DOI:
10.1039/C7RA06503E
(Paper)
RSC Adv., 2017,
7, 37636-37642
Hormesis of some organic solvents on Vibrio qinghaiensis sp.-Q67 from first binding to the β subunit of luciferase†
Received
11th June 2017
, Accepted 17th July 2017
First published on 31st July 2017
Abstract
Hormesis is a biphasic concentration–response relationship. During the luminescence inhibition test of Vibrio qinghaiensis sp.-Q67 (Q67), some organic solvents display the hormesis phenomenon. However, the mechanism of hormesis with respect to organic solvents remains unclear. This study focuses on luciferase, which is the key factor in luminescent reactions, and explores its role in the mechanism of hormesis. Q67 luciferase has two subunits, α and β. Molecular docking and molecular dynamics simulations were carried out by taking organic solvents as ligand and the two subunits as receptor. In addition, the binding free energies of the complexes formed by the ligand and Q67 luciferase were calculated. The results showed that the organic solvent ligands exhibiting hormesis bind to the β subunit first, while those that do not exhibit hormesis bind more easily to the α subunit. The hormetic organic solvents bind to β subunit first at low concentration, and change the flexibility of residues Ser145–Arg165 located on the α subunit; this enables flavin mononucleotide (FMN) to bind to the α subunit, exhibiting the hormesis phenomenon. With the increasing concentration, redundant molecules start to bind to the α subunit and compete with/block FMN binding to the α subunit, resulting in inhibition.
Introduction
Hormesis is a biphasic concentration–response phenomenon, which shows beneficial effects at low-concentrations and inhibition at high-concentrations.1 The hormetic concentration response (HCR) is different from the S-shaped concentration response curve (CRC) in terms of threshold level in the classical monotonic model, which challenges the way of chemical risk assessment.2 Calabrese et al.3 suggested that hormesis is a generalized phenomenon. Thus far, in numerous studies, many chemicals, such as antibiotics,4 endocrine disruptors5 and pesticides,6 have shown hormesis. In our previous study, we also observed hormesis of some ionic liquids (ILs)7–10 and organic solvents11 to Vibrio qinghaiensis sp.-Q67 (Q67).
The mainstream theories regarding the mechanism of hormesis are overcompensation and direct stimulation.12 At the molecular level, receptor-mediated and cell signalling-mediated mechanisms are the main theories to interpret hormesis.13 However, for different toxic endpoints, the specific mechanisms of hormesis are different.14 Morre et al.15 suggested that the TIP/NOX protein was the molecular target of the biological effects of hormesis involved in the stimulation of plant growth. Chen et al.16 speculated that the occupation of the AMP pocket by the imidazolium ring is responsible for hormetic stimulation. Therefore, the mechanisms of hormesis are different for diverse targets. In addition, different chemicals such as organic solvents and ILs may have varying mechanisms of hormesis for the same tested organism. When Q67 was the tested organism and luminescence inhibition was the toxicity endpoint, some organic solvents exhibited the hormesis phenomenon in the short-term (15 min) toxicity test,11 but ionic liquids did not show hormesis until after exposure for 12 h (the long-term toxicity test).9
Some researchers found a common pattern;17 the hormesis of 1-alkyl-3-methylimidazolium chloride on Q67 was accompanied by the stimulation of flavin mononucleotide (FMN)/nicotinamide adenine dinucleotide (NADH) and antioxidant inductions. However, the mechanism of hormesis with respect to organic solvents remains elusive during the luminescence inhibition test on bioluminescent bacteria. Based on our previous studies,11 we screened some organic solvents with good water solubility that are widely used in the pharmaceutical industry, electronics industry and oil processing.18 Herein, we classified these organic solvents with respect to their CRCs.
Different types of bioluminescent bacteria share the same luminescence mechanism.19 They produce blue-green light with a wavelength of 450–490 nm by employing luciferase as the catalyst and long-chain aliphatic aldehydes (RCHO), dioxygen and FMN as the substrates.20,21 The reaction proceeds as follows:
|
 | (1) |
Therefore, luciferase as catalyst is vital for the luminescence process. In this context, it is of interest to identify whether the different binding patterns of organic solvents and Q67 luciferase lead to different concentration–response relationships. The Q67 luciferase has two subunits, α and β (Fig. 1),22 which we named as Q67Lucα and Q67Lucβ. As the natural substrates of the luminous reaction in photobacterium, FMN binds to Q67Lucα.22
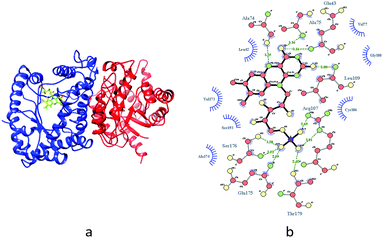 |
| Fig. 1 (a) The complex of FMN and luciferase in Q67, where the left part (blue) of the ribbon structure and the right part (red) represent the α and β subunits, respectively. (b) The hydrogen bond contact between FMN and the side chain and backbone of binding residues in the α subunits is expressed by green lines and hydrophobic contact groups are expressed by eyelash shaped curves. | |
Molecular docking23 examines whether the two molecules can bind and predicts the binding mode based on the three-dimensional structures of molecules. Docking methods are fast and often able to identify the correct binding site and ligand poses, though they rarely yield accurate binding affinities. Molecular dynamics (MD) simulations, particularly the binding free energy simulation module, can yield more information to help us understand protein function through the modelling of the protein stable states24 and ligand–protein binding affinities.25 However, the accuracy of MD depends on the optimization of force fields26,27 and the simulation time scale is limited by computer performance. The combination of molecular docking and MD is often used to reveal the mechanism of toxic pollutants or hazardous materials in toxicology and environmental science.28–30
In this study, we first conducted the toxicity test to verify the different concentration–response relationships of organic solvents to Q67. Then, we docked the organic solvents to Q67Lucα and Q67Lucβ separately and applied molecular dynamics to simulate the interaction. Finally, the binding free energies were calculated by Molecular Mechanics/Generalized Born Surface Area (MM/GBSA) energy calculations.31 Analysis of the results of the toxicity test and molecular simulations revealed the mechanism of hormesis displayed by the organic solvents during the Q67 toxicity test. When the CRC of hormesis is J-shaped, the corresponding organic solvents are called J-shaped organic solvents. Similarly, organic solvents with S-shaped CRCs are called S-shaped solvents.
Experimental
Chemicals
Eight organic solvents were selected in this study: tetrahydrofuran (THF), isopropanol, acetone, methanol, formaldehyde, phenol, ethyl acetate (EAC) and dimethyl sulfoxide (DMSO). All solutions were prepared with Milli-Q water and stored in darkness. Some physical properties of the chemicals are listed in Table 1.
Table 1 Basic information regarding the eight organic solvents
Name |
Formula |
CAS |
MWa (g mol−1) |
Source |
Purity |
MW refers to molecular weight. |
Tetrahydrofuran (THF) |
C4H8O |
109-99-9 |
72.11 |
Sigma-Aldrich |
≥99.9% |
Isopropanol |
(CH3)2CHOH |
67-63-0 |
60.1 |
Aladdin |
≥99.5% |
Acetone |
CH3COCH3 |
67-64-1 |
58.08 |
Sinopharm |
≥99.5% |
Methanol |
CH3OH |
67-56-1 |
32.04 |
Sigma-Aldrich |
≥99.9% |
Formaldehyde |
HCHO |
50-00-0 |
30.03 |
Aladdin |
10.4 mg mL−1 |
Phenol |
C6H5OH |
108-95-2 |
94.11 |
Aladdin |
≥99.5% |
Ethyl acetate (EAC) |
C4H8O2 |
141-78-6 |
88.11 |
Sigma-Aldrich |
≥99.8% |
Dimethyl sulfoxide (DMSO) |
C2H6OS |
67-68-5 |
78.13 |
Sigma |
≥99.5% |
Toxicity test
The freeze-dried luminescent bacterium Vibrio qinghaiensis sp.-Q67 was purchased from Beijing Hamamatsu Corp., Ltd. (Beijing, China). The components and preparation of the culture medium were the same as reported in the literature.32 The methods of bacteria activation, inoculation and culture were based on Wang's research.33
Microplate toxicity analysis (MTA) was used to determine the effect of organic solvents on Q67. The test details are described in our previous study.34,35 The effect (E) is expressed as a percentage inhibition of bioluminescence of Q67, which is calculated as follows:
|
 | (2) |
where
I0 is an average of the relative light units (RLU) of Q67 exposed to the controls (12 parallels), and
I is an average of RLU to the test chemical or mixture (three parallels) in one microplate.
Concentration–response curve (CRC) characterization
The CRCs of the organic solvents to Q67 were modelled by the nonlinear least squares fit.36 The monotonic (S-shaped) CRC was fitted by the assessment and prediction of the toxicity of chemical mixture (APTox) program,37 while the non-monotonic (J-shaped) CRC was fitted by the least squares support vector regression (LSSVR) procedure.38 The uncertainty of the experimental concentration inhibition was expressed as the 95% observation-based confidence intervals (OCIs).39,40 The goodness of fit was described by the determination coefficient (R2) and root mean square error (RMSE).
Molecular docking and molecular dynamics simulation
The atomic coordinates of Q67 luciferase with FMN (flavin mononucleotide) were taken from the structure file constructed by Chen et al.22 We named the subunits of Q67 luciferase as Q67Lucα and Q67Lucβ. The molecular docking and molecular dynamics (MD) simulations were used to simulate the structure of the organic solvent–luciferase complex. The ligand files for eight organic solvents were read in Chimera 1.10.2,41 all of the hydrogen atoms and AM1-BCC42 charges were added, and non-polar hydrogen atoms were merged.
UCSF DOCK (version 6.6)43 was run to dock the ligand into Q67Lucα. A subset of spheres within 8.0 Å around FMN in Q67Lucα was selected to represent the binding site. The scoring grids were calculated using the accessory program GRID. Flexible ligand docking was performed and the maximum orientation was set as 50
000. The grid score was the first filtration and Amber score was the second. The conformation with the lowest negative Amber score was chosen as the initial conformation for MD. To identify the accuracy of molecular docking, we docked FMN with Q67 luciferase, and the result is shown in Fig. S1.† Thus, the best Amber score conformation overlaps well with original conformation, and the RMSD is 0.8 Å, less than the docking credibility scope of RMSD ≤ 2.0 Å,44 which implies that the docking method is credible.
The structure of ligand–Lucβ was obtained using a semi-flexible docking approach with AutoDockVina.45 Q67Lucβ is used as a receptor and considered to be fully rigid, while the organic solvent ligand is flexible. For the docking calculations, a box of size 40 × 40 × 40 Å was used, centred at the geometric centre of the ligand–Lucβ structure. The exhaustiveness value (exhaustiveness of finding the global minimum) was changed to 25 (default is 8), and the program was allowed to generate 10 binding modes (default is 9). The maximum energy difference between the best binding mode and the worst displayed was 3 kcal mol−1. The docking pose with the lowest negative score (highest binding affinity) was chosen as the initial conformation for MD.
MD was performed using the AMBER 12 software package.46 The organic solvent–Q67 luciferase (Q67Lucα or Q67Lucβ) complex (selecting the general AMBER force field (GAFF)47 and RESP charges for the organic solvent ligand and ff12SB force field48 for Q67 luciferase) was solvated by TIP3P water molecules, with a minimum distance of 8.5 Å from the complex surface. The system was gradually heated from 0 to 300 K within 50 ps and subsequently simulated at 300 K for the equilibration and production phases.22 The ptraj module was used to analyze the root mean-square displacements (RMSD) between the trajectory structures and the first snapshot structure in 1st ns trajectory and root mean-square fluctuation (RMSF) of various residues. All systems were equilibrated at 5 ns, and the MDs were prolonged for another 3 ns. One hundred snapshots of the simulated structures were sampled within the last 1 ns with a step of 10 ps.
Binding free energy and its components
The MM/GBSA procedure49–51 was employed to calculate the binding free energies (ΔG) of the organic solvents bound to Q67 luciferase using 100 snapshots of each complex (every 10 ps) generated from the last 1 ns MD trajectories.
Results and discussion
Concentration–response relationships of eight organic solvents
The concentration–response curves (CRCs) of the organic solvents on Q67 are monotonic (S-shaped) for THF, isopropanol, acetone and methanol and non-monotonic (J-shaped) for formaldehyde, phenol, EAC and DMSO (Fig. 2). The statistical results are listed in Table 2, and the values of RMSE (<0.05) and R2 (>0.992) indicated that the S- and J-shaped CRCs were well fitted by the APTox program and LSSVR procedure, respectively. The fitted CRCs were subsequently used to calculate the median effective concentration (EC50) and the minimum inhibitory effect (Emin) or the maximum stimulation effect. The Emin values of the four J-shaped organic solvents are −20.5% (THF), −21.6% (isopropanol), −33.5% (acetone) and −16.6% (methanol). Thus, acetone has the maximum stimulation effect.
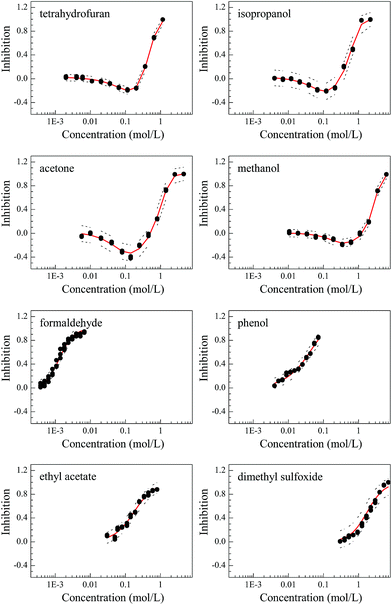 |
| Fig. 2 The concentration–response curves (CRCs) of the eight organic solvents, where the scattered points, solid lines (in red), and short dashed lines represent the experimental values, fitted CRCs, and the 95% confidence intervals of the CRCs, respectively. | |
Table 2 Statistics (the determination coefficient, R2, and root mean square error, RMSE), EC50, lower and upper limits of 95% confidence intervals of EC50 and Emin values of the eight organic solvents
Name |
Fitting function |
R2 |
RMSE |
Lowera (mol L−1) |
EC50 (mol L−1) |
Upperb (mol L−1) |
Eminc (%) |
Lower refers to lower limit of 95% confidence intervals of EC50. Upper refers to upper limit of 95% confidence intervals of EC50. Emin refers to the minimum inhibited effect or the maximum stimulated effect. |
Tetrahydrofuran (THF) |
LSSVR |
0.998 |
0.020 |
4.50 × 10−1 |
5.08 × 10−1 |
5.55 × 10−1 |
−20.5 |
Isopropanol |
LSSVR |
0.992 |
0.048 |
4.64 × 10−1 |
6.35 × 10−1 |
8.01 × 10−1 |
−21.6 |
Acetone |
LSSVR |
0.994 |
0.047 |
7.60 × 10−1 |
1.074 |
1.403 |
−33.5 |
Methanol |
LSSVR |
0.997 |
0.023 |
2.314 |
2.783 |
3.102 |
−16.6 |
Formaldehyde |
Logit |
0.994 |
0.029 |
1.14 × 10−3 |
1.32 × 10−3 |
1.55 × 10−3 |
— |
Phenol |
Weibull |
0.990 |
0.034 |
2.39 × 10−2 |
3.08 × 10−2 |
3.95 × 10−2 |
— |
Ethyl acetate (EAC) |
Logit |
0.992 |
0.036 |
1.35 × 10−1 |
1.75 × 10−1 |
2.31 × 10−1 |
— |
Dimethyl sulfoxide (DMSO) |
Logit |
0.994 |
0.030 |
1.761 |
2.031 |
2.318 |
— |
Root mean-square displacements (RMSD)
The root mean square displacement (RMSD) is used to evaluate the kinetic stability of the model system. The lower the RMSD is, the more stable the model system. From Fig. 3, after 8 ns simulation in each system, the fluctuation of RMSD is relatively small. In the last 3 ns of the model systems, the average values and standard deviations of RMSD are 1.56 ± 0.09 Å (methanol–Q67Lucα), 1.32 ± 0.06 Å (THF–Q67Lucα), 1.51 ± 0.07 Å (isopropanol–Q67Lucα), 1.52 ± 0.10 Å (acetone–Q67Lucα), 1.32 ± 0.08 Å (phenol–Q67Lucα), 1.25 ± 0.08 Å (DMSO–Q67Lucα), 1.52 ± 0.06 Å (formaldehyde–Q67Lucα), 1.55 ± 0.06 Å (EAC–Q67Lucα), 1.41 ± 0.06 Å (methanol–Q67Lucβ), 1.23 ± 0.07 Å (THF–Q67Lucβ), 1.48 ± 0.07 Å (isopropanol–Q67Lucβ), 1.36 ± 0.06 Å (acetone–Q67Lucβ), 1.36 ± 0.06 Å (phenol–Q67Lucβ), 1.42 ± 0.07 Å (DMSO–Q67Lucβ), 1.49 ± 0.05 Å (formaldehyde–Q67Lucβ), and 1.52 ± 0.06 Å (EAC–Q67Lucβ). All standard deviations of RMSD are less than 0.1 Å, indicating that the model systems are tending towards stability.
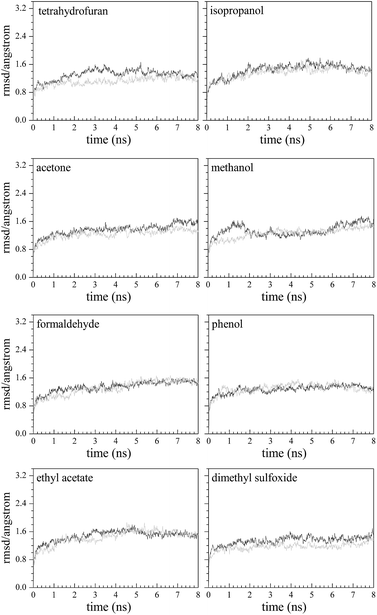 |
| Fig. 3 Plots of root mean square deviation (RMSD) vs. time for eight complexes of organic ligand–luciferase in Q67 where the gray and black lines represent the Q67Lucα– and Q67Lucβ–organic ligand complexes, respectively. | |
Binding free energy
The binding free energy (ΔG) refers to how easily a ligand binds to a protein. The higher the absolute value of ΔG (negative value), the stronger the binding affinity. The calculated ΔG for the four J-shaped organic solvents and four S-shaped organic solvents binding to Q67Lucα and Q67Lucβ are presented in Tables 3 and 4, respectively. The organic solvent–Q67 luciferase interactions of the lowest energy conformation are shown in Fig. S2 and S3.†
Table 3 The binding free energy and components for the complexes of the four J-shaped ligands with the Q67 luciferase α and β subunits
Component (kcal mol−1) |
THF-α |
Isopropanol-α |
Acetone-α |
Methanol-α |
THF-β |
Isopropanol-β |
Acetone-β |
Methanol-β |
ΔEvdw |
−10.36 |
−12.43 |
−9.16 |
−4.28 |
−14.08 |
−14.31 |
−13.75 |
−7.07 |
ΔEele |
−2.04 |
−2.61 |
−0.65 |
−12.22 |
−2.57 |
−9.87 |
−8.27 |
−6.04 |
ΔEvdw + ΔEele |
−12.40 |
−15.03 |
−9.82 |
−16.50 |
−16.65 |
−24.17 |
−22.02 |
−13.11 |
ΔGpolar,sol |
4.14 |
7.20 |
5.92 |
14.60 |
3.96 |
8.68 |
16.34 |
7.73 |
ΔGnonpolar,sol |
−1.94 |
−2.17 |
−2.15 |
−1.42 |
−1.85 |
−1.90 |
−1.90 |
−1.26 |
ΔGsol |
2.20 |
5.03 |
3.77 |
13.18 |
2.11 |
6.78 |
14.45 |
6.48 |
ΔEvdw + ΔGnonpolar,sol |
−12.30 |
−14.60 |
−11.32 |
−5.70 |
−15.93 |
−16.20 |
−15.65 |
−8.32 |
ΔEele + ΔGpolar,sol |
2.10 |
4.59 |
5.26 |
2.38 |
1.39 |
−1.19 |
8.08 |
1.69 |
ΔG |
−10.20 |
−10.01 |
−6.06 |
−3.32 |
−14.54 |
−17.39 |
−7.57 |
−6.63 |
Table 4 The binding free energy and components for the complexes of the four S-shaped ligands with the Q67 luciferase α and β subunits
Component (kcal mol−1) |
Formaldehyde-α |
Phenol-α |
EAC-α |
DMSO-α |
Formaldehyde-β |
Phenol-β |
EAC-β |
DMSO-β |
ΔEvdw |
−6.89 |
−14.42 |
−17.22 |
−12.73 |
−5.92 |
−9.95 |
−12.43 |
−7.12 |
ΔEele |
−8.77 |
−13.07 |
−8.85 |
−11.40 |
−8.95 |
−18.11 |
−2.61 |
−8.47 |
ΔEvdw + ΔEele |
−15.66 |
−27.49 |
−26.07 |
−24.14 |
−14.86 |
−28.06 |
−15.03 |
−15.58 |
ΔGpolar,sol |
13.94 |
14.95 |
14.56 |
16.62 |
13.08 |
18.43 |
7.20 |
11.85 |
ΔGnonpolar,sol |
−1.37 |
−2.61 |
−2.53 |
−2.09 |
−1.19 |
−2.18 |
−2.17 |
−1.43 |
ΔGsol |
12.57 |
12.34 |
12.05 |
14.53 |
11.90 |
16.25 |
5.03 |
10.42 |
ΔEvdw + ΔGnonpolar,sol |
−8.26 |
−17.03 |
−19.75 |
−14.82 |
−7.11 |
−12.13 |
−14.60 |
−8.56 |
ΔEele + ΔGpolar,sol |
−24.44 |
−40.56 |
−34.91 |
−35.55 |
−23.83 |
−46.17 |
−17.64 |
−24.04 |
ΔG |
−3.09 |
−15.15 |
−14.02 |
−9.61 |
−2.98 |
−11.81 |
−10.01 |
−5.17 |
From Tables 3 and 4, the values of van der Waals interaction energy (ΔEvdw) and electrostatic energy (ΔEele) are negative, which means that the gas-phase electrostatic energy (ΔEMM = ΔEvdw + ΔEele) is a favorable factor for the binding of the organic solvent. Moreover, the values of the nonpolar solvation energy (ΔGnonpolar,sol) are negative, but those of the polar solvation free energy (ΔGpolar,sol) are positive. However, the contribution from ΔGnonpolar,sol could not compensate for the unfavorable effect from ΔGpolar,sol. Thus, the solvation energy is an unfavorable factor for binding of organic solvents. Similar results were observed in Chen's report that predict the mixture effects of three pesticides by integrating molecular simulation with concentration addition modeling. As he explained, the contribution from ligand–protein polar interactions could not compensate for the large desolvation penalty.52
As is shown in Tables 3 and 4, the ΔG values for the J-shaped organic solvents binding to Q67Lucβ (−14.54, −17.39, −7.57 and −6.63 kcal mol−1 for THF, isopropanol, acetone and methanol, respectively) are more negative than those of its binding to Q67Lucα (−10.20, −10.01, −6.06 and −3.32 kcal mol−1), but the opposite was observed for the S-shaped organic solvents. Thus, the binding of the J-shaped organic solvents occurs more easily with Q67Lucβ than with Q67Lucα.
Root mean square fluctuation (RMSF)
The root mean square fluctuation (RMSF) reflects the fluctuation of residues in the model system. The parts of conformations are easier to change when RMSF is higher. As the natural substrates of the photobacterium luminous reaction, FMN binds to Q67Lucα.22 For Q67Lucα, the fluctuation of the residue Thr179α of the α-helix can make it bigger and easier for FMN binding to occur. In addition, the loop of the residues Ser145α–Arg165α has high flexibility, which enables the fluctuation of the α-helix.22 As observed from Fig. 4, the RMSF of J-shaped organic solvents binding to Q67Lucβ first are slightly higher for pure Q67 luciferase without binding FMN and organic solvents. This indicates that the loop of the residues Ser145α–Arg165α became more flexible because of the J-shaped organic solvents binding to Q67Lucβ, assisting FMN to bind to Q67Lucα.
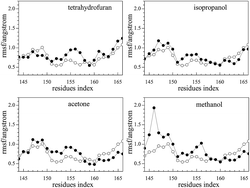 |
| Fig. 4 Plots of the root mean square fluctuation (RMSF) vs. the residue index for Q67Lucα, where the solid dots represent the complexes of the four J-shaped organic solvent ligands and Q67 luciferase. The hollow dots represent Q67 luciferase without any ligand such as FMN or organic solvent. | |
Mechanistic hypothesis of the hormesis
Overcompensation stimulation hormesis (OCSH)53 is an adaptive response for organisms facing low levels of stress, which results in enhanced fitness for some physiological systems in finite periods. The key conceptual features of OCSH are the disruption of homeostasis, the modest overcompensation, reestablishment of homeostasis and the adaptive nature of the process.53 Hormetic concentration responses without the observation of an overcompensating response are called direct stimulation hormesis (DSH).13 DSH includes receptor-mediated and cell signalling-mediated mechanisms. The specific difference between OCSH and DSH is seen in Fig. S4.†54 In this study, the mechanism of hormesis of organic solvents was based on Q67 luciferase as the receptor and the organic solvents as the xenobiotic substances. The hormesis phenomenon occurred after exposure for 15 min. These characteristics were in line with direct stimulation hormesis (DSH) and it could be the mechanism for the organic solvents.
Allosteric regulation occurs when an allosteric modulator binds at an allosteric site, a site distinct from the orthosteric site, inducing a change in conformation of the protein and its function and activity.55 In this study, J-shaped organic solvents bind to Q67Lucβ first, while the S-shaped organic solvents bind to Q67Lucα. At low concentrations, J-shaped organic solvents do not block Q67Lucα and do not prevent the combination of FMN and Q67Lucα. In addition, the loop of the residues Ser145α–Arg165α becomes more flexible, which assists FMN to bind to Q67Lucα. Thus, a stimulatory effect is observed on the luminous reaction. With increase of concentration, the Q67Lucβ is gradually filled up, and the J-shaped organic solvent starts to bind to Q67Lucα and prevents FMN from binding to Q67Lucα. This inhibits the luminous reaction. In the case of S-shaped organic solvents, FMN cannot bind to Q67Lucα at low concentrations, because the solvents bind to Q67Lucα in the first place. The luminous reaction is thus inhibited from the start. To summarize, precisely because of the binding order, the organic solvents display different concentration–response relationships.
Conclusions
In this study, we found that four organic solvents, THF, isopropanol, acetone and methanol, have hormetic concentration–response relationships with Q67, but the other four solvents, formaldehyde, phenol, EAC and DMSO, do not. Molecular simulation reveals that the hormetic organic solvents first bind to Q67Lucβ at low concentrations, and along with the binding, slightly change the flexibility of the residues Ser145–Arg165 located on Q67Lucα; this enables FMN to bind to Q67Lucα and finally exert the hormesis phenomenon. With increasing concentrations, redundant molecules start to bind to Q67Lucα and compete with/block FMN binding to Q67Lucα, resulting in an inhibitory effect. It can be concluded that the inhibition is induced by competition with FMN, while the simulation is derived from binding with Q67Lucβ.
Acknowledgements
The authors are particularly grateful to the National Natural Science Foundation of China (21377097).
References
- E. J. Calabrese, Environ. Pollut., 2013, 182, 452–460 CrossRef CAS PubMed.
- L. N. Vandenberg, T. Colborn, T. B. Hayes, J. J. Heindel, D. R. Jacobs, D. H. Lee, J. P. Myers, T. Shioda, A. M. Soto, F. S. vom Saal, W. V. Welshons and R. T. Zoeller, Reprod. Toxicol., 2013, 38, 1–15 CrossRef CAS PubMed.
- E. J. Calabrese and L. A. Baldwin, Toxicol. Sci., 2003, 71, 246–250 CrossRef CAS PubMed.
- Y. Fan, S. S. Liu, R. Qu, K. Li and H. L. Liu, RSC Adv., 2017, 7, 6080–6088 RSC.
- S. Jenkins, J. Wang, I. Eltoum, R. Desmond and C. A. Lamartiniere, Environ. Health Perspect., 2011, 119, 1604–1609 CrossRef CAS PubMed.
- R. G. Belz and H.-P. Piepho, Chemosphere, 2017, 178, 88–98 CrossRef CAS PubMed.
- L. J. Wang, S. S. Liu, J. Yuan and H. L. Liu, Chemosphere, 2011, 84, 1440–1445 CrossRef CAS PubMed.
- J. Zhang, S. S. Liu, Z. Y. Yu and J. Zhang, Chemosphere, 2013, 91, 462–467 CrossRef CAS PubMed.
- J. Zhang, S. S. Liu, Z. Y. Yu, H. L. Liu and J. Zhang, J. Hazard. Mater., 2013, 258, 70–76 CrossRef PubMed.
- R. Qu, S. S. Liu, F. Chen and K. Li, RSC Adv., 2016, 6, 21012–21018 RSC.
- S. S. Liu, F. Liu and H. L. Liu, China Environ. Sci., 2007, 27, 371–376 CAS , in Chinese.
- E. J. Calabrese, Homeopathy, 2015, 104, 90–96 CrossRef PubMed.
- E. J. Calabrese, Crit. Rev. Toxicol., 2013, 43, 580–606 CrossRef CAS PubMed.
- M. Z. Hashmi, Naveedullah, H. Shen, S. Zhu, C. Yu and C. Shen, Environment International, 2014, 64, 28–39 CrossRef CAS PubMed.
- D. J. Morre, Hum. Exp. Toxicol., 1998, 17, 272–277 CAS.
- F. Chen, S. S. Liu, M. Yu, R. Qu and M. C. Wang, Chemosphere, 2015, 132, 108–113 CrossRef CAS PubMed.
- J. Zhang and S. S. Liu, J. Hazard. Mater., 2015, 283, 568–573 CrossRef CAS PubMed.
- S. H. Hsieh, C. H. Hsu, D. Y. Tsai and C. Y. Chen, Environ. Toxicol. Chem., 2006, 25, 2920–2926 CrossRef CAS PubMed.
- E. A. Meighen, Annu. Rev. Genet., 1994, 28, 117–139 CrossRef CAS PubMed.
- A. R. Madvar, S. Hosseinkhani, K. Khajeh, B. Ranjbar and A. Asoodeh, FEBS Lett., 2005, 579, 4701–4706 CrossRef CAS PubMed.
- E. V. Vetrova, N. S. Kudryasheva, A. Visser and A. van Hoek, Luminescence, 2005, 20, 205–209 CrossRef CAS PubMed.
- F. Chen, S. S. Liu and X. T. Duan, Acta Chim. Sin., 2013, 71, 1035–1040 CrossRef CAS , in Chinese.
- D. Rajesh, S. Muthukumar, G. Saibaba, D. Siva, M. A. Akbarsha, B. Gulyas, P. Padmanabhan and G. Archunan, Sci. Rep., 2016, 6, 35900 CrossRef CAS PubMed.
- B. Yoo, B. Jing, S. E. Jones, G. A. Lamberti, Y. Zhu, J. K. Shah and E. J. Maginn, Sci. Rep., 2016, 6, 19889 CrossRef CAS PubMed.
- A. Perez, J. A. Morrone, C. Simmerling and K. A. Dill, Curr. Opin. Struct. Biol., 2016, 36, 25–31 CrossRef CAS PubMed.
- R. B. Best, X. Zhu, J. Shim, P. E. M. Lopes, J. Mittal, M. Feig and A. D. MacKerell Jr, J. Chem. Theory Comput., 2012, 8, 3257–3273 CrossRef CAS PubMed.
- W. Wang, W. Ye, C. Jiang, R. Luo and H. F. Chen, Chem. Biol. Drug Des., 2014, 84, 253–269 CAS.
- F. Li, Q. Xie, X. Li, N. Li, P. Chi, J. Chen, Z. Wang and C. Hao, Environ. Health Perspect., 2010, 118, 602–606 CrossRef CAS PubMed.
- S. D. Walker and S. McEldowney, Chemosphere, 2013, 93, 2568–2577 CrossRef CAS PubMed.
- W. Yang, S. Wei, H. Liu and H. Yu, Chemosphere, 2011, 84, 328–335 CrossRef CAS PubMed.
- G. Rastelli, A. Del Rio, G. Degliesposti and M. Sgobba, J. Comput. Chem., 2010, 31, 797–810 CAS.
- M. Yu, S. S. Liu, M. C. Wang, F. Chen and H. X. Tang, Chin. J. Chem., 2014, 32, 545–552 CrossRef CAS , in Chinese.
- M. C. Wang, S. S. Liu and F. Chen, Acta Chim. Sin., 2014, 72, 56–60 CrossRef CAS , in Chinese.
- S. S. Liu, X. Q. Song, H. L. Liu, Y. H. Zhang and J. Zhang, Chemosphere, 2009, 75, 381–388 CrossRef CAS PubMed.
- Y. H. Zhang, S. S. Liu, X. Q. Song and H. L. Ge, Ecotoxicol. Environ. Saf., 2008, 71, 880–888 CrossRef CAS PubMed.
- M. Scholze, W. Boedeker, M. Faust, T. Backhaus, R. Altenburger and L. H. Grimme, Environ. Toxicol. Chem., 2001, 20, 448–457 CrossRef CAS PubMed.
- S. S. Liu, J. Zhang, Y. H. Zhang and L. T. Qin, Acta Chim. Sin., 2012, 70, 1511–1517 CrossRef CAS , in Chinese.
- L. T. Qin, S. S. Liu, H. L. Liu and Y. H. Zhang, Chemosphere, 2010, 78, 327–334 CrossRef CAS PubMed.
- X. W. Zhu, S. S. Liu, L. T. Qin, F. Chen and H. L. Liu, Ecotoxicol. Environ. Saf., 2013, 89, 130–136 CrossRef CAS PubMed.
- X. W. Zhu, S. S. Liu, H. L. Ge and Y. Liu, China Environ. Sci., 2009, 29, 113–117 CAS , in Chinese.
- Z. Yang, K. Lasker, D. Schneidman-Duhovny, B. Webb, C. C. Huang, E. F. Pettersen, T. D. Goddard, E. C. Meng, A. Sali and T. E. Ferrin, J. Struct. Biol., 2012, 179, 269–278 CrossRef CAS PubMed.
- A. Jakalian, B. L. Bush, D. B. Jack and C. I. Bayly, J. Comput. Chem., 2000, 21, 132–146 CrossRef CAS.
- P. T. Lang, S. R. Brozell, S. Mukherjee, E. F. Pettersen, E. C. Meng, V. Thomas, R. C. Rizzo, D. A. Case, T. L. James and I. D. Kuntz, RNA, 2009, 15, 1219–1230 CrossRef CAS PubMed.
- G. J. Howard and T. F. Webster, Environ. Health Perspect., 2013, 121, 1–6 Search PubMed.
- O. Trott and A. J. Olson, J. Comput. Chem., 2010, 31, 455–461 CAS.
- D. A. Case, T. E. Cheatham, T. Darden, H. Gohlke, R. Luo, K. M. Merz, A. Onufriev, C. Simmerling, B. Wang and R. J. Woods, J. Comput. Chem., 2005, 26, 1668–1688 CrossRef CAS PubMed.
- J. M. Wang, R. M. Wolf, J. W. Caldwell, P. A. Kollman and D. A. Case, J. Comput. Chem., 2004, 25, 1157–1174 CrossRef CAS PubMed.
- D. A. Case, T. A. Darden, T. E. Cheatham III, C. L. Simmerling, J. Wang, R. E. Duke, R. Luo, R. C. Walker, W. Zhang and K. M. Merz, AMBER 12, 2012 Search PubMed.
- T. J. Hou, J. M. Wang, Y. Y. Li and W. Wang, J. Chem. Inf. Model., 2011, 51, 69–82 CrossRef CAS PubMed.
- H. Sun, Y. Li, S. Tian, L. Xu and T. Hou, Phys. Chem. Chem. Phys., 2014, 16, 16719–16729 RSC.
- L. Xu, H. Sun, Y. Li, J. Wang and T. Hou, J. Phys. Chem. B, 2013, 117, 8408–8421 CrossRef CAS PubMed.
- F. Chen, S. S. Liu, X. T. Duan and Q. F. Xiao, RSC Adv., 2014, 4, 32256–32262 RSC.
- E. J. Calabrese and L. A. Baldwin, Hum. Exp. Toxicol., 2002, 21, 91–97 CAS.
- E. J. Calabrese and L. A. Baldwin, Annu. Rev. Public Health, 2001, 22, 15–33 CrossRef CAS PubMed.
- N. M. Goodey and S. J. Benkovic, Nat. Chem. Biol., 2008, 4, 474–482 CrossRef CAS PubMed.
Footnote |
† Electronic supplementary information (ESI) available. See DOI: 10.1039/c7ra06503e |
|
This journal is © The Royal Society of Chemistry 2017 |
Click here to see how this site uses Cookies. View our privacy policy here.