DOI:
10.1039/C8RA06347H
(Paper)
RSC Adv., 2018,
8, 33960-33967
A colorimetric biosensor based on enzyme-catalysis-induced production of inorganic nanoparticles for sensitive detection of glucose in white grape wine†
Received
27th July 2018
, Accepted 25th September 2018
First published on 3rd October 2018
Abstract
Sensitive and selective colorimetric sensors have come into a high demand due to their simplicity, rapidity, precision and use of common laboratory instruments. In this study, as a new colorimetric nanoprobe, enzyme-catalysis-induced production of Prussian blue nanoparticles (PBNPs) was employed to develop a colorimetric biosensor which was simple and inexpensive for the rapid detection of glucose in wine. Briefly, glucose as the detection target was added into a solution of glucose oxidase (GOx), FeCl3 and K3Fe(CN)6, which turned the solution color from light-yellow to blue within 10 min. Thus, it could be probed by UV-vis spectroscopy. Unlike common colorimetric methods based on a sole color change mechanism, this method has two paths to generate PBNPs. Because both K3Fe(CN)6 and O2 are involved in the turnover of GOx catalysis, they generate K4Fe(CN)6 and H2O2 that reduces Fe3+, respectively, and both paths finally produce PBNPs. This dual-path method enhances the yield of PBNPs and the detection performance. Under optimized conditions, the method presented a linear detection range of 4 μM to 0.5 mM (r2 = 0.998) and a limit of detection of 3.29 μM, which is comparable to or better than analogues, as well as excellent selectivity. This method also worked well in white grape wine samples with detection results varying within 1% to those obtained by the standard HPLC method. The proposed biosensing method is rapid, simple, low-cost, sensitive and selective, therefore, it is a promising method for daily detection of glucose in food in households and markets.
Introduction
Sugar-sweetened beverages, such as wine, fruit drinks sports drinks, sweetened tea and coffee with added sugar, are popular daily drinks.1 The total sugar content is one of the important indexes that affect food quality. Sugar is easy to be absorbed by human body in the form of glucose and fructose, which not only provides materials for the body's metabolism and storage capability, but also helps digestion and mediation of lipid and protein metabolism. Moreover, sugar is a key factor related to the taste. Glucose, as a major kind of reducing sugar existing in most beverages, has a direct relationship with the total sugar content. Therefore, it is significant to detect glucose content in the food industry for ensuring good food quality.2 Commonly, glucose is detected by conventional methods, such as titration and chromatography. However, the end-point of the titration method is difficult to judge, especially for beginners.3,4 Standard methods based on chromatography for the detection of glucose in foods, such as liquid chromatography (LC)/high-performance liquid chromatography (HPLC), have high sensitivity, specificity, and repeatability, but they are time-consuming. However, due to the requirement of expensive instruments and skilled personnel,5–7 the chromatography method obviously cannot satisfy the need for a simple, rapid and cost-effective detection. With development of nanomaterials and technology, numerous glucose biosensors have been fabricated.8–10 However, these glucose biosensors more or less suffer from complicated principles, difficult design, and relatively expensive.11 Thus, it is necessary to develop an innovative in-field or on-site test method to detect glucose in wine in a processing plant, food market, restaurant or household.
Colorimetric methods have shown great potential for inexpensive daily-life applications for their obvious advantages of simplicity, low cost, and no requirement of any expensive instruments. Besides, the color change of substrates can be observed easily by naked eyes.10,12–14 For example, gold nanoparticle-based colorimetric biosensing assays have attracted considerable attentions in many commercial applications due to their simplicity and versatility.15,16 Prussian blue (PB) has been extensively used as a pigment in the formulation of paints, lacquers, and printing inks throughout several hundred years history, due to its highly distinguishable blue color.17,18 PB is a kind of mixed-valence compounds which can be readily obtained by simply mixing Fe3+ and Fe(CN)64− or mixing Fe2+ and Fe(CN)63−.19,20 Therefore, PB has been developed as a kind of colorimetric probes to design colorimetric detection methods for a variety of targets such as phenolic,21 ascorbate,22 morphine23 and hydralazine,24 which were mainly based on the reaction of these targets with one of precursors of PB. However, for, it is a challenge to develop PB-based colorimetric method for detection of those targets that are not reactive to precursors of PB, such as glucose.
In this research, a new colorimetric biosensor was developed by bridging the production of PB nanoparticles (PBNPs) as colorimetric probe with the existence of glucose by enzyme-catalysis for simple, inexpensive and rapid detection of glucose in white grape wine. The PBNPs generated through two paths related to the enzyme catalysis in this system doubled the efficiency of the yield of PBNPs and in turn amplified the detection signal. Accordingly, the proposed colorimetric biosensor presented satisfactory performance for the detection of glucose in white grape wines.
Experimental section
Materials and apparatus
Potassium ferricyanide (K3Fe(CN)6), potassium chloride (KCl), ferric chloride (FeCl3·6H2O), 30% H2O2 aqueous solution, potassium hydroxide (KOH) and hydrochloric acid (HCl, 12 M), were obtained from Sinopharm Chemical Reagent Co., Ltd. (Shanghai, China). GOx (EC 1.1.3.4; type II from Aspergillus niger, 150 kU g−1) was purchased from Sigma. Potassium ferrocyanide (K4Fe(CN)6·3H2O), 2-morpholinoethanesulfonic acid (MES, C6H13O4NS·H2O), maltose, lactose, galactose, mannose and fructose were purchased from Sangon Biotech (Shanghai, China). MES solution of 50 mM was used. HPLC grade glucose standard sample and acetonitrile were purchased from Sigma-Aldrich (St. Louis, MO, USA). All other chemicals were of analytical-reagent-purity grade and were used as received without further purification. Milli-pore ultrapure water (18.2 MΩ cm, Millipore, Billerica, MA, USA) was used throughout. The solution pH was adjusted with HCl (12 M) and KOH (12 M). Unless especially noted, all experiments were performed at room temperature.
Transmission electron microscopy (TEM) images were collected on JEOL JEM-1200EX transmission electron microscope (Tokyo, Japan). UV-vis spectrophotometry was conducted on Synergy H1 Hybrid Multi-Mode Microplate reader (BioTek, Winooski, VT, USA). HPLC was performed using a Shimadzu LC-20A HPLC system with refractive index detector (RIA-10A), NH2 column (250 mm × 4.6 mm, 3.5 μm), SIL-20A Prominence autosampler and Prominence CTO-20AC column oven (Shimadzu Corporation, Japan).
Samples and pretreatment
Two types of white grape wine (half sweet and sweet), J.J.Muller wine (Jerry Moselle, Germany) and J.W.Morris wine (California, USA), were bought from a local supermarket (Hangzhou, China). The white grape wine samples were filtered by 0.22 μm-pore size filtration membrane (Millipore, Billerica, MA, USA) three times, and stored at 4 °C.
HPLC analysis of glucose
Standard HPLC method was used for the determination of glucose in different samples.25 HPLC parameters included using the auto-sampler at a flow rate of 0.5 mL min−1, mobile phase of 75% acetonitrile and 25% sterile ultrapure water, with 20 μL injection of each sample through a guard column followed by a NH2 column at 35 °C, and refractive index detector (RIA-10A) with the evaporator temperature of 60 °C, and the nebuliser temperature of 40 °C. The concentrations of glucose were calculated based on the signal of a sample at corresponding retention times.25
Procedures for detection of glucose using the colorimetric biosensor
For glucose assay, the mixture of 5 μL of 2.2 to 44 mM glucose, 50 μL of 1.5 mM K3Fe(CN)6 and 50 μL of 1.5 mM FeCl3 solution in 50 mM MES (pH 3.0) were prepared in a well of a 96-well plate, followed by the adding of 5 μL of 1.1 mg mL−1 GOx in 50 mM MES (pH 3.0). Then, the absorbance of the solution was measured at a wavelength of 706 nm against a blank prepared with the same reagent concentrations after 10 min.
For testing the selectivity of the glucose assay, procedures were the same as that for the detection of glucose, except the replacement of glucose solution with other sugar solutions of 110 mM (maltose, lactose, galactose, mannose or fructose).
Enzymatic kinetic analysis
Enzymatic kinetic measurements were carried out by monitoring the absorbance change at 706 nm. First, the mixture of 5 μL of 2.2 to 176 mM glucose, 50 μL of 0.5 mM K3Fe(CN)6 and 50 μL of 0.5 mM FeCl3 solution in 50 mM MES (pH 3.0) were prepared in a well of 96-well plate, followed by the addition of 5 μL of 0.4 mg mL−1 GOx in 50 mM MES (pH 3.0). Then, the absorbance of solution was monitored at 706 nm against a blank prepared with the same reagent concentrations. The catalytic efficiency of GOx was evaluated by absorbance versus time. The Michaelis–Menten constant was calculated from Lineweaver–Burk plots of the double-reciprocal form of the Michaelis–Menten equation (eqn (1)). |
1/V = (Km/Vmax)(1/[S]) + 1/Vmax
| (1) |
where V is the initial velocity, [S] is the concentration of the substrate, Km is the Michaelis–Menten constant and Vmax is the maximal reaction velocity.
Results and discussion
The mechanism of the proposed enzyme-catalysis-induced production of PBNPs
As the key part of the proposed colorimetric method, the mechanism of the enzyme-catalysis-induced production of PBNPs was investigated. First, FeCl3 and K3Fe(CN)6 solutions were mixed with glucose or/and GOx, and the UV-vis spectra and digital pictures of each sample were collected. As shown in Fig. 1, the mixture of FeCl3 solution and K3Fe(CN)6 (sample 1) was clear and presented light-yellow in color, which had minor change after mixing with glucose (sample 2) or GOx (sample 3). The UV-vis spectra showed peaks at 429 nm, which was ascribed to the presence of K3Fe(CN)6. No peak appeared at a wavelength range from 500 to 1000 nm. However, after the mixing with glucose and GOx with the mixture of FeCl3 and K3Fe(CN)6 solutions (sample 4), the solution gradually turned to blue after 2 min. The UV-vis spectra clearly showed a new peak at 706 nm, which was the classic peak for PB formation.26 The results demonstrated the production of PB through the GOx catalysis.
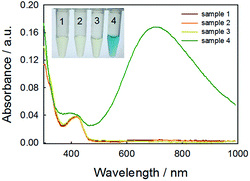 |
| Fig. 1 UV-vis spectra and digital image (inset) of several reaction systems: FeCl3/K3Fe(CN)6 (1), FeCl3/K3Fe(CN)6/glucose (2), FeCl3/K3Fe(CN)6/GOx (3), and FeCl3/K3Fe(CN)6/GOx/glucose (4). Reaction conditions: 0.25 mM FeCl3, 0.25 mM K3Fe(CN)6, 0.05 mg mL−1 GOx, and 1 mM glucose in 50 mM MES (pH 3.0) at 25 °C, reaction for 10 min. | |
Furthermore, we used TEM to investigate the formation of PBNPs. Sample 4 was centrifuged (10
000 rpm) for 10 min and the supernatant was removed. The precipitates were washed with diluted hydrochloric acid (0.2 M) three times. The size and morphology of the precipitates were imaged by TEM, as shown in Fig. 2. The samples of PBNPs prepared by conventional method, namely, mixing FeCl2 and K3Fe(CN)6, were characterized by TEM. Both samples present nanoparticles. The diameters are 21.9 ± 4.5 nm and 8.1 ± 2.6 nm for conventionally prepared and the GOx-catalysis-based PBNPs, respectively. Therefore, the TEM also proves the production of PBNPs based on GOx-catalysis. The averaged diameter of the PBNPs from conventional method was much bigger than the ones from GOx-catalysis. The reason may be that amide moieties (GOx here) can weakly bind to iron ions through a coordinating interaction. So, GOx stabilized the PB nanoparticles and prevented the further aggregation of the particles.27
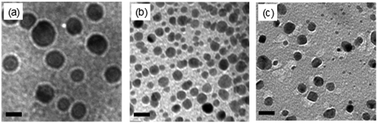 |
| Fig. 2 TEM images of PBNPs prepared from FeCl2 and K3Fe(CN)6 (a), FeCl3, K3Fe(CN)6, glucose, and GOx (b), FeCl3, K3Fe(CN)6 and H2O2 (c). Reaction conditions: 0.25 mM FeCl3, 0.25 mM K3Fe(CN)6, 0.05 mg mL−1 GOx, 1 mM glucose and 1 mM H2O2 in 50 mM MES (pH 3.0) at 25 °C. Scale bar: 20 nm. | |
Although we have validated the production of PBNPs, the specific mechanism was still not clear. It's well known that the H2O2 is generated from GOx-catalyzed glucose oxidation with the presence of O2. Therefore, we investigated the possibility that H2O2 led to the formation of PBNPs. To verify this reaction, H2O2 was added to the mixtures of FeCl3 and K3Fe(CN)6 in MES solution (pH 3.0). The mixture of FeCl3 and K3Fe(CN)6, and the one of FeCl2 and K3Fe(CN)6, were used as the controls. UV-vis absorption spectra and the photographs of sample were collected. As shown in Fig. 3, the mixture of FeCl3 and K3Fe(CN)6 kept clear and light-yellow (sample 1), and the mixture of FeCl2 and K3Fe(CN)6 rapidly turned to blue (sample 2). The UV-vis spectra also showed no peaks and an obvious peak at 706 nm for those two control samples, respectively. Obviously, the addition of H2O2 (sample 3) immediately turned into blue too, and UV-vis spectrum shows a peak at 706 nm, clearly indicating the production of PB. TEM image of the sample 3 (Fig. 2c) also displayed nanoparticles of 11.3 ± 3.5 nm. Based on above results and previous reports,19,26 the mechanism of the formation of the PBNPs based on GOx-catalysis was proposed as bellow. First, H2O2 is generated through the glucose oxidation catalyzed by GOx in the presence of O2 (eqn (2)).10 Secondly, H2O2 reduces Fe3+ to be Fe2+, which reacts with the co-existing K3Fe(CN)6 to form PB.28–30
|
 | (2) |
|
2Fe3+ + 2Fe(CN)63− + H2O2 + 2K+ → 2KFe[Fe(CN)6] + O2 + 2H+
| (3) |
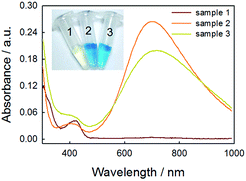 |
| Fig. 3 UV-vis spectra and digital image (inset) of several reaction systems: FeCl3/K3Fe(CN)6 (1), FeCl2/K3Fe(CN)6 (2), and FeCl3/K3Fe(CN)6/H2O2 (3). Reaction conditions: 0.25 mM FeCl2, 0.25 mM FeCl3, 0.25 mM K3Fe(CN)6 and 1 mM H2O2 in 50 mM MES (pH 3.0) at 25 °C, reaction for 10 min. | |
After verifying the first path for the formation of PBNPs, we also explored other possibility. Here, we tried the way by mixing GOx, glucose, FeCl3 and K3Fe(CN)6 similarly, but all of solutions were free of O2 by pumping N2 for 30 min. Interestingly, we still observed the formation of PB, and UV-vis spectrum also displayed a peak of PB at 706 nm, as shown in Fig. 4. Because the path that relied on the enzyme-catalysis-generated H2O2 was excluded, the path turns to the reaction of GOx and Fe(CN)63−. As well known, Fe(CN)63− has well adopted as electron donor to mediate the turn-over of the reduction state to oxidation state of GOx, which is the basic fact of the second-generation glucose electrochemical biosensor.10,31 According to this fact, we propose the second path of PBNPs formation based on GOx-catalysis as below. After the oxidation of glucose by GOx, the GOx turns to reduction state (eqn (4))10,31,32 and the latter is reduced to Fe(CN)64− (eqn (5)). Finally, Fe(CN)64− reacts with Fe3+ and forms PB. For the catalysis of glucose by GOx, the first step is always the oxidation of glucose by GOx, and during this process the original oxidative GOx is turned to reductive GOx. Then, if it is the oxygen that oxidizes the reductive GOx and generates H2O2, it is the first path; if it is the K3Fe(CN)6 that oxidizes the reductive GOx, it is the second path. Both paths regenerate the reductive GOx to be the oxidative GOx and to initiate another catalysis cycle. Because there are both oxygen and K3Fe(CN)6 existing in the reaction solution, two paths occurred simultaneously.
|
GOxox + Glucose → GOxred + Gluconic acid
| (4) |
|
GOxred + Fe(CN)63− → GOxox + Fe(CN)64−
| (5) |
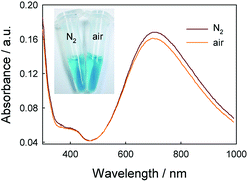 |
| Fig. 4 UV-vis spectra of FeCl3/K3Fe(CN)6/GOx/glucose under air and nitrogen. Reaction conditions: 0.25 mM FeCl3, 0.25 mM K3Fe(CN)6, 0.05 mg mL−1 GOx, and 1 mM glucose in 50 mM MES (pH 3.0) at 25 °C, reaction for 10 min. | |
It's interesting that the UV-vis spectra recorded in the N2 atmosphere has a higher absorbance than in presence of oxygen in Fig. 4, which is incompatible with common sense. There is the difference between UV-vis spectra in the presence and in absence of O2. In order to discriminate the path that generates the larger amount of PBNPs, the effect of oxygen on the catalysis reaction was studied. From the UV-vis spectra recorded by mixing GOx, glucose, FeCl3 and K3Fe(CN)6 similarly in presence of different concentrations of O2 in Fig. S1,† the absorbance was lower with higher O2 concentration. It meant that PBNPs was produced under oxygen catalyst and inhibited in higher O2 concentration during GOx catalysis. In order to explore the role of H2O2 during PBNPs generation, we tried to mixing FeCl2 and K3Fe(CN)6 in presence of H2O2 (Fig. S2†). It was found that the absorbance of PBNPs was lower with higher H2O2 concentration. Moreover, the lowest absorbance existed in 0.8 mM H2O2 which was 87.86% of the absorbance without H2O2, when the concentration of H2O2 was lower than 2 mM. The yield of PBNPs was inhibited in higher H2O2 concentration during FeCl2/K3Fe(CN)6 reaction. So, there was a balance in FeCl3/K3Fe(CN)6/H2O2 reaction. A reasonable explanation was that the production of PBNPs are coupled with the Fenton reaction, in which hydroxyl radicals (HO˙) are generated through the catalytic reaction of Fe2+/Fe3+in the presence of hydrogen peroxide.33,34 After verifying two paths for the formation of PBNPs, the path without O2 that generated the larger amount of PBNPs was the major path.
Therefore, we have confirmed that there were two paths simultaneously existing in this system to produce PBNPs, as illustrated in Scheme 1. In detail, GOx oxidizes glucose and turned itself to reduction state, followed by recovering to oxidation state, which was realized by the reaction with co-exiting O2 (path 1) and K3Fe(CN)6 (path 2). Between the two ways, the signal was mainly generated by path 2. As well known, GOx catalysis suffered from O2 deficiency when the glucose concentration was high, because the concentration of O2 in water was ∼1.3 mM.35 This shortcut often brought inconvenience to the detection of glucose at higher concentrations. Therefore, this dual-path method should effectively overcome the deficiency of O2 and increase the efficiency of the formation of PB, in turn amplify the detection signal.
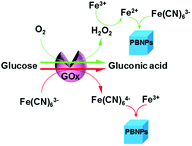 |
| Scheme 1 Illustration for the proposed colorimetric biosensor method for detection of glucose. | |
Optimization of reaction parameters
The enzyme activity and PBNPs stability are both affected by pH. Because GOx generally exhibits the largest activity in neutral solution, while PB is stable in acidic solution. Therefore, a balance of pH for both paths is a key point. The effect of the acidity of the solution on the absorbance of the reaction system was investigated in 50 mM MES solution. The experiment was carried out by changing the pH of 50 mM MES solutions from 1.0 to 7.0. First, the absorbance of PBNPs by mixing FeCl2 and K3Fe(CN)6 in different pH MES solutions were studied. It can be seen that the absorbance of PBNPs through FeCl2/K3Fe(CN)6 reaction in pH 6.0–7.0 were low (Fig. S3†), in which it was not suitable for PBNPs formation. Then the absorbance of PBNPs by GOx-catalysis in different pH MES solutions were studied. The results showed that the absorbance of the PBNPs in pH 3.0 MES solution was the highest and the typical blue color was clearly observed by naked eyes in the corresponding digital image (Fig. S4†). The optimum pH 3.0 for the GOx-catalysis was very closed to pH 2.8 which was suitable for the homogeneous Fenton process.33 This GOx-catalytic reaction was propagated by the reduction of Fe3+ to Fe2+ in Fenton reaction, which was the key reaction step in path 1. In Fig. S4,† the absorbance has higher value at pH 7. The reason may be that GOx enzyme had high activity in neutral pH. However, Fe3+ was stable at acidic solution rather than at neutral pH. Considering the generation of PB was determined both by the GOx catalysis and the supply of Fe3+ ions, there should be a point to balance the conflicted reaction parameters. The role of MES solution was not a buffer but a solution to provide enough ion strength for the dissolution of the enzyme. The buffer role of the solution was from the acid itself. In the proposed method, 0.5 mM glucose could theoretically generate 0.5 mM gluconic acid through the GOx catalysis. The pKa of gluconic acid is 3.86,36 therefore, 0.5 mM gluconic acid could generate 6.9 × 10−8 M H+, which should be negligible to the pH change of the reaction system. To verify this, we monitored the pH of the reaction solution during the experiments in Fig. S5.† It clearly showed that there was minor pH change during the reaction. In Fig. S6,† there was 3.1 ± 1.3% of absorbance change at 706 nm in MES compared with that in hydrochloric acid, demonstrating that the presence (reducing ability) of MES had negligible influence on the detection system. MES solution was just used to keep suitable ionic strength for enzyme reaction. Therefore, we carefully optimized the influence of pH and found that pH 3.0 was the best to the production of PB for detection.
To obtain the high sensitivity, GOx should be enough for catalytic reaction, the effect of GOx concentration was evaluated from 0 to 0.8 mg mL−1. The dissolved O2 acts as a natural mediator for GOx turnover, but it is of only ∼1.3 mM saturated concentration in water.35 Although K3Fe(CN)6 could also supply the catalysis, in order to ensure catalysis efficiency, glucose concentration was selected to be 1 mM. As shown in Fig. S7,† when the GOx concentration was in the range of 0.05–0.1 mg mL−1 with the presence of 1 mM glucose, the absorption at 706 nm increases rapidly, because, obviously, more GOx should catalyze to produce more PBNPs. When the GOx concentration was in the range of 0.1–0.8 mg mL−1, the absorbance reached a platform, which meant GOx in these concentrations were sufficient for the catalysis. Therefore, the GOx concentration of 0.05 mg mL−1 was used in the further study.
To ensure the highest yield of PBNPs, the molar ratio of precursors of PBNPs at 706 nm was examined. The total volume of 0.5 mM FeCl3 and 0.5 mM K3Fe(CN)6 were 200 μL while the volume ratio of two solutions varied from 0 to 19. As shown in Fig. S8,† increasing the amount of FeCl3 gradually deep the blue color of the solutions. When the molar ratio was from 1 to 1.5, the absorbance of PBNPs at 706 nm became the highest. Therefore, the molar ratio of Fe3+ to Fe(CN)63− of 1 was used further.
Furthermore, the effect of FeCl3 concentrations on the absorption of the reaction was investigated, and the results were shown in Fig. S9.† When the FeCl3 concentration was 0.75 mM with the presence of 1 mM glucose, the absorption of the system reached the maximum. When the Fe3+ concentration was higher than 2 mM, the absorption decreased rapidly due to the precipitation of large-size PBNPs. Therefore, the FeCl3 concentration of 0.75 mM was used in the further study.
The reaction time was optimized by monitoring absorbance change for 24 min at 706 nm. As shown in Fig. S10,† after the initiation of the catalysis, the color of the solution rapidly turned to blue and the absorbance increased significantly. When reaction time increased to 10 min, the absorbance reached the plateau, which meant the saturation of catalysis. Therefore, 10 min was selected for further detection. This short reaction time should highlight the advantage in time of the proposed method favored for rapid detection.
Enzymatic kinetic analysis
To investigate the catalytic activity of GOx in this system and to evaluate the performance of the proposed method, we examined enzymatic kinetic parameters using PBNPs as chromogenic probe and glucose/O2/K3Fe(CN)6 as substrates. The catalytic activity of GOx was evaluated by means of steady-state kinetics by changing the concentrations of glucose (Fig. S11a†). Typical Michaelis–Menten curve was obtained by monitoring the absorbance change at 706 nm for 10 min. Michaelis–Menten constant Km and maximum initial rate Vmax were obtained from a Lineweaver–Burk plot (Fig. S11b†). Km value and Vmax for GOx to glucose were 5.40 mM and 1.46 × 10−3 mM s−1, respectively. The Km value obtained was lower than that of the enzyme in other studies (25–27 mM).37,38 This reflected the higher affinity of GOx for glucose in the reaction with the high activity, which should facilitate the production of PBNPs and the final performance of detection.
Evaluation of the colorimetric biosensor for glucose detection
Using the optimal parameters, quantitative detection of glucose was performed on the basis of the absorbance change at 706 nm. As shown in Fig. 5a, the typical blue color reaction was clearly observed by naked eyes and the variation of color depth with the glucose concentration was visual. This method showed good linearity over the range of 0.004–0.5 mM (Fig. 5b), and the linear regression equation was y = 0.87x + 0.01 with a correlation coefficient (r2) of 0.998. The limit of detection (LOD) was calculated to be 3.29 μM (S/N = 3). This indicated good performance of this reaction system for glucose determination. We also compared the sensing performances of this method with those of analogues, as summarized in Table 1. Obviously, although this method has not used any signal-amplification materials (such as nanomaterials), the performance was comparable to or better than other analogues. Besides the good performance, the low-cost, rapidity and the simplicity should add more to the whole value of this method.
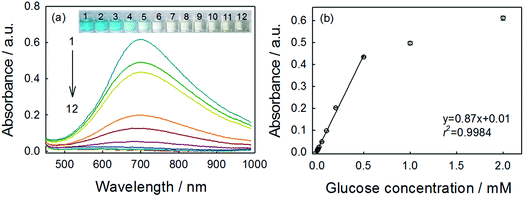 |
| Fig. 5 UV-vis spectra (a) of different concentrations of glucose in the presence of 0.05 mg mL−1 GOx, 0.75 mM FeCl3 and 0.75 mM K3Fe(CN)6 in 50 mM MES (pH 3.0) reaction for 10 min. Glucose concentrations (all in mM) from top to bottom (1 to 12): 2, 1, 0.5, 0.2, 0.1, 0.05, 0.02, 0.01, 0.008, 0.004, 0.001, and 0. The inset represented the corresponding samples. The linear relationship between the absorbance and concentrations of glucose (b) was also given. | |
Table 1 Comparison of the performances of glucose colorimetric sensors
Colorimetric sensora |
Linear range (μM) |
LOD (μM) |
Reference |
TMB: 3,3′,5,5′-tetramethylbenzidine; CTDI: coronene bisimide derivative; ABTS: 2,2′-azino-bis(3-ethylbenzothiazoline-6-sulfonic acid) diammonium salt; H2TCPP: 5,10,15,20-tetrakis(4-carboxyl pheyl)-porphyrin. |
Fe3O4@C/TMB |
6–100 |
2 |
39 |
Graphene oxide/GOx/TMB |
1–20 |
1 |
40 |
CuZnFeS/GOx/TMB |
16–60 |
4.1 |
41 |
MnSe-g-C3N4/GOx/TMB |
160–1600 |
8 |
42 |
CTDI/GOx/TMB |
1–40 |
1 |
43 |
H2TCPP-NiO NPs/GOx/TMB |
50–500 |
20 |
44 |
Au@TiO2/GOx/TMB |
0–10 |
3.5 |
45 |
PBNPs/GOx/ABTS |
0.1–50 |
0.03 |
46 |
PBNPs/GOx |
4–500 |
3.29 |
This work |
The selectivity of biosensor was extremely important for detecting the target accurately. To verify the sensing selectivity of GOx-catalysis-induced PBNPs system toward glucose, several glucose analogues, such as lactose, maltose, galactose, mannose and fructose, were tested. As shown in Fig. 6, even the concentration of these analogues were 5 times higher than that of glucose, their presence did not result in a significant variation of the absorbance, indicating that these analogues had almost no interferences with the detection of glucose. This proved that the proposed method had a high selectivity toward glucose sensing, which should be ascribed to the specificity of GOx to glucose in the catalytic reaction.
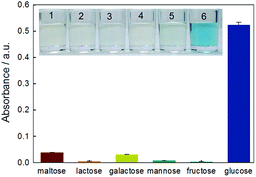 |
| Fig. 6 UV-vis absorbance responses of the proposed biosensor to 5 mM maltose, 5 mM lactose, 5 mM galactose, 5 mM mannose, 5 mM fructose and 1 mM glucose (1 to 6). Inset: sample images of above reactions. Conditions: 0.75 mM FeCl3, 0.75 mM K3Fe(CN)6 and 0.05 mg mL−1 GOx in 50 mM MES (pH 3.0) at 25 °C. | |
Determination of glucose concentration in white grape wine samples
In order to evaluate the feasibility for food sample, the proposed method was applied to the determination of glucose concentration in white grape wine samples. Two kinds of white grape wine (half sweet type and sweet type) purchased from a local supermarket were selected. Wine liquids were filtered by 0.22 μm-pore size water filtration membrane three times to remove solid contents. Then, the wine samples were diluted 5 times with deionized water and analyzed by the proposed method. The two types of white grape wine treated with the proposed method had strong absorbance at 706 nm, as shown in Fig. 7. Simultaneously, the typical blue color reaction was clearly observed by naked eyes in the experimental samples, while the control samples without GOx were light yellow. It's known that there was a variety of reducing species, such as phenolic, ascorbate, and more existing in white grape wine. Here we tested the effect of co-existing species, for example, sulfite, polyphenolic, ascorbate acid in white grape wine. According to the previous reports,47–49 the sodium sulfite, gallic acid and ascorbate acid were added into two types of white grape wine, in which the added concentration of the sodium sulfite, gallic acid and ascorbate acid were equal to their original concentrations, followed by collecting absorbance at 706 nm with UV-vis spectroscopy using the proposed method. For comparison, the original wine samples were adopted as the controls and detected by the proposed method too. As shown in Fig. S12,† the additions of sodium sulfite, gallic acid and ascorbic acid caused 1.3 ± 1.0%, 3.2 ± 0.1% and 1.3 ± 0.6% changes in the sweet wine (Fig. S12a†), respectively, as well as 2.9 ± 2.2%, 1.3 ± 0.5% and 0.6 ± 0.9% in the half-sweet wine (Fig. S12b†), respectively. These changes were all less than 4% of that for the original sample, demonstrating that the natively-existing reductants in the examined wines had minor influence on the detection of glucose. We also tested higher concentrations of gallic acid (2 times), sodium sulfite (10 times), and ascorbic acid (10 times) and obtained the great change (16.7 ± 3.8%) for gallic acid but minor changes (2.0 ± 0.9% and 2.7 ± 1.3%) for sodium sulfite and ascorbic acid in sweet wine, respectively, as well as the changes of 17.6 ± 3.8%, 3.8 ± 1.6% and 0.8 ± 0.7% in the half-sweet wine, respectively. Therefore, the proposed method should be reliable in the detection of glucose in white grape wine.
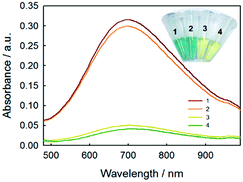 |
| Fig. 7 UV-vis spectra and digital image (inset) of two kinds of white grape wine: sweet wine (1), half sweet wine (2), sweet wine control (3), half sweet wine control (4). Reaction conditions: 0.75 mM FeCl3, 0.75 mM K3Fe(CN)6 and 0.05 mg mL−1 GOx in 50 mM MES (pH 3.0) at 25 °C, reaction for 10 min. No GOx for control sample. | |
In order to validate the method developed in this study, the white grape wine samples were also analyzed by HPLC method, which was a national standard method of China (please see the Experimental part for details). As shown in Fig. 8, the calibration curve of glucose based on the HPLC method was first made showing regression coefficient of 0.9997. The comparative results were shown in Table 2. The relative standard deviations (RSD) of samples were less than 2%, illustrating that the proposed method could be applied to the detection of glucose in food samples. These results confirmed the accuracy of the sensor for measuring glucose in the food samples.
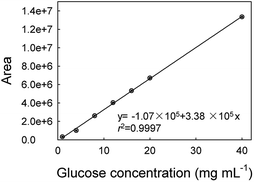 |
| Fig. 8 Calibration curve of glucose measurement using a HPLC method. | |
Table 2 Comparison of the glucose contents in white grape wine by HPLC method and the colorimetric method developed in this study (n = 3)
White grape wine |
HPLC method |
Colorimetric method (this study) |
Mean ± SDa (mM) |
RSD (%) |
Mean ± SD (mM) |
RSD (%) |
SD: standard deviation. |
Half sweet |
28.58 ± 0.07 |
0.25 |
29.51 ± 0.13 |
0.44 |
Sweet |
31.28 ± 0.07 |
0.21 |
32.11 ± 0.38 |
1.18 |
Conclusions
A simple, rapid and sensitive colorimetric biosensing method has been developed for the detection of glucose based on the use of GOx-catalysis-induced PBNPs as nano-reporter. Two paths for the PBNPs formation upon GOx catalysis were identified and evaluated, which enhanced PBNPs yield and the detection performance. Between the two paths, the PBNPs was mainly produced in absence of O2. After the optimization, this reaction system responded linearly to glucose in a wide analyte concentration range within 10 min. The limit of detection was down to 3.29 μM, which was comparable to or better than analogues. Furthermore, glucose could be quasi-quantified by naked eyes based on the color change. This method also provided good sensitivity for detecting glucose without resort to advanced or complex readout instruments. The method was successfully applied to monitoring glucose in the white grape wine. The proposed biosensing method showed significant advantages of rapidity, simplicity, low-cost and high sensitivity and selectivity, therefore, it is promising for use in daily detection of glucose in food processing plants and markets, restaurants and households.
Conflicts of interest
There are no conflicts to declare.
Acknowledgements
This research was supported in part by the National Natural Science Foundation of China (No. 21775137).
Notes and references
- J. M. Zoellner, V. E. Hedrick, W. You, Y. Chen, B. M. Davy, K. J. Porter, A. Bailey, H. Lane, R. Alexander and P. A. Estabrooks, International Journal of Behavioral Nutrition and Physical Activity, 2016, 13, 38 CrossRef PubMed.
- C. E. Kearns, L. A. Schmidt and S. A. Glantz, JAMA Intern. Med., 2016, 176, 1680–1685 CrossRef PubMed.
- G. Marrubini, A. Papetti, E. Genorini and A. Ulrici, Food Analytical Methods, 2017, 10, 1556–1567 CrossRef.
- J. J. Gao, T. Hu and W. J. Cai, J. Food Saf. Food Qual., 2015, 6, 3120–3123 Search PubMed.
- M. Chunmei, S. Zhen, C. Changbao, Z. Lili and Z. Shuhua, Food Chem., 2014, 145, 784–788 CrossRef PubMed.
- M. Brokl, O. Hernandez-Hernandez, A. Cristina Soria and M. Luz Sanz, J. Chromatogr. A, 2011, 1218, 7697–7703 CrossRef CAS PubMed.
- Y. Westphal, H. A. Schols, A. G. J. Voragen and H. Gruppen, J. Chromatogr. A, 2010, 1217, 689–695 CrossRef CAS PubMed.
- A. Chen and S. Chatterjee, Chem. Soc. Rev., 2013, 42, 5425–5438 RSC.
- K. E. Toghill and R. G. Compton, Int. J. Electrochem. Sci., 2010, 5, 1246–1301 CAS.
- C. Chen, Q. Xie, D. Yang, H. Xiao, Y. Fu, Y. Tan and S. Yao, RSC Adv., 2013, 3, 4473–4491 RSC.
- S. A. Zaidi and J. H. Shin, Talanta, 2016, 149, 30–42 CrossRef CAS PubMed.
- K. Wu, X. Zhao, M. Chen, H. Zhang, Z. Liu, X. Zhang, X. Zhu and Q. Liu, New J. Chem., 2018, 42, 9578–9587 RSC.
- Y. Gao, K. Wu, H. Li, W. Chen, M. Fu, K. Yue, X. Zhu and Q. Liu, Sens. Actuators, B, 2018, 273, 1635–1639 CrossRef CAS.
- H. Liu, Y. Ding, B. Yang, Z. Liu, Q. Liu and X. Zhang, Sens. Actuators, B, 2018, 271, 336–345 CrossRef CAS.
- W. Zhao, M. A. Brook and Y. F. Li, ChemBioChem, 2008, 9, 2363–2371 CrossRef CAS PubMed.
- Z. Wang and L. Ma, Coord. Chem. Rev., 2009, 253, 1607–1618 CrossRef CAS.
- R. S. Casey, Ind. Eng. Chem., 1940, 32, 1584–1587 CrossRef CAS.
- A. Kraft, Nachr. Chem., 2010, 58, 1124–1127 CrossRef CAS.
- V. Hornok and I. Dekany, J. Colloid Interface Sci., 2007, 309, 176–182 CrossRef CAS PubMed.
- M. Pyrasch and B. Tieke, Langmuir, 2001, 17, 7706–7709 CrossRef CAS.
- T. Margraf, A. R. Karnopp, N. D. Rosso and D. Granato, J. Food Sci., 2015, 80, C2397–C2403 CrossRef CAS PubMed.
- H. Zhang, J. Li, K. Wang, X. Du and Q. Li, Anal. Biochem., 2009, 388, 40–46 CrossRef CAS PubMed.
- H. Hsu, L. Chen and K. C. Ho, Anal. Chim. Acta, 2004, 504, 141–147 CrossRef CAS.
- B. Zargar and A. Hatamie, Anal. Methods, 2014, 6, 5951–5956 RSC.
- S. S. T. Hua, B. J. Hernlem, W. Yokoyama and S. B. L. Sarreal, World J. Microbiol. Biotechnol., 2015, 31, 729–734 CrossRef CAS PubMed.
- J. D. Qiu, H. Z. Peng, R. P. Liang, J. Li and X. H. Xia, Langmuir, 2007, 23, 2133–2137 CrossRef CAS PubMed.
- T. Uemura, M. Ohba and S. Kitagawa, Inorg. Chem., 2004, 43, 7339–7345 CrossRef CAS PubMed.
- J. Qiu, H. Peng, R. Liang, J. Li and X. Xia, Langmuir, 2007, 23, 2133–2137 CrossRef CAS PubMed.
- K. Itaya, I. Uchida and V. D. Neff, Acc. Chem. Res., 1986, 19, 162–168 CrossRef CAS.
- S. Liu, J. Xu and H. Chen, Electrochem. Commun., 2002, 4, 421–425 CrossRef CAS.
- A. Heller and B. Feldman, Chem. Rev., 2008, 108, 2482–2505 CrossRef CAS PubMed.
- S. Chen, R. Yuan, Y. Chai and F. Hu, Microchim. Acta, 2013, 180, 15–32 CrossRef CAS.
- E. G. Garrido-Ramirez, B. K. G. Theng and M. L. Mora, Appl. Clay Sci., 2010, 47, 182–192 CrossRef CAS.
- P. Xu, G. M. Zeng, D. L. Huang, C. L. Feng, S. Hu, M. H. Zhao, C. Lai, Z. Wei, C. Huang, G. X. Xie and Z. F. Liu, Sci. Total Environ., 2012, 424, 1–10 CrossRef CAS PubMed.
- E. Wilhelm, R. Battino and R. J. Wilcock, Chem. Rev., 1977, 77, 219–262 CrossRef CAS.
- Y. Fu, Z. Callaway, J. Lum, R. Wang, J. Lin and Y. Li, Anal. Chem., 2014, 86, 1965–1971 CrossRef CAS PubMed.
- P. He, G. Greenway and S. J. Haswell, Microfluid. Nanofluid., 2010, 8, 565–573 CrossRef CAS.
- C. Wang, D. K. Ye, Y. Y. Wang, T. Lu and X. H. Xia, Lab Chip, 2013, 13, 1546–1553 RSC.
- Q. Li, G. Tang, X. Xiong, Y. Cao, L. Chen, F. Xu and H. Tan, Sens. Actuators, B, 2015, 215, 86–92 CrossRef CAS.
- Y. Song, K. Qu, C. Zhao, J. Ren and X. Qu, Adv. Mater., 2010, 22, 2206–2210 CrossRef CAS PubMed.
- A. Dalui, B. Pradhan, U. Thupakula, A. H. Khan, G. S. Kumar, T. Ghosh, B. Satpati and S. Acharya, Nanoscale, 2015, 7, 9062–9074 RSC.
- F. Qiao, Q. Qi, Z. Wang, K. Xu and S. Ai, Sens. Actuators, B, 2016, 229, 379–386 CrossRef CAS.
- M. Yang, H. Zhou, Y. Li, Q. Zhang, J. Li, C. Zhang, C. Zhou and C. Yu, J. Mater. Chem. B, 2017, 5, 6572–6578 RSC.
- Q. Liu, Y. Yang, H. Li, R. Zhu, Q. Shao, S. Yang and J. Xu, Biosens. Bioelectron., 2015, 64, 147–153 CrossRef CAS PubMed.
- X. Peng, G. Wan, L. Wu, M. Zeng, S. Lin and G. Wang, Sens.
Actuators, B, 2018, 257, 166–177 CrossRef CAS.
- W. Zhang, D. Ma and J. Du, Talanta, 2014, 120, 362–367 CrossRef CAS PubMed.
- Y. Liu, C. J. Walkey, T. J. Green, H. J. J. van Vuuren and D. D. Kitts, Food Chem., 2016, 194, 26–31 CrossRef CAS PubMed.
- J. Ballester, M. Magne, P. Julien, L. Noret, M. Nikolantonaki, C. Coelho and R. D. Gougeon, Beverages, 2018, 4, 19 CrossRef.
- M. P. Bradshaw, C. Barril, A. C. Clark, P. D. Prenzler and G. R. Scollary, Crit. Rev. Food Sci. Nutr., 2011, 51, 479–498 CrossRef CAS PubMed.
Footnote |
† Electronic supplementary information (ESI) available. See DOI: 10.1039/c8ra06347h |
|
This journal is © The Royal Society of Chemistry 2018 |