DOI:
10.1039/C6RA28595C
(Paper)
RSC Adv., 2017,
7, 16494-16504
Metabolomics insights into diabetes nephropathy and protective effects of Radix Scutellariae on rats using ultra-high performance liquid chromatography coupled with quadrupole time-of-flight mass spectrometry
Received
23rd December 2016
, Accepted 7th March 2017
First published on 15th March 2017
Abstract
Diabetic nephropathy (DN) is the most prevalent microvascular complication in diabetes contributing to high mortality. Radix Scutellariae is one of the most commonly used traditional Chinese medicines (TCM) and has played an important role in treating DN. In this study, a urine metabolomics method based on ultra-high performance liquid chromatography coupled with quadrupole time-of-flight mass spectrometry (UHPLC-Q-TOF-MS) has been established to explore the metabolic variations in DN rats and investigate the therapeutic effect of Radix Scutellariae on DN in the different stages (6th week and 10th week). Multivariate analysis approaches were applied to differentiate the components between a Radix Scutellariae-treated group, a model group and a normal group. A series of urinary endogenous metabolites were screened for DN rats, suggesting metabolic dysfunction in pathways of tryptophan, phenylalanine, tyrosine, purine, pyrimidine, and energy metabolism. Treatment with Radix Scutellariae could reverse urinary metabolite abnormalities, and subsequently influenced the above disordered pathways, which were remarkably involved in inhibition of oxidative stress, inflammation and attenuation of several enzymes altered in DN. Our results thus provide an overall view of the progression of DN, and nephroprotective effects of Radix Scutellariae against DN.
1 Introduction
Diabetes mellitus (DM), characterized by hyperglycemia, is a chronic metabolic disease which spreads at epidemic rates, with 415 million adults affected worldwide in 2015.1 The occurrence of diabetic complications has presented to be the major cause of mortality in diabetic patients. The International Diabetes Federation has reported that in every six seconds there will be one diabetes patient death, and diabetes related complications are thought to contribute to the high mortality.2 Diabetic nephropathy (DN) is the most prevalent microvascular complication in DM, which commonly causes end-stage renal failure.3 It is characterized by excessive amassing of extracellular matrix (ECM) with thickening of glomerular and tubular basement membranes and an increased amount of mesangial matrix, which progresses to glomerulosclerosis and tubulo-interstitial fibrosis, and subsequently leads to albuminuria and glomerular filtration rate.4
To date, the therapeutic strategies for DN mainly target on management of blood pressure and intensification of glycaemic control. Inhibitors of renin-angiotensin system (RAS) were considered as the first line therapeutic drugs for DN, providing renoprotective effect over blood pressure reduction.5 Traditional Chinese medicines (TCM) have attracted much attention for their long time clinic test and reliable efficacy on prevention and treatment of diabetes and its complications.6,7
Radix Scutellariae (RS) is one of the valuable TCM which have been comprehensively studied and applied for centuries in regard to DN.8 Flavonoids are the most abundant and active constituents in RS, that were responsible for therapeutic effects on renal protection in DN, especially baicalein and baicalin.9,10 Experimental evidence showed that anti-diabetic function of baicalin and baicalein were related to glucose homeostasis regulation by stimulating insulin secretion and β-cell proliferation.11,12 RS and its components could inhibit apoptosis in vascular endothelial cells and ameliorate angiotensin II-induced endothelial dysfunction.13,14 RS had shown a beneficial role in anti-hypertension via anti-glycation and anti-inflammatory mechanisms, which may result in management of renal dysfunction.15 All these evidences indicate the critical role of RS in prevention and management of DN.
Recently, modern technologies have been utilized to uncover the benefits of using TCM in the management of particular diabetic complications. Metabolomics has emerged as a valuable tool to get comprehensive metabolic analysis and discover novel endogenous biomarkers of DM and its complications, which may reveal the pathophysiology of the complex metabolic disorder and aid to evaluate therapeutic strategies.16,17 Mass spectrometry is a powerful tool for metabolomic analysis with the advantages of high selectivity and sensitivity, coupled with liquid chromatography to make it suitable for throughput metabolic profiling. In this study, an ultra-high performance liquid chromatography coupled with quadrupole time-of-flight mass spectrometry (UHPLC-Q-TOF-MS) method integrated with statistical tools was applied to augment the understanding of DN and investigate the therapeutic efficacy of Radix Scutellariae on DN.
2 Method and materials
2.1 Chemicals
Streptozotocin (STZ), hippuric acid, leucine, uridine, glucose, phenyllactic acid, citric acid, xanthurenic acid, xanthosine dehydrate, phenylacetylglycine, 4-pyridoxic acid, L-ornithine monohydrochloride and cysteine were purchased from Sigma Chem. Co. (St. Louis, MO, USA). Leucine enkephalin and sodium formate were obtained from Waters (Milford, USA). Formic acid and acetonitrile of HPLC grade were supplied by Tedia Company, Inc. (USA) and Fisher Scientific (Fair Lawn, NJ, USA), respectively. Deionized water was prepared using a Milli-Q water purification apparatus (Bedford, MA, USA). All other chemicals and reagents were of analytical grade. Triglyceride (TG) kits, total cholesterol (TC) kits, superoxide dismutase (SOD) kits, malondialdehyde (MDA) kits, urea nitrogen (BUN) kits, creatinine kits, monoamine oxidase (MAO) kits, were obtained from Nanjing Jiancheng Bioengineering Institute (Nanjing, China).
2.2 Preparation of Radix Scutellariae extract
RS was purchased from Tongrentang Pharmacy (Changchun, China) and authenticated by Professor Shumin Wang (Changchun University of Traditional Chinese Medicine, China). The RS was weighted and reflux extracted with 70% ethanol at a sample-to-solvent ratio 1
:
10 (w/v) for 40 minutes. Afterward, the RS residue was reflux extracted twice with the same multiple of water for 1.5 h. Finally the filtrates were combined, concentrated and lyophilized to obtain extract powder.
2.3 DN model construction and treatment
Sprague-Dawley male rats aged 8 weeks were purchased from Experimental Animal Center of Jilin University (China). All the following animal studies were performed according to institutional guidelines for the care and use of laboratory animals, and protocols were approved by the Animal Research Ethics Committee of Jilin University. All animals were kept in a barrier system with regulated temperature (21 ± 2 °C) and humidity (50 ± 5%), and under light–dark cycle of 12 h per day. Prior to initiation of the experiments, all the rats were acclimated for 7 days. They were randomly divided into healthy control group, diabetic model group, Radix Scutellariae treated group (RS group). The rats in healthy control group were fed with standard chow, and rats in the other two groups were fed with high-sucrose and high-fat diet (18% lard, 3% cholesterol, 20% sucrose and 59% standard rat chow) for 8 weeks. Afterward, the rats in diabetic model groups were injected intraperitoneally with STZ (35 mg kg−1 in citrate buffer pH 4.5), while the healthy control group was injected with citrate buffer. Then, the tail-blood glucose level was measured by OneTouch Ultra Meter (Lifescan Inc., CA, USA) after a week. Only those rats whose glycemia was higher than 16.7 mmol L−1 were considered as diabetic and included in our schedule of treatment. Rats in RS treated group were orally administered with RS extract 3 g per kg per day, while the other two groups were given an equal volume of distilled water. All drugs were dissolved in distilled water and administered by gastric irrigation for 10 weeks.
2.4 Sample collection and preparation
During the experimental period, body weights were recorded weekly. Overnight (24 h) urine samples were collected from fasting rats at the 6th week and the end of experiment. The collected urine was centrifuged at 3000 rpm for 10 min. The supernatant was transferred and stored at −80 °C until analysis. At the end of 10 weeks, blood was collected and placed on ice for 30 min and then centrifuged at 3000 rpm for 10 min at 4 °C to get the serum samples that were kept at −80 °C for later biochemical analysis. Prior to UHPLC-MS analysis, urine samples were thawed at 4 °C. After centrifuging at 10
000 rpm for 10 min, the samples were filtered through a 0.22 μm filter membrane. Every 5 μL sample solution was injected directly into UHPLC system for each run.
2.5 Biochemical analyses
Serum triglyceride (TG) and cholesterol (TC) were measured enzymatically using commercial kits. The activity of an antioxidant enzyme-superoxide dismutase (SOD) as well as the concentration of malondialdehyde (MDA), were measured.
The renal function related parameters in different groups were also assayed. The concentrations of albumin in urine samples were determined by the commercial assay kits (Nanjing Jiancheng, Institute of Biotechnology, Nanjing, China), and 24 h urinary albumin excretion rate (UAER) was calculated. The serum creatinine (Scr) and BUN were measured by the commercial assay kits (Nanjing Jiancheng, Institute of Biotechnology, Nanjing, China).
2.6 Histopathological examination
The kidney samples were fixed in 10% buffered formalin, dehydrated and then embedded with paraffin. Sections about 5 μm thickness were sliced from each embedded tissues for histological analysis. Haematoxylin-eosin (HE) staining and Masson's trichrome staining were applied according to the standard protocols. The examination of slides was performed under light microscopy by a pathologist in a blind to the experimental profile.
2.7 UHPLC-Q-TOF-MS analysis
A Waters Acquity UHPLC system coupled with a Q-TOF SYNAPT G2 High Definition Mass Spectrometer (Waters, USA) was used to analyze urine samples. Chromatographic separation was archived on an ACQUITY UHPLC BEH C18 column (1.7 μm, 2.1 mm × 50m; Waters) with column temperature kept at 35 °C and injection volume of 5 μL. Mobile phase A and B were 0.1% formic acid (v/v) in water and acetonitrile respectively. The flow rate was 0.4 mL min−1. The LC gradient was from 5 to 100% B in 8 min, kept at 100% B for 2 min then went back to initial condition. During the period of analysis, all the samples were maintained at 4 °C.
A Q-TOF MS with ESI source was operated in both positive and negative ion modes. The source temperature and desolvation gas temperature were 120 °C and 350 °C separately. Nitrogen was used as cone and desolvation gas. The flow rates of cone and desolvation gas were set at 50 L h−1 and 600 L h−1, respectively. Capillary, cone and extraction cone voltages were set at 3.0 kV, 30 V and 5.0 V in positive ion mode, respectively, while at 2.0 kV, 30 V and 5.0 V in negative ion mode, respectively. MS data was collected in full-scan mode in mass range of 100–1000 Da with a 0.2 s scan time. Sodium formate was used to set up mass spectrometer calibration. A lock-mass of leucine enkephalin at a concentration of 2 ng mL−1 was employed with an infusion flow of 5 μL min−1 via a lock spray interface to ensure accuracy during the MS analysis. Argon was used as the collision gas. MSE was applied for the MS/MS analysis with the low collision energy of 5 eV and the high collision energy of 20–30 eV.
The quality control (QC) samples were prepared by pooling same volume of urine from all samples. All extracts and QC samples were analyzed in parallel on the analytical instrument. Before analysis, 6 QC samples were run to equilibrate the system, and then a QC sample was injected at a regular interval (every ten samples) throughout the analytical run to observe the repeatability and stability of the system.
2.8 Data processing and pattern recognition analysis
The raw data were processed with MarkerLynx 4.1 software (Waters) and MarkerLynx Application Manager (Waters) for peak detection, alignment and normalization. A data matrix was generated to include normalized peak intensity, exact mass and retention time. Then, the data matrix was subjected to principal component analysis (PCA), a program module, and orthogonal projection to latent structures squares-discriminant analysis (OPLS-DA), another program module, for pattern recognition using EZinfo 2.0 software. The application of PCA is to reduce the dimensionality of the raw data set to enable easy visualization of any metabolic clustering in the different groups of samples. OPLS-DA was used to maximize covariance between the data. The S-plots and VIP-plots corresponding with OPLS-DA analysis were conducted to extract potential biomarkers. The significance was expressed by using Student's t-test. The potential biomarkers with p values less than 0.05 were considered significant.
Potential biomarkers were identified by searching in HMDB (http://www.hmdb.ca) and verified by standards. The related metabolic pathway was searched in KEGG (http://www.genome.jp/kegg/ligand.html). The potential biomarkers were evaluated by the enrichment analysis using the MetaboAnalyst.18 For further interpretation of metabolomics data, a network was established based on KEGG pathway analysis using Metscape (a plugin for Cytoscape). By subjecting biomarkers' information to this database, Metscape provides a visual map that integrates connections among metabolites, reactions, enzymes and genes.
3 Results
3.1 Biochemical parameters
The biochemistry parameters of different groups were compared to evaluate therapeutic effects of RS, which are presented in Table 1. Oxidative stress were induced in rats of model group, with a significant reduction of serum SOD activities while increased the content of serum MDA compared with normal rats (p < 0.05). Treatment of RS could mitigate the oxidative stress damage in diabetic rats, along with regulated serum SOD activities and MDA contents. In addition, RS partially reduced serum TC and TG levels.
Table 1 Physiological, biochemical, and renal functional parameters of rats in different groups after RS-treated for 10 weeksa
Group |
Sample size |
SOD (U mL−1) |
MDA (μmol L−1) |
TC (mmol L−1) |
TG (mmol L−1) |
BUN (mmol L−1) |
Scr (μmol L−1) |
UAER (mg per 24 h) |
*p < 0.05 versus model group. **p < 0.01 versus model group. |
Normal group |
8 |
66.33 ± 6.72** |
3.94 ± 0.58** |
0.98 ± 0.11** |
0.44 ± 0.04** |
4.52 ± 0.67** |
97.67 ± 4.58** |
4.72 ± 0.92** |
Model group |
8 |
50.95 ± 4.88 |
5.22 ± 0.35 |
1.58 ± 0.14 |
0.78 ± 0.06 |
8.01 ± 0.93 |
143.34 ± 7.41 |
31.54 ± 3.7 |
RS group |
8 |
58.73 ± 5.69* |
4.23 ± 0.65* |
1.32 ± 0.23* |
0.67 ± 0.09* |
6.24 ± 0.72** |
122.42 ± 11.48** |
21.32 ± 4.84** |
In addition, Table 1 shows the improvement effect of RS on biochemical parameters related to renal functions. Levels of renal function markers, BUN, Scr and UAER presented significant elevations in model group to compare with normal group (shown in Table 1), suggesting that marked DN has been developed in model group. Compared with the control group, the levels of BUN, Scr and UAE were dramatically increased in model group. After being treated for 10 weeks, levels of these parameters showed significant decrease in RS group compared with model group.
3.2 Histopathological findings
To investigate the effects of RS on the renal lesions in DN rat models, HE staining and Masson's trichrome staining of these renal tissues were performed. HE staining showed that, no histological alterations in the kidney of normal rat were observed (Fig. 1A). However, kidney sections from diabetic rats (Fig. 1B) revealed typical pathological features of kidney damage, including the moderate expansion in mesangial matrix, thickening of glomerular basement membrane, tubular vacuolization and dilation atrophy. In contrast, treatment with RS for 10 weeks could noticeably improve the pathological abnormalities (Fig. 1C), which showed kidney protective function of RS. On the other hand, Masson staining results revealed the renal fibrosis in the tubulointerstitial area. The area of renal interstitial fibrosis in model group (Fig. 1E) was significantly larger than that in control group (Fig. 1D), however, the renal interstitial area was modified by RS treatment (Fig. 1F). These results indicated the therapeutic effect of RS on ameliorating renal lesions.
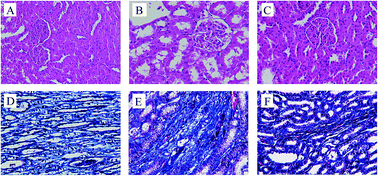 |
| Fig. 1 Effect of RS on the histological morphology of rats' kidney by HE staining (A–C) and Masson's trichrome staining (D–F). (A, D) Normal group; (B, E) Model group; (C, F) RS group; (magnification: 400). | |
3.3 Validation of UHPLC-MS
In metabolomic studies, the detection aims to get a holistic and global picture of the metabolome to be relatively unbiased. The analytical technology applied in metabolomic study could provide repeatable, robust and high-quality data. Thus, QC samples have been applied in this study as a pragmatic solution to fulfill the requirement to simultaneously assess repeatability for hundreds of endogenous metabolites. The PCA score plots (Fig. 2) demonstrate that the system is relatively stable during sample analysis. To evaluate the system consistency, 18 species of ions (0.42–114.067, 0.88–367.152, 1.44–206.046, 2.67–216.064, 3.29–225.110, 4.75–170.061, 5.17–321.095, 6.04–203.110, 7.96–231.142 in positive ion mode, and 0.54–191.025, 0.96–258.03, 1.30–242.018, 2.65–192.071, 3.38–283.087, 4.56–219.068, 5.14–297.102, 6.38–201.118, 8.40–141.134 in negative in mode, respectively) were monitored as extracted ion chromatograms through the entire data set of QC injections in both positive and negative ion modes. The relative standard deviations of retention times and peak areas of the 18 selected ions were 0.36–8.62% and 1.89–7.55%, respectively. All these results were very satisfactory, which show that the UHPLC-Q-TOF-MS system was robust with good reproducibility and stability.
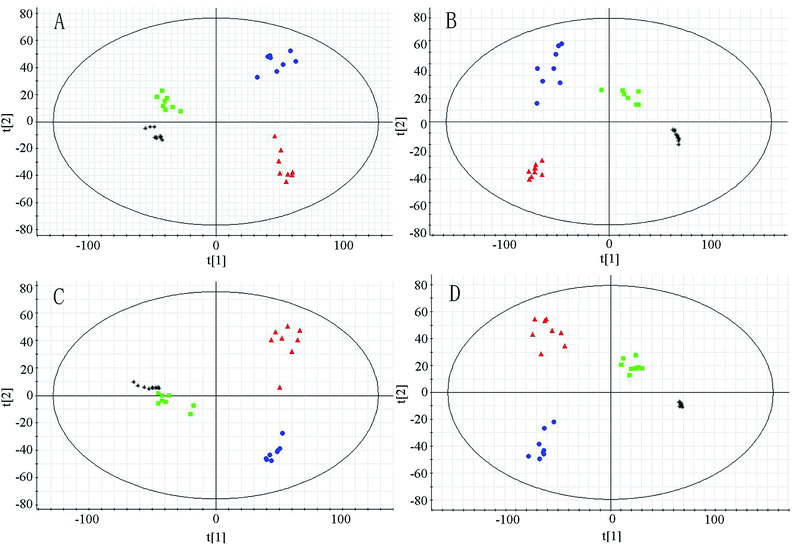 |
| Fig. 2 PCA score plots derived from urinary metabolic profile of normal group (■), model group (●), RS group (▲) and QC samples (*) in positive mode (A, C) and negative mode (B, D) at week 6 (A, B) and week 10 (C, D). | |
3.4 Multivariate analysis of UHPLC-Q-TOF-MS data
The system of UHPLC-Q-TOF-MS was applied for analysis of urinary metabolism in both positive and negative ion modes. The PCA to be used as a pattern recognition method was applied to visualize metabolic differences among different groups at week 6 and week 10 (Fig. 2). In the PCA score plots, each spot represents a sample. The model group and normal group could be clearly separated in PCA score plots, which indicated group differences were remarkable and marked alteration of urinary metabolites in model group. Moreover, PCA analysis showed RS group was separated from model group and close to normal group, implied the treatment effects of RS.
The OPLS-DA model to be also used as a pattern recognition approach was further conducted to maximize class separation and recognize the potential biomarkers from the control group and model group at the week 6 and week 10. The OPLS-DA score plots present good fitness and high predictability of model, with high statistical values of R2Y and Q2. In the 6th week, the parameters separately were R2Y = 0.973, Q2 = 0.815 in positive mode and R2Y = 0.970, Q2 = 0.830 in negative mode. In the 10th week, the values individually presented to be R2Y = 0.995, Q2 = 0.923 in positive mode and R2Y = 0.995, Q2 = 971 in negative mode. The control and model groups were distinguished clearly, indicating that metabolic profiles changed significantly in model group along with different time and endogenous substances in urine were disturbed significantly as the progress of diabetes.
3.5 Identification of potential biomarkers
The variable importance in the projection (VIP) of OPLS-DA model was generated using centroid scaling to identify the metabolite contribution to the discrimination. The metabolites with VIP value above 1.0 and p-value below 0.05 were considered as potential biomarkers. The S-plot, a further loading plot, was used for verification of the significance of potential biomarkers. Based on this rule, potential biomarkers in urine were screened for further study.
The possible molecular formulas of those potential biomarkers were calculated by high-accuracy quasi-molecular ion within a mass error of 10 ppm, and the fractional isotope abundance detected by Q-TOF-MS was used to filter out the interference. MS/MS fragmentation patterns also provided necessary information for the structure of biomarkers. Twelve of them were confirmed with available reference standards by matching their retention time and accurate mass measurement. What follows is a description of the identification procedure taking one ion as an example. In positive mode, the ion exhibited a retention time of 1.72 and the [M + H]+ ion at m/z 180.0667. This ion might contain an odd number of nitrogen atoms because its precise molecular weight was 179.0582. According to the analysis of its elemental composition, the molecular formula was speculated as C9H10NO3. By calculation of the degree of unsaturation (the double bond equivalent is 5), this compound might have an aromatic ring. In MS/MS experiments, we obtained fragment ion at m/z 105.0350 and 77.0385, which could correspond to loss –C2H5NO2 and then further loss –CO. By searching related database, the candidate compound was found to be hippuric acid. To further confirm the structure, its retention time and accurate mass were compared with related reference standard. Finally it was identified to be hippuric acid. The extracted ion chromatograph of reference standard and MS/MS spectrum of hippuric acid are displayed in Fig. 3.
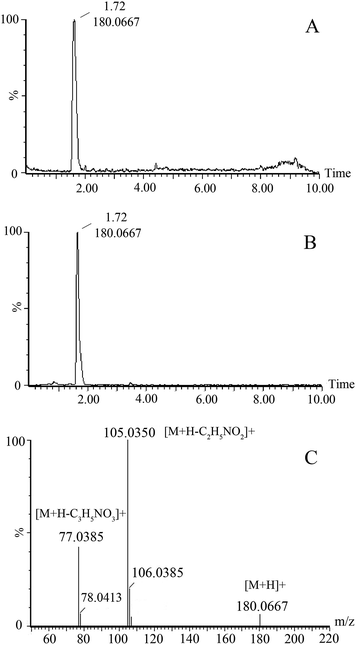 |
| Fig. 3 The extracted ion chromatograph of hippuric acid in urine (A) and standard (B); MS/MS spectrum (C) of hippuric acid. | |
The information of potential biomarkers screened from the 6th week and the 10th week are summarized in Table 2. We successfully identified 25 potential biomarkers in the 6th week and 34 potential biomarkers in the 10th week for DN rats respectively. In the potential biomarkers, several metabolites, such as hippuric acid, phenylacetylglycine, citric acid and indoxyl sulphate have been reported in other DN studies,19 with similar variation tendencies that consistent with our results presented in this study. In the current study, more DN-related novel potential biomarkers that independent from known risk indicators were screened based on metabolomics, which may contribute to understanding the pathophysiological mechanisms and metabolic response to DN. Among these biomarkers, 15 and 25 biomarkers separately in week 6 and week 10, were partially reversed in RS treated group, reflecting renoprotective effects of RS.
Table 2 Identification and change trends of biomarkers in the 6th week and 10th weeka
Mode |
Identification |
RT (min) |
Mass error (ppm) |
Measured mass (Da) |
6 weeks |
10 weeks |
Change trend (M/K) b |
Change trend (RS/M) c |
Change trend (M/K) b |
Change trend (RS/M) c |
The up (↑) and down (↓) arrows represent the relative increasing or decreasing trend of the metabolites, “—” represents no significant differences between the RS group and model group, “✗” denotes the metabolite were not identified as potential biomarkers. Change trend of model group vs. control group. Change trend of RS group vs. model group. (*p < 0.05; **p < 0.01). |
ESI+ |
3-Indole carboxylic acid glucuronide |
1.89 |
1.0 |
338.0867 |
↑ |
↓** |
↑ |
↓** |
Hippuric acid |
1.72 |
6.5 |
180.0667 |
↑ |
↓* |
↑ |
↓** |
Xanthosine |
3.04 |
2.7 |
285.0797 |
↑ |
— |
↑ |
↓** |
Glucose |
1.21 |
8.6 |
181.0691 |
↑ |
↓* |
↑ |
↓* |
Indole-3-carboxylic acid |
2.69 |
9.5 |
162.0565 |
↑ |
↓* |
↑ |
↓** |
3-Methyldioxyindole |
2.76 |
3.7 |
164.0712 |
↑ |
↓* |
↑ |
↓* |
4,6-Dihydroxyquinoline |
3.62 |
9.5 |
162.0565 |
↓ |
↑** |
↓ |
↑** |
Aldosterone |
6.84 |
6.5 |
361.2033 |
↑ |
— |
✗ |
✗ |
4-Oxo-retinoic acid |
9.31 |
0.7 |
315.1957 |
↑ |
↓** |
✗ |
✗ |
Deoxyribose |
1.17 |
4.4 |
135.0646 |
↑ |
— |
✗ |
✗ |
Metanephrine |
4.50 |
3.2 |
198.1131 |
↓ |
— |
✗ |
✗ |
Pantetheine |
2.47 |
2.9 |
279.1381 |
✗ |
✗ |
↑ |
↓** |
Phenylacetic acid |
2.60 |
3.6 |
137.0602 |
✗ |
✗ |
↑ |
↓** |
5-Hydroxykynurenamine |
2.97 |
6.9 |
181.0959 |
✗ |
✗ |
↓ |
— |
Histidinal |
0.68 |
8.8 |
140.0806 |
✗ |
✗ |
↑ |
↓** |
Cervonoyl ethanolamide |
7.32 |
4.1 |
373.2722 |
✗ |
✗ |
↑ |
— |
Imidazoleacetic acid |
0.61 |
7.1 |
127.0493 |
✗ |
✗ |
↑ |
↓** |
Phenylacetaldehyde |
3.00 |
9.2 |
121.0659 |
✗ |
✗ |
↑ |
— |
Ornithine |
4.81 |
7.9 |
133.0982 |
✗ |
✗ |
↑ |
↓* |
4-Hydroxybenzaldehyde |
4.70 |
2.1 |
123.0438 |
✗ |
✗ |
↑ |
↓** |
Leucine |
2.30 |
7.6 |
132.1009 |
✗ |
✗ |
↑ |
↓** |
Uridine |
4.51 |
2.0 |
245.0773 |
✗ |
✗ |
↑ |
↓** |
Hydrocinnamic acid |
5.55 |
0.4 |
151.0753 |
✗ |
✗ |
↑ |
— |
L-Cysteine |
3.20 |
8.0 |
122.0280 |
✗ |
✗ |
↓ |
— |
ESI− |
Indoxyl sulfate |
2.35 |
7.5 |
212.0104 |
↓ |
↑* |
↓ |
↑** |
p-Cresol sulfate |
4.09 |
7.7 |
187.0085 |
↑ |
↓* |
↓ |
— |
4-Pyridoxic acid |
0.46 |
1.7 |
182.0462 |
↓ |
↑* |
↓ |
— |
Indolelactic acid |
3.91 |
4.3 |
204.0675 |
↓ |
— |
↓ |
— |
5-Thymidylic acid |
0.51 |
2.6 |
321.0485 |
↑ |
↓* |
↑ |
↓** |
Phenyllactic acid |
2.27 |
6.5 |
165.0568 |
↑ |
— |
↑ |
↓** |
Xanthurenic acid |
1.20 |
6.2 |
204.0315 |
↓ |
— |
↓ |
— |
2-Phenylethanol glucuronide |
2.80 |
3.4 |
283.0833 |
↑ |
↓* |
✗ |
✗ |
5-L-Glutamyl-taurine |
5.57 |
0.7 |
253.0508 |
↑ |
— |
✗ |
✗ |
Cholic acid |
8.61 |
3.9 |
407.2819 |
↑ |
↓ |
✗ |
✗ |
3,4-Dihydroxybenzylamine |
0.46 |
7.5 |
138.0571 |
↓ |
— |
✗ |
✗ |
Suberic acid |
3.09 |
5.6 |
173.0829 |
↓ |
↑** |
✗ |
✗ |
Glycocholic acid |
7.80 |
4.8 |
464.304 |
↑ |
↓** |
✗ |
✗ |
3-Oxooctanoic acid |
5.70 |
9.3 |
157.0885 |
↓ |
— |
✗ |
✗ |
7-Methylguanosine |
4.47 |
3.6 |
297.1068 |
✗ |
✗ |
↑ |
↓** |
Citric acid |
0.29 |
7.2 |
191.0211 |
✗ |
✗ |
↓ |
↑** |
3,4-Dihydroxybenzeneacetic acid |
0.89 |
6.6 |
167.0361 |
✗ |
✗ |
↑ |
↓** |
Phenylpyruvic acid |
1.82 |
5.7 |
163.0410 |
✗ |
✗ |
↑ |
↓** |
3,4-Dihydroxymandelaldehyde |
1.20 |
3.7 |
167.0356 |
✗ |
✗ |
↑ |
↓* |
Phenylacetylglycine |
2.09 |
6.7 |
192.0679 |
✗ |
✗ |
↓ |
— |
Phenylacetylglutamine |
3.54 |
4.3 |
263.1026 |
✗ |
✗ |
↑ |
↓** |
To illustrate the correlations among the biomarkers, a network diagraph was generated based on searching results from the KEGG database and presented in Fig. 4. In addition, the heatmap generated from biomarkers (Fig. 5) showed distinct segregation between these three groups, and revealed the metabolic variation influenced by DN.
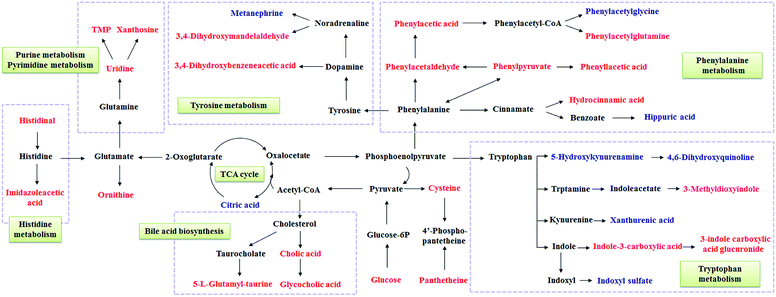 |
| Fig. 4 Correlation networks of main potential biomarkers in response to DN. The metabolites marked in red denote the increased potential biomarkers, and blue denote the decreased ones. | |
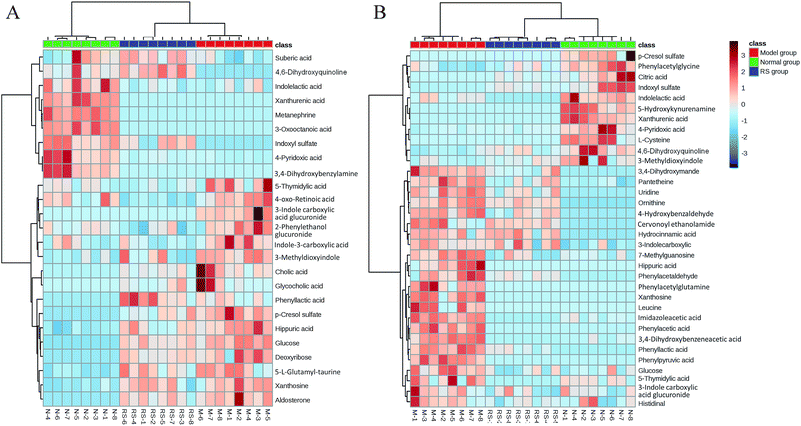 |
| Fig. 5 Heatmap visualizing the intensities of potential biomarkers in different groups at week 6 (A) and week 10 (B). Increasing expression values are coded with blue to red colors. Rows indicate potential biomarkers; columns indicate samples. | |
4 Discussion
In this study, high fat diet and streptozotocin-induced diabetic rats have developed markedly DN which was confirmed by biochemical and histological examinations. UHPLC-Q-TOF-MS based metabolomics approach was established to investigate the metabolic variations in urine of diabetic rats which involved in the progression of DN. The variations of biomarkers revealed perturbations of several metabolism pathways that significantly correlated with the development of DN. The current study also presented therapeutic effects of RS in ameliorating renal lesions, kidney function and urinary metabolite abnormalities in rats with DN. To evaluate the reversal of potential biomarkers by treatment of RS, the relative intensities of biomarkers from different groups in the 6th week and 10th week were compared, respectively. The results in this study revealed that RS treatment was able to regulate the metabolic disorders caused by DN, thereby blocking the progression of DN.
4.1 Changes in phenylalanine metabolism pathway
Phenylalanine is an aromatic amino acid that presented to be highly significant predictors of diabetes risk.20 Changes in phenylalanine metabolism showed close association with chronic kidney failure, including extensive urinary excretion of its down products.21 In DN rats, there is an impairment of phenylalanine metabolism, especially in the 10th week, including remarkable elevation of urine phenylacetic acid (PAA), phenylacetaldehyde, phenylpyruvic acid, phenyllactic acid, hippuric acid, phenylacetylglutamine (PAG), hydrocinnamic acid as well as reduction of phenylacetylglycine and p-cresol sulfate compared with those in control group. Whereas only changes of phenyllactic acid, hippuric acid, and p-cresol sulfate were found in the 6th week.
Interestingly, PAA, PAG, hippuric acid and p-cresol sulfate are protein-bound uremic toxins that contributed to the progression of chronic kidney disease (CKD) and cardiovascular disease. They were proved to induce endothelial ROS productions in human umbilical vein endothelial cells.22 In the current study, alterations of these uremic toxins were prevented by RS treatment, except for p-cresol sulfate in the 6th week and 10th week.
As shown in the network, PAA served as a key node that closely connected with several potential biomarkers in phenylalanine metabolism. Endogenous PAA is transformed from phenylalanine by gut microbiota actions, via the generation process, phenylacetaldehyde, phenylpyruvate were presented as intermediate products. Up-regulated contents of PAA, phenylacetaldehyde and phenylpyruvate indicated the formation of activated PAA. Excess PAA exerts the abilities to stimulate reactive oxygen species (ROS) by inhibiting expression of inducible NO synthase (iNOS) in vascular smooth cells, and enhance the activation of polymorphonuclear leucocytes caused inflammation, denoting its role linking oxidative stress and inflammation to cardiovascular risk.23,24 PAG and phenylacetylglycine are generated from PAA by conjugating with glutamine in liver, and subsequently derived from kidney filtration and excreted in urine. The production of PAG was considered as a vital route to dispose waste nitrogen in urea cycle disorders and CKD.25 In the 10th week, elevated urinary PAG was observed reflecting excess nitrogen excretion in DN, resulted from urea accumulation induced by damaged renal function. Up-regulations of PAA, PAG, phenylpyruvic acid and phenyllactic acid in model group were reversed to normal levels by RS-treatment indicating the inhibition effect of RS on PAA metabolism in DN rats, consequently, RS-treatment protected against oxidative stress and progression of DN.
Moreover, these potential biomarkers involved in phenylalanine metabolism were mammalian-microbial co-metabolites.26 The gut microbiota function was found to be an important environmental factor in the development of DM. For instance, hippuric acid is a glycine conjugate of benzoic acid transformed by intestinal microorganisms, and its urinary level was involved in the distribution of intestinal microbial colonies.27,28 Overload of hippuric acid has been shown to accelerate renal damage and early development of vasculopathy. Hydrocinnamic acid is also a gut microbiota related metabolite produced by certain species of Clostridium which also contribute substantially to the generation of PAA.29 Variations of these metabolites in urine of model group suggest the prominent change of gut microbiota over time. Long-term administration of RS partially ameliorated the DN-associated abnormalities in phenylalanine metabolism, which is linked to regulation of altered gut microbiota.
4.2 Changes in tryptophan metabolism pathway
In tryptophan metabolic pathway, 3-methyldioxyindole, indole-3-carboxylic acid, 3-indole carboxylic acid glucuronide, 4,6-dihydroxyquinoline, indolelactic acid, 5-hydroxykynurenamine, indoxyl sulfate and xanthurenic acid were the related metabolites that significantly differentiated between the model group and control group. Tryptophan is metabolized mainly through 2 pathways in vivo, either by kynurenine or via indolic pathway. Disturbance of these two pathways were found in DN rats.
In indole pathway, obvious increase of 3-methyldioxyindole, indole-3-carboxylic acid, 3-indole carboxylic acid glucuronide and decrease of indoxyl sulfate in both 6th week and 10th week were observed. Indoxyl sulfate, known as indolic uremic toxin that accelerates ROS production and inflammation, linked cardiovascular toxicity to CKD by activating the nuclear translocation of aryl hydrocarbon receptor (known as dioxin receptor).30,31 Accumulation of its serum level has been demonstrated to be correlated with renal functions in DM patients.32 However, decreased urinary indoxyl sulfate level was observed in model group, which may be in part due to its strong protein-binding ratios and declined glomerular filtration rate caused by renal damage. Xanthurenic acid is a downproduct via kynurenine pathway. The level of xanthurenic acid decreased significantly in the urine of DN rats compared to the control rats, which was consistent with the result reported in CKD rats, indicating disorder in kynurenine pathway.33
Taken together, overload of indole pathway and down-regulated of kynurenine pathway suggested disorders in tryptophan metabolism. Treatment with RS could effectively regulate the disturbed indole pathway from the 6th week to 10th week, except for reversing the rise of indoelactic acid. Whereas results revealed that RS could not reverse the changes of xanthurenic acid. These findings indicating therapeutic effects of RS might base on the regulation of the dysfunction in tryptophan metabolism, especially in indole pathway.
4.3 Changes in tyrosine metabolism pathway
In week 10, the DN rats exhibited markedly elevation of urine 3,4-dihydroxybenzeneacetic acid (DOPAC) and 3,4-dihydroxymandelaldehyde (DHMAL) in tyrosine metabolism pathway compared with the normal rats. Tyrosine served as the precursor of monoamine neurotransmitters, like dopamine, norepinephrine and epinephrine. Intracellular turnover of monoamines were regulated by monoamine acid (MAO). MAO, known as flavin-containing amine oxidase with A and B two isoforms, located at the outer mitochondrial membrane and emerged as an important mitochondrial source of ROS. MAO-derived tissue redox imbalance situation contributed to endothelial dysfunction in cardiovascular pathology and diabetes.34,35 Manni et al. have demonstrated that activation of MAO-A is a part of the pathogenenic program activated by angiotensin-II in diabetes leading to DN.36 Notably, MAO is responsible for the formation of DOPAC and DHMAL from dopamine. Enhanced MAO activity may in part contribute to excess urinary excretion of these two metabolites. It has been demonstrated that, known inhibitor of mitochondrial MAO, such as clorgyline, could attenuate DOPAC production.37 Additionally, MAO was also involved in the generation of phenylacetaldehyde and 4,6-dihydroxyquinoline (shown in Fig. 6A), two potential biomarkers in phenylalanine metabolism pathway. In current study, up-regulated contents of DOPAC and DHMAL could be reversed by treatment with RS for 10 weeks leading to the hypothesis that was the MAO inhibitory effect of RS. To explore this hypothesis we measured MAO activity in serum of different groups at week 10. Significantly increased serum MAO activity was observed in DN rats compared to that in the normal rats (Fig. 6B), which in part explain the changes of MAO-related down products. Such an increase trend was weakened by long-term treatment of RS, with declined MAO activity detected in RS group. These data, taken collectively, indicated the therapeutic effect of RS on DN by modulating MAO activity and consequently regulating production of ROS.
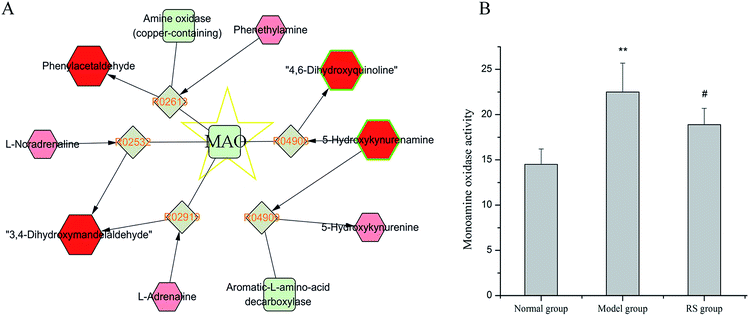 |
| Fig. 6 (A) The MAO related network generated by Metscape 2. Hexagons (red) represent the biomarkers; hexagons (pink) represent precursor metabolites or down products; squares (gray) represent reactions with KEGG reaction identification numbers and round cornered squares (green) are the associated enzymes. (B) Serum MAO activities in different groups. #p < 0.05 compared with normal group; *p < 0.05 compared with model group. | |
4.4 Changes in purine and pyrimidine metabolism pathways
Several metabolites involved in purine and pyrimidine metabolism pathways were proposed to be potential biomarkers that significantly differentiated from the model group and control group. The obvious increases of xanthosine, 5-thymidylic acid (TMP), 7-methylguanosine and uridine were observed in DN rats in the 10th week, however, increased xanthosine and TMP also occurred in the 6th week. Purine catabolism was reported to be an important component of the homeostatic response of mitochondria to oxidant stress and altered in the liver mitochondria of diabetic rats.38 Xanthosine and 7-methylguanosine are nucleosides derived from purine catabolism. Xanthosine was one of the major physiological precursors of xanthine. Elevation of these two potential biomarkers suggested the shifts to favor breakdown to xanthine in purine metabolism, linking to oxidant stress and nucleoside impairment. Uridine is a nucleoside presented in pyrimidine metabolism pathway. It was considered as a predictor for kidney deterioration.39 Uridine and its nucleotides were observed to effectively suppress renin secretion in kidney leading to its vasoconstrictor effect.40 TMP is an ester of phosphoric acid with the nucleoside thymidine. Interestingly, the generations of xanthosine, uridine and thymindine are closely related with 5′-nucleotidase. Endogenous 5′-nucleotidase inhibited basal lipid oxidation and glucose transport in skeletal muscle by suppressing AMP-activated protein kinase.41 Up-regulation of these potential biomarkers implicated the impairments of 5′-nucleotidase-dependent nucleosides metabolism in DN rats, while they were reversed by intervention of RS in week 10, though not to normal levels. These findings reflected the therapeutic action of RS in regulating nucleosides metabolism may in part base on its suppressive effect on 5′-nucleotidase in DN.
4.5 Changes in energy metabolism pathway
The declined citric acid (10th week) and increased glucose excretions (6th and 10th weeks) were evident in model group compared with the control group. These two potential biomarkers were also involved in energy metabolism. Hyperglycemia is the main characteristic of diabetes. Blood glucose is filtered at the glomerulus and reabsorbed in the proximal convoluted tubule via the sodium-dependent glucose transporters, SGLT2 and SGLT1.42 Excess renal glucose excretion induced by increased blood glucose, actually reflected the loss of energy and negative energy balance.43 Activation of SGLTs may also in part give rise to urinary glucose excretion. Studies have demonstrated SGLT inhibitors could modify energy balance by efficiently reducing urinary glucose excretion, and be useful in the treatment of DM and renal impairment.44,45 Moreover, recent research demonstrated that RS treatment regulated urinary glucose in chronic renal failure.46 Down-regulated urinary glucose level by RS treatment throughout the progress of DN implicated the potential suppressive effect of RS on SGLT1 or SGLT2.
Citric acid is a major intermediate in the tricarboxylic acid (TCA) cycle. Declined urinary citric acid is attributed to the impairment of the TCA cycle, reflecting reduction of aerobic glycolysis and decreased ATP production. It serves as a signaling molecule in management of glucose stimulated cytosolic ATP/ADP ratio and insulin secretion.47,48 Thus, it can be inferred that citric acid involved disordered energy metabolism via modulating the production of ATP. Down-regulated citric acid in DN rats was partially restored in response to RS intervention, indicating that DN was involved in energy metabolism and RS exhibited a protective effect on DN.
5 Conclusion
In this study, an UHPLC-Q-TOF-MS based urine metabolomics approach has been established to explore the metabolic variations in DN rats and investigate the therapeutic effects of RS. We successfully identified 25 potential biomarkers in the 6th week and 34 potential biomarkers in the 10th week for DN rats respectively. Among these biomarkers, 15 and 25 biomarkers in week 6 and week 10 were partially reversed in RS treated group separately. These potential biomarkers involved in perturbations in amino acids metabolism, purine and pyrimidine metabolism, and energy metabolism pathways that closely related with oxidative stress and inflammation. RS intervention could effectively modulate these disturbed metabolism pathways, thereby, block the progression of DN. Based on identification of pathological metabolic changes, metabolomics pointed to potential targets for therapeutic effect of RS against DN. There are still some limitations in clarifying the mechanisms of RS on progression of DN, thus further studies will be followed up.
Acknowledgements
This work was supported by the National Natural Science Foundation of China (No. 81373952).
References
- S. Sadikot, Diabetes Res. Clin. Pract., 2016, 114, 180–181 CrossRef PubMed.
- I. D. A. Grp, Diabetes Res. Clin. Pract., 2013, 100, 277–279 CrossRef PubMed.
- H. Nasri, J. Renal Inj. Prev., 2013, 2, 31–32 Search PubMed.
- R. M. Mason and N. A. Wahab, J. Am. Soc. Nephrol., 2003, 14, 1358–1373 CrossRef CAS PubMed.
- S. Clotet, M. Riera, J. Pascual and M. J. Soler, Am. J. Physiol., 2016, 310, F945–F957 CrossRef CAS PubMed.
- K. Hamid, A. Alqahtani, M. S. Kim, J. L. Cho, P. H. Cui, C. G. Li, P. W. Groundwater and G. Q. Li, Curr. Top. Med. Chem., 2015, 15, 2406–2430 CrossRef CAS PubMed.
- M. Makni, H. Fetoui, N. K. Gargouri, M. Garoui el and N. Zeghal, J. Diabetes Complications, 2011, 25, 339–345 CrossRef PubMed.
- C. Li, G. Lin and Z. Zuo, Biopharm. Drug Dispos., 2011, 32, 427–445 CrossRef CAS PubMed.
- A. Ahad, M. Mujeeb, H. Ahsan and W. A. Siddiqui, Biochimie, 2014, 106, 101–110 CrossRef CAS PubMed.
- N. Su, P. Zhao, L. Q. Zhou, B. Y. Du, H. Luo and J. Y. Lang, Amino Acids, 2011, 41, S34 Search PubMed.
- S. M. Park, S. M. Hong, S. R. Sung, J. E. Lee and D. Y. Kwon, Genes Nutr., 2008, 2, 347–351 CrossRef PubMed.
- Y. Fu, J. Luo, Z. Jia, W. Zhen, K. Zhou, E. Gilbert and D. Liu, Int. J. Endocrinol., 2014, 2014, 846742 Search PubMed.
- K. S. Suh, Y. H. Nam, Y. M. Ahn, N. J. Kim, C. Y. Park, G. Koh, S. Oh, J. T. Woo, S. W. Kim, J. W. Kim and Y. S. Kim, Biol. Pharm. Bull., 2003, 26, 1629–1632 CAS.
- X. Wei, X. Zhu, N. Hu, X. Zhang, T. Sun, J. Xu and X. Bian, Biochem. Biophys. Res. Commun., 2015, 465, 101–107 CrossRef CAS PubMed.
- H. M. El-Bassossy, N. A. Hassan, M. F. Mahmoud and A. Fahmy, Phytomedicine, 2014, 21, 1742–1745 CrossRef CAS PubMed.
- N. Tian, J. S. Wang, P. R. Wang, X. F. Song, M. H. Yang and L. Y. Kong, Metabolomics, 2013, 9, 1228–1242 CrossRef CAS.
- A. Hirayama, E. Nakashima, M. Sugimoto, S. Akiyama, W. Sato, S. Maruyama, S. Matsuo, M. Tomita, Y. Yuzawa and T. Soga, Anal. Bioanal. Chem., 2012, 404, 3101–3109 CrossRef CAS PubMed.
- J. Xia, I. Sinelnikov, B. Han and D. S. Wishart, Nucleic Acids Res., 2015, 43, W251–W257 CrossRef PubMed.
- D. F. Stec, S. Wang, C. Stothers, J. Avance, D. Denson, R. Harris and P. Voziyan, Biochem. Biophys. Res. Commun., 2015, 456, 610–614 CrossRef CAS PubMed.
- N. Friedrich, J. Endocrinol., 2012, 215, 29–42 CrossRef CAS PubMed.
- J. D. Kopple, J. Nutr., 2007, 137, 1586S–1590S CAS.
- Y. Itoh, A. Ezawa, K. Kikuchi, Y. Tsuruta and T. Niwa, Anal. Bioanal. Chem., 2012, 403, 1841–1850 CrossRef CAS PubMed.
- J. F. Saldanha, D. Yi, M. B. Stockier-Pinto, H. A. Soula, S. Chambert, D. Fouque, D. Mafra and C. O. Soulage, Biochimie, 2016, 125, 53–58 CrossRef CAS PubMed.
- G. Cohen, J. Raupachova and W. H. Horl, Nephrol., Dial., Transplant., 2013, 28, 421–429 CrossRef CAS PubMed.
- M. Posada-Ayala, I. Zubiri, M. Martin-Lorenzo, A. Sanz-Maroto, D. Molero, L. Gonzalez-Calero, B. Fernandez-Fernandez, F. de la Cuesta, C. M. Laborde, M. G. Barderas, A. Ortiz, F. Vivanco and G. Alvarez-Llamas, Kidney Int., 2014, 85, 103–111 CrossRef CAS PubMed.
- M. Li, B. Wang, M. Zhang, M. Rantalainen, S. Wang, H. Zhou, Y. Zhang, J. Shen, X. Pang, M. Zhang, H. Wei, Y. Chen, H. Lu, J. Zuo, M. Su, Y. Qiu, W. Jia, C. Xiao, L. M. Smith, S. Yang, E. Holmes, H. Tang, G. Zhao, J. K. Nicholson, L. Li and L. Zhao, Proc. Natl. Acad. Sci. U. S. A., 2008, 105, 2117–2122 CrossRef CAS PubMed.
- A. R. Rechner, G. Kuhnle, H. L. Hu, A. Roedig-Penman, M. H. van den Braak, K. P. Moore and C. A. Rice-Evans, Free Radical Res., 2002, 36, 1229–1241 CrossRef CAS PubMed.
- E. Bohus, M. Coen, H. C. Keun, T. M. Ebbels, O. Beckonert, J. C. Lindon, E. Holmes, B. Noszal and J. K. Nicholson, J. Proteome Res., 2008, 7, 4435–4445 CrossRef CAS PubMed.
- D. Mayrand and G. Bourgeau, J. Clin. Microbiol., 1982, 16, 747–750 CAS.
- M. B. Stockler-Pinto, J. F. Saldanha, D. Yi, D. Mafra, D. Fouque and C. O. Soulage, Free Radical Res., 2016, 50, 337–344 CrossRef CAS PubMed.
- B. Gondouin, C. Cerini, L. Dou, M. Sallee, A. Duval-Sabatier, A. Pletinck, R. Calaf, R. Lacroix, N. Jourde-Chiche, S. Poitevin, L. Arnaud, R. Vanholder, P. Brunet, F. Dignat-George and S. Burtey, Kidney Int., 2013, 84, 733–744 CrossRef CAS PubMed.
- K. Atoh, H. Itoh and M. Haneda, Diabetes Res. Clin. Pract., 2009, 83, 220–226 CrossRef CAS PubMed.
- Y. Y. Zhao, H. T. Li, Y. L. Feng, X. Bai and R. C. Lin, J. Ethnopharmacol., 2013, 148, 403–410 CrossRef CAS PubMed.
- O. M. Duicu, R. Lighezan, A. Sturza, R. A. Ceausu, C. Borza, A. Vaduva, L. Noveanu, M. Gaspar, A. Ionac, H. Feier, D. M. Muntean and C. Mornos, BioMed Res. Int., 2015, 2015, 515437 Search PubMed.
- R. Lighezan, A. Sturza, O. M. Duicu, R. A. Ceausu, A. Vaduva, M. Gaspar, H. Feier, M. Vaida, V. Ivan, D. Lighezan, D. M. Muntean and C. Mornos, Can. J. Physiol. Pharmacol., 2016, 94, 1040–1047 CrossRef CAS PubMed.
- M. E. Manni, E. Bigagli, M. Lodovici, M. Zazzeri and L. Raimondi, Pharmacol. Res., 2012, 65, 465–471 CrossRef CAS PubMed.
- D. S. Goldstein, Y. Jinsmaa, P. Sullivan, C. Holmes, I. J. Kopin and Y. Sharabi, J. Pharmacol. Exp. Ther., 2016, 356, 483–492 CrossRef CAS PubMed.
- B. S. Kristal, K. E. Vigneau-Callahan, A. J. Moskowitz and W. R. Matson, Arch. Biochem. Biophys., 1999, 370, 22–33 CrossRef CAS PubMed.
- K. Sharma, B. Karl, A. V. Mathew, J. A. Gangoiti, C. L. Wassel, R. Saito, M. Pu, S. Sharma, Y. H. You, L. Wang, M. Diamond-Stanic, M. T. Lindenmeyer, C. Forsblom, W. Wu, J. H. Ix, T. Ideker, J. B. Kopp, S. K. Nigam, C. D. Cohen, P. H. Groop, B. A. Barshop, L. Natarajan, W. L. Nyhan and R. K. Naviaux, J. Am. Soc. Nephrol., 2013, 24, 1901–1912 CrossRef CAS PubMed.
- G. Macdonald, R. Asse, A. Guiffre and E. Lo, Clin. Exp. Pharmacol. Physiol., 1984, 11, 381–384 CrossRef CAS PubMed.
- S. S. Kulkarni, H. K. Karlsson, F. Szekeres, A. V. Chibalin, A. Krook and J. R. Zierath, J. Biol. Chem., 2011, 286, 34567–34574 CrossRef CAS PubMed.
- K. Rave, L. Nosek, J. Posner, T. Heise, K. Roggen and E. J. van Hoogdalem, Nephrol., Dial., Transplant., 2006, 21, 2166–2171 CrossRef CAS PubMed.
- R. Elvert, A. Wille, J. Wandschneider, U. Werner, H. Glombik and A. W. Herling, Diabetes, Obes. Metab., 2013, 15, 324–334 CrossRef CAS PubMed.
- B. Zambrowicz, P. Lapuerta, P. Strumph, P. Banks, A. Wilson, I. Ogbaa, A. Sands and D. Powell, Clin. Ther., 2015, 37, 71–82 CrossRef CAS PubMed e12.
- V. Perkovic, M. Jardine, U. Vijapurkar and G. Meininger, Curr. Med. Res. Opin., 2015, 31, 2219–2231 CrossRef CAS PubMed.
- J. Fang, W. Wang, S. Sun, Y. Wang, Q. Li, X. Lu, Z. Hao and Y. Zhang, RSC Adv., 2015, 5, 75612–75621 RSC.
- V. Iacobazzi and V. Infantino, Biol. Chem., 2014, 395, 387–399 CrossRef CAS PubMed.
- M. J. MacDonald, L. A. Fahien, L. J. Brown, N. M. Hasan, J. D. Buss and M. A. Kendrick, Am. J. Physiol., 2005, 288, E1–E15 CrossRef CAS PubMed.
|
This journal is © The Royal Society of Chemistry 2017 |
Click here to see how this site uses Cookies. View our privacy policy here.