DOI:
10.1039/D1NR06195J
(Minireview)
Nanoscale, 2021,
13, 19352-19366
Artificial intelligence-powered microfluidics for nanomedicine and materials synthesis
Received
20th September 2021
, Accepted 29th October 2021
First published on 9th November 2021
Abstract
Artificial intelligence (AI) is an emerging technology with great potential, and its robust calculation and analysis capabilities are unmatched by traditional calculation tools. With the promotion of deep learning and open-source platforms, the threshold of AI has also become lower. Combining artificial intelligence with traditional fields to create new fields of high research and application value has become a trend. AI has been involved in many disciplines, such as medicine, materials, energy, and economics. The development of AI requires the support of many kinds of data, and microfluidic systems can often mine object data on a large scale to support AI. Due to the excellent synergy between the two technologies, excellent research results have emerged in many fields. In this review, we briefly review AI and microfluidics and introduce some applications of their combination, mainly in nanomedicine and material synthesis. Finally, we discuss the development trend of the combination of the two technologies.
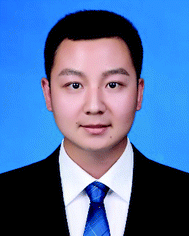 Linbo Liu | Linbo Liu received his Ph.D. in mechanical engineering from Jiangsu Key Laboratory for Design and Manufacture of Micro-Nano Biomedical Instruments, Southeast University, China, in 2021. He has been working as a visiting scholar in the School of Engineering and Applied Sciences and Department of Physics at Harvard University since 2018. His research interests include droplet microfluidics, inertial microfluidics, dielectrophoresis, and molecular diagnostic instruments. |
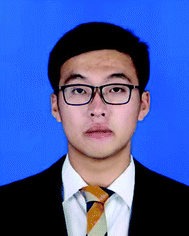 Mingcheng Bi | Mingcheng Bi is currently a postgraduate candidate at the Energy Engineering College at Zhejiang University (ZJU), Hangzhou, China. He completed his Bachelor's degree at Dalian University of Technology (DLUT), Dalian, China in 2020. His current research interests include artificial intelligence and polymer processing engineering, especially microwave foaming materials. |
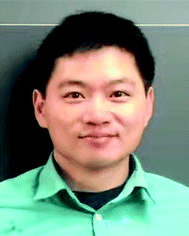 Xingcai Zhang | Dr Xingcai Zhang is a Harvard/MIT Research Fellow with expertise in sustainable nature/bio-derived/inspired materials/technologies for advanced biomed/energy/environment applications. He has published more than 100 peer-reviewed papers in top journals, including Nat. Rev. Mater., Nat. Nanotechnol., Matter, Nat. Commun., Sci. Adv., Proc. Natl. Acad. Sci. U. S. A., Natl. Sci. Rev., J. Am. Chem. Soc., Angew. Chem. Int. Ed., Adv. Mater., Mater. Today, Prog. Mater. Sci., Chem. Soc. Rev., and much more. He has served as a science writer/editor/advisory board member for Nature, Springer, Elsevier, Materials Today, American Chemical Society (ACS), Royal Society of Chemistry, and Wiley. He has been acknowledged by Nature VP for the publication of Nature Outlook Tea. He is a Nature Nano Ambassador and a member of multiple societies. Dr Zhang received multiple awards including the Nature Nano Award. |
1. Introduction
Artificial intelligence (AI) has become a hot topic in recent years, which can be used to research and develop theories, methods, technologies, and application systems for simulating and extending human intelligence. The concept of AI was first proposed by McCarthy et al.1 in 1956, but it failed to show its potential due to algorithm limitation and insufficient data in the early stage. In recent years, with the emergence of a variety of neural network models represented by Convolutional Neural Networks (CNN) and a large number of algorithms,2–11 as well as the development of open-source frameworks such as TensorFlow,12 AI has achieved leapfrog development. It has gained incomparable computing and analytical capabilities of traditional computing tools and rapidly become an emerging technology with great potential. In the past few years, the wave of artificial intelligence has rapidly swept across various industries, significantly impacting them.13–34
The rapid development of AI has brought new influence in many fields, and microfluidic technology is one of the beneficiaries. Microfluidics is a system that can process or manipulate a small number of fluids (10−9 to 10−18 L) in a microscale structure and has found diverse applications.35–37 It can be traced back to the Miniaturized Total Analysis System (μ-TAS) proposed by Manz and Harison et al. in the 1990s. Since the fluid in the microfluidic system is at the micro/nano-level, it produces completely different behaviors from macro-fluids, such as high heat transfer efficiency, large laminar flow, and significant surface effect,38–40 and it can precisely control nanoliter-scale liquids. Therefore, microfluidics has brought a new perspective to many traditional disciplines. In nearly 30 years of history, microfluidics has developed by leaps and bounds, showing great potential in many fields such as biology, new materials, chemistry, and energy,41–48 and has become an interdisciplinary discipline involving engineering, physics, micro-processing, etc. Besides, microfluidic systems have the advantages of high throughput, high integration, high sensitivity, low power consumption, and can produce many data,49–54 including size, shape, structure, composition, etc. Microfluidic systems often cannot mine and process these data well.
However, the large amount of complex data generated by microfluidics is a fertile ground for AI. The core of AI is data support. The rapid acquisition of valuable information from a large amount of data mined by microfluidic systems can provide a good foundation for machine learning and other AI algorithms. The training process of the AI algorithm model directly determines its ability to process data.55–58 Moreover, the AI model trained by the microfluidics can better feedback the microfluidic system, so that it can better mine data and lead the microfluidic system towards automation and intelligence. And the artificial intelligence model can be verified and adjusted. Therefore, these two emerging technologies can be mutually beneficial, creating new research in many fields (Table 1).
Table 1 Application of artificial intelligence-powered microfluidics
Microfluidic technology |
AI algorithm |
Applications |
Advantages |
Ref. |
Microfluidic chip |
Deep convolutional neural network |
Intelligent image-activated cell sorting |
High integration and automation |
53
|
Microfluidic cytometer |
Convolutional neural network and extreme learning machine |
Lensless blood cell counting system |
High resolution, low cost, and high throughput |
68
|
A microfluidic chip and mobile lensless blood cell image acquisition device |
Convolutional neural network |
An entire mobile lensless microfluidic blood image acquisition and analysis system |
Equipment miniaturization and high accuracy |
69
|
3D microfluidic devices |
Convolutional neural networks backward Kalman filtering and multiple hypothesis tracking |
Multicell migration tracking |
Migration tracking and high accuracy |
70
|
Digital microfluidic (DMF) |
Convolutional neural network |
Digital microfluidic Isolation of Single Cells for -Omics |
Unique levels of selectivity, context, and accountability |
71
|
Microfluidic microscopy |
Support vector machine |
Cancer screening |
Cost-effective and label-free |
73
|
Single-cell microfluidic method and quantitative deformability cytometry (q-DC) |
A k-nearest neighbor machine learning algorithm |
Predicting cancer cell invasion |
Classification of cancer cell types |
74
|
A microfluidic device consisted of luer-lock inlet and outlet ports |
Random-forest decision trees |
Risk stratification of cancer patients |
High accuracy and operability |
75
|
A microfluidic blood–brain niche (μBBN) chip |
Neural network, random forest, logistic regression, etc. |
Identify the possibility of tumor cells extravasating into the brain metastatic niche |
Precise |
76
|
2D light scattering microfluidic cytometry |
Deep learning |
Detection of leukemia cells |
Label-free, high efficiency, and high automation |
77
|
A microfluidic device with channels and mazes |
Support vector machine |
Diagnosis of sepsis |
High accuracy |
78
|
PDMS microfluidic channel |
Extreme learning machine |
Microfluidic imaging cytometer |
Continuous high throughput flowing cell recognition and counting |
79
|
Optofluidic time-stretch microscope with microfluidic device |
Support vector machine |
Detection of cellular drug responses |
High throughput and label-free |
82
|
Extreme-throughput, label-free, whole-blood imaging flow cytometer |
A deep convolutional autoencoder |
Ex vivo drug-susceptibility testing of leukemia |
Highly simple, rapid, and cost-effective |
83
|
Microfluidic chip with both physical stability and optical transparency |
Convolutional neural network |
Optofluidic time-stretch quantitative phase imaging |
Highly reliable, high-throughput label-free |
84
|
Vitro microfluidic cancer models |
Custom image analytic algorithms based on machine learning |
Recapitulation of the tumor microenvironment |
Sustain tumor fragments for multiple days, permit real-time, high-resolution imaging of the interaction and provide automated and quantitative assessment of experimental results |
90
|
16-Channel microfluidic reactor |
Black box optimization |
Optimization of quantum dot reaction conditions |
Fast and flexible |
98
|
Integrated silicon microreactor |
Black-box algorithms |
Optimize the yield and the throughput of a Knoevenagel condensation reaction |
|
101
|
Microreactor |
Artificial neural network |
Rapid data analysis in combinatorial nanoparticle syntheses |
Extract condition–property relations rapidly from the combinatorial synthesis data |
102
|
Tubular micro-reactor |
Artificial neural network |
Study the fluid dynamics of VOC oxidation over the nano-catalyst under the different operating conditions |
The model can be employed for the complex simultaneous oxidation of VOCs in the plug reactor without involving in the kinetic mechanism. |
103
|
Microreactor |
Genetic algorithm |
Autonomously driven materials-evolution robotic platform |
Discover complex nano-constructs using desired spectroscopic responses |
104
|
Oscillatory microfluidic platforms |
Bayesian optimization |
Self-driving platform for metal nanoparticle synthesis |
Time- and resource-saving |
105
|
Microfluidic reactors |
Ensemble neural networks and Bayesian optimization |
Self-driven multistep quantum dot synthesis |
Rapid, autonomous and low cost |
107
|
Microfluidic reactors |
Artificial neural network |
Identify relationships between variables affecting drug nanoprecipitation |
|
108
|
Microfluidic devices |
Artificial neural network |
Predict PLGA particle sizes produced by different microfluidic systems either individually or jointly merged |
Rapid and economical |
109
|
Microfluidic high-throughput experimental |
Bayesian optimization with a deep neural network |
Produce silver nanoparticles with the desired absorbance spectrum |
|
110
|
Artificial intelligence and microfluidics have excellent synergy.51 Therefore, in this minireview, we provide a brief overview of how the combination of microfluidic technology and artificial intelligence can help in other fields and mainly introduce the applications in nanomedicine and material synthesis. Finally, we discuss the future development trend of the combination of the two technologies.
2. Applications in nanomedicine
Nanomedicine is an emerging science that uses the technology and principles of nanoscience to treat, detect, and diagnose biological systems.59 Many biological mechanisms in the human body and the pathogenic mechanisms of viruses and bacteria occur on the nanoscale.60 It uses nanoparticles to enter the human body to provide imaging feedback to specific areas, or use high-throughput equipment to quickly detect blood and other samples in vitro to diagnose diseases, and use nanoparticles as drug carriers for disease treatment, which solves many problems that are difficult to be solved by traditional medical technology.61–63 Besides, empowered by AI, nanomedicine is increasingly used in cell identification and classification, disease diagnosis, drug sensitivity testing, disease treatment, etc.
2.1 Cell detection and recognition
A complete blood count (CBC) is one of the most commonly used laboratory tests in medicine. It reflects the health of the human body by counting cells or substances such as red blood cells, white blood cells, and hemoglobin in the blood. Some common diseases can be diagnosed by complete blood count, such as leukemia, inflammation, and scurvy.64–67 The traditional complete blood count method usually uses a high-power microscope for manual counting, which is often time-consuming and labor-intensive, and has a small amount of processing. Although the flow cytometers based on microfluidic technology have high throughput, they are usually large in size and expensive. Recently, a portable and low-cost technology for the timely detection of blood cell counts has been developed. In 2016, Huang et al.68 reported a single-frame super-resolution processing system for lensless blood cell counting based on machine learning (Fig. 1A). They built a miniaturized lensless imaging system by integrating a microfluidic channel on a miniature image sensor. To solve the problem of low throughput of multi-frame super-resolution processing and a large amount of storage space, they developed a microfluidic lensless cell counting system that can achieve high single-cell image quality without the limitation of throughput through super-resolution processing based on extreme learning machine (ELM) and convolutional neural network (CNN). The results show that the cell resolution of CNNSR had been increased by four times, the results are in good agreement with the commercial flow cytometer, and single-cell level analysis could be performed. In 2019, Liao et al.69 built a mobile CNN lensless sensor blood cell collection and analysis system (Fig. 1B). In the system, a pure integer quantization algorithm is proposed, which maintains high analysis accuracy while making the equipment miniaturized. Compared with the traditional floating-point method, the area is reduced by 45% while the calculation accuracy is only reduced by 0.56%. What's more, experimental results show that when the frequency is 100 MHz, the classification speed can reach 17.9 fps. These results show that the ingenious combination of artificial intelligence and lensless microfluidic imaging technology can pave the way for the miniaturization, portability, and real-time detection of complete blood count instruments.
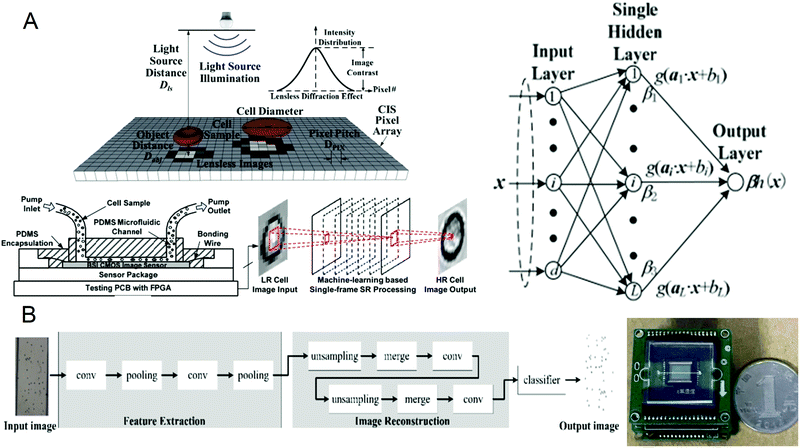 |
| Fig. 1 (A) Schematic diagram of the lens-free cell counting system and model structure of the extreme learning machine. Reproduced from ref. 68 with permission from Molecular Diversity Preservation International, 2016. (B) Cell division network structure in lensless imaging systems and comparison between the microfluidic chip and a coin. Reproduced from ref. 69 with permission from Molecular Diversity Preservation International, 2019. | |
Identifying different cell types and exploring the relationship between cell genes, compositions, structures and morphologies and cell physiology are important in biomedical research. In recent years, more and more technologies have emerged to identify and screen different cells, and conduct research. In 2018, Nao Nitta et al.53 proposed intelligent image-activated cell sorting (IACS) technology, which combined high-throughput cell microscopy, cell focusing, and sorting technology to achieve real-time automated operation of cell data collection, data processing, decision-making and driving. The system has high flexibility and high scalability. When this technology uses a five-layer deep CNN to screen platelets with high components, the high classification purity of aggregation can reach 79.5%, and even single platelets and double platelets can be distinguished. The whole process can be completed in 32 milliseconds (Fig. 2A). Wang et al.70 proposed an automatic image analysis system to vascular endothelial cells’ migration in a three-dimensional microfluidic device. The cell detection algorithm based on CNN obtained by using five-fold cross-validation achieved 87.4% accuracy and 91.2% recall rate in cell candidate detection, and the association accuracy reached 86.4% when a multi-hypothesis Kalman filter was used to correlate detected candidate cells (Fig. 2B). Regarding single-cell detection, Julian Lamanna et al.71 have launched a Digital microfluidic Isolation of Single Cells for -Omics (DISCO) platform, which uses lasers to lyse cells and collects and analyzes the contents of target cells on the microfluidic platform, and then uses artificial intelligence-driven image processing technology to analyze the contents (Fig. 2C). To verify the selectivity of the system, a mixture of human glioblastoma cells and murine melanoma cells transfected with different substances was inoculated and cultured and analyzed on a microfluidic device. The results showed that the positioning rate for the correct species was more than 80% and the positioning rate for the wrong species was less than 8%. These methods can help people better understand the role of different cells and evaluate the capabilities of different components of the cell, and master the cell mechanism.
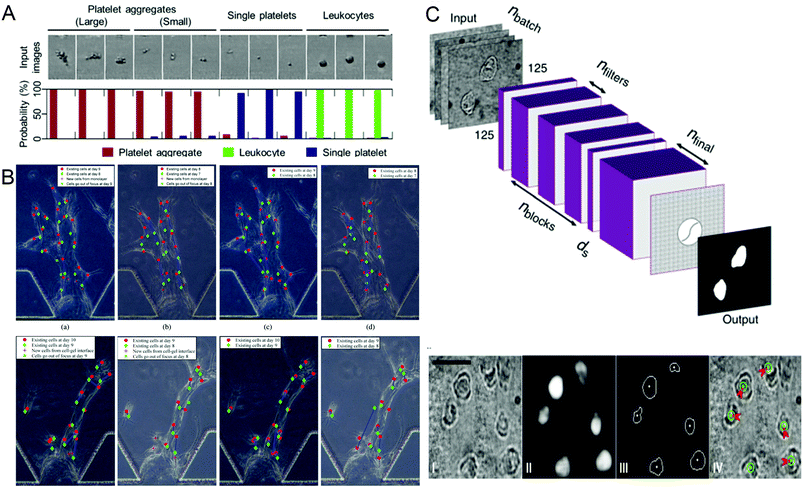 |
| Fig. 2 (A) Probability distribution of representative platelet aggregation, white blood cell, and single platelet images obtained by IACS using CNN. Reproduced from ref. 53 with permission from Cell Press, 2018. (B) Example of cell tracking after one day of migration, where green is the previous day. Reproduced from ref. 70 with permission from Spie-Soc Photo-Optical Instrumentation Engineers, 2018. (C) The structure of the CNN used by the DISCO platform for cell localization and segmentation and examples of artificial intelligence processing images. Reproduced from ref. 71 with permission from Nature Research, 2020. | |
2.2 Diagnosis of disease
Cancer is one of the most life-threatening diseases. According to statistics, there were 18.1 million new cancer cases and 9.6 million cancer deaths in 2018.72 The mortality rate of cancer can be reduced through early detection and treatment. In cancer detection, the cytopathological examination is critical, and the physical characteristics of cells are usually used as biomarkers for cancer diagnosis. But most cancer screenings are time-consuming and expensive. In recent years, many studies have been devoted to solving these problems. In 2016, Veerendra Kalyan Jagannadh et al.73 developed a low-cost and label-free method for microfluidic microscopy to identify cancer cells (Fig. 3A). This technology is based on principal component analysis (PCA) for feature extraction, support vector machine (SVM) for classification, and combined microfluidic microscopy with artificial intelligence. The “malignant cell surveillance system” suitable for cancer screening has been demonstrated for the first time, and the observation accuracy is up to 98.01%. In terms of cancer cell invasion ability to predict, Kendra D. Nyberg et al.74 used quantitative deformable cell count (Q-DC) to measure 6 physical phenotypes (Fig. 3B) at the rate of 100 cells per second, including elastic modulus, maximum strain, cell fluidity and size, transit time, and entry time. And using the k-nearest neighbor machine learning algorithm classified cell lines, the accuracy of cancer cell line classification is nearly doubled compared with single parameter analysis (up to 96%). They used a set of experimental data of human ovarian cancer cells overexpressing tumor suppressor microRNAs to train a multiple linear regression model to generate a physical phenotype model of invasion and successfully validated the model with human cancer cells of breast and ovarian cancer. This allows people to better predict the invasion of cancer cells through the physical phenotype of single cells. By stratifying the risk of cancer patients, appropriate treatment can be given to different degrees of cancer. In 2018, Michael S. Manak et al.75 reported a phenotypic biomarker assay for living primary cells. Unlike conventional formalin fixation histochemical biomarker analysis, this method can measure previously unavailable phenotypic biomarkers for living cells. This technology combines machine vision analysis and random forest decision trees, uses a microfluidic device as a cell measurement environment, and uses dynamic live cell biomarkers to stain prostate cancer and breast cancer tissue samples. The results are highly sensitive and specific. It divided cancer patients into three levels, which successfully provided a method for the clinical application of risk stratification (Fig. 3C). In 2019, C. Ryan Oliver et al.76 built a platform to quantitatively analyze the aggressiveness of cancer cells (Fig. 3D). They used the microfluidic blood–brain niche (μBBN) chip to describe the extravasation of cancer cells, combined with advanced live-cell imaging algorithms and artificial intelligence algorithms such as neural network, naive Bayes, random forest tree, logistic regression, knowledge network, and stochastic gradient descent, and successfully identified cancer cells with brain metastasis phenotypes with an accuracy of up to 0.87. It can be seen that the combination of artificial intelligence and microfluidic technology provides a new, convenient, and cheap method for cancer diagnosis and classification, which is of great significance for humans to fight cancer.
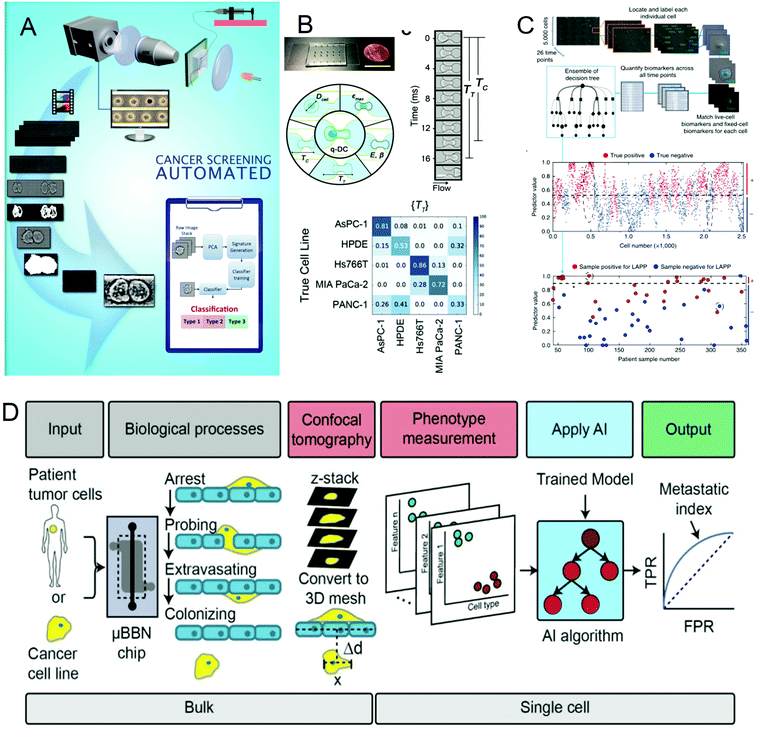 |
| Fig. 3 (A) Label-free cancer cell identification and screening framework. Reproduced from ref. 73 with permission from Springer Heidelberg, 2017. (B) Comparison of the Q-DC microfluidic device with US penny, schematic diagram of the Q-DC physical phenotype, six physics are obtained through microfluidic contraction to denature cells, parameters and the confusion matrix of Q-DC's predictive ability for cell classification. Reproduced from ref. 74 with permission from Oxford University Press, 2018 (C) Artificial intelligence algorithm integrates biomarkers to score and predict cells and patients. Reproduced from ref. 75 with permission from Nature Research, 2018. (D) Flow chart for the identification of the potential of tumor cells to extravasate into the brain metastatic niche. Reproduced from ref. 76 with permission from Royal Society Chemistry, 2019. | |
2.3 Drug-susceptibility testing
Drug sensitivity testing can help people accurately and effectively formulate disease treatment strategies and use drugs for treatment. In the past few years, high-content screening based on multivariate single-cell imaging has been proven effective in drug discovery, which can assess drug-induced phenotypic variation in gene expression, protein localization,80,81 and cytoskeletal structure. However, high-content screening requires fluorescent labels, most of which are analyses of inactivated cells, which will increase the cost and time-consumption of the experiment. These disadvantages hinder large-scale analysis. To overcome these shortcomings, in 2017, Hirofumi Kobayashi et al.82 proposed a label-free method that only uses high-throughput brightfield imaging and machine learning algorithms to evaluate cellular drug responses (Fig. 4A). They used the anticancer drug paclitaxel as a model drug, and MCF-7 as the model cell line to induce cell morphological changes. The cells were collected through an optical flow time stretching microscope at a high throughput of up to 10
000 cells per second bright-field images and researchers could use unlabeled cell images and SVM to identify their morphological changes, the discrimination accuracy rate can reach 92%. These studies have laid the foundation for unlabeled drug screening in pharmaceutical science and industry. In 2018, Hirofumi Kobayashi et al.83 proposed a liquid biopsy method for leukemia drug sensitivity test in vitro, which overcomes the disadvantages of poor clinical applicability and time-consuming and labor-consuming techniques in the past methods (Fig. 4B and C) and uses a high-throughput whole blood imaging flow cytometer (>1 million cells per second) and a deep convolution autoencoder for label-free detection. It can be used to analyze drug-treated whole blood cells within 24 hours. The drug sensitivity of whole blood leukocytes is obtained based on image recognition. In addition, based on the dose-dependent morphological changes, they verified through experiments that the drug sensitivity of the human chronic myeloid leukemia cell line K562 resistant strain is the same as the detection result of the traditional method. This method paves establishing of a drug susceptibility group for all available anticancer drugs for each patient and can improve the survival rate of leukemia patients. In 2020, Wu et al.84 proposed a high-throughput (up to 15
000 cells per second), high-accuracy frequency-shifted optical fluid time-stretch quantitative phase imaging technology, using this technology to obtain a large number of images of white blood cells and cancer cells. And they used more than 30
000 images to train a convolutional neural network (CNN), according to the images to display the intelligent classification of various types of leukemia cells and white blood cells, with an average accuracy of 96.2% (Fig. 4D). Based on this, they evaluated the efficacy of anti-cancer drugs on leukemia cells, and through CNN analysis, the drug-induced differentiation of cells can be identified in image recognition. This technology can monitor and evaluate the efficacy of drug-treated leukemia cells in a label-free manner. Through the combination of microfluidics and artificial intelligence technology, these technologies have successfully provided a basis for selecting disease drugs and brought hope to leukemia patients.
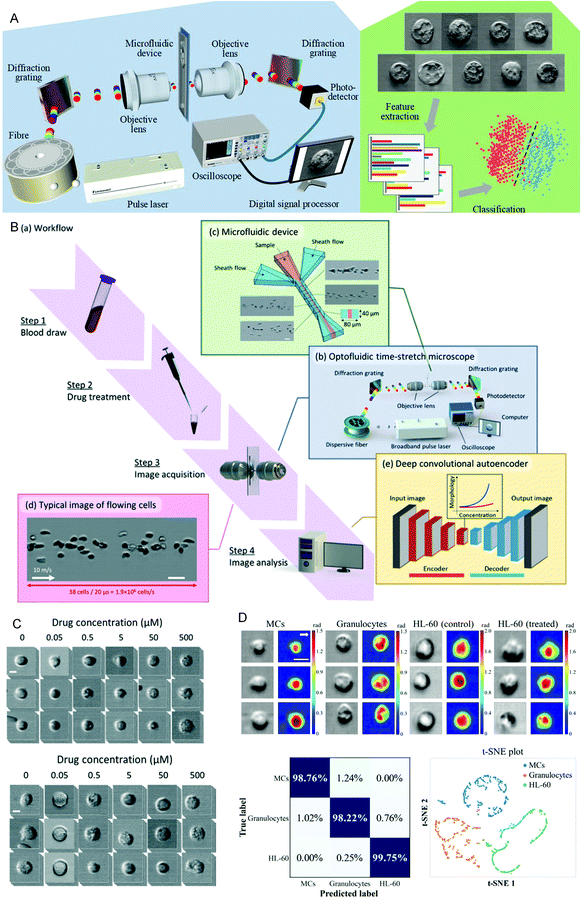 |
| Fig. 4 (A) Schematic diagram of the optical flow time stretching microscope and machine learning-assisted image analysis. Reproduced from ref. 82 with permission from Nature Publishing, 2017. (B) Intelligent whole blood imaging flow cytometer drug sensitivity test process and principle diagram. (C) Comparison of the exported leukocyte susceptibility test images of healthy people and patients with acute lymphoblastic leukemia from an intelligent whole blood imaging flow cytometer. Reproduced from ref. 83 with permission from Royal Society Chemistry, 2019. (D) Intelligent evaluation results of leukemia treatment. Reproduced from ref. 84 with permission from Optical Society America, 2020. | |
2.4 Treatment of diseases
Cancer treatment is limited by the complexity of the microenvironment in which tumor cells are located. The regeneration of the tumor microenvironment is the key to explore the tumorigenesis mechanism, targeted therapy, and immunotherapy and evaluate chemotherapy.85–88 With the emergence of microfluidic technology, the ability to model the tumor microenvironment in vitro has been greatly improved, and the “cancer on a chip” platform has developed rapidly. In 2016, Jose M. Ayuso et al.89 proposed a microfluidic device that can model and study the tumor microenvironment in real-time and is easy to use (Fig. 5A and B). The device can simulate the three-dimensional structure of multicellular spheroids to generate visible, live tumor chips. It is easy to observe cells in different areas in the simulated environment and their response to different drugs in real-time and evaluate the ability of drugs to penetrate several layers of cells to improve the effective rate of drug treatment. In 2018, N. Moore et al.90 combined the microfluidic technology with artificial intelligence and reported a microfluidic model of an in vitro immune tumor dynamic environment for tumor biopsy (Fig. 5C and D). They put live tumor slices (up to 12) in the model to simulate the interaction of tumor cells and tumor-infiltrating lymphocytes (TILs) in real-time and dynamically, and can obtain high-resolution images in real-time. On this basis, they use the machine learning custom image analysis algorithm for the quantitative evaluation of experimental results. They used the system to demonstrate the interaction of mouse tumor cells with anti-PD-1 immune checkpoint inhibitors and to evaluate the response of human tumor cells to immune checkpoint inhibitors. These studies show that the development of in vitro modeling of the tumor microenvironment provides a powerful platform for in vitro experiments of cancer and other diseases, allowing researchers to better develop drugs and treat cancer more effectively.
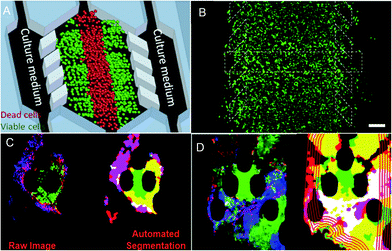 |
| Fig. 5 (A) The principle of “tumor slice” in vivo, in which the red area simulates the area of tumor necrosis. (B) Cells in the microdevice are observed under the microscope (stained). Reproduced from ref. 89 with permission from Nature Publishing Group, 2016. (C) Image analytic mapping of the tumor using automated segmentation technology. (D) Recognition map of the tumor infiltration process. Reproduced from ref. 90 with permission from Royal Society Chemistry, 2018. | |
3. Applications in materials synthesis
Due to their good mechanical and optical properties, nanomaterials have an extensive range of applications and broad development prospects. Traditional preparation methods have limited the synthesis of some nanoparticles due to expensive equipment, low yields, and high requirements for reaction conditions. And it is difficult to produce nanoparticles with more complex structures. With the emergence of microfluidic technology, it has become an excellent synthesis platform for nanoparticles due to its excellent droplet manipulation ability, excellent efficient heat transfer and mass transfer rates. It has been widely used in the synthesis of nanoparticles, such as composite materials, metal particles, nanofibers, and quantum dots,91–100 opening a new direction in nanoparticle synthesis. However, during the synthesis of nanomaterials, many parameters need to be controlled, including temperature, pressure, reagent concentration, flow rate, etc. These parameters are directly related to the performance of the prepared nanoparticles, and it is challenging to adjust these parameters manually, which also limits the development of nanomaterials. In recent years, with the development of artificial intelligence technology, people have begun to combine it with microfluidic technology to synthesize nanomaterials, optimize and predict the synthesis of nanomaterials, and reduce the required cost while preparing high-quality nanoparticles.
3.1 Synthetic optimization
The reaction parameters during material synthesis can determine the performance of the product. Finding the optimal conditions for material synthesis has always been one of the key research contents in chemical synthesis. Although microfluidic technology has greatly optimized material synthesis, it is still challenging to manually adjust a large number of reaction parameters and make them optimal. As early as in 2010, Jonathan P. McMullen et al.101 began to consider using artificial intelligence to adjust response conditions and obtain optimal results. They proposed an automated microfluidic system for the online optimization of chemical synthesis and carried out 46 oxidation experiments of benzoic acid using benzoyl formaldehyde. The simplex method was used for multi-parameter optimization, and the optimal reaction conditions were successfully determined when the yield of benzoyl formaldehyde was 80%, which provided a way for optimizing chemical synthesis (Fig. 6A). In 2012, Yuuichi Orimoto et al.102 trained 1600 different neural networks to construct an integrated neural network to determine the relationship between reaction conditions and the properties of produced nanomaterials, and successfully extracted the basic model of the conditional-attribute relationship of CdSe nanoparticles. The key parameters of control synthesis were extracted by data interpolation and sensitivity analysis of the neural network, which guided the optimization of the properties of nanoparticles. In 2020, Amin Sokhansanj et al.103 developed an intelligent hybrid model (Fig. 6B) using computational fluid dynamics analysis and artificial neural networks to simulate the simultaneous catalytic oxidation of benzene and toluene. The result showed that at 350 °C, using nano-supported cobalt oxide for catalysis, the maximum conversion rates of benzene and toluene are 89.74% and 82.37%, which are consistent with the experimental results. This model can not only avoid the more complicated kinetic mechanism in the reaction process, solve the reaction rate more accurately, but also can explore the influence of reaction conditions on the catalytic oxidation reaction. This work provides a useful tool for studying the hydrodynamics of VOC oxidation on the nano-catalyst under different operating conditions. In the same year, Daniel Salley et al.104 proposed an autonomously driven material evolution robot platform (Fig. 6C). They used genetic algorithms to synthesize designated nanoparticles. After analyzing the synthesized nanoparticles, they optimized the synthesis again. This method can not only synthesize known structures can also be used to discover more complex nanostructures. Experiments have proved that the system can produce spherical nanoparticles and rod-shaped particles. Finally, octahedral nanoparticles are synthesized by optimizing rod-shaped particles as seeds. In 2021, Tao et al.105 reported a platform for the automatic synthesis of nanoparticles.
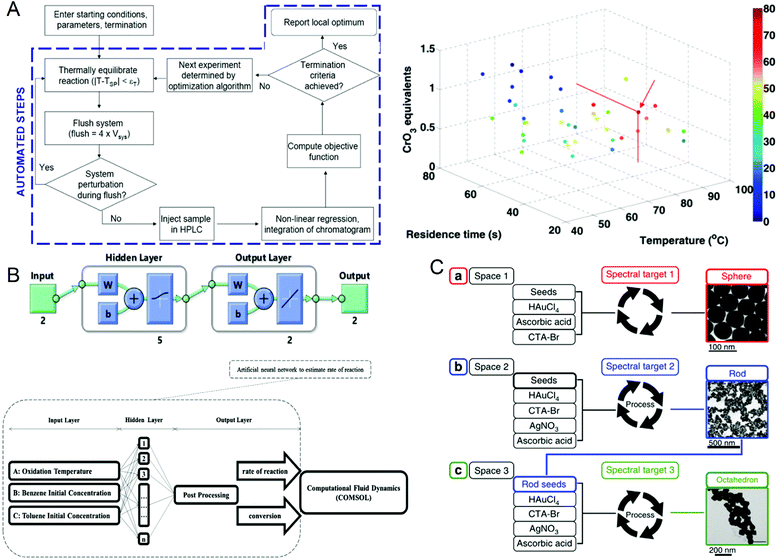 |
| Fig. 6 (A) Operation flow chart of the automated microfluidic system and the best reaction conditions for maximum benzaldehyde yield obtained by the simplex method. Reproduced from ref. 101 with permission from American Chemistry Society, 2010. (B) CFD-ANN hybrid modeling architecture for studying the simultaneous catalytic oxidation of benzene and toluene in the air on nano-catalysts. Reproduced from ref. 103 with permission from Elsevier, 2020. (C) Intelligent evolution of gold nanoparticles. Reproduced from ref. 104 with permission from Nature Publishing Group, 2020. | |
They combined the oscillating microfluidic platform with machine learning for the first time and used the oscillating microfluidic platform to synthesize nanoparticles of different dimensions. They used Bayesian optimization to analyze the nanoparticles and only a few experiments were performed to obtain the optimal nanoparticle synthesis formula. Furthermore, the relationship between the reaction conditions and the properties of the nanoparticles was also obtained through the ML analysis. It provides support for the automatic synthesis technology of nanoparticles. These studies provide a more convenient method for optimizing the conditions of materials synthesis, which can greatly reduce costs and increase the yield of target products. Furthermore, it can provide a theory for people to discover new structures.
In recent years, quantum dots have attracted attention due to their unique optical and electrical properties, and are very popular in fields such as solar cells, displays, and cell imaging. However, the synthesis of quantum dots involves a series of processes such as the nucleation and growth of crystals, which have a complex reaction mechanism. This leads to a large dispersion of the performance of the produced quantum dots, which limits their application to a certain extent. Recently, more and more scientists have researched this. In 2019, Oleksandr Voznyy et al.106 optimized the synthesis of monodisperse PbS nanoparticles (Fig. 7A), using Bayesian optimization implemented by neural networks to establish a continuous numerical model of the reaction parameter space, and using its optimized synthesis parameter results to synthesize PbS nanoparticles, whose half-width at half-maximum are 55 meV and 24 meV at 950 nm and 1500 nm (the best values previously announced are 75 and 26 meV). In 2020, Wang et al.98 used feedback control for the first time to synthesize high-throughput nanomaterials in a continuous flow parallel reactor. They developed a 16-channel microfluidic reactor to produce light-emitting quantum dots, which solved the problem of low throughput required for industrial production. The reactor is based on the real-time monitoring of the photoluminescence characteristics of quantum dots and uses a black-box algorithm for feedback control to self-optimize product quality. It is used to produce CsPbBr3 quantum dots with a yield of 1L h−1. With the help of artificial intelligence, these studies have a more thorough understanding of the nucleation and growth mechanism of quantum dots, and can better control and optimize reaction conditions to obtain high-quality quantum dots quickly and in large quantities (Fig. 7B). In 2021, to find the optimal synthesis formula for inorganic lead halide perovskite quantum dots, Kameel Abdel-Latif et al.107 developed an autonomous microfluidic synthesis strategy using integrated neural networks and Bayesian optimization. The process for determining the optimal synthesis route to the continuous manufacturing of ideal quantum dots is realized, and the tedious steps in the middle are omitted, saving a lot of time.
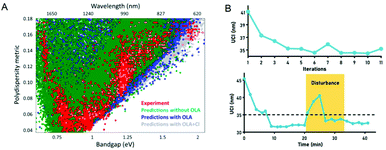 |
| Fig. 7 (A) The effects of oleylamine and chloride on PbS synthesis predicted by the machine learning model are the same as experimental results. Reproduced from ref. 106 with permission from American Chemical Society, 2019. (B) Self-optimization and anti-interference performance of microfluidic reactor for nanoparticle synthesis. Reproduced from ref. 98 with permission from Royal Society Chemistry, 2020. | |
3.2 Synthetic prediction
It is essential to accurately predict the properties of synthetic materials. During the synthesis of materials, the performance of the products may vary greatly due to the different reaction conditions. However, in microfluidic synthesis, there are many reaction conditions involved, and a small change may lead to a large change in the properties of the product. Therefore, a lot of research is devoted to this. In 2009, Hany S. M. Ali et al.108 trained a neural network using 53 samples prepared under different processing conditions and created a model to identify the relationship between variables that affect drug precipitation. They used this model to input the reaction conditions such as the angle and inner diameter of the microreactor during prednisolone nanoprecipitation, and successfully predicted the resulting particle size, which was consistent with the experimental data (Fig. 8A). In 2020, Safa A. Damiati et al.109 developed an artificial neural network prediction model for droplet and particle size generated by three different microfluidic systems to control the size uniformity of synthesized PLGA particles (Fig. 8B), which can predict the size of PLGA particles and quickly and effectively produce PLGA particles with adjustable size. To synthesize nanomaterials with specific properties, Flore Mekki-Berrada et al.110 proposed a two-step framework for a machine learning-driven high-throughput microfluidic platform in 2020 (Fig. 8C). They combined Bayesian optimization of the Gaussian process with a deep neural network. After sufficient data training, it can be used to predict the reaction synthesis of accessible color palettes and successfully optimize the synthesis of nanomaterials. In 2020, Jonathan A. Fine et al.111 trained a neural network to use a combination of Fourier transform infrared and mass spectroscopy data to predict the functional groups of molecules. After experimental verification, the neural network model can predict and recognize compound mixtures containing different functional groups (Fig. 8D). Artificial intelligence not only helps to understand the relationship between reaction conditions and product properties but also provides a basis for optimizing the properties of target products and quickly synthesizing materials with specific properties in large quantities.
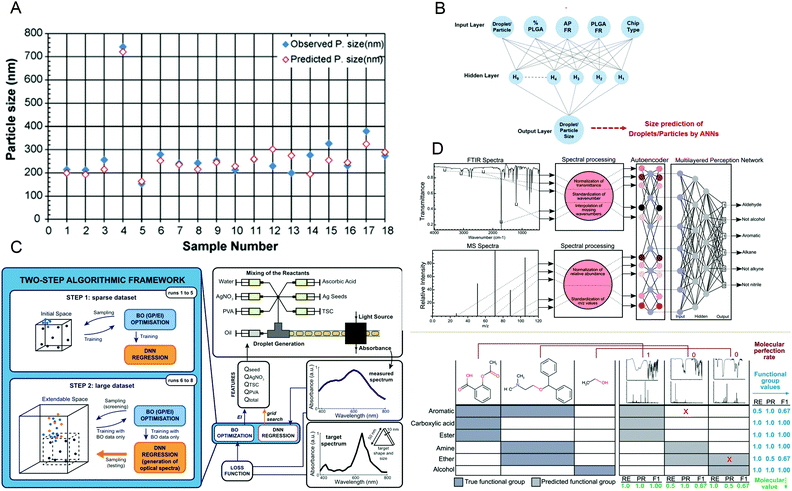 |
| Fig. 8 (A) The predicted particle size obtained by the artificial neural network is almost the same as the observed size. Reproduced from ref. 108 with permission from Elsevier, 2009. (B) The schematic diagram of the artificial neural network is used to predict the size of PLGA particles. Reproduced from ref. 109 with permission from Nature Research, 2020. (C) The two-step algorithm framework of the high-throughput microfluidic platform. Reproduced from ref. 110 with permission from Nature Research, 2021. (D) Algorithm schematic diagram and prediction examples for functional group prediction classification. Reproduced from ref. 111 with permission from Royal Society of Chemistry, 2020. | |
4. Summary and outlook
With the development of AI, more and more microfluidics scholars have begun to devote themselves to the study of AI, aiming to create new ideas for their research fields. The advantages of the two technologies complement each other as if they are a natural pair. Although microfluidic systems have advantages of small scale and high throughput, they often produce a large amount of complex data which are difficult to be processed manually, while artificial intelligence technology is efficient and has powerful computing and analysis capabilities, but needs a large amount of data to train the model. Their characteristics determine that they will inevitably be combined to bring a new and rapidly developing direction to nanomedicine and material synthesis. In the field of nanomedicine, these two technologies are helping medical workers gain a deeper understanding of human cells and their disease-causing mechanisms. It has made some traditional detection instruments intelligent and miniaturized112 and has made great contributions to disease diagnosis, drug development, and disease treatment.113–117,121,122 In materials synthesis, microfluidic technology has become an excellent material synthesis platform with the characteristics of high throughput, efficient mass transfer, excellent heat transfer rate, and precise liquid manipulation. With the addition of artificial intelligence, we have a deeper understanding of the reaction process, successfully optimized and predicted the synthesis results, and obtained products with better performance and higher yield.114,118–120
However, reviewing existing research, it is not difficult to find that there are still shortcomings. First of all, most of the artificial intelligence currently used is supervised learning, which requires manual use of a large amount of data to train the model, which will consume a lot of time and labor and it may be more convenient to use unsupervised learning. Secondly, the general applicability of existing research is poor. In the application of disease diagnosis, most of them are for the identification and sorting of cancer cells, while less attention is paid to other diseases.
In the future, common diseases that are difficult to cure, such as AIDS, diabetes, and COVID-19, can be studied. In the field of materials synthesis, most of the current programs are only for the synthesis of a certain material, and a public program has yet to be developed. In short, as emerging technologies, artificial intelligence and microfluidics have achieved satisfactory results, and as we further study them, they will definitely collide with more intense novel ideas.
Conflicts of interest
There are no conflicts to declare.
Notes and references
- J. Mccarthy, M. L. Minsky and C. E. Shannon, AI Mag., 2006, 27, 12–14 Search PubMed.
- G. E. Hinton, S. Osindero and Y. Teh, Neural Comput., 2006, 18, 1527–1554 CrossRef PubMed.
-
B. E. Boser, I. M. Guyon and V. N. Vapnik., Proceedings of the fifth annual workshop on Computational learning theory, 1992, pp. 144–152.
- M. W. Fagerland, Stata J., 2014, 14, 947–964 CrossRef.
-
L. Breiman, Arcing classifiers, 1996 Search PubMed.
- W. Y. Loh, Wiley Interdiscip. Rev. Data Min. Knowl. Discov., 2011, 1, 14–23 CrossRef.
- D. E. Rumelhart, G. E. Hinton and R. J. Williams, Nature, 1986, 323, 533–536 CrossRef.
-
D. S. Broomhead and D. Lowe, Radial basis functions, multi-variable functional interpolation and adaptive networks, Royal Signals and Radar Establishment Malvern, United Kingdom, 1988 Search PubMed.
- K. Fukushima, Biol. Cybern., 1980, 36, 193–202 CrossRef CAS PubMed.
- C. Cortes and V. Vapnik, Mach. Learn., 1995, 20, 273–297 Search PubMed.
-
V. Vapnik, The nature of statistical learning theory, Springer science & business media, 2013 Search PubMed.
- L. Rampasek and A. Goldenberg, Cell Syst., 2016, 2, 12–14 CrossRef CAS PubMed.
- X. Chen, H. Chen, Z. Chen, J. Gong and C. Y. Chen, J. Mater. Chem. B, 2020, 8, 2063–2081 RSC.
- B. Huang, Z. Li and J. Li, Nanoscale, 2018, 10, 21320–21326 RSC.
- A. Thakkar, S. Johansson, K. Jorner, D. Buttar, J. Reymond and O. Engkvist, React. Chem. Eng., 2021, 6, 27–51 RSC.
- J. C. Caicedo, S. Cooper, F. Heigwer, S. Warchal, P. Qiu, C. Molnar, A. S. Vasilevich, J. D. Barry, H. S. Bansal, O. Kraus, M. Wawer, L. Paavolainen, M. D. Herrmann, M. Rohban, J. Hung, H. Hennig, J. Concannon, I. Smith, P. A. Clemons, S. Singh, P. Rees, P. Horvath, R. G. Linington and A. E. Carpenter, Nat. Methods, 2017, 14, 849–863 CrossRef CAS PubMed.
- M. Xiao, H. Yang, Q. Xiao, H. Zhang and X. Chen, Nanoscale, 2019, 11, 19179–19189 RSC.
- C. Zhai, T. Li, H. Shi and J. Yeo, J. Mater. Chem. B, 2020, 8, 6562–6587 RSC.
- J. Bostrom, D. G. Brown, R. J. Young and G. M. Keseru, Nat. Rev. Drug Discovery, 2018, 17, 709–727 CrossRef PubMed.
- Y. D. Hezaveh, L. P. Levasseur and P. J. Marshall, Nature, 2017, 548, 555–557 CrossRef CAS PubMed.
- C. Lei, H. Kobayashi, Y. Wu, M. Li, A. Isozaki, A. Yasumoto, H. Mikami, T. Ito, N. Nitta, T. Sugimura, M. Yamada, Y. Yatomi, D. Di Carlo, Y. Ozeki and K. Goda, Nat. Protoc., 2018, 13, 1603–1631 CrossRef CAS PubMed.
- G. Wetzstein, A. Ozcan, S. Gigan, S. Fan, D. Englund, M. Soljačić, C. Denz, D. A. B. Miller and D. Psaltis, Nature, 2020, 588, 39–47 CrossRef CAS PubMed.
- M. Liu, P. Karuso, Y. Feng, E. Kellenberger, F. Liu, C. Wang and R. J. Quinn, MedChemComm, 2019, 10, 1667–1677 RSC.
- X. Zou, J. Pan, Z. Sun, B. Wang, Z. Jin, G. Xu and F. Yan, Energy Environ. Sci., 2021, 3965–3975 RSC.
- H. Yang, Z. Zhang, J. Zhang and X. C. Zeng, Nanoscale, 2018, 1, 1992–1999 Search PubMed.
- M. Zhang, J. Li, L. Kang, N. Zhang, C. Huang, Y. He, M. Hu, X. Zhou and J. Zhang, Nanoscale, 2020, 12, 3988–3996 RSC.
- S. Wang, Z. Shen, Z. Shen, Y. Dong, Y. Li, Y. Cao, Y. Zhang, S. Guo, J. Shuai, Y. Yang, C. Lin, X. Chen, X. Zhang and Q. Huang, Nano Today, 2021, 38, 101152 CrossRef CAS.
- Z. Tang, L. Zhao, G. Chen and C. Y. Chen, RSC Adv., 2021, 11, 6423–6446 RSC.
- J. He, C. He, C. Zheng, Q. Wang and J. Ye, Nanoscale, 2019, 11, 17444–17459 RSC.
- H. S. Stein, J. M. Gregoire and P. C. U. S. California Institute Of Technology Caltech, Chem. Sci., 2019, 1, 964–9649 Search PubMed.
- P. L. Gentili, RSC Adv., 2013, 3, 25523 RSC.
- G. K. Anumanchipalli, J. Chartier and E. F. Chang, Nature, 2019, 568, 493–498 CrossRef CAS PubMed.
- P. W. Hatfield, J. A. Gaffney, G. J. Anderson, S. Ali, L. Antonelli, S. D. P. Başeğmez, J. Citrin, M. Fajardo, P. Knapp, B. Kettle, B. Kustowski, M. J. Macdonald, D. Mariscal, M. E. Martin, T. Nagayama, C. A. J. Palmer, J. L. Peterson, S. Rose, J. J. Ruby, C. Shneider, M. J. V. Streeter, W. Trickey and B. Williams, Nature, 2021, 593, 351–361 CrossRef CAS PubMed.
- O. Inderwildi, C. Zhang, X. Wang and M. Kraft, Energy Environ. Sci., 2020, 13, 744–771 RSC.
- Y. Wang, L. Lu, G. Zheng and X. Zhang, ACS Nano, 2020, 14, 9861–9872 CrossRef CAS PubMed.
- G. M. Whitesides, Nature, 2006, 442, 368–373 CrossRef CAS PubMed.
- Z. Tang, N. Kong, X. Zhang, Y. Liu, P. Hu, S. Mou, P. Liljeström, J. Shi, W. Tan, J. S. Kim, Y. Cao, R. Langer, K. W. Leong, O. C. Farokhzad and W. Tao, Nat. Rev. Mater., 2020, 5, 847–860 CrossRef CAS PubMed.
- P. N. Nge, C. I. Rogers and A. T. Woolley, Chem. Rev., 2013, 113, 2550–2583 CrossRef CAS PubMed.
- J. Atencia and D. J. Beebe, Nature, 2005, 437, 648–655 CrossRef CAS PubMed.
- A. A. Darhuber and S. M. Troian, Annu. Rev. Fluid Mech., 2005, 37, 425–455 CrossRef.
- M. Chowdhury, W. Zheng, S. Kumari, J. Heyman, X. Zhang, P. Dey, D. Weitz and R. Haag, Nat. Commun., 2019, 10, 4546 CrossRef PubMed.
- Z. Li, X. Zhang, J. Ouyang, D. Chu, F. Han, L. Shi, R. Liu, Z. Guo, G. X. Gu, W. Tao, L. Jin and J. Li, Bioact. Mater., 2021, 6, 4053–4064 CrossRef CAS PubMed.
- S. Han, Q. Zhang, X. Zhang, X. Liu, L. Lu, J. Wei, Y. Li, Y. Wang and G. Zheng, Biosens. Bioelectron., 2019, 143, 111957 CrossRef PubMed.
- R. Yang, Z. Gong, X. Zhang and L. Que, J. Nanosci. Nanotechnol., 2019, 19, 7591–7595 CrossRef CAS PubMed.
- Z. Li, D. Chu, Y. Gao, L. Jin, X. Zhang, W. Cui and J. Li, Mater. Today Adv., 2019, 3, 100014 CrossRef.
- Q. Zhang, X. Zhang, X. Zhang, L. Jiang, J. Yin, P. Zhang, S. Han, Y. Wang and G. Zheng, Mar. Pollut. Bull., 2019, 144, 20–27 CrossRef CAS PubMed.
- Z. Gong, S. Karandikar, X. Zhang, V. Kotipalli, Y. Lvov and L. Que, IEEE Sensors, 2010, 10, 29–32 Search PubMed.
- G. Zheng, Q. Gao, Y. Jiang, L. Lu, J. Li, X. Zhang, H. Zhao, P. Fan, Y. Cui, F. Gu and Y. Wang, Anal. Chem., 2021, 93, 9728–9736 CrossRef CAS PubMed.
- E. Brouzes, M. Medkova, N. Savenelli, D. Marran, M. Twardowski, J. B. Hutchison, J. M. Rothberg, D. R. Link, N. Perrimon and M. L. Samuels, Proc. Natl. Acad. Sci. U. S. A., 2009, 106, 14195–14200 CrossRef CAS PubMed.
- J. J. Agresti, E. Antipov, A. R. Abate, K. Ahn, A. C. Rowat, J. C. Baret, M. Marquez, A. M. Klibanov, A. D. Griffiths and D. A. Weitz, Proc. Natl. Acad. Sci. U. S. A., 2010, 107, 4004–4009 CrossRef CAS PubMed.
- L. Liu, N. Xiang, Z. Ni, X. Huang, J. Zheng, Y. Wang and X. Zhang, BioTechniques, 2020, 68, 114–116 CrossRef CAS PubMed.
- A. Isozaki, J. Harmon, Y. Zhou, S. Li, Y. Nakagawa, M. Hayashi, H. Mikami, C. Lei and K. Goda, Lab Chip, 2020, 20, 3074–3090 RSC.
- N. Nitta, T. Sugimura, A. Isozaki, H. Mikami, K. Hiraki, S. Sakuma, T. Iino, F. Arai, T. Endo, Y. Fujiwaki, H. Fukuzawa, M. Hase, T. Hayakawa, K. Hiramatsu, Y. Hoshino, M. Inaba, T. Ito, H. Karakawa, Y. Kasai, K. Koizumi, S. Lee, C. Lei, M. Li, T. Maeno, S. Matsusaka, D. Murakami, A. Nakagawa, Y. Oguchi, M. Oikawa, T. Ota, K. Shiba, H. Shintaku, Y. Shirasaki, K. Suga, Y. Suzuki, N. Suzuki, Y. Tanaka, H. Tezuka, C. Toyokawa, Y. Yalikun, M. Yamada, M. Yamagishi, T. Yamano, A. Yasumoto, Y. Yatomi, M. Yazawa, D. Di Carlo, Y. Hosokawa, S. Uemura, Y. Ozeki and K. Goda, Cell, 2018, 175, 266–276 CrossRef CAS PubMed.
- A. F. Chrimes, K. Khoshmanesh, P. R. Stoddart, A. Mitchell and K. Kalantar-Zadeh, Chem. Soc. Rev., 2013, 42, 588–596 RSC.
- M. Uddin, Y. Wang and M. Woodbury-Smith, NPJ Digit. Med., 2019, 2, 112 CrossRef PubMed.
- S. Y. Wang, S. Pershing and A. Y. Lee, Curr. Opin. Ophthalmol., 2020, 31, 318–323 CrossRef PubMed.
- N. L. Bragazzi, H. Dai, G. Damiani, M. Behzadifar, M. Martini and J. Wu, Int. J. Environ. Res. Public Health, 2020, 17, 3176 CrossRef CAS PubMed.
- A. F. de Almeida, R. Moreira and T. Rodrigues, Nat. Rev. Chem., 2019, 3, 589–604 CrossRef.
- S. M. Moghimi, A. C. Hunter and J. C. Murray, FASEB J., 2004, 19, 311–330 CrossRef PubMed.
- E. H. Chang, J. B. Harford, M. A. W. Eaton, P. M. Boisseau, A. Dube, R. Hayeshi, H. Swai and D. S. Lee, Biochem. Biophys. Res. Commun., 2015, 468, 511–517 CrossRef CAS PubMed.
- B. Pelaz, C. Alexiou, R. A. Alvarez-Puebla, F. Alves, A. M. Andrews, S. Ashraf, L. P. Balogh, L. Ballerini, A. Bestetti, C. Brendel, S. Bosi, M. Carril, W. C. W. Chan, C. Chen, X. Chen, X. Chen, Z. Cheng, D. Cui, J. Du, C. Dullin, A. Escudero, N. Feliu, M. Gao, M. George, Y. Gogotsi, A. Grünweller, Z. Gu, N. J. Halas, N. Hampp, R. K. Hartmann, M. C. Hersam, P. Hunziker, J. Jian, X. Jiang, P. Jungebluth, P. Kadhiresan, K. Kataoka, A. Khademhosseini, J. Kopeček, N. A. Kotov, H. F. Krug, D. S. Lee, C. Lehr, K. W. Leong, X. Liang, M. L. Lim, L. M. Liz-Marzán, X. Ma, P. Macchiarini, H. Meng, H. Möhwald, P. Mulvaney, A. E. Nel, S. Nie, P. Nordlander, T. Okano, J. Oliveira, T. H. Park, R. M. Penner, M. Prato, V. Puntes, V. M. Rotello, A. Samarakoon, R. E. Schaak, Y. Shen, S. Sjöqvist, A. G. Skirtach, M. G. Soliman, M. M. Stevens, H. Sung, B. Z. Tang, R. Tietze, B. N. Udugama, J. S. Vanepps, T. Weil, P. S. Weiss, I. Willner, Y. Wu, L. Yang, Z. Yue, Q. Zhang, Q. Zhang, X. Zhang, Y. Zhao, X. Zhou and W. J. Parak, ACS Nano, 2017, 11, 2313–2381 CrossRef CAS PubMed.
- X. Ji, L. Ge, C. Liu, Z. Tang, Y. Xiao, W. Chen, Z. Lei, W. Gao, S. Blake, D. De, B. Shi, X. Zeng, N. Kong, X. Zhang and W. Tao, Nat. Commun., 2021, 12, 1124 CrossRef CAS PubMed.
- D. Gao, X. Guo, X. Zhang, S. Chen, Y. Wang, T. Chen, G. Huang, Y. Gao, Z. Tian and Z. Yang, Mater. Today Bio, 2020, 5, 100035 CrossRef CAS PubMed.
- M. Buttarello and M. Plebani, Am. J. Clin. Pathol., 2008, 130, 104–116 CrossRef PubMed.
- L. A. Bertschi, Am. J. Nurs., 2021, 121, 38–45 CrossRef PubMed.
- L. R. Dixon, J. Perinat. Neonatal. Nurs., 1997, 11, 1–18 CrossRef CAS PubMed.
- C. P. Hornik, D. K. Benjamin, K. C. Becker, D. K. Benjamin, J. Li, R. H. Clark, M. Cohen-Wolkowiez and P. B. Smith, Pediatr. Infect. Dis. J., 2012, 31, 803–807 CrossRef PubMed.
- X. Huang, Y. Jiang, X. Liu, H. Xu, Z. Han, H. Rong, H. Yang, M. Yan and H. Yu, Sensors, 2016, 16, 1836 CrossRef PubMed.
- Y. Liao, N. Yu, D. Tian, S. Li and Z. Li, Sensors, 2019, 19, 5103 CrossRef PubMed.
- M. Wang, L. S. Ong, J. Dauwels and H. H. Asada, J. Med. Imaging, 2018, 5, 1 Search PubMed.
- J. Lamanna, E. Y. Scott, H. S. Edwards, M. D. Chamberlain, M. D. M. Dryden, J. Peng, B. Mair, A. Lee, C. Chan, A. A. Sklavounos, A. Heffernan, F. Abbas, C. Lam, M. E. Olson, J. Moffat and A. R. Wheeler, Nat. Commun., 2020, 11, 5632 CrossRef CAS PubMed.
- F. Bray, J. Ferlay, I. Soerjomataram, R. L. Siegel, L. A. Torre and A. Jemal, CA-Cancer J. Clin., 2020, 70, 313 Search PubMed.
- V. K. Jagannadh, G. Gopakumar, G. R. K. Sai Subrahmanyam and S. S. Gorthi, Med. Biol. Eng. Comput., 2017, 55, 711–718 CrossRef PubMed.
- K. D. Nyberg, S. L. Bruce, A. V. Nguyen, C. K. Chan, N. K. Gill, T. Kim, E. K. Sloan and A. C. Rowat, Integr. Biol., 2018, 10, 218–231 CrossRef CAS PubMed.
- M. S. Manak, J. S. Varsanik, B. J. Hogan, M. J. Whitfield, W. R. Su, N. Joshi, N. Steinke, A. Min, D. Berger, R. J. Saphirstein, G. Dixit, T. Meyyappan, H. Chu, K. B. Knopf, D. M. Albala, G. R. Sant and A. C. Chander, Nat. Biomed. Eng., 2018, 2, 761–772 CrossRef CAS PubMed.
- C. R. Oliver, M. A. Altemus, T. M. Westerhof, H. Cheriyan, X. Cheng, M. Dziubinski, Z. Wu, J. Yates, A. Morikawa, J. Heth, M. G. Castro, B. M. Leung, S. Takayama and S. D. Merajver, Lab Chip, 2019, 19, 1162–1173 RSC.
-
J. Sun, L. Wang, Q. Liu and X. Su, Optics in Health Care and Biomedical Optics IX, 2020, 11190 Search PubMed.
- F. Ellett, J. Jorgensen, A. L. Marand, Y. M. Liu, M. M. Martinez, V. Sein, K. L. Butler, J. Lee and D. Irimia, Nat. Biomed. Eng., 2018, 2, 207–214 CrossRef CAS PubMed.
- X. Huang, J. Guo, X. Wang, M. Yan, Y. Kang and H. Yu, PLoS One, 2014, 9, e104539 CrossRef PubMed.
- S. Heynen-Genel, L. Pache, S. K. Chanda and J. Rosen, Expert Opin. Drug Discovery, 2012, 7, 955–968 CrossRef CAS PubMed.
- M. M. Usaj, E. B. Styles, A. J. Verster, H. Friesen, C. Boone and B. J. Andrews, Trends Cell Biol., 2016, 26, 598–611 CrossRef PubMed.
- H. Kobayashi, C. Lei, Y. Wu, A. Mao, Y. Jiang, B. Guo, Y. Ozeki and K. Goda, Sci. Rep., 2017, 7, 955–968 CrossRef PubMed.
- H. Kobayashi, C. Lei, Y. Wu, C. Huang, A. Yasumoto, M. Jona, W. Li, Y. Wu, Y. Yalikun, Y. Jiang, B. Guo, C. Sun, Y. Tanaka, M. Yamada, Y. Yatomi and K. Goda, Lab Chip, 2019, 19, 2688–2698 RSC.
- Y. Wu, Y. Zhou, C. Huang, H. Kobayashi, S. Yan, Y. Ozeki, Y. Wu, C. Sun, A. Yasumoto, Y. Yatomi, C. Lei and K. Goda, Opt. Express, 2020, 28, 519 CrossRef CAS PubMed.
- D. Gao, T. Chen, Y. Han, S. Chen, Y. Wang, X. Guo, H. Wang, X. Chen, M. Guo, Y. S. Zhang, G. Hong, X. Zhang, Z. Tian and Z. Yang, Nano-Micro Lett., 2021, 13, 99 CrossRef CAS PubMed.
- Z. Yang, D. Gao, X. Guo, L. Jin, J. Zhang, Y. Wang, S. Chen, X. Zheng, L. Zeng, M. Guo, X. Zhang and Z. Tian, ACS Nano, 2020, 14, 17442–17457 CrossRef CAS PubMed.
- Y. Wang, D. Gao, Y. Liu, X. Guo, S. Chen, L. Zeng, J. Ma, X. Zhang, Z. Tian and Z. Yang, Bioact. Mater., 2020, 6, 1513–1527 CrossRef PubMed.
- C. Liu, S. Sun, Q. Feng, G. Wu, Y. Wu, N. Kong, Z. Yu, J. Yao, X. Zhang, W. Chen, Z. Tang, Y. Xiao, X. Huang, A. Lv, C. Yao, H. Cheng, A. Wu, T. Xie and W. Tao, Adv. Mater., 2021, 33, 2102054 CrossRef CAS PubMed.
- J. M. Ayuso, M. Virumbrales-Muñoz, A. Lacueva, P. M. Lanuza, E. Checa-Chavarria, P. Botella, E. Fernández, M. Doblare, S. J. Allison, R. M. Phillips, J. Pardo, L. J. Fernandez and I. Ochoa, Sci. Rep., 2016, 6, 36086 CrossRef CAS PubMed.
- N. Moore, D. Doty, M. Zietstorff, I. Kariv, L. Y. Moy, A. Gimbel, J. R. Chevillet, N. Lowry, J. Santos, V. Mott, L. Kratchman, T. Lau, G. Addona, H. Chen and J. T. Borenstein, Lab Chip, 2018, 18, 1844–1858 RSC.
- M. S. Chowdhury, X. Zhang, L. Amini, P. Dey, A. K. Singh, A. Faghani, M. Schmueck-Henneresse and R. Haag, Nano-Micro Lett., 2021, 13, 147 CrossRef CAS PubMed.
- X. Chen, Y. Chen, L. Zou, X. Zhang, Y. Dong, J. Tang, D. J. Mcclements and W. Liu, J. Agric. Food Chem., 2019, 67, 6574–6584 CrossRef CAS PubMed.
- A. R. Kirmani, J. M. Luther, M. Abolhasani and A. Amassian, ACS Energy Lett., 2020, 5, 3069–3100 CrossRef CAS.
- J. Puigmartí-Luis, M. Rubio-Martínez, U. Hartfelder, I. Imaz, D. Maspoch and P. S. Dittrich, J. Am. Chem. Soc., 2011, 133, 4216–4219 CrossRef PubMed.
- D. Liu, H. Zhang, E. Mäkilä, J. Fan, B. Herranz-Blanco, C. Wang, R. Rosa, A. J. Ribeiro, J. Salonen, J. Hirvonen and H. A. Santos, Biomaterials, 2015, 39, 249–259 CrossRef CAS PubMed.
- C. L. C. Smith, D. K. C. Wu, M. W. Lee, C. Monat, S. Tomljenovic-Hanic, C. Grillet, B. J. Eggleton, D. Freeman, Y. Ruan, S. Madden, B. Luther-Davies, H. Giessen and Y. Lee, Appl. Phys. Lett., 2007, 91, 121103 CrossRef.
- J. Wu, S. Yadavali, D. Lee and D. A. Issadore, Appl. Phys. Rev., 2021, 8, 31304 CAS.
- L. Wang, L. R. Karadaghi, R. L. Brutchey and N. Malmstadt, Chem. Commun., 2020, 56, 3745–3748 RSC.
- I. Lignos, S. Stavrakis, G. Nedelcu, L. Protesescu, A. J. Demello and M. V. Kovalenko, Nano Lett., 2016, 16, 1869–1877 CrossRef CAS PubMed.
- R. Huang, X. Chen, Y. Dong, X. Zhang, Y. Wei, Z. Yang, W. Li, Y. Guo, J. Liu, Z. Yang, H. Wang and L. Jin, ACS Appl. Bio Mater., 2020, 3, 2125–2131 CrossRef CAS.
- J. P. Mcmullen and K. F. Jensen, Org. Process Res. Dev., 2010, 14, 1169–1176 CrossRef CAS.
- Y. Orimoto, K. Watanabe, K. Yamashita, M. Uehara, H. Nakamura, T. Furuya and H. Maeda, J. Phys. Chem. C, 2012, 116, 17885–17896 CrossRef CAS.
- A. Sokhansanj, S. M. Abdoli and M. Zabihi, Process Saf. Environ., 2020, 141, 321–332 CrossRef CAS.
- D. Salley, G. Keenan, J. Grizou, A. Sharma, S. Martín and L. Cronin, Nat. Commun., 2020, 11, 2771 CrossRef CAS PubMed.
- H. Tao, T. Wu, S. Kheiri, M. Aldeghi, A. Aspuru-Guzik and E. Kumacheva, Adv. Funct. Mater., 2021, 2106725 CrossRef.
- O. Voznyy, L. Levina, J. Z. Fan, M. Askerka, A. Jain, M. Choi, O. Ouellette, P. Todorović, L. K. Sagar and E. H. Sargent, ACS Nano, 2019, 13, 11122–11128 CrossRef CAS PubMed.
- K. Abdel-Latif, R. W. Epps, F. Bateni, S. Han, K. G. Reyes and M. Abolhasani, Adv. Intell. Syst., 2021, 3, 2000245 CrossRef.
- H. S. M. Ali, N. Blagden, P. York, A. Amani and T. Brook, Eur. J. Pharm. Sci., 2009, 37, 514–522 CrossRef CAS PubMed.
- S. A. Damiati, D. Rossi, H. N. Joensson and S. Damiati, Sci. Rep., 2020, 10, 19517 CrossRef CAS PubMed.
- M. Flore, R. Zekun, H. Tan, W. W. Kuan, Z. Fang, X. Jiaxun, I. P. S. Tian, J. Senthilnath, M. Zackaria, B. Daniil, H. Kedar, K. Saif, B. Tonio, L. Qianxiao and W. Xiaonan, npj Comput. Mater., 2021, 7, 1–10 CrossRef.
- J. A. Fine, A. A. Rajasekar, K. P. Jethava and G. Chopra, Chem. Sci., 2020, 11, 4618–4630 RSC.
- J. R. Mejía-Salazar, K. R. Cruz, E. M. M. Vásques and O. N. D. Oliveira Jr., Sensors, 2020, 20, 1951 CrossRef PubMed.
- F. Grisoni, B. Huisman, A. L. Button, M. Moret, K. Atz, D. Merk and G. Schneider, Sci. Adv., 2021, 7, eabg3338 CrossRef CAS PubMed.
- E. A. Galan, H. Zhao, X. Wang, Q. Dai, W. T. S. Huck and S. Ma, Matter, 2020, 3, 1893–1922 CrossRef.
- K. Jiang, D. S. Jokhun and C. T. Lim, J. Biomech., 2021, 117, 110235 CrossRef PubMed.
- J. Liu, Z. Xu, Y. Shan and X. Huang, Analyst, 2021, 146, 1529–1537 RSC.
- J. Yang, X. Zhang, C. Liu, Z. Wang, L. Deng, C. Feng, W. Tao, X. Xua and W. Cui, Prog. Mater. Sci., 2021, 118, 100768 CrossRef CAS.
- W. Xiao, L. Xin, R. Cao, X. Wu, R. Tian, L. Che, L. Sun, P. Ferraro and F. Pan, Lab Chip, 2021, 21, 1385–1394 RSC.
- A. A. Volk, R. W. Epps and M. Abolhasani, Adv. Mater., 2021, 33, 2004495 CrossRef CAS PubMed.
- B. A. Rizkin, A. S. Shkolnik, N. J. Ferraro and R. L. Hartman, Nat. Mach. Intell., 2020, 2, 200–209 CrossRef.
- Y. Yang, X. Wei, N. Zhang, J. Zheng, X. Chen, Q. Wen, X. Luo, C. Lee, X. Liu, X. Zhang, J. Chen, C. Tao, W. Zhang and X. Fan, Nat. Commun., 2021, 12, 4876 CrossRef CAS PubMed.
- Y. Li, B. Liu and X. Zhang, Mater. Today, 2021 DOI:10.1016/j.mattod.2021.10.008.
Footnote |
† These authors contributed equally to this work. |
|
This journal is © The Royal Society of Chemistry 2021 |