DOI:
10.1039/D3NR06057H
(Review Article)
Nanoscale, 2024,
16, 1471-1489
Organic iontronic memristors for artificial synapses and bionic neuromorphic computing
Received
28th November 2023
, Accepted 14th December 2023
First published on 15th December 2023
Abstract
To tackle the current crisis of Moore's law, a sophisticated strategy entails the development of multistable memristors, bionic artificial synapses, logic circuits and brain-inspired neuromorphic computing. In comparison with conventional electronic systems, iontronic memristors offer greater potential for the manifestation of artificial intelligence and brain–machine interaction. Organic iontronic memristive materials (OIMs), which possess an organic backbone and exhibit stoichiometric ionic states, have emerged as pivotal contenders for the realization of high-performance bionic iontronic memristors. In this review, a comprehensive analysis of the progress and prospects of OIMs is presented, encompassing their inherent advantages, diverse types, synthesis methodologies, and wide-ranging applications in memristive devices. Predictably, the field of OIMs, as a rapidly developing research subject, presents an exciting opportunity for the development of highly efficient neuro-iontronic systems in areas such as in-sensor computing devices, artificial synapses, and human perception.
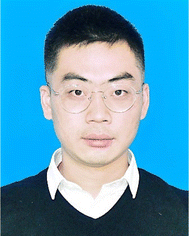 Yang Li | Yang Li is an associate professor at the School of Physical Science and Technology, Suzhou University of Science and Technology. He received his BS and PhD degrees from Soochow University, under the supervision of Prof. Jianmei Lu. From 2016 to 2017, he got financial support from the Chinese government to complete his joint PhD research at Nanyang Technological University, in Prof. Qichun Zhang's group. He is currently a Vebleo Fellow. His research interests focus on the preparation of novel organic/inorganic memristive materials and devices, and their applications for data storage and neuromorphic computing. |
1. Introduction
The conventional von Neumann architecture, which has been the foundation for computing since its inception, segregates processing and memory units and thus results in a well-known bottleneck commonly referred to as the “von Neumann bottleneck”.1–3 The bottleneck arising from the constant shuttle of data between the processing and memory units not only produces significant energy consumption but also imposes limitations on the computing speed.4,5 The academia and industry are actively seeking alternative computing architectures to sustain the advancement of computational power, given the termination of Moore's law and the limitations in further transistor miniaturization.6–8 The most promising alternative is neuromorphic computing, which draws inspiration from the human brain and integrates processing and memory into a unified entity.9,10 The brain serves as the central processing unit, and it is known that information transmission only consumes about 10–20 W.11 Therefore, scientists have replicated brain-inspired computing by developing new principle paradigms known as neuromorphic computing, which aim to emulate the cognitive functions observed in the human brain.
To the best of our knowledge, the human nervous system is comprised of over 86 billion neurons. As depicted in Fig. 1a, these neurons form an intricate network interconnected through synapses, facilitating the transmission of chemical mediators (e.g., Ca+, Na+, and K+) from presynaptic to postsynaptic terminals. Inspired by this, iontronics have emerged as an integral component in the field of electronics, affording the intricate interplay between ion regulation, coupled ion/electron charge transfer and signal exchange,12–14 and exerting a significant influence in areas such as data storage and bionics.15,16 As shown in Fig. 1b, the earliest studies of ion/electron coupling can be traced back to the galvanic frog muscle electrophysiological experiments in the 18th century, covering interdisciplinary subjects such as energy, sensing, and information transmission.17 Afterward, investigation into these fundamental scientific issues, such as iontronic materials, ion/electron coupling interfaces, iontronic devices and so on, has established a solid groundwork for future ion/electron logic circuits and neuromorphic computing.18,19
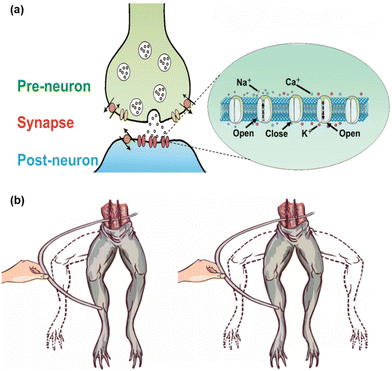 |
| Fig. 1 (a) Schematic image of an artificial synapse. (b) Stimulation of frog's leg muscles under electrical stimulation.47 Reprinted with permission from ref. 47, © 2019 American Chemical Society. | |
The ionic-type organic semiconductor materials, which typically consist of electrovalent bonds and charged groups, possess distinct advantages and attract greater attention compared to conventional neutral organic functional materials.20,21 These materials exhibit electron and ion conduction, providing enhanced functionalities like ion diffusion and adsorption, electrochemical activity, and tunable conductivity.22,23 Besides, their high ion mobility, selectivity, and reversible redox make them ideal for multifunctional devices performing both electronic and ionic functions.24,25 Therefore, ion-doped organic materials and their iontronic devices have been further applied to various areas, such as light-emitting devices (LEDs), field-effect transistors (FETs), sensors, and especially memristors and neuromorphic computing.26–32 Accordingly, organic ionic memristive materials (OIMs) and memristive devices have emerged as new electronics relying on ion migration and electron permeation in the solid ion transport layer.33–36 OIMs hold great promise in artificial intelligence (AI), neuromorphic computing, brain–computer interfaces, etc. to enable information processing, recognition, memorizing and forgetting, learning, and decision-making.37–45
This review aims to summarize the development and status of various OIMs in the field of memristor and neuromorphic computing, which possess an organic backbone and exhibit stoichiometric ionic states. As a class of emerging memristive materials,46 OIMs have garnered great attention due to their low cost, easy synthesis, high stability and ion/electron coupling properties. Here, we discuss in depth the progress and prospects of OIMs, including their inherent advantages, diverse types, synthesis methodologies, and wide-ranging applications in memristive devices, and propose their applications in highly efficient neuro-iontronic systems, such as in-sensor computing devices, artificial synapses, and human perception. However, the future challenges of OIMs in stability and reproducibility still need to be addressed, which are also summarized and discussed.
2. Organic iontronic memristive materials (OIMs)
2.1. Brief concept and advantages of OIMs
A plethora of inorganic memristive materials have been utilized to construct memristor devices, and continued to dominate the field of memristor preparation, due to their exceptional processing efficiency and immense storage capacity.47–51 Nevertheless, they often lack sufficient functional groups to be modified for diverse functionalities. By contrast, OIMs exhibit simultaneous electronic and ionic conductivity, and have attracted considerable attention in recent years due to their simple tuning ability and potential for a wide range of electronic applications, particularly in the field of organic iontronic memristors.52,53 Generally, these OIMs consist of skeletons embedded with charged ions, which confers additional advantages compared to traditional memristive materials.54 As summarized in Fig. 2, the primary advantage of OIMs lies in their capability to address intricate issues and specific needs through appropriate structural and functional design, thereby solving challenges pertaining to energy conversion, sensing, memory, and logic applications.55 Secondly, OIM-based memristors may exhibit enhanced energy efficiency in both operation and control.56 Furthermore, due to their insensitivity to magnetic fields, OIMs enable effective mitigation of electromagnetic interference in high-frequency and high-density environments, distinguishing them from electronic devices.57 Finally, the deformable and flexible properties of OIMs facilitate more natural and comfortable contact with the human body, inducing the prospects for intelligent applications, such as wearable devices, implantable devices, and smart surfaces. To sum up, OIMs possess the advantages of addressing complex problems, exhibiting low energy consumption, resisting interference, and facilitating seamless human–computer interaction,53,54,56 and can serve as robust contenders for the development of innovative electronic devices and systems.58–60
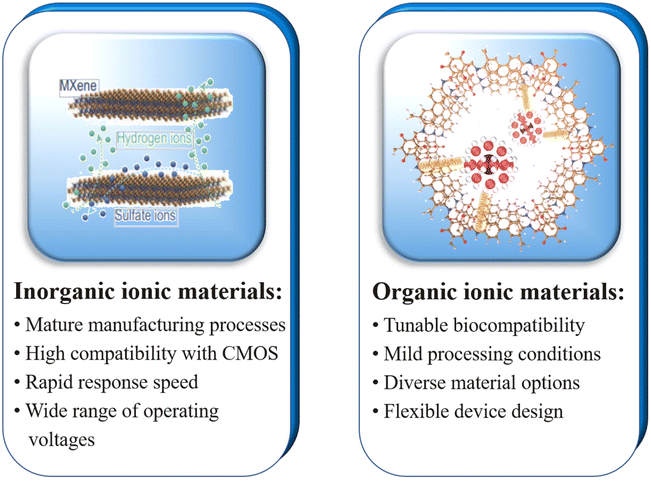 |
| Fig. 2 The performance comparison between inorganic ionic memristive materials and organic ionic memristive materials.71,72 Reprinted with permission from ref. 71, © 2022 Oxford University Press; ref. 72, © 2021 Wiley. | |
Until now, a series of OIMs have been developed and yielded several distinctive advantages. For ionic small molecules, they usually exhibit good conductivity, enabling rapid response to external electrical signals and enhanced sensitivity. They also have high mechanical flexibility and plasticity, rendering them suitable for fabricating flexible electronic devices that afford extensive applications in smart watches, medical stickers and other fields.61,62 Ionic polymers have excellent ion transport properties, which can realize data storage through ion migration and energy storage through ion conductance. Consequently, this kind of material finds wide applications not only in memristors but also in supercapacitors and battery energy storage systems.63,64 Due to their exceptional chemical stability and precise control over ions, ionic liquids have also been extensively studied and applied in the fields of solar cells and flexible fuel cells, while they can also be prepared into thin films for metal protection, electronic device packaging, biomedicine, and so on.65–67 In addition, OIMs can be applied in the domains of configurable circuits and multilevel memory, owing to their adjustable molecular structure, excellent optoelectronic properties, large specific surface area, controllable porosity, etc.68–70 Overall, the unique characteristics of OIMs enable them to exert excellent performance and application in diverse fields, thereby manifesting their significance for further development and investigation in the future.
2.2. Specific categories of OIMs
OIM-based memristors typically exhibit ion transport characteristics, showing nonlinear resistance–current characteristics.73 When a voltage bias is applied, the transport speed and direction of ions in OIM-based memristors will change, leading to a corresponding variation in the resistance value. According to the principle of memristors, the inherent nonlinearity performance enables memristive devices to efficiently store and process vast amounts of data, facilitating computation and logic operations based on ion transport. In addition, owing to the high diffusion velocity and low response time of the ion-active medium, OIM-based memristors hold immense potential for diverse applications encompassing artificial intelligence, neuromorphic computing, memory devices, and energy storage.32
Generally, OIMs can be divided into two categories, namely, ionically rigid conjugated materials (IICs) and ionically flexible spacer materials (IIFs).74 IICs pertain to organic compounds featuring rigid conjugated molecular frameworks and cationic or anionic groups. Once an external electric field is applied, the IIC-based memristor will present resistive switching behavior. Considering the existence of rigid molecular frameworks, IICs usually exhibit high stability and a prolonged lifetime.74–76 IIFs refer to organic materials with ions attached to flexible molecular chains. Under the application of an electric field, a reorganization of ions in the ionic liquid or colloid may lead to a modified resistance. Due to the presence of a flexible molecular framework, IIFs have a high ion transport speed and a fast response speed.77,78 These two aforementioned categories of OIMs have broad application potential in the fields of energy storage, information storage, and sensing. However, it is imperative to meticulously select the appropriate material category according to the specific application requisites.
To give a more refined classification, OIMs can be divided into organic ionic small molecules and polymers, including ionic liquids,79–81 polymeric ionic liquids,82 ionic gels,160 ionic conducting polymers,83–86 coordination compounds (e.g., metal–organic frameworks (MOFs)),87–89 some specific complexes, and so on.84,90–93,161 All of these categories are introduced as follows (Fig. 3). (a) Ionic liquids can be designed and synthesized with varying compositions of ion pairs. They normally possess the advantages of low melting point, wide chemical resistance window, excellent ion transport properties, high adjustability, good thermal stability, and low volatility. Due to the relatively straightforward design and preparation process, ionic liquids with low fabrication costs can be easily synthesized on a large scale with a controllable size.79–81 (b) Polyionic liquids are composed of ionic groups attached to polymer chains. In comparison with ionic liquids, polyionic liquids exhibit enhanced stability and plasticity. Besides, they exhibit exceptional ionic conductivity, rapid ion transport velocity and reduced energy consumption. Polyionic liquids also demonstrate relatively high electrochemical stability and thermal stability.82 (c) Ionic gels utilize organic gels containing ionic liquids as the active layer. The ionic liquid components provide high ionic conductivity, while the gel network offers mechanical stability and flexibility. At the applied voltage, the migration of cations and anions within the ionic gel enables resistive switching. The crosslinked gel structure helps maintain the ion distribution after removing the voltage. The integration of high conductivity and shape retainability makes ionic gels suitable for high-performance memristive devices. Ionic gels combine the dynamics of ionic liquids and robustness of gels, exhibiting both fast ion transport capability and form stability.160 (d) Besides polyionic liquids and gels, other ion-conducting polymers are widely used in iontronic memristors. These ion-conducting polymers enhance ion transport properties by incorporating ionic groups into their molecular structures. They possess remarkable tunability and flexibility, where their electrochemical characteristics can be tuned by chemical modification. In addition, this kind of polymer generally exhibits exceptional conductivity and good durability.83–86 (e) Ionic coordination compounds represent a category of structures wherein metal atoms and organic ligands are linked by coordinate bonds. As a representative, MOFs show unique properties of high tunability, porosity and large surface areas. These materials can offer precise control over ion transport channels in iontronic memristors, while facilitating the storage and release of various ions within the material. Additionally, MOFs have superior thermal and chemical stability, along with remarkable scalability.87–89 (f) Organic complexes exhibit both electronic and ion conductivity, serving as active layers in memristive devices. Under the stimulus of voltage, the resistive state of memristors can be modulated by the migration of ions in and out of the active layer. The unique electrochemical properties inherent to organic complexes make them attractive for utilization in memristors, resulting in low-power and energy-efficient operation.84,90–93 Conclusively, ionic liquids and polyionic liquids have the advantages of low cost, easy preparation, high stability and plasticity. Other ion-conducting polymers, ionic coordination compounds and complexes exhibit the benefits of high tunability, excellent ion transport properties and good stability. All of these materials can cater to diverse application requirements including memristors.94
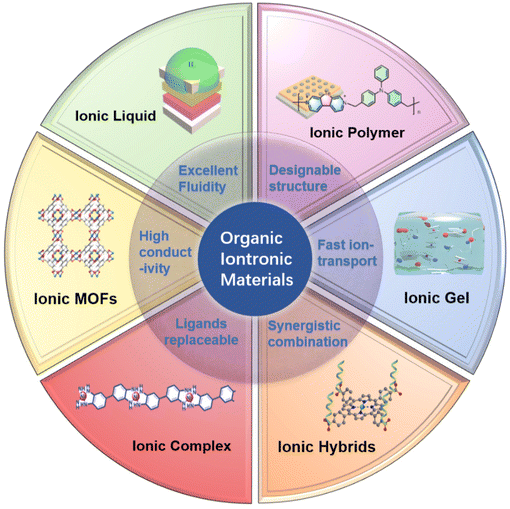 |
| Fig. 3 Different types of ionic memristive materials.93,95–97,160,161 Reprinted with permission from ref. 93, © 2017 Wiley; ref. 95, © 2023 Wiley; ref. 96, © 2021 American Chemical Society; ref. 97, © 2021 Open Access; ref. 160, © 2023 Open Access; and ref. 161, © 2023 American Chemical Society. | |
2.3. Preparation methods
To achieve these OIMs, various synthesis methods have been developed, including the ion exchange method, free radical polymerization method, solvothermal method and self-assembly method. (1) For the ion exchange method, the ion exchange reactions are performed between organic ligands and ions to form organic ionic materials,98 which are suitable for the synthesis of organic ionic materials with specific structures and functions at high selectivity and a fast reaction rate. For instance, cation exchange resin is one of the most commonly used organic ion materials, due to its excellent ion exchange capacity and chemical stability.99 However, it has limited regularity in terms of methodological procedures and preparation diversity, restricting its applicability to the preparation of specific types of ions.100 (2) Free radical polymerization involves the polymerization of monomers containing ionic groups, resulting in the formation of ionic polymer materials with desired properties.101 The key to this method lies in conducting monomer polymerization reactions using catalysts to form organic ionic polymer materials.102 During atom transfer radical polymerization (ATRP), transition metal catalysts and upstream radicals can realize high selectivity and controllable polymerizations, offering predictable and diverse chemical structures.103 (3) The solvothermal method needs the elevated temperature and pressure conditions of organic solvents to facilitate the formation of OIMs through dissolution and recrystallization.104 This technique is particularly suitable for synthesizing OIMs with high crystal quality and uniform distribution.105 Notably, solvothermal synthesis is employed to produce organic perovskite materials by the solvothermal method,106 showing the high photoelectric conversion efficiency of solar cells and LED performances.107 (4) The process of self-assembly involves the spontaneous arrangement of organic molecules into specific structures through intermolecular interaction.108 By selecting suitable organic ligands and the corresponding metal ions, organic ionic materials with specific morphologies and structures can be readily formed for various applications, such as liquid crystal displays, optoelectronic devices and sensors.109,110 Overall, the ongoing research efforts in developing OIMs are expected to discover more novel synthetic methodologies that improve the properties and performance of OIMs for various electronic applications.
3. Memristive switching mechanisms
Unlike traditional electronic components, OIM-based memristors demonstrate a different operational mechanism. The memristive effect in OIM-based memristors depends on the quantity of charge and ions, holding promise to mimic human memory functions.111,112 Specifically, when current flows through the memristor, the corresponding resistance may change as the increased applied voltage. If the resistance state of the device can be well maintained even after the removal of voltage, this device demonstrates typical nonvolatile memory behaviors.113 Until now, some mechanisms have been promoted, including conductive filament formation,114–118 charge trapping modulation,119–122etc.
3.1. Formation of conductive filaments
The formation of conductive filaments in memristors is attributed to the oxidation of active metal ions (e.g. Cu and Ag) into cations under an electric field, which migrate toward the cathode and are reduced back into metals, forming conductive paths between the electrodes and decreasing resistance.114 After power removal, the instability of such paths causes the rupture and recovery of the high resistance state.115 The formation and dissolution of conductive filaments can be modulated by the magnitude and polarity of the applied voltage. Recently, Sun et al. reported a memristor with a Ag/IGZO–TiO2/Al structure whose switching mechanism can be tuned by ionic liquids.96 As illustrated in Fig. 4a, the operating principle of this device was elucidated through the following steps: (1) upon positive bias, metal ions (Ag+, Vo2+, H+) drift toward the cathode while O2− and e− move to the anode. (2) When Ag+ is reduced into Ag atoms and connected with Vo2+, a conductive filament forms around the cathode. (3) Under reversed bias, opposite cations migrate back to the anode and anions back to the cathode. (4) As the reverse bias further increases, gradual rupture of the filamentary path occurs, recovering the high-resistance state.
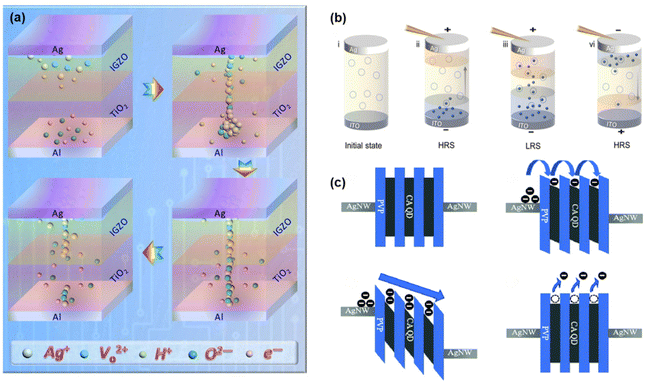 |
| Fig. 4 (a) Schematic illustration of the formation of conductive filaments.96 (b) Model of the resistive switching mechanism.118 (c) Schematic band diagrams to explain the resistive switching model.122 Reprinted with permission from ref. 96, © 2021 American Chemical Society; ref. 118, © 2022 Science China Press and Springer; ref. 122, © 2018 Royal Society of Chemistry. | |
In addition, ion migration is a critical mechanism governing resistive switching in memristors, participating in the formation and rupture of conductive filaments.116 External stimuli such as light, electricity, heat, or chemical effects can induce the migration of ions, leading to the formation of conductive channels or non-conductive barriers and the corresponding switching of memristor resistance. Therefore, precise control of ion migration is crucial for the design of memristors with desired performance.117 Zhang et al. reported an ultrathin bio-memristor based on silk nanofiber films and analyzed its resistive switching behavior.118 As depicted in Fig. 4b, multiple traps associated with sodium ions (Na+) exist in the nanofiber layer. When a positive voltage is applied on the top electrode, electrons migrate from the bottom electrode while cations in the layer move toward the negatively biased bottom electrode. An electric double layer (EDL) enriched with Na+ forms at the interface, facilitating carrier trapping and migration. As the voltage further increases, instant saturated trap-filling leads to redundant carriers forming a conductive channel, inducing an abrupt transition from the high-resistance state (HRS) to the low-resistance state (LRS). The memristor recovers to the HRS when an opposite voltage is applied, releasing the trapped electrons toward the bottom electrode.
3.2. Modulation of charge trapping
The charge trapping mechanism refers to the process of modulating the trapping and release of charge carriers in the memristive layer.119 Some certain carriers become trapped in defects or impurities in the insulating layer, leading to reduced resistance of the memristor.120 Conversely, when a reverse bias is applied, the trapped charge carriers are released from their confinement, resulting in an increased resistance of the memristor. This charge-trapping modulation serves as a fundamental mechanism for the operation of specific types of memristors such as organic and amorphous oxide variants.121 Zhou et al. employed a full solution process to prepare an Ag nanowire/citric acid quantum dot-PVA/Ag nanowire structure volatile memory device, and proposed a resistance switching mechanism model for memory devices.122 As shown in Fig. 4c, the thermally excited carriers dominate the conduction at a low bias voltage (0–0.7 V), wherein the device shows a high-resistance state. At a medium bias voltage (0.7–1 V), the injected carriers are dominant through trap-controlled space charge-limited mechanisms, which fall within the secondary region. When all traps are fully saturated, the injected carriers can move freely, leading to a sharp transition of the device into a low-resistance state. Upon removal of the bias voltage, the shallow traps fail to trap carriers, causing the device to revert back to a high-resistance state. The model elucidates the formation and destruction process of the conductive path in the dielectric layer driven under an external electric field, and explains the reversible resistance-switching behaviors.
3.3. Redox mechanism
Redox reactions serve as a significant mechanism for resistive switching in certain types of memristors. The active layer in these devices typically contains electrochemically active species that can undergo oxidation or reduction when subjected to an electric field.151 For example, in electrochemical metallization cells with a solid electrolyte, metal ions such as Cu2+ and Ag+ can be reduced at the cathode, leading to the formation of conductive metallic filaments that bridge the electrodes.152 Reversing the voltage bias will result in the oxidation and dissolution of these filaments, thereby increasing the resistance of the device.153
In organic memristors, the redox-active groups embedded in the organic matrix play a crucial role in modulating charge transport and resistance states, which have been observed in certain types of memristive devices. These redox-active groups, such as nitro and acene, undergo reversible oxidation/reduction reactions when subjected to external voltage. During these redox reactions, ion migration occurs as counterions need to balance the charges generated by the oxidation or reduction processes. The movement of ions within the organic matrix further influences the overall resistance state of the memristor.154 To develop high-performance memristors, it is essential to have precise control over these redox processes. Understanding the reaction kinetics involved and studying ion migration dynamics can greatly contribute to optimizing the memristor performance, including device stability, switching speed, and increasing endurance.155 Therefore, this knowledge will pave the way for advancements in memristor technology that could revolutionize various fields including data storage, neuromorphic computing systems, and flexible electronics.
4. OIM-based memristor applications
OIM-based memristors utilize both the semiconductor characteristics and ionic transport properties during the device operation. Active media encompass various substances, such as small molecules, complexes, ionic liquids, ion-conducting polymers and polymeric ionic liquids.81,82,91,93,123,124 In the context of memristive devices, the resistive states can be modulated by the migration of ions in and out of the active layer under an externally applied electric field.32,125 The potential of these devices in low-cost and flexible electronics, as well as their ability to exhibit analog behavior, has garnered significant attention (Table 1). Therefore, developing high-performance OIMs is crucial to advancing the field of organic iontronic memristors and realizing their potential in a range of electronic applications.43
Table 1 The summary of the performance of different OIM-based devices
Materials |
Category |
Current ratio |
Other features |
Ref. |
NCPy |
Small molecule |
103 |
— |
73
|
NCPy-salt |
Small molecule |
106/102 |
Retention time > 5 × 103 s |
73
|
DEME-TFSI |
Small molecule |
— |
Retention time > 105 s |
96
|
SA-Bu |
Zwitterionic |
105/102 |
Retention time > 104 s |
128
|
Response time 190 s (NO2) |
CA-Bu |
Zwitterionic |
107 |
Retention time > 104 s |
128
|
PTeVTPA |
Polymer |
— |
High-pass filter |
97
|
Ni-BTA |
Complex |
107/103 |
Retention time > 104 s |
93
|
Ni-BPTA |
Complex |
108/104 |
Retention time > 104 s |
93
|
Au-NPs |
MOFs |
104 |
High stability > 200 times |
87
|
Ag-NPs |
MOFs |
103 |
High stability > 200 times |
87
|
SNFs |
Bio-nanofibrils |
102 |
Retention time > 105 s |
118
|
High stability > 180 times |
4.1. Small molecule OIM-based memristors
Small molecule OIM-based memristors utilize organic small molecule OIMs as the data-storage medium, where the migration of ions or electrons is controlled to adjust their resistance/conductance. Typically, ions or electrons can migrate within the active layer at an applied voltage. Different ion or electron migration behaviors result in varying resistance alterations, leading to information storage and reading operations. Unlike inorganic materials with well-defined crystalline properties, organic materials often present isotropic molecular orientations and diverse organizational forms, giving rise to unpredictable morphologies and device performance. Commonly, the performance of organic small molecule memristors could be enhanced by modifying the chemical structure of the active layer. For example, Zhang et al. synthesized two fresh ionic and zwitterionic donor/acceptor (D/A) conjugated small molecules (LD-IOM and LD-ZIOM).126 Compared to neutral parent structures, these two newly-synthesized ionic and zwitterionic D/A conjugated small molecules show improved optoelectronic performances (Fig. 5a). In addition, Zhang et al. also synthesized a new pyridine-based organic conjugated molecule (NCPy) and converted it into an organic pyridinium salt (NCPy-salt).73 The transition from NCPy to ionic NCPy salts produced highly oriented nanofibers and high-quality films. The NCPy salt-based devices undergo two “writing” processes, transitioning from the OFF state to the medium conductivity (ON1) state and further to the high conductivity (ON2) state. The device exhibits a current switching ratio of 106/102/1. Owing to the salification, a unique ternary resistive switching memory behavior was observed, implying potential applications in high-density data storage and memory (Fig. 5b). The stability and repeatability of the small molecule memristor were fully confirmed through measurements conducted on over 50 device units, as well as a persistence test lasting more than 5000 s.
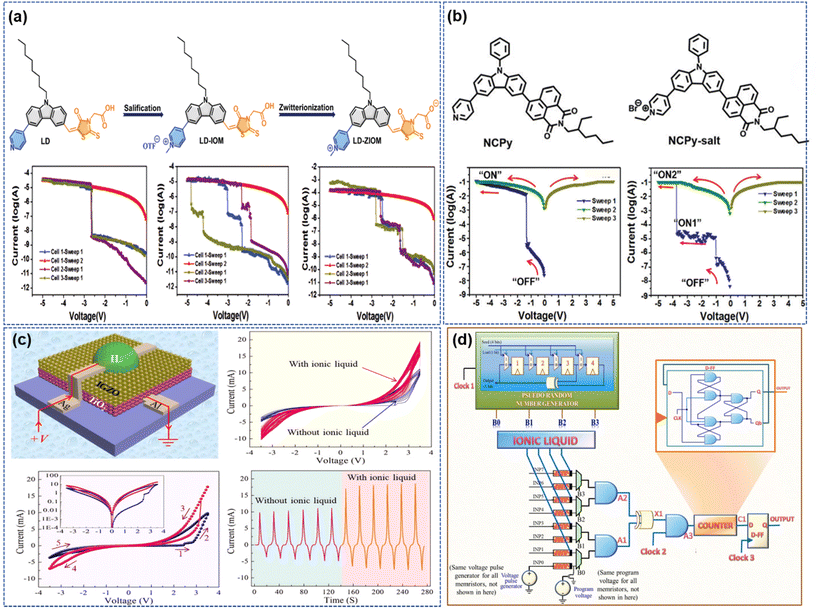 |
| Fig. 5 (a) Synthesis schemes of LD and its ionic and zwitterionic derivatives (LD-IOM and LD-ZIOM) and I–V characteristics.126 (b) Molecular structures of NCPy, NCPy-salt and their I–V characteristics.73 (c) Schematic diagram of the device modulated by ionic liquids. (d) Circuit diagram of the true random number generator.96 Reprinted with permission from ref. 73, © 2018 the Royal Society of Chemistry; ref. 96, © 2021 American Chemical Society; and ref. 126, © 2020 the Royal Society of Chemistry. | |
The inclusion of small molecule ionic liquid materials has expanded the selection range of memristive materials. Ionic liquids have high ionic conductivity, resulting in reduced threshold voltage and low power consumption for these memristors. To increase the storage density, Sun et al. used an indium gallium zinc oxide (IGZO)–TiO2 bilayer film as the functional layer within the memristive device (Fig. 5c).96 By utilizing diethylmethyl(2-methoxyethyl)ammonium bis(trifluoromethylsulfonyl)imide (DEME-TFSI), an ionic liquid known for its flexibility, it was able to fine-tune the memory behavior of the device. The device exhibits excellent switching stability and retention, with the HRS and the LRS lasting for a minimum of 150 cycles when subjected to a readout voltage of 1.0 V. Additionally, the device successfully passed a hold test lasting 105 s. This research demonstrates that integrating memristors into computing systems can lead to high-density storage capabilities and advanced artificial intelligence processing (Fig. 5d). However, the research in the field of small molecule OIM-based memristors is still in its early stage. Some exciting results have been shown in terms of device performance and stability. The ongoing advancement of novel small organic molecules, along with the optimization of device architectures and processing methods, is anticipated to propel this field further and facilitate a wide range of potential applications in areas such as flexible electronics, wearable devices, and neuromorphic computing.
4.2. Zwitterionic OIM-based memristors
Zwitterionic materials refer to a material containing both positive and negative functional groups, showing reversible ion migration behaviors under an electric field. Zwitterion-based memristors are also known as ion conductive memristors, which can realize information storage and retrieval. Zwitterionic memristors offer several advantages, including high durability and stability, reduced power consumption, and scalability. Furthermore, they can exhibit multilevel resistive switching states for memory, logic operations, and neuromorphic computing. Recently, some zwitterionic memristors have been developed and investigated. How to design high-quality zwitterionic materials for high-performance memory devices become an important research topic. Wei et al. designed two molecules named SA-Bu and CA-Bu to explore the impact of conjugation on the properties of IIC materials (Fig. 6a and b).128 These molecules are used as components of the active layer for memristors. As shown in Fig. 6c and d, they found that SA-Bu exhibits favorable ternary memory behavior, displaying excellent conductivity and high sensitivity to NO2 detection at low concentrations. The perfect conjugation of the SA-Bu-based devices allowed for a remarkably low limit of detection, reaching as low as 10 ppb. To assess reproducibility, statistical analysis was performed on 120 samples, revealing an approximate device yield of 41% for the three-component system. Consequently, SA-Bu is able to realize three different resistance states in the memory device cell, giving it more capacity and flexibility for information storage. For CA-Bu, due to the steric distortion of a conjugated structure, it exhibits a binary memory behavior with reduced conductivity and responsiveness to NO2. The implication here is that the establishment and regulation of the conjugated structure play a pivotal role in attaining optimal electrical conductivity and memory performance when designing organic zwitterionic memristors.
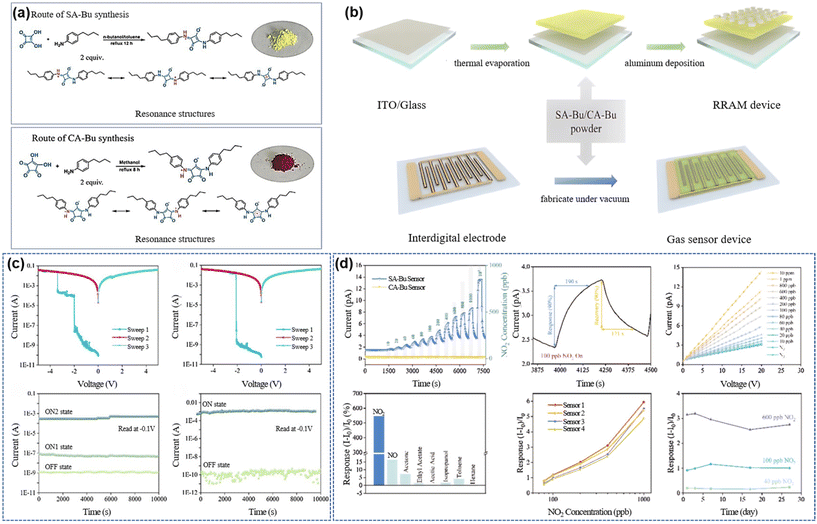 |
| Fig. 6 (a) Synthetic routes of SA-Bu, CA-Bu and their zwitterionic resonance structures. (b) Schematic diagram of fabricating RRAM and gas sensor devices. (c) Typical tri-state I–V and stability test characteristics of SA-Bu/CA-Bu based memory devices. (d) Transient response of SA-Bu and CA-Bu sensors with an increase in NO2 concentration.128 Reprinted with permission from ref. 128, © 2021 Elsevier. | |
Wang et al. adopted commercially available organic dyes, Rhodamine B and Rhodamine 6G, as active materials for low-cost, easy-to-manufacture multilevel RRAM devices.123 The as-fabricated RRAM exhibits a ternary write-once-read-many (WORM) type memory behavior with a retention time of up to 5000 s. Due to the strong intermolecular interactions between the dye molecules, these rhodamine-based memristors can work stably at temperatures up to 80 °C. Furthermore, they demonstrate that the flexible RRAMs can be fabricated on different substrates, such as polyethylene terephthalate (PET), postage stamps and leaves, while maintaining their retention even after being bent more than a thousand times. The research in the field of zwitterionic memristors is still in its nascent stage, yet it has exhibited promising device performance and functionality. The ongoing advancement of novel materials incorporating amphoteric ions, along with the optimization of the device architecture and manufacturing techniques, is in urgent need.
4.3. Polymer OIM-based memristors
Organic polymer memristors generally adopted D/A conjugated polymers as the active layer. These kinds of devices can operate by modulating the charge transport properties of the polymer under an external electric field, resulting in resistance changes and non-volatile memory behavior. Organic polymer memristors often exhibit high switching speeds and large on/off ratios, making them highly attractive for applications in high-speed memory and neuromorphic computing. In the field of organic polymer memristors, researchers are striving to improve the performance and stability of these devices. Through optimization of the geometric construction, ratio and synthesis method of the polymer, superior electrical conductivity and memristive properties have been achieved. As shown in Fig. 7a and b, Zhao et al. successfully designed and synthesized a series of D/A conjugated polymer PCVTPA by directly coupling cation–anion V-TPA units containing sulfur (PSVTPA), selenium (PSeVTPA) and tellurium (PTeVTPA).97 The sandwich structure device of Al/PCVTPA/ITO showed a typical memristive effect. By incorporating S, Se and Te into the V-TPA unit, the device demonstrated superior performance. The process of simulating the “learning, forgetting, and re-learning” of the human brain is achieved by applying continuous pulses. The third time it reaches the same learning level only requires 15 pulses out of the first 50 continuous pulses, while simultaneously exhibiting a significantly slower rate of forgetting compared to the second time (Fig. 7c and d). This study achieves the integration of high-performance synaptic simulation and brain-like computing functions within a single electronic device, presenting a novel approach to build intelligent computing systems. The research demonstrates the significant potential of this material in constructing artificial neural networks for neuromorphic computing and intelligent computing systems, which is capable of performing arithmetic operations on decimal numbers.
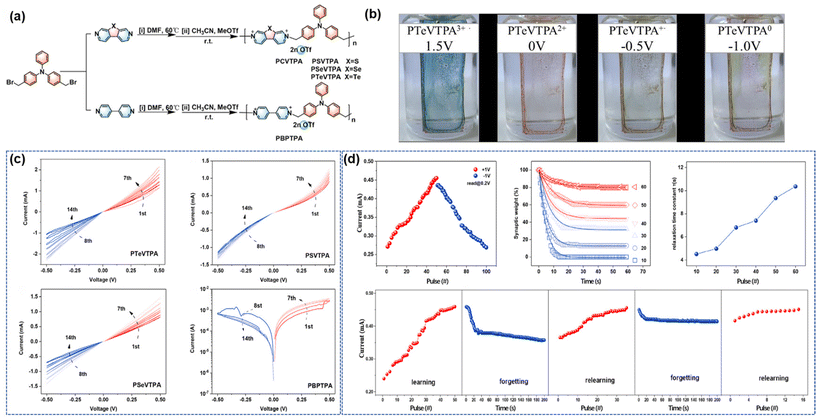 |
| Fig. 7 (a) Synthesis of PCVTPA (PSVTPA, PSeVTPA, PTeVTPA) and PBPTPA. (b) Electrochromic photographs of the PTeVTPA thin film coated onto the ITO substrate. (c) I–V characteristics of the Al/polymer/ITO devices. (d) Synaptic function simulation in human brain memory behavior, including repetitive learning forgetting (STP/STD).97 Reprinted with permission from ref. 97 © 2021 Open Access. | |
Wang et al. combined a MXene/thermoplastic polyurethane (TPU) tensile sensor to construct an Ag ionic polymer–metal composite (IPMC) actuator, a new flexible threshold switch (TS) device for an unconditional reflective arc system.124 By utilizing the internal silver nanowire (NW) channel, the system can be adjusted according to varying operating conditions, which can effectively emulate a fundamental reflection arc and enable basic self-control capabilities. NW-based memristors exhibit significant potential for various applications, because of their simple preparation process, versatile modulation constraints and inherent self-recovery characteristics. The relative resistance change rate reaches a remarkable value of 525.62 when the maximum effective stretching reaches 40%. Simultaneously, the actuator demonstrates its reversibility and reliability by withstanding 10 cycles of cyclic voltage switching from 3 to −3 V. The field of polymer OIM-based memristors has witnessed significant advancements in recent years, with numerous studies highlighting exceptional device performance and stability.83 However, challenges persist in terms of achieving prolonged stability and reproducibility. Continuous development of novel conjugated polymer OIMs, alongside the optimization of device architecture and processing techniques, is anticipated to propel this field forward.
4.4. Complex OIM-based memristors
Organic complex memristors utilize an organic complex as the active layer, which is composed of central metal ions and organic ligands. The performance of memristors can be regulated by adjusting the properties of metal ions and ligands. Compared with traditional inorganic memristors, organic memristors have higher adjustability and controllability, offering multi-level resistance changes and fast switching speeds. Similar to organic small molecule memristors, organic complex memristors also possess the advantage of low power consumption, flexibility and ease of processing. For organic complexes, they have been extensively investigated to improve the device performance through the optimization of the coordination environment, complex structure and ligand selection. For example, diverse storage behaviors and performances can be achieved by conducting devices under various synthetic conditions. Zhang et al. proposed a simple interfacial synthesis method and successfully prepared large-area 2D coordination polymer films.84 The polymer film can be transferred to any independent substrate, providing the possibility to develop solution-processed non-volatile memory devices. The device exhibits a stable current of about 0.04 A at a constant voltage stress of 0.5 V, lasting up to 104 s, maintaining a high on/off current ratio of 103, and precise control of the on and off states ensures the memory device's low misreading rate. This work demonstrates an innovative approach to designing memory devices based on 2D coordination polymer films through interface nanostructure engineering, and thus, proposing new ideas for the further development of non-volatile memory devices.
As shown in Fig. 8a and b, Cheng et al. reported the successful fabrication of ternary resistive random access memory (RRAM) devices using 1D π–d conjugated coordination polymer chains as the active layer.93 The two polymers, Ni-BTA and Ni-BPTA, were synthesized via a solution-based method and exhibited good thermal stability, attributed to their robust intermolecular interactions. As shown in Fig. 8c and d, these materials demonstrate maximum switching current ratios as high as 107 and 108, and all three levels of both aged devices can last and read for more than 104 s after 100 days of storage. High temperature stability and long-term environmental durability make it very promising for next-generation data storage through RRAM technology. Besides, these materials also own potential applications in molecular-based ferromagnets, synthetic metal conductors, nonlinear optical materials, iron electricity, and other electronic fields. The significance of molecular structure tuning in the design of organic complex memristors is evident from these studies. Rational design and optimization of molecular structures can facilitate diverse storage behaviors and superior performance. Therefore, this work offers novel ideas and prospects for advancing high-performance and tunable properties in complex OIM-based memristors.
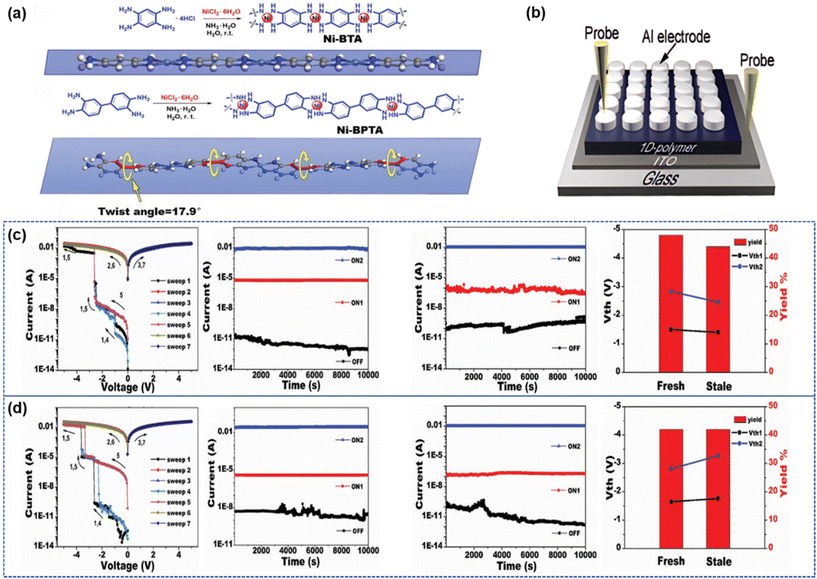 |
| Fig. 8 (a) Synthetic routes and geometry of Ni-BTA and Ni-BPTA. (b) Typical sandwiched structure of memory devices. (c and d) Characteristic I–V curves, stability, threshold voltage and ternary device yield of (c) Ni-BTA and (d) Ni-BPTA.93 Reprinted with permission from ref. 93, © 2017 Wiley. | |
4.5. Other OIM-based memristors
In addition to the aforementioned types of memristors, there are several other types of memristors that have been investigated in recent years. For instance, an organic composite memristor contains the interaction between the organic and inorganic components.129 Zhao et al. reported the synthesis of Au and Ag nanoparticles (NPs) embedded in 3D Cd-based MOF matrixes via a photoreduction method, producing Au(Ag)–NP@MOF composite materials.87 Electrical bistability measurements on the sandwiched ITO/NP@MOF/Ag device showed two switchable conductivity states of the device with non-volatile memory behaviors. This was attributed to an electric-field-induced charge transfer process aided by conformational changes in the active layer. Among the tested samples, the ITO/Au NP@2/Ag device with a neutral MOF matrix had the highest ON/OFF current ratio of 104, owing to a better dispersion of Au NPs in the MOF matrix resulting in more efficient electron tunneling.
Another type is a bio-memristor, inspired by biological systems like synaptic connections between neurons in the brain.130–133 Zhang et al. reported the fabrication of an ultra-thin bio-memristor based on silk nanofibrils (SNFs) (Fig. 9a). As shown in Fig. 9b–g, the SNF-based device showed a low operating voltage, good stability and environmental friendliness.118 Besides, the device demonstrated a high dielectric performance due to its intrinsic ionic conductivity and nanoscale thickness. As a result, the SNF-based memristor presented stable resistive switching over 180 cycles and a retention time of up to 105 s. The integration of SNF-based memristors into a crossbar array achieves consistent memristive performance for image memorization as well as logic operation functions. All of these types of memristors offer unique advantages and opportunities, prompting ongoing research efforts to develop novel materials and devices that can optimize their performance and facilitate new applications.
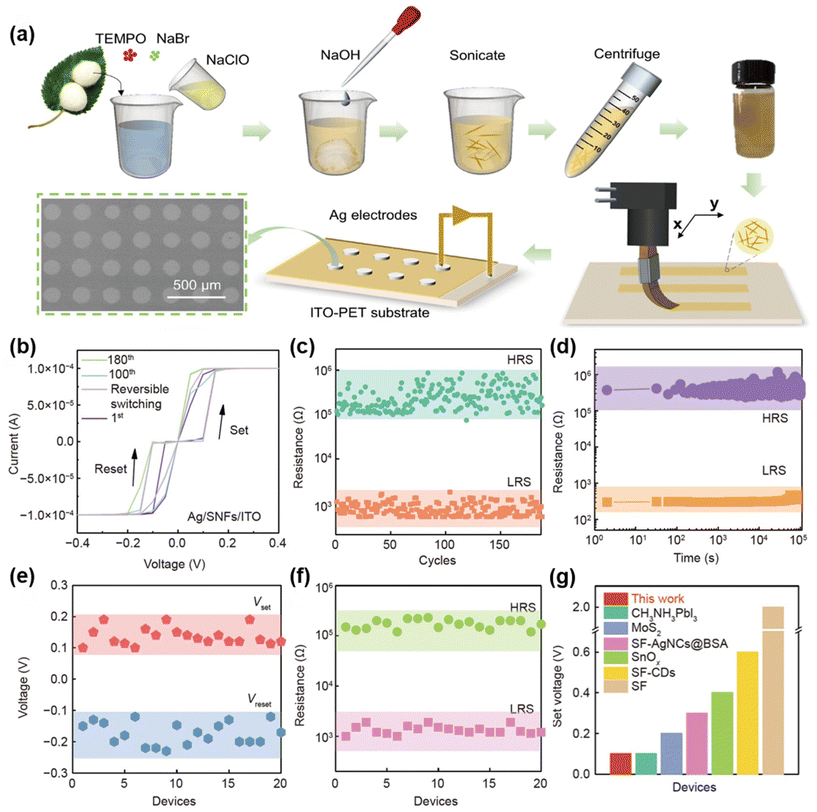 |
| Fig. 9 (a) Schematic diagram illustrating the preparation of the ultra-thin SNF-based memristor. (b) Typical I–V characteristics of the SNF-based memristor. (c) Endurance performance and (d) time retention measurements of the Ag/SNFs/ITO memristor. (e and f) The Vset, Vreset and the resistance distributions of Ag/SNFs/ITO memristors. (g) Comparison of the set voltages for different memristors.118 Reprinted with permission from ref. 118, © 2022 Science China Press and Springer. | |
5. Extended applications
The unique properties of memristors, such as nonvolatile memory, low power consumption, and analog computing capabilities, have demonstrated significant potential in various device applications.134,135 Specifically, OIM-based memristors offer distinct advantages in neural simulation, brain–computer interfaces and the efficient photoelectric effect, due to their utilization of ionic–electronic materials with exceptional properties.136–138 Some distinct properties of OIM-based memristors should be noted. Firstly, OIM-based memristors have good biocompatibility and can be directly integrated into organisms, providing the possibility to build bioelectronic interfaces. The polymorphism properties of OIMs can complete different oxidation states, simulating the potential functionality of neurons and multi-level data-storage behavior, thereby facilitating the realization of a more realistic neural network. OIMs can be easily prepared by simple solution deposition or printing techniques, which hold promise to produce large-scale neural networks. By adjusting the chemical structure of OIMs, their electrical properties can be precisely regulated. Therefore, the electrophysiological parameters of various neurons can be customized. Additionally, some OIMs have strong photo-responsiveness, which can directly convert light signals into electrical signals, meaning that the highly sensitive optoelectronic neurons can be mimicked. Consequently, OIMs play a significant role in fabricating flexible devices, which is conducive to achieving wearable neuroelectronic applications.
The utilization of OIM-based memristive materials offers distinct advantages in the field of biomimetic electronics, facilitating efficient emulation of neural networks and interfaces for human–computer interaction.138 Through fast switching and programmable conductivity states, these materials are able to mimic the excitatory and inhibitory states of neurons, enabling intricate computations within neural networks. In addition, its low power consumption and high stability render it highly suitable for the development of brain–computer interfaces.137 By integrating with biocompatible materials, OIMs can effectively interact with the human brain and promote the advancement of brain–computer interface technology. Meanwhile, OIMs also exhibit a diverse range of photosensitive properties, which can achieve fast and reversible responses. Such characteristics will greatly actuate the advancement of efficient light control devices and optical memory.136 OIMs have broad application prospects in the fields of biomimetic electronics, intelligent computing and medical electronics.
5.1. Simulated biological synapse simulation
The importance of human brain synapses in memory and learning lies in their remarkably low power consumption and superior performance compared to supercomputers.60 To develop suitable electronics for artificial neural networks (ANNs), the OIM-based device must demonstrate energy efficiency comparable to that of human brain synapses.139,140 Synapse simulation is considered an important step toward efficient ANNs similar to the brain.141,142 However, previous attempts to take advantage of complementary metal–oxide–semiconductor (CMOS) technology have resulted in increased energy consumption and complexity, posing challenges for high-density neural networks.143 By contrast, Zhang et al. produced an artificial synapse based on flexible PET substrates, exhibiting unique history-dependent memory behaviors,86 analogous to the synaptic enhancement and inhibition in neurobiology. The device also showed basic synaptic plasticity and some human memory and learning behaviors. Furthermore, the study showed that even with a bending radius of less than 10 mm, the device still showed a unique synaptic performance. This indicates the potential utilization of such devices in constructing wearable neuromorphic computing systems. In addition to mimicking neural pathways in the brain, OIMs are also used for simulating biological behaviors. Wang et al. presented an innovative artificial device to replicate the bladder relaxation reflex arc found in biological systems (Fig. 10a).124 This device successfully converted continuous extension signals into control signals, adhering to the all-or-nothing principle. As shown in Fig. 10c and d, by combining an MXene/TPU tensile sensor, an Ag IPMC actuator and a new flexible threshold-switching device, they constructed an unconditional reflective arc system, with a 10%–40% strain range similar to bladder expansion and a maximum strain response up to 225%. As the bladder expands when filled with fluid, the sensor is stretched and modulates the memristor's resistance through parallel connection, triggering the actuator contraction upon reaching a threshold to simulate the bladder relaxation reflex. They proposed to use flexible materials with neuromorphological control in medical and intelligent robotics applications, while simulating unconditional reflexes through the utilization of threshold-switching memristors as sympathetic nerve centers.
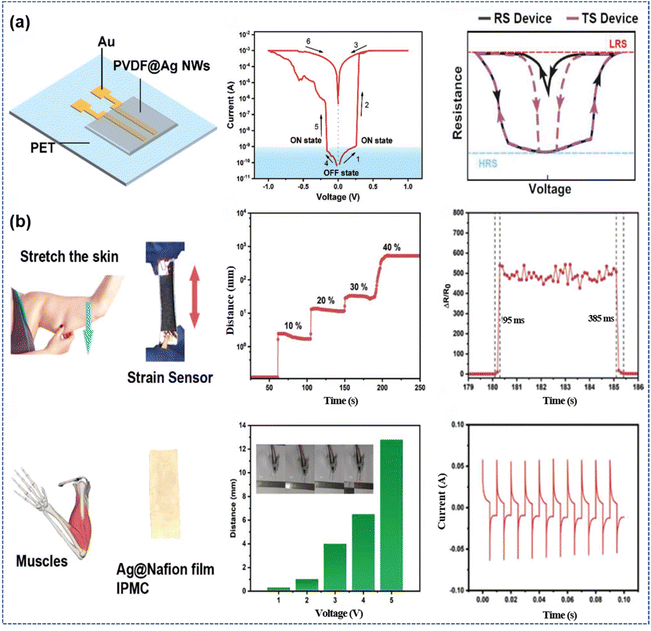 |
| Fig. 10 (a) Statistical diagram of urine accumulation and excretion in the bladder and schematic representation of the resistance. (b) The bladder diastolic reflex and corresponding electrical tests.124 Reprinted with permission from ref. 124, © 2022 Wiley. | |
5.2. High-density data storage
The utilization of OIMs in memristors presents numerous advantages in the realm of high-density data storage. These devices possess non-volatile memory properties, enabling them to retain the as-stored data for extended periods even during power outages.144 This property exhibits enormous advantages in scenarios involving temporary power disruptions or interruptions. Owing to their operating mechanism based on ion transport or charge injection, organic iontronic memristors can achieve higher information densities compared to conventional memory technology.145,146 Therefore, OIM-based memristor holds high promise for large-scale high-density data storage. Sun et al. designed an IGZO-based memristor regulated by DEME-TFSI ionic liquids. A memristor array was integrated to produce a true random number generator for secure communication or radar waveform design, etc.96 They found that such devices could be applied not only for data storage, but also opened up potential applications in cryptography. Besides, this device showed high packing density and excellent compatibility with the existing CMOS processes. Therefore, this work paves the way for advancing nanoscale technologies that can potentially supplant current CMOS technologies. In addition, OIM-based memristors also favor achieving high-frequency writing/reading speeds and low power consumption. Wang et al. successfully fabricated cost-effective and easily manufacturable multilevel RRAM using commercially available dyes Rhodamine B and Rhodamine 6G.123 The as-fabricated RRAM exhibited ternary WORM-type memory behaviors, which could be attributed to the strong intermolecular interactions between the dye molecules. These rhodamine-based reservoirs could even work stably at temperatures up to 80 °C.
5.3. Integrated photovoltaic memristors
The application of memristors in optoelectronic devices also presents broad prospects. By combining memristors with optoelectronic elements, it becomes feasible to realize memristor-based optoelectronic memory devices for controlling and storing optical properties.147 Simultaneously, memristors can also be used to achieve photoelectric conversion devices, thereby converting light energy into electrical energy or vice versa. By introducing light-absorbing materials into the device, OIM-based memristors can realize efficient photoelectric conversion. These applications will play a vital role in the fields of photoelectric information, photoelectric sensing and detection, and photoelectric devices, offering vast development potential.148
Yang et al. carried out the development of photovoltaic memristors based on bulk photovoltaic effect (BPVE) devices with similar synaptic plasticity to biological synapses.127 The photovoltaic synaptic plasticity in BPVE devices is closely associated with the dynamics of domain switching in ferroelectric thin films. This device demonstrates highly discernible changes in the photovoltaic response that can be continuously adjusted from low to high levels. Additionally, spike timing-related plasticity (STRP) can be harnessed through the diverse modes of domain switching dynamics of double pulses. Photovoltaic memristors employ optical reading instead of high-frequency electrical reading, thereby increasing the reading speed and reducing the energy consumption.
6. Conclusions and perspective
In summary, OIMs and their memristors, as a novel category of memristive materials and devices, have received increasing attention due to their advantages such as a high switching ratio, low power consumption, substantial switching durability, and compatibility with flexible substrates.149 Over the past decade, they have been extensively investigated. These newly-designed materials and their doped complexes have significantly enhanced device performance, making them suitable for various applications, including neuromorphic computing, artificial intelligence, energy storage, and so on.150 In this review, we introduced the basic concept and synthetic methods of OIMs, and discussed the various applications of OIM-based memristors. Their advantages relative to other memristors were also highlighted. The different types of OIMs possess different transmission speeds, power consumption, storage capacities, etc. Therefore, the functionality of organic iontronic memristors can be determined by a comprehensive analysis. The topics covered in this section encompass ion transport kinetics and some other typical memory mechanisms. For OIMs, the kinetics of ion transport is a core factor influencing the performance of iontronic devices, including response speed, power consumption and stability of the devices. The modulation strategies of ion transport kinetics involve the design and optimization of the device structure, materials selection, device interface engineering, etc., which can be used to improve the efficiency and controllability of ion transport. Therefore, an in-depth understanding and control of ion transport kinetics will provide important guidance for the development of ion electronics. By exploring new materials, interface engineering, and optimizing device structures, it is expected to further improve the efficiency and controllability of ion transport properties, bringing more opportunities for the application fields of ion electronics.
Although significant progress has been achieved in the development of OIMs as well as their memristors, some challenges still remain to be addressed in the future. Firstly, the switching speed and response time of OIM-based memristors need to be further improved through interface engineering and nanostructure optimization to meet the demands of high-speed information processing and transmission. For example, novel electrode materials and device architectures could be explored to lower the energy barriers for ion migration. Secondly, the durability and cyclability of OIM-based devices should be enhanced by improving material stability and conducting appropriate encapsulation. The device lifespan could be extended by inhibiting the side reactions between the active layer and electrodes. Developing OIMs with high thermal/chemical stability is also vital. Thirdly, new electrode materials such as graphene, carbon nanotubes and conductive polymers could be explored to improve the charge injection efficiency at the electrode/OIM interface. Appropriate electrode selection and surface modification are keys to achieving ohmic contacts. Fourthly, more theoretical and experimental efforts are still needed to reveal the detailed ion transport mechanisms and kinetics in OIMs. Advanced characterization techniques, such as conductive atomic force microscopy, could provide valuable insights. Molecular dynamics simulations may help understand the ion conduction processes. Fifthly, the integration and compatibility of OIM-based memristors with CMOS circuits remain a challenge. Innovative device fabrication processes and creative circuit designs are required to address this integration issue. Finally, new OIMs with superior comprehensive performances, including high speed, large ON/OFF ratio, long endurance, and excellent stability, need to be developed through molecular design, synthesis and nanoengineering. Systematic material optimization is the key to achieving ideal OIMs.156,157 We believe that with continuous research efforts, the challenges faced by OIM-based memristive devices could be gradually conquered, unleashing their application potential in next-generation electronics.
Overall, OIM-based memristors have demonstrated great potential in various applications, such as data storage, artificial intelligence, neuromorphic computing, energy storage, sensors and other fields.158,159 We believe that the challenges discussed here can be effectively solved through collaborative efforts. Future work on OIMs will undoubtedly continue to pave the way for further development of memristive devices, and we remain optimistic regarding the applications of these devices in the forthcoming years. With the advancement of technology and the expansion of prospects, iontronic memristors will play a significant role in the future intelligent electronic field.
Author contributions
The manuscript was written through contributions of all authors. All authors have given approval to the final version of the manuscript.
Conflicts of interest
The authors declare no competing financial interest.
Acknowledgements
Y. L. acknowledges financial support from the National Natural Science Foundation of China (Grants No. 22008164 and 62304148), the Natural Science Foundation of Jiangsu Province (Grants No. BK20230074), the Youth Talent Support Program of Jiangsu Association for Science and Technology (TJ-2023-033), the Youth Talent Support Program of Suzhou Association for Science and Technology (2023-04), the Natural Science Foundation of the Jiangsu Higher Education Institutions of China (Grants No. 22KJB150037), and the Foundation of Key Laboratory of Synthetic and Biological Colloids, Ministry of Education, Jiangnan University (No. 1042050205225990/007). This work is also supported by the Postgraduate Research & Practice Innovation Program of Jiangsu Province (KYCX23_3304), Jiangsu Key Disciplines of the Fourteenth Five-Year Plan (2021135), and the Suzhou Key Laboratory for Low Dimensional Optoelectronic Materials and Devices (SZS201611), and Research in Cutting-Edge Technology of Suzhou (SYG202351).
References
- D. Feng, Z. Niu, J. Yang, W. Xu, S. Liu, X. Mao and X. Li, Nano Energy, 2021, 90, 106526 CrossRef CAS.
- A. M. Ionescu and H. Riel, Nature, 2011, 479, 329–337 CrossRef CAS PubMed.
- X. Zhao, Z. Wang, Y. Xie, H. Xu, J. Zhu, X. Zhang, W. Liu, G. Yang, J. Ma and Y. Liu, Small, 2018, 14, 1801325 CrossRef PubMed.
- J. B. Roldan, E. Miranda, D. Maldonado, A. N. Mikhaylov, N. V. Agudov, A. A. Dubkov, M. N. Koryazhkina, M. B. Gonzalez, M. A. Villena, S. Poblador, M. Saludes-Tapia, R. Picos, F. Jimenez-Molinos, S. G. Stavrinides, E. Salvador, F. J. Alonso, F. Campabadal, B. Spagnolo, M. Lanza and L. O. Chua, Adv. Intell. Syst., 2023, 5, 6 Search PubMed.
- F. Zahoor, T. Z. Azni Zulkifli and F. A. Khanday, Nanoscale Res. Lett., 2020, 15, 90 CrossRef CAS PubMed.
- Y. Ren, C.-L. Chang, L.-Y. Ting, L. Zhou, J.-Y. Mao, S.-R. Zhang, H.-H. Chou, J.-Q. Yang, Y. Zhou and S.-T. Han, Adv. Intell. Syst., 2019, 1, 1900008 CrossRef.
- N. Raeis-Hosseini, Y. Park and J.-S. Lee, Adv. Funct. Mater., 2018, 28, 1800553 CrossRef.
- Y.-Y. Zhao, W.-J. Sun, J. Wang, J.-H. He, H. Li, Q.-F. Xu, N.-J. Li, D.-Y. Chen and J.-M. Lu, Adv. Funct. Mater., 2020, 30, 2004245 CrossRef CAS.
- Y. G. Song, J. M. Suh, J. Y. Park, J. E. Kim, S. Y. Chun, J. U. Kwon, H. Lee, H. W. Jang, S. Kim, C.-Y. Kang and J. H. Yoon, Adv. Sci., 2022, 9, 2103484 CrossRef CAS PubMed.
- H. Xiong, S. Ling, Y. Li, F. Duan, H. Zhu, S. Lu and M. Du, J. Colloid Interface Sci., 2022, 608, 1126–1134 CrossRef CAS PubMed.
- R. Sarpeshkar, Neural Comput., 1998, 10, 1601–1638 CrossRef CAS PubMed.
- H. Chun and T. D. Chung, Annu. Rev. Anal. Chem., 2015, 8, 441–462 CrossRef CAS PubMed.
- M. Onoda, IEEJ Trans. Electr. Electron. Eng., 2020, 15, 320–334 CrossRef CAS.
- Y. Hou and X. Hou, Science, 2021, 373, 628–629 CrossRef CAS PubMed.
- T. Li and K. Xiao, Adv. Mater. Technol., 2022, 7, 2200205 CrossRef.
- R. J. Sengwa, V. K. Patel and M. Saraswat, J. Polym. Res., 2022, 29, 480 CrossRef CAS.
- G. Laucirica, M. E. Toimil-Molares, C. Trautmann, W. Marmisoll and O. Azzaroni, ACS Appl. Mater. Interfaces, 2020, 12, 28148–28157 CrossRef CAS PubMed.
- P. Zhang, W. Guo, Z. H. Guo, Y. Ma, L. Gao, Z. Cong, X. J. Zhao, L. Qiao, X. Pu and Z. L. Wang, Adv. Mater., 2021, 33, 2101396 CrossRef CAS PubMed.
- J. Yu, Y. Wang, S. Qin, G. Gao, C. Xu, Z. L. Wang and Q. Sun, Mater. Today, 2022, 60, 158–182 CrossRef CAS.
- J. Zhang, W. Liu, J. Dai and K. Xiao, Adv. Sci., 2022, 9, 2200534 CrossRef CAS PubMed.
- A. Zhang and C. M. Lieber, Chem. Rev., 2016, 116, 215–257 CrossRef CAS PubMed.
- Y. Lee, J. Park, A. Choe, S. Cho, J. Kim and H. Ko, Adv. Funct. Mater., 2020, 30, 1904523 CrossRef CAS.
- C. Yang and Z. Suo, Nat. Rev. Mater., 2018, 3, 125–142 CrossRef CAS.
- K. Raidongia and J. Huang, J. Am. Chem. Soc., 2012, 134, 16528–16531 CrossRef CAS PubMed.
- P. Jia, L. Wang, Y. Zhang, Y. Yang, X. Jin, M. Zhou, D. Quan, M. Jia, L. Cao, R. Long, L. Jiang and W. Guo, Adv. Mater., 2021, 33, 2007529 CrossRef CAS PubMed.
- H. Kim, M. Kim, A. Lee, H.-L. Park, J. Jang, J.-H. Bae, I. M. Kang, E.-S. Kim and S.-H. Lee, Adv. Sci., 2023, 10, 19 Search PubMed.
- S.-H. Lee, H.-L. Park, C.-M. Keum, I.-H. Lee, M.-H. Kim and S.-D. Lee, Phys. Status Solidi RRL, 2019, 13, 1900044 CrossRef.
- S.-H. Lee, H.-L. Park, M.-H. Kim, S. Kang and S.-D. Lee, ACS Appl. Mater. Interfaces, 2019, 11, 30108–30115 CrossRef CAS PubMed.
- J. Li, Y. Qian, W. Li, S. Yu, Y. Ke, H. Qian, Y.-H. Lin, C.-H. Hou, J.-J. Shyue, J. Zhou, Y. Chen, J. Xu, J. Zhu, M. Yi and W. Huang, Adv. Mater., 2023, 35, 23 Search PubMed.
- J. Meng, Z. Li, Y. Fang, Q. Li, Z. He, T. Wang, H. Zhu, L. Ji, Q. Sun, D. W. Zhang and L. Chen, IEEE Electron Device Lett., 2022, 43, 2069–2072 CAS.
- H.-L. Park, M.-H. Kim, M.-H. Kim and S.-H. Lee, Nanoscale, 2020, 12, 22502–22510 RSC.
- J. Shi, S. Kang, J. Feng, J. Fan, S. Xue, G. Cai and J. S. Zhao, Nanoscale Horiz., 2023, 8, 509–515 RSC.
- Y. Wang, Y. Gong, L. Yang, Z. Xiong, Z. Lv, X. Xing, Y. Zhou, B. Zhang, C. Su, Q. Liao and S.-T. Han, Adv. Funct. Mater., 2021, 31, 2100144 CrossRef CAS.
- Y. Pei, L. Yan, Z. Wu, J. Lu, J. Zhao, J. Chen, Q. Liu and X. Yan, ACS Nano, 2021, 15, 17319–17326 CrossRef CAS PubMed.
- J.-L. Meng, T.-Y. Wang, Z.-Y. He, L. Chen, H. Zhu, L. Ji, Q.-Q. Sun, S.-J. Ding, W.-Z. Bao, P. Zhou and D. W. Zhang, Mater. Horiz., 2021, 8, 538–546 RSC.
- M. Kumar, Y. H. Ahn, S. Iqbal, U. Kim and H. Seo, Small, 2022, 18, 2105585 CrossRef CAS PubMed.
- Y. Li, Y. Pan, C. Zhang, Z. Shi, C. Ma, S. Ling, M. Teng, Q. Zhang, Y. Jiang, R. Zhao and Q. Zhang, ACS Appl. Mater. Interfaces, 2022, 14, 44676–44684 CrossRef CAS PubMed.
- Z. Wang, L. Wang, Y. Wu, L. Bian, M. Nagai, R. Jv, L. Xie, H. Ling, Q. Li, H. Bian, M. Yi, N. Shi, X. Liu and W. Huang, Adv. Mater., 2021, 33, 2104370 CrossRef CAS PubMed.
- X. Zhao, J. Xu, D. Xie, Z. Wang, H. Xu, Y. Lin, J. Hu and Y. Liu, Adv. Mater., 2021, 33, 2104023 CrossRef CAS PubMed.
- C. Zhang, Y. Li, F. Yu, G. Wang, K. Wang, C. Ma, X. Yang, Y. Zhou and Q. Zhang, Nano Energy, 2023, 109, 108274 CrossRef CAS.
- C. Zhang, X. Wang, Y. Li, Y. Sun and Q. Zhang, Chem. – Eur. J., 2023, 29, e202300481 CrossRef CAS PubMed.
- H. Patil, H. Kim, K. D. Kadam, S. Rehman, S. A. Patil, J. Aziz, T. D. Dongale, Z. A. Sheikh, M. K. Rahmani, M. F. Khan and D.-K. Kim, ACS Appl. Mater. Interfaces, 2023, 15, 13238–13248 CrossRef CAS PubMed.
- X. Wen, W. Tang, Z. Lin, X. Peng, Z. Tang and L. Hou, Appl. Phys. Lett., 2023, 122, 173301 CrossRef CAS.
- M. Zhang, C. Ma, D. Du, J. Xiang, S. Yao, E. Hu, S. Liu, Y. Tong, W.-Y. Wong and Q. Zhao, Adv. Electron. Mater., 2020, 6, 2000841 CrossRef CAS.
- H. Yang, Z. Wang, X. Guo, H. Su, K. Sun, D. Yang, W. Xiao, Q. Wang and D. He, ACS Appl. Mater. Interfaces, 2020, 12, 34370–34377 CrossRef CAS PubMed.
- D. Goldhaber-Gordon, M. S. Montemerlo, J. C. Love, G. J. Opiteck and J. C. Ellenbogen, Proc. IEEE, 1997, 85, 521–540 CrossRef CAS.
- P. Zhang, M. Xia, F. Zhuge, Y. Zhou, Z. Wang, B. Dong, Y. Fu, K. Yang, Y. Li, Y. He, R. H. Scheicher and X. S. Miao, Nano Lett., 2019, 19, 4279–4286 CrossRef CAS PubMed.
- C. Li, L. Han, H. Jiang, M. H. Jang, P. Lin, Q. Wu, M. Barnell, J. J. Yang, H. L. Xin and Q. Xia, Nat. Commun., 2017, 8, 15666 CrossRef CAS PubMed.
- S. Choi, S. Jang, J.-H. Moon, J. C. Kim, H. Y. Jeong, P. Jang, K.-J. Lee and G. Wang, NPG Asia Mater., 2018, 10, 1097–1106 CrossRef CAS.
- S. Kim, S. Jung, M. H. Kim, Y. C. Chen, Y. F. Chang, K. C. Ryoo, S. Cho, J. H. Lee and B. G. Park, Small, 2018, 14, 1704062 CrossRef PubMed.
- F. Wan, Q. Wang, T. Harumoto, T. Gao, K. Ando, Y. Nakamura and J. Shi, Adv. Funct. Mater., 2020, 30, 2007101 CrossRef CAS.
- M. Ali, A. Sokolov, M. J. Ko and C. Choi, J. Alloys Compd., 2021, 855, 157514 CrossRef CAS.
- Q. Liao, Y. Wang, Z. Lv, Z. Xiong, J. Chen, G. P. Wang, S.-T. Han and Y. Zhou, Org. Electron., 2021, 90, 106062 CrossRef CAS.
- J. Chen, Z. Feng, M. Luo, J. Wang, Z. Wang, Y. Gong, S. Huang, F. Qian, Y. Zhou and S.-T. Han, J. Mater. Chem. C, 2021, 9, 15435–15444 RSC.
- U. Das, P. Sarkar, B. Paul and A. Roy, Appl. Phys. Lett., 2021, 118, 182103 CrossRef CAS.
- A. S. Sokolov, M. Ali, R. Riaz, Y. Abbas, M. J. Ko and C. Choi, Adv. Funct. Mater., 2019, 29, 1807504 CrossRef.
- K.-H. Son and H.-S. Lee, Appl. Surf. Sci., 2022, 575, 151754 CrossRef CAS.
- K. Sun, Q. Wang, L. Zhou, J. Wang, J. Chang, R. Guo, B. K. Tay and X. Yan, Sci. China Mater., 2023, 66, 2013–2022 CrossRef CAS.
- C. Wang, Z. Si, X. Jiang, A. Malik, Y. Pan, S. Stathopoulos, A. Serb, S. Wang, T. Prodromakis and C. Papavassiliou, IEEE J. Emerging Sel. Top. Circuits, 2022, 12, 723–734 Search PubMed.
- X. Yang, B. Taylor, A. Wu, Y. Chen and L. O. Chua, IEEE T. Circuits-I, 2022, 69, 1845–1857 Search PubMed.
- H. Liao, J. Chen, L. Lan, Y. Yu, G. Zhu, J. Duan, X. Zhu, H. Dai, M. Xiao, Z. Li, W. Yue and I. McCulloch, ACS Appl. Mater. Interfaces, 2022, 14, 16477–16486 CrossRef CAS PubMed.
- J. Qiu, D. Zeng, Y. Lin, W. Ye, C. Chen, Z. Xu, G. Hu and Y. Liu, Spectrochim. Acta, Part A, 2023, 285, 121913 CrossRef CAS PubMed.
- H. Niu, M. Ding, X. Li, X. Su, X. Han, N. Zhang, P. Guan and X. Hu, Mater. Chem. Phys., 2023, 293, 126971 CrossRef CAS.
- Y. Yuan, B. Wang, K. Xue, Y. Ma, X. Liu, X. Peng, M. Liu and H. Lu, ACS Appl. Mater. Interfaces, 2023, 15, 17144–17151 CrossRef CAS PubMed.
- H. Cheng and J. Ouyang, J. Phys. Chem. Lett., 2022, 13, 10830–10842 CrossRef CAS PubMed.
- A. Wolny and A. Chrobok, Molecules, 2022, 27, 5900 CrossRef CAS PubMed.
- M. Zunita, R. Hastuti, A. Alamsyah, G. T. M. Kadja, K. Khoiruddin, K. A. Kurnia, B. Yuliarto and I. G. Wenten, J. Ind. Eng. Chem., 2022, 113, 96–123 CrossRef CAS.
- H. Lv, L. Wei, S. Guo, X. Zhang, F. Chen, X. Qin, C. Wei, B. Jiang and Y. Gong, Front. Chem., 2022, 10, 807088 CrossRef CAS PubMed.
- J. Fu, J.-Y. Liu, G.-H. Zhang, Q.-H. Zhu, S.-L. Wang, S. Qin, L. He and G.-H. Tao, Small, 2023, 2302570 CrossRef CAS PubMed.
- M. Lee, M.-S. Kim, J.-M. Oh, J. K. Park and S.-M. Paek, ACS Nano, 2023, 17, 3019–3036 CrossRef CAS PubMed.
- S. F. Zhao, W. H. Ran, Z. Lou, L. L. Li, S. Poddar, L. L. Wang, Z. Y. Fan and G. Z. Shen, Natl. Sci. Rev., 2022, 9, nwac158 CrossRef CAS PubMed.
- F. Sheng, B. Wu, X. Li, T. Xu, M. A. Shehzad, X. Wang, L. Ge, H. Wang and T. Xu, Adv. Mater., 2021, 33, 2104404 CrossRef CAS PubMed.
- C. Zhang, Y. Li, Y. Zhou, Q. Zhang, H. Li and J. Lu, Chem. Commun., 2018, 54, 10610–10613 RSC.
- C. Yu, J.-H. He, X.-F. Cheng, H.-Z. Lin, H. Yu and J.-M. Lu, Angew. Chem., Int. Ed., 2021, 60, 15328–15334 CrossRef CAS PubMed.
- X. Xiao, X.-F. Cheng, X. Hou, J.-H. He, Q.-F. Xu, H. Li, N.-J. Li, D.-Y. Chen and J.-M. Lu, Small, 2017, 13, 1602190 CrossRef PubMed.
- J. Zhou, X.-F. Cheng, B.-J. Gao, C. Yu, J.-H. He, Q.-F. Xu, H. Li, N.-J. Li, D.-Y. Chen and J.-M. Lu, Small, 2019, 15, 1803896 CrossRef PubMed.
- C. Yu, H.-Z. Lin, J. Zhou, X.-F. Cheng, J.-H. He, H. Li, Q.-F. Xu, N.-J. Li, D.-Y. Chen and J.-M. Lu, J. Mater. Chem. A, 2020, 8, 1052–1058 RSC.
- J. Zhou, H. Lin, X.-F. Cheng, J. Shu, J.-H. He, H. Li, Q.-F. Xu, N.-J. Li, D.-Y. Chen and J.-M. Lu, Mater. Horiz., 2019, 6, 554–562 RSC.
- H. Yuan, H. Shimotani, A. Tsukazaki, A. Ohtomo, M. Kawasaki and Y. Iwasa, J. Am. Chem. Soc., 2010, 132, 6672–6678 CrossRef CAS PubMed.
- P. Bhunia, E. Hwang, M. Min, J. Lee, S. Seo, S. Some and H. Lee, Chem. Commun., 2012, 48, 913–915 RSC.
- K. Rajan, A. Chiappone, D. Perrone, S. Bocchini, I. Roppolo, K. Bejtka, M. Castellino, C. F. Pirri, C. Ricciardi and A. Chiolerio, RSC Adv., 2016, 6, 94128–94138 RSC.
- Y. Li, Y. Song, X. Zhang, X. Wu, F. Wang and Z. Wang, Macromol. Chem. Phys., 2015, 216, 113–121 CrossRef CAS.
- L. Dou, J. Gao, E. Richard, J. You, C. C. Chen, K. C. Cha, Y. He, G. Li and Y. Yang, J. Am. Chem. Soc., 2012, 134, 10071–10079 CrossRef CAS PubMed.
- Z. Zhang, Y. Nie, W. Hua, J. Xu, C. Ban, F. Xiu and J. Liu, RSC Adv., 2020, 10, 20900–20904 RSC.
- D. Wi, J. Kim, H. Lee, N.-G. Kang, J. Lee, M.-J. Kim, J.-S. Lee and M. Ree, J. Mater. Chem. C, 2016, 4, 2017–2027 RSC.
- C. Zhang, Y.-T. Tai, J. Shang, G. Liu, K.-L. Wang, C. Hsu, X. Yi, X. Yang, W. Xue, H. Tan, S. Guo, L. Pan and R.-W. Li, J. Mater. Chem. C, 2016, 4, 3217–3223 RSC.
- L. Zhao, W. Wu, X. Shen, Q. Liu, Y. He, K. Song, H. Li and Z. Chen, ACS Appl. Mater. Interfaces, 2019, 11, 47073–47082 CrossRef CAS PubMed.
- Y. He, X. Hou, Y. Liu and N. Feng, J. Mater. Chem. B, 2019, 7, 5602–5619 RSC.
- Z. Yao, L. Pan, L. Liu, J. Zhang, Q. Lin, Y. Ye, Z. Zhang, S. Xiang and B. Chen, Sci. Adv., 2019, 5, eaaw4515 CrossRef CAS PubMed.
- C. S. Dash, S. Sahoo and S. R. S. Prabaharan, Solid State Ionics, 2018, 324, 218–225 CrossRef CAS.
- C. Yun, M. Webb, W. Li, R. Wu, M. Xiao, M. Hellenbrand, A. Kursumovic, H. Dou, X. Gao, S. Dhole, D. Zhang, A. Chen, J. Shi, K. H. L. Zhang, H. Wang, Q. Jia and J. L. MacManus-Driscoll, J. Mater. Chem. C, 2021, 9, 4522–4531 RSC.
- M. Kim, M. A. Rehman, D. Lee, Y. Wang, D. H. Lim, M. F. Khan, H. Choi, Q. Y. Shao, J. Suh, H. S. Lee and H. H. Park, ACS Appl. Mater. Interfaces, 2022, 14, 44561–44571 CrossRef CAS PubMed.
- X.-F. Cheng, E.-B. Shi, X. Hou, J. Shu, J.-H. He, H. Li, Q.-F. Xu, N.-J. Li, D.-Y. Chen and J.-M. Lu, Adv. Electron. Mater., 2017, 3, 1700107 CrossRef.
- C. Zhang, Y. Li, Z. Li, Y. Jiang, J. Zhang, R. Zhao, J. Zou, Y. Wang, K. Wang, C. Ma and Q. Zhang, ACS Appl. Mater. Interfaces, 2022, 14, 3111–3120 CrossRef CAS PubMed.
- Q. Lu, Y. Xia, F. Sun, Y. Shi, Y. Zhao, S. Wang and T. Zhang, Adv. Mater. Technol., 2023, 2300059 CrossRef CAS.
- B. Sun, S. Ranjan, G. Zhou, T. Guo, C. Du, L. Wei, Y. N. Zhou and Y. A. Wu, ACS Appl. Electron. Mater., 2021, 3, 2380–2388 CrossRef CAS.
- Z. Zhao, Q. Che, K. Wang, M. E. El-Khouly, J. Liu, Y. Fu, B. Zhang and Y. Chen, iScience, 2022, 25, 103640 CrossRef CAS PubMed.
- T. S. Badessa and V. A. Shaposhnik, Electrochim. Acta, 2017, 231, 453–459 CrossRef CAS.
- G. Cho, Y. Park, Y.-K. Hong and D.-H. Ha, Nano Converg., 2019, 6, 17 CrossRef PubMed.
- I. Bejanidze, O. Petrov, V. Pohrebennyk, T. Kharebava, N. Nakashidze, N. Didmanidze, N. Davitadze and A. Petrov, Appl. Sci., 2020, 10, 7383 CrossRef CAS.
- G. Yilmaz, Polymers, 2019, 11, 1577 CrossRef CAS PubMed.
- M. A. Tasdelen, J. Lalevee and Y. Yagci, Polym. Chem., 2020, 11, 1111–1121 RSC.
- L. Breloy, O. Yavuz, I. Yilmaz, Y. Yagci and D.-L. Versace, Polym. Chem., 2021, 12, 4291–4316 RSC.
- K. Luo, H. Chen, Q. Zhou, Z. Yan, Z. Su and K. Li, Spectrochim. Acta, Part A, 2021, 247, 119046 CrossRef CAS PubMed.
- Y. Li, C. Li, B. Wang, W. Li and P. Che, J. Alloys Compd., 2019, 772, 770–774 CrossRef CAS.
- F. Li, J. Lu, Q. Zhang, D. Peng, Z. Yang, Q. Xu, C. Pan, A. Pan, T. Li and R. Wang, Sci. Bull., 2019, 64, 698–704 CrossRef CAS PubMed.
- S. A. Kurnosenko, O. I. Silyukov, A. S. Mazur and I. A. Zvereva, Ceram. Int., 2020, 46, 5058–5068 CrossRef CAS.
- G. G. Warr and R. Atkin, Curr. Opin. Colloid Interface Sci., 2020, 45, 83–96 CrossRef CAS.
- B. Shi, D. Shen, W. Li and G. Wang, Macromol. Rapid Commun., 2022, 43, 2200071 CrossRef CAS PubMed.
- Y. Zhong, J. Wang and Y. Tian, MRS Bull., 2019, 44, 183–188 CrossRef.
- Z. Zhou, J. Zhao, A. P. Chen, Y. Pei, Z. Xiao, G. Wang, J. Chen, G. Fu and X. Yan, Mater. Horiz., 2020, 7, 1106–1114 RSC.
- S. Pan, L. Liu, Q. Huang, J. He, H. Wang and S. Chang, Sci. China: Phys., Mech. Astron., 2023, 66, 276811 Search PubMed.
- A. A. Gismatulin, O. M. Orlov, V. A. Gritsenko and G. Y. Krasnikov, Chaos, Solitons Fractals, 2021, 142, 203502 CrossRef.
- F. Pan, S. Gao, C. Chen, C. Song and F. Zeng, Mater. Sci. Eng., R, 2014, 83, 1–59 CrossRef.
- J.-Q. Yang, R. Wang, Z.-P. Wang, Q.-Y. Ma, J.-Y. Mao, Y. Ren, X. Yang, Y. Zhou and S.-T. Han, Nano Energy, 2020, 74, 104828 CrossRef CAS.
- Y. P. Santos, E. Valenca, R. Machado and M. A. Macedo, Mater. Sci. Semicond. Process., 2018, 86, 43–48 CrossRef CAS.
- S. Zhu, B. Sun, G. Zhou, C. Ke, T. Guo, H. Zhao, F. Yang, Y. Zhang, Y. A. Wu and Y. Zhao, Mater. Today Chem., 2022, 26, 101169 CrossRef.
- Y. Zhang, S. Fan, Q. Niu, F. Han and Y. Zhang, Sci. China Mater., 2022, 65, 3096–3104 CrossRef CAS.
- Y. Wang, J. Yang, W. Ye, D. She, J. Chen, Z. Lv, V. A. L. Roy, H. Li, K. Zhou, Q. Yang, Y. Zhou and S.-T. Han, Adv. Electron. Mater., 2020, 6, 1900765 CrossRef CAS.
- L. Zhao, J. Xu, X. Shang, X. Li, Q. Li and S. Li, R. Soc. Open Sci., 2019, 6, 181098 CrossRef CAS PubMed.
- Y. Gong, Y. Wang, R. Li, J.-Q. Yang, Z. Lv, X. Xing, Q. Liao, J. Wang, J. Chen, Y. Zhou and S.-T. Han, J. Mater. Chem. C, 2020, 8, 2985–2992 RSC.
- Z. Zhou, H. Mao, X. Wang, T. Sun, Q. Chang, Y. Chen, F. Xiu, Z. Liu, J. Liu and W. Huang, Nanoscale, 2018, 10, 14824–14829 RSC.
- J. Wang, X.-F. Cheng, W.-H. Qian, Y.-Y. Zhao, J.-H. He, Q.-F. Xu, H. Li, D.-Y. Chen, N.-J. Li and J.-M. Lu, J. Mater. Chem. C, 2020, 8, 7658–7662 RSC.
- D. Wang, S. Zhao, L. Li, L. Wang, S. Cui, S. Wang, Z. Lou and G. Shen, Adv. Funct. Mater., 2022, 32, 2200241 CrossRef CAS.
- S. G. Kim, J. S. Han, H. Kim, S. Y. Kim and H. W. Jang, Adv. Mater. Technol., 2018, 3, 1800457 CrossRef.
- C. Zhang, H. Li, Y. Su, F. Yu, C. Li, Q. Zhang and J. Lu, Mater. Chem. Front., 2020, 4, 3280–3289 RSC.
- Y. Chen, H. Wei, Y. Wu, T. Yang and B. Cao, J. Mater. Chem. C, 2022, 10, 17386–17397 RSC.
- J. Wei, J. Li, C. Yu, Q. Sun, J. He and J. Lu, Chin. Chem. Lett., 2021, 32, 2463–2468 CrossRef CAS.
- T. R. Desai, S. S. Kundale, T. D. Dongale and C. Gurnani, ACS Appl. Bio Mater., 2023, 6, 1763–1773 CrossRef CAS PubMed.
- X. Li, L. Zhang, R. Guo, J. Chen and X. Yan, Adv. Mater. Technol., 2020, 5, 2000191 CrossRef CAS.
- M. Saha, S. M. Nawaz, B. K. Keshari and A. Mallik, ACS Appl. Bio Mater., 2022, 5, 833–840 CrossRef CAS PubMed.
- N. Sharma, K. Singh, C. C. Tripathi and M. K. Bera, J. Electron. Mater., 2023, 52, 3264–3280 CrossRef CAS.
- Q. Xue, Y. Peng, L. Cao, Y. Xia, J. Liang, C. C. Chen, M. Li and T. Hang, ACS Appl. Mater. Interfaces, 2022, 14, 25710–25721 CrossRef CAS PubMed.
- J. H. Cha, S. Y. Yang, J. Oh, S. Choi, S. Park, B. C. Jang, W. Ahn and S. Y. Choi, Nanoscale, 2020, 12, 14339–14368 RSC.
- C. Zhang, Y. Li, C. Ma and Q. Zhang, Small Sci., 2021, 2, 2100086 CrossRef.
- L. Cai, L. Yu, W. Yue, Y. Zhu, Z. Yang, Y. Li, Y. Tao and Y. Yang, Adv. Electron. Mater., 2023, 9, 2300021 CrossRef CAS.
- Z. Liu, J. Tang, B. Gao, P. Yao, X. Li, D. Liu, Y. Zhou, H. Qian, B. Hong and H. Wu, Nat. Commun., 2020, 11, 4234 CrossRef CAS PubMed.
- S. H. Sung, Y. Jeong, J. W. Oh, H.-J. Shin, J. H. Lee and K. J. Lee, Mater. Today, 2023, 62, 251–270 CrossRef CAS.
- W. Huh, D. Lee and C.-H. Lee, Adv. Mater., 2020, 32, 2002092 CrossRef CAS PubMed.
- J. Xu, Y. Huan, K. Yang, Y. Zhan, Z. Zou and L.-R. Zheng, IEEE Access, 2018, 6, 29320–29331 Search PubMed.
- R. C. Ivans, S. G. Dahl and K. D. Cantley, IEEE Trans. Neural Networks Learn. Syst., 2020, 31, 4206–4216 Search PubMed.
- J. T. Jang, D. Kim, W. S. Choi, S.-J. Choi, D. M. Kim, Y. Kim and D. H. Kim, ACS Appl. Electron. Mater., 2020, 2, 2837–2844 CrossRef CAS.
- R. Wang, Z. Mu, H. Sun and Y. Wang, Front. Phys., 2021, 690944 CrossRef.
- Y. Zhou, H. Wu, B. Gao, W. Wu, Y. Xi, P. Yao, S. Zhang, Q. Zhang and H. Qian, Adv. Funct. Mater., 2019, 29, 1900155 CrossRef.
- Y.-J. Zhang, X.-H. Chen, Z.-R. Wang, Q.-L. Chen, G. Liu, Y. Li, P.-J. Wang, R.-W. Li and X.-S. Miao, IEEE Trans. Electron Devices, 2019, 66, 4710–4715 CAS.
- H. Zhao, Z. Liu, J. Tang, B. Gao, Y. Zhang, H. Qian and H. Wu, Tsinghua Sci. Technol., 2022, 27, 455–471 Search PubMed.
- Y. Wang, L. Wu, G. Liu and L. Liu, J. Alloys Compd., 2018, 740, 273–277 CrossRef CAS.
- S. Wang, X. Dong, Y. Xiong, J. Sha, Y. Cao, Y. Wu, W. Li, Y. Yin and Y. Wang, Adv. Electron. Mater., 2021, 7, 2100014 CrossRef CAS.
- Y. Li, S. Ling, R. He, C. Zhang, Y. Dong, C. Ma, Y. Jiang, J. Gao, J. He and Q. Zhang, Nano Res., 2023, 16, 11278–11287 CrossRef CAS.
- C. Zhang, M. Chen, G. Wang, M. Teng, S. Ling, Y. Wang, Z. Su, K. Gao, X. Yang, C. Ma, Y. Li and Q. Zhang, Chin. J. Chem., 2022, 40, 2296–2304 CrossRef CAS.
- A. H. Jaafar, L. Shao, P. Dai, T. Zhang, Y. Han, R. Beanland, N. T. Kemp, P. N. Bartlett, A. L. Hector and R. Huang, Nanoscale, 2022, 14, 17170–17181 RSC.
- C. Zhang, M. Chen, Y. Pan, Y. Li, K. Wang, J. Yuan, Y. Sun and Q. Zhang, Adv. Sci., 2023, 10, 2207229 CrossRef CAS PubMed.
- Y. Li, C. Zhang, Z. Shi, C. Ma, J. Wang and Q. Zhang, Sci. China Mater., 2022, 65, 2110–2127 CrossRef.
- W. Zhang, H. Gao, C. Deng, T. Lv, S. Hu, H. Wu, S. Xue, Y. Tao, L. Deng and W. Xiong, Nanoscale, 2021, 13, 11497–11504 RSC.
- Z. Q. Li, Q. J. Zhang, C. Zhang, H. Li and J. M. Lu, Org. Electron., 2019, 66, 70–75 CrossRef CAS.
- S. Ling, C. Zhang, C. Ma, Y. Li and Q. Zhang, Adv. Funct. Mater., 2023, 33, 2208320 CrossRef CAS.
- T. Park, S. S. Kim, B. J. Lee, T. W. Park, H. J. Kim and C. S. Hwang, Nanoscale, 2023, 15, 6387–6395 RSC.
- Z. Feng, J. Yu, Y. Wei, Y. Wang, B. Tian, Y. Li, L. Cheng, Z. L. Wang and Q. Sun, Brain-X, 2023, 1, e24 CrossRef.
- B. Tian, Z. Xie, L. Chen, S. Hao, Y. Liu, G. Feng, X. Liu, H. Liu, J. Yang, Y. Zhang, W. Bai, T. Lin, H. Shen, X. Meng, N. Zhong, H. Peng, F. Yue, X. Tang, J. Wang, Q. Zhu, Y. Ivry, B. Dkhil, J. Chu and C. Duan, Exploration, 2023, 3, 20220126 CrossRef PubMed.
- Y. Wang, B. Han, M. Mayor and P. Samorì, Adv. Mater., 2023, 30, 2307359 CrossRef PubMed.
- Z. R. Wang, W. B. Zhu, J. H. Li, Y. D. Shao, X. H. Li, H. W. Shi, J. H. Zhao, Z. Y. Zhou, Y. C. Wang and X. B. Yan, ACS Appl. Mater. Interfaces, 2023, 15, 49390–49401 CrossRef CAS PubMed.
Footnote |
† These authors contributed equally to this work. |
|
This journal is © The Royal Society of Chemistry 2024 |