loading of 15.8 μg cm−2 for BAM 1020). This is an extremely high hourly BC concentration for an ambient environment, even for highly polluted ambient environments. Therefore, we do not expect to encounter a ‘maximum’ detection limit for typical ambient conditions.
We can compare the EDL to measurements of ambient BC. Fig. 4 shows a histogram of one year of hourly BC concentrations measured with an aethalometer at a near-road site in Pittsburgh, Pennsylvania, USA from August 2020–July 2021. The median for the BC histogram is 0.67 μg m−3. The measured BC at this site is above the EDL for >98% of all hours. BC concentrations in many developing nations are higher (Fig. S8†), indicating that this approach will be able to determine hourly BC concentrations from BAM tapes in many locations worldwide.
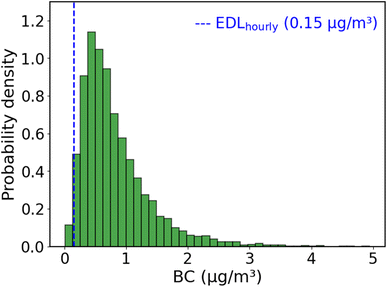 |
| Fig. 4 The histogram plot shows the probability distribution curve of hourly BC for a near-road site (AQS ID: 42-003-1376, latitude: 40.437430 and longitude: −79.863572) in Pittsburgh, Pennsylvania (USA). The vertical blue dashed line represents the EDL of the image reflectance method. The monitoring station is a roadside site and BC data is measured with an aethalometer, sampled between August 2020 to July 2021. The hourly BC concentration was higher than the EDL (0.15 μg m−3) more than 98% of the time. | |
We use the RMSE of R to BC model for low BC levels (below 1.66 μg m−3, or R > 224) to estimate the uncertainty of the method to be around 0.1 μg m−3 at the typical ambient BC concentrations shown in Fig. 4.
3.2 Validation of BAM estimated BC with EC at CSN sites
In this section, we apply the calibration model to BAM tapes collected at two EPA monitoring sites in Pittsburgh that have co-located filter measurements for thermal-optical EC. The Lawrenceville site (AQS ID: 42-003-0008, latitude: 40.465420 and longitude: −79.960757) is an urban background site. It is in a city neighborhood with a mix of residential and commercial land use. The Liberty site (AQS ID: 42-003-0064, latitude: 40.323761 and longitude: −79.868151) is located 2.65 km from the largest metallurgical coke plant in North America. Both sites are CSN sites with 1-in-3 days (Lawrenceville) and 1-in-6 days (Liberty) 24 h EC filter measurements.
We obtained BAM tapes from 12 July, 2020 to 6 September, 2020 for Lawrenceville and 10 March, 2020 to 29 May, 2020 for Liberty. We used the image reflectance method to determine hourly BC concentrations (BC-OPT) at each location. For days with concurrent filter sampling, we compared the daily mean BC-OPT to the filter EC (EC-CSN) at each site (Lawrenceville: 19 days; Liberty: 13 days). The comparison is shown as a box plot in Fig. 5. Daily BC-OPT estimates and EC-CSN for both sites are listed in Table S3.†
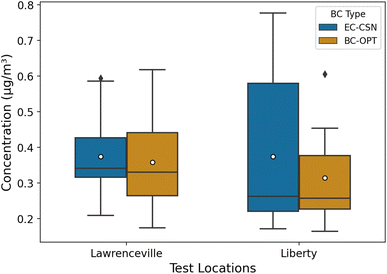 |
| Fig. 5 The boxplot is a comparison of the daily average BC-OPT with EC-CSN in Pittsburgh, Pennsylvania. Lawrenceville CSN site (AQS ID: 42-003-0008) measures EC 1-in-3 days, whereas the frequency is every 6th day in Liberty (AQS ID: 42-003-0064). In this plot, we compare daily average BC values at the two sites for 19 and 13 days, respectively. The boxes show the interquartile range and whiskers on the two ends extend to 1.5 times the interquartile range. The data points outside the whiskers are considered outliers. The white dots in the boxes represent mean values. | |
Overall, concentrations at both locations were typical of urban BC concentrations in the US. Mean (median) EC concentrations were ∼0.37 (0.34) μg m−3 for Lawrenceville and ∼0.37 (0.26) μg m−3 for Liberty. The mean concentration at Lawrenceville is similar to average background BC concentrations in Pittsburgh measured during mobile sampling between similar months (August–September) of 2016.50
The mean and median BC-OPT for Lawrenceville (mean: 0.35 μg m−3, median: 0.32 μg m−3) and Liberty (mean: 0.30 μg m−3, median: 0.24 μg m−3) agree well with EC-CSN. At Lawrenceville, the BC-OPT has a larger interquartile range (IQR). The IQR is 0.11 μg m−3 for the CSN EC and 0.19 μg m−3 for the optical method. Overall, the optical BC compares favorably with the EC measurements for this urban site.
At Liberty, the 25th percentile and medians agree well for the two methods, but the 75th percentile for the EC-CSN (0.58 μg m−3) is larger than for the optical method (0.38 μg m−3). This may be a result of the different measurement methods (optical for BC and thermal-optical for EC).51 Fig. S7† shows that while BC and EC are correlated at Liberty, the image reflectance-based BC is consistently lower when EC is above 0.5 μg m−3. This disparity in EC and BC-OPT is reflected in our quartz training filters (Fig. S2†). In those filters, EC was systematically higher than BC-OPT, with larger differences at high concentrations.
While some of the differences between BC-OPT and EC at Liberty might be due to methodological differences, the source of BC may also play a role. High BC (EC) concentrations both at Liberty and at the CMU site are often associated with industrial emissions from a metallurgical coke works located 3 km south of the Liberty site.52 If these industrial emissions have a different EC-to-BC ratio than typical traffic-dominated urban emissions, that could explain the poorer agreement at Liberty than at Lawrenceville or CMU.
3.3 Color channel correlation for samples from different sources
This section focuses on quantifying wood smoke BC from the filter spots. Previous studies have used the difference in BC at 370 nm (ultraviolet) and 880 nm (infrared) as a tracer for wood smoke. We hypothesized that data from multiple color channels from sample photographs could be used to differentiate between wood smoke BC and that from other sources.
Fig. 6 and Table S4† compare Red, Blue and Green channel values for filter samples from three sources: wood smoke, diesel-dominated traffic and ambient. The ambient filters are the same samples used for model calibration. The BC on these filters is likely dominated by traffic emissions, primarily from diesel vehicles. Subramanian et al.53 attributed >80% of BC in Pittsburgh to traffic sources. Robinson et al. further showed that Pittsburgh has minor impacts from biomass burning emissions.54
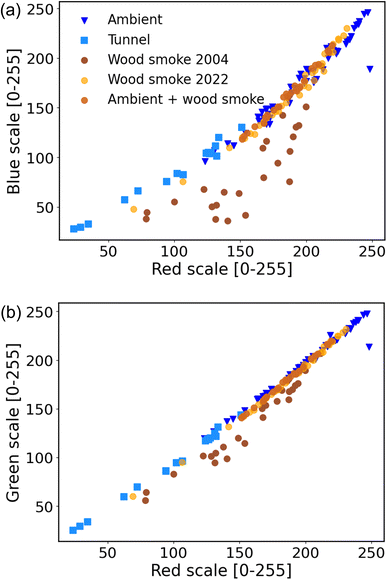 |
| Fig. 6 (a) B to R plots and (b) G to R plots for ambient, diesel and woodsmoke sources. Scatter points represent 156 individual samples. ‘Ambient’ samples (51) are the samples used for training R to BC models. ‘Tunnel’ samples (17) were collected inside the Squirrel Hill tunnel in Pittsburgh in 2004. ‘Wood smoke 2004’ (26) and ‘Wood smoke 2022’ (35) are wood smoke samples collected from direct wood burn experiments performed in the CMU laboratory in 2004 and 2022, respectively. ‘Ambient + wood smoke’ samples (27) are filters with wood burn emissions deposited over ambient samples. | |
The diesel-dominated samples were collected in the Squirrel Hill tunnel in Pittsburgh, Pennsylvania. Sampling details for the ‘Tunnel’ filters can be found in Grieshop et al.55 Briefly, the tunnel traffic is mostly comprised of heavy-duty diesel vehicles (HDDVs) and light-duty gasoline vehicles (LDVs). Grieshop et al. found that EC emission factor for HDDVs in the tunnel was up to 20 times larger than that for the LDVs, suggesting a diesel emission-dominated environment.55
The wood smoke filters were sampled by collecting direct emissions from controlled burning of wood logs. The wood smoke sampling setup is explained in Section S4 and Fig. S9 of the ESI.† ‘Wood smoke 2004’ filters were collected by Lipsky et al. in 2004.56 These samples were stored in a freezer since then. ‘Wood smoke 2022’ are freshly collected filters sampled in 2022.
Lastly, we generated filter samples that contained ambient PM ‘doped’ with wood smoke. These ‘Ambient + wood smoke’ samples are a subset of the ambient filters used for calibration (e.g., Fig. 2) with additional wood smoke sampled after the initial analysis. These filters should therefore represent a mix of diesel and wood smoke emissions.
For nearly all of the samples, there is a high correlation between both the Red and Blue channels (R2 ∼ 0.98 for a quadratic polynomial) and Red and Green channels (R2 ∼ 0.99 and gradient ∼1.03 for a linear model). Samples from different sources (ambient, tunnel and wood smoke) seem to fall along a common curve in both Red–Blue (Fig. 6a) and Red–Green (Fig. 6b) plots.
The exception is the ‘Wood smoke 2004’ samples. These samples have excess absorption of both Blue and Green light wavelengths. This excess absorption may be due to the presence of light-absorbing brown carbon formed during smoldering combustion.57 The wood smoke samples from 2022 were dominated by flaming combustion and therefore had minimal emissions of brown carbon. The excess absorption of Blue and Green light is not observed in the freshly collected ‘Wood smoke 2022’ samples nor in the ambient samples doped with wood smoke. The excess Blue and Green absorption for the 2004 samples might also be due to artifacts introduced to these samples due to aging of particle deposits during storage.
Our results suggest that excess absorption in the Blue and Green channels might be useful as a qualitative indicator for the presence of BC from biomass burning. However, since flaming combustion produces minimal brown carbon, samples dominated by flaming biomass combustion will not have excess absorption in the Blue and Green channels. Better separation of wood smoke BC from other sources, such as diesel, will require further investigation under a variety of biomass burning conditions.
4 Conclusion
Air quality monitoring in the Global South is hampered by the high cost of equipment. This paper presents a low-cost method to leverage existing measurements to extract PM2.5 composition data that can in turn be used to better understand PM sources and inform future policies. BAMs are deployed worldwide to quantify PM2.5 concentrations with hourly time resolution; for example, the US State Department has deployed BAMs at 76 embassies and consulates worldwide. Post-analysis of the BAM filter tapes using cell phone camera images can provide valuable information on BC concentrations with hourly time resolution.
The approach shown here has an effective minimum detection limit of 0.15 μg m−3. As we show in Fig. 4, this is sufficient to detect hourly BC at a roadside location in the US, and therefore the method should be widely applicable to locations worldwide.
There are a few key advantages to the approach outlined here. First, it leverages existing samples. Second, it is extremely low cost. Cell phone cameras are ubiquitous, and no special or expensive equipment is needed to collect the photographs; a lower resolution camera such as a webcam is could also be used in place of the cell phone camera. Third, all code is available freely online. Lastly, this method can provide high time resolution data on a PM component. One potential drawback to our approach is that BC detection is not achieved in real time. Instead, the BAM filter tapes can only be photographed after the entire filter tape has been used and removed from the BAM. Since filters last approximately 2–3 months, this can create a delay between data collection and analysis.
Conflicts of interest
There are no conflicts to declare.
Acknowledgements
This work was funded by a grant from the Wilton E. Scott Institute at Carnegie Mellon University and the US National Science Foundation (Award #2020666). We thank David Good and David Cobb for providing the BAM tapes for the Pittsburgh sites.
References
- B. Chen and H. Kan, Air Pollution and Population Health: A Global Challenge, Environ. Health Prev. Med., 2008, 13(2), 94–101, DOI:10.1007/S12199-007-0018-5.
- J. Lelieveld, J. S. Evans, M. Fnais, D. Giannadaki and A. Pozzer, The Contribution of Outdoor Air Pollution Sources to Premature Mortality on a Global Scale, Nature, 2015, 525(7569), 367–371, DOI:10.1038/nature15371.
- J. Zhang and K. R. Smith, Indoor Air Pollution: A Global Health Concern, Br. Med. Bull., 2003, 68(1), 209–225, DOI:10.1093/BMB/LDG029.
- M. H. Forouzanfar, L. Alexander, V. F. Bachman, S. Biryukov, M. Brauer, D. Casey, M. M. Coates, K. Delwiche, K. Estep, J. J. Frostad, K. C. Astha, H. H. Kyu, M. Moradi-Lakeh, M. Ng, E. Slepak, B. A. Thomas, J. Wagner, T. Achoki, C. Atkinson, R. M. Barber, K. Cooperrider, L. Dandona, D. Dicker, A. D. Flaxman, T. D. Fleming, K. J. Foreman, E. Gakidou, S. I. Hay, K. R. Heuton, M. L. Iannarone, T. Ku, H. J. Larson, S. S. Lim, A. D. Lopez, R. Lozano, M. F. MacIntyre, C. Margono, A. McLain, A. H. Mokdad, E. C. Mullany, C. J. L. Murray, M. Naghavi, G. Nguyen, A. W. Pain, L. Richardson, M. Robinson, L. Sandar, N. Stephens, A. M. Temesgen, B. Thomson, T. Vos, X. Wan, H. Wang, B. Wurtz, B. E. Ebel, R. G. Ellenbogen, J. L. Wright, R. Alfonso-Cristancho, B. O. Anderson, P. N. Jensen, D. A. Quistberg, A. Riederer, M. S. Vavilala, J. R. Zunt, H. R. Anderson, F. Pourmalek, C. C. Gotay, R. Burnett, H. H. Shin, S. Weichenthal, A. Cohen, A. Knudsen, G. Aasvang, J. M. Kinge, V. Skirbekk, S. Vollset, C. Abbafati, A. Abbasoglu Ozgoren, A. Çavlin, B. Kucuk Bicer, F. Abd-Allah, S. F. Abera, Y. A. Melaku, V. Aboyans, B. Abraham, J. Puthenpurakal Abraham, J. P. Abraham, A. L. Thorne-Lyman, E. L. Ding, S. Fahimi, S. Khatibzadeh, G. R. Wagner, G. Bukhman, I. R. Campos-Nonato, A. B. Feigl, J. A. Salomon, H. Benzian, I. Abubakar, N. M. E. Abu-Rmeileh, T. C. Aburto, M. A. Avila, S. Barquera, T. Barrientos-Gutierrez, J. C. Campuzano, A. J. Cantoral, A. G. Contreras, L. Cuevas-Nasu, V. De, F. A. García-Guerra, H. Gomez Dantes, T. Gonzalez de Cosio, D. González-Castell, I. B. Heredia-Pi, L. Hernandez, A. Jauregui, C. Medina, F. Mejia-Rodriguez, M. J. C. Hernandez, L. S. Pedraza, A. Pedroza, A. D. Quezada, D. Salvo, L. M. Sanchez, T. G. Sánchez-Pimienta, E. E. Servan-Mori, T. Shamah Levy, T. M. M. Rojo, S. Villalpando, A. Adelekan, K. Adofo, A. K. Adou, J. C. Adsuar, U. Fra Paleo, A. Afshin, R. Micha, D. Mozaffarian, S. Shahraz, S. Shangguan, G. M. Singh, E. E. Agardh, A. M. J. Khabouri, A. F. H. Lami, S. Alam, A. Naheed, D. Alasfoor, M. I. Albittar, M. A. Alegretti, F. Cavalleri, A. V. Aleman, V. Colistro, Z. A. Alemu, S. Alhabib, Z. Chen, P. Gething, R. Ali, D. A. Bennett, A. D. M. Briggs, K. Rahimi, P. Scarborough, E. P. Simard, M. K. Ali, S. Argeseanu Cunningham, Y. Liu, K. M. V. Narayan, S. B. Omer, F. Alla, F. Guillemin, P. Allebeck, N. Roy, M. Kivipelto, E. Weiderpass, S. Fereshtehnejad, R. Havmoeller, S. Sindi, P. J. Allen, U. Alsharif, M. Endres, S. Nolte, C. Papachristou, E. Alvarez, N. Alvis-Guzman, P. A. J. Caicedo, A. A. Amankwaa, A. T. Amare, H. W. Hoek, R. T. Gansevoort, M. Yenesew, E. A. Ameh, O. Ameli, H. Amini, M. Tanner, W. Ammar, H. L. Harb, C. A. T. Antonio, E. A. Faraon, C. A. Panelo, P. Anwari, J. Arnlöv, A. Larsson, A. V. S. Arsenijevic, A. Artaman, R. J. Asghar, R. Assadi, L. S. Atkins, B. Awuah, D. O. Laryea, A. Badawi, M. C. Bahit, T. Bakfalouni, K. Balakrishnan, S. Balalla, V. L. Feigin, T. B. J. Ao, R. Balu, R. Dandona, S. Goenka, G. Kumar, K. S. Murthy, K. Reddy, A. Banerjee, S. L. Barker-Collo, B. del Pozo-Cruz, L. Barregard, L. H. Barrero, A. C. Basto-Abreu, C. Batis Ruvalcaba, E. F. De Castro, N. Lopez, J. L. Texcalac, A. Basu, L. Gaffikin, S. Basu, M. O. Basulaiman, Z. A. Memish, J. Beardsley, N. Bedi, T. Bekele, M. L. Bell, J. J. Huang, C. Benjet, G. Borges, R. A. Gutiérrez, R. Orozco, L. Trasande, H. Hagan, E. Bernabé, C. D. A. Wolfe, T. J. Beyene, N. Bhala, S. Derrett, A. Bhalla, V. Jha, Z. A. Bhutta, M. I. Nisar, B. Bikbov, B. A. A. Abdulhak, L. Vijayakumar, P. P. Chiang, J. D. Blore, P. M. Brooks, A. Lakshmana Balaji, S. M. Colquhoun, R. G. Weintraub, F. M. Blyth, A. Meretoja, M. A. Bohensky, B. Bora Basara, G. K. Yentür, M. R. Kose, A. Pekericli, S. B. Uzun, N. M. Bornstein, D. Bose, S. Boufous, L. Degenhardt, R. R. Bourne, M. Brainin, A. Brazinova, M. Majdan, N. J. Breitborde, B. Schöttker, H. Brenner, D. M. Broday, R. Lunevicius, N. G. Bruce, M. K. Dherani, D. Pope, T. S. Brugha, B. Brunekreef, H. Kromhout, R. Buchbinder, B. Gabbe, K. B. Gibney, A. G. Thrift, L. N. Bui, N. T. Nguyen, A. G. Bulloch, S. B. Patten, M. Tonelli, J. Wang, M. Burch, P. G. J. Burney, D. L. Jarvis, A. Rodriguez, L. Rushton, M. Soljak, T. N. Williams, J. Caravanos, D. Nash, R. Cárdenas, E. Cardis, M. J. Nieuwenhuijsen, D. Rojas-Rueda, D. O. Carpenter, R. Leung, V. Caso, C. A. Castañeda-Orjuela, R. E. Castro, F. Catalá-López, V. K. Chadha, J. Chang, J. G. Scott, D. G. Hoy, L. D. Knibbs, F. J. Charlson, H. E. Erskine, A. J. Ferrari, H. N. Gouda, L. J. Veerman, H. A. Whiteford, W. Chen, X. Zou, H. Chen, S. J. London, Y. Jiang, K. Takahashi, O. Chimed-Ochir, R. Chowdhury, J. Powles, C. A. Christophi, T. Chuang, S. S. Chugh, M. Cirillo, T. K. D. Claßen, A. Kraemer, M. Tobollik, M. Colomar, C. Cooper, L. T. Cooper, J. Coresh, K. Matsushita, B. X. Tran, K. J. Courville, M. H. Criqui, M. B. Stein, J. Damsere-Derry, H. Danawi, A. H. Refaat, P. I. Dargan, A. Davis, D. F. J. Fay, J. C. Schmidt, D. V. Davitoiu, A. Dayama, D. DeLeo, G. de Lima, V. M. P. Machado, J. R. Nogueira, C. M. Teixeira, R. P. Dellavalle, K. Deribe, W. Mekonnen, D. C. Jarlais, M. Dessalegn, G. A. DeVeber, M. P. Lindsay, H. Hu, K. M. Devries, M. McKee, N. Pearce, H. Stöckl, T. Tillmann, C. H. Watts, S. D. Dharmaratne, K. Dokova, E. R. Dorsey, T. R. Driscoll, G. B. Marks, J. Leigh, L. Duan, Y. Li, S. Liu, J. Ma, L. Wang, P. Ye, M. Zhou, X. Liang, A. M. Durrani, Y. M. Elshrek, S. P. Ermakov, S. Soshnikov, B. Eshrati, F. Farzadfar, A. Esteghamati, N. Hafezi-Nejad, S. Sheikhbahaei, S. G. Sepanlou, P. Heydarpour, M. Sahraian, V. Rahimi-Movaghar, C. P. Ferri, N. Foigt, R. C. Franklin, A. Gamkrelidze, I. Khonelidze, L. Sturua, F. G. Gankpé, E. Gasana, N. Sabin, J. M. Geleijnse, B. D. Gessner, R. F. Gillum, I. A. M. Ginawi, M. Giroud, G. Giussani, K. Goginashvili, P. Gona, A. Goto, R. L. Guerrant, A. S. Terkawi, H. C. Gugnani, D. Gunnell, R. Gupta, M. Hagstromer, Y. A. Halasa, B. T. Idrisov, R. R. Hamadeh, M. Hammami, G. J. Hankey, Y. Hao, Y. Zheng, T. Haregu, S. van de Vijver, J. Haro, M. T. Hedayati, M. Hijar, H. J. Hoffman, G. A. Mensah, U. K. Sampson, J. C. Hornberger, H. Hosgood, M. Hsairi, G. Hu, C. Huang, B. J. Hubbell, L. Huiart, L. Racapé, A. Husseini, K. M. Iburg, N. Ikeda, K. Innos, M. Inoue, N. Kawakami, K. Shibuya, F. Islami, S. Ismayilova, K. H. Jacobsen, H. A. Jansen, S. K. Jassal, S. Jayaraman, P. Jeemon, D. Prabhakaran, F. Jiang, G. Jiang, M. R. Phillips, J. B. Jonas, K. Juel, J. She, H. Kan, K. S. S. Roseline, N. E. Karam, A. Karch, C. K. Karema, G. Karthikeyan, V. K. Paul, M. Satpathy, N. Tandon, A. Kaul, D. S. Kazi, A. H. Kemp, P. A. Lotufo, G. V. Polanczyk, I. S. Santos, A. P. Kengne, R. Matzopoulos, C. D. Parry, K. Sliwa, B. M. Mayosi, D. J. Stein, A. Keren, Y. S. Khader, S. E. Ali Hassan Khalifa, E. A. Khan, Y. Khang, C. Kieling, D. Kim, S. Kim, Y. Kim, R. W. Kimokoti, Y. Kinfu, B. M. Kissela, Y. Kokubo, S. Kosen, T. S. Warouw, M. Kravchenko, Y. Y. Varakin, S. Krishnaswami, B. Kuate Defo, E. J. Kuipers, S. Polinder, C. Kulkarni, V. S. Kulkarni, G. F. Kwan, T. Lai, R. Lalloo, T. Lallukka, R. Shiri, H. Lam, Q. Lan, V. C. Lansingh, P. M. Lavados, A. E. Lawrynowicz, J. L. Leasher, J. Lee, S. Yoon, M. Levi, J. Liang, Y. Wang, J. Zhu, S. E. Lipshultz, B. K. Lloyd, R. Room, G. Logroscino, J. Lortet-Tieulent, S. Ma, H. P. Phua, C. Magis-Rodriguez, A. A. Mahdi, R. Malekzadeh, S. Mangalam, C. C. Mapoma, F. Masiye, M. Marape, W. Marcenes, P. A. Meaney, D. J. Margolis, D. H. Silberberg, R. V. Martin, M. B. Marzan, M. T. Mashal, A. J. Mason-Jones, T. T. Mazorodze, A. C. McKay, M. Mehndiratta, M. Meltzer, W. Mendoza, F. Apolinary Mhimbira, T. R. Miller, E. J. Mills, S. Mishra, N. Mohamed Ibrahim, K. A. Mohammad, G. L. Mola, L. Monasta, M. Montico, L. Ronfani, A. R. Moore, L. Morawska, R. E. Norman, R. Mori, M. Tsilimbaris, J. Moschandreas, W. N. Moturi, A. Werdecker, U. O. Mueller, R. Westerman, M. Mukaigawara, Z. Nahas, K. S. Naidoo, L. Naldi, D. Nand, V. Nangia, B. Neal, C. Nejjari, S. P. Neupane, C. R. Newton, F. N. Ngalesoni, J. D. Ngirabega, J. M. Nolla, S. E. Vollset, O. F. Norheim, B. Norrving, L. Nyakarahuka, I. Oh, T. Ohkubo, B. O. Olusanya, J. N. Opio, R. S. Pagcatipunan, J. D. Pandian, E. Park, S. Seedat, B. I. Pavlin, L. Pejin Stokic, D. M. Pereira, R. Perez-Padilla, F. Perez-Ruiz, N. Perico, G. Remuzzi, M. Trillini, S. A. L. Perry, A. Pervaiz, K. Pesudovs, C. B. Peterson, M. Petzold, D. Plass, D. Poenaru, C. D. Pond, C. Pope, S. Popova, J. Rehm, N. M. Prasad, D. M. Qato, A. Rafay, S. M. Rana, S. Ur Rahman, M. Raju, I. Rakovac, M. Rao, H. Razavi, A. L. Ribeiro, G. Velasquez-Melendez, P. M. Riccio, L. A. Sposato, A. Roca, I. Romieu, K. Straif, G. M. Ruhago, B. F. Sunguya, R. L. Sacco, S. Saha, R. Sahathevan, J. R. Sanabria, L. Sanchez-Riera, A. Sapkota, J. E. Saunders, S. Soneji, M. Sawhney, M. I. Saylan, I. J. C. Schneider, D. C. Schwebel, J. A. Singh, B. Serdar, G. Shaddick, Y. Shinohara, K. Shishani, I. Shiue, I. D. Sigfusdottir, A. Singh, K. Søreide, C. T. Sreeramareddy, N. J. C. Stapelberg, V. Stathopoulou, N. Steckling, K. Stroumpoulis, S. Swaminathan, M. Swaroop, Y. Yano, B. L. Sykes, K. M. Tabb, R. T. Talongwa, D. Tanne, M. Tavakkoli, S. V. Thackway, G. D. Thurston, F. Topouzis, J. A. Towbin, H. Toyoshima, J. Traebert, U. Trujillo, Z. Tsala Dimbuene, E. Tuzcu, U. S. Uchendu, K. N. Ukwaja, R. Van Dingenen, C. H. van Gool, J. van Os, T. J. Vasankari, A. N. Vasconcelos, F. S. Violante, V. Victorovich Vlassov, S. G. Waller, M. T. Wallin, W. Wang, K. Wessells, J. D. Wilkinson, H. C. Williams, S. M. Woldeyohannes, J. Q. Wong, A. D. Woolf, G. Xu, L. L. Yan, G. Yang, P. Yip, N. Yonemoto, M. Z. Younis, Z. Younoussi, C. Yu, M. E. Zaki, Y. Zhao and S. Zhu, Global, Regional, and National Comparative Risk Assessment of 79 Behavioural, Environmental and Occupational, and Metabolic Risks or Clusters of Risks in 188 Countries, 1990-2013: A Systematic Analysis for the Global Burden of Disease Study 2013, Lancet, 2015, 386(10010), 2287–2323, DOI:10.1016/S0140-6736(15)00128-2.
- A. K. Amegah and S. Agyei-Mensah, Urban Air Pollution in Sub-Saharan Africa: Time for Action, Environ. Pollut., 2017, 220, 738–743, DOI:10.1016/J.ENVPOL.2016.09.042.
- A. K. Amegah, Proliferation of Low-Cost Sensors. What Prospects for Air Pollution Epidemiologic Research in Sub-Saharan Africa?, Environ. Pollut., 2018, 241, 1132–1137, DOI:10.1016/J.ENVPOL.2018.06.044.
-
Health Effects Institute, The State of Air Quality and Health Impacts in Africa. A Report from the State of Global Air Initiative, https://www.stateofglobalair.org/sites/default/files/documents/2022-10/soga-africa-report.pdf, (accessed Nov 8, 2022) Search PubMed.
- C. L. Weagle, G. Snider, C. Li, A. Van Donkelaar, S. Philip, P. Bissonnette, J. Burke, J. Jackson, R. Latimer, E. Stone, I. Abboud, C. Akoshile, N. X. Anh, J. R. Brook, A. Cohen, J. Dong, M. D. Gibson, D. Griffith, K. B. He, B. N. Holben, R. Kahn, C. A. Keller, J. S. Kim, N. Lagrosas, P. Lestari, Y. L. Khian, Y. Liu, E. A. Marais, J. V. Martins, A. Misra, U. Muliane, R. Pratiwi, E. J. Quel, A. Salam, L. Segev, S. N. Tripathi, C. Wang, Q. Zhang, M. Brauer, Y. Rudich and R. V. Martin, Global Sources of Fine Particulate Matter: Interpretation of PM 2.5 Chemical Composition Observed by SPARTAN Using a Global Chemical Transport Model, Environ. Sci. Technol., 2018, 52(20), 11670–11681, DOI:10.1021/ACS.EST.8B01658.
- J. Bachwenkizi, C. Liu, X. Meng, L. Zhang, W. Wang, A. van Donkelaar, R. V. Martin, M. S. Hammer, R. Chen and H. Kan, Fine Particulate Matter Constituents and Infant Mortality in Africa: A Multicountry Study, Environ. Int., 2021, 156, 106739, DOI:10.1016/J.ENVINT.2021.106739.
- Q. Di, P. Koutrakis and J. Schwartz, A Hybrid Prediction Model for PM2.5 Mass and Components Using a Chemical Transport Model and Land Use Regression, Atmos. Environ., 2016, 131, 390–399, DOI:10.1016/J.ATMOSENV.2016.02.002.
- K. Vohra, A. Vodonos, J. Schwartz, E. A. Marais, M. P. Sulprizio and L. J. Mickley, Global Mortality from Outdoor Fine Particle Pollution Generated by Fossil Fuel Combustion: Results from GEOS-Chem., Environ. Res., 2021, 195, 110754, DOI:10.1016/J.ENVRES.2021.110754.
- M. S. Hammer, A. Van Donkelaar, C. Li, A. Lyapustin, A. M. Sayer, N. C. Hsu, R. C. Levy, M. J. Garay, O. V. Kalashnikova, R. A. Kahn, M. Brauer, J. S. Apte, D. K. Henze, L. Zhang, Q. Zhang, B. Ford, J. R. Pierce and R. V. Martin, Global Estimates and Long-Term Trends of Fine Particulate Matter Concentrations (1998-2018), Environ. Sci. Technol., 2020, 54(13), 7879–7890, DOI:10.1021/ACS.EST.0C01764.
-
P. A. Solomon, D. Crumpler, J. B. Flanagan, R. K. M. Jayanty, E. E. Rickman and C. E. McDade, U.S. National PM2.5 Chemical Speciation Monitoring Networks—CSN and IMPROVE: Description of Networks, 2014, 64, 12, 1410–1438, DOI:10.1080/10962247.2014.956904.
- V. Ramanathan and G. Carmichael, Global and Regional Climate Changes Due to Black Carbon, Nat. Geosci., 2008, 1(4), 221–227, DOI:10.1038/ngeo156.
- G. T. Wolff, Particulate Elemental Carbon in the Atmosphere, J. Air Pollut. Control Assoc., 2012 DOI:10.1080/00022470.1981.10465298.
- H. Rosen, T. Novakov and B. A. Bodhaine, Soot in the Arctic, Atmos. Environ., 1981, 15(8), 1371–1374, DOI:10.1016/0004-6981(81)90343-7.
- M. O. Andreae, Soot Carbon and Excess Fine Potassium: Long-Range Transport of Combustion-Derived Aerosols, Science, 1983, 220(4602), 1148–1151, DOI:10.1126/SCIENCE.220.4602.1148.
- N. A. H. Janssen, G. Hoek, M. Simic-Lawson, P. Fischer, L. van Bree, H. T. Brink, M. Keuken, R. W. Atkinson, H. Ross Anderson, B. Brunekreef and F. R. Cassee, Black Carbon as an Additional Indicator of the Adverse Health Effects of Airborne Particles Compared with Pm10 and Pm2.5, Environ. Health Perspect., 2011, 119(12), 1691–1699, DOI:10.1289/EHP.1003369.
- T. J. Grahame and R. B. Schlesinger, Cardiovascular Health and Particulate Vehicular Emissions: A Critical Evaluation of the Evidence, Air Qual., Atmos. Health, 2010, 3(1), 3–27, DOI:10.1007/S11869-009-0047-X.
- H. Horvath, Atmospheric Light Absorption—A Review, Atmos. Environ., Part A, 1993, 27(3), 293–317, DOI:10.1016/0960-1686(93)90104-7.
- M. O. Andreae and A. Gelencsér, Black Carbon or Brown Carbon? The Nature of Light-Absorbing Carbonaceous Aerosols, Atmos. Chem. Phys., 2006, 6(10), 3131–3148, DOI:10.5194/acp-6-3131-2006.
- A. D. A. Hansen, H. Rosen and T. Novakov, The Aethalometer — An Instrument for the Real-Time Measurement of Optical Absorption by Aerosol Particles, Sci. Total Environ., 1984, 36, 191–196, DOI:10.1016/0048-9697(84)90265-1.
- D. Contini, R. Vecchi and M. Viana, Carbonaceous Aerosols in the Atmosphere, Atmos, 2018, 9(5), 181, DOI:10.3390/ATMOS9050181.
- G. O. Salako, P. K. Hopke, D. D. Cohen, B. A. Begum, S. K. Biswas, G. G. Pandit, Y. S. Chung, S. A. Rahman, M. S. Hamzah, P. Davy, A. Markwitz, D. Shagjjamba, S. Lodoysamba, W. Wimolwattanapun and S. Bunprapob, Exploring the Variation between EC and BC in a Variety of Locations, Aerosol Air Qual. Res., 2012, 12(1), 1–7, DOI:10.4209/AAQR.2011.09.0150.
-
G. Allen, P. Babich and R. Poirot, Evaluation of a New Approach for Real Time Assessment of Wood Smoke PM Urban-Scale Spat Ial-t Emporal Variabilit y of Black Carbon and Wint Er Resident Ial Wood Combust Ion Par., 2004.
- Y. Wang, P. K. Hopke, O. V. Rattigan, X. Xia, D. C. Chalupa and M. J. Utell, Characterization of Residential Wood Combustion Particles Using the Two-Wavelength Aethalometer, Environ. Sci. Technol., 2011, 45(17), 7387–7393, DOI:10.1021/ES2013984.
- Y. Wang, P. K. Hopke, O. V. Rattigan, D. C. Chalupa and M. J. Utell, Multiple-Year Black Carbon Measurements and Source Apportionment Using Delta-C in Rochester, New York., J. Air Waste Manage. Assoc., 2012, 62(8), 880–887, DOI:10.1080/10962247.2012.671792.
-
J. Y. W. Cheng, C. K. Chan and A. P. S. Lau, Quantification of Airborne Elemental Carbon by Digital Imaging, 2011, 45 (5), 581–586, DOI:10.1080/02786826.2010.550960.
-
N. Ramanathan, M. Lukac, T. Ahmed, A. Kar, P. S. Praveen, T. Honles, I. Leong, I. H. Rehman, J. J. Schauer and V. Ramanathan, A Cellphone Based System for Large-Scale Monitoring of Black Carbon, DOI:10.1016/j.atmosenv.2011.05.030.
- M. Jeronimo, Q. Stewart, A. T. Weakley, J. Giacomo, X. Zhang, N. Hyslop, A. M. Dillner, M. Shupler and M. Brauer, Analysis of Black Carbon on Filters by Image-Based Reflectance, Atmos. Environ., 2020, 223, 117300, DOI:10.1016/j.atmosenv.2020.117300.
- A. Mukherjee and D. W. Toohey, A Study of Aerosol Properties Based on Observations of Particulate Matter from the U.S. Embassy in Beijing, China, Earth’s Futur., 2016, 4(8), 381–395, DOI:10.1002/2016EF000367.
- R. Subramanian, A. S. Kagabo, V. Baharane, S. Guhirwa, C. Malings, N. J. Williams, E. Kalisa, H. Li, P. Adams, A. L. Robinson, H. L. Dewitt, J. Gasore and P. Jaramillo, Air Pollution in Kigali, Rwanda : Spatial and Temporal Variability, Source Contributions, and the Impact of Car-Free Sundays, Clean Air J., 30(2) DOI:10.17159/caj/2020/30/2.8023.
- H. Langley Dewitt, J. Gasore, M. Rupakheti, K. E. Potter, R. G. Prinn, J. De Dieu Ndikubwimana, J. Nkusi and B. Safari, Seasonal and Diurnal Variability in O3, Black Carbon, and CO Measured at the Rwanda Climate Observatory, Atmos. Chem. Phys., 2019, 19(3), 2063–2078, DOI:10.5194/acp-19-2063-2019.
- M. J. Gatari, P. L. Kinney, B. Yan, E. Sclar, N. Volavka-Close, N. S. Ngo, S. Mwaniki Gaita, A. Law, P. K. Ndiba, A. Gachanja, J. Graeff and S. N. Chillrud, High Airborne Black Carbon Concentrations Measured near Roadways in Nairobi, Kenya, Transp. Res. D: Transp. Environ., 2019, 68, 99–109, DOI:10.1016/J.TRD.2017.10.002.
- A. Retama, D. Baumgardner, G. B. Raga, G. R. McMeeking and J. W. Walker, Seasonal and Diurnal Trends in Black Carbon Properties and Co-Pollutants in Mexico City, Atmos. Chem. Phys., 2015, 15(16), 9693–9709, DOI:10.5194/ACP-15-9693-2015.
- B. Ambade, T. K. Sankar, A. S. Panicker, A. S. Gautam and S. Gautam, Characterization, Seasonal Variation, Source Apportionment and Health Risk Assessment of Black Carbon over an Urban Region of East India, Urban Clim., 2021, 38, 100896, DOI:10.1016/J.UCLIM.2021.100896.
- Y. Zhang, Y. Li, J. Guo, Y. Wang, D. Chen and H. Chen, The Climatology and Trend of Black Carbon in China from 12-Year Ground Observations, Clim. Dyn., 2019, 53(9–10), 5881–5892, DOI:10.1007/S00382-019-04903-0.
- M. A. Robert, M. J. Kleeman and C. A. Jakober, Size and Composition Distributions of Particulate Matter Emissions: Part 2—Heavy-Duty Diesel Vehicles, J. Air Waste Manage. Assoc., 2007, 57(12), 1429–1438, DOI:10.3155/1047-3289.57.12.1429.
- J. Jimenez, C. Claiborn, T. Larson, T. Gould, T. W. Kirchstetter and L. Gundel, Loading Effect Correction for Real-Time Aethalometer Measurements of Fresh Diesel Soot, J. Air Waste Manage. Assoc., 2007, 57(7), 868–873, DOI:10.3155/1047-3289.57.7.868.
- A. Virkkula, T. Mäkelä, R. Hillamo, T. Yli-Tuomi, A. Hirsikko, K. Hämeri and I. K. Koponen, A Simple Procedure for Correcting Loading Effects of Aethalometer Data, J. Air Waste Manage. Assoc., 2007, 57(10), 1214–1222, DOI:10.3155/1047-3289.57.10.1214.
-
R. Subramanian, C. A. Roden, P. Boparai and T. C. Bond, Yellow Beads and Missing Particles: Trouble Ahead for Filter-Based Absorption Measurements, 2007, 41 (6), 630–637, DOI:10.1080/02786820701344589.
- Z. Zhang, A Flexible New Technique for Camera Calibration, IEEE Trans. Pattern Anal. Mach. Intell., 2000, 22(11), 1330–1334, DOI:10.1109/34.888718.
- D. G. Lowe, Distinctive Image Features from Scale-Invariant Keypoints, Int. J. Comput. Vis., 2004, 60(2), 91–110, DOI:10.1023/B:VISI.0000029664.99615.94.
-
G. Bradski and A. Kaehler, Learning OpenCV, O'Reilly Media, Inc., 2009, vol. 16 Search PubMed.
- D. G. Lowe, Object Recognition from Local Scale-Invariant Features, Proc. IEEE Int. Conf. Comput. Vis., 1999, 2, 1150–1157, DOI:10.1109/ICCV.1999.790410.
- M. A. Fischler and R. C. Bolles, Random Sample Consensus: A Paradigm for Model Fitting with Applications to Image Analysis and Automated Cartography, Commun. ACM, 1981, 24(6), 381–395, DOI:10.1145/358669.358692.
-
Commission International Electrotechnical, Multimedia Systems and Equipment-Color Measurement and Management-Part 2-1. Color Manag. RGB Color space-sRGB, 1999.
- G. D. Finlayson, M. MacKiewicz and A. Hurlbert, Color Correction Using Root-Polynomial Regression, IEEE Trans. Image Process., 2015, 24(5), 1460–1470, DOI:10.1109/TIP.2015.2405336.
- E. S. Macias, S. Louis and R. B. Húsar, Atmospheric Particulate Mass Measurement with Beta Attenuation Mass Monitor, Environ. Sci. Technol., 1976, 18, 50 Search PubMed.
- P. Gu, H. Z. Li, Q. Ye, E. S. Robinson, J. S. Apte, A. L. Robinson and A. A. Presto, Intracity Variability of Particulate Matter Exposure Is Driven by Carbonaceous Sources and Correlated with Land-Use Variables, Environ. Sci. Technol., 2018, 11545–11554, DOI:10.1021/acs.est.8b03833.
- C. H. Jeong, P. K. Hopke, E. Kim and D. W. Lee, The Comparison between Thermal-Optical Transmittance Elemental Carbon and Aethalometer Black Carbon Measured at Multiple Monitoring Sites, Atmos. Environ., 2004, 38(31), 5193–5204, DOI:10.1016/J.ATMOSENV.2004.02.065.
- S. Rose Eilenberg, R. Subramanian, C. Malings, A. Hauryliuk, A. A. Presto and A. L. Robinson, Using a Network of Lower-Cost Monitors to Identify the Influence of Modifiable Factors Driving Spatial Patterns in Fine Particulate Matter Concentrations in an Urban Environment, J. Expo. Sci. Environ. Epidemiol., 2020, 30(6), 949–961, DOI:10.1038/s41370-020-0255-x.
- R. Subramanian, N. M. Donahue, A. Bernardo-Bricker, W. F. Rogge and A. L. Robinson, Contribution of Motor Vehicle Emissions to Organic Carbon and Fine Particle Mass in Pittsburgh, Pennsylvania: Effects of Varying Source Profiles and Seasonal Trends in Ambient Marker Concentrations, Atmos. Environ., 2006, 40(40), 8002–8019, DOI:10.1016/J.ATMOSENV.2006.06.055.
- A. L. Robinson, R. Subramanian, N. M. Donahue, A. Bernardo-Bricker and W. F. Rogge, Source Apportionment of Molecular Markers and Organic Aerosol. 2. Biomass Smoke, Environ. Sci. Technol., 2006, 40(24), 7811–7819, DOI:10.1021/ES060782H.
- A. P. Grieshop, E. M. Lipsky, N. J. Pekney, S. Takahama and A. L. Robinson, Fine Particle Emission Factors from Vehicles in a Highway Tunnel: Effects of Fleet Composition and Season, Atmos. Environ., 2006, 40(SUPPL. 2), 287–298, DOI:10.1016/J.ATMOSENV.2006.03.064.
- E. M. Lipsky and A. L. Robinson, Effects of Dilution on Fine Particle Mass and Partitioning of Semivolatile Organics in Diesel Exhaust and Wood Smoke, Environ. Sci. Technol., 2006, 40(1), 155–162, DOI:10.1021/ES050319P.
- R. K. Chakrabarty, H. Moosmüller, L. W. A. Chen, K. Lewis, W. P. Arnott, C. Mazzoleni, M. K. Dubey, C. E. Wold, W. M. Hao and S. M. Kreidenweis, Brown Carbon in Tar Balls from Smoldering Biomass Combustion, Atmos. Chem. Phys., 2010, 10(13), 6363–6370, DOI:10.5194/ACP-10-6363-2010.
|
This journal is © The Royal Society of Chemistry 2023 |