DOI:
10.1039/C8AN01219A
(Paper)
Analyst, 2019,
144, 220-229
Merging metabolomics and lipidomics into one analytical run†
Received
3rd July 2018
, Accepted 12th October 2018
First published on 9th November 2018
Abstract
A novel integrated metabolomics/lipidomics workflow is introduced enabling high coverage of polar metabolites and non-polar lipids within one analytical run. Dual HILIC and RP chromatography were combined to high-resolution mass spectrometry. As a major advantage, only one data file per sample was obtained by fully automated simultaneous analysis of two extracts per sample. Hence, the unprecedented high coverage without compromise on analytical throughput was not only obtained by the orthogonality of the chromatographic separations, but also by the implementation of dedicated sample preparation procedures resulting in optimum extraction efficiency for both sub-omes. Thus, the method addressed completely hydrophilic sugars and organic acids next to water-insoluble triglycerides. As for the timing of the dual chromatography setup, HILIC and RP separation were performed consecutively. However, re-equilibration of the HILIC column during elution of RP compounds and vice versa reduced the overall analysis time by one third to 32 min. Application to the Standard Reference Material SRM 1950 – Metabolites in Frozen Human Plasma resulted in >100 metabolite and >380 lipid identifications based on accurate mass implementing fast polarity switching and acquiring data dependent MS2 spectra with the use of automated exclusion lists. Targeted quantification based on external calibrations and 13C labeled yeast internal standards was successfully accomplished for 59 metabolites. Moreover, the potential for lipid quantification was shown integrating non-endogenous lipids as internal standards. In human plasma, concentrations ranging over 4 orders of magnitude (low nM to high μM) were assessed.
Introduction
Global scale metabolomics lags behind other omics disciplines.1 To date, the major caveats remaining in non-targeted metabolomics are the conflicting goals of analytical throughput and metabolite coverage.2 The chemical and physical diversity of the metabolome is huge as exemplified by partition coefficient values ranging over 40 orders of magnitude.3 Completely water soluble sugars are an integral part of the metabolome as well as completely water insoluble lipids such as triglycerides. This diversity ultimately led to the “divide and conquer” strategy of developing sub-ome approaches such as metabolomics and lipidomics. In fact, lipidomics workflows differ significantly from metabolomic workflows.4–8 This holds true for all steps of the analytical process from sampling, sample preparation, measurement, data evaluation and data reporting (peak picking, standardization of annotation, nomenclature). Although lipids constitute a major fraction of the metabolome (i.e. more than 60% of all metabolites within the human metabolome database are annotated lipids9), best practice guidelines for global large scale metabolome studies focus on extraction and separation procedures dedicated to polar metabolites. Even when omitting lipid coverage, application of multiple analytical runs utilizing different chromatographic separations and ionization methods is recommended in order to achieve comprehensive analysis of the metabolite sub-ome.10 On top of that, there is increasing unease that the chemical space one is looking at in a single liquid chromatography high-resolution mass spectrometry (LC-HRMS) based analytical run is much smaller than could be simply inferred by the number of features detected.11 There are current estimations that a fingerprint obtained by a typical ultra-high performance liquid chromatography (UHPLC) HRMS method of 15–20 min results from only several hundreds of molecules, the limitations originating from ionization and retention properties of the molecules present in the sample together with the limits imposed by the dynamic range of electrospray ionization (ESI). Ionization of less abundant molecules in the presence of highly abundant molecules (suppression effects) becomes a limiting factor when peak capacity and separation selectivity are limited. As a consequence, despite the fact that several thousands of features are detected, after thorough corroboration of the data, the number of molecules accessible by a single LC-HRMS run is only in the hundreds.11
Thus, increasing chromatographic peak capacity and separation selectivity which in turn improve the selectivity and dynamic range of MS detection is crucial when aiming at comprehensiveness.2,12 This holds true even when considering the sub-omes separately. Hydrophilic interaction liquid chromatography (HILIC) separation using alkaline conditions revealed to have a high coverage for primary metabolites such as amino acids, organic acids, nucleotides, and sugar phosphates.13 However, two-dimensional orthogonal separations combining HILIC with reversed phase (RP) chromatography remain a technological challenge14 due to solvent incompatibilities and dilution effects. Several studies proposed on-line combination of orthogonal separations either using parallel separations,15 single heart-cut16 or multiple heart cut LC separations.17–19 2D-LC has proved to be a powerful approach in lipidomics.20–23 Rampler et al. recently introduced an on-line combination of HILIC and reversed phase chromatography for increased coverage of hydrophilic and hydrophobic lipids.24 However, the majority of in-depth lipid profiling is carried out by applying reversed phase chromatography high-resolution mass spectrometry (HRMS) only using run times of 1–2 hours.4,6 To date, comprehensive two-dimensional separations are not widely spread in both fields.25,26 A successful alternative analytical strategy addressed multiple-tailored derivatization methods targeting different chemical moieties thereby covering the whole metabolome while at the same time allowing to resort to a single reversed phase separation.27–29 However, in these studies, lipids are largely omitted.
Despite these technological challenges of slow throughput, metabolomics and lipidomics became major driving forces in biomedical research over recent years.10 Many health disorders and diseases including cancer and neurodegenerative diseases are caused (or accompanied) by altered metabolism.30 The objectives are to uncover pathways underlying the disease, contributing to better classification and therapeutic strategies. The hypothesis is that metabolomics enables identification of fingerprints for early diagnosis, monitoring disease progression and ultimately resulting in a therapeutic strategy. Not surprisingly, comprehensiveness of fingerprint analysis is crucial in biomarker discovery.10 It becomes increasingly clear that it is pivotal to merge metabolomics and lipidomics strategies in these types of studies.30,31 Major efforts concerned the elaboration of protocols suitable for simultaneous metabolite and lipid extractions.32 However, to date only a few methods aiming at simultaneous analysis of the different fractions resulting from biphasic extraction were introduced.33 To the best of our knowledge, the simultaneous analysis of the metabolome and lipidome was first described in 2015 by the use of solid phase extraction followed by two RP separations.34 Wang et al.35 performed simultaneous analysis of lipids and metabolites in one analytical run by a fully automated novel 2D heart-cutting approach. However, the sample preparation did not consider the full coverage of both -omes. In this work, we developed the idea of parallel separations15 further and introduce a novel workflow which allows simultaneously analyzing two separate metabolite and lipid extracts. Fully automated parallel HILIC and RP-LC enabled merging metabolomics and lipidomics data into one analytical run, i.e. one data file. Although we focus on human plasma in this work, other application areas of metabolomics research such as e.g. plant science, microbiology, oceanography and geomicrobiology could benefit from this setup. For the sake of clarity, in the following the term metabolites/metabolomics is restricted to the sub-ome of small polar compounds omitting lipids.
Experimental
Standards and solvents
Acetonitrile (ACN), isopropanol (IPA), methanol (MeOH) and water were of LC-MS grade and ordered at Fisher Scientific (Vienna, Austria) or Sigma Aldrich (Vienna, Austria). Ammonium bicarbonate, ammonium formate and ammonium hydroxide were ordered as the eluent additive for LC-MS at Sigma Aldrich. Formic acid was also of LC-MS grade and ordered at VWR International (Vienna, Austria).
Metabolite standards were purchased from Sigma Aldrich (Vienna, Austria) or Carbosynth (Berkshire, UK). Lipid standards were purchased from Avanti Polar Lipids, Inc. (Alabama, USA) and Sigma Aldrich. All standards were weighed and dissolved in an appropriate solvent. A multi-metabolite mix (containing a fully 13C labeled internal standard from ISOtopic solutions e.U. (Vienna, Austria) reconstituted in 2 mL water to a final dilution of 1
:
10 (v/v)) and a multi-lipid mix were prepared. Dilutions between 0.01 μM and 25 μM were prepared in 50% ACN and starting conditions of reversed phase separation (30% B), respectively. SPLASH® Lipidomix® Mass Spec Standard was purchased from Avanti Polar Lipids, Inc. (Alabama, USA).
Standard reference material 1950
The standard reference material (SRM) 1950 Metabolites in Frozen Human Plasma was purchased at the National Institute of Standards & Technology (NIST) (Gaithersburg, USA). SRM 1950 contains human plasma from 100 individuals and constitutes a human plasma sample with certified concentration values for cholesterol, total glycerides, selected fatty acids, amino acids, vitamins, carotenoids, clinical markers, hormones, and electrolytes.36 Recently, also consensus values for 339 lipids obtained from an interlaboratory comparison were published.37 This study did not involve samples or procedures requiring informed consent of approval by an institutional review board.
MeOH extraction of SRM 1950.
The procedure was adapted from the study by Simón-Manso et al. (2013).38 SRM 1950 was thawed at room temperature. 50 μL of SRM 1950 were mixed with 50 μL yeast ISTD (extract out of 2 billion cells reconstituted in 2 mL water, n = 4) or 50 μL water (n = 4). 400 μL methanol (MeOH) were added to reach a final volume of 500 μL (80% MeOH, v/v), which resulted in a 1
:
10 dilution of SRM 1950 and yeast internal standard (ISTD). After thorough vortexing, it was kept on ice for 30 min. Then it was vortexed again and kept at −20 °C overnight. The samples were centrifuged (14
000g, 4 °C, 15 min) and two 200 μL aliquots were dried in a vacuum centrifuge. Afterwards, the samples were reconstituted in 200 μL 50% H2O/50% ACN (v/v), vigorously vortexed for 3 minutes to ensure complete dissolving of the sample and centrifuged (14
000g, 4 °C, 10 min) prior to being transferred to an HPLC vial.
MTBE extraction of SRM 1950.
The extraction of human plasma using methyl-tert-butyl ether (MTBE) was adapted from the Matyash39 protocol. Methanol (0.375 mL) was added to a 50 μL SRM 1950 plasma sample aliquot in a glass tube with a Teflon-lined cap. After vortexing, 1.25 mL MTBE was added and the mixture was incubated for 1 h at room temperature in a shaker. Phase separation was induced by adding 0.313 mL of MS-grade water. Upon 10 min of incubation at room temperature, the sample was centrifuged at 400 rpm (∼30g) for 5 min. The upper (organic) phase was collected, and the lower phase was re-extracted with 0.5 mL of the solvent mixture, whose composition was equivalent to the expected composition of the upper phase [obtained by mixing MTBE/methanol/water (10
:
3
:
2.5, v/v/v) and collecting the upper phase]. Combined organic phases were dried in a vacuum centrifuge and stored at −20 °C. Extracted lipids were dissolved in 180 μL of LC-MS starting buffer (30% B). 20 μL SPLASH Lipidomix was added prior to centrifugation (30g for 10 min) and transferred to an HPLC vial.
Hydrophilic interaction liquid chromatography (HILIC) for metabolites
A SeQuant® ZIC®-pHILIC column (150 × 2.1 mm, 5 μm, polymer, Merck-Millipore) was used with gradient elution under alkaline conditions. Mobile phase A was 90% 10 mM ammonium bicarbonate, pH 9.2/10% acetonitrile and mobile phase B 100% acetonitrile. The following gradient was used at a flow rate of 0.300 mL min−1 and 40 °C: 0.0–6.0 min 75%–45% B, 6.0–7.0 min decrease to 30% B, 7.0–10.0 min 30% B and at 10.0 min switch to 75% B. From 15 to 27 min, the flow rate was reduced to 0.050 mL min−1 in order to save solvents during RP elution. Five minutes prior to the next HILIC injection, the flow rate was increased to 0.300 mL min−1 to ensure stable column conditions. The injection volume was 5 μL and the injector needle was washed with ACN
:
MeOH
:
H2O 1
:
1
:
1 (v/v/v) for 5 s prior to each injection.
Reversed-phase (RP) chromatography for lipids
For RP chromatography of lipids, an Acquity HSS T3 (2.1 mm × 150 mm, 1.8 μm, Waters) with a VanGuard Pre-column (2.1 mm × 5 mm, 100 Å, 1.8 μm) was used. The flow rate was set to 0.250 mL min−1 and the column temperature to 40 °C. Solvent A was ACN/H2O (3
:
2, v/v), and solvent B was IPA/ACN (9
:
1, v/v). Both solvents contained 0.1% formic acid and 10 mM ammonium formate. The following gradient was used: 0–12.0 min 30% B, 12.0–25.0 min ramp to 75% B, 25.0–27.0 ramp to 100% B, 27.0–32.0 100% B, 32.0 min fast switch to 30% B. Equilibration (at least 5 min required) was continued during the following HILIC injection. The injection volume was 5 μL. The injector needle was washed with 75% IPA, 25% H2O, and 0.1% formic acid for 5 s prior to each injection.
Setup for HILIC-RP liquid chromatography
A Vanquish Duo UHPLC system (Thermo Fisher Scientific) equipped with an autosampler with two injection units, two binary pumps and a column compartment was used to enable parallel HILIC and RP analyses. As can be observed in Fig. 1, 2 post-column 2-position 6-port valves were installed, respectively, followed by a T-piece prior to the introduction into the ESI source of the mass spectrometer. This way, the two orthogonal separations could be performed consecutively, carrying only one (either HILIC or RP) eluent to the MS, while the other separation eluent was directed to the waste. The temperature of the autosampler was set to 10 °C. The injection routine (drawing the sample and washing the needle) started with parallel preparation of both samples, i.e. the metabolite extract and the lipid extract, so that both samples were in their corresponding autosampler needle. The analytical run was initialized upon injection of the former sample onto the HILIC column, while the injection of the lipid sample was delayed by 10 min corresponding to the run time of HILIC separation. The second injection on the RP column followed after 10 min and was synchronized to the switching of the two post column 6-port valves at 11 min. The RP separation was carried out, while the HILIC column was re-equilibrated. Overall, this resulted in a total run time of 32 min compared to 47 min if the two methods were run separately, thereby saving roughly one third of analysis time.
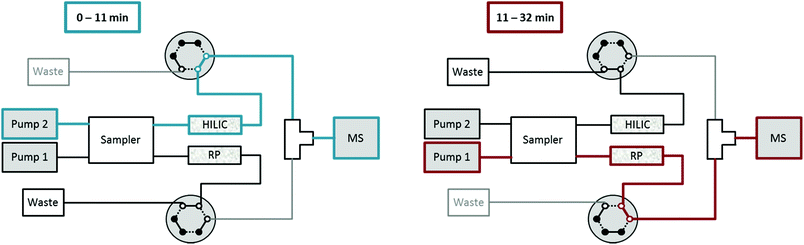 |
| Fig. 1 Dual setup for HILIC-RP MS with two pumps, an autosampler with two independent flow-paths and two 2-position-6-port valves. From 0–11 min HILIC elution of metabolites is carried out and directed into the MS, followed by RP elution of lipids which are injected after 10 min. During column re-equilibration of each column, the flow is directed to a waste bottle allowing for stable conditions during elution of the other column. | |
High-resolution mass spectrometry (HRMS)
High-resolution mass spectrometry was conducted on a high field Thermo Scientific™ Q Exactive HF™ quadrupole-Orbitrap mass spectrometer equipped with an electrospray source. The ESI source parameters were the following: sheath gas 40, auxiliary gas 3, spray voltage 2.8 kV in negative and 3.5 kV in the positive mode, capillary temperature 280 °C, S-Lens RF level 30 and auxiliary gas heater 320 °C. Spectral data were acquired in the profile mode. From 0–11 min full mass scan data in the range of 65–900 m/z were acquired followed by 200–2000 m/z from 11–32 min for lipid analysis. For full MS runs, positive and negative mode data were acquired in the polarity switching mode with a resolution of 120
000. The automatic gain control (AGC) target was set to 1e6 and the maximum injection time (IT) was 200 ms. For identification, data-dependent MS2 (ddMS2) fragmentation spectra were acquired in positive and negative modes separately with a resolution of 30
000 for MS2. A Top15 method was used from 0–11 min for metabolites, and Top10 was used for lipids. The AGC target was 2e5 and the maximum injection time was 60 ms. The ions were isolated with 1 m/z and fragmented with normalized collision energies of NCE 30 for HILIC metabolites and 28 for RP lipids. The dynamic exclusion of triggered m/z was set to 15 s. Iterative exclusion lists were generated using the IE-Omics tool.40
Data analysis
Targeted data evaluation was performed in Tracefinder for metabolites and lipids together. 13C internal standardization using external calibration was employed for metabolites. All calibration curves were linear and weighted 1/x. Limits of detection (LODs) were calculated according to EURACHEM, The Fitness for Purpose of Analytical Methods, 2nd edition (2014) by repetitive injections of a low concentrated standard.41 For untargeted data processing of metabolites, Thermo Scientific Compound Discoverer software was used as described in a previous publication.42 For untargeted data analysis of lipids, LipidSearch from Thermo Scientific was used. Details on the identification are given in the ESI.†
Results and discussion
Extraction of human plasma for metabolomics and lipidomics
Accurate quantification of metabolites and lipids is intrinsically dependent on the design of the extraction procedure. Well-established extractions in metabolomics are based on solvents such as methanol, ethanol or acetonitrile. This has been thoroughly validated for the sample matrix of human plasma in several studies.43,44 The extraction of lipids is typically performed with biphasic extraction using chloroform–methanol mixtures (Folch,45 Bligh & Dyer46) or more recently using methyl-tert-butyl ether (MTBE).39 The latter approach was adapted from a multi-omics procedure (denoted as the SIMPLEX protocol which enabled metabolomics, lipidomics and proteomics from the same cell sample). A detailed description and validation can be found elsewhere.32 Metabolites are recovered from the lower aqueous phase following removal of the MTBE fraction containing lipids and methanol addition. This latter step is required for completeness of protein precipitation. Accurate metabolite quantification is enabled provided that the complete volume of the metabolite extract is recovered and treated further for analysis as the accurate volume of the metabolite extraction solvent cannot be assessed. If this is accomplished properly, the method is equivalently suitable for metabolite analysis in human plasma as methanol/ethanol based extraction protocols with the advantage of reducing sample volume. This is exemplified by absolute quantification of amino acids in the certified reference material SRM1950 comparing both extraction procedures (see ESI Fig. S1†). Both MeOH extraction and biphasic MTBE extraction revealed similar peak areas and quantitative values (obtained by isotope dilution) in good agreement with the certified values. In the presented study, a low sample volume was not a requirement. Thus in the following, two separate extraction procedures were performed on two aliquots of human plasma, namely methanol extraction and MTBE extraction. After centrifugation and drying, the metabolite extract was directly analyzed, thereby reducing sample handling to a minimum.43,47
Metabolomics using HILIC
Metabolome analysis was addressed by hydrophilic interaction chromatography on a zwitterionic polymer-based stationary phase. Using alkaline conditions, the separation covered a metabolite panel comparable to the well-established ion pairing chromatography.48 Merging metabolomics/lipidomics workflows required switching from the negative ionization to positive ionization mode,49 which is impeded when using ion pairing reagents. Hence, the key advantage of not using ion pairing reagents predominated the fact that HILIC analysis was compromised regarding e.g. histidine and biogenic polyamines, most likely due to their multiple amine groups. Histidine eluted as an extremely broad peak of more than eight minutes, while spermine and spermidine could not be detected. With respect to anion chromatography,42 the metabolite panel could be extended to amino acids and other positively charged metabolites. In this work, HILIC was adapted to short gradient times and tested for a standard panel of 133 metabolites (see Table S1†). The target metabolites included organic acids, amino acids, sugar phosphates, nucleobases, nucleosides, nucleotides and coenzymes. As can be observed in Table S2,† several critical isomers of the primary metabolome were separated even when using a separation time of 11 min. Only leucine and isoleucine and the pentose-phosphates (ribose-5-phosphate and ribulose-5-phosphate) were not separated at all. Regarding the hexose-phosphates, fructose-6-phosphate and glucose-6-phosphate were baseline separated, while fructose-6-phosphate and glucose-1-phosphate co-eluted. For hexoses, at least an elution order (fructose-mannose-galactose-glucose) could be assessed. Limits of detection were assessed according to the Eurachem guidelines “The Fitness for Purpose of Analytical Methods, 2nd edition (2014)”41 using replicate measurements of a low concentrated standard containing a fully 13C labeled yeast extract as the matrix mimic (see Table S1†). Detection limits for organic acids were between 10 and 50 nM, for nucleotides and amino acids between 10 and 100 nM. Overall, the limits of detection were comparable to those of a previously published ion-exchange chromatography method.42 Moreover, excellent recoveries were assessed for 1 μM quality control (QC) standards (containing the 13C labeled yeast extract) representing the trueness bias of quantification by external and internal standards. A significant fraction of the studied metabolites could be determined using both ionization modes, while organic acids, sugars and sugar phosphates required negative ionization and a minor fraction of intrinsically positively charged metabolites (nicotinamide, carnitine, and propionyl-carnitine) could only be measured in the positive mode. Although slightly counterintuitive, alkaline conditions did not impede positive electrospray ionization, a fact that has also been previously reported.13,50 In the following, all data were acquired using the fast polarity switching feature of the orbitrap-MS with the aim of increased coverage. Finally, the HILIC retention of polar lipid classes potentially present in methanol extracts of plasma samples, i.e. phospholipids,43 which might cause ion suppression, was addressed. As can be observed in the ESI (Fig. S2†), all lipids eluted near the void volume (0.7–1.1 min). Among the target metabolites, only 3 compounds showed such poor retention and eluted in the void volume, namely 3-methyl-2-oxovaleric acid, dihydroxyisovalerate and ketoisovalerate. Despite this fact, their quantification in plasma samples was feasible due to 13C labeled internal standards. Compared to a previously published dual setup that used RP chromatography for both metabolites and lipids,35 improved retention and separation of polar metabolites including their isomers could be achieved by the HILIC method.
Lipid analysis using RP-LC
For lipid analysis, reversed phase chromatography separating lipids4,7 according to their acyl chain length and degree of unsaturation was integrated using a sub 2 μm stationary phase. Again, a standard panel covering all lipid classes, i.e. monoglycerides (MG), diglycerides (DG), triglycerides (TG), ceramides (Cer), hexosyl ceramides (HexCer), dihexosyl ceramides (Hex2Cer), cholesteryl esters (CE), phosphatidic acids (PA), phosphatidylcholines (PC), phosphatidylethanolamines (PE), phosphatidylglycerols (PG), phosphatidylserines (PS), lysophosphatidylcholines (LPC), lysophosphatidylethanolamines (LPE), fatty acids (FA), sphingomyelins (SM), and acyl carnitines (AcCa) (see Table S3†) served for establishing the chromatographic separation and assessing limits of detection.41Fig. 2 shows the retention order obtained by the implemented reversed phase gradient duration of 22 min (starting the data acquisition at 1 min): lysophospholipids (LPL including LPC and LPE), MG and AcCa were followed by FA, glycerophospholipids (GPL including PA, PC, PE, PG, PS), sphingolipids (SPL including Cer, HexCer, Hex2Cer, SM) and finally DGs, TGs and CEs eluted. Excellent limits of detection in the nM range were observed. Only for CEs poor ionization and for MGs and TGs elevated backgrounds led to LODs in the low μM range. In addition, the intermediate repeatability and recovery of 1 μM quality control standards were assessed by external calibration proving the suitability for quantification purposes (N = 12 replicate measurements over >45 hours). The short reversed phase gradient time of 22 min resulted in 250 lipid identifications (based on full MS peak detection, MS/MS spectral annotation), which is a typical value when analyzing human plasma samples. UHPLC peak widths typically ranged at 3–4 s (FWHM). Hence, the worst case scenario observed was for DGs and TGs where only 7 data points could be collected over the chromatographic peak. All lipids that were identified in the separately performed ddMS2 runs (positive and negative, respectively) could be recovered in the Full MS files acquired in the polarity switching mode showing that increasing the throughput by polarity switching is a valid approach. Overall, the identification numbers compared well with other established lipid profiling methods based on such short analysis time.4,7
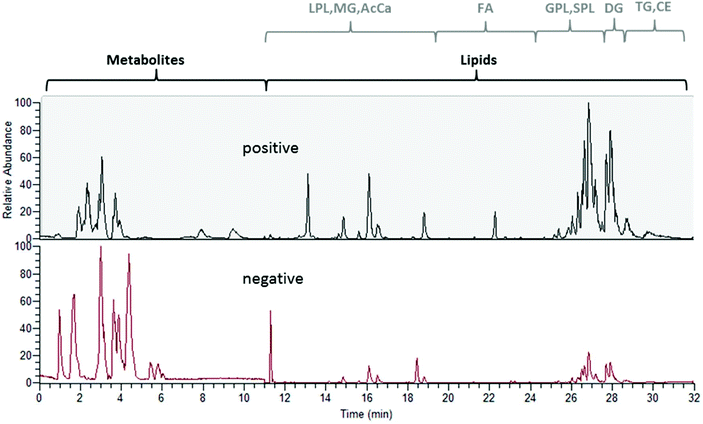 |
| Fig. 2 Base peak chromatograms of SRM 1950 measured with HILIC and RP chromatography with polarity switching. The upper chromatogram shows the positive mode, the lower one negative. The first 11 min comprise small molecules from HILIC separation followed by lipids from 11–32 min from RP separation. LPL (lysophospholipids), MG (monoglycerides), AcCa (acyl carnitines), FA (fatty acids), GPL (glycerophospholipids) SPL (sphingolipids), DG (diglycerides), TG (triglycerides), and CE (cholesteryl esters). | |
Combining metabolomics and lipidomics: two extracts on two columns in one run
The experimental set-up depicted in Fig. 1 enabled us to perform metabolomics and lipidomics in parallel. This way, not only chromatographic separation, but also sample preparation could be tailored to the respective sub-omes. The overall run time of the merged analysis was 32 min. In Fig. 2, the base peak chromatograms for positive and negative electrospray ionization acquired with polarity switching of a human plasma extract are shown. Initially, 11 min of analysis time were dedicated to HILIC separation and re-equilibration of RP separation, which was followed by 21 min of RP separation and simultaneous HILIC re-equilibration. The dual injection was timed according to the sequential separations as previously described. Remarkably, two chromatographic separations utilizing alkaline and acidic eluents, respectively, could be easily combined due to two additional post-column valves without compromising the analytical figures of merit. Implementing the fast polarity switching capability of the QE-HF MS, the total analysis time otherwise required for a measurement series could be reduced by one half nonetheless obtaining between 7 and 25 data points (R = 120
000) across the peak. Table 1 surveys retention time stability, intermediate repeatability and % of recovered target compounds in a QC sample and the SRM 1950 sample. Out of the investigated panel, only a small fraction could not be assessed by the designed approach. Some metabolites were poorly ionized under the selected conditions (see Table S1† for details). Excellent intermediate repeatabilities of both the peak area and retention time were obtained by the dual setup, considering replicate measurements of a 1 μM QC sample spiked with the 13C labeled yeast extract and of a pooled plasma extract from SRM 1950 over >45 hours.
Table 1 Total number of compounds and relative standard deviations (RSDs) of areas of metabolites separated by HILIC and lipids separated by RP over a time of >45 hours (N = 12 for QC and N = 8 for SRM 1950) with the dual setup. The values are representing the number of compounds below a certain RSD of the peak area over 45 h. Slightly more metabolites were detected in the negative ionization mode whereas the positive mode is preferable for lipids. Excellent RSDs were observed despite the alternation of pH 9.2 (HILIC) and ammonium formate/formic acid (RP). Numbers for SRM 1950 refer to the available standard compounds that were also found in human plasma
RSD [%] |
Metabolites (133 standards) |
Lipids (52 standards) |
QC 1 μM |
SRM 1950 |
QC 1 μM |
SRM 1950 |
Pos |
Neg |
Pos |
Neg |
Pos |
Neg |
Pos |
Neg |
Peak area
|
Number of compounds
|
≤5% |
17 |
32 |
12 |
18 |
12 |
10 |
9 |
7 |
≤10% |
37 |
63 |
24 |
35 |
30 |
16 |
17 |
12 |
≤15% |
52 |
86 |
29 |
44 |
33 |
21 |
19 |
13 |
≤20% |
64 |
92 |
31 |
46 |
37 |
22 |
20 |
14 |
≤30% |
81 |
104 |
33 |
50 |
37 |
22 |
23 |
15 |
≤50% |
91 |
110 |
33 |
50 |
38 |
22 |
26 |
15 |
|
Retention time
|
Number of compounds
|
≤0.1% |
2 |
7 |
1 |
5 |
33 |
14 |
27 |
11 |
≤0.2% |
8 |
10 |
4 |
11 |
37 |
18 |
29 |
14 |
≤0.5% |
59 |
78 |
27 |
35 |
38 |
22 |
29 |
15 |
≤1% |
85 |
107 |
32 |
46 |
38 |
22 |
29 |
15 |
≤2% |
92 |
111 |
32 |
49 |
38 |
22 |
29 |
15 |
|
Percentage of recovered target compounds
|
69% |
83% |
25% |
37% |
73% |
42% |
56% |
29% |
While in metabolomics the two essential tasks targeted quantification and non-targeted analysis are carried out by separate approaches (even using different platforms), these boundaries are somewhat blurry in lipid analysis. There are well accepted strategies based on HRMS merging lipid identification and quantification into one analytical run.4 Lipid class-specific internal standardization with non-endogenous lipids enabled quantification of up to several hundred lipids by LC-MS4,7 as well as shotgun MS.51,52 As data mining in such merged workflows is highly challenging, currently the resurgence of wide targeted lipid analysis can be observed.37,53 In metabolomics, only a few studies implement high resolution MS for merging absolute quantification and fingerprinting. A recent IC-HRMS study showed that simultaneous absolute quantification of 45 metabolites and non-targeted analysis was successfully performed in cancer cells upon integration of yeast derived 13C labeled standards.42 The number of annotated metabolites was not significantly influenced by the presence of an internal standard. Following the analogous strategy in this work, the metabolome extraction procedures comprised the addition of the yeast derived 13C internal standard with the aim of performing both simultaneously, absolute quantification of >50 primary metabolites and non-targeted fingerprinting. Thus, the introduced workflow addressed not only integrated metabolomics and lipidomics into one run, but merged quantification and identification for metabolomics as well. The novel method was evaluated using the SRM 1950, which is certified for several metabolites. Moreover, a recent laboratory intercomparison study provided excellent lipid characterization of this material.37 As a consequence, it was the ideal sample to evaluate the proposed novel workflow.
As can be observed in Fig. 3, the determined values were in good agreement with certified metabolite reference values in the plasma sample SRM1950. Besides amino acids, 59 metabolites were quantified based on external calibration with internal standardization (where possible; information in Table S1†). Quantitative values for 59 metabolites are given in the ESI (Fig. S3†) showing that concentrations ranging over 3–4 orders of magnitude can be assessed by the parallel HILIC-RP method. In untargeted data mining >1000 compounds (grouped features based on isotopologue pattern considering C, H, O, N, S, P present in common organic molecules and adducts, considering the first 11 min of analysis) with CVs <30% in the pooled sample measured over 45 hours (N = 8) were detected in the HILIC separation. This proved once more that this setup with switching between acidic and alkaline conditions within one run showed excellent intermediate repeatabilities and thus is suitable for untargeted analysis. This number also compares well with other separations used in untargeted metabolomics. In both positive and negative modes, ddMS2 spectra could be attributed to more than 50% of the features. Based on mzCloud as the MS2 library, >100 compounds were putatively annotated in the human plasma sample. Among amino acids and organic acids, which were already addressed in the targeted analysis, several other compounds of non-endogenous compounds such as gabapentin, caffeine, δ-valerolactam, pipecolic acid, paracetamol, benzoylecgonine, cotinine and ecgonine were detected.
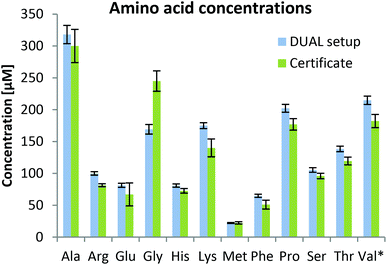 |
| Fig. 3 Calculated amino acid concentrations (error bars correspond to standard deviations of replicate extractions, N = 4) based on internal standardization with the fully 13C labeled yeast extract in human plasma SRM 1950 after MeOH extraction compared to the certificate provided by the National Institute of Standards & Technology (NIST). All amino acids were quantified in the positive mode except *valine, which was quantified in the negative mode because it was not baseline separated from betaine which shows a much higher signal in the positive mode. | |
Finally, lipid profiling enabled by parallel HILIC–RP analysis was addressed. Lipid identification was not impeded by the dual setup. As can be seen in the ESI (Fig. S4†), changing the pH during the analytical run did not affect the number of identified lipids as no difference was observed when using RP separation of 22 min separately. The slight differences arising from replicate analysis by the two methods are arbitrary and can be explained by the complexity of the sample compared to the ddMS2 acquisition speed. Precursor selection is therefore not completely repeatable when analyzing technical replicates. As shown in Fig. 4, the identified lipids are in excellent agreement with the lipids identified in an interlaboratory comparison.37 Considering the concentration range >1 μM, 77%, i.e. 145 out of the 188 lipids reported in the interlaboratory comparison were recovered. At concentrations of 1 and 0.5 μM 63% (31 out of 49) were annotated decreasing to still 36% (25 out of 69) at low concentrations <0.5 μM. Details on lipid identification with LipidSearch are given in the ESI† comparing RP chromatography and the dual setup. Using the SPLASH Lipidomix as the internal standard, we also addressed lipid quantification. As can be observed in the ESI (Fig. S5†), overall, the method could be used for quantification37,53 considering all the limitations imposed by the use of reversed phase chromatography.
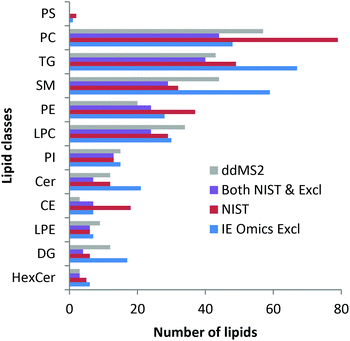 |
| Fig. 4 Lipid identifications in SRM 1950 measured with the dual setup with ddMS2 (grey) and automated exclusion list generation via the IE Omics tool (blue: IE Omics Excl) in comparison with the identifications stated in the NIST interlaboratory comparison (red: NIST).37 Most lipids identified with the presented setup are in very good agreement with the interlaboratory comparison. Lipids found in both the NIST report and by the dual setup with the IE Omics tool are depicted as violet bars. Data were evaluated with LipidSearch considering adducts and both polarities. | |
Increasing the number of putatively annotated compounds
In metabolomics, acquiring data dependent MS2 spectra on a pooled sample for identification purposes has already been established whereas in lipidomics, ddMS2 is used to increase the level of identification of putative lipid isomers and isobars. Recently, a tool that automatically creates exclusion lists for Q Exactive instruments was published (IE Omics).40 It is provided as R script and directly puts all m/z from the first ddMS2 run that were already fragmented on an exclusion list to allow for fragmentation of lower abundant ions. We applied it to our pooled plasma sample, i.e. pool of extraction replicates, and by only two additional ddMS2 runs we were able to significantly increase the number of identifications for both lipids and metabolites. In LipidSearch, 386 instead of 272 lipids (+40%) were identified when two iterative exclusion lists were used showing the power of this approach. Details on lipid identification are given in the ESI† comparing RP chromatography and the dual setup with automated exclusion list generation by the IE Omics tool. Metabolite identification was performed in Compound Discoverer. For both the pooled sample with and without the fully 13C labeled internal standard 20% more metabolites were putatively annotated by MS2 spectra comparison with mzCloud after two iterative exclusion lists. Also in total, for 25% more features MS2 spectra were triggered. The same result was found for both positive and negative ionization. The impact on the number of lipid identifications is supposed to be stronger than for metabolites because the RP peaks are narrower; thus there is less time to perform data dependent fragmentation. The described approach was straight-forward. The benefit of increasing the number of identifications justified the additional measurement time.
Conclusions
Due to the increasing importance of biomarker research leading to the development of high-throughput diagnostic assays, merging metabolomics and lipidomics is key but compromised by the fact that typically the two approaches deliver separate data sets. In this work, hundreds of metabolites and lipids were analyzed simultaneously without compromising the analytical throughput. The method enabled both absolute quantification based on isotopically labeled internal standards and identification of compounds. Such a screening approach has great potential in large scale global studies as only one data file per sample has to be handled. In future, the coverage of the dual approach could be even expanded by elongation of chromatographic separation time and the implementation of complementary fragmentation techniques. As currently very fast improvements regarding data analysis software can be observed, new packages that accommodate both the special requirements for metabolomics as well as lipidomics can be expected to be available soon.
Conflicts of interest
There are no conflicts of interest to declare.
Acknowledgements
The Mass Spectrometry Center (MSC), Faculty of Chemistry, University of Vienna is acknowledged for providing mass spectrometric instrumentation. The authors thank Thermo Fisher Scientific for providing the Vanquish Duo UHPLC system and their invaluable help regarding software as well as hardware.
References
- A. Doerr, Global Metabolomics, Nat. Methods, 2017, 14(1), 32 CrossRef CAS.
- K. Ortmayr, T. J. Causon, S. Hann and G. Koellensperger, Increasing Selectivity and Coverage in LC-MS Based Metabolome Analysis, TrAC, Trends Anal. Chem., 2016, 82, 358–366 CrossRef CAS.
- T. Cajka and O. Fiehn, Toward Merging Untargeted and Targeted Methods in Mass Spectrometry-Based Metabolomics and Lipidomics, Anal. Chem., 2016, 88(1), 524–545 CrossRef CAS PubMed.
- A. Triebl, M. Trötzmüller, J. Hartler, T. Stojakovic and H. C. Köfeler, Lipidomics by Ultrahigh Performance Liquid Chromatography-High Resolution Mass Spectrometry and Its Application to Complex Biological Samples, J. Chromatogr. B: Anal. Technol. Biomed. Life Sci., 2017, 1053, 72–80 CrossRef CAS PubMed.
- T. Cajka, J. T. Smilowitz and O. Fiehn, Validating Quantitative Untargeted Lipidomics Across Nine Liquid Chromatography–High-Resolution Mass Spectrometry Platforms, Anal. Chem., 2017, 89(22), 12360–12368 CrossRef CAS PubMed.
- M. Holčapek, G. Liebisch and K. Ekroos, Lipidomic Analysis, Anal. Chem., 2018, 90(7), 4249–4257 CrossRef PubMed.
- Y. H. Rustam and G. E. Reid, Analytical Challenges and Recent Advances in Mass Spectrometry Based Lipidomics, Anal. Chem., 2018, 90(1), 374–397 CrossRef CAS PubMed.
- A. Triebl, J. Hartler, M. Trötzmüller and C. Köfeler, H. Lipidomics: Prospects from a Technological Perspective, Biochim. Biophys. Acta, Mol. Cell Biol. Lipids, 2017, 1862(8), 740–746 CrossRef CAS PubMed.
- D. S. Wishart, T. Jewison, A. C. Guo, M. Wilson, C. Knox, Y. Liu, Y. Djoumbou, R. Mandal, F. Aziat and E. Dong,
et al. HMDB 3.0–The Human Metabolome Database in 2013, Nucleic Acids Res., 2013, 41(Database issue), D801–D807 CAS.
- L. Cui, H. Lu and Y. H. Lee, Challenges and Emergent Solutions for LC-MS/MS Based Untargeted Metabolomics in Diseases, Mass Spectrom. Rev., 2018, 37(6), 772–792 CrossRef CAS PubMed.
- N. G. Mahieu and G. J. Patti, Systems-Level Annotation of a Metabolomics Data Set Reduces 25 000 Features to Fewer than 1000 Unique Metabolites, Anal. Chem., 2017, 89(19), 10397–10406 CrossRef CAS PubMed.
- S. Wernisch and S. Pennathur, Evaluation of Coverage, Retention Patterns, and Selectivity of Seven Liquid Chromatographic Methods for Metabolomics, Anal. Bioanal. Chem., 2016, 408(22), 6079–6091 CrossRef CAS PubMed.
- A. Teleki, A. Sánchez-Kopper and R. Takors, Alkaline Conditions in Hydrophilic Interaction Liquid Chromatography for Intracellular Metabolite Quantification Using Tandem Mass Spectrometry, Anal. Biochem., 2015, 475, 4–13 CrossRef CAS PubMed.
- D. R. Stoll and P. W. Carr, Two-Dimensional Liquid Chromatography: A State of the Art Tutorial, Anal. Chem., 2017, 89(1), 519–531 CrossRef CAS PubMed.
- K. Klavins, H. Drexler, S. Hann and G. Koellensperger, Quantitative Metabolite Profiling Utilizing Parallel Column Analysis for Simultaneous Reversed-Phase and Hydrophilic Interaction Liquid Chromatography Separations Combined with Tandem Mass Spectrometry, Anal. Chem., 2014, 86(9), 4145–4150 CrossRef CAS PubMed.
- K. Ortmayr, S. Hann and G. Koellensperger, Complementing Reversed-Phase Selectivity with Porous Graphitized Carbon to Increase the Metabolome Coverage in an on-Line Two-Dimensional LC-MS Setup for Metabolomics, Analyst, 2015, 140(10), 3465–3473 RSC.
- K. Klavins, D. B. Chu, S. Hann and G. Koellensperger, Fully Automated On-Line Two-Dimensional Liquid Chromatography in Combination with ESI MS/MS Detection for Quantification of Sugar Phosphates in Yeast Cell Extracts, Analyst, 2014, 139(6), 1512–1520 RSC.
- D. R. Stoll, X. Wang and P. W. Carr, Comparison of the Practical Resolving Power of One- and Two-Dimensional High-Performance Liquid Chromatography Analysis of Metabolomic Samples, Anal. Chem., 2008, 80(1), 268–278 CrossRef CAS PubMed.
- D. R. Stoll, K. Shoykhet, P. Petersson and S. Buckenmaier, Active Solvent Modulation: A Valve-Based Approach To Improve Separation Compatibility in Two-Dimensional
Liquid Chromatography, Anal. Chem., 2017, 89(17), 9260–9267 CrossRef CAS PubMed.
- M. Holčapek, M. Ovčačíková, M. Lísa, E. Cífková and T. Hájek, Continuous Comprehensive Two-Dimensional Liquid Chromatography–Electrospray Ionization Mass Spectrometry of Complex Lipidomic Samples, Anal. Bioanal. Chem., 2015, 407(17), 5033–5043 CrossRef PubMed.
- W. C. Byrdwell, Dual Parallel Liquid Chromatography with Dual Mass Spectrometry (LC2/MS2) for a Total Lipid Analysis, Front. Biosci., 2008, 13, 100–120 CrossRef CAS.
- H. Nie, R. Liu, Y. Yang, Y. Bai, Y. Guan, D. Qian, T. Wang and H. Liu, Lipid Profiling of Rat Peritoneal Surface Layers by Online Normal- and Reversed-Phase 2D LC QToF-MS, J. Lipid Res., 2010, 51(9), 2833–2844 CrossRef CAS PubMed.
- M. Li, B. Feng, Y. Liang, W. Zhang, Y. Bai, W. Tang, T. Wang and H. Liu, Lipid Profiling of Human Plasma from Peritoneal Dialysis Patients Using an Improved 2D (NP/RP) LC-QToF MS Method, Anal. Bioanal. Chem., 2013, 405(21), 6629–6638 CrossRef CAS PubMed.
- E. Rampler, H. Schoeny, B. M. Mitic, Y. E. Abiead, M. Schwaiger and G. Koellensperger, Simultaneous Non-Polar and Polar Lipid Analysis by on-Line Combination of HILIC, RP and High Resolution MS, Analyst, 2018, 143(5), 1250–1258 RSC.
- A. Baglai, A. F. G. Gargano, J. Jordens, Y. Mengerink, M. Honing, S. van der Wal and P. J. Schoenmakers, Comprehensive Lipidomic Analysis of Human Plasma Using Multidimensional Liquid- and Gas-Phase Separations: Two-Dimensional Liquid Chromatography–Mass Spectrometry vs. Liquid Chromatography–Trapped-Ion-Mobility–Mass Spectrometry, J. Chromatogr. A, 2017, 1530, 90–103 CrossRef CAS PubMed.
- A. F. G. Gargano, M. Duffin, P. Navarro and P. J. Schoenmakers, Reducing Dilution and Analysis Time in Online Comprehensive Two-Dimensional Liquid Chromatography by Active Modulation, Anal. Chem., 2016, 88(3), 1785–1793 CrossRef CAS PubMed.
- T. Huan, Y. Wu, C. Tang, G. Lin and L. Li, DnsID in MyCompoundID for Rapid Identification of Dansylated Amine- and Phenol-Containing Metabolites in LC-MS-Based Metabolomics, Anal. Chem., 2015, 87(19), 9838–9845 CrossRef CAS PubMed.
- Z. Li, J. Tatlay and L. Li, Nanoflow LC-MS for High-Performance Chemical Isotope Labeling Quantitative Metabolomics, Anal. Chem., 2015, 87(22), 11468–11474 CrossRef CAS PubMed.
- X. Luo and L. Li, Metabolomics of Small Numbers of Cells: Metabolomic Profiling of 100, 1000, and 10000 Human Breast Cancer Cells, Anal. Chem., 2017, 89(21), 11664–11671 CrossRef CAS PubMed.
- E. L. Gill, J. P. Koelmel, R. A. Yost, M. S. Okun, V. Vedam-Mai and T. J. Garrett, Mass Spectrometric Methodologies for Investigating the Metabolic Signatures of Parkinson's Disease: Current Progress and Future Perspectives, Anal. Chem., 2018, 90(5), 2979–2986 CrossRef CAS PubMed.
- X. Liu and G. Xu, Recent Advances in Using Mass Spectrometry for Mitochondrial Metabolomics and Lipidomics - A Review, Anal. Chim. Acta, 2017, 1037, 3–12 CrossRef PubMed.
- C. Coman, F. A. Solari, A. Hentschel, A. Sickmann, R. P. Zahedi and R. Ahrends, SIMPLEX: A Combinatorial Multimolecular Omics Approach for Systems Biology, Mol. Cell. Proteomics, 2016, 15(4), 1453–1466 CrossRef CAS PubMed.
- C. D. Broeckling and J. E. Prenni, Stacked Injections of Biphasic Extractions for Improved Metabolomic Coverage and Sample Throughput, Anal. Chem., 2018, 90(2), 1147–1153 CrossRef CAS PubMed.
- Y. Li, Z. Zhang, X. Liu, A. Li, Z. Hou, Y. Wang and Y. Zhang, A Novel Approach to the Simultaneous Extraction and Non-Targeted Analysis of the Small Molecules Metabolome and Lipidome Using 96-Well Solid Phase Extraction Plates with Column-Switching Technology, J. Chromatogr. A, 2015, 1409, 277–281 CrossRef CAS PubMed.
- S. Wang, L. Zhou, Z. Wang, X. Shi and G. Xu, Simultaneous Metabolomics and Lipidomics Analysis Based on Novel Heart-Cutting Two-Dimensional Liquid Chromatography-Mass Spectrometry, Anal. Chim. Acta, 2017, 966, 34–40 CrossRef CAS PubMed.
- K. W. Phinney, G. Ballihaut, M. Bedner, B. S. Benford, J. E. Camara, S. J. Christopher, W. C. Davis, N. G. Dodder, G. Eppe and B. E. Lang,
et al. Development of a Standard Reference Material for Metabolomics Research, Anal. Chem., 2013, 85(24), 11732–11738 CrossRef CAS PubMed.
- J. A. Bowden, A. Heckert, C. Z. Ulmer, C. M. Jones, J. P. Koelmel, L. Abdullah, L. Ahonen, Y. Alnouti, A. M. Armando and J. M. Asara,
et al. Harmonizing Lipidomics: NIST Interlaboratory Comparison Exercise for Lipidomics Using SRM 1950-Metabolites in Frozen Human Plasma, J. Lipid Res., 2017, 58(12), 2275–2288 CrossRef CAS PubMed.
- Y. Simón-Manso, M. S. Lowenthal, L. E. Kilpatrick, M. L. Sampson, K. H. Telu, P. A. Rudnick, W. G. Mallard, D. W. Bearden, T. B. Schock and D. V. Tchekhovskoi,
et al., Metabolite Profiling of a NIST Standard Reference Material for Human Plasma (SRM 1950): GC-MS, LC-MS, NMR, and Clinical Laboratory Analyses, Libraries, and Web-Based Resources, Anal. Chem., 2013, 85(24), 11725–11731 CrossRef PubMed.
- V. Matyash, G. Liebisch, T. V. Kurzchalia, A. Shevchenko and D. Schwudke, Lipid Extraction by Methyl-Tert-Butyl Ether for High-Throughput Lipidomics, J. Lipid Res., 2008, 49(5), 1137–1146 CrossRef CAS PubMed.
- J. P. Koelmel, N. M. Kroeger, E. L. Gill, C. Z. Ulmer, J. A. Bowden, R. E. Patterson, R. A. Yost and T. J. Garrett, Expanding Lipidome Coverage Using LC-MS/MS Data-Dependent Acquisition with Automated Exclusion List Generation, J. Am. Soc. Mass Spectrom., 2017, 28(5), 908–917 CrossRef CAS PubMed.
-
The Fitness for Purpose of Analytical Methods: A Laboratory Guide to Method Validation and Related Topics, ed. B. Magnusson and U. Örnemark, 2nd edn, 2014 Search PubMed.
- M. Schwaiger, E. Rampler, G. Hermann, W. Miklos, W. Berger and G. Koellensperger, Anion-Exchange Chromatography Coupled to High-Resolution Mass Spectrometry: A Powerful Tool for Merging Targeted and Non-Targeted Metabolomics, Anal. Chem., 2017, 89(14), 7667–7674 CrossRef CAS PubMed.
- S. Tulipani, R. Llorach, M. Urpi-Sarda and C. Andres-Lacueva, Comparative Analysis of Sample Preparation Methods To Handle the Complexity of the Blood Fluid Metabolome: When Less Is More, Anal. Chem., 2013, 85(1), 341–348 CrossRef CAS PubMed.
- S. J. Bruce, I. Tavazzi, V. Parisod, S. Rezzi, S. Kochhar and P. A. Guy, Investigation of Human Blood Plasma Sample Preparation for Performing Metabolomics Using Ultrahigh Performance Liquid Chromatography/Mass Spectrometry, Anal. Chem., 2009, 81(9), 3285–3296 CrossRef CAS PubMed.
- J. Folch, M. Lees and G. H. S. Stanley, A Simple Method for the Isolation and Purification of Total Lipides from Animal Tissues, J. Biol. Chem., 1957, 226(1), 497–509 CAS.
- E. G. Bligh and W. J. Dyer, A Rapid Method of Total Lipid Extraction and Purification, Can. J. Biochem. Physiol., 1959, 37(8), 911–917 CrossRef CAS PubMed.
- D. Vuckovic, Current Trends and Challenges in Sample Preparation for Global Metabolomics Using Liquid Chromatography–Mass Spectrometry, Anal. Bioanal. Chem., 2012, 403(6), 1523–1548 CrossRef CAS PubMed.
- J. M. Buescher, S. Moco, U. Sauer and N. Zamboni, Ultrahigh Performance Liquid Chromatography-Tandem Mass Spectrometry Method for Fast and Robust Quantification of Anionic and Aromatic Metabolites, Anal. Chem., 2010, 82(11), 4403–4412 CrossRef CAS PubMed.
- J. Ivanisevic, Z.-J. Zhu, L. Plate, R. Tautenhahn, S. Chen, P. J. O'Brien, C. H. Johnson, M. A. Marletta, G. J. Patti and G. Siuzdak, Toward ‘Omic Scale Metabolite Profiling: A Dual Separation–Mass Spectrometry Approach for Coverage of Lipid and Central Carbon Metabolism, Anal. Chem., 2013, 85(14), 6876–6884 CrossRef CAS PubMed.
- B. A. Mansoori, D. A. Volmer and R. K. Boyd, Wrong-way-round’ Electrospray Ionization of Amino Acids, Rapid Commun. Mass Spectrom., 1997, 11(10), 1120–1130 CrossRef CAS.
- C. S. Ejsing, J. L. Sampaio, V. Surendranath, E. Duchoslav, K. Ekroos, R. W. Klemm, K. Simons and A. Shevchenko, Global Analysis of the Yeast Lipidome by Quantitative Shotgun Mass Spectrometry, Proc. Natl. Acad. Sci. U. S. A., 2009, 106(7), 2136–2141 CrossRef CAS PubMed.
- L. A. Heiskanen, M. Suoniemi, H. X. Ta, K. Tarasov and K. Ekroos, Long-Term Performance and Stability of Molecular Shotgun Lipidomic Analysis of Human Plasma Samples, Anal. Chem., 2013, 85(18), 8757–8763 CrossRef CAS PubMed.
- C. Z. Ulmer, J. M. Ragland, J. P. Koelmel, A. Heckert, C. M. Jones, T. J. Garrett, R. A. Yost and J. A. Bowden, LipidQC: Method Validation Tool for Visual Comparison to SRM 1950 Using NIST Interlaboratory Comparison Exercise Lipid Consensus Mean Estimate Values, Anal. Chem., 2017, 89(24), 13069–13073 CrossRef CAS PubMed.
Footnote |
† Electronic supplementary information (ESI) available. See DOI: 10.1039/c8an01219a |
|
This journal is © The Royal Society of Chemistry 2019 |
Click here to see how this site uses Cookies. View our privacy policy here.