Advantages and challenges associated with bisulfite-assisted nanopore direct RNA sequencing for modifications†
Received
31st May 2023
, Accepted 23rd August 2023
First published on 23rd August 2023
Abstract
Nanopore direct RNA sequencing is a technology that allows sequencing for epitranscriptomic modifications with the possibility of a quantitative assessment. In the present work, pseudouridine (Ψ) was sequenced with the nanopore before and after the pH 7 bisulfite reaction that yields stable ribose adducts at C1′ of Ψ. The adducted sites produced greater base call errors in the form of deletion signatures compared to Ψ. Sequencing studies on E. coli rRNA and tmRNA before and after the pH 7 bisulfite reaction demonstrated that using chemically-assisted nanopore sequencing has distinct advantages for minimization of false positives and false negatives in the data. The rRNA from E. coli has 19 known U/C sequence variations that give similar base call signatures as Ψ, and therefore, are false positives when inspecting base call data; however, these sites are refractory to reacting with bisulfite as is easily observed in nanopore data. The E. coli tmRNA has a low occupancy Ψ in a pyrimidine-rich sequence context that is called a U representing a false negative; partial occupancy by Ψ is revealed after the bisulfite reaction. In a final study, 5-methylcytidine (m5C) in RNA can readily be observed after the pH 5 bisulfite reaction in which the parent C deaminates to U and the modified site does not react. This locates m5C when using bisulfite-assisted nanopore direct RNA sequencing, which is otherwise challenging to observe. The advantages and challenges of the overall approach are discussed.
Introduction
The epitranscriptome is the collection of chemical modifications on RNA that are essential for the structure and stability of RNA, translation efficiency of mRNA, and recognition of self vs. non-self RNAs, and they are involved in nearly all other cellular functions of RNA.1,2 The epitranscriptome is a dynamic system under intense study, in which the benefits of future discoveries include an improved understanding of gene regulation, new targets for therapeutic intervention of disease, and personalized medicine when we understand the RNA modification signatures of particular diseases.1,2 Classically, modifications to RNA were found by harvesting cellular RNA and then completely digesting it with nucleases and phosphatase to its nucleoside components followed by TLC or LC-MS/MS analysis.3,4 These experiments have identified >140 base and sugar modifications on RNA polymers from all phyla of life. A drawback to the complete digestion of RNA to its nucleoside components is that the sequence information is lost.
Sequencing RNA with the goal of locating and ideally quantifying the modifications has been approached by many methods.5 The RNA can be reverse transcribed to a cDNA followed by high-throughput sequencing; modification-specific chemical reactions (e.g., CMC alkylation of pseudouridine) can be used for the introduction of a signature, such as a stop, in the cDNA revealed during sequencing. Immunoprecipitation of RNA targeting a specific modification can generate an enriched population of strands that is converted to cDNAs for sequencing to locate the modification.6 The RNA can undergo limited nuclease digestion followed by LC-MS analysis for sequencing modifications.7 Recently, direct sequencing of RNA for modifications has become possible with new technologies. The PacBio platform can directly sequence RNA and has the potential for locating modifications as was demonstrated for N6-methyladenine in RNA;8 however, it is nanopore direct RNA sequencing that has been applied to the greatest extent for modification-aware sequencing.6,9,10
The nanopore sequencer is a two-protein platform that uses an ATP-dependent helicase to deliver the RNA 3′ to 5′ into a lipid-bilayer-embedded protein nanopore under an electrophoretic force (Fig. 1A).11 As the nucleotides (nts) pass the central constriction zone of the nanopore protein they deflect the ionic current in a sequence-dependent fashion. In RNA, approximately 5 nts, referred to as a k-mer, contribute to the current level.12 The current vs. time data are then base called using a recurrent neural network trained on canonical nucleotides to reveal their identities. Thus, RNA modifications can yield signatures in the raw ionic current vs. time data and/or in the base-called data.13 There are many advances that this technology enables for epitranscriptomic studies but some challenges remain to be resolved.
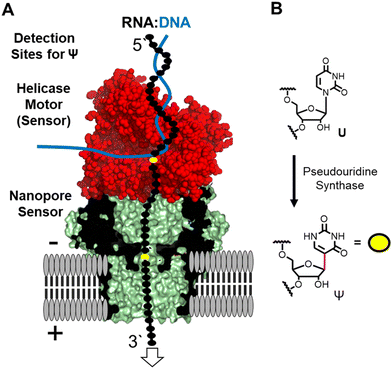 |
| Fig. 1 (A) The nanopore sequencer is used for nanopore direct RNA sequencing to locate the (B) uridine isomer pseudouridine in the helicase (red) and nanopore (green/black) proteins comprising the system. | |
Many different epitranscriptomic modifications have been sequenced with the nanopore system.14–17 The present discussion will focus on the uridine isomer pseudouridine (Ψ) because the prior work showcases the strengths and challenges of this approach (Fig. 1B).16,18–25 Pseudouridine is the most common modification in all RNA and is the second most commonly found in eukaryotic mRNA.26 There exist many writers for catalyzing the isomerization reaction with humans having 13 of these enzymes26 that can install Ψ in nearly any sequence context.27 In nanopore direct RNA sequencing for Ψ, this modification is “miscalled” as a C with the highest frequency, and miscalls to the other bases occur with lower frequencies16,18–25 Natural U/C sequence variations will present as Ψ in base-call data analyses. These base miscall signatures have allowed sequencing for Ψ directly in rRNA,17,18,25 mRNA,20,23,25 tRNA,14,15 and vRNA19 that can have lengths >5000 nts, demonstrating the ability for long-read modification-aware sequencing with this method. A challenge to using base-called data for quantitative analysis of Ψ is that the frequency of “miscalls” is sequence-context dependent.20,23,28 The sequencer has high overall error for RNA sequencing;29 therefore, a well-matched control void in the target modification is needed to make comparisons.12,17,23
Inspection of the raw data from the nanopore sequencer (i.e., ionic current vs. time traces) can provide data to bypass some of these challenges.18,19 The current levels do change for Ψ; however, the changes are sequence-context dependent similar to the base-call data, which is expected because the current level data is used for base calling, and to compound the issue, other U modifications can yield similar current-level differences.19,28 Our work and others revealed that the helicase stalls at Ψ sites that are found when analyzing the dwell time for this modification 10–11 nts before the nucleotide reaches the nanopore protein central constriction.19,24 We proposed using a consensus of base call, ionic current, and dwell time data as an approach for greater accuracy in Ψ sequencing, which we expanded to 16 of the 17 different chemical modifications found in E. coli rRNA.17,19
In the present report, we outline an alternative approach to use nanopore direct RNA sequencing to locate Ψ and differentiate these sites from a U/C sequence variation, to identify Ψ in sequence contexts that fail to give a strong miscall, and to provide a positive signal that can differentiate Ψ from other U modifications. The approach employs the bisulfite reaction at pH 7 to form stable Ψ adducts that upon nanopore direct RNA sequencing are revealed as an insertion–deletion (indel) signature.30 Additionally, the bisulfite reaction at pH 5 is the gold-standard method for sequencing 5-methylcytosine in DNA via deamination of the parent C base to uracil while the methylated base fails to react; therefore, we used this reaction to sequence for 5-methylcytidine (m5C) in RNA, which is challenging to achieve without the assistance of this reaction.16 The advantages and challenges of using modification-specific chemistry for nanopore direct RNA sequencing are discussed.
Results
Comparison of Ψ base call signatures in synthetic vs. biological k-mers
Total RNA comprised of >85% rRNA was purified from cultured E. coli or HCT116 human colon cancer cells to be used in nanopore direct RNA sequencing (Fig. S1, ESI†). To study the rRNA strands using the Oxford Nanopore Technologies (ONT) system, the strands were first poly-A tailed with a commercial poly-A tailing kit following the manufacturer's protocol. The poly-A-tailed RNAs were then used for library preparation following the reported protocol from ONT using the SQK-RNA002 direct RNA sequencing kit. Next, the RNA strands were sequenced on either the smaller Flongle or larger minION (v9.4.1) flow cells to obtain the ionic current vs. time reads using the standard settings for the selection of those that passed quality control (Q > 7). The passed reads were base called with Guppy 6.3.2, aligned to the references with minimap2 or BWA MEM,31,32 the alignment files were converted to BAM format and sorted with Samtools,33 and the results were visualized using Integrative Genome Viewer (IGV; Fig. S2 and S3, ESI†).34 From the IGV inspection, the base “miscalls” and indels were quantified for the plots provided. A pair of independent replicates for each sample, with and without the bisulfite reaction, were sequenced.
In the E. coli 16S and 23S rRNAs, there exist 10 Ψ sites, and in the human 5.8S, 18S, and 28S rRNAs there exist 103 Ψ sites (Fig. S4, ESI†).35,36 Nearly all of these have been verified by mass spectrometry to be present at high occupancy.35,37 In the first analysis, the base calling errors for all 113 Ψ sites were determined and plotted (Fig. 2A left). The error ranged from 17% to 100% that is consistent with previous studies reporting a large range of base calling errors associated with Ψ.19,23,25,28 Ribosomal RNA has many modifications, some of which are clustered closer than 5 nts apart, and the clustered modifications could present higher base calling errors than Ψ isolated in the same unmodified sequence contexts. Next, Ψ sites isolated in k-mers that do not also possess other modifications (n = 87) were inspected to find roughly the same range of base calling errors (Fig. 2A right). These data demonstrate that when the high occupancy Ψ sites in rRNA are sequenced with the nanopore, the base calling error is highly variable with dependency on the sequence context. The sequences that give low error would be false negatives when conducting de novo sequencing for Ψ.
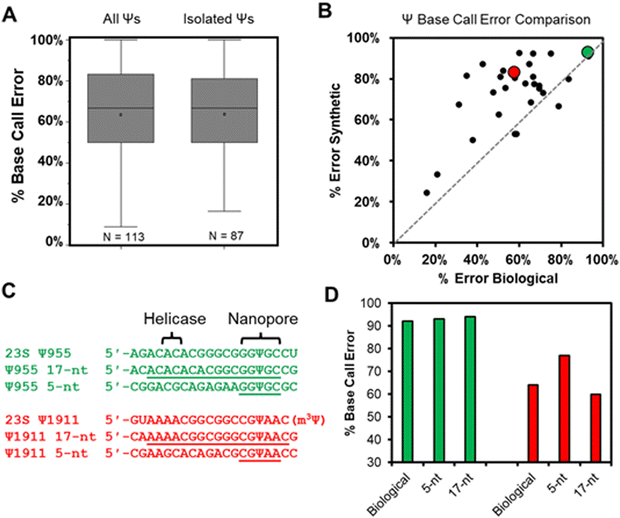 |
| Fig. 2 The base-call error profile for Ψ in cellular rRNA and synthetic RNA strands. (A) The base-call error profile for Ψ sites found in E. coli and human rRNA strands when all sites are considered that may have more than one modification per k-mer (left plot), and for those isolated as the only modification in the k-mer (right plot). (B) A plot that compares base-call error for Ψ in the same 5-nt k-mer in synthetic vs. biological RNA. The green data point is for E. coli 23S Ψ955 and the red data point is for E. coli 23S Ψ1911 that were studied further in the next two panels. (C) Sequences to explore how the inclusion of the sequence between the nanopore (k-mer) and helicase impacts base calling errors for Ψ. (D) The base-calling errors measured for the sequences in panel C. | |
Our next analysis compared the nanopore base miss calls for Ψ in cellular RNA against our previously published synthetic RNAs.28 The previous report synthesized RNA by in vitro transcription (IVT) with U or Ψ in all 5′-VV(U/Ψ)VV-3′ (where V ≠ U) sequence contexts.28 The sequences analyzed had the U/Ψ sites spaced >20 nts apart to interrogate the sequencer performance on individual modifications as they pass through the sensor rather than closely spaced modifications that could influence the signals from one another, an approach that has been reported in the literature.16,38 Out of the total 113 pseudouridinylation sites in the rRNAs from E. coli and humans, there exist 28 in 5-nt k-mer contexts that could be compared to the prior data set.28 The 28 sites in the rRNA used for the comparison were first corrected for the modification abundance on the basis of prior quantitative MS data (Fig. S4, ESI†).35,37 A plot of the base calling error for the synthetic 5-nt Ψ sequence contexts (y-axis) vs. the sequence-matched biological Ψ sites (x-axis) found a poor but positive trend existed between the datasets (Fig. 2B). The synthetic RNA strands predict a higher base calling error for Ψ than was found in the biological RNA.
We reasoned the base calling error differences between the synthetic RNA with Ψ vs. biological rRNA Ψ sites has to do with the 5-nt k-mer failing to reproduce the full complexity of the sequence between the helicase and the nanopore. The distance between the helicase active site and the k-mer centered in the central constriction zone of the nanopore is ∼11 nts (Fig. 1A); this distance is based on the helicase signature being 10–11 nts from the nanopore sensor for Ψ as previously reported.17,19,24 There exist two Ψ sites in the E. coli 23S rRNA (955 and 1911) in which the Ψ is in a sequence context spanning from the helicase active site to the 3′ side of the k-mer with only A, C, or G nucleotides, thus allowing the synthesis of these standards by IVT. We synthesized RNA to include 13 nts 5′ to the Ψ that should span slightly past the helicase active site and 3 nts 3′ to the Ψ to span 1 nt past the central constriction zone of the nanopore sensor where the k-mer resides (Fig. 2C).
The two sequence contexts selected are shown with either a green or red dot in Fig. 2B, in which the green one (23S Ψ955) represents a case that the synthetic 5-nt k-mer standard reproduced the biological data well, while the red one (23S Ψ1911) represents a case that the synthetic standard poorly reproduced the biological data (Fig. 2C). For the 23S Ψ955 data, extending the standard to include the sequence between the nanopore and helicase reproduced the base calling error obtained in the biological data similar to the 5-nt sequence (Fig. 2D, green; 5-nt standard = 93%, 17-nt standard = 94%, and biological = 92%). A point regarding 23S Ψ1911 is that there is an m3Ψ in this sequence at position 1915 that would have passed through the central constriction by the point at which 1911 is centered in this region; we acknowledge the synthetic system is not a perfect reproduction of the biological data. Nonetheless, when the sequence was extended, the 17-nt synthetic standard more closely represented the biological base calling error than the 5-nt standard (Fig. 2D, red; 5-nt standard = 77%, 17-nt standard = 60%, and biological = 64%). This example demonstrates that some sequence contexts require nanopore data with a much longer synthetic sequence standard that fully spans the region between the helicase and nanopore central constriction zone to reproduce biological nanopore data accurately. The utility of IVT to generate long control RNA with and without modifications has been used by us and others for comparisons to locate epitranscriptomic modifications;12,17,23 in the present work, an alternative approach for locating Ψ while minimizing the false positives and negatives is pursued. Use of chemical reagents to selectively label modifications can give rise to new sequencing signatures, and this method is employed in other studies for sequencing epitranscriptomic modifications.22,39–45
Bisulfite adducts to Ψ to minimize the nanopore signature sequence dependency
The bisulfite reaction conducted at pH 5 on DNA is the gold standard approach to locate 5-methyl-2′-deoxycytidine (5mC).46 The reaction induces 2′-deoxycytidine deamination to 2′-deoxyuridine (dU) while 5mC is resistant to deamination (Schemes 1A and B). Hence, 5mC is located by sites that continue to code like a dC, and the original dC sites now code like a thymidine (dU). This reaction has also been applied to find 5-methylcytidine (m5C) in RNA;41 however, the structured nature of RNA has led to concerns regarding the extent of bisulfite converting C to U nucleotides under the pH 5 reaction conditions.42 Our previous work used the bisulfite reaction to form stable ring-opened ribose adducts at pH 5 to Ψ in RNA that were revealed after reverse transcription to a cDNA via a deletion signature at the site in the sequencing data.42 Our initial report could sequence for Ψ as well as m5C and m1A in a single sequencing experiment, which significantly expands the number of modifications to be analyzed at one time. In our previous work, the chemical structures and mechanism of the bisulfite reaction with Ψ were investigated, which revealed two constitutional isomer adducts, each with a single diastereomer at the C1′ position.30 The adducts are the (R) stereoisomer attached via an oxygen atom of bisulfite ((R)-O adduct) or the (S) stereoisomer attached via the sulfur atom of bisulfite ((S)-S adduct; Scheme 1C). The bisulfite adducts to Ψ are collectively referred to as Ψ-(SO3−) adducts. This reaction can be Ψ selective by simply changing the conditions to pH 7, in which C fails to deaminate but Ψ continues to react.39,40,47 The ratios for the O- to S-adducts are 2
:
1 at pH 5 and 9
:
1 at pH 7.47 This method was employed by the He laboratory in the development of BID-seq and the Yi laboratory in the development of PRAISE sequencing for the quantitative sequencing of Ψ in human RNA using Illumina sequencing of amplified cDNA as the final read-out.39,40 At pH 7, bisulfite reaction conditions are reported that result in near quantitative conversion of Ψ to a bisulfite adduct leading to quantitative sequencing in singly and multiply modified sites in mammalian transcriptomes.39,40 The success of this reaction in our laboratory and others led us to consider using bisulfite adducts at Ψ as a means to differentiate the modification from the parent U by a substantial change in structure so that nanopore direct RNA sequencing would readily identify Ψ, independent of sequence. This would demonstrate the feasibility of using modification-specific chemical reactions as a tool for inducing signatures at modification sites that are hard to identify in the nanopore data.
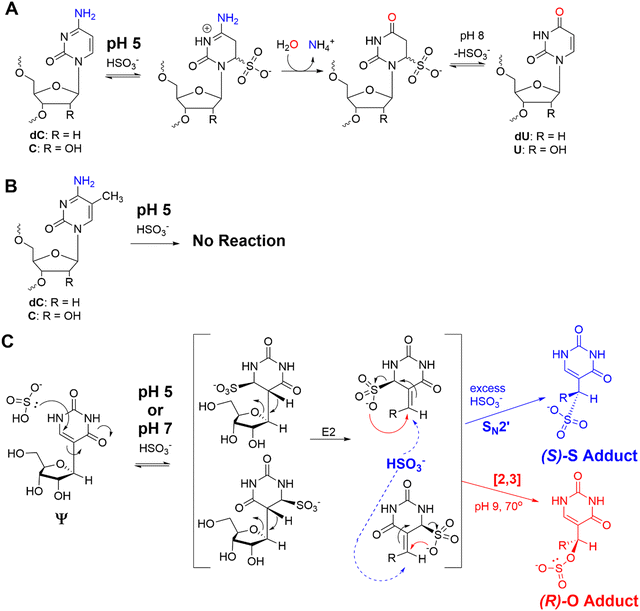 |
| Scheme 1 (A) The bisulfite reaction at pH 5 results in dC/C deamination while (B) 5mC/m5C is refractory to the reaction. (C) The products formed when Ψ is allowed to react with bisulfite at pH 5 or 7 to yield stable, ring-opened ribose adducts. | |
First, IVT-generated RNA strands with 14 model U/Ψ sites in different sequence contexts spaced >20 nts apart were synthesized and nanopore sequenced (Fig. S1, ESI†). These RNAs were successfully analyzed using the method described above that is standard for the nanopore direct RNA sequencing field.19 The Ψ-containing RNA strands were then subjected to the bisulfite reaction at either pH 5 or 7 under the reported conditions to generate stable Ψ-(SO3−) adducts consistent with our previous reports on this reaction (Fig. S5, ESI†).30,42 The alignment reference for the pH 5 reaction replaced the C nucleotides with U nucleotides because of the deamination that occurs under these conditions, while the data for the pH 7 reaction used the original alignment reference with C nucleotides. Determination that the adducts could pass through the helicase and nanopore sensors was first demonstrated using FastQC analysis of the passed reads from the sequencer to determine the mean read lengths, G:C content, and quality of the data. The Ψ-(SO3−) adducted RNA strands produced a similar population of read lengths as the U- and Ψ-containing RNAs, the read accuracy average decreased from Q20 for the U-containing RNA to Q12 for the Ψ- and Ψ-(SO3−) RNAs, and the %GC for the modified RNAs differed from the U-containing RNA in the expected direction based on our knowledge of the reactions (Fig. S6, ESI†). Next, we found that using minimap2 as the aligner software failed to map to the reference sequences. However, when the aligner software was changed to BWA MEM the mapped reads increased from the previous 0% to 42% (Fig. S2, ESI†). This change in the aligner program has been used to increase the mapped nanopore direct RNA sequencing reads for hypermodified tRNA to the reference sequences.14,15 For Ψ-containing synthetic RNA, the bisulfite reaction at pH 5 or 7 produced similar results, and those for pH 7 will be discussed.
The base calling error was analyzed using ELIGOS2, which reports an error of specific bases (ESB) for sites of interest;16,48 this computational tool also inspects base calling data for a modified RNA against a matched unmodified RNA to provide a statistical prediction of whether a modification resides at a target position or not. We used both features of the algorithm. Other programs exist for running base-calling error analysis to locate RNA modifications;20,25 however, they were not used in the present studies. When inspecting the ESB values from three nucleotides before and after the U, Ψ, or Ψ-(SO3−) sites (X in Fig. 3A and Fig. S7, ESI†), the ESB values were observed to increase from a low for the U sites, to a midrange value for Ψ sites, to a maximal value for Ψ-(SO3−) sites (Fig. 3A and Fig. S7, ESI†). The ESB values for Ψ in the contexts studied ranged from 0.2–0.9, while the Ψ-(SO3−) adducts gave ESB values ranging from 0.8–0.9; this suggests the sequence context effect on the error has been minimized for Ψ-(SO3−) adduct compared to Ψ, and in the sequence contexts studied, the adduct produced high base call errors generally in the form of deletion signatures that are reported as indels.
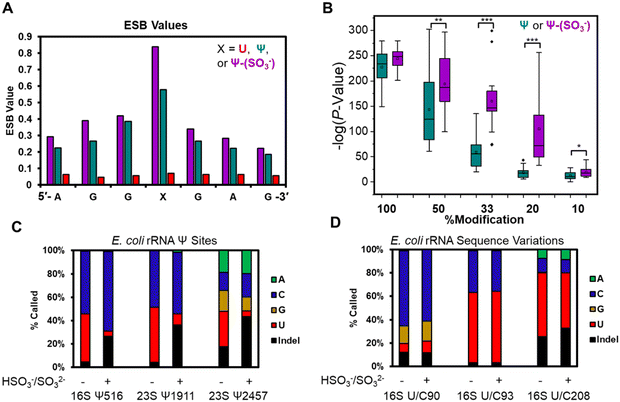 |
| Fig. 3 Bisulfite adducts to Ψ have greater base-calling error than Ψ, allowing detection of the modification to lower levels. (A) Example plot of the ESB values for U, Ψ, and a Ψ-(SO3−) adduct in a 7-nt sequence context. (B) Virtual titration followed by ELIGOS2 analysis to identify the lower limit of detection for Ψ and the Ψ-(SO3−) adducts in 14 sequence contexts. Statistical analysis was conducted using the Student's t-test with * = P value <0.05, ** = P value <0.01, and *** = P value <0.001. (C) Base-calling profiles for three established Ψ sites in E. coli rRNA before and after the bisulfite reaction at pH 7 to demonstrate that after adduct formation, the base-call error increases. (D) Base-calling profile for established U/C sequence variations before and after the bisulfite reaction yield nearly identical error profiles. This final study provides a method to differentiate sequence variations that masquerade as a Ψ (i.e., false positives) from real Ψ sites. | |
The U-containing RNA reads were mixed with known ratios of Ψ-containing or Ψ-(SO3−)-containing RNA (50%, 33%, 20%, or 10%) followed by ELIGOS2 analysis to determine the P-value of significance for a modification at the target sites (Fig. S8, ESI†). This examination provides insight into the bisulfite adduct to allow monitoring of sub-stochiometric levels of Ψ in the contexts studied. The P-values were negative-log transformed for visualization and plotted as box and whisker plots (Fig. 3B). At 50% occupancy of Ψ or Ψ-(SO3−), ELIGOS2 returned high values of significance for the presence of the modifications. When the level of the modifications was 20% or 33%, the significance levels reported for Ψ-(SO3−) were much greater than those for Ψ. At 10% modification, neither was predicted to be significantly modified, which is about the same level as the reported error for nanopore direct RNA sequencing.29 The bisulfite adducts to Ψ on these synthetic sequences increased the ability to detect Ψ down to ∼20% occupancy, which is lower than is possible for direct sequencing of Ψ based on the analysis (Fig. 3B).
Next, E. coli rRNA were allowed to react with bisulfite at pH 7 followed by nanopore sequencing and data analysis as described above. The 10 well-established Ψ sites were inspected for base calling error before and after the reaction to find the error increased after reaction (Fig. 3C and Fig. S9, ESI†). For example, 16S Ψ516 and 23S Ψ1911 before the reaction were base called to yield a nearly 1
:
1 ratio of C
:
U bases, and after bisulfite reaction, the ratio of U calls decreased to <10%, the C base calls stayed the same or increased, and the indel frequency increased to ∼30%. Similarly, 23S Ψ2457 before the reaction was base called as A, C, G, U, and indels, and after the reaction the U call decreased to <20% and the indels increased to >40%. The 23S Ψ2457 site before and after reaction was base called with all possible options (A, C, G, U, and indels) and one possible reason for this could be the dihydrouridine at 2449 that would influence the data because it is positioned between the nanopore and helicase when Ψ2457 is in the nanopore (Fig. 1A). The other seven Ψ sites in the E. coli rRNAs were inspected and those for which enough data was present gave similar results (Fig. S9, ESI†). These observations demonstrate on a biological sample that Ψ-(SO3−) adducts yield larger errors than Ψ or U.
The E. coli genome possesses 7 operons for expression of the rRNA strands, in which there are 62 known sequence variations of which 19 are U/C variants.17 The sites of U/C variation give high predicted values for Ψ occupancy when using base-calling error against a reference sequence with U at the variation sites instead of C (Fig. S10, ESI†). In Fig. 3D are the variant sites in the 16S rRNA at positions 90, 93, and 208. Position 16S 93 and 208 had U
:
C ratios that did not change before or after the reaction. The difference in ratios between the two sites is expected based on the frequency of this variation across the seven rRNA operons and the expression levels of the rRNAs in the cell when the RNA was harvested.17 Next, position 90 was predominantly called as a C, as expected,17 with lower levels of G and indels found that were similar before and after the reaction. This site resides centered in a homopolymer run of U nucleotides, which are known to be error prone when sequenced with the nanopore,29 as observed. Overall, this demonstrates the utility of using the bisulfite reaction for differentiation of U/C variant sites vs. Ψ sites because the variants failed to react with bisulfite at pH 7 while Ψ did react and showed a change in the base calling profile (Fig. 3C and D).
In a final study to demonstrate the utility of chemical labeling to enhance nanopore sequencing signatures, we sequenced the 363-nt tmRNA (a.k.a. 10Sa or SsrA) made by E. coli cells that functions both as a tRNA and an mRNA for labeling proteins translated without a stop codon.7 This RNA allows marking these failed proteins with an 11 amino acid tag on the C terminal end to target them for proteolysis. Prior MS and gel analysis found the tmRNA to have three modifications in the T-loop of the tRNA portion of this RNA.7 There resides a highly modified 5-methyluridine (m5U) at position 341, at 342 is a Ψ at high occupancy (>90%), and at 347 resides a lower occupancy Ψ (∼10%) predicted by gel analysis following a CMC-induced reverse transcriptase stop (Fig. 4A). Nanopore direct RNA sequencing of the tmRNA before and after the pH 7 bisulfite reaction provided base-calling data to confirm the presence of both Ψ sites in the T-loop. For the high occupancy Ψ342 site before the labeling reaction it was called as a C ∼90% of the time and after the reaction this site was called as a C or indel in nearly a 1
:
1 ratio; these data support the high penetrance of Ψ at position 342. As for position 347, before the reaction, a U was called with ∼90% frequency, and after the reaction indels were observed with an ∼25% frequency with the remainder comprised of U calls. This predicts ∼25% occupancy of Ψ at this site. This nanopore experiment predicts more Ψ at position 347 than the gel-based analysis by ∼2-fold.7 Reasons for the differences observed include the fact that the CMC reaction to locate Ψ requires treating the strands at high pH (10) and 37 °C for 3 h that results in highly degraded RNA due to its sensitivity to strand break formation under these conditions; this is why CMC sequencing for Ψ is poorly quantitative and often irreproducible resulting in bisulfite-based sequence for these sites to prevail as a better chemical reaction.39 Secondly, the E. coli previously studied and those in the present study are different strains grown under slightly different conditions. These differences likely explain the ∼2-fold difference in the occupancy of Ψ347 in the E. coli tmRNA. This result at position 347 supports the conclusion of control studies that found this approach could detect Ψ down to ∼20% (Fig. 3B). A final point regarding the pH 7 bisulfite reaction is the other nucleotides in this region (A, G, C, U, and m5U) were sequenced similarly before and after the reaction demonstrating the high degree of selectivity of the bisulfite reaction for Ψ at pH 7.
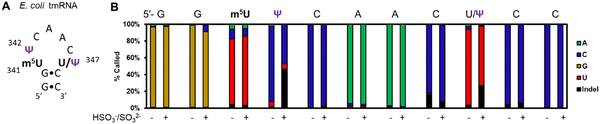 |
| Fig. 4 Nanopore direct RNA sequencing before and after the pH 7 bisulfite reaction can detect low and high occupancy Ψ sites in E. coli tmRNA. (A) The hairpin loop containing the only modifications in the tmRNA.7 (B) The base-calling data for the nucleotides comprising part of the hairpin stem and the entire loop where the modifications reside in this RNA before and after the pH 7 bisulfite reaction. | |
At pH 5, bisulfite reacts with RNA to reveal m5C sites and amplify the 5-hydroxymethylcytidine (hm5C) signature
The bisulfite reaction at pH 5 offers the opportunity to study m5C, a challenging RNA modification to find when using nanopore direct RNA sequencing. Its only difference to C is a small increase in indels at the site,16,17,25 and this is not an appealing way to find m5C in nanopore data. On the other hand, m5C sites could be readily revealed by the bisulfite reaction at pH 5 to deaminate the C nucleotides to U, while the m5C nucleotides do not react (Scheme 1A and B). Sequencing would then look for sites that differentially code as U (i.e., C) or C (i.e., m5C) to find the modifications. Additionally, 5-hydroxymethylcytidine (hm5C) reacts with bisulfite to form a stable base adduct (cytidine-5-methylsulfonate (CMS)) that was previously proposed as a way to find hm5C in nanopore data.49–51 Thus, we exposed IVT-generated RNA containing eight sequence contexts with m5C or hm5C to bisulfite at pH 5 followed by nanopore direct RNA sequencing. The reference sequence used before the reaction had a C at the position of interest, while the data after the reaction had a reference with U at the site of interest, which follows the convention for analyzing pH 5 bisulfite-treated RNA;42 therefore, it is expected C will give a low base-call error before and after the reaction, m5C will yield a low base-call error before the reaction and high error after the reaction, and hm5C is known to give intermediate error before the reaction,16 and after the reaction we hypothesize high base-call error will be found.
For C before the reaction we found a low error of ∼10% (Fig. 5A), and after bisulfite assisted deamination the error remained below 20% (Fig. 5B). The slightly higher base-call error after the pH 5 bisulfite reaction was comprised of ∼10% of C calls as a result of incomplete reaction, and ∼10% indels that were present before the reaction. For m5C, the base-call error was <25% before the bisulfite reaction (Fig. 5C), as expected,16,17,25 and after the reaction, the error was >70% (Fig. 5D). After the reaction, the m5C sites did not yield quantitative levels of error that likely results from low conversion of m5C deamination to m5U under these conditions. For hm5C, before the bisulfite reaction the base-call error was <40% (Fig. 5E), and after the reaction the sites gave >80% base-calling error to readily reveal these sites in RNA (Fig. 5F). The bisulfite reaction at pH 5 for m5C and hm5C is a viable way to differentiate these two modifications from the parent C nucleotide (Fig. S9, ESI†). These data suggest quantification of the reaction will be challenging without further optimizations. Studies on biological RNA were not explored.
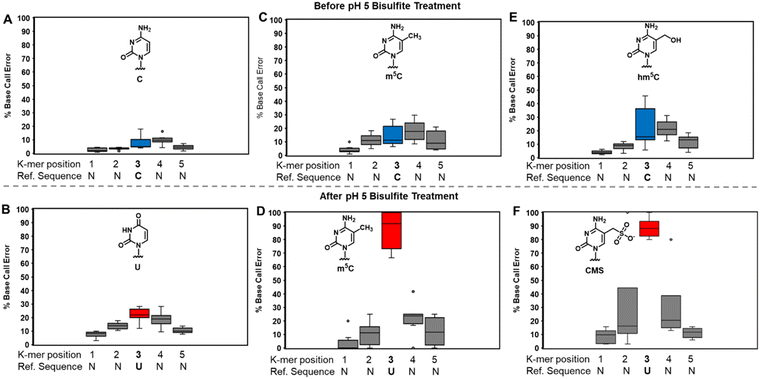 |
| Fig. 5 The pH 5 bisulfite reaction on RNA provides changes at C, m5C, and hm5C to reveal their presence in nanopore direct RNA sequencing data when analyzing base-calling error. The analysis inspected 8 different k-mers in IVT generated RNA before or after pH 5 bisulfite treatment for (A) and (B) C, (C) and (D) m5C, and (E) and (F) hm5C. | |
Discussion
Nanopore direct RNA sequencing for epitranscriptomic modifications has significantly grown in interest.14–25 The present report focused on sequencing the U isomer Ψ, and the C5 modified C nucleotides m5C and hm5C. Pseudouridine is written in virtually any sequence context when considering the full collection of pseudouridine synthases.27 The present work initially compared synthetic RNA strands with Ψ in a large number of 5-nt k-mers that were sequenced as benchmarks for comparison to biological samples. Comparing 28 rRNA Ψ sites found in E. coli and humans against synthetic standards in 5-nt k-mers, we found in general a >10% difference between the experimental and standard data (Fig. 2B). This points to a challenge to compare synthetic RNA strand standards to biological data for the detection and quantification of Ψ at sites of interest. This challenge likely will be faced with any of the >140 chemical modifications written into RNA. With one example, we demonstrated that including the sequence context between the nanopore and helicase (17 nts) can provide a better representation of the nanopore base-calling data than the 5-nt k-mer (Fig. 2D).
At present, two methods have been proposed to synthesize RNA standards null in modifications for comparison to biological datasets; one approach requires the ligation of a solid-phase-synthesized RNA to longer IVT-generated RNA strands, one sequence at a time,23,52 and the other uses cellular RNA for reverse transcription and PCR to yield DNA with the T7 promoter sequence to then re-synthesize RNA via IVT without modifications for comparison.12 Both approaches have their strengths and weaknesses. Synthesis of one mRNA modification at a time is very low throughput but can provide a well-defined control. The use of cellular RNA for cDNA synthesis to remake the RNA without modifications provides the best reproduction of a cell's RNA landscape that includes all the RNAs present and the cell-specific alternative splice forms of mRNA. However, this approach introduces reverse transcription errors particularly at some natural RNA modifications which are the sites of interest in epitranscriptomic studies (e.g., RNA base editing).53,54 Another approach for identification of RNA modifications in nanopore data is to study the RNA from cells with and without writer knockouts.21,38 This works well if the writer is known and knocking out the writer does not cause other biological impacts that interfere with the analysis.55,56
An appealing alternative is the application of chemical reagents to selectively modify target nucleic acid modifications, altering the nanopore signature, and it has been proposed by us and others.22,45,48,57,58 The bisulfite reaction at pH 7 to furnish stable adducts to Ψ is advantageous because the reaction conditions are fairly mild resulting in low degradation of the RNA (e.g., see Fig. 4B),39,47 unlike other approaches.27 The Ψ-(SO3−) adduct can minimize the sequence dependency in the nanopore base-calling errors (Fig. 3), which in turn minimizes the need for synthesizing such large libraries of benchmarking RNA strands for comparison to biological data. We studied E. coli rRNA as a test case for the bisulfite reaction and found the Ψ-(SO3−) adducts in many of the 10 Ψ sites produced enhanced base-calling error signatures (Fig. 3B and Fig. S9, ESI†); however, not all were able to be studied because the reaction did result in fewer successful sequence alignments as previously stated, which may result from the few clustered Ψ sites in the E. coli rRNAs. We did attempt the pH 7 bisulfite reaction on human rRNA followed by nanopore sequencing, but the results failed to align, likely as a consequence of the high density of Ψ adducts in this RNA failing to traverse and/or be analyzed by the nanopore sequencer; further studies were not conducted on these RNAs. The second demonstration of the reaction was to locate the high and low occupancy Ψ residues in E. coli tmRNA (Fig. 4). A key demonstration in this study was the low occupancy Ψ at position 347 before reaction was read predominantly as a U nucleotide, that is a false negative. This is likely because this Ψ is in a pyrimidine-rich k-mer (5′-ACΨCC) and resides at low occupancy. On the other hand, the bisulfite adduct created an indel signature that could be readily observed (Fig. 4B). This analysis demonstrates bisulfite adducts to Ψ can yield new signals to follow modification in k-mers where Ψ continues to code like a U. Application of this chemistry and nanopore sequencing on mRNA could result in success for detection and possibly quantification of Ψ sites via Ψ-(SO3−) adducts that change with cellular cues.17,25 Lastly, the bisulfite reaction at pH 5 can be used to locate both Ψ and m5C/hm5C (Fig. 3–5);42 however, a limitation to this approach is that the C nucleotides are converted to U nucleotides, which reduces the sequence complexity of the reads resulting in challenges for reference alignment.42,46
The Ψ-(SO3−) adduct additionally can differentiate U/C sequence variation sites that will give a mixture of U and C calls similar to a bonafide Ψ site (Fig. S10, ESI†). Naturally existing U/C variations will be false positives for Ψ. These natural variations are refractory to reacting with bisulfite and do not change when comparing sequencing data before and after the reaction (Fig. 3C); in contrast, Ψ sites will have altered base-calling behavior after the reaction to reveal them as actual modification sites (Fig. 3D). The present studies inspected the established U/C sequence variations found between the 7 operons for the rRNA strands in E. coli that have a 99.6% similarity.59 The recent release of the complete human genome sequence identifies humans have on average ∼400 ribosomal DNA sequences spread across multiple chromosomes that have sequence similarities ranging from 99.4–99.7%;60 the key point is human ribosomal RNA will harbor many false positive Ψ sites as a consequence of the U/C variations that naturally exist in the sequence. Other sources of T/C sequence variation include C-to-U editing in mRNA,61 and natural U/C variations in coding portions of the genome.62 The bisulfite reaction at pH 7 to label Ψ provides a method to differentiate sequence variations from RNA modifications when inspecting nanopore direct RNA base-calling data.
The use of chemical tools for site-selective labeling for RNA modifications can introduce challenges. The reactions must be highly selective and not cause degradation of the fragile RNA strand, and bisulfite chemistry at pH 7 fits these requirements; however, our data on the E. coli rRNA do show decreased alignment success suggesting some degradation or too many adducts on the strand resulted in decreased alignment to the reference (Fig. S3; ESI†). The data reported found that the Ψ-(SO3−) adduct when passing through the nanopore sensor yields current levels that the base-calling algorithm fails to call, which was observed as the high indel frequencies reported (Fig. 3). We were curious what the features of the raw current vs. time traces were that led to the loss in ability to base call these sites. Our analysis of two different sequence contexts found that when the Ψ-(SO3−) adduct passed through the nanopore sensor the current levels were noisier in ∼50% of the events compared to those of the unadducted nucleotides (Fig. S11; ESI†). This is problematic because the available software for the analysis of nanopore data filters this data out (Fig. S11; ESI†), likely as a consequence of these traces presenting raw data similar to that coming from failing nanopores. Future work in the software domain will be required for additional analysis of these adducts to Ψ and likely other larger adducts naturally or synthetically placed on the RNA nucleotides. The noisy feature may also have a benefit for development of machine learning tools to look at nanopore direct RNA sequencing data to find Ψ, especially in sequence contexts in which Ψ is called as a U (Fig. 4E. coli tmRNA Ψ347).
Chemical tools for site-specific labeling of Ψ expand past bisulfite to include the carbodiimide CMC that yields a stable N3 adduct to Ψ after a two-step reaction conducted under alkaline conditions known to degrade RNA.27,63 The CMC-Ψ adduct is likely too big (MW: bisulfite = 80; CMC = 252) to be successfully sequenced with the nanopore, in addition to the RNA degradation issue. Acrylonitrile and methyl vinyl sulfone are alternative Ψ alkylators for which the acrylonitrile adducts have been analyzed by nanopore sequencing;22,64 however, these reagents can also react with inosine3,64 and 4-thiouridine,65 and therefore, they are not site-selective reagents. There exists a library of chemical tools with varying degrees of selectivity for reacting with RNA modifications;66 these may become part of the toolbox for mapping RNA modifications in nanopore direct RNA sequencing data. As demonstrated in the present studies, site-selective chemical modification of Ψ provides data that minimizes or eliminates false positives at U/C sequence variation sites and false negatives at Ψ sites that code like U. Future advances in computational tools will need to occur to fully release the potential of using chemical tools to advance nanopore sequencing for epitranscriptomic modifications.
Experimental
RNA synthesis by in vitro transcription
In vitro transcription was performed using the MEGAscript T7 transcription kit (Thermo Fisher) according to the manufacturer's instructions. The duplex DNA templates for the IVT reactions were synthesized via commercial sources to have a T7 promoter for initiation of transcription and ended with a poly-A tail for sequencing library preparation (Fig. S1, ESI†). The IVT reactions were incubated for 6 h at 37 °C in a PCR thermocycler. After the incubation, DNase I treatment was performed on all samples at 37 °C, followed by purification using Quick Spin Columns for RNA purification (Sigma). To install Ψ, IVT was conducted in the presence of commercially available pseudouridine-5′-triphosphate (ΨTP; Trilink Biotechnologies with purities >99%) instead of UTP. Success in synthesizing the RNA transcripts was verified by agarose gel electrophoresis by comparison to a ladder of known lengths (Fig. S1, ESI†).
Cell growth and total RNA extraction
The E. coli DH5α cells (NEB) expressing a plasmid with an ampicillin resistance gene were grown as previously reported in LB media at 37 °C for 20 h.17 The colorectal carcinoma cells (HCT116) were obtained from ATTC. The cells were grown at 37 °C in Dulbecco's Modified Eagle Medium with 1× glutamax, 1× nonessential amino acids, 10% FBS, and 20 μg mL−1 gentamicin. The cells were grown to ∼80% confluency before pelleting. The total RNA from both cells was extracted using the Quick-RNA Miniprep Kit (Zymo Research) following the manufacturer's protocol. The RNA concentration was determined by Qubit analysis, and it was stored at −80 °C until ready for sequencing library preparation.
Bisulfite reaction conditions
The bisulfite reactions were conducted at pH 5 or 7 following prior reports as a guide.39,47 For the pH 5 reaction, the RNA (10 μg at 1 μg μL−1) was allowed to react with 3 M freshly prepared NaHSO3 (pH 5) at 50 °C for 10 h. For the pH 7 reaction, the RNA (10 μg at 1 μg μL−1) was allowed to react with 3 M freshly prepared NaHSO3/Na2SO3 (pH 7) at 70 °C for 3 h. The reacted strands were purified from the reacting salt using a GeneJET PCR cleanup kit (Thermo Scientific) following the manufacturer's protocol. The bisulfite adducted RNA strands were then desulfonated for 1 h at 37 °C in pH 9 buffer (20 mM Tris and 1 mM EDTA). Following the reaction, the RNA strands were again purified using the GeneJET PCR cleanup kit as described above and were stored at −80 °C prior to library preparation for sequencing.
Nanopore library preparation and sequencing
The total RNA from the cells was first poly-A tailed using a poly-A tailing kit following the manufacturer's protocol (Life Sciences Technologies). The IVT-generated RNA strands were designed to have a poly-A tail for library preparation. The poly-A tailed RNA strands were then library prepared using the direct RNA sequencing kit (SQK-RNA002) from Oxford Nanopore Technologies (ONT). The protocol was followed without changes and the library-prepared samples (1–5 ng) were directly used for sequencing. The samples were applied to the ONT Flongle or minION flow cells running the R9.4.1 chemistry following the manufacturer's protocol. The default settings were used with passed reads having a Q score greater than 7.
Data analysis
The ionic current vs. time traces in fast5 file format passed by the sequencer were base called using guppy v.6.3.8 to obtain the fastq sequencing read files used in the subsequent data analyses. The FastQC analysis was conducted on the reads with Q > 7.67 The fastq files were aligned to the reference sequences using minimap231 with the command line ‘-ax map-ont L’. For the BWA MEM32 alignments, the fastq files were first converted to DNA sequences with the fastx toolkit68 followed with alignment using the command line ‘-W13 k6 xont2d’. The aligned files were converted to bam format using Samtools. The bam file alignment statistics were determined with the flagstat function in Samtools33 and then the files were indexed with Samtools for visualization with IGV34 to obtain the base call information at the modification sites. The ELIGOS2 tool was used following the GitLab page reported for this tool.16 To study ELIGOS2 performance at lower levels of the Ψ or Ψ-(SO3−) adducts, a population of reads for U-containing RNA and the modified RNA strands were aligned to the reference followed by Samtool flagstat function to determine the alignment counts for each. Next, the known reads were mixed in predetermined ratios and then submitted to ELIGOS2 analysis for P-value prediction of the modification presence at each site of interest. The Tombo and Nanopolish tools were used as previously described.17 For the raw current-level analysis, the raw data stored in fast5 file format were opened with and extracted from HDView (v. 2.14.0). The digitized data were converted to current in pA vs. time in msec following a reported method.69 The data were plotted and analyzed in either python, Origin, or Excel for visualization.
Data availability
The base-called data are available on the Zenodo public repository for data, in which the E. coli rRNA before bisulfite treatment data were previously reported and are at DOI: https://doi.org/10.5281/zenodo.7746124,17 the data for the 5-nt k-mer stands with U or Ψ were previously reported and are at DOI: https://doi.org/10.5281/zenodo.7459451,28 and the human rRNA and bisulfite treated RNA sample data are new to this report and located at DOI: https://doi.org/10.5281/zenodo.7991319. These data are searchable in the OpenAIRE explorer.
Conflicts of interest
A. M. F. and C. J. B. have a patent licensed to Electronic BioSciences for nanopore sequencing.
Acknowledgements
The research was supported by the National Institute of General Medical Sciences grant no. R35 GM145237.
References
- M. M. Pomaville and C. He, Advances in targeting RNA modifications for anticancer therapy, Trends Cancer, 2023, S2405–8033, 00059 Search PubMed.
- K. Athanasopoulou, G. N. Daneva, M. A. Boti, G. Dimitroulis, P. G. Adamopoulos and A. Scorilas, The transition from cancer “omics” to “epi-omics” through next- and third-generation sequencing, Life, 2022, 12, 2010 CrossRef CAS PubMed.
- J. D. Jones, J. Monroe and K. S. Koutmou, A molecular-level perspective on the frequency, distribution, and consequences of messenger RNA modifications, Wiley Interdiscip. Rev.: RNA, 2020, e1586 CrossRef CAS PubMed.
- D. Su, C. T. Chan, C. Gu, K. S. Lim, Y. H. Chionh, M. E. McBee, B. S. Russell, I. R. Babu, T. J. Begley and P. C. Dedon, Quantitative analysis of ribonucleoside modifications in tRNA by HPLC-coupled mass spectrometry, Nat. Protoc., 2014, 9, 828–841 CrossRef CAS PubMed.
- S. Zaccara, R. J. Ries and S. R. Jaffrey, Reading, writing and erasing mRNA methylation, Nat. Rev. Mol. Cell Biol., 2019, 20, 608–624 CrossRef CAS PubMed.
- Y. Motorin and V. Marchand, Analysis of RNA modifications by second- and third-generation deep sequencing: 2020 update, Genes, 2021, 12, 278 CrossRef CAS PubMed.
- B. Felden, K. Hanawa, J. F. Atkins, H. Himeno, A. Muto, R. F. Gesteland, J. A. McCloskey and P. F. Crain, Presence and location of modified nucleotides in Escherichia coli tmRNA: structural mimicry with tRNA acceptor branches, EMBO J., 1998, 17, 3188–3196 CrossRef CAS PubMed.
- I. D. Vilfan, Y.-C. Tsai, T. A. Clark, J. Wegener, Q. Dai, C. Yi, T. Pan, S. W. Turner and J. Korlach, Analysis of RNA base modification and structural rearrangement by single-molecule real-time detection of reverse transcription, J. Biotechnol., 2013, 11, 8 CAS.
- M. C. Lucas and E. M. Novoa, Long-read sequencing in the era of epigenomics and epitranscriptomics, Nat. Methods, 2023, 20, 25–29 CrossRef CAS PubMed.
- M. Jain, R. Abu-Shumays, H. E. Olsen and M. Akeson, Advances in nanopore direct RNA sequencing, Nat. Methods, 2022, 19, 1160–1164 CrossRef CAS PubMed.
-
D. Branton and D. Deamer, Nanopore Sequencing An Introduction, World Scientific Publishing Co. PTE. Ltd, 2019 Search PubMed.
- R. E. Workman, A. D. Tang, P. S. Tang, M. Jain, J. R. Tyson, R. Razaghi, P. C. Zuzarte, T. Gilpatrick, A. Payne, J. Quick, N. Sadowski, N. Holmes, J. G. de Jesus, K. L. Jones, C. M. Soulette, T. P. Snutch, N. Loman, B. Paten, M. Loose, J. T. Simpson, H. E. Olsen, A. N. Brooks, M. Akeson and W. Timp, Nanopore native RNA sequencing of a human poly(A) transcriptome, Nat. Methods, 2019, 16, 1297–1305 CrossRef CAS PubMed.
- M. Furlan, A. Delgado-Tejedor, L. Mulroney, M. Pelizzola, E. M. Novoa and T. Leonardi, Computational methods for RNA modification detection from nanopore direct RNA sequencing data, RNA Biol., 2021, 18, 31–40 CrossRef CAS PubMed.
- N. K. Thomas, V. C. Poodari, M. Jain, H. E. Olsen, M. Akeson and R. L. Abu-Shumays, Direct nanopore sequencing of individual full length tRNA strands, ACS Nano, 2021, 15, 16642–16653 CrossRef CAS PubMed.
- M. C. Lucas, L. P. Pryszcz, R. Medina, I. Milenkovic, N. Camacho, V. Marchand and Y. Motorin, Ribas de Pouplana, L.; Novoa, E. M. Quantitative analysis of tRNA abundance and modifications by nanopore RNA sequencing, Nat. Biotechnol., 2023 DOI:10.1038/s41587-023-01743-6.
- P. Jenjaroenpun, T. Wongsurawat, T. D. Wadley, T. M. Wassenaar, M. Trudy, J. Liu, Q. Dai, V. Wanchai, N. S. Akel, A. Jamshidi-Parsian, A. T. Franco, G. Boysen, M. L. Jennings, D. W. Ussery, C. He and I. Nookaew, Decoding the epitranscriptional landscape from native RNA sequences, Nucleic Acids Res., 2020, 49, e7 CrossRef PubMed.
- A. M. Fleming, P. Bommisetti, S. Xiao, V. Bandarian and C. J. Burrows, Direct nanopore sequencing for the 17 RNA modification types in 36 locations in the E. coli ribosome enables monitoring of stress-dependent changes, ACS Chem. Biol., 2023 DOI:10.1021/acschembio.3c00166.
- A. M. Smith, M. Jain, L. Mulroney, D. R. Garalde and M. Akeson, Reading canonical and modified nucleobases in 16S ribosomal RNA using nanopore native RNA sequencing, PLoS One, 2019, 14, e0216709 CrossRef CAS PubMed.
- A. M. Fleming, N. J. Mathewson, S. A. Howpay Manage and C. J. Burrows, Nanopore dwell time analysis permits sequencing and conformational assignment of pseudouridine in SARS-CoV-2, ACS Cent. Sci., 2021, 7, 1707–1717 CrossRef CAS PubMed.
- S. Huang, W. Zhang, C. D. Katanski, D. Dersh, Q. Dai, K. Lolans, J. Yewdell, A. M. Eren and T. Pan, Interferon inducible pseudouridine modification in human mRNA by quantitative nanopore profiling, Genome Biol., 2021, 22, 330 CrossRef CAS PubMed.
- A. Leger, P. P. Amaral, L. Pandolfini, C. Capitanchik, F. Capraro, V. Miano, V. Migliori, P. Toolan-Kerr, T. Sideri, A. J. Enright, K. Tzelepis, F. J. van Werven, N. M. Luscombe, I. Barbieri, J. Ule, T. Fitzgerald, E. Birney, T. Leonardi and T. Kouzarides, RNA modifications detection by comparative nanopore direct RNA sequencing, Nat. Commun., 2021, 12, 7198 CrossRef CAS PubMed.
- S. Ramasamy, V. J. Sahayasheela, S. Sharma, Z. Yu, T. Hidaka, L. Cai, V. Thangavel, H. Sugiyama and G. N. Pandian, Chemical probe-based nanopore sequencing to selectively assess the RNA modifications, ACS Chem. Biol., 2022, 17, 2704–2709 CrossRef CAS PubMed.
- S. Tavakoli, M. Nabizadeh, A. Makhamreh, H. Gamper, C. A. McCormick, N. K. Rezapour, Y.-M. Hou, M. Wanunu and S. H. Rouhanifard, Semi-quantitative detection of pseudouridine modifications and type I/II hypermodifications in human mRNAs using direct long-read sequencing, Nat. Commun., 2023, 14, 334 CrossRef CAS PubMed.
- W. Stephenson, R. Razaghi, S. Busan, K. M. Weeks, W. Timp and P. Smibert, Direct detection of RNA modifications and structure using single-molecule nanopore sequencing, Cell Genom, 2022, 2, 100097 CrossRef CAS PubMed.
- O. Begik, M. C. Lucas, L. P. Pryszcz, J. M. Ramirez, R. Medina, I. Milenkovic, S. Cruciani, H. Liu, H. G. S. Vieira, A. Sas-Chen, J. S. Mattick, S. Schwartz and E. M. Novoa, Quantitative profiling of pseudouridylation dynamics in native RNAs with nanopore sequencing, Nat. Biotechnol., 2021, 39, 1278–1291 CrossRef CAS PubMed.
- N. M. Martinez, A. Su, M. C. Burns, J. K. Nussbacher, C. Schaening, S. Sathe, G. W. Yeo and W. V. Gilbert, Pseudouridine synthases modify human pre-mRNA co-transcriptionally and affect pre-mRNA processing, Mol. Cell, 2022, 82, 645–659 CrossRef CAS PubMed.
- T. M. Carlile, N. M. Martinez, C. Schaening, A. Su, T. A. Bell, B. Zinshteyn and W. V. Gilbert, mRNA structure determines modification by pseudouridine synthase 1, Nat. Chem. Biol., 2019, 15, 966–974 CrossRef CAS PubMed.
- A. M. Fleming and C. J. Burrows, Nanopore sequencing for N1-methylpseudouridine in RNA reveals sequence-dependent discrimination of the modified nucleotide triphosphate during transcription, Nucleic Acids Res., 2023, 51, 1914–1926 CrossRef CAS PubMed.
-
W. Liu-Wei, W. V. D. Toorn, P. Bohn, M. Hölzer, R. Smyth and M. V. Kleist, Sequencing accuracy and systematic errors in nanopore direct RNA sequencing. bioRxiv 2023 DOI:10.1011/2023.1003.1029.534691.
- A. M. Fleming, A. Alenko, J. P. Kitt, A. M. Orendt, P. F. Flynn, J. M. Harris and C. J. Burrows, Structural elucidation of bisulfite adducts to pseudouridine that result in deletion signatures during reverse transcription of RNA, J. Am. Chem. Soc., 2019, 141, 16450–16460 CrossRef CAS PubMed.
- H. Li, Minimap2: pairwise alignment for nucleotide sequences, Bioinformatics, 2018, 34, 3094–3100 CrossRef CAS PubMed.
- H. Li, Toward better understanding of artifacts in variant calling from high-coverage samples, Bioinformatics, 2014, 30, 2843–2851 CrossRef CAS PubMed.
- H. Li, B. Handsaker, A. Wysoker, T. Fennell, J. Ruan, N. Homer, G. Marth, G. Abecasis and R. Durbin, The sequence alignment/map format and SAMtools, Bioinformatics, 2009, 25, 2078–2079 CrossRef CAS PubMed.
- J. T. Robinson, H. Thorvaldsdóttir, W. Winckler, M. Guttman, E. S. Lander, G. Getz and J. P. Mesirov, Integrative genomics viewer, Nat. Biotechnol., 2011, 29, 24–26 CrossRef CAS PubMed.
- M. Taoka, Y. Nobe, Y. Yamaki, K. Sato, H. Ishikawa, K. Izumikawa, Y. Yamauchi, K. Hirota, H. Nakayama, N. Takahashi and T. Isobe, Landscape of the complete RNA chemical modifications in the human 80S ribosome, Nucleic Acids Res., 2018, 46, 9289–9298 CrossRef CAS PubMed.
- Z. L. Watson, F. R. Ward, R. Méheust, O. Ad, A. Schepartz, J. F. Banfield and J. H. Cate, Structure of the bacterial ribosome at 2 Å resolution, eLife, 2020, 9, e60482 CrossRef CAS PubMed.
- A. M. Popova and J. R. Williamson, Quantitative analysis of rRNA modifications using stable isotope labeling and mass spectrometry, J. Am. Chem. Soc., 2014, 136, 2058–2069 CrossRef CAS PubMed.
- H. Liu, O. Begik, M. C. Lucas, J. M. Ramirez, C. E. Mason, D. Wiener, S. Schwartz, J. S. Mattick, M. A. Smith and E. M. Novoa, Accurate detection of m6A RNA modifications in native RNA sequences, Nat. Commun., 2019, 10, 4079 CrossRef PubMed.
- Q. Dai, L.-S. Zhang, H.-L. Sun, K. Pajdzik, L. Yang, C. Ye, C.-W. Ju, S. Liu, Y. Wang, Z. Zheng, L. Zhang, B. T. Harada, X. Dou, I. Irkliyenko, X. Feng, W. Zhang, T. Pan and C. He, Quantitative sequencing using BID-seq uncovers abundant pseudouridines in mammalian mRNA at base resolution, Nat. Biotechnol., 2023, 41, 344–354 CrossRef CAS PubMed.
- M. Zhang, Z. Jiang, Y. Ma, W. Liu, Y. Zhuang, B. Lu, K. Li, J. Peng and C. Yi, Quantitative profiling of pseudouridylation landscape in the human transcriptome, Nat. Chem. Biol., 2023 DOI:10.1038/s41589-23-01304-7.
- S. Edelheit, S. Schwartz, M. R. Mumbach, O. Wurtzel and R. Sorek, Transcriptome-wide mapping of 5-methylcytidine RNA modifications in bacteria, archaea, and yeast reveals m5C within archaeal mRNAs, PLoS Genet., 2013, 9, e1003602 CrossRef CAS PubMed.
- V. Khoddami, A. Yerra, T. L. Mosbruger, A. M. Fleming, C. J. Burrows and B. R. Cairns, Transcriptome-wide profiling of multiple RNA modifications simultaneously at single-base resolution, Proc. Natl. Acad. Sci. U. S. A., 2019, 116, 6784–6789 CrossRef CAS PubMed.
- V. Marchand, F. Pichot, P. Neybecker, L. Ayadi, V. Bourguignon-Igel, L. Wacheul, D. L. J. Lafontaine, A. Pinzano, M. Helm and Y. Motorin, HydraPsiSeq: a method for systematic and quantitative mapping of pseudouridines in RNA, Nucleic Acids Res., 2020, 48, e110 CrossRef CAS PubMed.
- T. M. Carlile, M. F. Rojas-Duran, B. Zinshteyn, H. Shin, K. M. Bartoli and W. V. Gilbert, Pseudouridine profiling reveals regulated mRNA pseudouridylation in yeast and human cells, Nature, 2014, 515, 143–146 CrossRef CAS PubMed.
- A. E. Schibel, N. An, Q. Jin, A. M. Fleming, C. J. Burrows and H. S. White, Nanopore detection of 8-oxo-7,8-dihydro-2'-deoxyguanosine in immobilized single-stranded DNA via adduct formation to the DNA damage site, J. Am. Chem. Soc., 2010, 132, 17992–17995 CrossRef CAS PubMed.
- M. J. Booth, E.-A. Raiber and S. Balasubramanian, Chemical methods for decoding cytosine modifications in DNA, Chem. Rev., 2015, 115, 2240–2254 CrossRef CAS PubMed.
- A. M. Fleming, S. Xiao and C. J. Burrows, Pseudouridine and N1-methylpseudouridine display pH-independent reaction rates with bisulfite yielding ribose adducts, Org. Lett., 2022, 24, 6182–6185 CrossRef CAS PubMed.
- I. Nookaew, P. Jenjaroenpun, H. Du, P. Wang, J. Wu, T. Wongsurawat, S. H. Moon, E. Huang, Y. Wang and G. Boysen, Detection and Discrimination of DNA Adducts Differing in Size, Regiochemistry, and Functional Group by Nanopore Sequencing, Chem. Res. Toxicol., 2020, 33, 2944–2952 Search PubMed.
- Y. Huang, W. A. Pastor, Y. Shen, M. Tahiliani, D. R. Liu and A. Rao, The behaviour of 5-hydroxymethylcytosine in bisulfite sequencing, PLoS One, 2010, 5, e8888 CrossRef PubMed.
- X. Lu and C. He, Nonenzymatic labeling of 5-hydroxymethylcytosine in nanopore sequencing, ChemBioChem, 2013, 14, 1289–1290 Search PubMed.
- Q. Wu, S. M. Amrutkar and F. Shao, Sulfinate based selective labeling of 5-hydroxymethylcytosine: application to biotin pull down assay, Bioconjugate Chem., 2018, 29, 245–249 Search PubMed.
- H. Gamper, C. McCormick, A. Makhamreh, M. Wanunu, S. H. Rouhanifard and Y.-M. Hou, Enzymatic synthesis of RNA standards for mapping and quantifying RNA modifications in sequencing analysis, Methods Enzymol., 2023 DOI:10.1016/bs.mie.2023.04.024.
- A. M. Kietrys, W. A. Velema and E. T. Kool, Fingerprints of modified RNA bases from deep sequencing profiles, J. Am. Chem. Soc., 2017, 139, 17074–17081 Search PubMed.
- V. Potapov, X. Fu, N. Dai, I. R. Corrêa, Jr., N. A. Tanner and J. L. Ong, Base modifications affecting RNA polymerase and reverse transcriptase fidelity, Nucleic Acids Res., 2018, 46, 5753–5763 CrossRef CAS PubMed.
- C. Martinez Campos, K. Tsai, D. G. Courtney, H. P. Bogerd, C. L. Holley and B. R. Cullen, Mapping of pseudouridine residues on cellular and viral transcripts using a novel antibody-based technique, RNA, 2021, 27, 1400–1411 CrossRef PubMed.
- S. Kumar and T. Mohapatra, Deciphering epitranscriptome: modification of mRNA bases provides a new perspective for post-transcriptional regulation of gene expression, Front. Cell Dev. Biol., 2021, 9, 628415 CrossRef PubMed.
- N. An, A. M. Fleming, H. S. White and C. J. Burrows, Nanopore detection of 8-oxoguanine in the human telomere repeat sequence, ACS Nano, 2015, 9, 4296–4307 CrossRef CAS PubMed.
- V. Borsenberger, N. Mitchell and S. Howorka, Chemically labeled nucleotides and oligonucleotides encode DNA for sensing with nanopores, J. Am. Chem. Soc., 2009, 131, 7530–7531 CrossRef CAS PubMed.
- I. Gifford, A. Dasgupta and J. E. Barrick, Rates of gene conversions between Escherichia coli ribosomal operons, G3, 2021, 11, jkaa002 CrossRef CAS PubMed.
- S. Nurk, S. Koren, A. Rhie, M. Rautiainen, A. V. Bzikadze, A. Mikheenko, M. R. Vollger, N. Altemose, L. Uralsky, A. Gershman, S. Aganezov, S. J. Hoyt, M. Diekhans, G. A. Logsdon, M. Alonge, S. E. Antonarakis, M. Borchers, G. G. Bouffard, S. Y. Brooks, G. V. Caldas, N.-C. Chen, H. Cheng, C.-S. Chin, W. Chow, L. G. de Lima, P. C. Dishuck, R. Durbin, T. Dvorkina, I. T. Fiddes, G. Formenti, R. S. Fulton, A. Fungtammasan, E. Garrison, P. G. S. Grady, T. A. Graves-Lindsay, I. M. Hall, N. F. Hansen, G. A. Hartley, M. Haukness, K. Howe, M. W. Hunkapiller, C. Jain, M. Jain, E. D. Jarvis, P. Kerpedjiev, M. Kirsche, M. Kolmogorov, J. Korlach, M. Kremitzki, H. Li, V. V. Maduro, T. Marschall, A. M. McCartney, J. McDaniel, D. E. Miller, J. C. Mullikin, E. W. Myers, N. D. Olson, B. Paten, P. Peluso, P. A. Pevzner, D. Porubsky, T. Potapova, E. I. Rogaev, J. A. Rosenfeld, S. L. Salzberg, V. A. Schneider, F. J. Sedlazeck, K. Shafin, C. J. Shew, A. Shumate, Y. Sims, A. F. A. Smit, D. C. Soto, I. Sović, J. M. Storer, A. Streets, B. A. Sullivan, F. Thibaud-Nissen, J. Torrance, J. Wagner, B. P. Walenz, A. Wenger, J. M. D. Wood, C. Xiao, S. M. Yan, A. C. Young, S. Zarate, U. Surti, R. C. McCoy, M. Y. Dennis, I. A. Alexandrov, J. L. Gerton, R. J. O’Neill, W. Timp, J. M. Zook, M. C. Schatz, E. E. Eichler, K. H. Miga and A. M. Phillippy, The complete sequence of a human genome, Science, 2022, 376, 44–53 CrossRef CAS PubMed.
- S. Sharma, S. K. Patnaik, Z. Kemer and B. E. Baysal, Transient overexpression of exogenous APOBEC3A causes C-to-U RNA editing of thousands of genes, RNA Biol., 2017, 14, 603–610 Search PubMed.
- S. Aganezov, S. M. Yan, D. C. Soto, M. Kirsche, S. Zarate, P. Avdeyev, D. J. Taylor, K. Shafin, A. Shumate, C. Xiao, J. Wagner, J. McDaniel, N. D. Olson, M. E. G. Sauria, M. R. Vollger, A. Rhie, M. Meredith, S. Martin, J. Lee, S. Koren, J. A. Rosenfeld, B. Paten, R. Layer, C. S. Chin, F. J. Sedlazeck, N. F. Hansen, D. E. Miller, A. M. Phillippy, K. H. Miga, R. C. McCoy, M. Y. Dennis, J. M. Zook and M. C. Schatz, A complete reference genome improves analysis
of human genetic variation, Science, 2022, 376, eabl3533 CrossRef CAS PubMed.
- N. W. Ho and P. T. Gilham, Reaction of pseudouridine and inosine with N-cyclohexyl-N′-beta-(4-methylmorpholinium)ethylcarbodiimide, Biochemistry, 1971, 10, 3651–3657 CrossRef CAS PubMed.
- I. Behm-Ansmant, M. Helm and Y. Motorin, Use of specific chemical reagents for detection of modified nucleotides in RNA, J. Nucleic Acids, 2011, 2011, 408053 Search PubMed.
- Y. Chen, F. Wu, Z. Chen, Z. He, Q. Wei, W. Zeng, K. Chen, F. Xiao, Y. Yuan, X. Weng, Y. Zhou and X. Zhou, Acrylonitrile-mediated nascent RNA sequencing for transcriptome-wide profiling of cellular RNA dynamics, Adv. Sci., 2020, 7, 1900997 CrossRef CAS PubMed.
- M. Helm, M. C. Schmidt-Dengler, M. Weber and Y. Motorin, General principles for the detection of modified nucleotides in RNA by specific reagents, Adv. Biol., 2021, 5, e2100866 CrossRef PubMed.
-
S. Andrews, FastQC: a quality control tool for high throughput sequence data. https://www.bioinformatics.babraham.ac.uk/projects/fastqc, 2010.
-
G. Hannon, FASTX toolkit. https://hannonlab.cshl.edu/fastx_toolkit/, Ed.
- H. Gamaarachchi, H. Samarakoon, S. P. Jenner, J. M. Ferguson, T. G. Amos, J. M. Hammond, H. Saadat, M. A. Smith, S. Parameswaran and I. W. Deveson, Fast nanopore sequencing data analysis with SLOW5, Nat. Biotechnol., 2022, 40, 1026–1029 CrossRef CAS PubMed.
|
This journal is © The Royal Society of Chemistry 2023 |