DOI:
10.1039/C8RA08198K
(Paper)
RSC Adv., 2018,
8, 39477-39495
Multiple 3D-QSAR modeling, e-pharmacophore, molecular docking, and in vitro study to explore novel AChE inhibitors†
Received
3rd October 2018
, Accepted 16th November 2018
First published on 26th November 2018
Abstract
Ligand-based and energy-optimized structure-based approaches were considered to obtain excellent candidates as AChE inhibitors. The known AChE inhibitors were utilized to develop a pharmacophore hypothesis, HPRRR and X-ray crystallographic structures of AChE were used to produce three e-pharmacophore hypotheses viz. AHHRR, AHRR, and DHRR. Based on in silico approaches, we came across eight structurally diverse hits as non-competitive AChE inhibitors with good ADME properties. The best four hits, ZINC20592007, ZINC05354646, ZINC20649934, and ZINC39154782 were non-toxic, neuroprotective, and were selective AChE inhibitors (IC50 values 482 ± 1.88 nM, 580 ± 1.63 nM, 854 ± 2.65 nM, and 636 ± 1.79 nM respectively). The hits showed non-competitive inhibition of AChE at PAS site with attractive Ki values (0.21 ± 0.027 μM, 0.27 ± 0.064 μM, 0.3 ± 0.018 μM, and 0.28 ± 0.032 μM for ZINC20592007, ZINC05354646, ZINC20649934, and ZINC39154782 respectively), and increased the cholinergic activity as well as inhibited Aβ aggregation.
Introduction
Acetylcholinesterase (AChE), a 3.5 kDa protein, is a member of the carboxylesterase family with an α/β-hydrolase fold.1 The leading role of AChE is the hydrolysis of synaptic acetylcholine (ACh) and regulation of cholinergic neurotransmission in the body. It also plays a pivotal role in neuritogenesis, synaptogenesis, amyloidosis, dopamine neuron activation, regulation of apoptosis, nerve regeneration, hematopoiesis, and lymphocyte activation.2,3 The in vitro and in vivo exercises explain the relationship between amyloid precursor protein (APP) processing and cholinergic activation through muscarinic and nicotinic receptors.4,5
Structurally, AChE consists of ‘large central mixed β-sheets’ surrounded by ‘15 α-helices’.6 The catalytic anionic site (CAS) is located at the bottom of a narrow gorge of AChE consisting of esteratic site (Ser203, Glu334, and His447) and anionic site (Trp86). Another site, named as peripheral anionic site (PAS) (consisting of Tyr72, Asp74, Tyr124, Trp286, and Tyr341 residues) is 20 Å from the catalytic center. The aromatic residue's ring creates 40% surface of the gorge and is located in the loop, thus presenting greater conformational flexibility. The Trp86 residue forms π-cation interaction with quaternary nitrogen of the ACh along with Phe 338.7 The PAS of AChE acts as an adhesion site to non-amyloidogenic conformer of Aβ leading to its conformational change to produce amyloid fibrils.8 The Trp286 at the PAS site mimics response of the whole enzyme on amyloid formation.9 Further, AChE–Aβ complexes induce neurotoxicity and trigger more neurodegeneration than Aβ peptide alone. Thus, designing AChE inhibitor (AChEI) that blocks PAS of the enzyme will prevent Aβ aggregation as well as enhance cholinergic transmission for treating Alzheimer's disease (AD).
Both, β-amyloid protein (Aβ) and abnormally hyperphosphorylated tau (P-tau) can influence AChE overexpression in AD.10 The improvement of cholinergic transmission by using AChEI may boost cognitive impairment of patients with schizophrenia,11,12 and Parkinson's disease (PD).13 The acetylcholine receptors at neuromuscular junction are reduced in myasthenia gravis (MG),14 and AChEIs are considered essential for the treatment of MG. AChEIs can enhance cholinergic up-regulation by weakening the effect of neuroinflammation via immunocompetent cells expressing α-7-acetylcholine receptor (AChR).15
Butyrylcholinesterase (BuChE) level is increased by up to 2-fold during mild to moderate AD,16 and causes an imbalance between synthesis or synaptic release of ACh and its enzymatic hydrolysis. Therefore, AChEIs with BuChE inhibition property may provide better therapeutic value in neurological disorders.
The drug discovery process is time-consuming and cumbersome, but the use of in silico approaches helps to identify better hits and scaffolds for a target. The pharmacophore modeling is a mathematical modeling technique which may help in the quick prediction of hits. The combination of ligand-based and structure-based pharmacophore models help in better productivity of outcome.17 Earlier researchers attempted to develop pharmacophore models and utilized them for virtual screening of database molecules to find new AChE inhibitors.18–23
Present work combines both ligand-based (3D-QSAR) and energy optimized structure-based pharmacophore (e-pharmacophore) approaches for virtual screening of free ‘ZINC15’ database molecules. The hits, as AChE inhibitors, were recognized by utilizing HTVS and molecular docking studies of pharmacophore matched compounds after removal of pan-assay interference compounds (PAINS).24 The workflow of hit identification based on ligand-based 3D-QSAR and structure-based e-pharmacophore is explicit in Fig. 1.
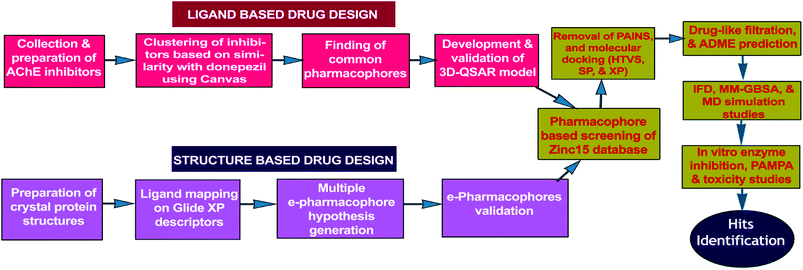 |
| Fig. 1 Flowchart of hit identification based on ligand-based and structure-based pharmacophore models. | |
The in vitro studies viz. enzyme inhibitions (AChE & BuChE), enzyme kinetics (AChE), propidium iodide displacement from AChE, parallel artificial membrane permeability assay for blood–brain barrier (PAMPA-BBB), effects on cell viability and neuroprotectivity against apoptosis triggered by L-glutamate, approved and validated the outcome from the in silico study.
Results and discussion
Development of ligand-based pharmacophore model
Atom-based 3D-QSAR model was developed by using 142 datasets, which was divided into actives, inactives, and moderately actives. Total 163 hypotheses were generated and the best pharmacophore hypothesis, HPRRR, was selected on the basis of good survival activity (3.7), survival-inactive score (2.22), vector score (0.998), volume (0.862), selectivity (2.576), energy scores, best active alignment, and number of site matches (Table 1).
Table 1 The 3D-QSAR pharmacophore hypothesis with various scores
Hypothesisa |
Survival score |
Survival-inactive score |
Site score |
Vector score |
Volume |
Selectivity |
H, hydrogen bond donor; P, positively ionizable group; and R, aromatic ring. |
HPRRR |
3.7 |
2.22 |
0.84 |
0.998 |
0.862 |
2.576 |
Hypothesis HPRRR: one hydrogen-bond acceptor, one positive ionizable group, and three aromatic rings showed the highest survival score. The developed 3D-QSAR pharmacophore model was statistically validated internally and externally to exhibit reliable predictions. We randomly selected 100 compounds in the training set and 42 in the test set to generate 3D-QSAR model. The statistical parameters were obtained by ‘leave one out’ (LOO) method and by partial least-square (PLS) analyze. HPRRR hypothesis showed better predictive ability, with PLS factor 5 than others (Table 2).
Table 2 PHASE 3D-QSAR and PLS statistics for internal validation of hypothesis
Hypothesis |
PLS factor |
SDa |
R2b |
Fc |
P |
Stability |
RMSEd |
Q2e |
Pearson-Rf |
Standard deviation of the regression. The square of correlation coefficient. Variance ratio. Root-mean-square error. Squared Q value for the predicted activities. Correlation between the predicted and observed activities for the test set. |
HPRRR |
1 |
0.735 |
0.570 |
129.7 |
1.204 × 10−19 |
0.933 |
0.651 |
0.314 |
0.588 |
2 |
0.482 |
0.817 |
216.5 |
1.706 × 10−36 |
0.843 |
0.480 |
0.627 |
0.797 |
3 |
0.376 |
0.890 |
257.8 |
8.733 × 10−46 |
0.770 |
0.468 |
0.646 |
0.813 |
4 |
0.288 |
0.936 |
347.5 |
8.721 × 10−56 |
0.702 |
0.437 |
0.691 |
0.834 |
5 |
0.225 |
0.961 |
465.7 |
1.13 × 10−64 |
0.678 |
0.409 |
0.729 |
0.857 |
Validation of ligand-based pharmacophore model
At PLS factor 5, hypothesis HPRRR showed low SD value of 0.225, RMSE of 0.409, and P value of 1.13 × 10−64, and higher R2 of 0.961 for the training set, and good Q2 of 0.729; Person-R of 0.857; F value of 465.7 for the test set. Therefore, HPRRR model had good predictivity at PLS factor 5 and was taken for further pharmacophore-based screening of database molecules. The pharmacophoric features of 3D-QSAR hypothesis are sketched in Fig. 2. The distance between pharmacophores was within the range of 2.174–11.329 Å (Table 3).
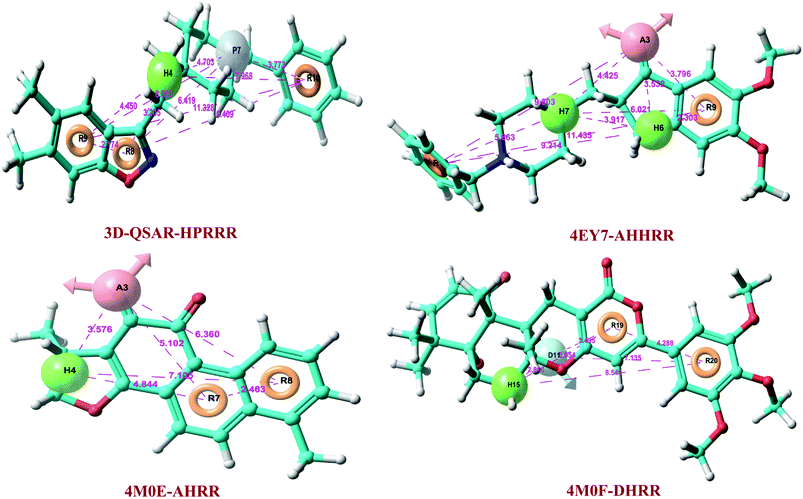 |
| Fig. 2 3D-QSAR pharmacophore hypotheses and structure-based pharmacophores models with their respective crystal structures. (A) H-bond acceptor, pink sphere containing arrow; (D) H-bond donor, sky blue sphere with arrow; (H) hydrophobic group, green sphere; (P) positive ionizable group, violet sphere; (R) aromatic ring, yellow circle. | |
Table 3 Distance between features of 3D-QSAR hypotheses and e-pharmacophores
Pharmacophore model a |
Distance from A to H (Å) |
Distance from A to R (Å) |
Distance from H to H (Å) |
Distance from H to P (Å) |
Distance from H to R (Å) |
Distance from P to R (Å) |
Distance from R to R (Å) |
Distance from D to H (Å) |
Distance from D to R (Å) |
Type of model written with pharmacophores; PDB used for the respective e-pharmacophore model. |
3D-QSAR-HPRRR |
|
|
|
4.703 |
3.205 |
6.419 |
2.174 |
|
|
|
|
|
|
4.45 |
8.05 |
9.409 |
|
|
|
|
|
|
7.968 |
3.773 |
11.328 |
|
|
4EY7-AHHRR |
3.532 |
3.796 |
3.917 |
|
2.303 |
|
11.435 |
|
|
4.425 |
|
|
|
9.214 |
|
|
|
|
|
9.803 |
|
|
6.021 |
|
|
|
|
|
|
|
|
5.863 |
|
|
|
|
4M0E-AHRR |
3.576 |
5.102 |
|
|
4.844 |
|
2.463 |
|
|
|
6.36 |
|
|
7.105 |
|
|
|
|
4M0F-DHRR |
|
|
|
|
5.054 |
|
4.288 |
2.816 |
3.495 |
|
|
|
|
8.549 |
|
|
|
7.135 |
All the parameters for external validation of ligand-based pharmacophore model helped to select best model (Table 4). The correlation coefficient (r2) value of 0.922, cross-validation coefficient (rcv2) value of 0.919, square of correlation coefficient value using the LOO method, (Rm(LOO)2), of 0.834, also helped to consider 3D-QSAR model as a better predictive model. The slopes of regression lines through origin (K and K′ value) and substantial values of correlation coefficients (R02 and
) were obtained from observed activity versus predictive activity plots (Fig. 3). The values were also within the limits and encouraged the model predictivity.
Table 4 External validation parameters for 3D-QSAR
External validation parameters |
HPRRR |
Limitations |
Cross-validated coefficient. Correlation coefficient between actual and predicted values. Slope values of regression lines. Slope values of regression lines. Correlation coefficients for regression lines through origin. Correlation coefficients for regression lines through origin. Modified squared correlation coefficient using LOO method. Predictive correlation coefficient value. |
rcv2a |
0.919 |
rcv2 > 0.5 |
r2b |
0.922 |
r2 close to 1 |
k valuec |
0.990 |
0.85 ≤ k ≤ 1.15 |
K′ valued |
1.008 |
0.85 ≤ k ≤ 1.15 |
R02e |
0.916 |
Close to r2 |
f |
0.921 |
Close to r2 |
Rm(LOO)2g |
0.834 |
Rm(LOO)2 > 0.5 |
rpred2h |
0.738 |
rpred2 > 0.5 |
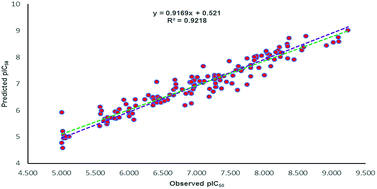 |
| Fig. 3 Plot of predicted pIC50 versus observed pIC50 of AChE inhibitors developed by model HPRRR with regression lines (original regression lines represented in green break line and regression lines with intercept zero in purple break line). | |
Development of energy-optimized structure-based pharmacophore
Total three human AChE (hAChE) crystal structures with a resolution between 2.0 Å and 2.35 Å and potent AChE inhibitory activity (IC50 from 5.3 to 7 nM and Ki 1.7 to 700 nM) were selected for developing e-pharmacophore. Protein preparation wizard was used to prepare the proteins with an OPLS_2005 force field. After refinement, the protein structures with ligand interaction showed that donepezil (cocrystal of 4EY7) interacted with Trp86, and TRP286 by a pi–pi stacking, H-bond interacted with Phe295, and pi-cationic with Phe338 residue. The dihydrotanshinone I (cocrystal of 4M0E) interacted with TRP286 by a pi–pi stacking, H-bond interaction was with Phe295; and territrem B (cocrystal of 4M0F) interacted with TRP286 by a pi–pi stacking and H-bond interaction was with Tyr124 at the PAS site (Fig. 4).
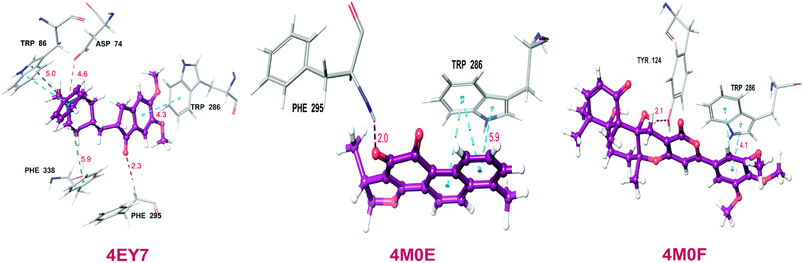 |
| Fig. 4 Crystal structures of AChE with cocrystal ligands (purple color) and bonding interactions. | |
The refined cocrystal ligands were redocked onto the respective prepared protein structures to generate energy-optimized structure-based pharmacophore (e-pharmacophore). The root-mean-square deviation (rmsd) was less than 1 Å for all the three cocrystal ligands. The e-pharmacophore hypotheses were generated by mapping Glide XP energetic terms onto pharmacophore sites, which were calculated from the structural and energy information between protein and ligand. Initially, the numbers of pharmacophore sites were set up to 10 for each of crystal structures for pharmacophore generation, but numbers of pharmacophore sites were selected, for the best hypothesis, on the basis of validation parameters. The total number of pharmacophore sites for each cocrystal ligand before energy-based site selection and selected sites for hypothesis generation for the three crystal structures with pharmacophoric feature scores are given in Table 5. The e-pharmacophore models generated were AHHRR with 5 sites from 4EY7, AHRR with 4 sites from 4M0E, and DHRR with 4 sites from 4M0F crystal structure (Fig. 2). In these pharmacophore modeling, A stranded for H-bond acceptor, D for H-bond donor, H for hydrophobic group, R for aromatic ring. The distance between e-pharmacophore features was within range of 2.303–11.435 Å (Table 3).
Table 5 e-Pharmacophore hypotheses with features scores
PDB |
No. of possible site |
No. of accepted site |
Hypothesesa |
Pharmacophore features with score |
A, H-bond acceptor; D, hydrogen bond donor; H, hydrophobic group; P, positively ionizable group; R, aromatic ring. |
4EY7 |
6 |
5 |
AHHRR |
H7: −1.64, H6: −1.5, R10: −1.48, R9: −1.2, A3: −0.73 |
4M0E |
4 |
4 |
AHRR |
A3: −1.7, R7: −1.62, H4: −1.5, R8: −1.13 |
4M0F |
7 |
4 |
DHRR |
R19: −1.5, D11: −1.49, R20: −1.3, H15: −0.66 |
Validation of energy-optimized structure-based pharmacophore
The database, consisting of 1053 compounds using 1000 drug-like decoys and 53 known AChE inhibitors, was utilized for e-pharmacophore validation., We evaluated enrichment factor (EF) and Goodness of hit score (GH) utilizing Güner–Henry scoring method To validate the e-pharmacophores (Table 6). The values of GH over 0.5 and EF higher than 10, ensured the suitability of pharmacophores for further pharmacophore-based virtual screening.
Table 6 Validation of e-pharmacophores with the Güner–Henry scoring method using a dataset consisting of total 1053 compounds with 53 total actives compounds
Parameters |
4EY7 |
4M0E |
4M0F |
Total hits. Active hits. Overall enrichment factor. Goodness of hit score. |
Hta |
74 |
69 |
71 |
Hab |
41 |
37 |
43 |
EFc |
11.008 |
10.654 |
12.033 |
GHd |
0.589 |
0.558 |
0.639 |
Pharmacophore matched screening and removal of pan-assay interference compounds (PAINS)
Pharmacophore matched molecules were separated out from the total 3530990 ZINC15 database compounds (without known AChE inhibitors) by utilizing advance pharmacophore screening option of PHASE. The fitness value is a measure of how well the ligand fits with the pharmacophore. The hits with high fitness value of more than 1.5 are probably very active inhibitors. We employed the validated three e-pharmacophores, and one ligand-based pharmacophore to screen the database of 2000 AChE inhibitor molecules by each model.
The reactivity towards proteins to develop poor potentiality or known toxicity of molecules, i.e., PAINS was removed from pharmacophore matched compounds by using RDKit, ZINC, and FAF-Drugs4 server. Only less than 1% of PAINS compounds were removed and mild PAINS were ignored for virtual screening.
High throughput virtual screening (HTVS) and molecular docking
High throughput screening of PAINS removed pharmacophore matched database and was a fruitful resource for initial hit identification. The number of hits from pharmacophore-based virtual screening and process of selection with their respective PDB are presented in Table 7.
Table 7 Number of compounds retrieved at each stage of screening of dataset
Pharmacophore models |
PDB |
HTVS hits |
SP hits |
XP hits |
No. of selected hits |
AHHRR |
4EY7 |
1991 |
200 |
20 |
1 |
AHRR |
4M0E |
1873 |
199 |
19 |
2 |
DHRR |
4M0F |
1993 |
200 |
20 |
2 |
HPRRR |
4M0F |
1945 |
200 |
20 |
3 |
Molecular XP docking was performed for all the outcome HTVS retrieves with 4M0E crystal structure to compare docking scores of hits with reference donepezil. We found that 55 molecules were having docking score more than −9.0. Finally, eight compounds with structural diversity, PAINS free (except ZINC20592007, a PAINS-ok molecule), better docking scores (−12.87 to −10.74) and Glide energies (−56.48 to −42.16 kcal mol−1) than donepezil were selected for further studies (Table 8). The hits outcome with respect to pharmacophore models are listed in ESI (Table S1†). The protein-ligand interactions with types of interactions and interacting residues with hits and donepezil are included in Table 8. The chemical structures of hits are sketched in Fig. 5 and ligand–protein interactions are pictured in Fig. 6; hits are represented in yellowish green, interacting amino acid residues of protein in gray, H-bond in red, pi-cationic interaction in green and pi–pi stacking in cyan color. The presence of more hydroxyl, keto, secondary amine and nitrogen-containing hetero aromatics in hits were responsible for formation of hydrogen bond and more docking score than donepezil.
Table 8 Hit molecules with their Glide docking score, number of H-bonds, interaction with essential amino acids, IFD docking score, and AutoDock binding energy
Salt bridge. H-bond interaction. Pi–Pi stacking. Pi-cation interaction. |
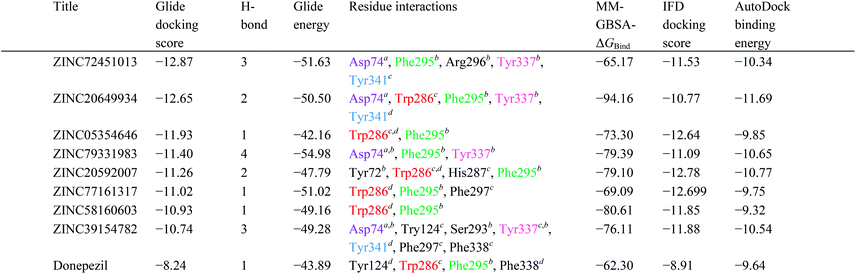 |
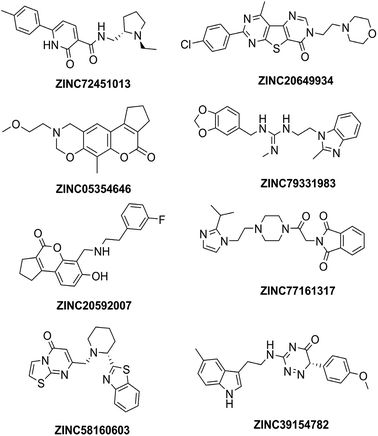 |
| Fig. 5 Structures of final hits with zinc database ids. | |
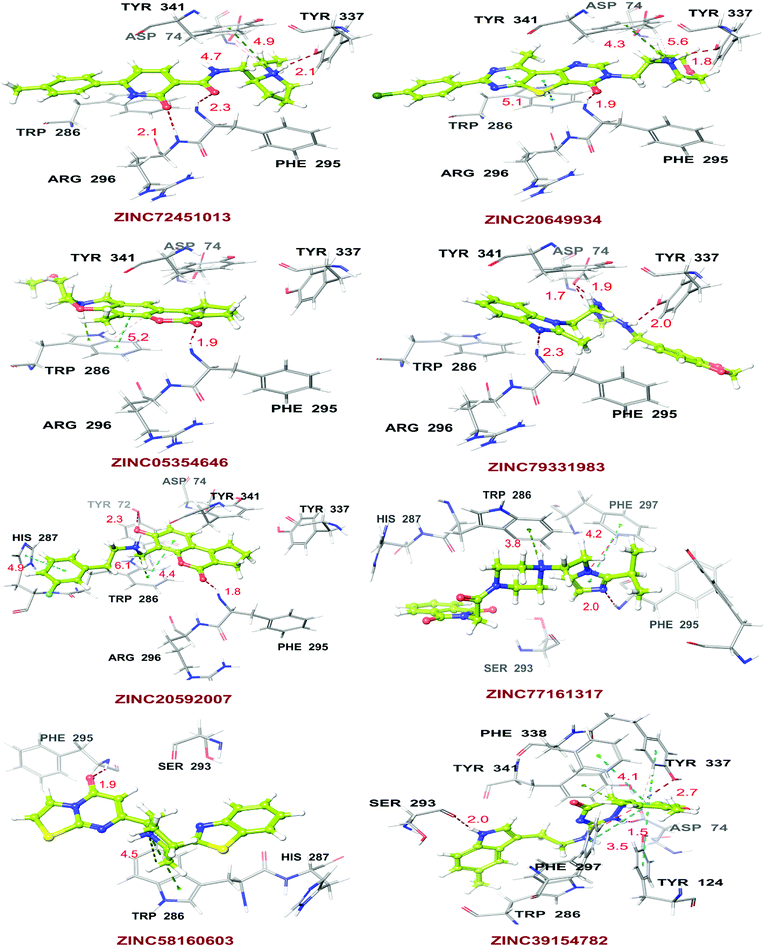 |
| Fig. 6 Docking poses of ZINC72451013, ZINC20649934, ZINC05354646, ZINC79331983, ZINC20592007, ZINC77161317, ZINC58160603, and ZINC39154782 with AChE crystal structure; hits represented in yellowish green, residues in gray, H-bond in red, pi-cationic interaction in green and pi–pi stacking in cyan color. | |
The hits mainly bind at PAS site of AChE through H-bond interactions with Phe295, Tyr337, and Phe338 residues (within 1.8–2.3 Å bond distance), pi–pi stacking with Trp286, His 287, Phe297, and Tyr341 residues (within 3.8–5.1 Å distance), and pi-cationic or salt bridge interactions with Asp74, Tyr341, and Trp286 residues (within 1.9–5.6 Å distance). Keto group of all hits, except ZINC77161317 and ZINC39154782 were formed H-bonding with Phe295 and Ser293, were formed hydrogen bonding with Phe295 reside of protein. Aromatic group of hits were produced π–π stacking interaction with Trp286, except ZINC39154782 interacting with Phe297.
Induced fit docking
The IFD scores of hits were close to the Glide XP docking scores (Table 8). The conformations generated from the IFD were little different from the docked poses produced from the rigid receptor docking. The Glide-based model gave an RMSD of 5.2 Å when compared to the native pose in the crystal structure. The IFD docking pose and score were supported by the binding positions, affinity, and stability of hits.
Calculation of prime MM-GBSA
To predict the binding mode and binding free energy (ΔGbind), the Prime MM-GBSA simulation was calculated for AChE–hits and AChE–cocrystal ligand complexes utilizing Maestro 10.1 (Table 8). All the hits showed better ΔGbind, and ZINC20649934 provided highest ΔGbind, −94.16 kcal mol−1. The binding free energy determination, based on Prime MM-GBSA, established the stability of AChE–hits complexes.
Docking with AutoDock
The AutoDock binding energy of the hits was calculated and presented in Table 8. The binding energy of final eight hits was between −9.32 to −11.69 kcal mol−1. The prediction of results was fully supported Glide XP docking and IFD results (Table 8). All the hits displayed similar binding affinity and docking pose with 4M0E utilizing AutoDock and Glide (ESI, Fig. S1 and S2†).
Predicted ADME properties
The predictions of drug-likeness and pharmacokinetics including absorption, distribution, metabolism, and excretion (ADME) were performed by utilizing QikProp tools of Maestro, Schrödinger. We evaluated physiochemically descriptors and pharmaceutically relevant properties of hits to analyze druggable properties (Table 9). All the hit molecules showed a good partition coefficient (QP
log
Po/w) values (1.6 to 3.854), which were critical for absorption and distribution of drugs. Factor QPPCaco, indicating permeability of these hits, was in the range of 79.257 to 1879.796, where QPPCaco was a predicted apparent Caco-2 cell permeability in nm s−1 value, a key factor for the estimation of cell permeability in biological membranes.
Table 9 Hit molecules with their physiochemical descriptors determined by Qikprop tools
Compound ida |
QP log Po/wb |
QP log Sc |
QP log HERGd |
QPPCacoe |
QPPMDCKf |
QP log Kpg |
% of human oral absorptionh |
Rule of fivei |
Zinc database compound id. QP log Po/w for octanol/water (−2.0 to 6.5). QP log S: predicted aqueous solubility, S in mol dm−3 (−6.5 to 0.5). log HERG: HERG K+ channel blockage (<−5). Apparent Caco-2 cell permeability (nm s−1) (<25 poor, >500 great). Apparent MDCK permeability (nm s−1) (<25 poor, >500 great). QP log Kp: skin permeability. % human oral absorption (>80% is high and <25% is poor). Rule of five: no. of violations of Lipinski's rule of five (0 is good and 4 is bad). |
ZINC72451013 |
3.586 |
−4.62 |
−6.76 |
249.141 |
121.859 |
−4.273 |
90.835 |
0 |
ZINC20649934 |
2.619 |
−3.37 |
−6.631 |
529.903 |
1193.73 |
−3.718 |
91.036 |
0 |
ZINC05354646 |
1.627 |
−1.92 |
−5.112 |
553.223 |
288.626 |
−4.359 |
85.562 |
0 |
ZINC79331983 |
3.854 |
−4.37 |
−5.055 |
1879.796 |
978.659 |
−3.407 |
100 |
0 |
ZINC20592007 |
3.448 |
−4.50 |
−6.75 |
199.231 |
173.105 |
−4.22 |
88.291 |
0 |
ZINC77161317 |
1.6 |
−2.38 |
−5.559 |
79.257 |
56.117 |
−4.617 |
70.301 |
0 |
ZINC58160603 |
2.674 |
−3.38 |
−6.619 |
393.786 |
546.198 |
−3.897 |
89.056 |
0 |
ZINC39154782 |
3.311 |
−5.64 |
−6.286 |
406.683 |
187.069 |
−2.709 |
93.035 |
0 |
All the hits successfully passed the entire pharmacokinetic requirements for a drug-like compound and were within the acceptable range as defined for human use. Overall, the percentage of human oral absorption for the compounds were between 70.301 to 100%, their water solubility (QP
log
S) ranged between −1.919 to −6.373, pMDCK (cell permeable parameter) values were between 56.117 to 1193.73, skin permeability (log
Kp) values were within −2.709 to −4.898; p
log
HERG (K+ channel blockage) values were less than −5. Additional parameters i.e., molecular weight, H-bond donors, H-bond acceptors, and log
P according to Lipinski's rule of five, were also evaluated for their drug-like behavior. Thus, hits with better binding interaction and good predicted pharmacokinetic properties were considered for in vitro studies.
Density functional theory
The HOMO and LUMO of chemical compounds are crucial indicators of their reactivity and also stability of ligand–receptor interactions.25 The stability of interactions is inversely correlated to energy gap between HOMO and LUMO orbitals. The orbital energy of all energetically stable hit molecules was calculated by using a DFT method. The high value of HOMO energy is likely to indicate the tendency of molecule to donate electrons to an appropriate acceptor molecule with LUMO. The correlation of HOMO energies with IC50 data suggested that the HOMO of inhibitor might transfer its electrons to less energy, LUMO, of some amino residues in the active site of the enzyme. The calculated DFT properties of all hits are given in ESI (Table S2†). The HOMO–LUMO energy gaps of hits were minimal, and between −0.182 to −0.012 eV. Leaser HOMO–LUMO energy gap facilitated electron(s) density exchanging properties or encouraged some interaction(s). The mean ESP indicated electron density distribution around nuclei of the molecules and was between −0.22 to 1.89 kcal mol−1. The ESP data indicated that most of the hits contained both low and high electron density sites in a molecule. The upper and low electron density regions may correspond to the hydrogen bonding between the hits and enzyme.
In silico AChE selectivity study
We performed Glide XP docking study against BuChE by utilizing 4BDS crystal structure to estimate selectivity of hits towards AChE. All the hits had BuChE binding affinity with more selectivity towards AChE (ESI Table S3†). The hit ZINC05354646 showed lowest Glide docking score (−5.66), and next lowest score compound was ZINC20649934 (−5.85) against BuChE crystal structure, 4BDS. The BuChE binding property of hits with AChE inhibition improved the therapeutic property of hits for cholinergic activity. The hits had moderate BuChE binding affinity which may improve cholinergic activity.
In vitro inhibition of AChE and BuChE
Four hits (ZINC20592007, ZINC05354646, ZINC20649934, and ZINC39154782) were selected, based on Glide docking score, AutoDock energy, AChE selectivity, PAS site selectivity, ADME properties, and interesting structural features for further in vitro studies. ZINC20592007 contains a 2,3-dihydrocyclopenta[c]chromen-4(1H)-one fused nucleus, which is PAINS-ok (mannich-A type) molecule. ZINC05354646, a 2,3,9,10-tetrahydro-8H-cyclopenta[3,4]chromeno[6,7-e][1,3]oxazin-4(1H)-one fused compound, is PAINS free with similar scaffold of ZINC20592007. ZINC20649934 has thieno[2,3-d:4,5-d′]dipyrimidin-4(3H)-one nucleus with attached morpholine ring to ethylene linker. ZINC39154782 contains 1,2,4-triazin-5(6H)-one with indole ring attached through an ethyl amino linker (Fig. 5).
The selected hits were evaluated for their anti-cholinesterase (anti-ChE) activity. AChE and BuChE inhibition activities were evaluated by the method described by Ellman,26 wherein donepezil was used as reference standard. Compound ZINC20592007 exhibited higher AChE inhibitory activity than ZINC05354646, ZINC20649934, and ZINC39154782 [IC50 values (nM) of 482 ± 1.88, 580 ± 1.63, 854 ± 2.65, and 636 ± 1.79, respectively (Table 10)]. All the hits has selective AChE inhibitory activity than BuChE enzyme (Table 10). The half maximal enzyme inhibitory concentration (IC50), a measure of potency of hits inhibiting AChE and BuChE, was calculated by constructing a dose-response curve (ESI, Fig. S3†) by utilizing GraphPad Prism 5.0.
Table 10 Inhibitory activity on AChE (electric eel) and BChE (horse serum) and propidium competition assay results
Compounds |
IC50 AChEa (nM) |
IC50 BChEa (nM) |
Selectivityb (AChE/BChE) |
Propidium displacement (%) |
0.24 μM |
1 μM |
3 μM |
Each assay was repeated three times independently. Selectivity for AChE = [IC50 (BuChE)]/[IC50 (AChE)]. |
ZINC20592007 |
482 ± 1.88 |
23954 ± 5.69 |
49.7 |
44 |
70 |
100 |
ZINC05354646 |
580 ± 1.63 |
147424 ± 6.66 |
254.2 |
0 |
42 |
57 |
ZINC20649934 |
854 ± 2.65 |
148654 ± 6.24 |
174.1 |
0 |
25 |
58 |
ZINC39154782 |
636 ± 1.79 |
128064 ± 5.13 |
201.4 |
29 |
58 |
100 |
Donepezil |
24 ± 0.29 |
7421 ± 2.00 |
309.2 |
0 |
0 |
0 |
The mechanism of AChE enzyme inhibition of the four hits was determined by an enzyme kinetic study. Lineweaver–Burk reciprocal plots were generated by plotting reciprocal of reaction rates and reciprocal of substrate concentrations using different concentrations of hit molecules. Michaelis–Menten kinetics curve resulting from velocity of AChE activity with varying concentrations of substrate (0.15–1.15 μM) in absence and presence hit molecules (0.25, 0.5 and 1 μM of ZINC20592007 and ZINC05354646, and 0.5, 1, and 2 μM for ZINC20649934, and ZINC39154782) are shown in Fig. 7, 8, 9, and 10 respectively. The Ki values of hits were determined by Yonetani–Theorell method from Lineweaver–Burk plots and presented in ESI as Fig. S4–S7† for ZINC20592007, ZINC05354646, ZINC20649934, and ZINC39154782 respectively.
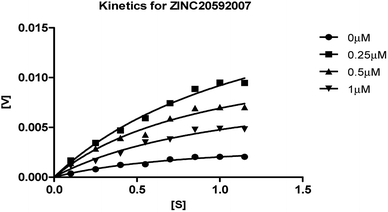 |
| Fig. 7 Michaelis–Menten kinetics curve resulting from velocity of AChE activity with different substrate concentrations (0.15–1.15 μM) in absence and presence of 0.25, 0.5 and 1 μM of ZINC20592007. | |
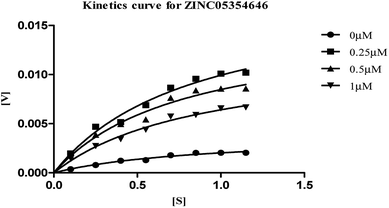 |
| Fig. 8 Michaelis–Menten kinetics curve resulting from velocity of AChE activity with different substrate concentrations (0.15–1.15 μM) in absence and presence of 0.25, 0.5 and 1 μM of ZINC05354646. | |
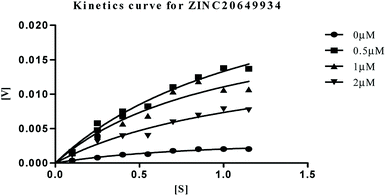 |
| Fig. 9 Michaelis–Menten kinetics curve resulting from velocity of AChE activity with different substrate concentrations (0.15–1.15 μM) in absence and presence of 0.5, 1 and 2 μM of ZINC20649934. | |
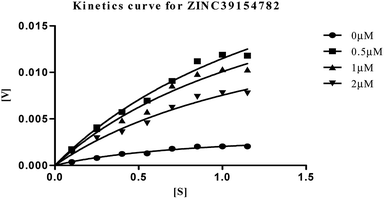 |
| Fig. 10 Michaelis–Menten kinetics curve resulting from velocity of AChE activity with different substrate concentrations (0.15–1.15 μM) in absence and presence of 0.5, 1 and 2 μM of ZINC39154782. | |
The plots revealed that with increasing the concentrations of inhibitor, an increase in slope (decreased Vmax) and the intercept (higher Km) occurred. The lower apparent value of Vmax in Michaelis–Menten plot to increase, decrease, or leave unaffected apparent value of Km, (ESI, Table S4†), indicated as non-competitive inhibitor on the kinetic constants. The double reciprocal Lineweaver–Burk displayed a nest of lines that intersect at a point other than y-axis, and intersecting lines converge to the left of y-axis, and below the x-axis, i.e., α < 1, and indicating that the inhibitor binds with greater affinity to the enzyme–substrate (ES) complex or subsequent species. The calculated inhibitor constant (Ki) of hits (ZINC20592007, ZINC05354646, ZINC20649934, and ZINC39154782) were 0.21 ± 0.027 μM, 0.27 ± 0.064 μM, 0.3 ± 0.018 μM, and 0.28 ± 0.032 μM respectively and were attractive.
Propidium iodide displacement assay
The particular PAS site binding affinity through Trp286 amino acid residue was established by propidium iodide displacement method. The hits successfully displaced propidium, and were selective PAS ligands (Table 10). Molecule ZINC20592007 and ZINC39154782 displaced 100% propidium from PAS of AChE at 3 μM concentration, but ZINC05354646 and ZINC20649934 displaced 57% and 58% respectively, at the same concentration.
In vitro blood–brain barrier permeation assay
A parallel artificial membrane permeation assay of blood–brain barrier (PAMPA-BBB) was performed, as the method described by Di L. et al.,27 to explore infiltration of the selected hits into brain. The in vitro permeability (Pe) of the four hits (Table 11) and nine commercially available drugs (Table S5, ESI†) was determined through a lipid extract of porcine brain lipid in PBS. The assay was validated by comparing the experimentally obtained permeability [Pe(exp)] of the nine drugs with reported values of permeation [Pe(ref)] offering a linear relationship, i.e.,Pe(exp) = 1.308 Pe(literature) − 0.8394, (R2 = 0.9317).
Table 11 Permeability, Pe (10−6 cms−1) determined by BBB-PAMPA study of hit compounds
Compounds |
Pe(exp)a [10−6 cm s−1] |
Pe(actual)a [10−6 cm s−1] |
Predictionb |
Data expressed as mean ± SEM of three independent experiments. CNS + indicates good passive CNS permeation. |
ZINC20592007 |
5.00 ± 0.3 |
5.7 ± 0.20 |
CNS+ |
ZINC05354646 |
7.8 ± 0.25 |
9.39 ± 0.36 |
CNS+ |
ZINC20649934 |
3.74 ± 0.136 |
5.45 ± 0.79 |
CNS+ |
ZINC39154782 |
4.69 ± 0.2 |
5.29 ± 0.31 |
CNS+ |
The permeability values (Pe) greater than 4.3 × 10−6 cm s−1 were capable of CNS permeability (Fig. S8 & Table S6, ESI†) and the tested compounds demonstrated permeability values above it. Thus, the experimentally determined permeability values (Pe) of the test compounds were a pointer towards their potential to comfortably cross the BBB by passive diffusion.
Cellular cytotoxicity and neuroprotection assessment
The cell viability and neuroprotective potential, against apoptosis of selected hits, were evaluated by utilizing human neuroblastoma SH-SY5Y cell line. To investigate the cytotoxicity of compounds, cells were exposed to considerably high concentrations of the test compounds (50 μM and 100 μM) for 24 h. The cell viability was determined by 3-(4,5-dimethyl thiazol-2-yl)-2,5-diphenyltetrazolium bromide (MTT) assay. The selected compounds showed insignificant cell death even at high concentrations (Table 12). The neuroprotective potential of selected hit molecules was assessed by using L-glutamate as excitotoxicity. In this assay, addition of L-glutamate (100 μM) to growth media caused significant cell death as was evidenced by reduction in cell viability. The results (Table 12) are mean ± SEM of at least three independent experiments.
Table 12 Cell viability, and neuroprotection of hit molecules in human neuroblastoma SH-SY5Y cell line
Compounds |
Cell viabilitya (%) |
Neuroprotectionb (%) |
50 μM |
100 μM |
25 μM |
Percentage cell viability of SH-SY5Y cells exposed at relatively high concentrations (50 μM and 100 μM) of test compounds. Percentage neuroprotection of SH-SY5Y cells at relatively lower concentrations (25 μM) of test compounds against L-glutamate(100 μM). |
ZINC20592007 |
98.0 ± 0.34 |
95.3 ± 0.32 |
18.2 ± 0.086 |
ZINC05354646 |
90.2 ± 0.39 |
88.7 ± 0.77 |
20.0 ± 0.061 |
ZINC20649934 |
94.8 ± 0.49 |
93.0 ± 0.45 |
67.8 ± 0.013 |
ZINC39154782 |
98.6 ± 0.55 |
97.1 ± 0.08 |
26.3 ± 0.077 |
Molecular dynamics (MD) simulation
The analyses of molecular dynamic (MD) simulation of ZINC20592007, ZINC20649934 and donepezil with AChE were performed to establish the binding potency and amino acid residue interactions. In MD simulations, RMSD of the protein backbone C-α atoms and individual inhibitor, Root Mean Square Fluctuation (RMSF) in the individual amino acid side chain and ligand–AChE interactions were recorded concerning time over a period of 50 ns of simulation. The total energy of dynamic ligand–protein complexes was found stable in last 40 ns of entire simulation. Furthermore, temperature, pressure, volume, and potential energy of the complex remained constant, indicating the robustness and reliability of MD simulations. The RMSD of simulation converging between 1.5 and 2.5 Å, denoted the stability of macromolecular ligand–protein complexes during 50 ns simulation. The RMSF in individual amino acid residues during the entire simulation was below 4.0 Å, indicating a lower degree of conformational changes in the side chains.
After initial 10 ns simulation, RMSD of protein backbone C-α along with the ligand RMSD values were stabilized. RMSD plot of RMSD values for protein on the left Y-axis and for ligand these values were displayed on right Y-axis in Fig. 11; protein backbone in green color, and ligand in maroon color. The mean RMSD value for donepezil–AChE complex was 2.04 Å, whereas ZINC20592007–AChE and ZINC20649934–AChE complexes were 1.76 and 2.11 Å respectively. RMSF was useful for characterizing local changes along the protein chain C-α and peaks indicated areas of the protein that fluctuate the most during the simulation. RMSF values of hits and donepezil were below 4.0 Å, indicated less fluctuation and better stability of ligand–protein complex during simulation (Fig. 12). The interaction of hits with AChE enzyme higher than 30% after MD simulation is provided in Fig. 13.
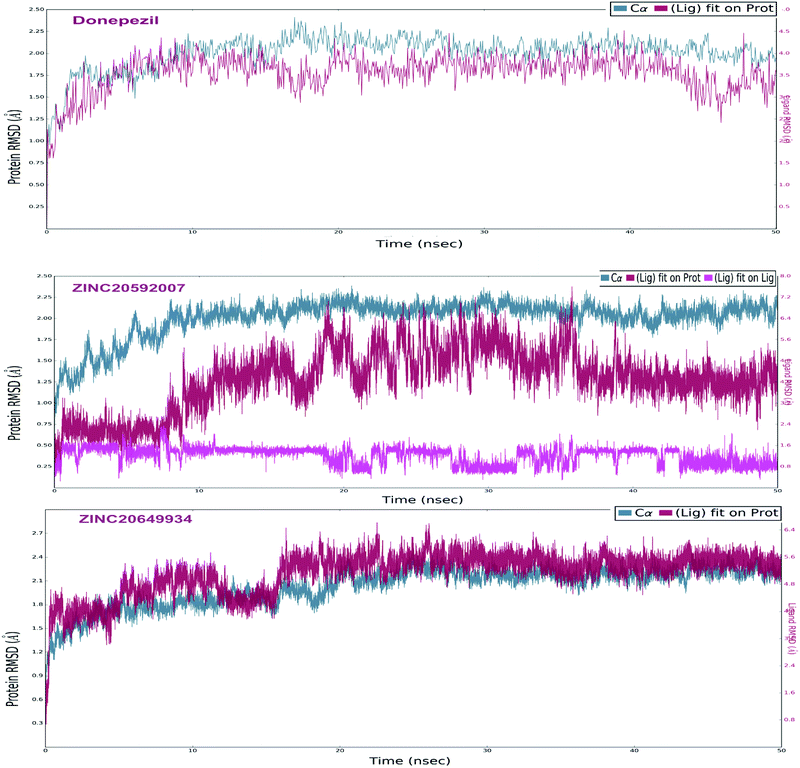 |
| Fig. 11 RMSD plot (donepezil–AChE, ZINC20592007–AChE, and ZINC20649934–AChE complexes) of RMSD values for protein on the left Y-axis and for ligand these values were displayed on right Y-axis; protein backbone in green color, and ligand in maroon color. | |
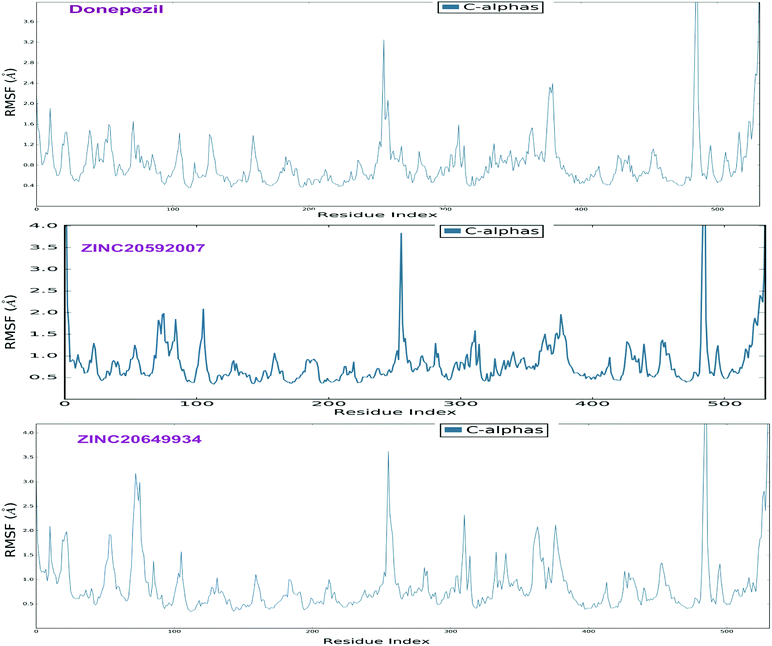 |
| Fig. 12 RMSF of the protein C-α chain in donepezil–AChE, ZINC20592007–AChE, and ZINC20649934–AChE complexes. | |
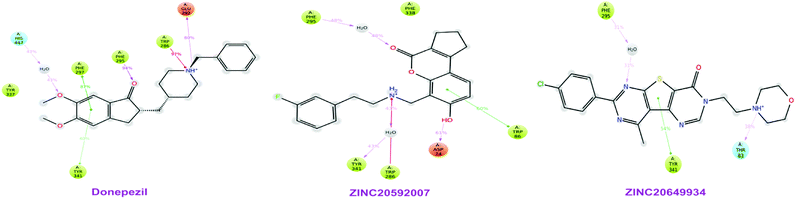 |
| Fig. 13 Schematic diagram of detailed ligand (donepezil, ZINC20592007, and ZINC20649934) interactions with AChE protein amino acid residues after MD simulation. | |
MD study revealed that ZINC20649934 was interacted Phe 295 with H-bonding through a water molecule, Thr 83 residue with direct H-bonding, and Tyr 341 amino acid with π–π stacking; ZINC20592007 interacted Tyr341, Trp 286, and Phe 295 with H-bond formation through a water molecule, Trp86 with direct H-bonding, and Asp 74 with π–π stacking; and donepezil interacted Trp286, Phe297, and Tyr241 with π–π stacking, and Glu292, and Phe295 with H-bond, and His447 through water involvement H-bonding. MD simulation displayed that all the ligands were interacting with protein at Phe 295 and Trp286, which were present at PAS site of AChE.
Materials and methods
Computational details
The computational tasks, except MD, were performed on an Intel(R) Core (TM) i5-3210M CPU @ 2.50 GHz processor with a memory of 8.0 GB RAM running on a Linux 64 operating system. Schrödinger suite 2015-1 (Schrödinger, LLC, New York, NY, 2015) was utilized to develop structure-based and ligand-based pharmacophore models and for the screening of publicly free ‘ZINC15’ database. MD simulation was perform using Desmond package on an Intel(R) Xeon(R) CPU E3-1225v5@ 3.30 GHz 3.31 GHz processor, RAM 32.0 GB system with Nvidia ‘Quadro P600’ GPU running on a Linux 64 operating system.
Development of ligand-based pharmacophore
Total 1062 structurally diverse AChE inhibitors with known and wide range of IC50 values (0.043–20
000 nM) were collected from Binding DB database (2017). The LigPrep in Maestro, Schrödinger 2015-1 was utilized to minimize the energy of inhibitor molecules by applying OPLS_2005 force field.28 As, donepezil has mixed type (PAS and CAS site) of binding properties with AChE, therefore, all the compounds were clustered by Tanimoto similarities against donepezil using linear fingerprint descriptors with Canvas v2.3. Compounds were collected depending upon canvas similarity higher than and equal to 0.15 and molecular weight below 500. Finally, 142 inhibitors (Fig. S9 in ESI†) were selected based on Glide docking study at PAS of AChE. The IC50 values of inhibitors were converted to pIC50 for the generation of 3D-QSAR model. PHASE v4.2, Schrödinger 2015-1 was used to generate 3D-QSAR model.29 The ConfGen, Schrödinger 2015-1 was used to create maximum 1000 number of conformers per structure utilizing force field OPLS_2005. The threshold of actives was above 8.0 and inactives was below 5.7. The PHASE randomly divided all ligands into two sets, i.e., the test set contained 42, and the training set included 100 compounds, to develop an Atom-based 3D-QSAR model in 1.00 Å of grid spacing. The ligands used for development of pharmacophore hypothesis are listed (ESI, Table S7†) with their fitness score, observed pIC50, phase predictive activity, and errors (the difference between observed and predicted activity). The common pharmacophore was obtained from the score of hypotheses having the best alignment of the active set ligands. All 142 compounds were aligned with the template pharmacophore hypothesis of the highly active molecule (Fig. S10 in ESI†).
Validation of ligand-based pharmacophore
The QSAR model was developed with partial least-squares (PLS) factors one to five and was validated by predicting pIC50 value of molecules. The QSAR model with PLS factor 5 was considered as the best model. The 3D-QSAR models were externally validated by using LOO method to evaluate the predictivity of hypotheses.30,31
Energy-optimized structure-based pharmacophore generation
Out of total 15, three X-ray crystal structures of hAChE were collected with good resolution and PAS site AChE inhibition activity of cocrystal ligand from the Protein Data Bank (https://www.rcsb.org). The cocrystal ligands of three PDB structures viz. 4EY7 (donepezil, IC50 5.3 nM), 4M0E (dihydrotanshinone I, Ki 700 nM), and 4M0F (territrem B, IC50 7 nM, & Ki 1.7 nM) are shown in Fig. 14.
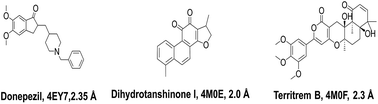 |
| Fig. 14 Cocrystal ligands structure with PDB id and resolution. | |
Protein structures were prepared using protein preparation wizard in Maestro 10.1, Schrödinger 2015-1 with an OPLS_2005 force field. The Grids of all three PDB structures were prepared at the center of cocrystal ligand using receptor Grid Generation tool in Maestro 10.1, Schrödinger 2015-1. The refined crystal ligands were docked by utilizing Glide XP (extra precision) docking with corresponding protein structures. The Glide XP energy was ranked by their contribution for the binding of pharmacophoric sites to cocrystal ligand.32 PHASE v4.2, Schrödinger, 2015-1 was applied to generate pharmacophore features based on XP energy descriptor information. It was used to develop pharmacophore sites viz. H-bond acceptor (A), H-bond donor (D), hydrophobic group (H), negative ionizable group (N), positive ionizable group (P), and aromatic ring (R). H-bond acceptor and H-bond donor were pointed as vectors, directed to corresponding H-bond donor and acceptor positions at the binding site of receptors respectively. The Glide XP descriptors consisted of hydrophobic enclosure, hydrophobically packed associated hydrogen bonds, electrostatic rewards, π–π stacking, π-cation, and other interactions. The most favorable sites were selected for the development of e-pharmacophore hypothesis by using excluded volume.
Energy-optimized structure-based pharmacophore validation
Enrichment factor (EF) and goodness of hit (GH) were calculated to validate e-pharmacophore hypotheses (eqn (1) and (2) respectively). A dataset of compounds was prepared using 1000 drug-like decoys (http://www.schrödinger.com/glide_decoy_set) with an average molecular weight of 400 D (the “dl-400” dataset) and known actives of 53 AChE inhibitors (inhibitors with IC50 less than 100 nM and out of molecules utilized for the 3D-QSAR model), to validate e-pharmacophore models. LigPrep in Schrödinger 2015-1 with Epik was applied to prepare database ligands with an OPLS_2005 force field. EF is the fraction of known actives retrieved after a screening of decoy database compounds.33 |
 | (1) |
|
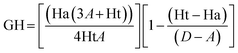 | (2) |
where, EF = enrichment factor, GH = goodness of hit, D = total compounds in the data set, A = total number of actives in the data set, Ht = total hits, and Ha = active hits.
Pharmacophore-based screening of the database
Only ‘hit-like’ compounds without known AChE inhibitors were collected from ‘ZINC15’ database utilizing Lipinski's filter. LigPrep with Epik was employed to prepare database ligands utilizing OPLS_2005 force field. One ligand-based pharmacophore and three e-pharmacophores based matched molecules were separately screened against prepared database compounds with PHASE v4.2, Schrödinger 2015-1.34 Pharmacophore matching was required for the most energetically favorable site, and score of more than 1.0 kcal mol−1 was selected for the pharmacophore screening, four sites for hypotheses with 3 or 4 and five sites for hypotheses with 4 or 5 were required to match. The tolerance of distance matching was set up to 2.0 Å. The aligned conformer of molecule matches the hypothesis based on rmsd, site matching, vector alignments, and volume terms expressed as fitness score.35 The pharmacophore matched database was ranked in order of the fitness score ranging from 0 to 3, as applied in the PHASE. The ligands were selected based on highest fitness scores up to 2000 molecules for each pharmacophore and scores above 1.5 were considered as suitable inhibitors. The molecules with best fitness score were docked into the binding sites of AChE crystal structure.32
Removal of pan-assay interference compounds (PAINS)
Baell and Holloway reported a list of structural features which generated frequent false positives across screening, known as PAINS.24 Jasial S. et al. established a large-scale analysis of behavior of PAINS in biological screening assays.36 The ‘ZINC15’ database molecules are categories within (A) anodyne, and (B) clean (PAINS-ok),37 were selected as hits from HTVS retrieves. A KNIME (freely available Konstanz Information Miner, http://knime.org)38 workflow distributed with the RDKit39 software package utilizing GUI data analysis platform was developed by Saubern S. et al.40 The obtained HTVS hits were screened in silico for PAINS to avoid false positives in biochemical and pharmacological assays using three public filters, including RDKit,39 ZINC,37 and FAF-Drugs4 server.41
High throughput virtual screening (HTVS) and molecular docking
Glide HTVS (high throughput virtual screening) is faster than Glide SP and XP, has higher tolerance to suboptimal fits than Glide XP and thus is selected for the study.33 After removal of PAINS, e-pharmacophore matched compounds were docked into binding sites of respective crystal structures, and ligand-based matched molecules were docked into 4M0E structure with Glide, Schrödinger 2015-1.42 The grid was generated at the center position of cocrystal ligand, through Grid Generation tools in Glide. Post-docking MM-GBSA minimization was performed to optimize the ligand geometries. The Glide HTVS screened molecules with best docking scores were selected for Glide SP (standard precision), and XP (extra precision) screenings. Top 10% of retrieves out from each step were taken up for next step. Finally, all the non-peptide retrieves from HTVS and donepezil were docked in Glide XP molecular docking using 4M0E crystal structure (highest resolution PDB with cocrystal ligand, 2.0 Å) to compare the docking score of screened out retrieves with reference donepezil.
Induced fit docking
We applied a mixed molecular docking and dynamics method known as induced fit docking (IFD),43 where the receptor was flexible in docking study. After ADME screening, selected hits were prepared by OPLS_2005 force field utilizing LigPrep. The hits were docked to rigid protein by using Glide, Schrödinger 2015-1 with scaling of ligand van der Waals (vdW) radii 0.5 for nonpolar atoms.44 Constrained energy minimization was performed on AChE (PDB: 4M0E) crystal structure, keeping it close to the original crystal structure while removing bad steric contacts. The energy minimization of protein structure was performed using OPLS_2005 force-field. The Glide XP was utilized for initial docking, and 20 ligand poses were retained for protein structural refinement. Prime, Schrödinger 2015-1 was used to refine residue within 5.0 Å of ligand poses and to generate the induced-fit protein–ligand complexes. Each of the 20 complexes was subjected to refinements of side-chain and backbone,44 and were ranked according to Prime energy. The receptor structures within 30 kcal mol−1 were redocked for final round of Glide docking and scoring. The Prime refinement included at least one atom of all residue located within 4.0 Å of corresponding ligand pose. In the last step, every ligand was redocked into each refined low-energy receptor structure generated in the refinement step. The new 20 receptor conformations were taken forward for Glide XP redocking. The binding affinity of each complex was reported in the docking score. The more negative docking score indicates more favorable binding with receptor.
Prime MM-GBSA simulation
The free binding energies of highest scoring docked complexes were computed utilizing molecular mechanic-generalized Born surface area (MM-GBSA)45 followed by default parameters. Based on the docking score and MM/GBSA binding-free energy, Jin et al. developed correlation model between docking scores or calculated binding-free energies and experimental pIC50 values.46 The Prime (Maestro v10.1, Schrödinger, LLC, New York, NY, 2015) was employed to calculate the MM-GBSA energy of Glide XP docked complex. The OPLS_2005 force field in conjunction with GBSA continuum model47 was utilized to determine energies of selected complexes of ligands. Computationally, the binding free energies (ΔGbind) of ligands were calculated using the following equation.48
ΔGbind = ΔEMM + ΔGsolv + ΔGSA |
where ΔEMM is the difference between minimized energies of the AChE-inhibitor complex and sum of the minimized energies of unliganded AChE and its inhibitor, ΔGsolv is difference between GBSA solvation energies of enzyme–inhibitor complex and sum of the GBSA solvation energies of unliganded AChE and inhibitor, and ΔGSA is the difference between surface area energies of the complex and sum of the surface area of unliganded enzyme and its inhibitor.
Docking using Autodock
AutoDockTools-1.5.6. and AutoDock 4.2 suite were utilized to redock the selected hits as AChE inhibitor for comparison of the Glide XP docking, IFD, and AutoDock results. AChE crystal structure, 4M0E, was prepared using AutoDock Tools. Atom charges, solvation parameters, and polar hydrogens were added to enzyme structure for docking simulation before applying to PDBQT file format. The Chem3D 16.0 chemical structure drawing software was utilized to draw hits with standard 3D structures and to minimize energies of the compounds using MM2 energy minimization method.49 The AutoDock 4.2 ligand optimization was performed using Gasteiger charges optimization, non-polar hydrogens were merged, and saved as PDBQT file. AutoDock requires pre-calculated grid maps, and the grid must surround the region of active site of AChE. Therefore, the grid box was centered at the active site including Leu 289, Arg 296, Phe 297, Phe 338, Trp 286, Ser 293, Val 294, Phe 295, Tyr 72, Tyr 341, Asp 74, Tyr 124 and Tyr 337 amino acid residues. The grid box size was positioned at 40, 42, and 48 Å and the grid center was set to 20.683, −16.615, 19.006 for x, y and z respectively, covering the active pocket. AutoGrid 4.0 was used to produce a grid with 0.375 Å spacing between grid points. The Lamarckian Genetic Algorithm (LGA) was used to search best conformers, and a maximum of 50 conformers was considered for each compound with the default setting. The Discovery Studio Visualizer was used for visualization of interactions. AutoDock Tools provided various methods for analyzing the results of docking simulations, viz. conformational similarity, visualizing the binding site and its energy, intermolecular energy and inhibition constant.
ADME properties prediction
The QikProp in Maestro 10.1, Schrödinger 2015-1 (ref. 50) was used to predict ADME properties of hit molecules. As the QikProp was unsuitable to neutralize the compounds and generate the descriptors, in the normal mode, hence, neutralization of all molecules was essential before performing QikProp. The QikProp predicted physicochemically significant and pharmaceutically applicable 44 descriptors for the hits. These included principle descriptors, physiochemical properties as well as log
P (octanol/water), QP%, log
HERG, Caco-2 cell membrane permeability, MDCK cell permeability, skin permeability log
Kp and Lipinski's rule of five, which were crucial for rational drug design.51,52
Density functional theory
Density functional theory (DFT) is utilized to determine and validate enzymatic reaction mechanisms and the enzyme active sites. Electronic effects of drug-like compounds play an essential role in the pharmacological effects.53 The most and least active AChE inhibitors of training set were optimized with the final hits in Jaguar (Jaguar v8.7, Schrödinger, LLC, New York, NY, 2015) program utilizing Lee–Yang–Parr correlation functional (B3LYP) theory, and Becke's three–parameter exchange potential54,55 with 6-31G* basis set. The molecular orbital surfaces, atomic electrostatics potential charges (EPS) and molecular electrostatic potential (MESP) were determined to calculate the HOMO and LUMO. The HOMO energy of small ligand molecules can donate electrons during the drug–enzyme complex formation, while LUMO energy manifests the capacity of the molecule to accept the electrons from the protein. The HOMO–LUMO gap energy (difference in HOMO and LUMO energy), expresses the electronic excitation energy, that is essential to compute the molecular reactivity and stability of the drug–protein complex.25
In silico AChE selectivity study
To determine binding affinity of hits towards BuChE, we carried out XP docking of hits using crystal structure of 4BDS (highest resolution PDB of human BuChE, 2.1 Å). The Glide (Glide, Schrödinger, LLC, New York, NY, 2015) was used to perform Glide XP docking in default setting for all docking steps, and the Grid was centralized at the PAS site of BuChE i.e., centralized the residues ASp70, Trp 82, Asn83, Ser198, and Tyr332.56
In vitro AChE and BuChE enzyme inhibition
The AChE and BuChE inhibition studies were performed by Ellman et al. method.26 Four selected hit molecules (ZINC20592007, ZINC05354646, ZINC20649934, and ZINC39154782) out of ten, were procured from MolPort SIA, Riga, Latvia (MolPort id: MolPort-002-672–705, MolPort-002-658-497, MolPort-005-915-644, and MolPort-004-876-009 respectively). The AChE from Electrophorus electricus and BuChE from horse serum (lyophilized powder) (CAS No. 9000-81-1, CAS No. 9001-08-5, respectively) were purchased from Sigma Aldrich, India. Acetylthiocholine iodide (ATCI), butyrylthiocholine iodide (BTCI), 5,5′-dithio-bis(2-nitrobenzoic acid) (DTNB–Ellman's reagent) and phosphate buffer saline (PBS), pH 7.4 were procured from HiMedia Laboratories, India, and donepezil (Sigma Aldrich, India) was used as reference. Six different concentrations (75 μM, 15 μM, 7.5 μM, 3 μM, 0.6 μM, and 0.12 μM) of hits, 0.25 mM DTNB, 0.06unit mL−1 of AChE or BuChE were combined in PBS and incubated at 37 °C for 30 min to determine inhibition of AChE or BuChE. 0.36 mM of the substrate (ATCI or BTCI) was added to reaction mixture before measuring absorbance at 415 nm wavelength by Synergy HTX multi-mode reader (BioTek, USA). The process was performed in triplicate with a blank and control, to calculate the percentage inhibition due to selected hits. The IC50 values, i.e., the concentration of the drug resulting in 50% inhibition of enzyme activity, were determined graphically from inhibition curves (log inhibitor concentration vs. percent inhibition) utilizing GraphPad Prism 5.0, GraphPad Software Inc.57
The enzyme kinetics (the mechanism of inhibition by ligands) of were determined by previously described method.26 Eight concentrations of substrate (ATCI; 0.1–1.15 μM) were incubated with AChE in absence and presence of different concentrations of test molecules (0.25 μM, 0.5 μM & 1 μM for ZINC20592007, and ZINC05354646; and 0.5 μM, 1 μM & 2 μM for ZINC20649934, and ZINC39154782). The absorbance was measured for 30 min at intervals of 5 min at 415 nm wavelength. The products formed during the time frame of 30 min were estimated by Beer–Lambert law. Vmax and Km values of Michaelis–Menten kinetics were computed by nonlinear regression from substrate–velocity curves using GraphPad Prism 5. Linear regression was used to calculate inhibition constant (Ki) utilizing Lineweaver–Burk plots.58 Ki value was determined by Yonetani–Theorell method in which the lines from the double reciprocal Lineweaver–Burk plot were extrapolated to intersect at a point.59 The positive reciprocal x-values of intersecting point were the determined Ki value of hits. The enzyme kinetics assays were performed in triplicate.
Assay of propidium iodide displacement
The molecular modeling studies illustrated that selected hits were PAS selective AChE inhibitors. Propidium iodide is a specific PAS selective ligand, which displays 10-fold fluorescence enrichment when bound to AChE. The displacement by hits was measure of their affinity towards PAS of AChE. Three concentrations (0.24, 1.0, and 3.0 μM) of test compounds, 5 μM AChE from electric eel (eeAChE) in PBS, pH 7.4, were added in black 96-well plates and were kept at room temperature for 6 h.60 The sample solutions were incubated for 15 min with 20 μM of propidium iodide (HiMedia, India), and intensity of fluorescence was measured in excitation and emission modes at 485 and 620 nm, respectively. The assay was carried out in triplicate.
In vitro blood–brain barrier permeation assay
The possible in vitro blood–brain barrier (BBB) permeation of compounds was predicted by parallel artificial membrane permeation assay (PAMPA) of BBB as described by Di L. et al.27 The donor microplates (PVDF membrane, pore size 0.45 μm) and acceptor microplates were obtained from Millipore, Bengaluru, India. The filter surface of donor microplate was impregnated with 4 μL of 20 mg mL−1 porcine brain lipid (Avanti polar lipids, Alabaster) in dodecane (Avra Synthesis, Hyderabad, India), and the acceptor microplates were filled with 200 μL of PBS, pH 7.4. 5 mg mL−1 of test compounds were dissolved in DMSO and diluted with PBS to obtain a final concentration of 100 μg mL−1. The donor well plates were filled with 200 μL of test solution and were carefully placed on the acceptor plate like a sandwich, carrying it undisturbed for 18 h at 25 °C. The donor plates were then removed, and concentration of compounds in acceptor, and donor wells were determined by measuring absorbance. Each well was analyzed at five different wavelengths with three independent performances, and results were explicit as mean ± SEM. The nine commercial drugs with known BBB permeability (verapamil, diazepam, progesterone, atenolol, dopamine, lomefloxacin, alprazolam, chlorpromazine, and oxazepam) were utilized to validate PAMPA model. The above-described method was followed to determine the experimental permeability, Pe(exp) values of these drugs, and data were regressed against Pe(ref) from literature to establish a linear correlation.61
Determination of cellular cytotoxicity and neuroprotection
Neuronal cell line cultures. The human neuroblastoma SH-SY5Y cell line was procured from National Centre for Cell Science (NCCS) Pune, India. Cells were cultured into T25 flasks containing Dulbecco's modified Eagle's medium nutrient mixture F-12 (DMEM-F12), supplemented with 10% fetal bovine serum (FBS), 1 μM glutamine, 50 U mL−1 penicillin, and 50 μg mL−1 streptomycin and were maintained at 37 °C in 5% CO2 humidified air. For MTT assay and neuroprotection study, SH-SY5Y cells were subcultured in 96-well plates at seeding density of 5 × 104 cells per well.
Determination of cell viability and neuroprotection. The MTT (3-(4,5-dimethyl thiazol-2-yl)-2,5-diphenyltetrazolium bromide) assay62 was performed to determine cytotoxicity of selected hits. After 24 h incubation at 37 °C, the medium was changed with test compounds having concentrations of 50 μM and 100 μM, for another 24 h at previously described conditions. 5 mg mL−1 of MTT (Sigma-Aldrich, India) in PBS was added to the culture medium for 4 h at 37 °C. The medium was removed, and the blue formazan crystals formed were dissolved in DMSO and evaluated by measuring absorbance at 570 nm. The test was carried out in triplicate, and results were explicit as mean ± SEM.Neuroprotectivity of selected hits was determined by evaluating their ability to protect SH-SY5Y cells against induced apoptosis by L-glutamate excitotoxicity. Amyloid beta (Aβ) neurotoxicity was triggered by L-glutamate in SH-SY5Y cell line.63 The cells were treated with test compounds, at 25 μM concentration, and incubated for 2 h. After incubation, cells were treated with a medium containing 100 μM of L-glutamate and left for an additional 24 h. The cell viability, after the treatment of L-glutamate, was assessed by MTT assay. The medium was further replaced with 80 μL of fresh medium and 20 μL of MTT (0.5 mg mL−1) in PBS. After 4 h of incubation, MTT solution was removed, and the crystals of formazan were dissolved in DMSO to measure the absorbance at 570 nm. Percentage of neuronal cell protection against L-glutamate was calculated by considering the absorbance of the control cells as 100% of the cell viability.
Molecular dynamics (MD) simulation
MD simulations of ZINC20592007 (most active in in vitro tests and 100% PAS selective), ZINC20649934 (higher docking score in in silico and 58% PAS selective in in vitro), and donepezil were performed utilizing Desmond v2.2, Schrödinger 2015-1 with the OPLS 2005 force field to model all peptide interactions,64,65 and TIP3P (transferable intermolecular potential with 3 points) model was used for solvent. Protein-ligand docked complex (.pv file) from XP docking was taken for solvation using open TIP3P water model in an orthorhombic core box of 20 Å radius. The overall complex had six negative charges and was neutralized by adding Na+ counter ion for simulation. Ligand–protein complex was minimized by steepest descent method followed by BFGS (Broyden–Fletcher–Goldfarb–Shanno) algorithm having a convergence threshold of 2.0 kcal mol−1 and 41
667 iterations. Ewald method (PME)66 was used to calculate long-range electrostatic interactions with a grid spacing of 0.8. van der Waals and short-range electrostatic interactions were truncated at 9.0. Nose–Hoover thermostats67 were utilized to maintain constant simulation temperature, and Martina–Tobias–Klein method68 was used to control pressure throughout simulation. The equations of motion were integrated utilizing the multistep RESPA integrator69 with an internal time step of 2.0 fs for bonded interactions and non-bonded interactions within 6.0 fs cut off. MD simulations were conceded out at 300 K temperature and 1.01325 bar pressure. The overall model system was relaxed for 2 ns before a 50 ns simulation, and coulombic interactions were defined by a short-range cut off radius of 9.0 Å and by a long-range smooth particle mesh Ewald tolerance to 1 × 10−9. Further, for energy calculation and trajectory analysis, recording interval of 1.2 ps was defined.
Conclusions
A 3D-QSAR and three e-pharmacophore models were developed from known AChE inhibitors, structurally similar to donepezil and available AChE crystal structures with cocrystal ligand at PAS site. Virtual screening of ZINC15 compounds afforded new excellent, non-toxic AChE inhibitors. The hits interacted with Trp 286, Phe 295, Asp 74, Tyr 337, and Tyr 124 residues of AChE crystal structure through one to three H-bond(s) and one to three pi–pi stacking interaction(s). MD strongly supported that the identified hits bound at PAS of AChE only. In vitro enzyme assays, with propidium iodide displacement of ZINC20592007, ZINC05354646, ZINC20649934, and ZINC39154782, also supported the in-silico results. ZINC20592007 and ZINC39154782, interacting with Try 286 amino acid residue, provided 100% propidium displacement at 3 μM concentration. The PAS site-selective mimics responded to inhibition of amyloid formation. The hits had attractive Ki values (0.21 ± 0.027 μM, 0.27 ± 0.064 μM, 0.3 ± 0.018 μM, and 0.28 ± 0.032 μM) with insignificant toxicity against neuroblastoma SH-SY5Y cell, good BBB permeability, and neuroprotectivity against L-glutamate induced excitotoxicity.
Further, ZINC20592007 molecule had potent, selective AChE inhibition at PAS, i.e., non-competitive, CNS permeability, non-toxicity, neuroprotectivity, and Aβ formation and aggregation inhibition, which increased cholinergic activity and also prevented Aβ aggregation to control AD. We consider that these compounds are excellent candidates to develop further as leads for AChE inhibition.
Conflicts of interest
The authors declare no competing financial interest.
Abbreviations
AChE | Acetylcholinesterase |
AChEI | AChE inhibitor |
AD | Alzheimer's disease |
ADME | Absorption distribution metabolism and excretion |
APP | Amyloid precursor protein |
BBB | Blood brain barrier |
BuChE | Butyrylcholinesterase |
CNS | Central nervous system |
3D-QSAR | 3-Dimentional-quantitative structure activity relationship |
EF | Enrichment factor |
e-pharmacophore | Energy-optimized pharmacophore |
Glide XP | Glide extra precision |
GH | Goodness of hit |
HTVS | High throughput virtual screening |
IFD | Induced fit docking |
MD | Molecular dynamics |
MTT | 3-(4,5-Dimethylthiazol-2-yl)-2,5-diphenyltetrazolium bromide |
OPLS | Optimized potential for liquid simulations |
PAINS | Pan assay interference compounds |
PAMPA | Parallel artificial membrane permeation assay |
RMSD | Root mean square deviation |
SP | Standard precision |
THA | Tacrine |
1YL | (1R)-1,6-Dimethyl-1,2-dihydrophenanthro[1,2-b]furan-10,11-dione |
Acknowledgements
The authors are thankful to Department of Biotechnology, Ministry of Science & Technology, New Delhi, India for financial support (BT/PR9624/MED/30/1253/2013 dated-29/11/2014). We are grateful to Dr Ozair Alam, Department of Pharmaceutical Chemistry, School of Pharmaceutical Education & Research, Jamia Hamdard, Hamdard Nagar, New Delhi, India, for his assistance and support. S. J. and A. G. would like to thank Ministry of Human Resource Development, New Delhi, India, for the award of senior research fellowships to them.
References
- Y. Bourne, P. Taylor, P. E. Bougis and P. Marchot, J. Biol. Chem., 1999, 274, 2963–2970 CrossRef CAS PubMed.
- G. Johnson and S. Moore, Curr. Pharm. Des., 2006, 12, 217–225 CrossRef CAS PubMed.
- H. Soreq and S. Seidman, Nat. Rev. Neurosci., 2001, 2, 294–302 CrossRef CAS PubMed.
- M. Pakaski and P. Kasa, Curr. Drug Targets: CNS Neurol. Disord., 2003, 2, 163–171 CAS.
- N. P. L. Verhoeff, Expert Rev. Neurother., 2005, 5, 277–284 CrossRef CAS PubMed.
- J. Massoulié, J. Sussman, S. Bon and I. Silman, Prog. Brain Res., 1993, 98, 139–146 Search PubMed.
- J. Massoulie and S. Bon, Annu. Rev. Neurosci., 1982, 5, 57–106 CrossRef CAS PubMed.
- E. O. Campos, A. Alvarez and N. C. Inestrosa, Neurochem. Res., 1998, 23, 135–140 CrossRef CAS PubMed.
- N. C. Inestrosa, A. Alvarez, C. A. Perez, R. D. Moreno, M. Vicente, C. Linker, O. I. Casanueva, C. Soto and J. Garrido, Neuron, 1996, 16, 881–891 CrossRef CAS PubMed.
- M.-S. García-Ayllón, D. H. Small, J. Avila and J. Sáez-Valero, Front. Mol. Neurosci., 2011, 4, 22 Search PubMed.
- F. Ferreri, C. Agbokou and S. Gauthier, J. Psychiatry Neurosci., 2006, 31, 369 Search PubMed.
- S. R. Ribeiz, D. P. Bassitt, J. A. Arrais, R. Avila, D. C. Steffens and C. M. Bottino, CNS Drugs, 2010, 24, 303–317 CrossRef CAS PubMed.
- A. A. Kehagia, R. A. Barker and T. W. Robbins, Lancet Neurol., 2010, 9, 1200–1213 CrossRef PubMed.
- T. Brenner, Y. Hamra-Amitay, T. Evron, N. Boneva, S. Seidman and H. Soreq, FASEB J., 2003, 17, 214–222 CrossRef CAS PubMed.
- T. Brenner, E. Nizri, M. Irony-Tur-Sinai, Y. Hamra-Amitay and I. Wirguin, J. Neuroimmunol., 2008, 201, 121–127 CrossRef PubMed.
- E. Giacobini, Int. J. Geriatr. Psychiatry, 2003, 18, S1–S5 CrossRef PubMed.
- S. Jana and S. K. Singh, J. Biomol. Struct. Dyn., 2018, 1–22 CrossRef PubMed.
- J. K. Dhanjal, S. Sharma, A. Grover and A. Das, Biomed. Pharmacother., 2015, 71, 146–152 CrossRef CAS PubMed.
- P. Ambure, S. Kar and K. Roy, BioSystems, 2014, 116, 10–20 CrossRef CAS PubMed.
- G. Brahmachari, C. Choo, P. Ambure and K. Roy, Bioorg. Med. Chem., 2015, 23, 4567–4575 CrossRef CAS PubMed.
- Y. Zhang, S. Zhang, G. Xu, H. Yan, Y. Pu and Z. Zuo, Mol. BioSyst., 2016, 12, 3734–3742 RSC.
- S. Bag, R. Tulsan, A. Sood, S. Datta and M. Torok, Curr. Comput.-Aided Drug Des., 2013, 9, 2–14 CAS.
- R. Malik, B. S. Choudhary, S. Srivastava, P. Mehta and M. Sharma, J. Biomol. Struct. Dyn., 2016, 1–17 Search PubMed.
- J. B. Baell and G. A. Holloway, J. Med. Chem., 2010, 53, 2719–2740 CrossRef CAS PubMed.
- Y. Zheng, M. Zheng, X. Ling, Y. Liu, Y. Xue, L. An, N. Gu and M. Jin, Bioorg. Med. Chem. Lett., 2013, 23, 3523–3530 CrossRef CAS PubMed.
- G. L. Ellman, K. D. Courtney, V. Andres Jr and R. M. Featherstone, Biochem. Pharmacol., 1961, 7, 88–95 CrossRef CAS PubMed.
- L. Di, E. H. Kerns, K. Fan, O. J. McConnell and G. T. Carter, Eur. J. Med. Chem., 2003, 38, 223–232 CrossRef CAS PubMed.
- Schrödinger, LLC, S. Release, New York, NY, 2015 Search PubMed.
- S. L. Dixon, A. M. Smondyrev and S. N. Rao, Chem. Biol. Drug Des., 2006, 67, 370–372 CrossRef CAS PubMed.
- P. Pratim Roy, S. Paul, I. Mitra and K. Roy, Molecules, 2009, 14, 1660–1701 CrossRef PubMed.
- K. Loving, N. K. Salam and W. Sherman, J. Comput.-Aided Mol. Des., 2009, 23, 541–554 CrossRef CAS PubMed.
- N. K. Salam, R. Nuti and W. Sherman, J. Chem. Inf. Model., 2009, 49, 2356–2368 CrossRef CAS PubMed.
- T. A. Halgren, R. B. Murphy, R. A. Friesner, H. S. Beard, L. L. Frye, W. T. Pollard and J. L. Banks, J. Med. Chem., 2004, 47, 1750–1759 CrossRef CAS PubMed.
- J. J. Irwin, T. Sterling, M. M. Mysinger, E. S. Bolstad and R. G. Coleman, J. Chem. Inf. Model., 2012, 52, 1757–1768 CrossRef CAS PubMed.
- S. L. Dixon, A. M. Smondyrev, E. H. Knoll, S. N. Rao, D. E. Shaw and R. A. Friesner, J. Comput.-Aided Mol. Des., 2006, 20, 647–671 CrossRef CAS PubMed.
- S. Jasial, Y. Hu and J. r. Bajorath, J. Med. Chem., 2017, 60, 3879–3886 CrossRef CAS PubMed.
- T. Sterling and J. J. Irwin, J. Chem. Inf. Model., 2015, 55, 2324–2337 CrossRef CAS PubMed.
- M. R. Berthold, N. Cebron, F. Dill, T. Gabriel, T. Kötter, T. Meinl, P. Ohl, C. Sieb, K. Thiel and B. Wiswedel, Data Analysis, and Knowledge Organisation (GfKL 2007), Springer, 2007 Search PubMed.
- G. Landrum, RDKIT. ORG, 2013 Search PubMed.
- S. Saubern, R. Guha and J. B. Baell, Mol. Inf., 2011, 30, 847–850 CrossRef CAS PubMed.
- D. Lagorce, L. Bouslama, J. Becot, M. A. Miteva and B. O. Villoutreix, Bioinformatics, 2017, 33, 3658–3660 CrossRef CAS PubMed.
- R. A. Friesner, R. B. Murphy, M. P. Repasky, L. L. Frye, J. R. Greenwood, T. A. Halgren, P. C. Sanschagrin and D. T. Mainz, J. Med. Chem., 2006, 49, 6177–6196 CrossRef CAS PubMed.
- H. Wang, R. Aslanian and V. S. Madison, J. Mol. Graphics Modell., 2008, 27, 512–521 CrossRef CAS PubMed.
- R. A. Friesner, J. L. Banks, R. B. Murphy, T. A. Halgren, J. J. Klicic, D. T. Mainz, M. P. Repasky, E. H. Knoll, M. Shelley and J. K. Perry, J. Med. Chem., 2004, 47, 1739–1749 CrossRef CAS PubMed.
- N. Huang, C. Kalyanaraman, J. J. Irwin and M. P. Jacobson, J. Chem. Inf. Model., 2006, 46, 243–253 CrossRef CAS PubMed.
- M. Jin, N. Shepardson, T. Yang, G. Chen, D. Walsh and D. J. Selkoe, Proc. Natl. Acad. Sci. U. S. A., 2011, 108, 5819–5824 CrossRef CAS PubMed.
- Z. Yu, M. P. Jacobson and R. A. Friesner, J. Comput. Chem., 2006, 27, 72–89 CrossRef CAS PubMed.
- P. D. Lyne, M. L. Lamb and J. C. Saeh, J. Med. Chem., 2006, 49, 4805–4808 CrossRef CAS PubMed.
- G. M. Morris, D. S. Goodsell, R. S. Halliday, R. Huey, W. E. Hart, R. K. Belew and A. J. Olson, J. Comput. Chem., 1998, 19, 1639–1662 CrossRef CAS.
- E. M. Duffy and W. L. Jorgensen, J. Am. Chem. Soc., 2000, 122, 2878–2888 CrossRef CAS.
- C. A. Lipinski, F. Lombardo, B. W. Dominy and P. J. Feeney, Adv. Drug Delivery Rev., 1997, 23, 3–25 CrossRef CAS.
- F. Ntie-Kang, SpringerPlus, 2013, 2, 353 CrossRef PubMed.
- J. Matysiak, Eur. J. Med. Chem., 2007, 42, 940–947 CrossRef CAS PubMed.
- P. M. Gill, B. G. Johnson, J. A. Pople and M. J. Frisch, Chem. Phys. Lett., 1992, 197, 499–505 CrossRef CAS.
- P. Stephens, F. Devlin, C. Chabalowski and M. J. Frisch, J. Phys. Chem., 1994, 98, 11623–11627 CrossRef CAS.
- P. Masson, W. Xie, M.-T. Froment, V. Levitsky, P.-L. Fortier, C. Albaret and O. Lockridge, Protein Struct. Mol. Enzymol., 1999, 1433, 281–293 CrossRef CAS.
- H. Motulsky, GraphPad Software, 2007, vol. 31, pp. 39–42 Search PubMed.
- Y. Wang, X.-L. Guan, P.-F. Wu, C.-M. Wang, H. Cao, L. Li, X.-J. Guo, F. Wang, N. Xie and F.-C. Jiang, J. Med. Chem., 2012, 55, 3588–3592 CrossRef CAS PubMed.
- R. A. Copeland, Evaluation of enzyme inhibitors in drug discovery: a guide for medicinal chemists and pharmacologists, John Wiley & Sons, 2013 Search PubMed.
- J. Eichler, A. Anselment, J. L. Sussman, J. Massoulié and I. Silman, Mol. Pharmacol., 1994, 45, 335–340 CAS.
- D. Kumar, S. K. Gupta, A. Ganeshpurkar, G. Gutti, S. Krishnamurthy, G. Modi and S. K. Singh, Eur. J. Med. Chem., 2018, 150, 87–101 CrossRef CAS PubMed.
- J. van Meerloo, G. J. L. Kaspers and J. Cloos, in Cancer Cell Culture: Methods and Protocols, ed. I. A.Cree, Humana Press, Totowa, NJ, 2011, pp. 237–245, DOI:10.1007/978-1-61779-080-5_20.
- X. Di, J. Yan, Y. Zhao, J. Zhang, Z. Shi, Y. Chang and B. Zhao, Neuroscience, 2010, 168, 778–786 CrossRef CAS PubMed.
- W. L. Jorgensen, D. S. Maxwell and J. Tirado-Rives, J. Am. Chem. Soc., 1996, 118, 11225–11236 CrossRef CAS.
- G. A. Kaminski, R. A. Friesner, J. Tirado-Rives and W. L. Jorgensen, J. Phys. Chem. B, 2001, 105, 6474–6487 CrossRef CAS.
- U. Essmann, L. Perera, M. L. Berkowitz, T. Darden, H. Lee and L. G. Pedersen, J. Chem. Phys., 1995, 103, 8577–8593 CrossRef CAS.
- W. G. Hoover, Phys. Rev. A: At., Mol., Opt. Phys., 1985, 31, 1695 CrossRef.
- G. J. Martyna, D. J. Tobias and M. L. Klein, J. Chem. Phys., 1994, 101, 4177–4189 CrossRef CAS.
- S. J. Basha, P. Mohan, D. P. Yeggoni, Z. R. Babu, P. B. Kumar, A. D. Rao, R. Subramanyam and A. G. Damu, Mol. Pharm., 2018, 15, 2206–2223 CrossRef CAS PubMed.
Footnote |
† Electronic supplementary information (ESI) available: Results of DFT study, graphs, and calculations of enzyme inhibition study, experimental procedure, and calculations of PAMPA study; Structures of known AChE inhibitors with test and training sets for development of pharmacophore; identified virtual hits alignment with their pharmacophores and interaction images of hits. See DOI: 10.1039/c8ra08198k |
|
This journal is © The Royal Society of Chemistry 2018 |