DOI:
10.1039/D1EA00102G
(Critical Review)
Environ. Sci.: Atmos., 2022,
2, 867-890
Terrestrial carbon cycle: tipping edge of climate change between the atmosphere and biosphere ecosystems†
Received
8th December 2021
, Accepted 17th July 2022
First published on 18th July 2022
Abstract
Owing to its tendency to couple with multiple elements, carbon forms complex molecules, which is the basic chemistry of life. Given that the climate system is inextricably coupled with the biosphere, understanding the terrestrial mechanistic pathway of carbon is critical in the transformation of the augmenting atmospheric carbon dioxide (CO2) in future. Although the global terrestrial carbon sink reduces the accumulation of atmospheric CO2, which is contingent on the climate and ecosystem, the underlying key biophysical function that controls the ecosystem-carbon-climate responses and their feedback is uncertain. Accordingly, numerous unprecedented multi-scale studies have highlighted the dynamics of terrestrial carbon by strategically employing in situ, earth observation and process-based models; however, to date, the driving force for its dynamics remains unclassified. Besides, the significant variability in carbon is related to the large uncertainties from changes in land use, unambiguously increasing the regional carbon source from the seasonal to interannual scale but without long-term positive or negative feedback. Accordingly, in this review, we attempt to present a holistic understanding of the terrestrial carbon cycle by addressing its nature and different key drivers. The heterogenetic data platforms that reliably address the terrestrial carbon sink and its source dynamics are discussed in detail to demonstrate the potential of systematic quantification. Moreover, we summarize the complexity of carbon-climate feedbacks and their associates, extending the pathway for understanding the recent terrestrial carbon allocation, where India's environment is highlighted. This comprehensive review can be valuable to the research community in understanding the importance of the present and future carbon-climate feedback.
Environmental significance
A very tiny layer (atmosphere) holds our planet's life structures in an aesthetically complex mix of abiotic and biotic factors, which interact in an ever-changing subtle unique inter-play. In this case, the carbon cycle is inevitable as it forms the functional interface between the terrestrial ecosystems and the atmosphere. It often plays a decisive role in shaping the climate from the regional to global scales, cascading it to the ecosystem function and changing the biosphere interactions with atmospheric CO2 concentration and climate. Given that the climate is dynamic, with a lack of observations at large spatial and temporal scales, quantifying its changes, evaluating and projecting its trend have been extremely challenging. Thus far, our knowledge on the environmental and climate impact on terrestrial ecosystems, and consequently, their control of the carbon cycle is limited. This has led to an unsolved question by the scientific community, whether terrestrial systems will remain as a carbon sink under climate stress or an increase in carbon may offset this negative feedback. As climate change continues to intensify, a crucial limitation is to unravel how the carbon cycle regulates terrestrial systems.
|
1 Introduction
Carbon, the global metabolic element that sustains life, is the key controller of various physical and chemical dynamics of processes on Earth.1–3 The phase shift of carbon through various systems in the terrestrial realm, which modify carbon in the bio-, geo-, atmo-, and pedo-sphere through various energy transfer states and dominated by the biosphere from minutes to the decadal-scale, is known as the terrestrial carbon cycle.4 Thus, it is indispensable to understand the carbon dynamics at the regional and global scales for better climate prediction. The dynamics in the transfer of carbon between the terrestrial system to the atmosphere holds the second-largest uncertainty in climate projection. Multiple aspects of anthropogenic, biophysical, chemical, ecological and hydrological processes create an imbalance in understanding the carbon dynamics at various scales.5,6 Therefore, these processes are responsible for the high degree of heterogeneous feedback to the terrestrial system, making constant carbon monitoring necessary. Additionally, human interventions in the modification of this system make the quantification of carbon even more difficult, which alters the basic key fluxes and stocks, cascading to form an imbalance in the carbon sources and sinks.3,7,8
Global terrestrial carbon pools accelerate/decelerate the accumulation of atmospheric CO2, thus triggering climate change. Compared to other natural carbon buffers, the terrestrial system is more sensitive given that the nature of carbon dynamics is pragmatically determined by various multi-nodal agents and also proportionally controlled by anthropogenic interventions.4,9,10 Studies have proven that there has been a significant increase in global photosynthesis since the pre-industrial epoch. Experimental studies based on theory have shown that CO2 is responsible for the enhancement in the terrestrial carbon sink. Supporting this, Hari et al.,11 Asner and Mascaro12 and Worden et al.13 reported that the traditional carbon sinks of the tropical forests have been tipped off their balance due to the alarming climate change, anthropogenic factors, fires, etc., acting as a periodic carbon source. The accelerated alterations in the climatic system by CO2 assimilation make in necessary for researchers to understand the flow of carbon to mitigate the risks of enviro-climatic (environmental and climatic) changes.14–16 Consequently, within the last three decades, many researchers have addressed various mediums of carbon dynamics and its feedback through direct and indirect analysis on a regional to global scale. With the emerging research on the carbon cycle, studies have focused on understanding the feedback not only for stakeholders and the research community but also for communicating its effects to the public for mitigation.17–19
With the record of more than fifty years of atmospheric datasets, thirty years of satellite datasets and twenty years of continuous in situ datasets, the science community has presented many fundamental insights into climate change and its interface with the terrestrial system in terms of the carbon dynamics.2,15 However, due to its heterogeneous property in various mediums, a standard method for quantifying the carbon content or its direct feedbacks (intensification of CO2 concentration) and indirect feedbacks (response towards cascading climate change) is still lacking,6,10,16,20 and given that the carbon cycle interactions are multi-nodal, they may amplify or modify the atmospheric carbon dramatically.
This review describes the fundamentals of the carbon cycle at the terrestrial level and its paradigms by discussing their feedback and associates. Principally, this study focuses on the state of knowledge rather than the quantitative analytics. This article is divided into six sections, excluding the introduction and summary. The section “Background: terrestrial carbon source and sink” presents a brief outline of the terrestrial carbon sinks and sources and their outliers in quantification. Subjectively, in this section we discuss the ‘textbook perspective’ of the terrestrial carbon system. The section “Key drivers of the terrestrial carbon cycle” summarizes the various key fluxes and stocks that drive the terrestrial carbon system at the regional and global scales. This section summarizes the general terminologies of various carbon key drivers, supported by the global trend and magnitude. Subsequently, in the section “Methods for quantifying carbon fluxes and stocks”, the multi-variate methods for estimating terrestrial carbon are discussed. This section is classified briefly as model, earth observation, in situ-based methods, etc., where their ability to quantify terrestrial carbon at various spatial scales is described. This section also outlines their mode of uncertainty in projecting the trend. Next, the “Associates of terrestrial carbon” section deals with various interactive mediums of carbon in the terrestrial regime, which exhibit multi-nodal feedback to the system. In the section “Carbon-climate feedback”, we briefly discuss the shift and cascading nature of bi-nodal feedback between the terrestrial ecosystem and the climate, highlighting the qualitative assessment of the observational trend at the regional and global scales. Finally, in the “Terrestrial carbon cycle magnitude and trend” section, we outline the global carbon trend and focus on the carbon flux and stock trends in India. Herein, we generally pay less attention to other associates of carbon such as methane, aerosols and extreme events. Additionally, we do not focus on a particular terrestrial ecosystem such as grassland, peatland, tropical, agroecosystems and dryland to maintain the scope and make the text of this article more practical.
2 Background: terrestrial carbon source and sink
The spatiotemporal magnitude and the residence time of carbon exchange between the terrestrial system and other spheres through photosynthesis and autotrophic/heterotrophic respiration is known as the terrestrial carbon cycle.21,22Fig. 1 presents a schematic diagram of the terrestrial carbon cycle, focusing on the important fluxes of the terrestrial ecosystem. In the refined IPCC23 report, it was estimated that the terrestrial carbon sink has drastically changed in the last few decades, which has aroused concern regarding the future of the terrestrial carbon sink.2,24 Thus, a balanced study to understand the carbon source and the sink must be performed to gain insight for future carbon budgeting and managing.
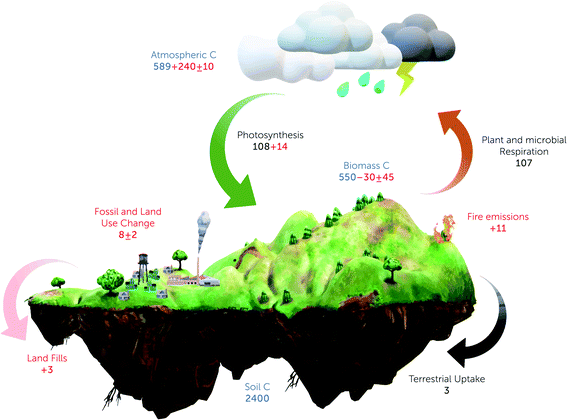 |
| Fig. 1 Storage and exchange of carbon in various spheres of the terrestrial carbon cycle. The blue text indicates the reservoir mass, black text indicates the natural carbon flux among the spheres, and red text indicates the change in the particular sphere. “+” indicates the cumulative gain of carbon, “−” indicates the cumulative loss of carbon and “±” indicates the net annual change. Carbon fluxes are represented in Pg C per year and carbon stocks are in Pg C. Values are adapted and approximated from Ciais et al.204 | |
2.1 Terrestrial carbon sources
From an ecological viewpoint, various studies have highlighted that the primary source of the carbon cycle from the terrestrial ecosystem is land-use conversion (LUC) from agricultural practices (slash-burn practices)25,26 and deforestation (chiefly the primary forests).27 According to global carbon budget studies, such as that by Sitch et al.28 and Fu et al.,29 it is roughly estimated that about 2.0 Pg C per year was shifted from the terrestrial system to the atmosphere in the late 90's. Thus, an imbalance in carbon in the restoration was retained by the carbon sink, maintaining the holding capacity of 0.2 Pg C per year, traumatizing the sink to the residual of 2.2 Pg C per year and forming an imbalance in the terrestrial ecosystem.1,30 These constraints break down the traditional view of the decadal trend of the terrestrial carbon sink and sources. With the advent of drastic climate change, researchers are eagerly analyzing the nature of the carbon fluxes between the biosphere and atmosphere, whereas an uncertainty estimation of the terrestrial sink and source is still lacking.10,22,31,32
2.2 Terrestrial carbon sinks
Terrestrial carbon sinks are assessed by various key environmental and atmospheric factors,1,33 which deliver positive feedback on carbon fluxes, including the factors that intensify the carbon residual time in the biota, factors that iterate the change in carbon loss, and both anthropogenic and natural factors.34–36 Researchers have employed sophisticated measurement techniques and achieved a consistent understanding of the fluctuations in the carbon sink; however, the importance of the factors in its assessment is still under debate.33,37,38 This variability in understanding the sinks requires them to be explicitly studied by considering the factors of biospheric recovery from the LUC together with the climate change with the recent increase in the atmospheric carbon concentration.39,40 This empirical estimation presents the missing gaps in the sink by representing the existing knowledge in the biospheric response to various factors.38,41,42 Friedlingstein et al.,37 Luo43 and Liu et al.36 elucidated that in the late 90's, about 0.3 to 1.5 Pg C per year was restored in the sink, suggesting that the biospheric recovery from the LUC and increased atmospheric CO2 concentration catalyzed the nature of the sink. These conclusions were drawn by the researchers using various parametric ensembles of ecological and atmosphere models, which are predominately based on in situ data, which is limited in the case of ecology and climatology. The alarming increase in CO2 concentration in the carbon cycle has resulted from natural and anthropogenic factors, which portray the terrestrial realm as a sink at the global scale,17,44–46 whereas at the local spatial extent, it exhibits the opposite trend by being a source.11 This variability in spatial scale is conditioned by the local climatic factors and LUC. Specifically, the terrestrial regime of the carbon cycle has the potential of being both a sink and source to the atmosphere, with the question of the spatial location remaining.2,46
To date, a complete frame of the “systematic terrestrial carbon cycle” that points out the uncertainty inducer is still lacking.22,47 The challenge in understanding the nature of the carbon cycle is predominant because of its future response to the current geography. Thus, continuous studies at the regional and global scales are necessary to elucidate the missing pieces, understand the nature and process of the sources and the sinks, quantify real-time carbon in the atmosphere and project the future CO2 level with a high degree of accuracy.
3 Keys drivers of the terrestrial carbon cycle
The carbon stocks and fluxes are considered to be the key aspects of the terrestrial carbon cycle. They frame a quantification tool for better understanding the terrestrial carbon cycle. Ecosystem respiration (ER), gross primary production (GPP), net biome productivity (NBP), net ecosystem exchange (NEE), net ecosystem production (NEP) and net primary production (NPP) form the prime fluxes, and above ground carbon (AGC), below ground carbon (BGC) and soil organic carbon (SOC) are the important stocks of the terrestrial carbon cycle. These terms are defined in Table 1. Upon encountering a small disturbance, these drivers significantly influence the level of atmospheric CO2.
Table 1 Definitions and descriptions of the key driving components of the terrestrial carbon cyclea
Terrestrial C |
Key driver |
Acronym |
Definition |
Expression |
Reference |
(*) Allometric equations for individual species; CF: conversion factor; n: number of plots; D: depth of soil horizon; BD: bulk density; and Ccon: carbon concentration.
|
C flux |
Ecosystem respiration |
ER |
Efflux of carbon from autotrophic and heterotrophic respiration |
ER = Ra + Rh |
53
|
Gross primary production |
GPP |
Total carbon fixed by the autotrophs during photosynthesis |
GPP = Total C |
69
|
Net primary production |
NPP |
Potential biomass/C available in the ecosystem after autotrophic metabolism |
NPP = GPP − Ra |
68
|
Net biome production |
NBP |
NEE with non-respiratory carbon loss and heterotrophic carbon gain (or) GPP with total ER and anthropogenic carbon flux |
NBP = NEE − Cefflux − Cinflux (or) NBP =GPP − ER − Alulc |
36
|
Net ecosystem exchange |
NEE |
Net exchange of carbon between ecosystem and atmosphere |
NEE = NPP − Rh |
58
|
Net ecosystem production |
NEP |
Net carbon flux to or from an ecosystem |
NEP = GPP − ER (or) NEP = GPP − ER − Alulc |
74
|
C stocks |
Above ground carbon |
AGC |
Carbon stored in living plant tissues above surface of earth |
|
98
|
Below ground carbon |
BGC |
Carbon stored in living plant tissues below surface of earth |
|
98
|
Soil organic carbon |
SOC |
Carbon in terms of soil organic matter compounds in dry weight |
SOC = CF × D × BD × Ccon |
55
|
3.1 Carbon fluxes
The carbon flux is the flow of carbon from the biosphere to the atmosphere and vice versa, which is dependent on the nature of the sink and source by various biophysical associate key drivers.28,34 It mediates the carbon flow between the ecosystem and is measured in mass per unit area and time.48,49 The carbon pool in an ecosystem holds multiple key fluxes of varying sizes and these key drivers affect the carbon by various factors depending upon the variability of the ecosystem. From an ecosystem viewpoint, based on the theory of resource allocation and optimization,50 biotic systems regulate their primary production within weeks to months by numerous ecological processes based on their environmental stability.36,51,52 This variability influences the fluxes and is quantified in parts to analyze the total flux between the terrestrial ecosystem and the atmosphere. The chief drivers that influence the carbon flux variability are CO2 fertilization,39,53 nitrogen (N) availability and nutrient variation,54,55 LUC trend,6 climate change,20,42 and forest fires.56
3.1.1 Ecosystem respiration (ER).
Ecosystem respiration (ER), which is the total efflux of carbon from autotrophic (plants) and heterotrophic (micro-organism) respiration, plays a pivotal and complex role in the carbon cycle given that it exhibits a multi-dimensional response to the ecological drivers in an ecosystem.57,58 After GPP, ER is the largest carbon flux. Zeng et al.,34 Reichstein et al.53 and Vetter et al.59 highlighted that ER influences the climatic structure with a positive response. According to its multifactor components of Ra (autotrophic), Raa (aboveground autotrophic), Rab (belowground autotrophic) and Rh (heterotrophic), ER exhibits heterogenous feedbacks to the same atmospheric and environmental variables such as temperature, precipitation, and soil moisture.60,61 A higher degree of sensitivity analysis is needed to understand the relation between the ER and the factors that control the carbon cycle. The quantification of ER and its factors are mainly processed by conventional direct methods such as chamber measurements (minimal spatial extent),62 eddy-flux (integration of micrometeorological factors),63 contemporary indirect remote sensing (RS) methods (larger spatial scale)15 and mathematical modelling.64,65
3.1.2 Gross primary production (GPP) and net primary production (NPP).
Gross primary production (GPP) is the most important key flux in the terrestrial carbon cycle. GPP is the total carbon fixed by the autotrophs in a unit scale of CO2, reduced during photosynthesis, and provides organic compounds for ER.66 Alternatively, net primary production (NPP) is the substantial difference between GPP and ER per unit scale, forming the biomass of residual carbon stored in the structural part of autotrophs.67 Waring et al.,68 Veroustraete et al.48 and Giardina et al.69 highlighted that the average GPP of autotrophs is estimated to be 5.83 × 106 cal m−2 per year and their NPP is about 4.95 × 106 cal m−2 per year. This, on an ecological standpoint, is the stored solar energy made available to the top order of the food chain. In contrast, from a biogeochemical viewpoint, GPP and NPP form the key drivers between the atmosphere and biosphere, integrating the global hydrological, nitrogen, energy and carbon cycles.54,63
3.1.3 Net biome production (NBP).
Net biome production (NBP) is the pertinent key flux that analyses the carbon transfer between the biosphere and atmosphere, including turbulences by the influence of anthropogenic LUC.35,36,64 NBP in an ecosystem is also defined as the residual carbon from the difference in ER and GPP from the prevailing carbon.35,40 Due to constant micro-disturbances, NBP is challenging to calculate at the biotic level and is estimated mostly over a larger biome. These micro-disturbances only affect the NBP flux and not the others due to their various timescales and ecological processes. In general, the NBP is the descending flux and is estimated after other upstream fluxes such as GPP, ER, and NEP. Studies by Cervarich et al.40 and Gahlot et al.35 indicated that NBP showed an increasing trend from 0.2 Gt C per year to 1.4 Gt C per year in the late 90's, which included dramatic LUC, suggesting a higher degree of carbon flux change globally,70,71 which significantly disturbed the terrestrial equilibrium.
3.1.4 Net ecosystem exchange (NEE) and net ecosystem production (NEP).
Net ecosystem exchange (NEE), which is the difference between NPP and Rh, also includes the NEP to the fluxes of carbon sources and sinks in an ecosystem.19,58 Alternatively, net ecosystem production (NEP) is the total difference between carbon fixed during photosynthesis (GPP) to that of ER, i.e., the accumulation of carbon in an ecosystem.56,72,73 NEP is also defined as the total flux of GPP and ER with CO2 fertilization by the anthropogenic LUC.39,53 NEP consists of different concepts, where one is similar to NPP, while different from Rh. Similar to NPP, NEP basically controls the carbon balance in the terrestrial system. Unlike the other key fluxes, the estimation of NEP is challenging due to its vagueness.72,74,75 Researchers have widely relied on the eddy covariance method to estimate NEP by the vertical exchange of carbon between the biosphere system and the atmosphere.65,76,77 NEE and NEP significantly refer to the same ecological process, without any formal definitions, where NEE is often measured at a minimal timestep ranging from hours to days,78 while NEP is typically quantified from months to years.79 These fluxes are the key drivers of the terrestrial carbon cycle, which are estimated by integrated models and RS (indirect estimation) and are augmented with eddy covariance and field inventories (a reliable direct estimation). Zeng et al.,34 Rödenbeck et al.80 and Worden et al.13 highlighted that the carbon flux anomalies increased from 0.03 Pg C per year in the late 20th century to 1.4 Pg C per year early in the 21st century, which was attributed to the increase in LUC and other anthropogenic factors. This also included a high degree of interannual and annual uncertainty due to the cascading regular and occasional anthropogenic, climatic and biophysical factors.10,81
3.2 Carbon stocks
Carbon stocks are the storage units of carbon (in terms of biomass/phytomass) across various pools of the ecosystem.82,83 They are highly proportional to the sequestration of atmospheric CO2 through various flux components and quantified and measured in terms of mass.48,84 Carbon stocks mostly act as sinks, which are the regulator of regional climate change, whereas the LUC in the past decades disrupted the trend of carbon sock availability and stressed the ecosystem to fall under insurgent enviro-climate change.85,86
3.2.1 Above ground carbon (AGC).
Above ground carbon/biomass (AGC/AGB), the paramount and visible dominant carbon pool of an ecosystem, is the dry weight of carbon stored in the live woody plant tissues (which include twigs, stem, and branches) above the Earth's surface (excluding roots and tree stumps).87,88 AGC accounts for a major proportion of the stored terrestrial carbon and is potentially a large contributor of carbon flux to the atmosphere by anthropogenic and natural disturbances.84,89 Thus, it demands higher importance in managing and quantifying the terrestrial carbon cycle at a regional scale, which requires effective constant spatial and temporal monitoring. In terms of estimation, the inventory-based destructive method90 and allometric models91 are the most reliable sources among the RS and biophysical models. However, they are resource constrained and imprecise at the ecosystem level.90,92 At the plot level, they are estimated by the important physical parameters of a biota, i.e., height, canopy density, DBH, age, and species composition,93–95 where the estimations are broadly based on the first four physical parameters.
3.2.2 Below ground carbon (BGC).
Below ground carbon/biomass (BGC/BGB) is one of the carbon pools where carbon is stored in the living roots below ground.87,96,97 BGC acts as a potential sink and is mostly an unobstructed pool compared to AGC.92,98 BGC is dynamically linked to AGC. With limited studies on BGC, understanding the distribution of carbon between the terrestrial system and the soil remains difficult due to various complications in understanding nitrogen coupling, LUC, litter influence, Rh flux inclusion, etc.99,100 The little-known concept of BGC possesses high uncertainty by biotic composition, stand age and density, but has a significant proportion in the terrestrial carbon stock quantification. The uncertainty in the estimation of BGC is mainly due to the environmental variabilities, nutrient availability, influence of hydrological and nitrogen cycle.101 BGC is mainly considered to be a driving factor in the formation of SOC.3,27,102 Compared to AGC, the estimation of BGC has been neglected mostly because of the difficulties in various measurement practices and its assessment at the stem base from proximal roots.
3.2.3 Soil organic carbon (SOC) and dead biomass component (DBC).
Soil organic carbon (SOC) is potentially the largest carbon pool in the terrestrial regime, forming about 54% of the terrestrial carbon stocks,103–105 which is the balance between the dead and decaying organic biota and the carbon loss due to the mineralization by Rh (heterotrophic respiration; decomposition of organic matter).64,106 The return of the inward carbon to the atmosphere and biota through ER to the soil through the organic biota is known as the soil carbon flux. Unlike other fluxes, the SOC fluxes are destabilized by enviro-physical factors such as pH, redox potential (Eh), and nitrogen content, complicating the quantification of soil carbon stocks. The SOC potentially includes the dead biomass component (DBC) or dead organic matter (DOM), which are the deadwood and litter of the biota, respectively.23,103 The SOC fluxes are remarkably accurate with the inclusion of DOM, which is dynamic in nature, depending on the nature of the ecosystem. DOM is virtually absent in other land cover classes, excluding the forest and other biotic ecosystems.
4 Methods for quantifying carbon fluxes and stocks
The carbon in an ecosystem is measured either directly/indirectly or a combination of both. Specifically, the destructive (direct) estimations are the most accurate but are limited to a small spatial extent107,108 compared to the non-destructive (indirect) estimations.90,109 However, both methods possess distinctive advantages and disadvantages. Some of the traditional and contemporary measures of carbon flux and stock include eddy covariance,76,79,110 chamber method,62,74 community descriptions,22 inventory-based harvest method,87 allometric equation models,111 RS measurements,109 and integrated models.77,112 Broadly these methods can be discussed as follows.
4.1 Destructive methods
Assessing carbon through the direct (destructive) method is a more accurate method compared to the indirect method,107,108 which involves quantification by harvesting the biota (either as a whole or by strata). This method is spatially, temporally and economically constrained due to its catastrophic nature, which also leads to regional environmental deterioration. However, this is the only robust method that validates other methods of estimation to critically analyze the factors that influence the terrestrial ecosystem. The inventory-based harvest method is one of the direct methods for the estimation of primary production (NPP) and aboveground/belowground carbon stored in the terrestrial system as biomass.87 The direct methods are used as calibrators to scale up the non-destructive estimation methods from the regional to global scale. However, most of these inventories are materialized in a forest-based ecosystem, which limits the heterogeneity of distribution in a non-forest environment.41 Destructive methods often result in errors such as biased sampling errors based on discrepancies in the inventory seasoning, plot location, measurement errors, imperfect local estimation models, etc.
4.2 Non-destructive methods
Long-term analysis of an enviro-climate response demands the estimation of terrestrial ecosystem carbon, for which a method that is non-destructive to the biota in an ecosystem should be employed. Although these methods of estimation lead to multiple inevitable uncertainties,92,111 they are broadly stimulated due to their proximity to higher temporal and spatial steps. The various important non-destructive estimations employed in the estimation of terrestrial carbon are discussed in the following sections.
4.2.1 Eddy covariance.
The eddy covariance method is one of the few reliable sources of surface-atmosphere flux estimation63,65,76 that quantifies the various biogeochemical process vertical turbulent fluxes (C, N, methane (CH4), energy (solar radiation and photosynthetically active radiation (PAR)), water (H2O), etc.). The flux measurements are mostly made in the unstable stratification in the surface boundary layer of the atmosphere, at about 30–50 m.113,114 However, eddy flux measurements at ∼10 m are conventionally employed due their high-quality measurements and maximized flux footprint, given that they share high consistency with subsurface fluxes and roughness properties.76,115
The bedrock of the eddy covariance principle is the measurement of the horizontal and vertical components of horizontal flowing eddies through a unit area per unit time.115 These desirable fluctuating variables are quantified to analyze the key parameters of the terrestrial carbon flux. Eddy covariance measurement explicitly provides site-specific fluxes at higher temporal resolution.19,116 These location-specific estimations can be upscaled to the regional and global scales with a series of multiple sites and studies, resulting in good agreement. A comprehensive list of the eddy covariance sites and their networks across the globe are listed in Table 2 and spatially represented in Fig. S1.† These flux networks strengthen RS measurements and process-based models to tackle the questions based on the ecosystem-climate feedbacks and uncertainties.53,62,63 However, the accuracy of this upscaling process is restrained by the heterogeneity of the site distributions. Eddy fluxes mostly show variations above the canopy level, which miss the subsurface and surface fluxes. Furthermore, the eddy covariance method indicates uncertainty in the measurement due to mechanical (equipment maintenance and calibration), biophysical (suboptimal wind turbulence) and climatic factors, which exerts a data gap that affects the magnitude of the terrestrial carbon flux3 However, these issues can be overcome using various gap-filling methods.
Table 2 Summary of the global distribution of flux measurement networks and their availability. The reader should refer to Fig. S1 in the ESI for the spatial representation of the flux network sites and their data record
Spatial domain |
Network |
Established year |
Towersa |
Statusb |
Data accessc |
Link |
Sites involved with multi projects.
Based on the maximum availability of data till 2019.
Based on the maximum number of site accessibility.
Consortium of US and China flux networks.
Network also includes BrasFLUX.
Non-detailed networks.
|
Africa |
CarboAfrica |
1999 |
026 sites |
Inactive |
Private |
https://www.carboafrica.eu/
|
Safari2000 |
1999 |
005 sites |
Inactive |
Public |
https://daac.ornl.gov/
|
Asia |
AsiaFlux |
1995 |
111 sites |
Active |
Public |
https://asiaflux.net/
|
ChinaFlux |
2002 |
068 sites |
Inactive |
Public |
https://www.chinaflux.org/
|
FFPRI FluxNet |
1999 |
006 sites |
Inactive |
Public |
https://www.ffpri.affrc.go.jp/
|
JapanFlux |
1996 |
039 sites |
Inactive |
Public |
https://www.japanflux.org/
|
India C project |
2012 |
016 sites |
Inactive |
Private |
https://www.nrsc.gov.in/
|
KoFlux |
2002 |
010 sites |
Inactive |
Public |
https://www.ncam.kr/
|
RusFluxNet |
1998 |
010 sites |
Inactive |
Public |
https://fluxnet.org/
|
TCOS-Siber |
1998 |
007 sites |
Inactive |
Private |
https://www.bgc-jena.mpg.de/
|
ThaiFlux |
2001 |
012 sites |
Inactive |
Private |
https://asiaflux.net/
|
Australia |
OzFlux |
2000 |
043 sites |
Active |
Public |
https://www.ozflux.org.au/
|
Europe |
CarboEurope |
1996 |
166 sites |
Active |
Public |
https://www.carboeurope.org/
|
CarboItaly |
1996 |
028 sites |
Active |
Public |
https://www.europe-fluxdata.eu/
|
CarboMont |
2002 |
013 sites |
Inactive |
Public |
https://www.uibk.ac.at/
|
EuroFlux |
1996 |
016 sites |
Active |
Public |
https://www.europe-fluxdata.eu/
|
ICOS |
1996 |
070 sites |
Active |
Public |
https://www.icos-cp.eu/
|
IMECC |
1996 |
014 sites |
Active |
Public |
https://www.europe-fluxdata.eu/
|
MEDEFLU |
1996 |
004 sites |
Inactive |
Public |
https://www.europe-fluxdata.eu/
|
Swiss FluxNet |
1997 |
008 sites |
Active |
Public |
https://www.swissfluxnet.ethz.ch/
|
TERENO |
2011 |
004 sites |
Active |
Public |
https://www.tereno.net/
|
UrbanFluxes |
2011 |
021 sites |
Active |
Public |
https://urbanfluxes.eu/
|
N. America |
Ameriflux |
1991 |
526 sites |
Active |
Public |
https://ameriflux.lbl.gov/
|
BERMS |
1994 |
004 sites |
Inactive |
Public |
https://daac.ornl.gov/
|
FluxnetCanada |
1993 |
032 sites |
Inactive |
Public |
https://daac.ornl.gov/
|
MexFlux |
2004 |
012 sites |
Active |
Public |
https://fluxnet.org/
|
USCCCd |
2004 |
052 sites |
Active |
Public |
https://lees.geo.msu.edu/
|
S. America |
EUCFLUX |
2010 |
010 sites |
Inactive |
Public |
https://www.ipef.br/
|
LBA ECOe |
1999 |
009 sites |
Inactive |
Public |
https://daac.ornl.gov/
|
SulFlux |
2009 |
009 sites |
Inactive |
Private |
https://www.sulflux.ufsm.br/
|
TropiFlux |
2001 |
002 sites |
Inactive |
Private |
https://daac.ornl.gov/
|
Miscf |
TaiwanFlux |
|
|
|
|
|
Tropi-dry |
|
|
|
|
|
4.2.2 Allometric models.
Allometric models are traditional statistical estimation models for quantifying the carbon stock and an alternative development to the destructive methods of estimation using the biometric variables (such as age, height, diameter at breast height (DBH), and canopy cover) of the biota.91,92,95 They possess diverged frames as the biomass equation (C quantification in the multi-component of a biota)102 and the volume equation (C quantification limited to the woody stem),90,117 which are conditional to the biotic variables. The allometric models are robust at the regional scale given that they are considerably plot-based. They are generic to the global scale for species-based models with similar traits (such as plantations). Statistically significant multispecies allometric models have been developed for estimating multi-variate biotic species in an ecosystem.111 Conversely, geographical-specific species allometric models provide higher accuracy in the estimation of carbon. Most of the studies based on allometric models highlight the importance of their integration with the RS variable predictors (such as vegetation index) for structural heterogenicity of the biome.83,118 Potentially, allometric models are species variants and carry anomalies by various ecological and climatic factors, which increase the level of uncertainty. Also, validation of this model estimation reverts to the destructive sampling type (at least ≤10 of sample biota) by cutting and weighing the biometric components.78
4.2.3 Integrated models.
The integrated numerical models indirectly estimate the carbon sources and sinks to quantify the carbon magnitude at higher spatial and temporal scales. The two major modelling approaches include the top-down modelling (understanding the nature of terrestrial carbon sink and sources by atmospheric CO2 inversion)40,119,120 and the bottom-up modelling (quantifying the magnitude of terrestrial carbon fluxes and stock by eco-physiological processes).58,103 The various model configurations are grouped and listed in Table 3 and discussed in detail in the following sub-sections.
Table 3 Comprehensive list of integrated models extensively used in terrestrial carbon quantification
Model integration |
Name |
Acronym |
Reference |
Consortium of multiple earth system models.
|
Bottom-up |
BioGeochemical Cycles model |
BIOME – BGC |
99
|
Carnegie-Ames-Stanford Approach model |
CASA |
106
|
CENTURY soil organic matter model |
CENTURY |
67
|
Community Land Model 4 with Carbon–Nitrogen |
CLM4CN |
189
|
Coupled Model Intercomparison Project |
CMIP |
190
|
Dynamic Land Ecosystem Model |
DLEM |
191
|
Ecosystem Demography model |
ED |
131
|
High Resolution Biosphere Model |
HRBM |
130
|
Hybrid LAND model |
HYLAND |
163
|
Integrated Biosphere Simulator |
IBIS |
112
|
Integrated Terrestrial Ecosystem Carbon model |
InTEC |
62
|
Jena Scheme for Biosphere-Atmosphere Coupling in Hamburg |
JSBACH |
37
|
Joint UK Land Environment Simulator |
JULES |
192
|
Land surface Processes and Exchanges |
LPX |
17
|
LINKAGES forest ecosystem biogeochemistry model |
LINKAGES |
193
|
LPJ – Dynamic Global Vegetation Model |
LPJ – DGVM |
28
|
LPJ – General EcoSystem Simulator |
LPJ – GUESS |
128
|
Lund-Potsdam-Jena model |
LPJ |
105
|
Mapped Atmosphere-Plant-Soil System model |
MAPSS |
194
|
MCFIRE 1 |
MC1 |
195
|
ORCHIDEE – coupled terrestrial Carbon and Nitrogen |
O – CN |
152
|
Organizing Carbon and Hydrology In Dynamic EcosystEms model |
ORCHIDEE |
156
|
Photosynthetic/EvapoTranspiration model |
PnET |
100
|
QUantifying the effects of Interacting Nutrient Cycles on Terrestrial Biosphere |
QUINCY |
101
|
Sheffield Dynamic Global Vegetation Model |
SDGVM |
60
|
Spatially Explicit Individual-Based Dynamic Global Vegetation Model |
SEIB – DGVM |
124
|
Surface Externalisée |
SURFEX |
196
|
Terrestrial Ecosystem Model |
TEM |
129
|
Terrestrial Observation and Prediction System model |
TOPS |
134
|
TRENDs in net land atmosphere carbon exchange |
TRENDYa |
197
|
VEgetation COntinuous DEscription |
VECODE |
198
|
Vegetation-Global-Atmosphere-Soil model |
VEGAS |
34
|
Top-down |
Atmospheric tracer TRANSport model interCOMparison |
TransCom |
199
|
Carbon TrackEr model |
CTE |
200
|
Community Atmosphere Biosphere Land Exchange model |
CABLE |
127
|
Conformal Cubic Atmospheric Model |
CCAM |
31
|
Integrated Science model for Assessment of climate change |
ISAM |
201
|
Japan Meteorological Agency model |
JMA |
32
|
JENA CarboScope-Max Planck Institute model |
JENA – MPI |
80
|
Laboratoire des Sciences du Climat et de l'Environnement model |
LSCE |
202
|
Mesoscale Atmospheric Transport and CHemistry model |
MATCH |
31
|
Monitoring Atmospheric Composition and Climate-Interim Implementation model |
MACC – II |
123
|
Non-hydrostatic ICosahedral Atmospheric Model-Transport Model |
NICAM – TM |
180
|
Research Institute for Global Change model |
RIGC |
203
|
Top-down Representation of Interactive Foliage and Flora Including Dynamics model |
TRIFFID |
150
|
4.2.3.1 Top-down modelling.
The methods for the quantification of carbon in an ecosystem have been multi-modal in recent years, but their validation can be analyzed at a point scale with a reliable source, causing the nature of the quantifying system to be complex. This demands a bilateral estimation of carbon. One example is the atmospheric inversion model or the top-down approach.57 This method estimates the carbon flux between the atmosphere and the biosphere based on the concentrations of atmospheric CO2 to understand the regional and global nature of the sinks and sources.119,121 An inverse atmospheric transport model is used to project the magnitude of uncertainties in atmospheric CO2, reflecting the terrestrial carbon flux to estimate the terrestrial carbon sink and source.122 The uncertainty gradient is highly suitable to constrain the estimation with nominal error. This also demands an improvement in calibrated observational measurements to enhance the quantification of the flux. However, the spatial coverage of the observation sites is uneven (e.g., the spatial distribution of the flux sites in Table 2 and Fig. S1†), which exhibits a higher uncertainty in the results.34,121 Given that the inverse atmospheric tracer transport models involve natural and anthropogenic fluxes, the estimated terrestrial carbon flux is affected. Thus, multiple data constraints such as hydrological and energy fluxes from other models are needed to reduce its ambiguity.5,121,122 These improvements in the spatial and temporal scale can potentially improve the agreement of the estimated terrestrial carbon flux with the observed CO2 concentrations.
Recent research has indicated that various inversion methods for estimating the flux variations of CO2 result in a substantial improvement in the simulation, but inconsistent due to uncertainties.109,113,120,123 Due to the multi-step temporal and spatial constraints, the variations in the estimations are not dependent on the model aggregation.8,33,71 This is predicted to cause errors in projecting the nature of terrestrial carbon (i.e., analysis with homogenous spatial and temporal observational data constraints with multiple scales of seasonal data with seasonal inverse atmospheric tracer transport model estimation, annual data with annual inverse atmospheric tracer transport model estimation and seasonal data with annual inverse atmospheric tracer transport model estimation can result in a variation in the nature of the carbon balance of the ecosystem to sink or source).36,70,113 Supporting this, Kawamiya et al.,124 Gregory et al.,9 Stocker et al.,17 Thum et al.101 and Walker et al.71 indicated that this variability in the models resulted in the observation of a carbon sink-source trend. Corresponding to these inconsistencies, inverse top-down modelling results in worse spatial resolution.
4.2.3.2 Bottom-up modelling.
Modelling for quantifying the terrestrial carbon stock and flux based on the integration of various ecological, biological, climatological, chemical, and physical processes in response to environmental variations6,19,77,125,126 involves terrestrial and bottom-up models. They vary from ‘simple response variable’ statistical models to complex ‘multi-constrain enviro-climatic’ driver models in relation to carbon flux. The bottom-up model is considered to be a process-based model, which is ensembled under dynamic global vegetation models (DGVM)28,40 and terrestrial biosphere models (TBM).19,112,113,127 These models have inherent ability to highlight the system dynamics and functionalities of terrestrial carbon sinks and sources. Various models have been developed and analyzed in manifold studies to quantify and predict the magnitude of the terrestrial carbon flux and atmospheric CO2 concentrations.
Specifically, the DGVM has the ability to understand the past, estimate the present and project the future dynamics of terrestrial carbon stocks and fluxes and other biogeochemical cycles through enviro-climatic interactions.51,120,128 DGVM captures these dynamics under the present climate change scenario and acts as an earth system model by capturing the terrestrial energy budget.9,47 In this alarming case of climate change and atmospheric CO2, DGVM accounts for the dynamics of the biotic stratum using the plant functioning types (PFTs)101,112,129 to understand its reversible nature spatially and temporally towards an infinite set of natural and anthropogenic behaviours. This ability to investigate the terrestrial ecosystem is mainly driven by the historical climatology, LUC, atmospheric CO2 concentration, nitrogen deposition and key drivers of terrestrial fluxes such as GPP and NPP, which relatively exhibit a collinear response towards the terrestrial carbon.6,51,75
Alternatively, the TBM mainly focuses on the key drivers of the terrestrial carbon fluxes, i.e., GPP, NPP and NEE, which explicitly estimate the interaction of carbon flux, nitrogen flux, hydrological flux and energy flux in the ecosystem.15,57,130 TBM delivers significant information on the nature of the terrestrial regime in the carbon cycle. TBMs are evolved process-based models, which explore the correlation among multiple ecosystem constraints based on natural and anthropogenic alterations at the regional and global scales.57,113,131 They are dramatically self-administered in estimating the various key drivers of carbon flux based on geographical and temporal precision.
Bottom-up modelling possesses limitations based on the key parameters and drivers, which cause the accuracy the fluctuate by increasing the uncertainty to a broader extent of temporal scale, which depends on multi-timescale data traits for better projection of terrestrial carbon.2,15,57,66,132 Further, the most complicated processes that limit the understanding of terrestrial carbon flux are generally simplified to achieve a better estimation, triggering multiple uncertainties in the magnitude of carbon and variation in the sinks/sources.5,122,125 The validation of these estimations is challenging due to the complications in uncertainty at various scale trends. However, these models are amplified for a larger time scale at multiple nodes for future and past projection and considered to be more appropriate for the estimation of the quantification of terrestrial carbon stocks and fluxes.
4.2.4 Remote sensing estimations.
Since the early 80's, terrestrial carbon fluxes and stock estimations extended their peak with the support of remote sensing (RS)-based estimation. RS enables researchers to achieve a brief view of the considered enigmatic terrestrial ecosystems, which are inaccessible with multiple temporal (diurnal to decadal) and spatial (regional to global) scale data sets.7,89,133 To date, the spatial viability of the RS platform ranges with a coarser-resolution of higher than 250 m (ref. 118 and 134) (e.g., MODIS and AVHRR), medium-resolution of between 10–200 m (ref. 94) (e.g., Landsat and Sentinel), and finer-resolution of less than 10 m (ref. 15, 89 and 135) (e.g., QuickBird and IKONOS). Monitoring the carbon flux is important to quantify the terrestrial carbon cycle, which can be achieved using RS with high spatial heterogenetic consistency and repeated cycles.15,83,120 In this case, RS provides solutions to most of the challenges encountered with other methods.83 To provide an outlook of RS in carbon quantification, Table 4 highlights the properties of contemporary RS platforms. RS also assists in modelling and observational measurements to quantify the nature of various terrestrial carbons in an ecosystem.
Table 4 Characteristics of representative spaceborne sensor properties in analysing terrestrial carbon fluxes, stocks and parameters
Remote sensing |
Sensor |
Platform |
Optimal spectral range |
Example |
Attributes |
FIR – far infrared; NIR – near infrared; B – blue; SWIR – shortwave infrared; TIR – thermal infrared; MW – microwave.
|
Optical |
Active |
LiDAR |
750 nm to 1.1 μm (FIR–NIR)a |
Carbon – 3D |
• Carbon stock estimator with canopy assessment |
• Higher accuracy |
• Regional scale |
Passive |
Multi spectra |
380 nm to 2.5 μm (B-SWIR)a |
MODIS |
• Carbon flux and carbon stock estimator |
LANDSAT |
• Nominal to highest accuracy |
SENTINEL |
• Global scale |
|
Thermal spectra |
10.3–12.5 μm (TIR)a |
|
• Carbon flux estimator (ER with LST) |
• Nominal to highest accuracy |
• Global scale |
Microwave |
Active |
RADAR |
0.3–100 cm (MW)a |
ALOS-PALSAR |
• Carbon stock estimator (vertical forest structure and canopy strata) |
• High accuracy |
• Global scale |
Passive |
|
|
SMAP |
• NEE and soil carbon estimator |
• High accuracy |
• Global scale |
4.2.4.1 RS carbon flux estimations.
The RS of carbon fluxes is mainly manifested by the optical spectrum channels and IR channels in various methods using vegetation indices (VIs), land surface temperature (LST), land cover, model integration, etc.15,134 Flux measurements are mainly estimated based on the biotic primary production (photosynthesis), canopy structure, vegetational shift, biotic ontogeny, phenology28,131 and with several VIs to understand the spatial and temporal productivity and variability in an ecosystem. Briefly, RS captures the variation in the light absorption spectrum by the biota using multiple VIs, an indicator to identify the chlorophyll content.101 Besides the primary productions, phenology and VIs, RS is integrated to estimate the canopy level N, CH4 and other biophysical parameters to estimate the key carbon flux drivers at variable scales.16,45 Various studies such as that by Turner et al.,83 Potter et al.118 and Tang et al.136 highlighted the prevailing correlation between VIs and key flux drivers (NPP, NEE, and ER). This has been further diversified by developing the light use efficiency (LUE) models,15,47,137 which are purely based on RS datasets and the integration of meteorological parameters. The studies by Potter et al.118 and Wang et al.138 indicated the high accuracy of NPP assessment by VIs, which is significant in the eddy covariance method, simplifying the hurdles in the carbon flux models from the regional to global scale. Similar correlated studies have been conducted in the tropical belt with homogenous meteorological, biophysical and phenological variability across space and time, which have been widely used since the early 90's.119 Further, the growing number of global flux towers provide continuous data integration with RS and multiple biophysical and biogeochemical cycle variables. Nevertheless, relatively few studies have indicated the impact of higher spectral hindrance and enviro-climate-driven parameters in ecosystems, leading to a negative correlation between VIs and flux measurements, which are mostly observed in the mid-latitudes that exhibit heterogeneous characteristics between the biotic system and the enviro-climate variables.15 Studies over the arid regions indicate reasonable accuracy in the GPP and NEE estimations, where the surface carbon fluxes vary with the biotic system and the meteorological variables, which are highly sensitive to other biophysical variable-based carbon flux estimation methods.85,88,89 Since the development of spaceborne platforms, tracing and quantifying the ecosystem dynamics have become robust and promising through various proxies. One of these proxies is the solar-induced chlorophyll fluorescence (SIF), which exhibits an inherent relationship with photosynthesis and an apparent equivalent connection with GPP.139–141 Since the first spaceborne measured SIF was retrieved by Joiner et al.142 and Frankenberg et al.,143 various earth observation missions have been explored constantly to retrieve SIF, unlocking a new prospect to trace the dynamics of ecosystem productivity. The integration of these proxy variables potentially reduces the uncertainty in the carbon flux estimation83,101 and used to assist various mediums of estimation, such as the model-driven eddy flux measurements and allometric models, by interpolated and extrapolated data profiles to increase the temporal and spatial scale with finer resolution.
Specifically, RS provides various accuracy indexes depending on the nature of the carbon flux. Studies on GPP indicate its better accuracy114,136 compared to NEP, ER and other carbon fluxes given that the micrometeorological variability in the subsurface is not well constrained. Supporting this, Ravindranath and Ostwald,144 Xiao et al.15 and Worden et al.13 reported that the RS estimation of GPP was underestimated for the occluded cloudy region. Additionally, the lower accuracy in estimation persists due to the absence of field sources on the biotic strand, distribution, heterogenicity, pedological veracity, etc., which leads to various uncertainties in quantifying the nature of the carbon sink and source.45,135 The integration of in situ flux analysis with RS may significantly provide other sets of uncertainties and regional/global flux variability due to the biased distribution of the sites (study accuracy was higher in the temperate belt than in the tropics, where the site distribution can be inferred from Table 2 and Fig. S1†).145
The RS environment significantly boosts the accuracy of the model, especially for production efficiency models such as the LUE models, to analyze the regional carbon flux.15,47,137,146 Also, these models are reliable sources of regional GPP and NPP in RS carbon flux estimation, given that they provide knowledge on the biotic strata, growth, conditions, etc. These models are dynamically related to RS with the biotic absorbed photosynthetically active radiation (APAR)15 and fraction of photosynthetically active radiation (fPAR)48 in estimating the key fluxes. Few RS systems directly provide APAR and fPAR for longer time steps (e.g., MODIS) in the estimation of GPP. Most of the LUE models are integrated with LUC, enviro-climatic regulations, pedological characteristics and the concentration of atmospheric CO2.137 These models are widely developed and incorporated with most of the prognostic and diagnostic process-based models explicitly in quantifying the surface carbon fluxes such as ER, GPP, NPP, NEP and NBP (e.g., CASA and GLO-PEM). They quantify capricious surface terrestrial flux to understand the variability of an ecosystem in a realistic approach with response to the enviro-climatic conditions. Few models optimize the RS data as parameters in the estimation to reduce the uncertainty in the simulated carbon fluxes.146
Limitations in the RS-based estimation exist in the dataset in terms of atmospheric contamination (cloud cover and reflectivity), sensor errors, retrieval algorithm errors, etc., which significantly increase the uncertainty.15 These data errors pertain to the data sources, such as the errors in data acquisition, processing, and validation. This cascades to the quantification accuracy, where the point error causes a higher frame in terms of other estimation sources. These various sources of error result in spatial and temporal variability in the terrestrial carbon dynamics,118,136 which obscure the magnitude and trend of carbon flux estimations and potentially alter the interannual variability and long-term trend of primary productivities.13,76 Given that RS is an inevitable source for terrestrial carbon estimation, reducing these errors will help in understanding the carbon dynamics, both spatially and temporally. Together with the inherent data errors, other sources of errors persist, such as the model parametrization/structure, key data parameters, and field-level validation sample bias errors.15,89 The RS models have been reported to exhibit many uncertainties due to the substantial model parametrization, where rectifying these uncertainties remains a challenge to date.
4.2.4.2 RS carbon stock estimations.
The application of RS technology for quantifying terrestrial carbon stock commenced in the early 90's using an optical medium, which was later improved towards microwaves (RADAR) and LiDAR.89,135 These platforms are widely employed in estimating various carbon stocks such as AGC, BGC and SOC. Optical RS,15,93 which exhibits significant properties towards biota, is considered to be a sensitive estimator of AGC to date. Similar to carbon flux estimation, carbon stocks are estimated with various VIs to understand the biotic attributes in estimating AGC and BGC.111 The most widely used VIs in the estimation include DVI, EVI, NDVI, SAVI, and SRI by optimizing multispectral bands.52,134 Subsequently, they are supported with texture analysis (using GLCM or FOTO method) to understand the biotic canopy attributes to differentiate the multi-variate structure in an ecosystem, which is an effective carbon stock predictor.111 Also, RS can be integrated with ground-based estimators such as allometric models and inventory datasets, i.e., VIs, LAI, canopy structure, and spectral mixture analysis, which are considered to be the most important biotic variables in estimating the available carbon stocks using empirical, regression, and integrated models.113
Generally, regression (OLS, SMLR, GWR, etc.) models are widely employed because of their local precision with minimal uncertainty, which is optimal for multivariable-independent predictors in carbon stock estimations.111,147 These models are further improved with various biophysical, spatial and enviro-climatic variables for higher accuracy with minimal uncertainty.94 Discerning an important linear variable is difficult given that many optimal variables unanimously estimate carbon stocks. These complications with the defined variables lead to the use of non-parametric methods (machine learning, neural networks, random forest, etc.) in estimating the carbon stocks, which are highly efficient in current research.147 They are employed to consistently estimate the carbon stocks from the regional to global scale at multiple and continuous timesteps with plausible uncertainties. The integration of non-parametric methods with allometric models also gives better results than the empirical models, given that they extend the system with the heterogenetic properties of the biotic environment.15,89 Although multispectral RS is highly advantageous in estimating carbon stocks at the regional scale, their estimation at the global scale diverges with errors due to the heterogenetic phenological state of the biotic environment, which are regionally defined.93,135
Besides the multispectral optical medium, microwave platforms (radiometers, i.e., passive RS, and scatterometers, i.e., active RS) are also used to estimate and quantify carbon stocks.95,104,135 Mostly, microwave RS has the potential to detect environmental properties even with high atmospheric disturbances (e.g., cloud cover and aerosol). Various studies on the RADAR-based estimation of carbon stocks indicted that radar backscattering117 is proportional to the carbon stock, which is substantial in the estimation, and showed a strong correlation with various environmental factors based on the level of its sensitivity in the assessment. Given that the use of RADAR in the estimation of carbon stocks is comparatively less than the optical RS, where the future development programs such as BIOMASS, SAOCOM, NISAR, TanDEM-X, and ALOS-4, will be qualitatively helpful for carbon stock estimation in association with optical RS.15,89
Similar to RADAR, LiDAR, which is an active RS medium, provides information on physical variables such as the vertical canopy structure, LAI, and canopy cover to estimate the terrestrial carbon stocks.104,148 These are regional-based estimator methods that provide precise estimation of the heterogeneous ecosystem, which are highlighted towards the estimation of variable biotic traits (leaves, trunk, foliage, etc.).95,148 LiDAR-based estimations also assist in producing allometric models with a non-destructive perception. LiDAR-based estimation of carbon stock is widely employed for ecosystems with a heterogenous biotic stratum, especially over the tropical belt.135 This is highly possible with the accurate capturing of the canopy density and the vertical structure, which are then used as a physical variable in the estimation of the carbon stock. This estimation is integrated with in situ measurements to construct a high-profile regional carbon stock estimation model, which is reasonably consistent. Future carbon estimation using LiDAR sensors depends on missions such as ATLAS, GEDI, and MOLI for higher precision at a large scale.15,89 The main disadvantages of RADAR and LiDAR are their temporal inconsistency and spatial discontinuity over a larger area, which hinder the continuous carbon stock assessment, unlike the optical RS system,12,148,149 exhibiting significant inconsistencies towards the estimation. Similar to the estimation frameworks in quantifying the magnitude of carbon stocks, the same aspects for carbon uncertainties have to be perused.
5 Associates of terrestrial carbon
The terrestrial carbon dynamics is controlled by ER and GPP, which are highly disturbed by various associated factors both positively and negatively. Studies state that about 270 Pg C per year is involved in the biotic terrestrial flux, which exhibits intra- and inter-annual variability at a significant level.35,76,136,150 These uncertainties are highly associated with various carbon responses, i.e., the intra-annual associates such as climate variables and inter-annual associates such as hydrology, LUC and soil N.
5.1 C and N: the rejoinder
Experimental studies such as that Parton et al.,67 Thum et al.101 and Reich et al.73 suggest that nitrogen supports and boosts biotic growth with a higher carbon concentration by enhanced NPP and GPP. This materializes in soil rich in inorganic nitrogen by decomposition (mineralization), which substantially increases the biota to act as a carbon sink more than a source.101,113 In other cases, it influences the carbon response towards climate change at a regional scale by coercing the removal of carbon from the terrestrial environment to the atmosphere. Due to the structural modulation in driving plant growth, nitrogen is usually neglected in most analyses, which leads to an overestimation of the terrestrial carbon balance.16,151 A handful of studies analyzed the reluctance in the interactions of C–N and prove that the estimation of terrestrial and atmospheric carbon interactions is overrated due to the deficient magnitude of N,152i.e., the terrestrial carbon sink was reduced by about ∼25% with C–N coupling.35 Supporting this, recent studies considering C–N restraints emphasized the increase in terrestrial carbon storage with a restrained increase in temperature and by neglecting N, resulted in a divergent trend with global warming,1,30,101,132 noticeably shifting the aspect of carbon-climate feedback. Due to the shifting pattern in agriculture and LUC, the influence of an increased magnitude in nitrogen to the naturally nitrogen-deficient terrain can potentially influence the carbon storage and alter the climate dynamics.45,54 Anthropogenic induction increases the reactive nitrogen (Nr), which potentially increases the carbon sink in the ecosystem,79,153 as concluded by various global studies. Prior to the late 90's, it was widely presumed that terrestrial nitrogen does not alter the nature of the terrestrial carbon.54 However, geographically explicit studies on C–N indicate the strong response of nitrogen towards carbon. Especially, the study by Jain et al.151 indicated that moist temperate and boreal forests, which lacked terrestrial N, acted as a carbon source in the 90's. Due to rapid climate change and the increase in nitrogen mineralization over the region during the early 21st century, where the meagre impact of nitrogen dynamics on terrestrial carbon flux reduced the GPP and changed the nature of the ecosystem.35,79,113 These studies bring out an aspect of the nature of terrestrial carbon under periodical stress of global climate and environmental change with the impact of nitrogen dynamics.
5.2 Hydrological linkages to carbon
The carbon cycle in the terrestrial biome is strongly influenced by various ecological and climatic variables.127,154 One of these regulators is hydrology. The effects of carbon dynamics and the hydrological cycle on the changing climate scenarios have not been studied closely.155 The hydrological cycle is significant in determining the variability of photosynthesis and biotic growth. Given that GPP is directly proportional to the increasing concentration of atmospheric CO2, it ultimately cascades to higher NPP availability in the ecosystem with higher AGC and BGC storage.16,156 Studies suggest that the decadal increase in CO2 concentration enhanced the NPP availability by 20–25%.10,153,157 The increase in CO2 concentration impacts the C-hydrological cycle balance due to the rapid photosynthesis, which decreases the evapotranspiration (ET) flux in water-deficient areas.110,126,158
In another aspect, evapotranspiration (ET), which is an unavoidable component of the water cycle, is mediated by stomatal closure of the biota, corresponding to the efflux and influx of carbon through ER.44,75,81 Under climate change, with the induced ET, the ecotypes in the terrestrial ecosystem experience soil drying with decreased soil-leaf water potential.44,154 The traditional paradigm of ER and climate proportionality was demonstrated in recent studies such as that by Green et al.,126 Nakayama and Pelletier,155 Sharma et al.110 and Worden et al.,13 highlighting that the hydrological factors are strongly correlated with the key carbon flux drivers to a high degree. However, an underestimation or overestimation of the factorial consideration of hydrology with the carbon cycle occurs due to the challenges in assessing the water storage in various regions.13,154 The development in RS has improved the understanding of the coupling nature of carbon and water in recent decades, but multifaceted relation of carbon with other factors increases the difficulty in quantifying the exact magnitude of these driving factors.
5.3 Agriculture and carbon cycle
The carbon flux is altered by the agricultural LUC, which profoundly affects the regional and global carbon cycle. At present, 40% of the planet's terrestrial geography is covered by agriculture and pasture lands,6,25 where the rate of carbon released from agriculture to the atmosphere increases by about 0.9 ± 0.8 Pg C per year.10,156 Further, the changes induced by LUC affect the nutrient value and species composition of the region, leading to a decrease in carbon-fixing potential. With the alarming increase in population, humans have emerged as a pilot in driving the carbon cycle by agriculture, which increased before the industrial era.8,17,28 However, the lack of direct information on the effect of agricultural LUC in changing the carbon cycle is due to its importance.18 For example, in India, the total cropland during 2018–2019 was about ∼200 Mha, which was comparatively higher in the past decades (change of ∼5 Mha), and consequently the carbon stock in the cropped area was estimated to be 138 Pg C per year.159 Furthermore, these changes also caused a large loss in the SOC. Crop-associated fires, such as in the Indo-Gangetic Plains, provide carbon to other systems, increasing the uncertainty range.26 These variations create an imbalance in the nature of the carbon source or sinks by modifying the terrestrial carbon cycle. This has prompted researchers to proposed an impossible state to quantify the uncertainties in the carbon variations due to the agricultural LUC.6,160 They also affect other biogeochemical cycles such as the CH4, nitrogen and hydrological cycles at the ecosystem level, leading to regional climate changes, which are blind spots in climate change.
5.4 Urbanization and carbon cycle
Although urbanization is considered a highly intriguing spatial phenomenon, it is rarely considered in carbon cycle studies (apart from LUC), given that the trend in population is irregular with the urban topology.161 According to IPCC,23 the carbon storage in built-up areas is ∼10% of the stored terrestrial carbon, where urban areas tend to play a great role in the carbon fluctuation in systems on Earth and global climate change. The tendency in carbon accumulation in urban areas is the transfer of carbon material (in various forms) to the city from matter produced in remote ecosystems, which creates two pools in cities, i.e., buildings and landfills (∼1.6% of the terrestrial carbon cycle).40,161 Substantially, these new carbon pools are small (mostly a century old) and are also framed in urbanized vegetation (∼0.4% global vegetation carbon) and soils (∼0.22% global soil carbon). The studies by Vetter et al.59 and Messori et al.61 estimated that the urban vegetation carbon storage accounts for about ∼0.16% (∼112 Pg C) in the terrestrial carbon cycle, indicating the substantial magnitude of the global carbon sink. The relative dynamics of the carbon footprint transfer on an urban scale and its trend should be studied deeply. This small portion of carbon in urban systems will increase over time, and thus studies investigating the effect of urbanization on the carbon cycle on a global scale should be conducted.
6 Carbon-climate feedback
Human intervention in the environment has led to global warming by increasing the atmospheric CO2 concentration (from 280 ppm in the mid-19th century to 410 ppm in the 21st century), resulting in an increase in temperature of about 0.5–0.7 °C in the last few decades.17,18,153 A variation in carbon in a system results in extreme seasonal variations and redistributes the global and regional climatic patterns. Studies on carbon-climate feedback modelling provide evidence for the binodal impact between terrestrial carbon and the climate,16,56 although their interdependent effects are not well understood. Fig. 2 schematically represents the feedbacks of various factors that affect the climate and carbon system.
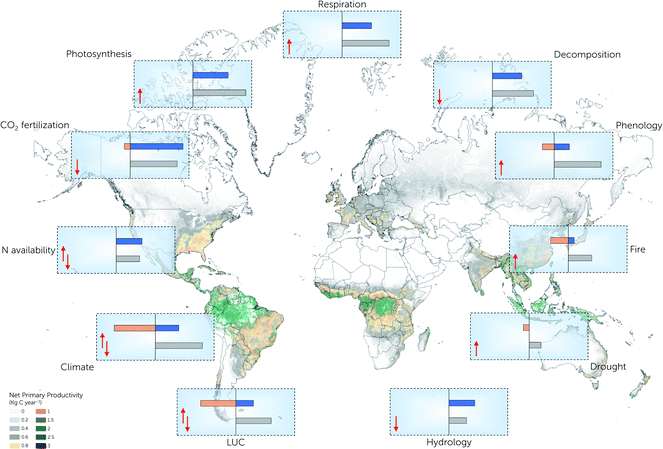 |
| Fig. 2 Schematic of major terrestrial carbon drivers that lead to positive (blue bar) or the negative (red bar) feedback loops to the enviro-climate medium. The bar plots signify the simplified form of understanding the feedback in the system. The confidence in the scientific understanding of the system is signified by the grey bar. The upward arrow represents the source and the downward arrow signifies the concurrent sink for predominant carbon pools under changing climate. The superimposed NPP map is for the year 2020, produced from MODIS, https://lpdaac.usgs.gov/products/mod17a3hv006/. | |
Gregory et al.,9 Piao et al.113 and Friedlingstein10 reported multiple contrary responses, where the carbon flux increased with an extended phenological season with positive precipitation and negative temperature variance. With the higher uncertainty in the quantification of terrestrial carbon, the past and the future climatic responses exhibit a variation across different models, which is from the carbon-climatic feedbacks and the increasing trend in CO2 concentration.10,37,120,156 Most of the carbon fluxes (both in terms of sink and source) are sensitive to temperature variations, leading to diverging changes in primary production. These kinetic sensitive carbon fluxes alter other enviro mechanisms such as the phenology of the biota, variations in the seasonal production of an ecosystem, and hydrological and nutritional dynamics in response to global warming.15,16,124 A few important feedbacks to be considered in the altering nature of the terrestrial carbon cycle include the dynamics in the Ra and Rh, increase in the regional LUC, variability in the growing season of the biota, changes in the species composition and regional drought.6,10,43
Some studies indicated that the ecosystem exhibits different responses with respect to the temporal variability.16,73,101 For a short timestep, increasing temperature, as a catalyst to the carbon fluxes, increases the primary production.17,43,45,116 In contrast, for a longer timestep, the carbon fluxes are insensitive to the temperature in simulating the primary production as the ecotypes adapt to the recent past temperature variability,45,116 which reduces the carbon loss for a longer period. The long-term effect of climate change on the ecosystem is highly noted by the dynamics in the primary production of the biota and in the carbon fluxes. In the projected future climate scenarios, the ecotypes adapt to it at a faster rate in ER and photosynthesis.33,37,101 Consequently, this reduces the residual time of carbon in the terrestrial system, which is also strongly influenced by the hydrological cycle.21,81,132 The biotic phenology has been used in research as a long-term climate indicator that is highly sensitive to various climatic and environmental variables.71 The RS studies on this variability by Piao et al.113 and Xiao et al.15 demonstrated the advancement in the phenology by three days in spring and a delay by two days in autumn per decade. These changes directly affect the nature of terrestrial carbon.
As stated earlier, nitrogen regulates the key drivers of the terrestrial carbon fluxes, whereas a climate-induced alteration in the soil nutrients has a long-term effect on the terrestrial ecosystem.29,48,73 The demand for nitrogen for accelerated primary production eventually leads to limited nitrogen under a potential climate change scenario.43,113 The accelerated increase/reduction in the nitrogen level by climate change potentially increases/restrains the carbon fluxes and stocks, altering the nature of the terrestrial carbon. The influence of climatic change on the hydrological cycle, together with drought and LUC, changes the carbon trend by soil drying with decreased soil-leaf water potential.79 This variation in the hydrological cycle induces improved plant growth/mortality, resulting in a shift in the biotic composition at the ecosystem level, which ultimately changes the terrestrial system from carbon sink to source and vice versa.8,43 Drought potentially plays a crucial role in the terrestrial carbon budget, given that the precipitation trend is significantly altered by global warming, resulting in the projection of increased drought frequencies in response to the current climate trend.20 These climate-driven droughts are projected to be short and intense.
Hypothetically, almost all the model-based studies agree with this carbon-climate feedback. However, the challenges in encountering uncertainties persist across various levels in these models.9,10,75 The model-based assumptions in carbon-climate feedbacks include various biophysical and enviro-climatic variables, which have large uncertainties due to their methods and scales. To date, it remains a great challenge to assess carbon-climate feedback even with high-performance models and experimental evidence.
7 Terrestrial carbon cycle magnitude and trend
Due to recent technological advancements in the quantification of carbon, research on the carbon balance signifies a synoptic understanding of the terrestrial cycle despite its system-driven uncertainties.10,22 Due to the disparities in the quantification methods, a general agreement on the dynamic nature of the sink and sources in the world is still under debate. However, most research indicates a decline in the carbon sinks due to the loss of the forest ecosystem, with the support of RS-based quantification.14 However, they are regionally constrained for a precise ecosystem, but then these associated trends are not ideal for analyzing the overall projection trend.15,47 These long-term trends and variability in an ecosystem rely on the employed process-based model and RS monitoring source. In time, the rapid anthropogenic trend and demand for resources, which are likely to simulate the rate of LUC, will eventually disturb the nature of the carbon sources/sink. Experimental studies indicate that LUC is pragmatically higher in the forest ecosystem,66,120 which is unlikely to change due to the growing population trend, and the carbon captured in the terrestrial sink is not likely to be trapped for a longer period. These aspects induce a higher degree of CO2 fertilization owing to climate change, which was scientifically agreed by the mounting trend of CO2 concentrations.17,18,153 Theoretical studies tried to reduce this significant increase; however, a consensus in the amplitude of the CO2 concentration remains elusive. Wang et al.138 supported this by depicting the increased seasonal trend of atmospheric CO2 over the past few decades with the increase in CO2 fertilization effects. Although biophysical and climate-mediated factors are reasoned to be responsible for the breakdown of atmospheric CO2 fertilization, the existing CO2, which has been sufficiently high since the pre-industrial epoch, was considered to be the primary driver in strengthening the terrestrial carbon uptake.122 Empirical evidence suggests that this feedback link is four- or more times stronger and more uncertain than the direct carbon-climate feedback.16,52,162 The understanding of this effect was strengthened by the findings reported across various ecosystems including cropland,163 grassland,114 tropics,49 and boreal forest.14
However, climate-change-induced drought stress consistently balanced the seasonal trend. This was reflected in the South American and Eastern Asian regions, where the stress was significant. Given that the drought influenced the moisture availability substantially, the trend was proportional to the GPP, and thus the carbon sink.2 Further, the competence of the biophysical effects driven by the ecosystem to mitigate this changed with the climate. Although this mediation is non-persistent across longer temporal scales, local-scale perturbation is highly critical. Given that climate change prompts reverse pressure, it further affects the overall terrestrial carbon cycle. The enviro-climate and biophysical factors may contradict this scenario and demand an innovative multi-aspect integrated model to predict the nature of the sink and sources under this variability.
Besides atmospheric CO2, at the continental scale, the dynamics in the spatial and seasonal variability of terrestrial carbon are expressed in response to ecosystem respiration, photosynthesis, productivity, LUC, fires, etc. However, the degree to which they affect the carbon cycle remains uncertain. In fact, extreme environmental conditions (drying and greening) across the tropics recently may have seriously transformed the carbon storage capacity of the tropical ecosystems. On account of the absence of uniform in situ networks, the tropics are conjectured to be the home of the largest uncertainty in the terrestrial carbon balance. For instance, tropical forests are generally ruled out as moisture-limited ecosystems. However, episodic drought-mediated moisture limitation introduces stress on the primary productivity, which weakens the carbon uptake potential of the tropics. This can be compared to the 2010 Amazonia drought, where the Brazilian forests were shaped from a sink to source of 0.5 Pg C per year.13,164 Although tropical regions act as a host of the largest terrestrial carbon fluxes, there is a lack of agreement on their contribution to the global carbon cycle. There are multiple lines of evidence suggesting that in the past five decades, they tipped their balance by 2 Pg C per year.5,10,14,165 The inference by Walker71 and Schlesinger3 highlights that hardly one-third of this balance is attributed to tropical forests and one quarter from the tropical savannas.
Besides the fluctuations in the environmental drivers such as temperature and precipitation, the tropical regions are the nexus of global LUC. Multiple studies shared the consensus of the neutrality of tropical carbon pools in the 90's; however, the global demand for various means from the tropics caused frequent tipping of the carbon balance. Nevertheless, the global terrestrial carbon estimations indicate that the terrestrial sink is large and escalating.
In recent decades, the terrestrial ecosystems have acted as a carbon sink, with an average uptake of 2.5 Pg C per year.5,33,166 However, the biosphere is not a static carbon trapper; in fact, the tropical forest alone reverts to ∼65 Pg C per year,13,132,167 through Ra and Rh. However, studies14,16,36,49,138 claim that these estimations are attributed to the difference in the quantification methods, processes and the region of interest, and thus subject to a wide range of uncertainties. If we consider the most extensively explored region with a sophisticated in situ network, i.e., North America, most studies claim that this region is a carbon sink with a range of 0.28–0.89 Pg C per year. This large difference arises from the methods employed and the geography, where the inversion models exhibit larger sinks than the in situ-based models, and the lack of dense in situ networks besides the contiguous United States. On the contrary, with uniform in situ sites (spatial distribution of the Fluxnet network shown in Fig. S1†), estimations in Europe depicted good correspondence between the inversion models and in situ network, suggesting that the terrestrial sink of Europe is 0.89 Pg C per year.168–170 Unlike Western Europe and North America, studies on the carbon sink in the Eastern Europe countries highlighted the agreement between the methods (0.55–0.66 Pg C per year),168,171 despite the poor data network. The regions with dominant tropical forests, such as South America and Africa, are often debated with respect to the distribution and the magnitude of the carbon pools, with large uncertainties, where forest fires and LUC are likely to influence the order of magnitude irrespective of the estimation method. Overall, the global terrestrial system is considered to act as a carbon sink of 2.2 Pg C per year,71,172 which is consistent with the ensemble of various DVGMs (2.7 Pg C per year).166
Because of the sparse ecological significance in the western front and in situ networks in the southern front of the Asian countries, little attention has been given to them in the global-scale analysis. However, studies that quantified the fluxes over these regions proved their vitality for constraining estimates of the global terrestrial carbon cycle.40,46,79,173,174 Cervarich et al.,40 by integrating atmospheric inversion models and DVGMs, estimated the terrestrial carbon budget of South Asian countries for the last few decades. In their study, they assessed the increasing trend of the terrestrial sink from 0.41 Pg C per year (1980s) to 0.54 Pg C per year (2000s). Supporting this, Harris et al.175 further showed that the carbon sink in Asia was relatively stronger (0.65 Pg C per year (2010's and 20's)40,175), reflecting carbon removal similar to that of the Congo Basin.176 This surge in South Asia is attributed to the CO2 fertilization effect,57 with significant interannual variability.11 Considering this, India and other South-East Asian countries exhibited a substantial normalized growth trend in carbon uptake. However, the border-sharing countries of India, i.e., Nepal,177 Bhutan,40 Bangladesh178 and Sri Lanka,179 had a negative growth in terrestrial carbon, i.e., decreasing carbon sink and increasing carbon source.46 Being home to monsoons and rapid urbanization, the higher variability in precipitation, temperature and LUC highly modulates the carbon fluxes for the countries in South Asia, where studies40,173,178–180 attempted to quantify the nature of the flux, i.e., 200.6 Tg C per year (India); 14.2 Tg C per year (Pakistan); 9.2 Tg C per year (Nepal); 2.2 Tg C per year (Bhutan); 3.6 Tg C per year (Sri Lanka) and 10.6 Tg C per year (Bangladesh). Studies focusing on the South Asian countries are limited; however, their results were significant enough to emphasize the transforming nature of the biosphere. For example, the mean NBP of South and South-East Asian countries was estimated to be 0.55 Pg C per year. Further, by summarizing the results, Cervarich et al.40 depicted that the carbon fluxes of the South Asian countries have large uncertainties due to the persistence of year-to-year variability across various biomes.
Despite its huge biospheric domain, the lack of a continuous observational network, similar to that in the Western world (Fig. S1†), different modelling studies over India have indicated a considerable fraction of uncertainty in the global carbon cycle. With its geographical significance and enviro-climatic threat, the decadal and seasonal variability of India's terrestrial carbon cycle remain unknown. The following sections summarize the past and the present nature of India's terrestrial carbon cycle, which was explored by a few studies considering its global significance.
7.1 Flux trend in India
India contains 2% of the planet's forest cover and is at the top of tropical nations in terms of afforestation.144 As a significant marker in the biospheric-atmospheric carbon exchange, India plays a crucial role in the regional and global carbon flux. Due to its heterogenetic geographical nature, studies on carbon fluxes in India are site-specific on a regional scale.160 Without the assistance of models and RS, estimations of carbon fluxes are inventory based, which inherits high spatial and temporal bounds in the estimated magnitude.
A few studies attempted to quantify the magnitude of the fluxes on a national scale. Historical studies on the carbon fluxes in India primarily focused on NEP and GPP. Studies by Brown et al.174 and Houghton181 estimated that the net carbon flux in India during the 80's varied from ∼40–20 Tg C. These results were contradicted by Ravindranath et al.182 with an estimation of 5 Tg C. The studies by Dadhwal et al.,82 Kaul et al.183 and Salunkhe et al.107 indicate that the terrestrial system of the Indian subcontinent acted as a source in the 80's, which later turned to a sink in the 20's. The decadal studies by Dadhwal77 and Rao et al.58 estimated the nationwide NEP for 1980–2000, which portrayed a higher variability trend. Haripriya184 quantified that the annual carbon flux accounted for ∼12 Tg C in the 90's. With the model assimilation, Chhabra and Dadhwal185 estimated that the net carbon flux was 47 Tg C in the 80's and 90's and this estimation is relatively consistent with that from the studies by Brown et al.174 and Houghton.181 The negative trend in the NEP and GPP fluxes is linear with a higher atmospheric CO2 concentration. Kaul et al.183 reported that the net carbon sink in India during the 90's was ∼1 Tg C per year using RS and inventories. Haripriya184 and Chhabra and Dadhwal97 used model assimilation by integrating LUC to assess the net carbon flux, which was ∼5 Pg C during the late 90's.
The carbon fluxes in India during the last decades increased steadily (GPP = ∼6.00 Tg C per year, Ra = ∼4 Tg C per year, NPP = ∼1.5 Tg C per year and Rh = ∼1.8 Tg C per year).11,35,46 Studies potentially stated the impact of CO2 fertilization on the positive trend of carbon fluxes in the ecosystems in India. Due to the higher rate of fluxes, the net carbon stock in plants was considerably lower, which exhibited a negative decadal trend.46 By employing the ISAM model, Gahlot et al.35 stated that the variability in carbon over the decades is due to the cascading of positive temperature and negative precipitation trends, resulting in carbon loss through ER and partial loss by LUC. They further stated that a higher rate of carbon was lost from the biosphere by various carbon fluxes during the 90's to 2000's.
With multi-model assimilation, Rao et al.,58 Ramachandra and Bharath18 and Hari et al.11 estimated the NEE to be ∼195 Tg C per year, for which India and other South Asian countries acted as a carbon sink in the 2000's. Cervarich et al.40 and Gahlot et al.35 employed multiple DGVMs and stated that the Indian terrestrial system acts as a carbon sink with ∼185 Tg C per year of NBP and ∼201 Tg C per year of NEP (both values signify a carbon sink). However, the analyzed simulation excluded fossil fuel emissions, ensuring India is a carbon sink. Consistently, multi-model assimilation studies suggested higher uncertainty in the estimation based on the model approaches.35,82,160,186 Furthermore, forest fires emit carbon similar to the NBP, about ∼3 Pg C per year,137 which significantly varies inter-annually and drives the global change. In the study by Sannigrahi et al.,137 using the CASA and VPM model, they showed that various burnt indices on coupling with NPP cause synergistic and trade-off interactions between the ecosystem productivity and the fire attributes. Few pilot studies have attempted to analyze individual carbon fluxes for a shorter time step; however, studies assessing all the carbon flux drivers at a national scale are limited and lack in projecting the future trend.58
7.2 Stock trend in India
Due to the heterogenetic ecosystem traits, carbon stock assessments in India are non-systematic, sporadic, and bound to a particular ecosystem. Thus, no robust data or methodological study has been carried out for a uniform estimation of the carbon stocks in India.84,107 As an agrarian country, the agricultural areas hold high potential carbon stocks with seasonal dynamics. Most of the studies are based on the characteristics of the forest-based ecosystem. This limitation in the studies in India is mainly due to the disparities in the data and methodical frame by edaphic, climatic and ecosystemic variations, which include alpine to tropical evergreen and arid to swamps.107,133 This variability can be traced back the systematic analysis of carbon estimation with a complete invariable dataset. The earliest evidence of carbon stock quantification in India can be traces back to the 90's. The estimation by Hingane56 using historical ecological data indicated a decrease in the carbon stock by about 4.52 Pg C from the 19th to 20th century.
Under the National Carbon Project, efficient long-term analysis of the carbon stock and flux by multi-variate RS datasets was performed by Reddy et al.133 to provide a synoptic overview of the trend of carbon stock in India. The study stated that the carbon stocks in India subsequently decreased from 98.09 t ha−1 to 93.27 t ha−1 in the last century, which correlates with the change in the world biomass, with a decrease of 10 Gt C in recent decades. Most of the research on Indian carbon stocks is based on RS estimation, where the study by Dadhwal et al.82 estimated the carbon stock to be ∼8000 Mt in the 80's, which increased to ∼8100 Mt in the 90's with the LUC change in the forest area of ∼64 Mha. The other significant studies attempted on a national scale were by Chhabra et al.97 and Haripriya184 for the period of 1980 to 1990. Recent decadal studies133,186 estimated the total change in carbon stock in India to be ∼570 Mt per year at the rate of ∼185 Mt per year in 2003–2005, whereas ∼540 Mt per year at the rate of ∼115 Mt per year in 2005–2007, significantly representing the negative trend of the stock. Reddy et al.133 reported the highest trend of carbon stocks in the 90's with a gain of ∼670 Tg C. In contrast, the lowest historical trend was in the 1940s with an annual loss of ∼50 Tg C. Similarly, in the last few years, a loss of ∼55 Tg C was observed in 2010 with an overall quantification of ∼3000 Tg C. The annual report by the Forest Survey of India (FSI)86 indicates an increase in carbon stocks of about 1.48% in 2015 with a net stock of ∼2647 Mt, whereas, in 2016, the AGC stocks in India were estimated to be ∼2200 Mt and the BGC and SOC ∼4500 Mt with an annual increase of 19 Mt.
The dense forests are reported with the highest carbon stocks of ∼60 Mg C ha−1, contributing 56%, moderately dense forests are estimated to have ∼49 Mg C ha−1 with 32%, whereas the open forests are estimated to be with ∼30 Mg C ha−1 with 12% of the total forest carbon stock in India.84,107 The mean carbon stock for the forest ecosystem was reported to be ∼52 Mg C ha−1. The Indian forest cover changed positively by about ∼4000 Sq km in 2019 compared to that in 2015, but with a negative change in the carbon stocks, the Forest Survey of India (FSI)86 reported low carbon regenerating forest areas, indicating the temporal and spatial loss of carbon stocks and gain of forest biota. These national studies with different estimation approaches produced multi-variate trends in the carbon stocks. Thus, based on the various study trends, India cannot be reported to be either a carbon sink or source.
The SOC in India was first assessed by Jenny and Raychaudhuri187 based on field inventory. This was then followed up by Brown et al.,174 who estimated ∼7 Pg C of SOC in 1980. Chhabra et al.97 estimated the trend of SOC to be around ∼6.5 Pg C during the 1950s and around ∼6 Pg C during the 1985s, which almost correspond with the estimations of Brown et al.174 The RS-based estimations of SOC were reported by Chhabra and Dadhwal185 to be ∼10 Pg C for the 1880 s and ∼6.5 Pg C for the 1980s. Other significant studies exhibited similar trends to the former studies, with no significant positive/negative trend in SOC over India.
Pilot studies111,117,183,186 based on the historical inventories, regional ecological data and contemporary RS data are widely employed at the regional scale. At the regional level, maximum carbon stocks were found in the states of Arunachal Pradesh (995 Mt C), Madhya Pradesh (700 Mt C), Chhattisgarh (560 Mt C), Maharashtra (500 Mt C) and Karnataka (470 Mt C) by carbon pool contribution, i.e., AGC of 32%, BGC of 12%, and SOC of 56%.86 This structurally varying trend was idealized by complex gradients of enviro-climatic factors, biophysical, forest management, ecological management and several other attributes. Specifically, the states of Arunachal Pradesh, Telangana, Tamil Nadu, Karnataka and Odisha act as the major sources of carbon, whereas Madhya Pradesh, Kerala, Chhattisgarh and Uttarakhand are actively considered as sinks.18,107,133,160 The reduction of carbon stocks by natural (removal of topsoil by erosion, nitrogen reduction, etc.) and anthropogenic (shifting agriculture, forest fires, etc.) factors are reasons for the carbon sources in the above-mentioned states of India. The Forest Survey of India (FSI)86 indicated that ∼10 Mt C ha−1 was removed from the northeastern states due to the sifting of agricultural practices at the rate of 1.5 Mt C ha−1. The uncertainty in the estimation with models and methods used in these studies was emphasized by the varying higher magnitude of carbon stocks.
8 Concluding remarks
8.1 Summary
Climate change studies have inevitably focused on the atmospheric carbon concentration in the last two centuries, where the dynamics and the response are determined by the various biosphere and atmospheric ecosystems. At various spatial and temporal scales, the dynamics of carbon in the terrestrial ecosystem are regulated by multi-modal carbon fluxes and stocks, which are natural processes, whereas the impromptu removal of carbon from an ecosystem leads to higher variability in the environment, which are mainly of the anthropogenic medium, i.e., LUC and fires. Various questions such as “how does the biosphere respond to a spontaneous amplification in atmospheric CO2?”, “at what spatial and temporal scale does an ecosystem resist climate change?”, “what is the steady-state of the terrestrial system in the carbon cycle, and does it remain neutral?”, and “will the terrestrial carbon system continue to act the same with the alarming climate change and urbanization in the near future?” are still unanswered by the research community in terms of carbon geography. The international forum on climate change struggles to articulate global policies due to the persistence of large uncertainties, which amplifies the gap in understanding the global and regional terrestrial carbon cycle.
According to this review, with advanced knowledge about the carbon cycle, the science community is confident in predicting the nature of the terrestrial ecosystem in the dynamics of carbon in response to global and regional enviro-climate changes. However, to precisely forecast these changes, the ambiguities in terrestrial carbon are not only determined by the atmospheric CO2 concentration but also by anthropogenic interventions and the bimodal impact of climate. The rate of climate change will reduced if the terrestrial system acts as a carbon sink, whereas it accelerates global warming and cascades to climate change to a greater extent if the terrestrial system transforms into a carbon source.
Although the understanding of the carbon cycle by the science community has been facilitated by various advanced process-based estimations/assessments, the level of uncertainties has equally increased. The advancement in RS strongly compliments the model and observational-based assessment with quantitative knowledge globally. With its bias, RS aids the analysis with relatively higher spatial and temporal resolution but then complexes the system medium with the multiple integrations. To overcome the complexity of the analysis methods, various associates of the carbon cycle (hydrological process, nutrient budget, LUC, fires, etc.) are ignored, which further increases the uncertainty. Again, the uncertainty follows the traits of the various mediums as in a closed loop. Thus, developing a systematic (fundamental, pragmatic, probabilistic) approach may provide a deeper understanding to predict and quantify the future trend by addressing these unanswered questions about the terrestrial carbon cycle and its feedback on the enviro-climate system.
8.2 Future perspective
The nature of climatic regimes solely depends on the role of the terrestrial carbon dynamics, which are altered by anthropogenic and natural factors. Deeper knowledge to understand the uncertainties and dynamics is necessary for strategic management and protection of the planetary systems to mitigate climate change.
This understanding can be acquired with the integration of well-designed networks of observational systems, spaceborne platforms and systematic models. Also, the sampling biases in the observational networks need to be considered for multiple ecotypes to understand the variability. On a large scale, the high-resolution flux monitoring is geographically biased, which possibly omits the missing pieces of the global carbon cycle intentionally. Additionally, observational coverage on the associate drivers realistically provides the neglected properties and their relative significance in the carbon dynamics.
Compiling RS and observational traits based on biotic structure, functional diversity and spatial variability provides quantitatively unbiased terrestrial properties. Multiple methods reveal multiple constraints at the same spatial and temporal scale, and thus there needs to be a consistent system. Integrated models self-propagate the temporal variability in the projection given that a systematic stochastic model (includes multi-modal key drivers) requires extensive studies on the response between the ecosystem and climate. The aggregation of the various temporal and spatial scales exhibits complexity in understanding the terrestrial heterogeneity in carbon geography, which is likely to be scaled up by the high-confident RS measurements. Close monitoring of the LUC trend should be considered in the inclusion of regional carbon studies as a persuasive factor. The combination of RS and integrated models pushes the boundaries in understanding the sensitive dynamics in the biogeochemical cycles by bringing interdisciplinary scientific communities together.
Despite the complexity of the carbon cycle, various systematic studies exhibit sufficient results to comprehend the outline of the nature of carbon in the terrestrial system. Qualitative and quantitative studies of terrestrial carbon dynamics in response to climate change are likely to advance the understanding of climate prediction knowledge in the near future. We, the inhabitants of Earth, live in an epoch that constantly needs to be informed about the alarming enviro-climatic system changes by atmospheric CO2 in our home, by us, which perturbs us; as stated by Broecker188 “The inhabitants of planet Earth are quietly conducting a gigantic experiment. We play Russian roulette with climate and no one knows what lies in the active chamber of the gun”.
Author contributions
MH: conceptualization, writing – original draft preparation, review and editing, visualization; BT: conceptualization, writing – review and editing, supervision. Both the authors have read and approved the final manuscript.
Conflicts of interest
There are no conflicts to declare.
Acknowledgements
The authors would like to acknowledge the National Institute of Technology Rourkela for providing lab facilities. The authors are thankful to Dr Guillermo Murray-Tortarolo for his valuable suggestions and stimulating scientific discussions. We sincerely apologize to all of the authors whose valuable contributions to the terrestrial carbon cycle could not be individually cited due to space limitations.
References
- A. Glikson, The lungs of the Earth: review of the carbon cycle and mass extinction of species, Energy Procedia, 2018, 146, 3–11 CrossRef CAS.
- T. F. Keenan and C. A. Williams, The terrestrial carbon sink, Annu. Rev. Environ. Resour., 2018, 43, 219–243 CrossRef.
- W. H. Schlesinger and E. S. Bernhardt, The Carbon Cycle of Terrestrial Ecosystems, Biogeochemistry, 2020, 141–182 Search PubMed.
- L. P. Wackett, Global biogeochemical cycles, Environ. Microbiol., 2016, 18, 1088–1089 CrossRef.
- F. A. Busch, Reducing the gaps in our understanding of the global terrestrial carbon cycle, New Phytol., 2015, 206, 886–888 CrossRef CAS PubMed.
- A. D. Jones,
et al., Quantifying Human-Mediated Carbon Cycle Feedbacks, Geophys. Res. Lett., 2018, 45(11), 370–379 Search PubMed.
- P. J. Sellers, D. S. Schimel, B. Moore, J. Liu and A. Eldering, Observing carbon cycle-climate feedbacks from space, Proc. Natl. Acad. Sci. U. S. A., 2018, 115, 7860–7868 CrossRef CAS PubMed.
- D. Wu,
et al., Accelerated terrestrial ecosystem carbon turnover and its drivers, GCB Bioenergy, 2020, 26, 5052–5062 Search PubMed.
- J. M. Gregory, C. D. Jones, P. Cadule and P. Friedlingstein, Quantifying carbon cycle feedbacks, J. Clim., 2009, 22, 5232–5250 CrossRef.
- P. Friedlingstein, Carbon cycle feedbacks and future climate change, Philos. Trans. R. Soc., A, 2015, 373, 20140421 CrossRef PubMed.
- M. Hari, B. Tyagi, M. O’Sullivan and S. Sitch, India is drying out its terrestrial carbon: an inference by multi-model estimation of primary productivities, Earth and Space Science Open Archive, 2021, 1, 10509106 Search PubMed.
- G. P. Asner and J. Mascaro, Mapping tropical forest carbon: calibrating plot estimates to a simple LiDAR metric, Remote Sens. Environ., 2014, 140, 614–624 CrossRef.
- J. Worden,
et al., Satellite Observations of the Tropical Terrestrial Carbon Balance and Interactions With the Water Cycle During the 21st Century, Rev. Geophys., 2021, 59, e2020RG000711 CrossRef.
- D. Schimel,
et al., Observing terrestrial ecosystems and the carbon cycle from space, GCB Bioenergy, 2015, 21, 1762–1776 Search PubMed.
- J. Xiao,
et al., Remote sensing of the terrestrial carbon cycle: a review of advances over 50 years, Remote Sens. Environ., 2019, 233, 111383 CrossRef.
- D. A. Way, A. Cook and A. Rogers, The effects of rising CO2 concentrations on terrestrial systems: scaling it up, New Phytol., 2021, 229, 2383–2385 CrossRef PubMed.
- B. D. Stocker,
et al., Multiple greenhouse-gas feedbacks from the land biosphere under future climate change scenarios, Nat. Clim. Change, 2013, 3, 666–672 CrossRef CAS.
- T. V. Ramachandra and S. Bharath, Carbon Sequestration Potential of the Forest Ecosystems in the Western Ghats, a Global Biodiversity Hotspot, Nat. Resour. Res., 2020, 29, 2753–2771 CrossRef CAS.
- J. Zeng,
et al., Global terrestrial carbon fluxes of 1999–2019 estimated by upscaling eddy covariance data with a random forest, Sci. Data, 2020, 7, 1–11 CrossRef PubMed.
- H. D. Matthews, A. J. Weaver and K. J. Meissner, Terrestrial carbon cycle dynamics under recent and future climate change, J. Clim., 2005, 18, 1609–1628 CrossRef.
- R. A. Houghton, F. Hall and S. J. Goetz, Importance of biomass in the global carbon cycle, J. Geophys. Res.: Biogeosci., 2009, 114, 1–13 Search PubMed.
- Z. Wu,
et al., Climate data induced uncertainty in model-based estimations of terrestrial primary productivity, Environ. Res. Lett., 2017, 12, 064013 CrossRef.
-
IPCC, 2019 Refinement to the 2006 IPCC Guidlines for National Greenhouse Gas Inventories, Volume 4: Agriculture, Forestry and Other Land Use: Forest Land, Forestry, vol. 4, 2006, pp. 1–29 Search PubMed.
- Z. Xie,
et al., Past, present and future of the carbon cycle, Natl. Sci. Rev., 2014, 1, 18–21 CrossRef.
- N. Ramankutty and J. A. Foley, Characterizing patterns of global land use: an analysis of global croplands data, Global Biogeochem. Cycles, 1998, 12, 667–685 CrossRef CAS.
-
K. Lorenz and R. Lal, Carbon Sequestration in Agricultural Ecosystems. Carbon Sequestration in Agricultural Ecosystems, Springer International Publishing, 2018 Search PubMed.
- T. Toru and K. Kibret, Carbon stock under major land use/land cover types of Hades sub-watershed, eastern Ethiopia, Carbon Balance Manage., 2019, 14, 1–14 CrossRef PubMed.
- S. Sitch,
et al., Evaluation of ecosystem dynamics, plant geography and terrestrial carbon cycling in the LPJ dynamic global vegetation model, GCB Bioenergy, 2003, 9, 161–185 Search PubMed.
- Z. Fu,
et al., Recovery time and state change of terrestrial carbon cycle after disturbance, Environ. Res. Lett., 2017, 12, 104004 CrossRef.
- A. Arneth,
et al., Terrestrial biogeochemical feedbacks in the climate system, Nat. Geosci., 2010, 3, 525–532 CrossRef CAS.
- P. J. Rayner,
et al., Interannual variability of the global carbon cycle (1992–2005) inferred by inversion of atmospheric CO2 and δ13CO2 measurements, Global Biogeochem. Cycles, 2008, 22, GB3008 CrossRef.
- T. Maki,
et al., New technique to analyse global distributions of CO2 concentrations and fluxes from non-processed observational data, Tellus B, 2010, 62, 797–809 CrossRef.
- S. Piao,
et al., Interannual variation of terrestrial carbon cycle: issues and perspectives, GCB Bioenergy, 2020, 26, 300–318 Search PubMed.
- N. Zeng, A. Mariotti and P. Wetzel, Terrestrial mechanisms of interannual CO2 variability, Global Biogeochem. Cycles, 2005, 19, 1–15 CrossRef.
- S. Gahlot, S. Shu, A. K. Jain and S. Baidya Roy, Estimating Trends and Variation of Net Biome Productivity in India for 1980–2012 Using a Land Surface Model, Geophys. Res. Lett., 2017, 44(11), 573–579 Search PubMed.
- Y. Liu, S. Piao, X. Lian, P. Ciais and W. K. Smith, Seasonal responses of terrestrial carbon cycle to climate variations in CMIP5 models: Evaluation and projection, J. Clim., 2017, 30, 6481–6503 CrossRef.
- P. Friedlingstein,
et al., Climate-Carbon Cycle Feedback Analysis: Results from the C4MIP Model Intercomparison, J. Clim., 2006, 19, 3337–3353 CrossRef.
- B. Lahn, A history of the global carbon budget, Wiley Interdiscip. Rev. Clim. Change, 2020, 11, 1–9 Search PubMed.
- T. Erbrecht and W. Lucht, Impacts of large-scale climatic disturbances on the terrestrial carbon cycle, Carbon Balance Manage., 2006, 1, 1–7 CrossRef.
- M. Cervarich,
et al., The terrestrial carbon budget of South and Southeast Asia, Environ. Res. Lett., 2016, 11, 1–11 Search PubMed.
- J. I. House, I. C. Prentice, N. Ramankutty, R. A. Houghton and M. Heimann, Reconciling apparent inconsistencies in estimates of terrestrial CO2 sources and sinks, Tellus B, 2003, 55, 345–363 CrossRef.
- A. A. Bloom, J. F. Exbrayat, I. R. Van Der Velde, L. Feng and M. Williams, The decadal state of the terrestrial carbon cycle: global retrievals of terrestrial carbon allocation, pools, and residence times, Proc. Natl. Acad. Sci. U. S. A., 2016, 113, 1285–1290 CrossRef CAS PubMed.
- Y. Luo, Terrestrial carbon-cycle feedback to climate warming, Annu. Rev. Ecol. Evol. Syst., 2007, 38, 683–712 CrossRef.
- L. Cheng,
et al., Recent increases in terrestrial carbon uptake at little cost to the water cycle, Nat. Commun., 2017, 8, 110 CrossRef PubMed.
- T. Tharammal, G. Bala, N. Devaraju and R. Nemani, A review of the major drivers of the terrestrial carbon uptake: model-based assessments, consensus, and uncertainties, Environ. Res. Lett., 2019, 14, 093005 CrossRef.
- M. Hari and B. Tyagi, India's Greening Trend Seems to Slow Down. What Does Aerosol Have to Do with It?, Land, 2022, 11, 538 CrossRef.
- W. K. Smith, A. M. Fox, N. MacBean, D. J. P. Moore and N. C. Parazoo, Constraining estimates of terrestrial carbon uptake: new opportunities using long-term satellite observations and data assimilation, New Phytol., 2020, 225, 105–112 CrossRef PubMed.
- F. Veroustraete, H. Sabbe and H. Eerens, Estimation of carbon mass fluxes over Europe using the C-Fix model and Euroflux data, Remote Sens. Environ., 2002, 83, 376–399 CrossRef.
- D. Schimel, B. B. Stephens and J. B. Fisher, Effect of increasing CO2 on the terrestrial carbon cycle, Proc. Natl. Acad. Sci. U. S. A., 2015, 112, 436–441 CrossRef CAS PubMed.
- M. A. McCarthy,
et al., Resource allocation for efficient environmental management, Soil Ecol. Lett., 2010, 13, 1280–1289 CrossRef PubMed.
- I. C. Prentice, S. P. Harrison and P. J. Bartlein, Global vegetation and terrestrial carbon cycle changes after the last ice age, New Phytol., 2011, 189, 988–998 CrossRef CAS PubMed.
- J. Yun,
et al., Enhanced regional terrestrial carbon uptake over Korea revealed by atmospheric CO2 measurements from 1999 to 2017, GCB Bioenergy, 2020, 26, 3368–3383 Search PubMed.
- M. Reichstein,
et al., Determinants of terrestrial ecosystem carbon balance inferred from European eddy covariance flux sites, Geophys. Res. Lett., 2007, 34, L01402 CrossRef.
- C. A. Varotsos, V. F. Krapivin and V. Y. Soldatov, Modeling the carbon and nitrogen cycles, Front. Environ. Sci. Eng., 2014, 2, 1–6 Search PubMed.
- J. Lehmann and M. Kleber, The contentious nature of soil organic matter, Nature, 2015, 528, 60–68 CrossRef CAS PubMed.
- L. S. Hingane, Some aspects of carbion dioxide exchange between atmosphere and Indian plant biota, Clim. Change, 1991, 18, 425–435 CrossRef CAS.
- S. Fatichi, C. Pappas, J. Zscheischler and S. Leuzinger, Modelling carbon sources and sinks in terrestrial vegetation, New Phytol., 2019, 221, 652–668 CrossRef CAS PubMed.
- A. S. Rao, G. Bala, N. H. Ravindranath and R. Nemani, Multi-model assessment of trends, variability and drivers of terrestrial carbon uptake in India, J. Earth Syst. Sci., 2019, 128, 1–19 CrossRef CAS.
- M. Vetter,
et al., Analyzing the causes and spatial pattern of the European 2003 carbon flux anomaly using seven models, Biogeosciences, 2008, 5, 561–583 CrossRef CAS.
- F. I. Woodward, T. M. Smith and W. R. Emanuel, A global land primary productivity and phytogeography model, Global Biogeochem. Cycles, 1995, 9, 471–490 CrossRef CAS.
- G. Messori, G. Ruiz-Pérez, S. Manzoni and G. Vico, Climate drivers of the terrestrial carbon cycle variability in Europe, Environ. Res. Lett., 2019, 14, 063001 CrossRef CAS.
- J. Chen, J. Liu, J. Cihlar and M. Goulden, Daily canopy photosynthesis model through temporal and spatial scaling for remote sensing applications, Ecol. Modell., 1999, 124, 99–119 CrossRef CAS.
- H. Chu, D. D. Baldocchi, R. John, S. Wolf and M. Reichstein, Fluxes all of the time? A primer on the temporal representativeness of Fluxnet, J. Geophys. Res.: Biogeosci., 2017, 122, 289–307 CrossRef.
- W. Chen, J. Chen and J. Cihlar, An integrated terrestrial ecosystem carbon-budget model based on changes in disturbance, climate, and atmospheric chemistry, Ecol. Modell., 2000, 135, 55–79 CrossRef CAS.
- G. Pastorello,
et al., The Fluxnet2015 dataset and the ONEFlux processing pipeline for eddy covariance data, Sci. Data, 2020, 7, 225 CrossRef PubMed.
- P. Shao, X. Zeng, K. Sakaguchi, R. K. Monson and X. Zeng, Terrestrial carbon cycle: climate relations in eight CMIP5 earth system models, J. Clim., 2013, 26, 8744–8764 Search PubMed.
- W. J. Parton, J. W. B. Stewart and C. V. Cole, Dynamics of C, N, P and S in grassland soils: a model, Biogeochemistry, 1988, 5, 109–131 CrossRef CAS.
- R. H. Waring, J. J. Landsberg and M. Williams, Net primary production of forests: a constant fraction of gross primary production?, Tree Physiol., 1998, 18, 129–134 CrossRef PubMed.
- C. P. Giardina, M. G. Ryan, D. Binkley and J. H. Fownes, Primary production and carbon allocation in relation to nutrient supply in a tropical experimental forest, GCB Bioenergy, 2003, 9, 1438–1450 Search PubMed.
- Y. Pan,
et al., A large and persistent carbon sink in the world’s forests, Science, 2011, 333, 988–993 CrossRef CAS PubMed.
- A. P. Walker,
et al., Integrating the evidence for a terrestrial carbon sink caused by increasing atmospheric CO2, New Phytol., 2021, 229, 2413–2445 CrossRef CAS PubMed.
- N. Delpierre,
et al., Quantifying the influence of climate and biological drivers on the interannual variability of carbon exchanges in European forests through process-based modelling, Agric. For. Meteorol., 2012, 154–155, 99–112 CrossRef.
- P. B. Reich,
et al., Synergistic effects of four climate change drivers on terrestrial carbon cycling, Nat. Geosci., 2020, 13, 787–793 CrossRef CAS.
- J. T. Randerson, F. S. Chapin, J. W. Harden, J. C. Neff and M. E. Harmon, Net Ecosystem Production:a Comprehensive Measure of Net Carbon Accumulation By Ecosystems, Appl. Ecol. Environ., 2002, 12, 937–947 CrossRef.
- Z. Wang,
et al., Sensitivity of terrestrial carbon cycle to changes in precipitation regimes, Ecol. Indic., 2020, 113, 106223 CrossRef.
- D. Papale,
et al., Towards a standardized processing of Net Ecosystem Exchange measured with eddy covariance technique: algorithms and uncertainty estimation, Biogeosciences, 2006, 3, 571–583 CrossRef CAS.
- V. K. Dadhwal, Assessment of Indian Carbon Cycle Components Using Earth Observation Systems and Ground Inventory, Int. Arch. Photogramm. Remote Sens. Spat. Inf. Sci., 2012, 8, 249–254 CrossRef.
-
D. Beerling and F. I. Woodward, Vegetation and the terrestrial carbon cycle: modelling the first 400 million years, Vegetation and the terrestrial carbon cycle: modelling the first 400 million years, Cambridge University Press, 2001 Search PubMed.
- B. Jia, Y. Wang and Z. Xie, Responses of the terrestrial carbon cycle to drought over China: modeling sensitivities of the interactive nitrogen and dynamic vegetation, Ecol. Modell., 2018, 368, 52–68 CrossRef CAS.
- C. Rödenbeck, S. Houweling, M. Gloor and M. Heimann, CO2 flux history 1982–2001 inferred from atmospheric data using a global inversion of atmospheric transport, Atmos. Chem. Phys., 2003, 3, 1919–1964 CrossRef.
- S. Mystakidis, S. I. Seneviratne, N. Gruber and E. L. Davin, Hydrological and biogeochemical constraints on terrestrial carbon cycle feedbacks, Environ. Res. Lett., 2017, 12, 014009 CrossRef.
- V. K. Dadhwal, S. Singh and P. Patil, Assessment of phytomass carbon pools in forest ecosystems in India, NNRMS Bull., 2009, 1–7 Search PubMed.
- D. P. Turner, S. V. Ollinger and J. S. Kimball, Integrating remote sensing and ecosystem process models for landscape- to regional-scale analysis of the carbon cycle, Bioscience, 2004, 54, 573–584 CrossRef.
- A. A. Wani, P. K. Joshi, O. Singh and R. Pandey, Carbon Inventory Methods in Indian Forests – A Review, Int. J. Agric. Sci., 2012, 2, 315–323 Search PubMed.
- R. Lal, Carbon Cycling in Global Drylands, Curr. Clim. Change Rep., 2019, 5, 221–232 CrossRef.
-
Forest Survey of India (FSI), Carbon Stock in India's Forest, Isfr, 2017, pp. 121–136 Search PubMed.
- G. Petrokofsky,
et al., Comparison of methods for measuring and assessing carbon stocks and carbon stock changes in terrestrial carbon pools. How do the accuracy and precision of current methods compare? A systematic review protocol, Environ. Evid., 2012, 1, 1–21 Search PubMed.
- L. Qie,
et al., Long-term carbon sink in Borneo’s forests halted by drought and vulnerable to edge effects, Nat. Commun., 2017, 8, 014009 CrossRef PubMed.
- S. Issa, B. Dahy, T. Ksiksi and N. Saleous, A review of terrestrial carbon assessment methods using geo-spatial technologies with emphasis on arid lands, Remote Sens., 2020, 12, 2008 CrossRef.
- F. J. Aguilar, A. Nemmaoui, A. Peñalver, J. R. Rivas and M. A. Aguilar, Developing allometric equations for teak plantations located in the coastal region of ecuador from terrestrial laser scanning data, Forests, 2019, 10, 1050 CrossRef.
- C. P. De Oliveira,
et al., Estimation of the aboveground biomass and carbon stocks in open Brazilian Savannah developed on sandy soils, Carbon Balance Manage., 2019, 14, 1–10 CrossRef PubMed.
- T. Kenzo,
et al., General estimation models for above- and below-ground biomass of teak (Tectona grandis) plantations in Thailand, For. Ecol. Manage., 2020, 457, 117701 CrossRef.
- O. Cartus,
et al., A national, detailed map of forest aboveground carbon stocks in Mexico, Remote Sens., 2014, 6, 5559–5588 CrossRef.
- P. Vicharnakorn, R. P. Shrestha, M. Nagai, A. P. Salam and S. Kiratiprayoon, Carbon stock assessment using remote sensing and forest inventory data in Savannakhet, Lao PDR, Remote Sens., 2014, 6, 5452–5479 CrossRef.
- N. A. Mohd Zaki, Z. A. Latif and M. N. Suratman, Modelling above-ground live trees biomass and carbon stock estimation of tropical lowland Dipterocarp forest: integration of field-based and remotely sensed estimates, Int. J. Remote Sens., 2018, 39, 2312–2340 CrossRef.
- A. Chhabra, S. Palria and V. K. Dadhwal, Growing stock-based forest biomass estimate for India, Biomass Bioenergy, 2002, 22, 187–194 CrossRef.
- A. Chhabra, S. Palria and V. K. Dadhwal, Spatial distribution of phytomass carbon in Indian forests, GCB Bioenergy, 2002, 8, 1230–1239 Search PubMed.
- R. D. Bardgett, D. A. Wardle and G. W. Yeates, Linking above-ground and below-ground interactions: how plant responses to foliar herbivory influence soil organisms, Soil Biol. Biochem., 1998, 30, 1867–1878 CrossRef CAS.
- P. E. Thornton and N. A. Rosenbloom, Ecosystem model spin-up: estimating steady state conditions in a coupled terrestrial carbon and nitrogen cycle model, Ecol. Modell., 2005, 189, 25–48 CrossRef CAS.
- S. S. Gbondo-Tugbawa, C. T. Driscoll, J. D. Aber and G. E. Likens, Evaluation of an integrated biogeochemical model (PnET-BGC) at a northern hardwood forest ecosystem, Water Resour. Res., 2001, 37, 1057–1070 CrossRef CAS.
- T. Thum,
et al., A new model of the coupled carbon, nitrogen, and phosphorus cycles in the terrestrial biosphere (QUINCY v1.0; revision 1996), Geosci. Model Dev., 2019, 12, 4781–4802 CrossRef CAS.
- T. Atsbha, A. Belayneh Desta and T. Zewdu, Carbon sequestration potential of natural vegetation under grazing influence in Southern Tigray, Ethiopia: implication for climate change mitigation, Heliyon, 2019, 5, e02329 CrossRef PubMed.
- M. Reichstein and N. Carvalhais, Aspects of Forest Biomass in the Earth System: Its Role and Major Unknowns, Surv. Geophys., 2019, 40, 693–707 CrossRef.
- S. Brown and A. E. Lugo, Trailblazing the carbon cycle of tropical forests from puerto rico, Forests, 2017, 8, 1–18 Search PubMed.
- A. Bondeau,
et al., Modelling the role of agriculture for the 20th century global terrestrial carbon balance, GCB Bioenergy, 2007, 13, 679–706 Search PubMed.
- C. S. Potter and S. A. Klooster, Global model estimates of carbon and nitrogen storage in litter and soil pools: response to changes in vegetation quality and biomass allocation, Tellus B, 1997, 49, 1–17 CrossRef.
- O. Salunkhe, P. K. Khare, R. Kumari and M. L. Khan, A systematic review on the aboveground biomass and carbon stocks of Indian forest ecosystems, Ecological processes, 2018, 7, 17 CrossRef.
- B. Huy, G. T. Thanh, K. P. Poudel and H. Temesgen, Individual plant allometric equations for estimating aboveground biomass and its components for a common bamboo species (Bambusa procera A. Chev. and A. Camus) in tropical forests, Forests, 2019, 10, 316 CrossRef.
- B. Poulter,
et al., Global Biogeochemical Cycles to variability in satellite-observed burned area, Global Biogeochem. Cycles, 2015, 29, 207–222 CrossRef CAS.
- S. Sharma,
et al., Carbon and evapotranspiration dynamics of a non-native perennial grass with biofuel potential in the southern U.S. Great Plains, Agric. For. Meteorol., 2019, 269–270, 285–293 CrossRef.
- M. Hari, S. Srinivasan, A. Rajasekaran and B. Tyagi, Above ground carbon stock mapping over Coimbatore and Nilgiris Biosphere: A key source to the C sink, Carbon Manag., 2021, 12, 411–428 CrossRef CAS.
- J. A. Foley,
et al., An integrated biosphere model of land surface processes, terrestrial carbon balance, and vegetation dynamics, Global Biogeochem. Cycles, 1996, 10, 603–628 CrossRef CAS.
- S. Piao,
et al., Evaluation of terrestrial carbon cycle models for their response to climate variability and to CO2 trends, GCB Bioenergy, 2013, 19, 2117–2132 Search PubMed.
- M. Umair, D. Kim, R. L. Ray and M. Choi, Evaluation of atmospheric and terrestrial effects in the carbon cycle for forest and grassland ecosystems using a remote sensing and modeling approach, Agric. For. Meteorol., 2020, 295, 108187 CrossRef.
-
G. Burba and D. Anderson, A Brief Practical Guide to Eddy Covariance Flux Measurements: Principles and Workflow Examples for Scientific and Industrial Applications, LI-COR Biosciences, 2010, 9780615430133 Search PubMed.
- C. Yi,
et al., Climate control of terrestrial carbon exchange across biomes and continents, Environ. Res. Lett., 2010, 5, 034007 CrossRef.
- K. C. Thumaty,
et al., Estimation of above ground biomass for central Indian deciduous forests using ALOS PALSAR L-band data, J. Indian Soc. Remote Sens., 2016, 44, 31–39 CrossRef.
- C. Potter,
et al., Carbon fluxes in ecosystems of Yellowstone National Park predicted from remote sensing data and simulation modeling, Carbon Balance Manage., 2011, 6, 1–16 CrossRef PubMed.
- S. Crowell,
et al., The 2015–2016 carbon cycle as seen from OCO-2 and the global in situ network, Atmos. Chem. Phys., 2019, 19, 9797–9831 CrossRef CAS.
- A. Bastos,
et al., Sources of Uncertainty in Regional and Global Terrestrial CO2 Exchange Estimates, Global Biogeochem. Cycles, 2020, 34, 1–21 CrossRef.
- G. Monteil,
et al., The regional European atmospheric transport inversion comparison, EUROCOM: first results on European-wide terrestrial carbon fluxes for the period 2006–2015, Atmos. Chem. Phys., 2020, 20, 12063–12091 CrossRef CAS.
- T. F. Keenan,
et al., Recent pause in the growth rate of atmospheric CO2 due to enhanced terrestrial carbon uptake, Nat. Commun., 2016, 7, 13428 CrossRef CAS PubMed.
- F. Chevallier,
et al., CO2 surface fluxes at grid point scale estimated from a global 21 year reanalysis of atmospheric measurements, J. Geophys. Res.: Atmos., 2010, 115, D21307 CrossRef.
- M. Kawamiya,
et al., Development of an Integrated Earth System Model on the Earth Simulator, Journal of the Earth Simulator, 2005, 1–13 Search PubMed.
- C. E. Yang,
et al., Assessing terrestrial biogeochemical feedbacks in a strategically geoengineered climate, Environ. Res. Lett., 2020, 15, 104043 CrossRef CAS.
- J. K. Green,
et al., Regionally strong feedbacks between the atmosphere and terrestrial biosphere, Nat. Geosci., 2017, 10, 410–414 CrossRef CAS PubMed.
- E. A. Kowalczyk, Y. P. Wang and R. M. Law, The CSIRO Atmosphere Biosphere Land Exchange (CABLE) model for use in climate models and as an offline model, CSIRO Mar. Atmos. Res. Pap., 2006, 13, 1–42 Search PubMed.
- B. Smith, I. C. Prentice and M. T. Sykes, Representation of vegetation dynamics in the modelling of terrestrial ecosystems: comparing two contrasting approaches within European climate space, Glob. Ecol. Biogeogr., 2001, 10, 621–637 Search PubMed.
- Y. Pan,
et al., A biogeochemistry-based dynamic vegetation model and its application along a moisture gradient in the continental United States, J. Veg. Sci., 2002, 13, 369–382 CrossRef.
- G. Esser, J. Hoffstadt, F. Mack, W. Qu and U. Wittenberg, The high resolution biosphere model: status of development, validation, results/Le modèle biosphère haute résolution (HRBM): état des travaux, validation, résultats, Sci. Geol., Bull., 1997, 50, 73–88 CrossRef CAS.
- P. R. Moorcroft, G. C. Hurtt and S. W. Pacala, A Method for Scaling Vegetation Dynamics: The Ecosystem Demography Model, Ecol. Monogr., 2001, 71, 557 CrossRef.
- D. Baldocchi, Y. Ryu and T. Keenan, Terrestrial Carbon Cycle Variability, F1000Research, 2016, 5, 2371 Search PubMed.
- C. S. Reddy,
et al., Geospatial assessment of long-term changes in carbon stocks and fluxes in forests of India (1930–2013), Glob. Planet. Change, 2016, 143, 50–65 CrossRef.
- R. Nemani,
et al., Monitoring and forecasting ecosystem dynamics using the Terrestrial Observation and Prediction System (TOPS), Remote Sens. Environ., 2009, 113, 1497–1509 CrossRef.
- S. Abbas, M. S. Wong, J. Wu, N. Shahzad and S. M. Irteza, Approaches of satellite remote sensing for the assessment of above-ground biomass across tropical forests: Pan-tropical to national scales, Remote Sens., 2020, 12, 1–38 Search PubMed.
- X. Tang,
et al., Remotely monitoring ecosystem respiration from various grasslands along a large-scale east-west transect across northern China, Carbon Balance Manage., 2020, 15, 1–14 CrossRef PubMed.
- S. Sannigrahi, S. Rahmat, V. Rana and S. Chakraborti, Effects of forest fire severity on terrestrial carbon emission and ecosystem production in the Himalayan region, India, Sci. Total Environ., 2020, 725, 138331 CrossRef CAS PubMed.
- K. Wang,
et al., Causes of slowing-down seasonal CO2 amplitude at Mauna Loa, GCB Bioenergy, 2020, 26, 4462–4477 Search PubMed.
- X. Li and J. Xiao, TROPOMI observations allow for robust exploration of the relationship between solar-induced chlorophyll fluorescence and terrestrial gross primary production, Remote Sens. Environ., 2022, 268, 112748 CrossRef.
- H. Gao,
et al., Global analysis of the relationship between reconstructed solar-induced chlorophyll fluorescence (SIF) and gross primary production (GPP), Remote Sens., 2021, 13, 2824 CrossRef.
- G. Badgley, L. D. L. Anderegg, J. A. Berry and C. B. Field, Terrestrial gross primary production: using NIR V to scale from site to globe, GCB Bioenergy, 2019, 25, 3731–3740 Search PubMed.
- J. Joiner,
et al., First observations of global and seasonal terrestrial chlorophyll fluorescence from space, Biogeosciences, 2011, 8, 637–651 CrossRef CAS.
- C. Frankenberg and et al,
et al., New global observations of the terrestrial carbon cycle from GOSAT: patterns of plant fluorescence with gross primary productivity, Geophys. Res. Lett., 2011, 38, L17706 CrossRef.
-
N. H. Ravindranath and M. Ostwald, Carbon Inventory Methods: Handbook for Greenhouse Gas Inventory, Carbon Mitigation and Roundwood Production Projects, Springer, 2008 Search PubMed.
- P. Olofsson,
et al., Implications of land use change on the national terrestrial carbon budget of Georgia, Carbon Balance Manage., 2010, 5, 1–13 CrossRef PubMed.
- K. D. Noumonvi and M. Ferlan, Empirical vs. light-use efficiency modelling for estimating carbon fluxes in a mid-succession ecosystem developed on abandoned karst grassland, PLoS One, 2020, 15, e0237351 CrossRef CAS PubMed.
- L. Bai, Y. Shu and Y. Guo, Estimating aboveground biomass of urban trees by high resolution remote sensing image: a case study in Hengqin, Zhuhai, China, IOP Conf. Ser.: Earth Environ. Sci., 2020, 569, 012053 CrossRef.
- Q. Man, P. Dong, H. Guo, G. Liu and R. Shi, Light detection and ranging and hyperspectral data for estimation of forest biomass: a review, J. Appl. Remote Sens., 2014, 8, 081598 CrossRef.
- P. d. C. Bispo,
et al., Woody aboveground biomass mapping of the brazilian savanna with a multi-sensor and machine learning approach, Remote Sens., 2020, 12, 2685 CrossRef.
-
J. K. Hughes, P. J. Valdes and R. A. Betts, Dynamical properties of the TRIFFID dynamic global vegetation model. October Hadley Centre technical note 56, 2004 Search PubMed.
- A. Jain,
et al., Nitrogen attenuation of terrestrial carbon cycle response to global environmental factors, Global Biogeochem. Cycles, 2009, 23, 1–13 CrossRef.
- S. Zaehle and A. D. Friend, Carbon and nitrogen cycle dynamics in the O-CN land surface model: 1. Model description, site-scale evaluation, and sensitivity to parameter estimates, Global Biogeochem. Cycles, 2010, 24, GB1005 Search PubMed.
-
P. Ciais, et al., Carbon and Other Biogeochemical cycles. Clim. Chang. 2013 Phys. Sci. basis. Contrib. Work. Gr. I to Fifth Assess. Rep. Intergov. Panel Clim. Chang, 2013 Search PubMed.
- A. Govind and J. Kumari, Understanding the terrestrial carbon cycle: an ecohydrological perspective, Int. J. Ecol. Environ. Sci., 2014, 2014, 712537 Search PubMed.
- T. Nakayama and G. J. Pelletier, Impact of global major reservoirs on carbon cycle changes by using an advanced eco-hydrologic and biogeochemical coupling model, Ecol. Modell., 2018, 387, 172–186 CrossRef CAS.
- G. Krinner,
et al., A dynamic global vegetation model for studies of the coupled atmosphere-biosphere system, Global Biogeochem. Cycles, 2005, 19, 1–33 CrossRef.
-
G. N. Murray-tortarolo, Recent Trends in the Land Carbon Cycle, University of Exeter, 2015 Search PubMed.
- M. Hari, B. Tyagi, M. S. K. Huddar and A. Harish, Satellite-based regional-scale evapotranspiration estimation mapping of the rice bowl of Tamil Nadu: a little water to spare, J. Irrig. Drain., 2021, 70, 958–975 CrossRef.
-
Directorate of Economics & Statistics, Agricultural Statistics at a Glance 2019, 2020, https://eands.dacnet.nic.in/PDF/At%20a%20Glance%202019%20Eng.pdf Search PubMed.
- K. V. Satish,
et al., Geospatial assessment and monitoring of historical forest cover changes (1920–2012) in Nilgiri Biosphere Reserve, Western Ghats, India, Environ. Monit. Assess., 2014, 186, 8125–8140 CrossRef CAS PubMed.
- G. Churkina, The role of urbanization in the global carbon cycle, Front. Ecol. Evol., 2016, 3, 1–9 Search PubMed.
- C. Chen, W. J. Riley, I. C. Prentice and T. F. Keenan, CO2 fertilization of terrestrial photosynthesis inferred from site to global scales, Proc. Natl. Acad. Sci. U. S. A., 2022, 119, e2115627119 CrossRef CAS PubMed.
- P. E. Levy, M. G. R. Cannell and A. D. Friend, Modelling the impact of future changes in climate, CO2 concentration and land use on natural ecosystems and the terrestrial carbon sink, Glob. Environ. Change, 2004, 14, 21–30 CrossRef.
- E. T. A. Mitchard, The tropical forest carbon cycle and climate change, Nature, 2018, 559, 527–534 CrossRef CAS PubMed.
- L. D. M. Fonseca,
et al., Phenology and seasonal ecosystem productivity in an Amazonian floodplain forest, Remote Sens., 2019, 11, 1–17 Search PubMed.
- P. Friedlingstein,
et al., Global Carbon Budget 2021, Earth Syst. Sci. Data, 2021, 1–191 Search PubMed.
- Y. Y. Liu,
et al., Recent reversal in loss of global terrestrial biomass, Nat. Clim. Change, 2015, 5, 470–474 CrossRef.
- S. Luyssaert,
et al., The European land and inland water CO2, CO, CH4 and N2O balance between 2001 and 2005, Biogeosciences, 2012, 9, 3357–3380 CrossRef CAS.
- A. J. Dolman,
et al., An estimate of the terrestrial carbon budget of Russia using inventory-based, eddy covariance and inversion methods, Biogeosciences, 2012, 9, 5323–5340 CrossRef CAS.
- M. O’Sullivan,
et al., Small global effect on terrestrial net primary production due to increased fossil fuel aerosol emissions from East Asia since the turn of the century, Geophys. Res. Lett., 2016, 43, 8060–8067 CrossRef PubMed.
- I. E. Teubner, M. Forkel, B. Wild, L. Mösinger and W. Dorigo, Impact of temperature and water availability on microwave-derived gross primary production, Biogeosciences, 2021, 18, 3285–3308 CrossRef.
- Y. Zhang,
et al., Increased Global Land Carbon Sink Due to Aerosol-Induced Cooling, Global Biogeochem. Cycles, 2019, 33, 439–457 CrossRef CAS.
-
E. P. Flint and J. F. Richards, Trends in Carbon Content of Vegetation in South and Southeast Asia Associated with Changes in Land Use, 1994, pp. 201–299 Search PubMed.
- S. Brown, A. J. R. Gillespie and A. E. Lugo, Biomass of tropical forests of south and southeast Asia, Can. J. For. Res., 1991, 21, 111–117 CrossRef.
- N. L. Harris,
et al., Global maps of twenty-first century forest carbon fluxes, Nat. Clim. Change, 2021, 11, 234–240 CrossRef.
- P. Ploton,
et al., A map of African humid tropical forest aboveground biomass derived from management inventories, Sci. Data, 2020, 7, 1–13 CrossRef PubMed.
-
N. R. Joshi, K. Gauli and B. P. Subedi, Forest Carbon Assessment in Community-Managed Forest in the Nepal Himalayas: Strengthening Local Communities to Monitor Carbon Stocks under REDD + Initiatives, 2007, pp. 62–67 Search PubMed.
- M. R. Ullah and M. Al-Amin, Above- and below-ground carbon stock estimation in a natural forest of Bangladesh, J. Forensic Sci., 2012, 372–379 Search PubMed.
- K. C. Seto, B. Güneralp and L. R. Hutyra, Global forecasts of urban expansion to 2030 and direct impacts on biodiversity and carbon pools, Proc. Natl. Acad. Sci. U. S. A., 2012, 109, 16083–16088 CrossRef CAS PubMed.
- Y. Niwa,
et al., Imposing strong constraints on tropical terrestrial CO2 fluxes using passenger aircraft based measurements, J. Geophys. Res.: Atmos., 2012, 117, D11303 CrossRef.
- R. A. Houghton, Tropical deforestation and atmospheric carbon dioxide, Clim. Change, 1991, 19, 99–118 CrossRef CAS.
- N. H. Ravindranath, B. S. Somashekhar and M. Gadgil, Carbon flow in Indian forests, Clim. Change, 1997, 35, 297–320 CrossRef CAS.
- M. Kaul, V. K. Dadhwal and G. M. J. Mohren, Land use change and net C flux in Indian forests, For. Ecol. Manage., 2009, 258, 100–108 CrossRef.
- G. S. Haripriya, Carbon budget of the Indian forest ecosystem, Clim. Change, 2003, 56, 291–319 CrossRef CAS.
- A. Chhabra and V. K. Dadhwal, Estimating terrestrial net primary productivity over India using satellite data, Curr. Sci., 2004, 86, 269–271 Search PubMed.
- M. A. Sheikh, M. Kumar, R. W. Bussman and N. P. Todaria, Forest carbon stocks and fluxes in physiographic zones of India, Carbon Balance Manage., 2011, 6, 15 CrossRef CAS PubMed.
- H. Jenny and S. P. Raychaudhuri, Effect of climate and cultivation on nitrogen and organic matter reserves in Indian soils, Indian Counc. Agric. Res. New Delhi, 1960, 105–106 Search PubMed.
- W. S. Broecker, Unpleasant surprises in the greenhouse?, Nature, 1988, 328, 123–126 CrossRef.
- G. B. Bonan and S. Levis, Quantifying carbon-nitrogen feedbacks in the Community Land Model (CLM4), Geophys. Res. Lett., 2010, 37, L07401 CrossRef.
- G. A. Meehl,
et al., The WCRP CMIP3 multimodel dataset: a new era in climatic change research, Bull. Am. Meteorol. Soc., 2007, 1383–1394 CrossRef.
- H. Tian,
et al., North American terrestrial CO2 uptake largely offset by CH4 and N2O emissions: toward a full accounting of the greenhouse gas budget, Clim. Change, 2015, 129, 413–426 CrossRef CAS PubMed.
- P. Alton, L. Mercado and P. North, A sensitivity analysis of the land-surface scheme JULES conducted for three forest biomes: biophysical parameters, model processes, and meteorological driving data, Global Biogeochem. Cycles, 2006, 21, GB1008 Search PubMed.
- B. Franck, A. Rowold, C. Wegner and H. G. Eckert, Synthesis of probable and improbable precursors for porphyrin biosynthesis, Philos. Trans. R. Soc., B, 1976, 273, 181–189 CAS.
- R. P. Neilson, A model for predicting continental-scale vegetation distribution and water balance, Appl. Ecol. Environ., 1995, 5, 362–385 CrossRef.
- D. Bachelet,
et al., MC1: a dynamic vegetation model for estimating the distribution of vegetation and associated ecosystem fluxes of carbon, nutrients, and water, Gen. Tech. Reports, 2001, 1–101 Search PubMed.
- D. Vikhamar-Schuler, K. Müller and T. Engen-Skaugen, Snow modeling using SURFEX with the CROCUS snow scheme, Met. Rep., 2011, 2238 Search PubMed.
- S. SITCH,
et al., Evaluation of the terrestrial carbon cycle, future plant geography and climate-carbon cycle feedbacks using five Dynamic Global Vegetation Models (DGVMs), GCB Bioenergy, 2008, 14, 2015–2039 Search PubMed.
- A. Mouchet and L. M. François, Sensitivity of a global oceanic carbon cycle model to the circulation and to the fate of organic matter: preliminary results, Phys. Chem. Earth, 1996, 21, 511–516 CrossRef.
- K. R. Gurney,
et al., TransCom 3 CO2 inversion intercomparison: 1. Annual mean control results and sensitivity to transport and prior flux information, Tellus B, 2003, 55, 555–579 CrossRef.
- W. Peters,
et al., An atmospheric perspective on North American carbon dioxide exchange: CarbonTracker, Proc. Natl. Acad. Sci. U. S. A., 2007, 104, 18925–18930 CrossRef CAS PubMed.
- A. K. Jain, H. S. Kheshgi and D. J. Wuebbles, A globally aggregated reconstruction of cycles of carbon and its isotopes, Tellus B, 1996, 48, 583–600 CrossRef.
- P. Peylin,
et al., Daily CO2 flux estimates over Europe from continuous atmospheric measurements: 1, inverse methodology, Atmos. Chem. Phys., 2005, 5, 3173–3186 CrossRef CAS.
- P. K. Patra, M. Ishizawa, S. Maksyutov, T. Nakazawa and G. Inoue, Role of biomass burning and climate anomalies for land-atmosphere carbon fluxes based on inverse modeling of atmospheric CO2, Global Biogeochem. Cycles, 2005, 19, 1–10 Search PubMed.
-
P. Ciais, et al., Carbon and Other Biogeochemical Cycles, in Climate Change 2013 – The Physical Science Basis (ed. Intergovernmental Panel on Climate Change), Cambridge University Press, 2013, pp. 465–570 Search PubMed.
|
This journal is © The Royal Society of Chemistry 2022 |