DOI:
10.1039/C9LC01209E
(Paper)
Lab Chip, 2020,
20, 1792-1806
Development of two-photon polymerised scaffolds for optical interrogation and neurite guidance of human iPSC-derived cortical neuronal networks
Received
9th December 2019
, Accepted 12th April 2020
First published on 16th April 2020
Abstract
Recent progress in the field of human induced pluripotent stem cells (iPSCs) has led to the efficient production of human neuronal cell models for in vitro study. This has the potential to enable the understanding of live human cellular and network function which is otherwise not possible. However, a major challenge is the generation of reproducible neural networks together with the ability to interrogate and record at the single cell level. A promising aid is the use of biomaterial scaffolds that would enable the development and guidance of neuronal networks in physiologically relevant architectures and dimensionality. The optimal scaffold material would need to be precisely fabricated with submicron resolution, be optically transparent, and biocompatible. Two-photon polymerisation (2PP) enables precise microfabrication of three-dimensional structures. In this study, we report the identification of two biomaterials that support the growth and differentiation of human iPSC-derived neural progenitors into functional neuronal networks. Furthermore, these materials can be patterned to induce alignment of neuronal processes and enable the optical interrogation of individual cells. 2PP scaffolds with tailored topographies therefore provide an effective method of producing defined in vitro human neural networks for application in influencing neurite guidance and complex network activity.
Introduction
Understanding the cellular and network mechanisms underlying the functioning of the human brain is a major goal of neuroscience. In addition to gaining insight into how neurons interact to generate functions such as memory and complex human attributes such as consciousness, such understanding is also an essential step in enabling the tackling of a range of neurological disease states. Conditions such as schizophrenia, epilepsy, traumatic brain injury and Alzheimer's disease continue to present major challenges to sufferers and increasing socio-economic pressure worldwide.1–3 The scientific and medical challenge is that understanding human brain function at the cellular level is constrained by limited access to diseased and non-diseased living brain tissue.
Animal models have played a central role in elucidating key mechanisms of neurodevelopment and neurotransmission, but despite many attempts at “humanising” models by implementing disease-causing mutations from human studies, it is not always possible to replicate complex neurological disorders in animals.4–7 To determine relevant functional human mechanisms and their disease related dysfunction it is therefore becoming clear that patient-derived human cell models are required that allow functional interrogation of cell–cell interactions from single cells to the whole-network level.8–14
The last decade has seen the emergence of neuronal cultures derived from human induced pluripotent stem cells (iPSCs) that has promise to be a technology that addresses many of the major issues currently facing the field. Neuronal cultures derived from iPSCs retain the disease genotype of the original donor, and therefore present a highly relevant model.
Developments with iPSC-derived neural cultures are advancing rapidly, however a majority have so far focused on deriving two dimensional planar cultures featuring a single subtype of neural cells, and a limited number of functional endpoints.15,16 In addition, a high variability of derived neuronal cell types and a lack of defined network architecture makes deriving conclusions from the analysis potentially problematic.
Recent focus has moved to using organoids which exhibit an in vivo-like morphogenic development program that generates self-organising structures and a wide spectrum of neural cells.17–19 Whilst organoids present an excellent emerging model for neurodevelopmental studies, robust cellular functional interrogation presents difficulties due to variable organoid size and structure.20–22
A potential solution to achieving the goal of developing controlled, low variability human neural networks with a fully definable cell population is the use of biomaterial scaffolds to guide and constrain cell interactions in three-dimensions in a top-down modelling approach.14,23–28 The field of tissue engineering has developed a multitude of research directions including; printing of functionalised proteins, microfluidics, polymer fibre extrusion, and polymer hydrogels.29–32 For those interested in a comprehensive review of this field that covers all these approaches please refer to Zhuang et al.33 In particular there is a large medical movement for reintroducing biological scaffolds as a regenerative medicine for neuronal damage.34 However, there is a key need for human in vitro structured neuronal networks for basic functional research and pharmaceutical testing. In recent years, micro-topographical surface relief has shown promise in aligning neurite-like structures in human and animal cell models.29,35–37 Production of aligned electrospun nanofibers has similarly shown neurite alignment and represents a promising model for investigating spinal cord injury.30,38,39 Perhaps most excitingly, technological advances in stereolithography, such as two-photon polymerisation (2PP), have provided a precise sub-micron-scale resolution for microfabrication of intricate 3D designs.40–42 Prior enlightening use of neuroblastoma and carcinoma lines (e.g. NT2, SH-SY5Y, Neuro2a) has shown the ability of these cell types for modelling neurite extension in bespoke scaffolds.34 However, little is known about the growth of human iPSC-derived neurons on 2PP scaffold models, whether they are functionally viable over physiologically relevant time periods and how scaffold structure can influence network development in a controllable manner to generate a defined underlying functional architecture.
Alongside advances in tissue modelling, similar advances have been made in the detection and monitoring of functional network-based activity. Electrophysiology remains the main methodology for single-cell and network functional characterisation in human in vitro brain models, with multi-well, high density and 3D multi-electrode arrays recently becoming commercially available.43,44 However, single cell resolution in networks where neurons may be in dense or irregular arrangements is problematic. Current solutions to these problems come from the emerging field of optogenetics, particularly with the development of genetically-encoded calcium and voltage indicators.45–47 In combination with fast imaging systems such as light-sheet fluorescence microscopy the use of genetically encoded indicators enables whole-network 3D activity acquisition.48 However, to make full use of these technologies in vitro, similar reproducibility and defined architecture of in vivo neural networks is desirable.
In this study we aimed to identify suitable materials to enable establishment of defined human neuronal networks on two-photon polymerised scaffolds. Putative polymer material(s) for 2PP should have three main properties once polymerised: (1) optical properties compatible with bright field and fluorescence functional imaging methodologies. (2) Biocompatibility to sustain normal iPSC-derived neuronal network development. (3) Printable via polymerisation at micron scale resolution relevant for cell interaction and guidance.
Here we report the identification and analyses of two suitable biomaterials, polyethylene glycol diacrylate with Irgacure 2959 (PEG2959) and Dental LT Clear (DClear), for 2PP to generate neural scaffolds using the criteria defined above. Human neural precursors derived from iPSCs were grown upon polymerised materials and differentiated to form functional cortical neurons. We observed that neurons cultured upon the surface of the materials matured ‘normally’ to form spontaneously active neuronal networks that could be optically interrogated using fluorescent calcium imaging and immunofluorescent staining. Furthermore, we determined that micropatterning of 2PP materials could be utilised to control the orientation of extending neurites, influencing overall network development. Together, our results demonstrate the utility of these materials and 2PP to shape complex architectures in vitro, and pave the way towards the development and monitoring of functional, tailored neuronal microcircuits.
Methods
Generation of UV-polymerised pellets and two-photon polymerised scaffolds
Four commercially available polymer materials were considered as candidate materials: (i) SR-259, a polyethylene glycol (200) diacrylate (PEG; Sartomer, Warrington, USA); (ii) Dental LT Clear (DClear) a proprietary combination of methyacrylic oligomers and glycol methacrylate (Formlabs, Berlin, Germany); (iii) polylactic acid (PLA); and (iv) organically modified silicon (Ormosil).49 These materials were used to produce planar pellets. To obtain a photopolymerisable formulation, the photoinitiators Irgacure 369, Irgacure 651 or Irgacure 2959 (Ciba, Basel, Switzerland) were added to the SR-259 at a concentration of 1% by weight.50 For the PEG (200) and photoinitiator combinations, these materials are referred to as PEG369, PEG651 and PEG2959 in text and figures. As many commercially-available photoinitiators are considered toxic, DClear was chosen due to its established medical approval as a Class IIa biocompatible resin (EN-ISO 10993-1:2009/AC:2010) indicating that the proprietary photoinitiator supplied was also identified as biocompatible. Planar polymer pellets with diameters of 6 mm and a thickness of approximately 800 μm were prepared from PEG369, PEG651, PEG2959, and DClear material formulations by photopolymerisation with ultraviolet (UV) light. For this purpose multi-well chambered coverslips (Grace Bio-labs, Oregon, USA) were filled with respective polymer and photoinitiator combinations, and subsequently cured using UV light (254 nm, dose 24 J cm−2) to form pellets. PLA and Ormosil photopolymers were prepared using a concentration of 1% by weight of 4,4-bis(diethylamino)benzophenone (Michler's ethyl ketone) photoinitiator (Sigma Aldrich, Missouri, USA) and fabricated via 2PP using a microscope objective (Zeiss Epiplan, 20×, NA: 0.4) at a laser wavelength of 780 nm (140 fs, 100 mW) as previously described.51–53 Unexposed material was removed by developing the PLA-4Bis samples in Ormodev (Micro resist technology GmbH, Germany) and Ormosil-4Bis samples in 1-propanol. These test samples are referred as PLA-4Bis and Ormosil-4Bis in text and figures.
Triangular surface topographies from DClear were produced using 2PP in a further step on the prepared UV cured DClear pellets as a surface substrate. A droplet of DClear was applied to the pellet surface and covered with a 170 μm thick cover glass (#1.5 coverslip) using a 200 μm spacer for creation of structuring volume. Triangular structures (50 μm length × 20 μm width × 20 μm height, with minimum 5 μm spacing in all directions) were then built on the surface of the pellets by three-dimensional direct laser writing using a commercial 2PP system (M4D, Laser nanoFab, Hannover, Germany) on the basis of a CAD model. A schematic illustration shows the experimental setup applied for 2PP fabrication (Fig. 1). A microscope objective (Zeiss Epiplan, 20×, NA: 0.4) was used for production, to focus the femtosecond laser beam (780 nm, 140 fs, 100 mW) into the photopolymer. The structuring was carried out at a writing speed of 2 mm second−1 with 0.5 μm hatching and 5 μm slicing parameters. After removal of the coverslip the structure was developed by 2-propanol to remove unexposed material. Prior to usage all samples were sterilised for 24 hours in 70% volume/volume (v/v) ethanol, then washed three times with sterile distilled water, followed by 7 days curing in sterile distilled water.
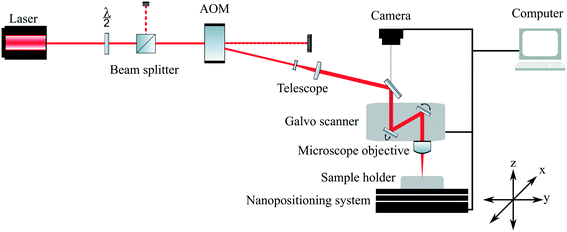 |
| Fig. 1 Schematic illustration of the experimental setup applied for 2PP fabrication. A femtosecond laser beam (780 nm, 140 fs, 100 mW) was focused onto a glass coverslip covering a photopolymer droplet using a microscope objective (Zeiss Epiplan, 20×, NA: 0.4). Using a XYZ stage, 2PP samples were produced at a writing speed of 2 mm second−1. | |
Human iPSC-derived NPC plating and differentiation
Human iPSC-derived neural progenitor cells (ax0016, Axol Bioscience Ltd., Cambridge, UK) were plated from frozen at 1 × 105 cells per cm2 onto SureBond (Axol Bioscience) coated 6-well plates using neural maintenance medium (Axol Bioscience) and Y-27632 2HCl (10 μM, Selleck Chemicals, Texas, USA) and cultured for 24 hours at 37 °C in a 5% CO2 environment.54 The plating medium was then replaced with Neural Maintenance medium with recombinant human FGF2 (20 ng mL−1) for initial expansion. Once the necessary number of cells was obtained, cells were terminally plated at a density of 7.5 × 104 cells per cm2 on tissue culture plastic, 13 mm ϕ glass coverslips (Thermo Fisher Scientific, Massachusetts, USA), as well as UV- and 2PP-fabricated constructs in a 48-well plate (Corning, New York, USA). All substrates were pre-coated with SureBond, a mouse laminin solution, and Readyset, a polyethyleneimine solution (Axol Bioscience) prior to seeding. Pre-coating with an extracellular matrix substrate was used to support adhesion of human NPCs and neurons, due to the typically bio-inert nature of unmodified polymers and hydrogels.55 During initial plating neural maintenance medium and Y-27632 2HCl (10 μM) was used, which was replaced 24 hours later, with neural maintenance medium without further supplementation and then fully exchanged every 2–3 days. For the rapid examination of neural projections on 2P-polymerised substrates, after a further 24 hours in neural maintenance medium alone, media was replaced with neural differentiation-XF medium (Axol Biosciences) supplemented with recombinant human BDNF (10 ng mL−1, Stem Cell Technologies, Cambridge, UK) and recombinant human GDNF (10 ng mL−1, Stem Cell Technologies) every 3 days.
Calcium imaging
Cultures were incubated in 10 μM Fluo-4 AM in DMSO (Thermo Fisher Scientific) diluted in cell culture medium for 35 minutes at 37 °C in a 5% CO2 environment. Samples were then washed with cell culture medium alone and incubated for a further 10 minutes to recover. Samples were transferred to a tissue perfusion chamber (RC-25, Warner instruments) on the stage of a Nikon FN1 microscope (Nikon, Kingston-upon-Thames, UK) and coverslip adhered in place using silicon grease. The chamber was perfused with 37 °C pre-warmed artificial cerebrospinal fluid (ACSF), a standard buffer solution for electrophysiological recording, containing the following: 126 mM NaCl, 5 mM KCl, 26 mM NaHCO3, 1.25 KH2PO4, 1 mM MgSO4, 2 mM CaCl2 and 10 mM glucose.56 Calcium activity was acquired using an OptoLED (Cairn Research, Faversham, UK) light source and FITC filter cube (Chroma Technology, Vermont, USA) with Optomorph image acquisition software (Cairn Research). Images were acquired at 0.5 Hz with a 30 ms exposure time. Tetrodotoxin citrate (TTX, 1 μM, Hellobio, Bristol, UK) and L-glutamate (100 μM, Hellobio) were used to inhibit and excite calcium transients respectively. Non-overlapping cells that displayed spontaneous calcium transients and had a typical neuronal morphology/soma size (<20 μm) were selected with an ROI surrounding their soma and then followed throughout the rest of the experiment.57
Live/dead cell viability staining
To determine viability, live cultures were incubated in DMEM:F12 (Thermo Fisher Scientific) supplemented with 2 μM calcein-AM (green – live cells, Thermo Fisher Scientific) and 4 μM ethidium homodimer-1 (red – dead cells, Thermo Fisher Scientific) at 37 °C for 15 minutes before immediately imaging using fluorescence microscopy to determine a live/dead ratio. After washing with DMEM:F12 once to remove excess dye, the cultures were imaged using 4× and 10× objectives (Olympus, Tokyo, Japan) on a Olympus CKX53 microscope with a GXCAM Hichrome-MET camera (GT Vision, Suffolk, UK), and pE-300 LED illumination source (CoolLED, Andover, UK) for acquisition. Acquired images were then processed and quantified using Image J – FIJI (NIH, USA) to determine the percentage of live cells from the total population.58,59 Viability was estimated from samples harvested at 1 day in vitro (DIV), 7 DIV and 14 DIV post-plating.
Immunocytochemistry
Cultures were fixed in 4% v/v formaldehyde (Sigma-Aldrich, Missouri, USA) in 4% sucrose w/v (weight/volume) DPBS for 15 minutes at room temperature (RT), then rinsed thrice with DPBS. Fixed cultures were incubated in permeabilisation buffer (0.2% Triton X-100 (v/v, Sigma-Aldrich) in DPBS), for 10 minutes at RT, then transferred into blocking buffer (3% Bovine serum albumin (w/v, Sigma-Aldrich) in permeabilisation buffer) and incubated for 1 hour at RT. Primary antibodies were prepared in blocking buffer and incubated with cultures overnight at 4 °C. Primary antibodies used were: anti-doublecortin (newborn neuron marker, 1
:
1000, Abcam), nestin (intermediate progenitors marker, 1
:
200, Millipore), anti-beta III tubulin (neuronal marker, 1
:
500, Abcam), anti-KI67 (mitotic marker, 1
:
1000, Abcam), anti-paxillin 6 (telencephalic progenitor marker, 1
:
300, Biolegend), anti-SOX2 (neuroepithelial progenitor marker, 1
:
300, R&D Systems), anti-TBR1 (1
:
200, early-born cortical neuronal marker, Abcam), anti-CTIP2 (1
:
200 early-born cortical neuronal marker, Abcam). Samples were then rinsed thrice with DPBS and incubated in secondary antibodies prepared in blocking buffer, protected from light, for 1 hour at RT. Secondary antibodies used were FITC Goat anti-Rabbit (Jackson ImmunoResearch, Cambridgeshire, UK), Alexa Fluor 633 Goat anti-Mouse (Abcam), and Alexa Fluor 543 Goat anti-Rat (Abcam). Cultures were then rinsed thrice in DPBS, including DAPI (a nuclear stain, 1
:
1000, Thermo Fisher Scientific) in the final rinse. Samples were left protected from light, unmounted in DPBS until imaging. Z-stacks of images were acquired using a TCS SP5 laser scanning confocal microscope (Leica, Wetzlar, Germany) with a 20× Leica water immersion objective. A minimum of three randomly selected regions of interest were imaged per replicate. To determine the number of bound cells post-plating, the same protocol was followed with fixation and permeabilisation, then was followed with a 30 minutes incubation of ActinGreen 488 Readyprobes (Thermo Fisher Scientific), followed with two DPBS rinses and incubation with DAPI in DPBS. To quantify immunocytochemical staining of cells positive for protein markers, a thresholded ROI mask was generated from DAPI+ staining in FIJI, which was then used to measure the mean pixel intensity in each ROI on its respective protein marker image. Once a minimum threshold was set for positive staining, the total number of ROIs that exceeded the threshold was divided by the total number of ROIs to generate a percentage of cells positive for the specific marker.58 To quantify neurite filament intensity, a randomised mask with 10 square ROIs was overlaid on DAPI+ and filament images and the mean pixel intensity measured. The mean pixel intensity of each filament marker ROI was then divided by its respective DAPI ROI to normalise the staining to quantity of cells. All statistical analysis was performed using GraphPad Prism 8 (GraphPad, San Diego, USA).
Quantitative and qualitative assessment of transmission spectra, auto-fluorescence, and opacity of sample materials
To register the transmission spectra of candidate materials, a LAMBA 950 spectrophotometer (Perkin Elmer, Massachusetts, USA) was used. A calibration spectrum for air was acquired, and followed by a control for a glass microscope slide. Small flakes of candidate material were then adhered to a microscope slide and later normalized to their spectra. Spectra were recorded in the range 380–720 nm with steps of 5 nm.
The background auto-fluorescence of samples was assessed using a TCS SP8 laser scanning confocal microscope (Leica). A 405 nm diode laser was used to excite the polymers. Illumination intensity was measured beforehand with a S121C photodiode (Thorlabs, New Jersey, USA) to guarantee comparability between different materials. Using the xyλ scan mode, images were registered of the first surface of the polymer at different wavelengths (λ), ranging from 425 to 705 nm, with a bandwidth of 10 nm. An emission spectra was produced by averaging the intensity over a set area of the polymer flake using FIJI. Continuous spectra were achieved by interpolating the data.
Candidate materials were imaged under an inverted fluorescence microscope to qualitatively assess their transparency under typical imaging conditions. Excitation and acquisition were achieved using a 60× Nikon (CFI APO NIR, 1.0 NA, 2.8 mm WD) water immersion objective, and a 2× Nikon (CFI Plan Achro UW, 0.06 NA, 7.5 mm WD) air objective, a mercury lamp, a FITC filter cube (Nikon), and a QICAM Fast 1394 monochrome CCD digital camera (QImaging, British Columbia, Canada). The transparency of the scaffolds was evaluated by imaging 2 μm green fluorescent beads (Estapor microspheres, Merck KGaA, Darmstadt, Germany) through them. The beads were attached to a coverslip coated with poly-L-lysine. Imaging was performed by placing a single flake of each polymer within a petri dish with a Corning cover-glass bottom, and, on top of the polymer, the coverslip with the micrometric beads. The dish was filled with distilled water and an exposure time of 50 ms was used to simulate imaging conditions. Contrast and brightness were set equally for all images in FIJI.59
Assessment of neurite alignment
To calculate orientation of neuronal filaments, Z-stacks acquired at 2 μm steps were first projected at maximum intensity within each field of view, to ensure continuous filament display. FIJI plugin “OrientationJ” was used to quantitatively assess the distribution of orientations for neurites (DCX+/TUJ1+) throughout the field of view.59,60
The orientation distribution measure A(θ) was normalised to enable inter-sample comparisons. The peak distribution angle A(θmax) was identified as the angle with the greatest number of detected alignments. The mean value of the orientation distribution is the integral of A(θ) over the range of angles (Atotal) divided by the absolute value of angular range (180°). The normalised distribution factor (NDF) is obtained by dividing the peak value of the distribution A(θmax) by the mean value of the orientation distribution, as given by the formula below:
A higher NDF value indicates greater unidirectional alignment of neurites. NDF values close to 1 indicate isotropic growth of neurites.
Scanning electron microscopy (SEM) imaging of cultures on scaffold material
To obtain high resolution images of the cultured cells on the surface of the scaffold material, triangular patterned samples were prepared for SEM imaging. Sample scaffold cultures were initially fixed with 2.5% v/v Glutaraldehyde at RT for 1 hour, followed by several 5 minute washes. After fixation, samples were dehydrated with graduated steps from graded 70% ethanol to 100% ethanol, followed by critical point drying. The sample was mounted upon a metal stub and sputter coated with platinum. Finally, the samples were imaged using a JSM-6060 scanning electrode microscope (Jeol USA, Massachusetts, USA) and Oxford Inca 300 EDS system (Oxford Instruments, UK). Scanning electron microscopy was conducted at the School of Metallurgy and Materials, University of Birmingham.
Results
Material optical properties
While a number of studies have reported substrates for two photon polymerisation, there is incomplete information on biocompatibility and imaging capability of these polymer scaffolds to examine network development and function.61 Optical transparency and low auto-fluorescence of the polymerised scaffolds is a prerequisite to achieving fluorescence-based protein visualisation and calcium activity monitoring in 3D neural tissue constructs. Opaque or dispersive polymers will impede imaging through the material surface, a problem that will worsen once series of layers are stacked upon each other, leading to incomplete mapping of three-dimensional structures. Likewise, materials with a high auto-fluorescence profile would reduce the signal to noise ratio, limiting the ability to discern distinct calcium indicator-visualised transients that are indicative of the opening of voltage gated calcium channels during neuronal transmission, and fluorescence-conjugated markers of proteins. To determine the suitability for brightfield and fluorescence imaging of the polymerised materials, UV-cured pellets or two photon polymerised scaffolds were produced using several candidate materials and photoinitiator combinations, namely DClear, Ormosil-4Bis, PEG and PLA-4Bis.
Initially, a transmission spectrum was acquired to determine the spectral absorption by the materials (Fig. 2A). Whilst many of the PEG samples permitted transmission of the majority of the 380–720 nm spectrum, which is relevant to widely used biological commercially available fluorophores (e.g. DAPI, FITC, GFP, TRITC, RFP, rhodamine Red-X, Texas Red, Cy5), DClear demonstrated a transmission spectra comparable to glass coverslips between 440–720 nm. Ormosil-4Bis and PLA-4Bis, which were structured by 2PP, exhibited reduced light transmission across the entire spectrum with over 50% reduction in signal relative to control. There was almost complete loss of transmission with Ormosil-4Bis between 380–525 nm. Due to their low spectral transmission Ormosil-4Bis and PLA-4Bis are poor candidate materials for multi-layered scaffolds.
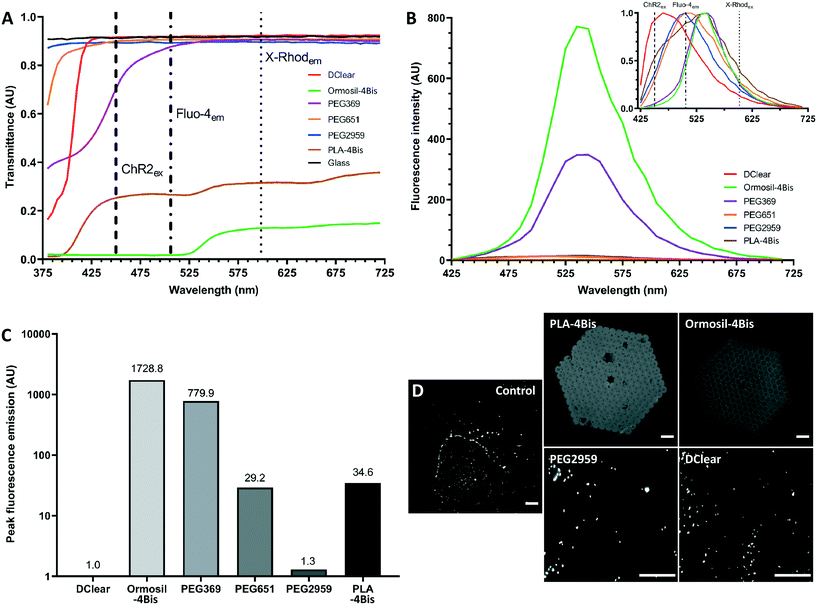 |
| Fig. 2 Optical characteristics of 2PP candidate materials. (A) Transmission spectra of candidate biomaterials over 380–720 nm in 5 nm steps. Channelrhodopsin Chr2/H134 excitation wavelength (450 nm) and calcium indicators Fluo-4 AM (506 nm) and X-Rhod-1 AM (602 nm) emission wavelengths are marked to represent widely used examples of optical interrogation tools for neuronal function. (B) Interpolated spectra of fluorescence emission intensity from 405 nm laser-excited candidate materials, recorded from 425–705 nm, with a spectral bandwidth of 10 nm. Inset, normalised graph highlighting emission peaks. (C) A normalised comparison between candidate materials to determine relative auto-fluorescence emission using maximal values. (D) Images of candidate materials structured by UV (PEG2959, DClear) or two-photon polymerisation (PLA, Ormosil) placed over green fluorescent beads and imaged using 405 nm-laser excitation. Scale bars = 50 μm. Arbitrary unit (AU). | |
To determine the contribution of auto-fluorescence, 405 nm-excited signal intensity was measured over a wide visible spectrum of 425–715 nm in 10 nm steps (Fig. 2B), then normalised to produce a continuous spectrum (Fig. 2B, inset). DClear exhibited the shortest auto-fluorescence wavelength peak at 465 nm, a wavelength shorter than the emission peak of key calcium indicators (Fluo4em 506 nm; GCaMP6em 512 nm; R-GECOem 590 nm; X-Rhod-1em 602 nm). PEG651 and PEG2959 exhibited emission peaks between 500 to 525 nm making them potentially unsuitable for imaging ultraviolet – green spectrum fluorophores but not yellow – infrared spectrum fluorophores. PEG369, PLA-4Bis and Ormosil-4Bis exhibited emission peaks at 525–565 nm, making them potentially unusable for distinguishing conventional green spectrum fluorophores.
The main determinant of substrate utility is however intensity of fluorescence. Ormosil-4Bis and PEG369 displayed intensity which were orders of magnitude higher than the lowest emitting samples PEG-2959 and DClear (Fig. 2C and D). Normalisation to DClear as lowest overall fluorescence (1 arbitary unit, AU), produced relative intensity magnitudes, with PEG2959 displaying lowest in the PEG-photoinitiator combinations (1.3 AU), with Ormosil-4Bis (1728.8 AU), PEG369 (779.9 AU) and PLA-4Bis (34.6 AU) displaying the highest relative auto-fluorescence intensities (Fig. 2C).
The most commonly used fluorescent calcium indicators are green emitting (500–565 nm). To further determine the utility of the candidate materials for imaging of such fluorescence we therefore determined whether 2 μm green fluorescent beads (470 nmex, 525 nmem) could be imaged through the test materials. DClear and PEG2959 permitted visualisation of the beads through the material (Fig. 2D). However, Ormosil-4Bis and PLA-4Bis did not allow visualisation of the beads through their structure.
Cell adhesion and viability
The spectral analysis revealed PEG2959 and DClear as the most promising biomaterials and thus were selected for continued study. Human iPSC-derived neural progenitors were cultured upon these candidate materials, tissue-culture treated plastic (TCPS), and glass coverslips to assess viability of cell-biomaterial interactions (Fig. 3Ai). To demonstrate suitability for adherent culture, density of adherent cells and average cell spreading was measured 24 hours post-plating. There was no significant difference (one-way ANOVA, F(3,16) = 1.178, p = 0.349) between the density of adhered cells determined by total nuclei visualised by DAPI+ staining in each of the four conditions; TCPS, Glass, DClear and PEG (Fig. 3Aiii and B). To determine whether nuclei density represented a good measure of adherence, cell spreading was also determined by calculating the average surface area per cell via the phallodin-labelled F-actin distribution per nuclei (Fig. 3Aii and C). F-Actin is an essential component of eurkaryotic cytoskeletons, and thus is largely distributed throughout the cell. Again no significant difference was seen between all conditions (one-way ANOVA, F(3,16) = 2.343, p = 0.112).
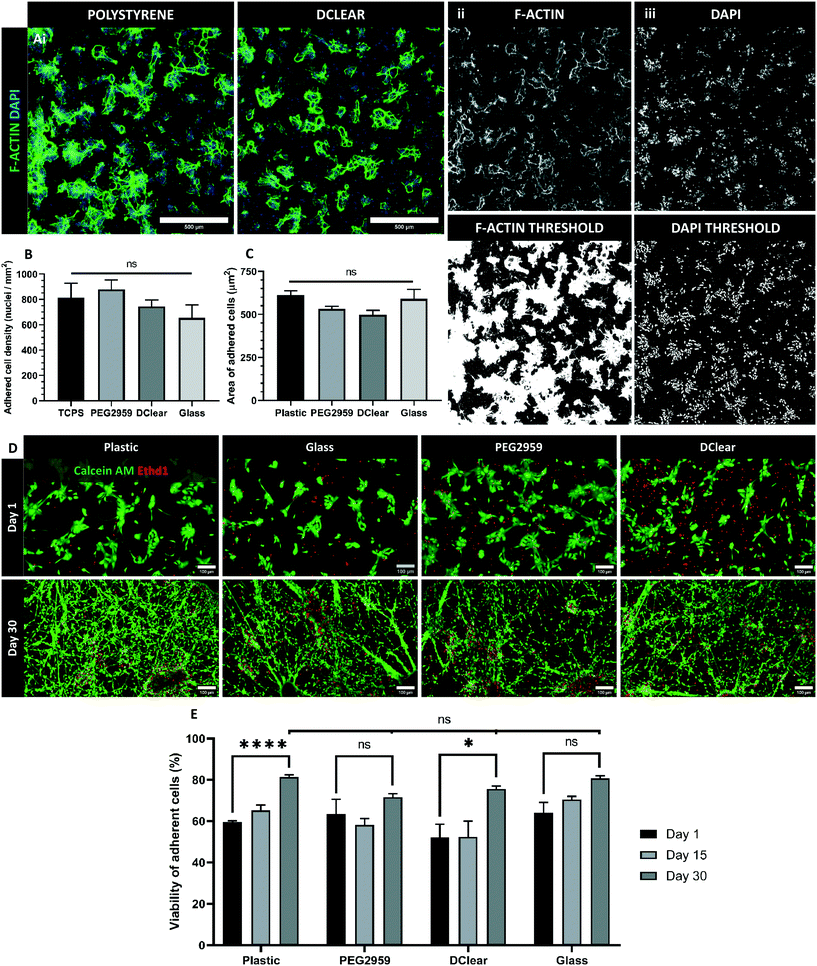 |
| Fig. 3 Candidate material biocompatibility for long-term adherent cultures. (A) i) Representative images of Phalloidin (green) and DAPI (blue) staining 24 hours post-plating on polystyrene and DClear with human neural progenitor cells. Scale bars = 500 μm. ii) F-Actin expression with thresholded image below. iii) Nuclei localisation with thesholded image below. (B) 24 hours post-plating adherent nuclei were quantified by number of thresholded DAPI+ regions of interest. (C) Mean surface area per cell (μm2) quantified by normalising total area of F-actin expression (Phallodin+ ROIs) against total number of nuclei (DAPI+ ROIs). (D) Representative images of live (calcein-AM+, green) and dead (Ethd1+, red) viability staining at 1 DIV (days in vitro) and 30 DIV post-plating of human neural progenitor cells. Scale bars = 100 μm. (E) % viability (calcein-AM+) of cells was quantified against total cell population. *p < 0.05, ***p < 0.001. Data represented as mean + s.e.m. | |
When using non-biological materials in cell culture it is important to determine any acute or chronic toxicity the material may present. However, as NPCs are a self-renewing population, the proportion of total viable cells should increase, rather than decrease over time. To determine the viability of adherent cultures grown directly on the candidate materials cell viability was assessed at 24 hours, 15- and 30-days post-plating (Fig. 3D). The percentage of living cells (green; calcein AM) was normalised against the total number of cells including dead (red; ethidium homodimer-1) for each sample. Whilst, there was a significant increase in cell viability over time on TCPS and DClear (paired T-test, TCPS T(3) = 16.79, p < 0.001; DClear T(3) = 3.454, p = 0.0408), PEG2959 and glass displayed no significant change in viability. At no time point was there significant difference (one-way ANOVA) in viability of cells grown on any of the materials tested compared to the control TCPS (Fig. 3D), suggesting that viability was not significantly impaired in any condition, despite lack of significant changes in viability for PEG2959 and glass.
Differentiation of hNPCs
A key consideration in the use of novel biomaterials are potential effects on differentiation capacity. To estimate differentiation efficiency, the proportion of cells staining for specific cell markers of neural progenitors, KI67+ (mitotically active), nestin+ (intermediate filaments), and those for TUJ1+ early post-mitotic neurons, were compared over time and between conditions. Additionally PAX6+ and SOX2+ expression was used to show cortical progenitor identity.
Initially, at 2 DIV post-plating, a high proportion of cells positively express KI67 (Glass, 72.06 ± 1.97%; DClear, 77.65 ± 2.65%; PEG2959, 69.51 ± 3.04%) with a high intensity of nestin (Glass, 0.639 ± 0.052 AU; DClear, 1.082 ± 0.130 AU; PEG2959, 0.777 ± 0.074 AU, relative intensity) relative to TUJ1 staining (Glass, 0.111 ± 0.026 AU; DClear, 0.120 ± 0.028 AU; PEG2959, 0.165 ± 0.034 AU, relative intensity) indicating a largely progenitor population (Fig. 4A–C). At 30 DIV post-plating a significant increase in the relative proportion of TUJ1+ neurons was identified (paired T-test, Glass, T(7) = 4.292, p < 0.01; DClear, T(7) = 5.794, p < 0.001; PEG2959, T(7) = 4.064, p < 0.01). Conversely, a significant decrease in overall KI67+ (paired T-test, Glass, T(8) = 18.94, p < 0.0001; DClear, T(8) = 20.72, p < 0.0001; PEG2959, T(8) = 12.28, p < 0.0001) and an increase in nestin+ intensity was also seen (paired T-test, Glass, T(8) = 3.196, p = 0.0127; Dclear, T(10) = 3.337, p < 0.01; PEG2959, T(8) = 3.920, p < 0.01). However it was a smaller fold increase compared with the increase in TUJ1+ intensity (Glass, 5.46× Nes, 31.36× TUJ1; DClear, 2.77× Nes, 16.32× TUJ1; PEG2959, 2.74× nestin, 9.71× TUJ1). Neither PEG2959 nor DClear appeared to significantly inhibit KI67+ progenitor maturation to post-mitotic (KI67−) neurons or post-mitotic neuronal survival over a 30 day period as previously shown by viability data (Fig. 4D).
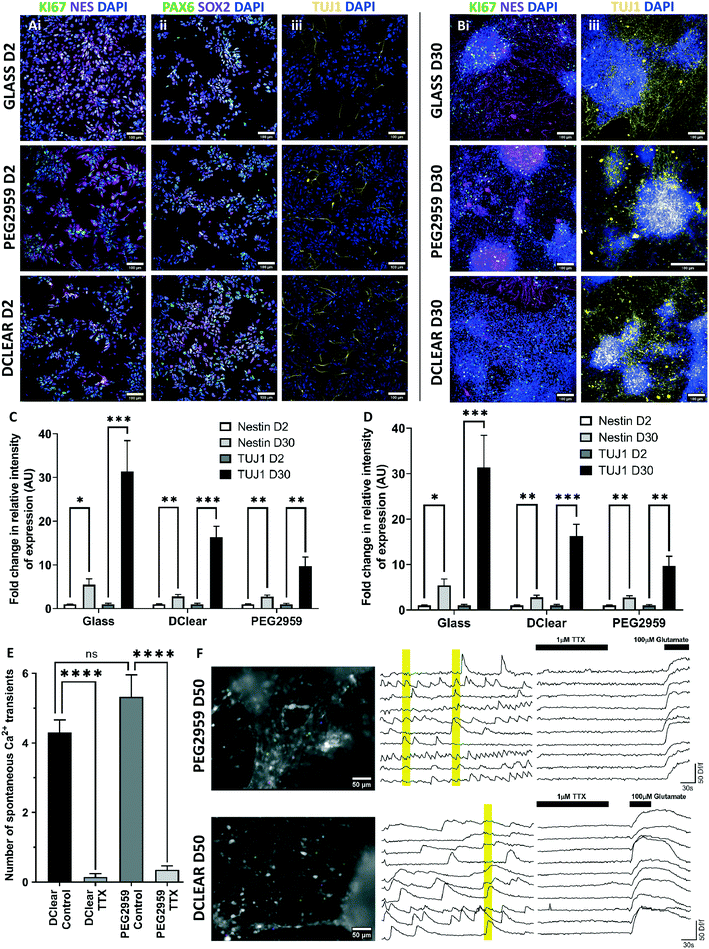 |
| Fig. 4 Assessment of neural progenitor efficiency in differentiation into functionally active post-mitotic neurons. (A) Representative images of fluorescence immunocytochemistry for protein markers. Mitotically active neural progenitors which are (i) SOX2+ (magenta) and PAX6+ (green), (ii) nestin+ (magenta) and KI67+ (green), naturally differentiated into post-mitotic neurons which are (iii) TUJ1+ (yellow) and KI67−/nestin−, from (A) 2 DIV (days in vitro) post-plating to (B) 30 DIV post-plating. Cells were grown on each DClear and PEG2959 for comparison against glass coverslips. DAPI+ (blue) staining indicates nuclear material. Scale bars = 100 μm. (C) Quantification of percentage positive staining for PAX6/SOX2 at 2 DIV, and KI76 at 2 DIV and 30 DIV. N ≥ 8 (D) relative intensity of nestin+ and TUJ1+ staining at 2 DIV and 30 DIV. N ≥ 8 (E) DIV 50 post-plating neural cultures on candidate materials DClear and PEG2959 cells displayed action potential dependent calcium transients that were blocked with TTX (1 μM) addition. N = 40 cells. *p < 0.05, **p < 0.01, ***p < 0.001, ****p < 0.0001. Data represented as mean + s.e.m. (F) Representative field of view with ROIs indicated for calcium imaging traces of 50 DIV post-plating cells loaded with Fluo-4 AM. Traces show spontaneous calcium elevations, which are blocked by TTX (1 μM) addition. Cells displayed elicited calcium transients to excitatory neurotransmitter glutamate (100 μM) addition. Yellow bars signify where ≥4 cells show synchronised firing. Scale bar = 50 μm. | |
When determining neuronal health it is crucial to consider both the viability of the cells and also their ability to form physiologically functional networks. To determine activity, 50 DIV cultures on PEG2959 and DClear were loaded with cell permeable Fluo-4 AM, a dye which shows fluorescence increase upon calcium ion influx into the cell, typically via voltage-gated calcium channels when depolarised by neuronal firing. These cultures displayed spontaneous calcium transients, which were significantly blocked (paired T test, DClear, T(39) = 12.25, p < 0.001); PEG2959, T(39) = 7.486, p < 0.001) by the presence of voltage-gated sodium channel blocker TTX (1 μM) in >90% of active cells (Fig. 4E). As voltage-gated sodium channels are required for action potential generation, this indicates a dependence on action potentials for the observed calcium transients. No significant difference in the amount of spontaneous activity was noted between DClear and PEG2959, meaning similar activity levels were present in cultures on different candidate materials. Synchronised calcium elevations in different imaged neurons confirmed network-level activity (Fig. 4F, yellow bars).57 The presence of functional glutamate receptors, the main neurotransmitter of the cortex, was established with 100% of cells displaying elicited calcium transients upon acute glutamate (100 μM) addition (Fig. 4F, N = 40).
Guidance of neuronal processes by 2PP generated microstructures
Morphogen gradients and precise spatial patterning enable the development of defined networks of neurons in vivo. However, controlling the development of networks in vitro is a significant challenge. Small scale studies using microfluidics, micropatterning and aligned fibres have demonstrated great promise.30,62 However, 2PP provides much greater resolution than can be achieved by using other methods. To determine whether directionally-oriented neuronal networks can be prepared with structural modifications, 2PP was used to print micron-scale triangular guidance motifs with DClear polymer (Fig. 5A). Previous studies in microfluidics and micropatterning have shown that constrictive funnel motifs may be employed to gate directional neurite extension.29,63–65 Building upon these findings, we used 2PP to fabricate a matrix of 2.5D triangles in a repeated pattern, with the aim of guiding directional neurite extension over relatively large distances (>500 μm). Progenitors cultured upon patterned and planar unpatterned platforms were synchronously differentiated and analysed to determine their distribution and orientation (Fig. 5B). Triangular micro-patterning demonstrated a highly clustered orientation distribution with a single central peak, whereas on the planar areas of patterned platforms (defined as a planar region >1000 μm distant from the surface modifications), or unpatterned platforms distribution appeared random (Fig. 5C).
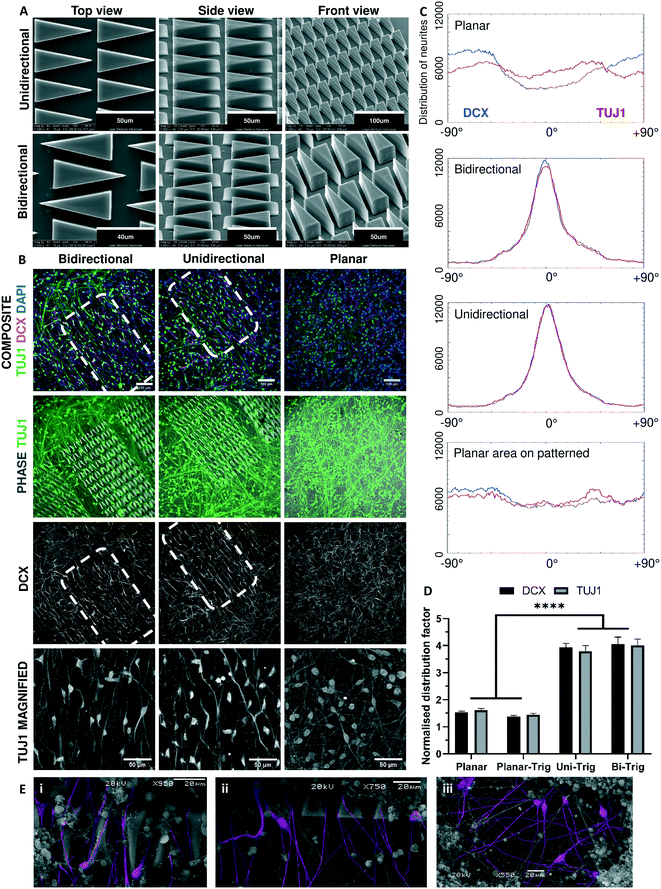 |
| Fig. 5 Alignment of neuronal filaments by two-photon micro-guidance patterning. (A) Representative scanning electron microscopy images of unidirectional and bidirectional two-photon polymerised micro-guidance triangles. Scale bars = 40, 50 and 100 μm. (B) Representative images of 14 DIV (days in vitro) post-mitotic neurons grown on patterned and planar platforms. Neurites are represented by TUJ1+ (green) and DCX+ (magenta) staining. DAPI represents nuclear material (blue). Dotted white line displays localisation of triangular patterning. Magnified regions display alignment of TUJ1+ neurites. Scale bars = 100 and 50 μm. (C) Representative distributions of orientation quantified from immunostained neurites. (D) Comparison of normalised distribution factor indicates significantly higher likelihood of alignment on triangular patterned pellets compared with planar pellets, or planar areas on patterned pellets. Planar pellets N = 18, bidirectional triangular pellets N = 6, unidirectional triangular pellets N = 12, planar areas on patterned pellets N = 6. ****p < 0.0001. (E) SEM images with false-coloured (magenta) neuronal cell bodies and neurites of (i) cells on DClear triangular micro-structure, (ii) aligned neurites exiting the edge of the patterned area, (iii) an unpatterned area of D-Clear. Scale bars = 20 μm. | |
To evaluate whether the degree to which neurites align on the triangular patterned surfaces was significant, a series of comparisons were made between three conditions, namely planar pellets (n = 18), triangular-patterned pellets (n = 18), and planar areas on patterned pellets (n = 6). For each condition, additional analyses were carried out on fluorescence localisation of (i) DCX+, a marker for migrating newborn post-mitotic neurons, and (ii) TUJ1+, a marker for maturing post-mitotic neurons, to determine if the effect is prevalent in migrating and maturing neurons. A significant difference was observed between alignment in planar pellets and triangular-patterned pellets in DCX (unpaired T-test, T(34) = 17.40, p < 0.0001) and TUJ1 images (unpaired T-test, T(34) = 12.67, p < 0.0001). As an internal control, triangular-patterned pellets tested against planar areas on patterned pellets displayed significant difference between both DCX (paired T-test, T(5) = 18.62, p < 0.0001) and TUJ1 images (paired T-test, T(5) = 5.683, P < 0.01). However, no significant difference was observed between planar pellets and planar areas on patterned pellets. Further tests performed between DCX and TUJ1 images in the same condition to determine whether neuronal maturity influenced orientation also reported no significant difference between DCX+ migrating newborn and TUJ1+ maturing neurons. Finally, no significant difference in neuronal alignment between unidirectional (n = 12) and bidirectional triangular (n = 6) motifs was observed. Using scanning electron microscopy, we can see that neurites extend along the surface of DClear, and often grown along the side of the triangular micro-pattern (Fig. 5E).
Discussion and conclusions
Human iPSC-derived in vitro CNS models have great potential in enabling the determination of mechanisms of human neuronal function and dysfunction in disease.13,15,21,66,67 However, due to the low complexity of monocultures and variability of patient-derived human cell models in forming neuronal networks, the process of developing novel therapeutics is currently hampered. Organoids, which exhibit more complex structure and cellular interactions have applications for investigating neurodevelopment, however, reported high variability in sample-sample and batch-batch preparations of CNS organoids make them currently less than optimal candidates for robust functional interrogation and standardised therapeutic screening.20,68,69 Micropatterning, 2.5 and 3D scaffolds therefore has great potential in providing a low variability structure on the basis of which defined networks can develop, resulting in predictable networks and a clearer understanding via functional interrogation.33
In this study, we identify two suitable materials for the fabrication of micropatterned surfaces using 2PP to guide human neuronal network development. Dental LT Clear (DClear), and a combination of PEG-DA and Irgacure 2959 were found to meet the required criteria of optical transparency with a low auto-fluorescence profile, amenability to 2PP at the micron scale and biocompatibility with cultured human neurons. These materials were not neurotoxic and permitted long-term adherence and normal differentiation of iPSC-derived neuronal progenitors to mature, functional neurons. PEG has previously been used with cultures of iPSC-derived neurons, however it is usually crosslinked with other molecules (e.g. hyaluronic acid) or as a high intensity phase emulsion (HIPE), and also employed as a hydrogel.31,70–72 PEG2959 has also been reported as suitable for two-photon polymerisation and culture with mouse fibroblasts, endothelial cells and bovine aortic cells, however to our knowledge this study is the first to report its suitability, alongside DClear, for usage as a 2PP substrate for human iPSC-derived neuronal cultures.61,73,74 Accardo et al. reported the development of 3D scaffolds for neuronal cultures from high molecular weight PEGDA (Mw = 700 DA) using Irgacure 819 as photoinitiator. In this study, scaffolds also featured very low auto fluorescence emission and were able to support detailed two-photon microscopy for immunofluorescence analysis of neuroblastoma cells cultured on these scaffolds.42 However, as reported in this and other work, PEGDA formulations are well known to exhibit a water-induced swelling ratio in the range of 11–60%.50,75,76 Such swelling properties of substrates are incompatible with the aim to fabricate micro-patterns with resilient and reproducible geometries when applied to the goal of precise neurite guidance. Material swelling may result in poor adhesion of micro-structures to the substrate, undesired micro-structure deformation, disruption of protein coatings, and even detachment of cell layers during prolonged culture. In our study, it was our intention to use biocompatible polymers with low or non-swelling behaviour (low molecular weight) to later fabricate surface structures of robust, highly reproducible geometry for studying the development of iPSC-derived neuronal networks in vitro. The geometry of 2PP fabricated triangular surface structures from DClear is characterized by a high conformity with the original CAD design (Fig. 5A). This surface patterning could be reproducibly fabricated and applied to the systematic evaluation of patterning effect on alignment of neuronal filaments.
Several previously reported materials used for two-photon polymerisation (e.g. PLA-4Bis, Ormosil-4Bis, PEG369) did not meet the required criteria, including transmission of standard fluorophore emission wavelengths (∼400 nm – 650 nm) and low auto-fluorescence. DClear displayed the lowest relative auto-fluorescence of the polymerised candidate materials tested, and a compatible spectrum of auto-fluorescence for imaging with fluorescent calcium indicators or protein-bound fluorophores. Furthermore, together with PEG2959 it exhibited near total transmission of light across the visible spectrum, making a suitable candidate for building multi-layered scaffolds for optical interrogation. Previous studies have similarly demonstrated the growth of iPSC-derived cells within 2PP fabricated scaffolds, with a notable mention being the development of a scaffold to graft iPSC-derived retinal cells in a physiologically relevant manner.77 However, these two-photon polymerised materials were auto-fluorescent, only allowing observation of the outward face of the scaffold.78–81 The use of DClear and PEG2959 enabled fluorescence calcium imaging at single neuron resolution revealing complex cellular and synchronised network activity. This promises the dual benefits of optical functional interrogation and investigation of protein expression, achievable at a high-throughput level, as well as the expansion of the materials usage towards developing bespoke 3D scaffolds.
The ability to influence neuronal network structural architecture and directionality of neurite outgrowth and cellular positioning is of great scientific and functional interest.34,62,82,83 Control of connectivity between separate populations of neurons is necessary to generate defined neuronal networks, with previous studies reporting neurite-selective or directional innervation using microfluidic devices.14,64,84 Other solutions include micro-contact printing of peptides, proteins and photopolymer resists, nanospun fibres, aligned polymer gels and many more, however resulting interactions are often low complexity, e.g. a single divider between population A and population B, low control, or the method of fabrication would not suit expansion to 3D paradigms.32,38,70,85–95 The benefit of 2PP fabricated structures is that they can be as complex or simple as the experiment necessitates with a micron-scale resolution for printing. Whilst large scale 2PP arrays take considerable time to prepare, a combination approach with UV-polymerised macro structures, as demonstrated in this study, may soon overcome the scalability issue of transitioning from micron to millimetre printing resolution.
Using 2PP micropatterned matrices of low angle of incidence triangles resulted in unidirectional alignment of neurites for up to 500 μm. Triangular surface modifications were chosen based on the hypothesis that during neurite guidance of in vitro neurons, growth cones tend to grow straight, and large turn angles can often be a marker for growth cone collapse or reversal.96,97 Thus the ideal situation was to offer shallow angles in a repeated pattern to encourage straight neurite extension, without forcing the neurites into separate tracts entirely (e.g. by use of a microgroove) as neuronal tracts in the cortex often have overlapping segments. Interestingly, there was no significant difference in neuronal alignment between unidirectional and bidirectional triangular motifs, an unexpected finding as the bidirectional triangular motifs offer frequent potential obstruction, and a lack of “funnelling” structure of gradually tightening restriction when compared with the unidirectional structures. This suggests that alignment was not dependent upon funnelling of neurons, as in a previous study but rather a regularly situated low-angle of incidence obstruction that guides neurite extension.63 Employing similar, yet more complex 2PP matrices to influence neurite extension over long distances may be a useful approach for developing optimal topography for programmed synaptic formation, resulting in reproducible neural networks with definable functional architectures.
In conclusion, DClear and PEG2959 represent suitable polymers for two-photon polymerisation of patterning at the micron scale, allowing the establishment of neuronal networks, and optical interrogation for functional and morphological studies. To our knowledge, this study is the first to report the growth of iPSC-derived cortical neurons on 2PP micropatterned surfaces, as well as the first to optically observe functionality of human iPSC-derived neurons upon suitable materials for two-photon polymerisation. Additionally, DClear has not previously been used to produce 2PP generated micropatterned surfaces or scaffolds for cell culture. Its properties met all our set criteria for a biocompatible 2PP scaffold material and its status Class IIa biocompatible resin with approval for prolonged contact with human tissues makes long term pharmacological/tissue engineering studies feasible. The compatible combination of iPSC-derived neural cultures with optically-interrogatable materials that can be intricately patterned to influence network architecture represents a promising advance in the development of 3D scaffolds for structurally-defined and reproducible network development.
Data availability
Data will be made available via Aston University's Data Explorer repository.
Author contributions
J. A. Crowe: conceptualization, methodology, formal analysis, investigation, data curation, writing – original draft, writing – review and editing, visualization. A. El-Tamer: Conceptualization, methodology, software, formal analysis, investigation, data curation, writing – original draft, writing – review and editing. D. Nagel: Methodology, investigation, conceptualization, funding acquisition, supervision. A. Koroleva: Investigation, writing – original draft, writing – review & editing, visualization, supervision. J. A. M. Wolff: Formal analysis, investigation, writing – original draft, data curation, visualization. O. E. Olarte: Formal analysis, investigation, writing – original draft, data curation, visualization. S. Sokolovsky: Funding acquisition. E. Estevez-Priego: Software, validation, writing – review & editing. A.-A. Ludl: Software, validation, writing – review & editing, validation, formal analysis. J. Soriano: Conceptualization, writing – review & editing, supervision, project administration, funding acquisition. P. Loza-Alvarez: Resources, conceptualization, writing – review & editing, supervision, project administration, funding acquisition. B. N. Chichkov: Resources, supervision, project administration, funding acquisition. E. U. Rafailov: Project administration, funding acquisition. E. J. Hill: Conceptualization, resources, writing – original draft, writing – review & editing, visualization, supervision, project administration, funding acquisition. H. R. Parri: Conceptualization, resources, writing – original draft, writing – review and editing, supervision, visualization, project administration, funding acquisition.
Conflicts of interest
Prof B. N. Chichkov is Scientific Director at Laser nanoFab GmbH (Hannover, Germany), a commercial company specialising in nanofabrication via two-photon microscopy systems, and commercial sales of the systems.
Acknowledgements
This project has received funding from the European Union's Horizon 2020 research and innovation programme under the grant agreement MESOBRAIN No 713140. JS and EE acknowledge financial support from the Spanish Ministerio de Economia y Competitividad through projects no. FIS2013-41144-P, FIS2016-78507-C2-2-P and FIS2017-90782-REDT (IBERSINC), and from the Generalitat de Catalunya through grant no. 2017-SGR-1061. PL-A, OO and JM-W acknowledge financial support from the Spanish Ministerio de Economia y Competitividad through the “Severo Ochoa” program for Centres of Excellence in R&D (SEV-2015-0522, FIS2016-80455-R; AEI/FEDER, UE), Fundació Privada Cellex, Fundacio'n Mig-Puig and from Generalitat de Catalunya through the “CERCA program” and EU H2020 LaserLab Europe grant 654148. EUR and BNC acknowledge further financial support from the European Union's Horizon 2020 programme under the grant agreement SCAFFOLD-NEEDS No. 851734. We thank Charlie Clark-Bland, Aston University, for assistance with confocal imaging.
References
- S. Trautmann, J. Rehm and H. U. Wittchen, EMBO Rep., 2016, 17, 1245–1249 CrossRef CAS PubMed.
- A. Gustavsson, M. Svensson, F. Jacobi, C. Allgulander, J. Alonso, E. Beghi, R. Dodel, M. Ekman, C. Faravelli, L. Fratiglioni, B. Gannon, D. H. Jones, P. Jennum, A. Jordanova, L. Jönsson, K. Karampampa, M. Knapp, G. Kobelt, T. Kurth, R. Lieb, M. Linde, C. Ljungcrantz, A. Maercker, B. Melin, M. Moscarelli, A. Musayev, F. Norwood, M. Preisig, M. Pugliatti, J. Rehm, L. Salvador-Carulla, B. Schlehofer, R. Simon, H. C. Steinhausen, L. J. Stovner, J. M. Vallat, P. Van den Bergh, P. V. den Bergh, J. van Os, P. Vos, W. Xu, H. U. Wittchen, B. Jönsson, J. Olesen and C. S. Group, Eur. Neuropsychopharmacol., 2011, 21, 718–779 CrossRef CAS PubMed.
- M. DiLuca and J. Olesen, Neuron, 2014, 82, 1205–1208 CrossRef CAS PubMed.
- B. P. Grone and S. C. Baraban, Nat. Neurosci., 2015, 18, 339–343 CrossRef CAS PubMed.
- L. Kandratavicius, P. A. Balista, C. Lopes-Aguiar, R. N. Ruggiero, E. H. Umeoka, N. Garcia-Cairasco, L. S. Bueno-Junior and J. P. Leite, Neuropsychiatr. Dis. Treat., 2014, 10, 1693–1705 CrossRef PubMed.
- T. M. Dawson, T. E. Golde and C. Lagier-Tourenne, Nat. Neurosci., 2018, 21, 1370–1379 CrossRef CAS PubMed.
- E. J. Nestler and S. E. Hyman, Nat. Neurosci., 2010, 13, 1161–1169 CrossRef CAS PubMed.
- K. J. Brennand, M. C. Marchetto, N. Benvenisty, O. Brustle, A. Ebert, J. C. Izpisua Belmonte, A. Kaykas, M. A. Lancaster, F. J. Livesey, M. J. McConnell, R. D. McKay, E. M. Morrow, A. R. Muotri, D. M. Panchision, L. L. Rubin, A. Sawa, F. Soldner, H. Song, L. Studer, S. Temple, F. M. Vaccarino, J. Wu, P. Vanderhaeghen, F. H. Gage and R. Jaenisch, Stem Cell Rep., 2015, 5, 933–945 CrossRef PubMed.
- K. J. Brennand, A. Simone, N. Tran and F. H. Gage, Mol. Psychiatry, 2012, 17, 1239–1253 CrossRef CAS PubMed.
- A. Falk, V. M. Heine, A. J. Harwood, P. F. Sullivan, M. Peitz, O. Brüstle, S. Shen, Y. M. Sun, J. C. Glover, D. Posthuma and S. Djurovic, Mol. Psychiatry, 2016, 21, 1321 CrossRef CAS PubMed.
- A. I. Grainger, M. C. King, D. A. Nagel, H. R. Parri, M. D. Coleman and E. J. Hill, Front. Neurosci., 2018, 12, 590 CrossRef PubMed.
- E. A. LaMarca, S. K. Powell, S. Akbarian and K. J. Brennand, Front. Pediatr., 2018, 6, 82 CrossRef PubMed.
- E. J. Hill, C. Jiménez-González, M. Tarczyluk, D. A. Nagel, M. D. Coleman and H. R. Parri, PLoS One, 2012, 7, e36098 CrossRef CAS PubMed.
- J. H. George, D. Nagel, S. Waller, E. Hill, H. R. Parri, M. D. Coleman, Z. Cui and H. Ye, Sci. Rep., 2018, 8, 15552 CrossRef PubMed.
- K. J. Yoon, H. N. Nguyen, G. Ursini, F. Zhang, N. S. Kim, Z. Wen, G. Makri, D. Nauen, J. H. Shin, Y. Park, R. Chung, E. Pekle, C. Zhang, M. Towe, S. M. Hussaini, Y. Lee, D. Rujescu, D. St Clair, J. E. Kleinman, T. M. Hyde, G. Krauss, K. M. Christian, J. L. Rapoport, D. R. Weinberger, H. Song and G. L. Ming, Cell Stem Cell, 2014, 15, 79–91 CrossRef CAS PubMed.
- D. St Clair and M. Johnstone, Philos. Trans. R. Soc., B, 2018, 373(1742), 20170037 CrossRef PubMed.
- T. Kadoshima, H. Sakaguchi, T. Nakano, M. Soen, S. Ando, M. Eiraku and Y. Sasai, Proc. Natl. Acad. Sci. U. S. A., 2013, 110, 20284–20289 CrossRef CAS PubMed.
- I. Kelava and M. A. Lancaster, Dev. Biol., 2016, 420, 199–209 CrossRef CAS PubMed.
- I. Kelava and M. A. Lancaster, Cell Stem Cell, 2016, 18, 736–748 CrossRef CAS PubMed.
- N. de Souza, Nat. Methods, 2017, 14, 655 CrossRef CAS.
- X. Qian, H. N. Nguyen, M. M. Song, C. Hadiono, S. C. Ogden, C. Hammack, B. Yao, G. R. Hamersky, F. Jacob, C. Zhong, K. J. Yoon, W. Jeang, L. Lin, Y. Li, J. Thakor, D. A. Berg, C. Zhang, E. Kang, M. Chickering, D. Nauen, C. Y. Ho, Z. Wen, K. M. Christian, P. Y. Shi, B. J. Maher, H. Wu, P. Jin, H. Tang, H. Song and G. L. Ming, Cell, 2016, 165, 1238–1254 CrossRef CAS PubMed.
- M. Huch, J. A. Knoblich, M. P. Lutolf and A. Martinez-Arias, Development, 2017, 144, 938 CrossRef CAS PubMed.
- A. M. Hopkins, E. DeSimone, K. Chwalek and D. L. Kaplan, Prog. Neurobiol., 2015, 125, 1–25 CrossRef PubMed.
- M. D. Tang-Schomer, J. D. White, L. W. Tien, L. I. Schmitt, T. M. Valentin, D. J. Graziano, A. M. Hopkins, F. G. Omenetto, P. G. Haydon and D. L. Kaplan, Proc. Natl. Acad. Sci. U. S. A., 2014, 111, 13811–13816 CrossRef CAS PubMed.
- R. D. Abbott, E. P. Kimmerling, D. M. Cairns and D. L. Kaplan, ACS Appl. Mater. Interfaces, 2016, 8, 21861–21868 CrossRef CAS PubMed.
- M. A. Lancaster, N. S. Corsini, S. Wolfinger, E. H. Gustafson, A. W. Phillips, T. R. Burkard, T. Otani, F. J. Livesey and J. A. Knoblich, Nat. Biotechnol., 2017, 35, 659–666 CrossRef CAS PubMed.
- R. J. McMurtrey, J. Tissue Eng., 2016, 7, 2041731416671926 Search PubMed.
- A. Koroleva, A. A. Gill, I. Ortega, J. W. Haycock, S. Schlie, S. D. Gittard, B. N. Chichkov and F. Claeyssens, Biofabrication, 2012, 4, 025005 CrossRef CAS PubMed.
- M. Kamudzandu, Y. Yang, P. Roach and R. A. Fricker, RSC Adv., 2015, 5, 22053–22059 RSC.
- B. Luo, L. Tian, N. Chen, S. Ramakrishna, N. Thakor and I. H. Yang, Biomater. Sci., 2018, 6, 3262–3272 RSC.
- P. Madhusudanan, G. Raju and S. Shankarappa, J. R. Soc., Interface, 2020, 17, 20190505 CrossRef PubMed.
- J. R. Ryu, J. H. Kim, H. M. Cho, Y. Jo, B. Lee, S. Joo, U. Chae, Y. Nam, I.-J. Cho and W. Sun, Lab Chip, 2019, 19, 291–305 RSC.
- P. Zhuang, A. X. Sun, J. An, C. K. Chua and S. Y. Chew, Biomaterials, 2018, 154, 113–133 CrossRef CAS PubMed.
- A. Accardo, C. Cirillo, S. Lionnet, C. Vieu and I. Loubinoux, Brain Res. Bull., 2019, 152, 202–211 CrossRef CAS PubMed.
- M. Pardo-Figuerez, N. R. W. Martin, D. J. Player, P. Roach, S. D. R. Christie, A. J. Capel and M. P. Lewis, ACS Omega, 2018, 3, 12383–12391 CrossRef CAS PubMed.
- A. K. Yip, A. T. Nguyen, M. Rizwan, S. T. Wong, K. H. Chiam and E. K. F. Yim, Biomaterials, 2018, 181, 103–112 CrossRef CAS PubMed.
- A. T. Nguyen, S. R. Sathe and E. K. Yim, J. Phys.: Condens. Matter, 2016, 28, 183001 CrossRef PubMed.
- F. Yang, R. Murugan, S. Wang and S. Ramakrishna, Biomaterials, 2005, 26, 2603–2610 CrossRef CAS PubMed.
- H. N. Kim, A. Jiao, N. S. Hwang, M. S. Kim, D. H. Kang, D. H. Kim and K. Y. Suh, Adv. Drug Delivery Rev., 2013, 65, 536–558 CrossRef CAS PubMed.
- M. Farsari and B. N. Chichkov, Nat. Photonics, 2009, 3, 450 CrossRef CAS.
- V. Melissinaki, A. A. Gill, I. Ortega, M. Vamvakaki, A. Ranella, J. W. Haycock, C. Fotakis, M. Farsari and F. Claeyssens, Biofabrication, 2011, 3, 045005 CrossRef CAS PubMed.
- A. Accardo, M.-C. Blatché, R. Courson, I. Loubinoux, C. Vieu and L. Malaquin, Biomed. Phys. Eng. Express, 2018, 4, 027009 CrossRef.
- H. Amin, A. Maccione, F. Marinaro, S. Zordan, T. Nieus and L. Berdondini, Front. Neurosci., 2016, 10, 121 Search PubMed.
- J. O. Muthmann, H. Amin, E. Sernagor, A. Maccione, D. Panas, L. Berdondini, U. S. Bhalla and M. H. Hennig, Front. Neuroinform., 2015, 9, 28 Search PubMed.
- H. H. Yang and F. St-Pierre, J. Neurosci., 2016, 36, 9977–9989 CrossRef CAS PubMed.
- A. Berndt, P. Schoenenberger, J. Mattis, K. M. Tye, K. Deisseroth, P. Hegemann and T. G. Oertner, Proc. Natl. Acad. Sci. U. S. A., 2011, 108, 7595–7600 CrossRef CAS PubMed.
- T. W. Chen, T. J. Wardill, Y. Sun, S. R. Pulver, S. L. Renninger, A. Baohan, E. R. Schreiter, R. A. Kerr, M. B. Orger, V. Jayaraman, L. L. Looger, K. Svoboda and D. S. Kim, Nature, 2013, 499, 295–300 CrossRef CAS PubMed.
- M. B. Ahrens, M. B. Orger, D. N. Robson, J. M. Li and P. J. Keller, Nat. Methods, 2013, 10, 413–420 CrossRef CAS PubMed.
- A. Ovsianikov, J. Viertl, B. Chichkov, M. Oubaha, B. MacCraith, I. Sakellari, A. Giakoumaki, D. Gray, M. Vamvakaki, M. Farsari and C. Fotakis, ACS Nano, 2008, 2, 2257–2262 CrossRef CAS PubMed.
- A. Ovsianikov, M. Malinauskas, S. Schlie, B. Chichkov, S. Gittard, R. Narayan, M. Lobler, K. Sternberg, K. P. Schmitz and A. Haverich, Acta Biomater., 2011, 7, 967–974 CrossRef CAS PubMed.
- P. Timashev, D. Kuznetsova, A. Koroleva, N. Prodanets, A. Deiwick, Y. Piskun, K. Bardakova, N. Dzhoyashvili, S. Kostjuk, E. Zagaynova, Y. Rochev, B. Chichkov and V. Bagratashvili, Nanomedicine, 2016, 11, 1041–1053 CrossRef CAS PubMed.
- D. Kuznetsova, A. Ageykin, A. Koroleva, A. Deiwick, A. Shpichka, A. Solovieva, S. Kostjuk, A. Meleshina, S. Rodimova, A. Akovanceva, D. Butnaru, A. Frolova, E. Zagaynova, B. Chichkov, V. Bagratashvili and P. Timashev, Biofabrication, 2017, 9, 025009 CrossRef CAS PubMed.
- A. Koroleva, A. Deiwick, A. Nguyen, S. Schlie-Wolter, R. Narayan, P. Timashev, V. Popov, V. Bagratashvili and B. Chichkov, PLoS One, 2015, 10, e0118164 CrossRef PubMed.
- Y. Shi, P. Kirwan, J. Smith, H. P. Robinson and F. J. Livesey, Nat. Neurosci., 2012, 15, 477–486 CrossRef CAS PubMed , S471.
- D. Fan, S. Urs and A. Angelo, Bioengineering, 2019, 6, 113 CrossRef PubMed.
- T. M. Pirttimaki and H. R. Parri, Neuroscience, 2012, 205, 18–28 CrossRef CAS PubMed.
- T. M. Pirttimaki, R. E. Sims, G. Saunders, S. A. Antonio, N. K. Codadu and H. R. Parri, J. Neurosci., 2017, 37, 9859–9870 CrossRef CAS PubMed.
- J. Schindelin, I. Arganda-Carreras, E. Frise, V. Kaynig, M. Longair, T. Pietzsch, S. Preibisch, C. Rueden, S. Saalfeld, B. Schmid, J. Y. Tinevez, D. J. White, V. Hartenstein, K. Eliceiri, P. Tomancak and A. Cardona, Nat. Methods, 2012, 9, 676–682 CrossRef CAS PubMed.
- C. T. Rueden, J. Schindelin, M. C. Hiner, B. E. DeZonia, A. E. Walter, E. T. Arena and K. W. Eliceiri, BMC Bioinf., 2017, 18, 529 CrossRef PubMed.
- R. Rezakhaniha, A. Agianniotis, J. T. Schrauwen, A. Griffa, D. Sage, C. V. Bouten, F. N. van de Vosse, M. Unser and N. Stergiopulos, Biomech. Model. Mechanobiol., 2012, 11, 461–473 CrossRef CAS PubMed.
- A. K. Nguyen and R. J. Narayan, Mater. Today, 2017, 20, 314–322 CrossRef CAS.
- L. J. Millet and M. U. Gillette, Trends Neurosci., 2012, 35, 752–761 CrossRef CAS PubMed.
- J. M. Peyrin, B. Deleglise, L. Saias, M. Vignes, P. Gougis, S. Magnifico, S. Betuing, M. Pietri, J. Caboche, P. Vanhoutte, J. L. Viovy and B. Brugg, Lab Chip, 2011, 11, 3663–3673 RSC.
- J. Harris, H. Lee, B. Vahidi, C. Tu, D. Cribbs, N. L. Jeon and C. Cotman, J. Visualized Exp., 2007, 7, e261 Search PubMed.
- M. Kamudzandu, M. Köse-Dunn, M. G. Evans, R. A. Fricker and P. Roach, Biomed. Phys. Eng. Express, 2019, 5, 045016 CrossRef.
- M. A. Lancaster, M. Renner, C. A. Martin, D. Wenzel, L. S. Bicknell, M. E. Hurles, T. Homfray, J. M. Penninger, A. P. Jackson and J. A. Knoblich, Nature, 2013, 501, 373–379 CrossRef CAS PubMed.
- S. P. Pasca, T. Portmann, I. Voineagu, M. Yazawa, A. Shcheglovitov, A. M. Pasca, B. Cord, T. D. Palmer, S. Chikahisa, S. Nishino, J. A. Bernstein, J. Hallmayer, D. H. Geschwind and R. E. Dolmetsch, Nat. Med., 2011, 17, 1657–1662 CrossRef CAS PubMed.
- J. Mariani, G. Coppola, P. Zhang, A. Abyzov, L. Provini, L. Tomasini, M. Amenduni, A. Szekely, D. Palejev, M. Wilson, M. Gerstein, E. L. Grigorenko, K. Chawarska, K. A. Pelphrey, J. R. Howe and F. M. Vaccarino, Cell, 2015, 162, 375–390 CrossRef CAS PubMed.
- A. M. Pasca, S. A. Sloan, L. E. Clarke, Y. Tian, C. D. Makinson, N. Huber, C. H. Kim, J. Y. Park, N. A. O'Rourke, K. D. Nguyen, S. J. Smith, J. R. Huguenard, D. H. Geschwind, B. A. Barres and S. P. Pasca, Nat. Methods, 2015, 12, 671–678 CrossRef CAS PubMed.
- Z. N. Zhang, B. C. Freitas, H. Qian, J. Lux, A. Acab, C. A. Trujillo, R. H. Herai, V. A. Nguyen Huu, J. H. Wen, S. Joshi-Barr, J. V. Karpiak, A. J. Engler, X. D. Fu, A. R. Muotri and A. Almutairi, Proc. Natl. Acad. Sci. U. S. A., 2016, 113, 3185–3190 CrossRef CAS PubMed.
- A. R. Murphy, I. Ghobrial, P. Jamshidi, A. Laslett, C. M. O'Brien and N. R. Cameron, Polym. Chem., 2017, 8, 6617–6627 RSC.
- M. W. Hayman, K. H. Smith, N. R. Cameron and S. A. Przyborski, J. Biochem. Biophys. Methods, 2005, 31, 231–240 CrossRef PubMed.
- A. Ovsianikov, M. Malinauskas, S. Schlie, B. Chichkov, S. Gittard, R. Narayan, M. Löbler, K. Sternberg, K. P. Schmitz and A. Haverich, Acta Biomater., 2011, 7, 967–974 CrossRef CAS PubMed.
- A. K. Nguyen, S. D. Gittard, A. Koroleva, S. Schlie, A. Gaidukeviciute, B. N. Chichkov and R. J. Narayan, Regener. Med., 2013, 8, 725–738 CrossRef CAS PubMed.
- K. McAvoy, D. Jones and R. R. S. Thakur, Pharm. Res., 2018, 35, 36 CrossRef PubMed.
- H. Seo, S. G. Heo, H. Lee and H. Yoon, RSC Adv., 2017, 7, 28684–28688 RSC.
- K. S. Worthington, L. A. Wiley, E. E. Kaalberg, M. M. Collins, R. F. Mullins, E. M. Stone and B. A. Tucker, Acta Biomater., 2017, 55, 385–395 CrossRef CAS PubMed.
- Z. Ma, S. Koo, M. A. Finnegan, P. Loskill, N. Huebsch, N. C. Marks, B. R. Conklin, C. P. Grigoropoulos and K. E. Healy, Biomaterials, 2014, 35, 1367–1377 CrossRef CAS PubMed.
- M. M. Nava, A. Piuma, M. Figliuzzi, I. Cattaneo, B. Bonandrini, T. Zandrini, G. Cerullo, R. Osellame, A. Remuzzi and M. T. Raimondi, Stem Cell Res. Ther., 2016, 7, 132 CrossRef PubMed.
- K. S. Worthington, A.-V. Do, R. Smith, B. A. Tucker and A. K. Salem, Macromol. Biosci., 2019, 19, 1800370 CrossRef PubMed.
- A.-V. Do, K. S. Worthington, B. A. Tucker and A. K. Salem, Int. J. Pharm., 2018, 552, 217–224 CrossRef CAS PubMed.
- A. Farrukh, S. Zhao and A. del Campo, Front. Mater., 2018, 5, 62 CrossRef.
- L. J. Millet, M. B. Collens, G. L. Perry and R. Bashir, Integr. Biol., 2011, 3, 1167–1178 CrossRef CAS PubMed.
- S. Bang, S. Na, J. M. Jang, J. Kim and N. L. Jeon, Adv. Healthcare Mater., 2016, 5, 159–166 CrossRef CAS PubMed.
- A. Solanki, S. T. Chueng, P. T. Yin, R. Kappera, M. Chhowalla and K. B. Lee, Adv. Mater., 2013, 25, 5477–5482 CrossRef CAS PubMed.
- C. D. James, R. Davis, M. Meyer, A. Turner, S. Turner, G. Withers, L. Kam, G. Banker, H. Craighead, M. Isaacson, J. Turner and W. Shain, IEEE Trans. Biomed. Eng., 2000, 47, 17–21 CrossRef CAS PubMed.
- R. Fricke, P. D. Zentis, L. T. Rajappa, B. Hofmann, M. Banzet, A. Offenhäusser and S. H. Meffert, Biomaterials, 2011, 32, 2070–2076 CrossRef CAS PubMed.
- T. Honegger, M. I. Thielen, S. Feizi, N. E. Sanjana and J. Voldman, Sci. Rep., 2016, 6, 28384 CrossRef CAS PubMed.
- N. E. Sanjana and S. B. Fuller, J. Neurosci. Methods, 2004, 136, 151–163 CrossRef PubMed.
- R. Beighley, E. Spedden, K. Sekeroglu, T. Atherton, M. C. Demirel and C. Staii, Appl. Phys. Lett., 2012, 101, 143701 CrossRef PubMed.
- M. D. Tang-Schomer, Brain Res., 2018, 1678, 288–296 CrossRef CAS PubMed.
- F. Larramendy, S. Yoshida, L. Jalabert, S. Takeuchi and O. Paul, J. Micromech. Microeng., 2016, 26, 095017 CrossRef.
- G. T. Christopherson, H. Song and H. Q. Mao, Biomaterials, 2009, 30, 556–564 CrossRef CAS PubMed.
- J. Ko, N. K. Mohtaram, F. Ahmed, A. Montgomery, M. Carlson, P. C. Lee, S. M. Willerth and M. B. Jun, J. Biomater. Sci., Polym. Ed., 2014, 25, 1–17 CrossRef CAS.
- N. K. Mohtaram, J. Ko, C. King, L. Sun, N. Muller, M. B. Jun and S. M. Willerth, J. Biomed. Mater. Res., Part A, 2015, 103, 2591–2601 CrossRef CAS PubMed.
- S. Geraldo and P. R. Gordon-Weeks, J. Cell Sci., 2009, 122, 3595 CrossRef CAS PubMed.
- S. M. Maskery, H. M. Buettner and T. Shinbrot, BMC Neurosci., 2004, 5, 22 CrossRef.
|
This journal is © The Royal Society of Chemistry 2020 |
Click here to see how this site uses Cookies. View our privacy policy here.