DOI:
10.1039/C8AN01701H
(Paper)
Analyst, 2018,
143, 5987-5998
Raman spectroscopic analysis of high molecular weight proteins in solution – considerations for sample analysis and data pre-processing†
Received
3rd September 2018
, Accepted 24th September 2018
First published on 8th October 2018
Abstract
This study explores the potential of Raman spectroscopy, coupled with multivariate regression techniques and a protein separation technique (ion exchange chromatography), to quantitatively monitor diagnostically relevant changes in high molecular weight proteins in liquid plasma. Measurement protocols to detect the imbalances in plasma proteins as an indicator of various diseases using Raman spectroscopy are optimised, such that strategic clinical applications for early stage disease diagnostics can be evaluated. In a simulated plasma protein mixture, concentrations of two proteins of identified diagnostic potential (albumin and fibrinogen) were systematically varied within physiologically relevant ranges. Scattering from the poorly soluble fibrinogen fraction is identified as a significant impediment to the accuracy of measurement of mixed proteins in solution, although careful consideration of pre-processing methods allows construction of an accurate multivariate regression prediction model for detecting subtle changes in the protein concentration. Furthermore, ion exchange chromatography is utilised to separate fibrinogen from the rest of the proteins and mild sonication is used to improve the dispersion and therefore quality of the prediction. The proposed approach can be expeditiously employed for early detection of pathological disorders associated with high or low plasma/serum proteins.
Introduction
Raman spectroscopy has emerged over the past 20 years as an increasingly routine analytical technique for a wide range of applications, as it provides specific biochemical information without the use of extrinsic labels. This technique can provide intrinsic vibrational signatures of the material of interest in a non-destructive fashion, and its potential for diagnostic applications has been well demonstrated, notably in human serum and plasma.1–4 Raman spectroscopy provides a vibrational signature of a complex biological mixture which is a result of the contributions from all the major components from that mixture, and changes in the concentrations of the components will give rise to notable changes in the Raman signal. However, although both Raman and Fourier-Transform Infrared (FTIR) spectroscopy have been widely explored to study bodily fluids over the last two decades, most of these studies have been carried out on air dried samples, in order to avoid the water contribution in the case of FTIR, and to increase the concentration of the analytes in the case of Raman.5–9 The major limiting factor in the use of dried samples is the so-called “coffee-ring” effect, or, specifically in terms of blood serum, the Vroman effect,10–12 whereby different analytes precipitate from solution at different rates, giving rise to variations in the spectral features due to chemical and physical inhomogeneity. This leads to spatially varying chemical compositions and sample thicknesses, and unreliable results.13 Ultimately, it is desirable to undertake the analysis in the native state of bodily fluids, in which the chemical composition is averaged out by molecular motion over the measurement time, and additional drying steps can be eliminated. This aim naturally favours Raman analysis, as water is a relatively weak Raman scatterer.
In this paper, the sensitivity of Raman spectroscopy to detect subtle changes in a simulated plasma protein-mixture concentration is explored, specifically for the higher molecular weight proteins. Albumin is the most abundant plasma protein, normally constituting about 50% of the plasma protein and has a molecular weight of 66 kDa.14 The normal concentration of albumin in the human body is 30 mg mL−1, although it dramatically decreases in critically ill patients and does not increase again until the recovery phase of the illness.15 Several studies have demonstrated that the functions of albumin, such as ligand binding and transport of various molecules, can be applied to the treatment of cirrhotic patients and patients suffering from other end stage liver diseases.16–18 It is clear that closely monitoring the variation in albumin concentration could act as an indicator of liver diseases and other related pathologies. Fibrinogen is a 340 kDa (0.4% in human plasma) dimeric plasma glycoprotein synthesised by the liver and plays a major role in blood coagulation.19 The normal concentration of fibrinogen in human body is ∼3 mg mL−1, and any variation in this concentration can be an indicator of disease states.20–22 Many clinical studies have consistently shown elevated levels of fibrinogen in patients with cardiovascular disease and thrombosis.23–25
The conventional test kits available in a hospital for plasma/serum analysis suffer from long time delays for the availability of results due to the need of specialised laboratories, which may in turn delay the therapy, and prolong patient anxiety. The potential of vibrational spectroscopy techniques coupled with multivariate analysis techniques have been previously investigated for a range of clinical applications.1–9,26–29 This paper evaluates the potential of Raman spectroscopy as a diagnostic tool to detect minute changes in the plasma protein concentrations in aqueous samples and explores the challenges to such liquid based biopsy techniques, including sample scattering and fractionation of individual constituent components.
A simulated plasma protein mixture of high and low molecular weight proteins, i.e. albumin, fibrinogen, cytochrome c and vitamin B12, at physiologically relevant concentrations, was prepared and variations were made to these concentrations over physiologically relevant ranges. Separation of proteins in the solution was performed by ion exchange chromatography to separate high molecular weight proteins from low molecular weight proteins, and high molecular weight fraction proteins from each other. The efficiency of data pre-processing methods (rubberband and Extended Multiplicative signal Correction (EMSC)) in removing the background, to build an accurate prediction model, was explored and mild sonication was used to improve the dispersion of fibrinogen. The standardisation of measurement protocol and other experimental parameters is detailed and the results of concentration dependence study of proteins, in isolation and protein mixtures, and the chemometric methods used to build the prediction model are presented. This study presents a systematic assessment of some of the challenges presented by measurements of high molecular weight protein mixtures, and some potential solutions to improve the protocols of liquid biopsy monitoring using Raman spectroscopy.
Materials and methods
Preparation of stock protein and protein mixture
Albumin (A9511), fibrinogen (F3879), cytochrome c (C2506) and vitamin B12 (V2876) were purchased from Sigma Aldrich, Ireland. Individual protein solutions of varying concentration were prepared in distilled water, to explore the accuracy of detection of each protein and sensitivity of vibrational spectroscopic techniques to subtle changes in the protein concentrations in its native state. In order to assess the ability of Raman spectroscopic techniques to detect subtle changes in the concentration of the protein-mixture, potentially usable as biomarkers of various disease states, varying concentrations of each protein in the protein-mixture were prepared in distilled water. Concentrations of albumin and fibrinogen, were varied in the protein mixture in the physiologically relevant ranges, from 5 mg mL−1 to 50 mg mL−1 (ref. 15) and 0.5 mg mL−1 to 5 mg mL−1 (ref. 22) respectively while maintaining the concentrations of cytochrome c and vitamin B12 constant. The stock solutions and the protein-mixture solutions and analysed in the liquid form using Raman spectroscopy.
Ultrasonication
A Sonics VCX-750 Vibra Cell Ultra Sonic Processor (Sonics & Materials Inc., USA), equipped with a model CV33 Sonic Tip was used to sonicate the fibrinogen stock solution for 5–10 seconds at 30% amplitude at room temperature to explore the effect of improved dispersion of the fibrinogen on the measurement procedure. Fibrinogen can withstand ultrasonication for 10 seconds at 30% amplitude at low frequency without cleavage of peptide and interchain disulfide bonds or formation of interchain and intermolecular cross-links.26
Ion exchange chromatography
Carboxymethyl-cellulose (C9481) was purchased from Sigma Aldrich, Ireland. It acts as a weak cationic exchanger and binds to the positively charged molecules.27 Glycine (G8898) was purchased from Sigma Aldrich, Ireland and glycine buffer of pH 10 was prepared as the elution buffer.28 1 mL of the protein-mixture was pipetted into a centrifuge tube and 0.08 g of carboxymethyl-cellulose. The solution was mixed for 10 minutes on a Spira-mix roller and then centrifuged at 14
000g for 5 minutes. The unbound material was present in the supernatant and was transferred to a fresh tube. The pellet was washed using 2 mL glycine buffer by repeated inversion, followed by centrifugation at 14
000g for 5 minutes. The supernatant that contains the fibrinogen was carefully transferred to a fresh centrifuge tube and Raman analysis was performed.
Raman spectroscopy
A Horiba Jobin–Yvon LabRam HR800 spectrometer with a 16-bit dynamic range Peltier cooled CCD detector was used to record the Raman spectra throughout this work. The spectrometer was coupled to Olympus 1X71 inverted microscope and a ×60 water immersion objective (LUMPlanF1, Olympus) was employed. In the following experiments 532 nm laser of 12 mW was used with the 600 lines per mm grating and the backscattered Raman signal was integrated for 3 accumulations and a total acquisition time of 80 seconds over the spectral range from 400–1800 cm−1.
Sample substrates
The Lab-Tek plate (154534) was chosen as the optimal substrate for this study. It has a 0.16–0.19 mm thick glass bottom, 1.0 borosilicate cover glass, and was purchased from Thermo Fischer Scientific, Ireland.
Spectral preprocessing
Pre-processing techniques are essential to remove the background signal and reduce the noise, before further analysis. Smoothing of the raw data was done by Savitzky–Golay at a polynomial order of 5 and window 13. Two pre-processing techniques, Extended Multiplicative Signal Correction (EMSC) and the rubberband method, were trialed on the raw dataset of the proteins in Matlab, at different stages of the study. EMSC was employed for the pre-processing of protein data to remove the underlying water spectrum,29 which has an OH bending vibration at ∼1640 cm−1 (ref. 30) which can obscure the protein signals at low concentrations. The reference for EMSC was prepared by adding a few drops of distilled water to the known concentration of protein powder and a thick paste is made (∼10 mg mL−1). Rubberband correction was carried out in Matlab by wrapping a ‘rubberband’ of defined length around the ends of the spectrum to be corrected and fitting against the curved profile of the spectrum.31 A Raman spectrum of the paste was recorded using the 532 nm laser as source and used as the reference spectrum.
Partial least squares regression
Partial Least Squares Regression (PLSR) algorithm was applied to construct a regression model that can be used to predict the outcome in varying concentration of proteins, and the performance model in predicting varying protein concentration was evaluated in this study.32 The PLSR model attempts to elucidate factors that account for the systematic majority of variation in predictors ‘X’ (spectral data) versus associated responses ‘Y’ (target values of protein concentration). The spectral data (X matrix) is thus related to the targets (Y matrix) according to the linear equation Y = XB + E, where B is a matrix of regression coefficients and E is a matrix of residuals. Leave – One – Out cross validation was applied to assess the validity of the model. In this case, the number of latent variables was assessed, enabling the assessment of the performance of a model when applied to an unknown data set. The number of latent variables used for building the PLSR model is optimised by finding the value that is equivalent to the minimum of the Root Mean Square Error of Cross Validation (RMSECV) and percent variance explained by the latent variables. The spectral data obtained from the 30 samples were split as 20% training and 30% test sets and the RMSECV was calculated. RMSECV is used to evaluate the robustness of the constructed model.33 The percent variance plot explains the number of components required for maximum variation in the input data. The appropriateness of various pre-processing methods can be determined through the performance of the PLSR model.
Results
Standardisation of measurement protocol
For the analysis of liquid protein samples, an optimised inverted set-up, previously demonstrated by Bonnier et al.13 was used. Better analysis of serum using Raman spectroscopy was reported when the sample was analysed in the inverted geometry using a water immersion objective with a 785 nm laser and CaF2 substrate. In this study, a ×60 water immersion objective is used with a 532 nm laser and the substrate used was a Lab-Tek plate. The 532 nm laser was chosen as it is compatible with (thin glass bottomed) Lab-Tek plate substrates and provides a strong Raman signal of water with minimal background interference. A drop of water is used to minimise the differences in the refractive indexes between sample, objective and the substrate. However, the water drop does not contribute to the data collected, as it is outside the focus of the beam. This set-up also has an added advantage of providing high quality, consistent Raman spectra from a sample volumes as low as 1 μL.
Fig. 1 presents the spectra of the fingerprint region of the stock solutions of proteins recorded in the inverted geometry. The raw spectra of the proteins were baseline corrected using the rubberband method and smoothed using the Savitzky–Golay algorithm (polynomial 5, window 13). Measurement in the inverted geometry, using a water immersion objective, is found to be the best instrumental set up that enables an increase in the overall spectral intensity accompanied by an improved signal to noise (S/N) ratio with small sample volume.
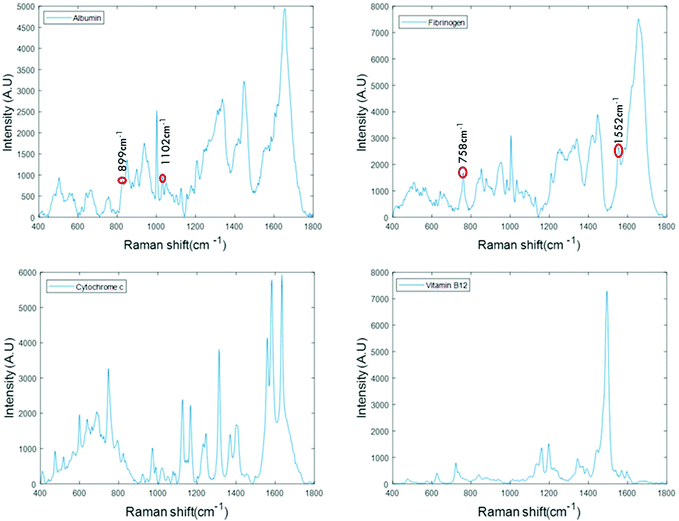 |
| Fig. 1 Raman spectra of the stock solutions of albumin, fibrinogen, cytochrome c and vitamin B12 recorded in the finger print region in the inverted geometry focused by water immersion ×60 objective. Well-defined Raman peaks with minimum background were obtained. | |
The spectra of albumin and fibrinogen shown in Fig. 1 clearly reveal the common Raman peaks of these two proteins. These include the amide I band around ∼1659 cm−1, a relatively sharp band at 1003 cm−1 associated with phenylalanine, intense bands at ∼1336 cm−1 and ∼1450 cm−1 due to C–H deformation, and a vibration band at ∼940 cm−1 related to C–C stretching mode backbone of α-helix structure. The signature peaks of albumin that differentiate it from fibrinogen are bands at 899 cm−1 and 1102 cm−1, that can be related to ν(CC) and ν(CN).34 The signature peaks of fibrinogen are sharp bands observed at 758 cm−1 and 1552 cm−1 that can be assigned to tryptophan.35 Raman bands of cytochrome c and vitamin B12 are highly specific and can be easily distinguished, as evidenced in Fig. 1.36,37
Monitoring the concentration dependence of proteins in aqueous solution
Albumin.
Protein solutions were prepared by varying the concentration of albumin in order to achieve the physiologically relevant range from 5 mg mL−1 to 50 mg mL−1. Fig. 2A show the raw unpre-processed spectra, which exhibit a steady increase in the spectral intensity when the concentration is increased from 5 mg mL−1 to 50 mg mL−1. The spectrum of the highest concentration clearly shows albumin features, whereas those of the lower concentrations are dominated by water, which has a characteristic OH bending mode at ∼1640 cm−1. As the concentration of albumin increases, a notable increase in the background can also be observed, which can be attributed to scattering. Although many studies suggest that the broad background present in Raman spectra is due to fluorescence,38 albumin is a non-resonant protein that is optically transparent at 532 nm, so the background is rather due to scattering of the source laser as well as the Raman scattered light, which enters the spectrometer as stray light, and is dispersed across the CCD in a wavelength independent fashion.39 In order to analyse the spectral variations and the albumin concentrations, the PLSR algorithm was applied. The percent variance plot in Fig. 2B gives a rough indication of how the algorithm progressively fits the spectral data, showing that nearly 68% of the variance is explained by the first component, while as many as four additional components make significant contributions.
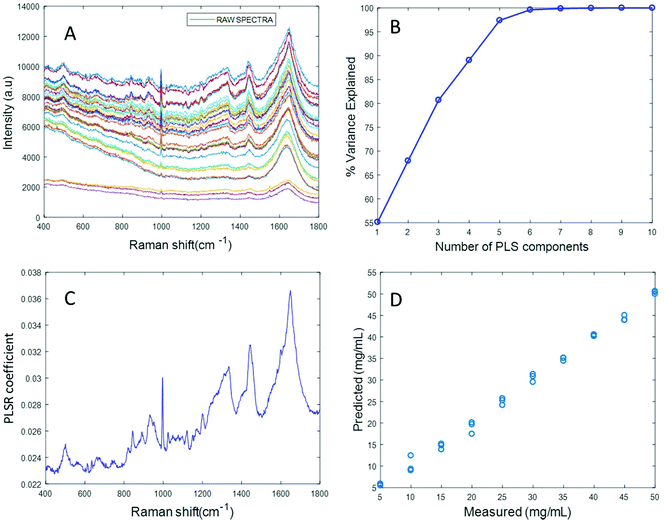 |
| Fig. 2 (A) Raw Raman spectra of varying concentrations of albumin (5 mg mL−1–50 mg mL−1) in distilled water recorded using 532 nm laser, (B) percent variance explained by the components, (C) plot of PLSR coefficient with Albumin features, (D) linear predictive model built from the PLSR analysis. | |
Based on the percent variance explained by the latent variables and the minimum value of RMSECV, the optimum number of latent variables to reach the best model is determined. The PLSR coefficient plot displayed in Fig. 2C, confirms the correlation of the data in Fig. 2D is based on albumin features, such as the peaks at ∼1665 cm−1, ∼1448 cm−1 and ∼1337 cm−1. Finally, after selecting the optimum number of components for the data set analysed, a predictive model is built from the PLSR analysis (Fig. 2D), to compare the observations to the known concentrations of albumin in the samples with the estimated concentrations from the spectral data sets. Fig. 2D indicates that a good linear model could be obtained with the raw data set. However, the PLSR coefficient is not a clean albumin spectrum and has a large background due to scattering, indicating that scattering could have influenced the model. Furthermore, the minimum value of RMSECV was found to be 22.59 mg mL−1, indicating a poor accuracy of prediction over the range 5 mg mL−1 to 50 mg mL−1. Analysis of the raw albumin concentration dependence serves as an initial illustration of some of the issues presented by measurement of high molecular weight macromolecules in solution. Appropriate pre-processing steps could help to minimise the background from scattering effects. Hence, rubberband pre-processing steps were performed on the data set before PLSR analysis and the model obtained is displayed in Fig. 3.
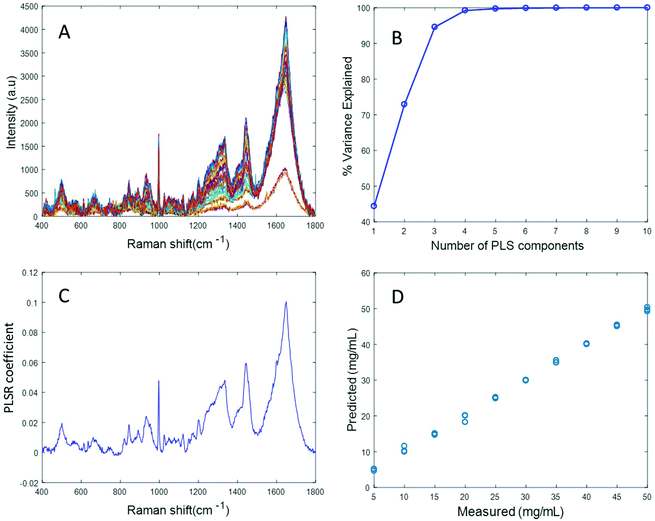 |
| Fig. 3 (A) Rubberband corrected Raman spectra of varying concentrations of Albumin (5 mg mL−1–50 mg mL−1) in distilled water, (B) % variance explained by the latent variables, (C) plot of PLSR coefficient with Albumin features, (D) linear predictive model built from the PLSR analysis. | |
Fig. 3A shows the albumin data set after background correction using the rubberband method. Fig. 3B shows the percent variance explained by the latent variables, indicating that three components accounted for the majority of the variance. Five latent variables were chosen for this model and the resultant PLSR coefficient exhibits strong albumin features, as shown in Fig. 3C. A linear predictive model can be defined from the rubberband corrected data set of varying concentration of albumin in water Fig. 3D. The RMSECV was found to be 1.58 mg mL−1 after applying the rubberband pre-processing steps for the same data set. The results suggest that there is a significant improvement in the predictive capacity of the constructed model when rubberband pre-processing steps are applied to the data set.
Simulated “pathological” plasma protein mixtures were prepared by varying the concentration of albumin in order to achieve the physiologically relevant range from 5 mg mL−1 to 50 mg mL−1 and by maintaining the concentrations of fibrinogen, cytochrome c and vitamin B12 constant at the concentrations of the “healthy” human plasma. The concentrations for hypoalbuminemia (>30 mg mL−1) and hyperalbuminemia (<30 mg mL−1) have been deliberately included in the set of samples being prepared. Based on the results of Fig. 2, rubberband correction was applied to the dataset in an attempt to improve the accuracy of the prediction by performing baseline correction. Notably, the Raman spectral features of the protein mixture were seen to decrease with increasing albumin concentration (Fig. S1A in ESI†), and the PLSR coefficient obtained from this data shows inverse albumin features (Fig. S1C†), indicating that the model built from this dataset is not reliable, as the high degree of scattering is effecting the dataset and the prediction model is not based on the albumin features. Hence, the EMSC based algorithm was applied to the data set in an attempt to eliminate the scattering associated with the albumin data in the simulated plasma and subsequently improve the prediction model. EMSC of polynomial order 4 was performed on the data set of varying concentration of albumin in simulated plasma protein mixture. The reference used for EMSC is a spectrum of albumin which has been diluted with a minimum amount of water, recorded with 532 nm.
Fig. 4A displays the albumin spectra after performing background correction using the EMSC algorithm. The amide 1 band at 1665 cm−1 and CH2 deformation band at 1445 cm−1 can be clearly seen in the corrected spectra. Based on the percentage variance explained by the latent variables (Fig. 4B) and the minimum value of RMSECV, seven latent variables were found to be optimal for this model. The PLSR coefficient shows albumin features (Fig. 4C), indicating that the prediction is now based on the variation in the albumin peak intensity. A linear prediction model was achieved from this model (Fig. 4D). The minimum value of RMSECV is 1.5844 mg mL−1, indicating an improved prediction capacity. This value is the same as the minimum value of RMSECV recorded for the varying concentration of albumin in distilled water, indicating that the PLSR model of EMSC corrected simulated plasma spectra is as accurate as the PLSR model of rubberband corrected spectra of varying concentrations of pure albumin in water. The results demonstrated in this section suggest that this model can be effectively used to detect variations in the concentration of albumin in human plasma, as a result, for example, of liver disorders at an early stage. A strong reduction in the RMSECV indicates that the EMSC algorithm can efficiently subtract the background without altering the albumin features, which in turn improves the prediction of the model.
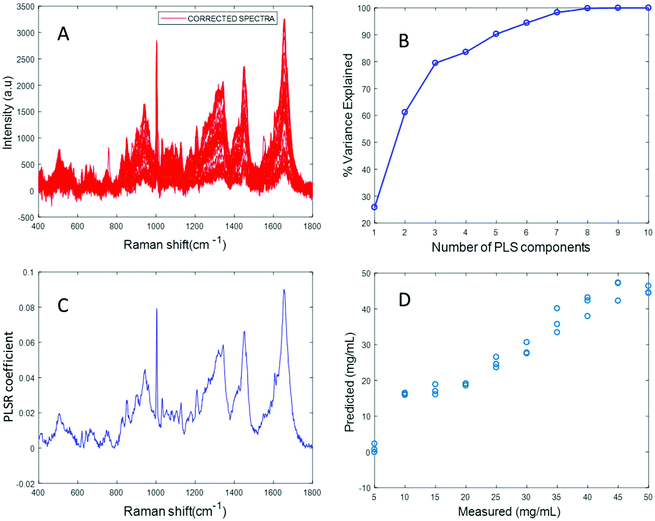 |
| Fig. 4 (A) EMSC corrected of varying concentrations of albumin in simulated plasma, and (B) percent variance explained by the latent variables, (C) PLSR coefficient showing albumin features, and (D) linear prediction model defined from the dataset. | |
Fibrinogen.
Fibrinogen solutions were prepared by diluting the stock solution of 100 mg ml−1 to the more physiologically relevant range of 0.5 mg mL−1 to 5 mg mL−1. Raman spectra were recorded from the protein samples and smoothed using Savitzky–Golay (polynomial 5, window 13). When the rubberband method was applied on this dataset to perform baseline correction, the PLSR coefficient spectrum obtained was an inverse water spectrum, as shown in ESI (Fig. S2†). Fibrinogen is poorly soluble in water, such that the fibrinogen solution is visually cloudier than the albumin solution. This significant problem of lack of solubility due to the protein aggregation leads to scattering of the more pronounced Raman signal of the water, in a concentration dependent fashion. Hence, EMSC with a polynomial of order 4 was performed on the same data set to pre-process the data prior to PLSR analysis. The reference spectrum was obtained under similar conditions as the albumin reference, from a fibrinogen paste with minimal amount of water. A polynomial of order 3 resulted in the best correction. The output, however, is a very noisy spectral data set with some indication of fibrinogen features in the spectra, notably at ∼758 cm−1, ∼1650 cm−1, ∼1450 cm−1, ∼1336 cm−1 and ∼1250 cm−1 (Fig. S3 in ESI†).
In an attempt to overcome the lack of solubility of the protein, the stock solution was ultrasonicated to enhance the dispersion of fibrinogen and obtain a clear solution. Ultrasonication for approximately 10 seconds at 30% amplitude resulted in a clear solution of fibrinogen with a significantly improved Raman signal (Fig. S4 in ESI†). Varying concentrations of fibrinogen samples in the physiologically relevant range were prepared using the ultrasonicated fibrinogen stock.
The spectrum of sonicated fibrinogen after background correction using the EMSC algorithm with polynomial of order 3 displays strong fibrinogen features with higher intensity over the same concentration range, compared to the non-sonicated fibrinogen samples (Fig. 5A). Applying PLSR, it is clear from Fig. 5B that a total of six components made significant contributions to explain the variance in the sonicated fibrinogen spectra. Based on the percent variance explained, six latent variables were used to build the prediction model. The PLSR coefficient plot shows signature peaks of fibrinogen, indicating that the prediction was based on variation in the fibrinogen spectral intensities (Fig. 5C). A linear prediction model was defined from the data set, showing correlation between the Raman peak intensity and concentration (Fig. 5D). The minimum value of RMSECV is found to be 0.0615 mg mL−1. The reduction in the RMSECV value recorded for fibrinogen data after sonication indicates that the accuracy of the model increases as a result of the improved solubility following sonication. Hence, it can be concluded that sonication improves the solubility of the fibrinogen and increases the spectral intensity, in turn leading to a considerable improvement in the predictive capacity of the model.
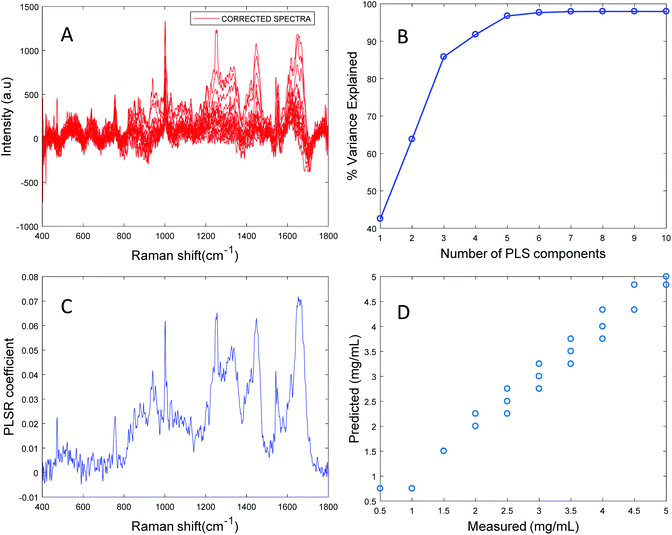 |
| Fig. 5 (A) Raman spectra of varying concentration of sonicated fibrinogen background corrected using EMSC algorithm (B) percent variance explained by the latent variables (C) PLSR coefficient plotted from the sonicated fibrinogen data set shows strong fibrinogen features, (D) linear predictive model built from the PLSR analysis showing correlation between concentration and peak intensity. | |
Simulated “pathological” plasma protein-mixture was prepared by varying the concentration of fibrinogen stock in order to achieve the physiologically relevant range from 0.5 mg mL−1 to 5 mg mL−1 and by maintaining the concentrations of albumin, cytochrome c and vitamin B12 constant at the normal concentrations in healthy human plasma. The concentrations for heart disorders (<3 mg mL−1) and liver disorders (<3 mg mL−1) have been deliberately included in the concentration range. The raw spectra of varying concentrations of fibrinogen in simulated plasma were smoothed by Savitzky–Golay, polynomial of 5, window 13 (Fig. 6).
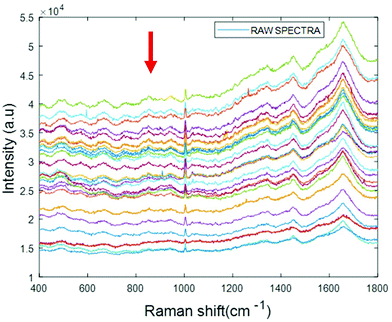 |
| Fig. 6 Smoothed spectra of varying concentration of fibrinogen in simulated plasma (0.5 mg mL−1 to 5 mg mL−1). The arrow indicates the order of increasing concentration. | |
The arrow indicates that both the background and spectral features themselves decrease with increasing concentration of fibrinogen. However, noting that albumin is the dominant contributor to the Raman signal, and that fibrinogen is the dominant scatterer, this can be understood as a (fibrinogen) concentration dependent loss of (albumin) Raman scattering.
The PLSR coefficient obtained after pre-processing the data using the EMSC based algorithm shows an inverse spectrum of albumin rather than fibrinogen, as shown in Fig. S5 in ESI.† As in the case of the water dispersions, the dominant effect of increasing concentrations of the poorly soluble fibrinogen is the scattering of the dominant Raman spectrum. Hence, although the predictive model built from this dataset shows a good correlation with fibrinogen concentration, it is not based on the characteristic spectroscopic signature of fibrinogen, and the variation of the albumin signal could equally be due to any other scatterer.
Ultracentrifugation using 100 kDa centrifugal filters failed to separate fibrinogen from the rest of the protein in the protein mixture. Fig. S6† shows that the Raman spectrum of the concentrate obtained has pronounced characteristic albumin features at 899 cm−1 and 1102 cm−1. Ion exchange chromatography was therefore explored as an alternative method for fibrinogen separation from the protein mixture, based on its charge. Carboxymethyl-cellulose acts as a weak cationic exchanger and fibrinogen is eluted out by altering the net charge of the bound protein, and thus its matrix binding capacity. Fibrinogen was detected in the unbound fraction. Albumin was not detected in the unbound fraction by Raman spectroscopy and it is concluded adsorption of the albumin fraction to the carboxymethyl cellulose resin occurred at the pH values employed. Other studies have shown carboxymethyl cellulose may form insoluble complexes with serum albumin.40
Fibrinogen was extracted from the protein mixtures over the full concentration range, and Raman spectra were recorded from the separated fibrinogen and EMSC was performed on the data set before doing PLSR analysis. In the absence of sonication the prediction model performed poorly, due to the high degree of scattering, as seen in Fig. S7.† Mild sonication can be employed to improve the solubility of and reduce the scattering from fibrinogen, and thus the performance of the prediction model.
The spectrum of sonicated fibrinogen separated by ion exchange chromatography after background correction using the EMSC algorithm displays strong fibrinogen features. In Fig. 7B, it is clear that nine components made significant contributions to the variance in the sonicated fibrinogen spectra. The minimum value of RMSECV is found to be 0.0568 mg mL−1. The PLSR coefficient plot shows the signature peaks of fibrinogen (Fig. 7C), indicating that the linear prediction model obtained was based on the correlation between the Raman spectral intensities of fibrinogen and concentration (Fig. 7D). Hence, it can be concluded that ion exchange chromatography can successfully separate fibrinogen for Raman analysis from the protein mixture within 30 minutes and an accurate prediction model can be built from the Raman data to detect subtle changes in the fibrinogen concentration. Early detection of fibrinogen concentration could help to prevent disorders that are associated with increased fibrinogen level in plasma such as thromboembolism,41 various cardiovascular events and post-surgical arterial reocclusion.42
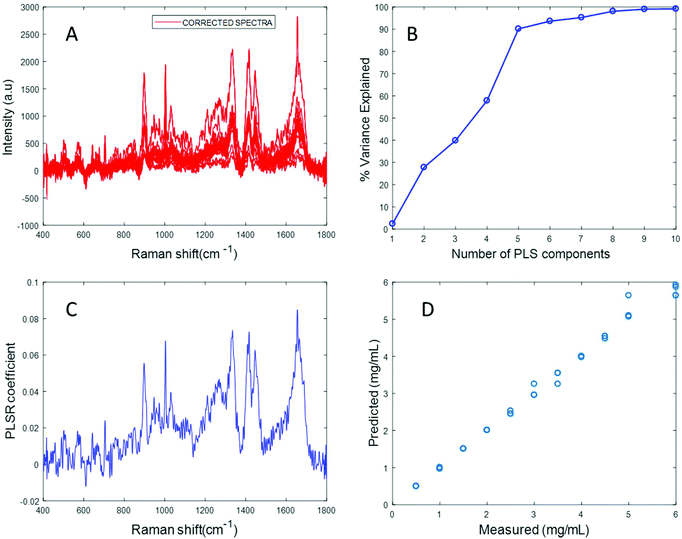 |
| Fig. 7 (A) EMSC corrected data of varying concentrations of fibrinogen separated by ion exchange chromatography, and (B) percent variance explained by the latent variables, (C) PLSR coefficient showing fibrinogen features and (D) linear prediction model defined from the dataset. | |
Discussion
In monitoring biological molecules in their native aqueous state in biofluids, Raman spectroscopy offers the potential advantage over other spectroscopic techniques such as infrared absorption, that water was a relatively low scattering cross section. However, applications of the technique face several challenges related to detection of relatively low concentrations and variations of concentrations of analytes, and low quality signals from poorly dispersed components, and there remains a considerable number of issues relating to the fundamental process of recording and extracting the spectral details using chemometric techniques.
Raman analysis in the inverted geometry using a water immersion objective is found to be the optimal method to record well defined spectra with minimal background, and notably samples of volumes as low as 1 μL can be measured. In a sample set of varying concentrations over physiologically relevant ranges, the albumin contributions to the spectrum dominate over those of the water, and, after minimal preprocessing, PLSR can be employed to establish a regression model whose predictive performance shows a close correlation between the concentrations of the proteins and the Raman spectral profile. However, in a the more complex simulated plasma mixture of proteins, improved data preprocessing techniques are required to account for the increased spectral background.
Although the broad background to Raman spectra is often attributed to fluorescence, this cannot be the case for materials with are nonresonant at the Raman source wavelength. Proteins such as albumin and fibrinogen can, however, contribute to stray Mie scattered light by causing diffusely scattered radiation that is not well collimated by the collection objective of the Raman microscope, enters the spectrometer effectively as stray light, and is dispersed across the detector.21 The rubberband pre-processing method appeared to efficiently remove the background from the data set of varying concentration of albumin in water, but failed to satisfactorily deal with the background of varying concentrations of albumin in the simulated plasma protein mixture. The more sophisticated EMSC based algorithm helped eliminate the scattering associated with the albumin data in the simulated plasma, improving the prediction model, and also helped to extract the spectral features of fibrinogen from water. In both cases, before subtraction, the primary effect of varying the protein concentrations was to decrease the contribution of the dominant Raman scatterer, which can be understood in terms of the presence of the poorly soluble, highly Mie scattering fibrinogen component. This proposed method can be efficiently used to detect albumin as a standard biomarker for detecting diseases associated with hypoalbuminemia (<30 mg mL−1), such as liver diseases, gastrointestinal protein loss, edema and hyperalbuminemia (>30 mg mL−1), such as severe dehydration and abnormal increase in body fat.43,44 The accuracy of the proposed method is comparable to that of the most commonly used method for detecting albumin from biological fluids, the enzyme linked immunosorbent assay (ELISA),45,46 which is sensitive and selective but is very time consuming and requires extensive sample preparation steps.
In varying concentrations of fibrinogen in aqueous solution, the Raman signal of the water itself is diffusely scattered, increasingly so with increasing fibrinogen concentration, and thus the PLSR identifies a decreasing Raman contribution of water as the dominant concentration dependent effect. In the case of albumin in the simulated protein mixture, a concentration dependent Mie scattering of the Raman signal of albumin itself is the dominant effect of increasing albumin concentration. While one would expect a linear concentration dependent increase in the Raman signal of albumin, the inability of the ultracentrifugation technique to separate the two high molecular weight proteins may suggest an interaction between the albumin and fibrinogen, such that increased albumin Raman scattering is overwhelmed by increased Mie scattering.
Mild sonication is seen to improve the dispersion of fibrinogen in aqueous solutions, and significantly improve the Raman signal. Removing the water contribution using EMSC is seen to significantly improve the predictive model (Fig. 5).
Separation of the fibrinogen by ion exchange chromatography from the plasma protein mixture and application of the ultrasonication technique to reduce aggregation helped to detect fibrinogen features from the plasma solution even at a concentration as low as 0.5 mg mL−1. The RMSECV of 0.0568 mg mL−1 compares favourably with similar observations, for example for attenuated total reflection – Fourier transform infrared absorption monitoring of glucose in blood serum.47 The accuracy of this study is closer to that of the most commonly used gold-standard method i.e., the Clauss assay, which has a detection limit of ∼0.4 mg mL−1.48 The Clauss assay is relatively time consuming and suffers from inconsistencies in the results due to calibration standards, methodologies and variation in the reagents from various manufacturers.41 These steps are relevant only in the case of human plasma and can be avoided while working with human serum as fibrinogen is absent in the serum. The optimised protocol can be applied to detect low abundant protein in bodily fluids after depletion of the abundant proteins to reduce the spectral variability. Currently, such studies are conducted and the results are promising.
Ion exchange chromatography is a quick method to separate the proteins from each other by altering their net surface charge, making it an ideal tool for separating all the protein constituents and a better alternative to ultracentrifugation. In this case, ultracentrifugation failed to separate HMWF proteins from one another, as they tend to form hydrophobic bonds and nonspecific binding interactions with the membrane material (Fig. S6†). However, the ion exchange chromatographic method has to be tailored to the specific protein, depending on its charge, and cannot be applied as a ‘one-for-all’ separation kit for all the proteins.
Conclusions
The potential advantages of using vibrational spectroscopy for disease diagnosis based on bodily fluids have been extensively explored over the last two decades. However, little consideration has been given to date to the optimisation of a Raman analysis protocol involving proteins in their native aqueous state, leading to irreproducible results due to high complexity of the plasma proteins. This study is a proof of concept that Raman spectroscopy can be successfully used to detect subtle changes in individual plasma protein concentration from simulated plasma samples to disease diagnostics purposes.
It has been shown that measurement in the inverted geometry using a water immersion objective yields high quality spectra and the sample volume can be as small as 1 μL. This experimental set up is advantageous for clinical purposes where the volumes of patient samples are minimal. In the simulated plasma protein mixture, the poorly soluble fibrinogen component was seen to obscure the systematic variations of the protein concentrations, due to the high degree of scattering. Extraction of the fibrinogen by ion exchange chromatography is seen to be more specific than by ultracentrifugal filtration, such that the variations of fibrinogen levels themselves can be quantified. In general, the scattering problems caused by fibrinogen favour the use of blood serum for the analysis of the remaining lower molecular weight fractions.
However, to further ensure relevancy and consistency of these results, experiments need to be carried out in pooled plasma/serum. The use of Raman spectroscopy coupled with chemometric techniques not only gives a mere estimate of whether the protein levels are high or low but also gives higher accuracy of quantification. Once appropriate experimental methods are established, a hypothesised point-of-care device that can be used in real clinical applications for spectroscopic analysis of body fluids can be realised. The proposed approach can be expeditiously employed for early detection of pathological disorders associated with high or low plasma proteins.
Conflicts of interest
There are no conflicts to declare.
Acknowledgements
This project was funded by DIT Fiosraigh scholarship. J. McIntyre was funded by Science Foundation Ireland, PI/11/08.
References
- F. Bonnier, M. J. Baker and H. J. Byrne, Vibrational spectroscopic analysis of body fluids: avoiding molecular contamination using centrifugal filtration, Anal. Methods, 2014, 6(14), 5155 RSC
. Available from: http://pubs.rsc.org/en/content/articlehtml/2014/ay/c4ay00891j.
- A. A. Bunaciu, Ş. Fleschin, V. D. Hoang and H. Y. Aboul-Enein, Vibrational Spectroscopy in Body Fluids Analysis, Crit. Rev. Anal. Chem., 2017, 47(1), 67–75 CrossRef CAS PubMed
.
-
M. J. Baker, C. S. Hughes and K. A. Hollywood, Biophotonics: Vibrational Spectroscopic Diagnostics, Morgan & Claypool Publishers, 2016. DOI:10.1088/978-1-6817-4071-3
.
- A. L. Mitchell, K. B. Gajjar, G. Theophilou, F. L. Martin and P. L. Martin-Hirsch, Vibrational spectroscopy of biofluids for disease screening or diagnosis: Translation from the laboratory to a clinical setting, J. Biophotonics, 2014, 7(3–4), 153–165 CrossRef CAS PubMed
.
- M. J. Baker, S. R. Hussain, L. Lovergne, V. Untereiner, C. Hughes and R. A. Lukaszewski,
et al., Developing and understanding biofluid vibrational spectroscopy: a critical review, Chem. Soc. Rev., 2015, 45(7), 1803–1818 RSC
. Available from: http://pubs.rsc.org/en/content/articlehtml/2016/cs/c5cs00585j.
- A. Oleszko, S. Olsztyńska-Janus, T. Walski, K. Grzeszczuk-Kuć, J. Bujok and K. Gałecka,
et al., Application of FTIR-ATR spectroscopy to determine the extent of lipid peroxidation in plasma during haemodialysis, BioMed Res. Int., 2015, 2015, 1–8 CrossRef PubMed
.
- A. Sahu, K. Dalal, S. Naglot, P. Aggarwal and C. M. Krishna, Serum based diagnosis of asthma using Raman spectroscopy: An early phase pilot study, PLoS One, 2013, 8(11), e78921 CrossRef CAS PubMed
.
- D. Sheng, Y. Wu, X. Wang, D. Huang, X. Chen and X. Liu, Comparison of serum from gastric cancer patients and from healthy persons using FTIR spectroscopy, Spectrochim. Acta, Part A, 2013, 116, 365–369, DOI:10.1016/j.saa.2013.07.055
.
- D. Perez-Guaita, J. Ventura-Gayete, C. Pérez-Rambla, M. Sancho-Andreu, S. Garrigues and M. De La Guardia, Protein determination in serum and whole blood by attenuated total reflectance infrared spectroscopy, Anal. Bioanal. Chem., 2012, 404(3), 649–656 CrossRef CAS PubMed
.
- L. Vroman, A. L. Adams, G. C. Fischer and P. C. Munoz, Interaction of high molecular weight kininogen, factor XII, and fibrinogen in plasma at interfaces, Blood, 1980, 55(1), 156–159 CAS
.
- A. H. Schmaier, L. Silver, A. L. Adams, G. C. Fischer, P. C. Munoz and L. Vroman,
et al., The effect of high molecular weight kininogen on surface-adsorbed fibrinogen, Thromb. Res., 2017, 33(1), 51–67, DOI:10.1016/0049-3848(84)90154-3
.
- A. L. Adams, G. C. Fischer, P. C. Munoz and L. Vroman, Convex-lens-on-slide: A simple system for the study of human plasma and blood in narrow spaces, J. Biomed. Mater. Res., 1984, 18(6), 643–654 CrossRef CAS PubMed
. Available from: http://doi.wiley.com/10.1002/jbm.820180606 [cited 2017 Oct 31].
- F. Bonnier, F. Petitjean, M. J. Baker and H. J. Byrne, Improved protocols for vibrational spectroscopic analysis of body fluids, J. Biophotonics, 2014, 7(3–4), 167–179 CrossRef CAS PubMed
.
- J. P. Nicholson, M. R. Wolmarans and G. R. Park, The role of albumin in critical illness, Br. J. Anaesth., 2000, 85(4), 599–610 CrossRef CAS PubMed
.
-
J. T. Busher, Serum Albumin and Globulin, in Clinical Methods: The History, Physical, and Laboratory Examinations, ed. H. K. Walker, W. D. Hall, and J. W. Hurst, Butterworths, Boston, 3rd edn, 1990, ch. 101, pp. 497–499. Available from: https://www.ncbi.nlm.nih.gov/books/NBK204/ Search PubMed
.
- V. Arroyo, R. García-Martinez and X. Salvatella, Human serum albumin, systemic inflammation, and cirrhosis, J. Hepatol., 2014, 61(2), 396–407, DOI:10.1016/j.jhep.2014.04.012
, European Association for the Study of the Liver.
- T. B. Vree, M. Shimoda, J. J. Driessen, P. J. Guelen, T. J. Janssen and E. F. Termond,
et al., Decreased plasma albumin concentration results in increased volume of distribution and decreased elimination of midazolam in intensive care patients, Clin. Pharmacol. Ther., 1989, 46(5), 537–544 CrossRef CAS PubMed
. Available from: http://www.ncbi.nlm.nih.gov/entrez/query.fcgi?cmd=Retrieve&db=PubMed&dopt=Citation&list_uids=2582710.
- V. Arroyo, Review article: albumin in the treatment of liver diseases–new features of a classical treatment, Aliment. Pharmacol. Ther., 2002, 16(Suppl 5), 1–5 CrossRef CAS PubMed
. Available from: http://www.ncbi.nlm.nih.gov/pubmed/12423447.
- R. M. Cappelletti, Fibrinogen and Fibrin: Structure and Functional Aspects, J. Thromb. Haemostasis, 2012, 263–291 Search PubMed
.
- L. Sheng, M. Luo, X. Sun, N. Lin, W. Mao and D. Su, Serum fibrinogen is an independent prognostic factor in operable nonsmall cell lung cancer, Int. J. Cancer, 2013, 133(11), 2720–2725 CAS
.
- K. T. Nyuwi, C. H. Gyan Singh, S. Khumukcham, R. Rangaswamy, Y. S. Ezung and S. R. Chittvolu,
et al., The role of serum fibrinogen level in the diagnosis of acute appendicitis, J. Clin. Diagn. Res., 2017, 11(1), PC13–PC15 CrossRef PubMed
.
- I. O. Tekin, B. Pocan, A. Borazan, E. Ucar, G. Kuvandik and S. Ilikhan,
et al., Positive correlation of CRP and fibrinogen levels as cardiovascular risk factors in early stage of continuous ambulatory peritoneal dialysis patients, Renal Failure, 2008, 30(2), 219–225 CrossRef CAS PubMed
.
- J. J. Stec, H. Silbershatz, G. H. Tofler, T. H. Matheney, P. Sutherland and I. Lipinska,
et al., Association of fibrinogen with cardiovascular risk factors and cardiovascular disease in the Framingham Offspring Population, Circulation, 2000, 102(14), 1634–1638 CrossRef CAS PubMed
.
- R. A. S. Ariëns, Elevated fibrinogen causes thrombosis, Blood, 2011, 4687–4688 CrossRef PubMed
.
- L. F. Hong, X. L. Li, S. H. Luo, Y. L. Guo, C. G. Zhu and P. Qing,
et al., Association of fibrinogen with severity of stable coronary artery disease in patients with type 2 diabetic mellitus, Dis. Markers, 2014, 2014, 485687 Search PubMed
. Available from: http://www.ncbi.nlm.nih.gov/pubmed/24803720%5Cn, http://www.ncbi.nlm.nih.gov/pmc/articles/PMC3997864/pdf/DM2014-485687.pdf.
- E. A. Cherniavsky, I. S. Strakha, I. E. Adzerikho and V. M. Shkumatov, Effects of low frequency ultrasound on some properties of fibrinogen and its plasminolysis, BMC Biochem., 2011, 12(1), 1–12 CrossRef PubMed
.
-
G. Healthcare, Ion Exchange Chromatography & Chromatofocusing: Principles and Methods, GE Heal Handbooks, 2016, pp. 170 Search PubMed
.
- W. Alan and F. Verna, Ion-Exchange Chromatography, Curr. Protoc. Mol. Biol., 1998, 44(1), 10.10.1–10.10.30 Search PubMed
. Available from: https://currentprotocols.onlinelibrary.wiley.com/doi/abs/10.1002/0471142727.mb1010s44.
- A. Kohler, J. Sulé-Suso, G. D. Sockalingum, M. Tobin, F. Bahrami and Y. Yang,
et al., Estimating and Correcting Mie Scattering in Synchrotron-Based Microscopic Fourier Transform Infrared Spectra by Extended Multiplicative Signal Correction, Appl. Spectrosc., 2008, 62(3), 259–266, DOI:10.1366/000370208783759669
.
- Z. Wang, A. Pakoulev, Y. Pang and D. D. Dlott, Vibrational substructure in the OH stretching transition of water and HOD, J. Phys. Chem. A, 2004, 108(42), 9054–9063 CrossRef CAS
.
- H. J. Byrne, P. Knief, M. E. Keating and F. Bonnier, Spectral pre and post processing for infrared and Raman spectroscopy of biological tissues and cells, Chem. Soc. Rev., 2016, 45(7), 1865–1878 RSC
. Available from: http://xlink.rsc.org/?DOI=C5CS00440C.
- S. Wold, M. Sjöström and L. Eriksson, PLS-regression: A basic tool of chemometrics, Chemom. Intell. Lab. Syst., 2001, 58(2), 109–130 CrossRef CAS
.
- B.-H. Mevik and R. Wehrens, The pls Package: Principle Component and Partial Least Squares Regression in R, J. Stat. Softw., 2007, 18(2), 1–24 Search PubMed
. Available from: http://www.jstatsoft.org/v18/i02/paper.
- D. N. Artemyev, V. P. Zakharov, I. L. Davydkin, J. A. Khristoforova, A. A. Lykina and V. N. Konyukhov,
et al., Measurement of human serum albumin concentration using Raman spectroscopy setup, Opt. Quantum Electron., 2016, 48(6), 337, DOI:10.1007/s11082-016-0610-2
.
- K. W. C. Poon, F. M. Lyng, P. Knief, O. Howe, A. D. Meade and J. F. Curtin,
et al., Quantitative reagent-free detection of fibrinogen levels in human blood plasma using Raman spectroscopy, Analyst, 2012, 137(8), 1807 RSC
.
- N. A. Brazhe, A. B. Evlyukhin, E. A. Goodilin, A. A. Semenova, S. M. Novikov and S. I. Bozhevolnyi,
et al., Probing cytochrome c in living mitochondria with surface-enhanced Raman spectroscopy, Sci. Rep., 2015, 5, 1–13, DOI:10.1038/srep13793
.
- Z. Zhang, B. Wang, Y. Yin and Y. Mo, Surface-enhanced Raman spectroscopy of Vitamin B12 on silver particles in colloid and in atmosphere, J. Mol. Struct., 2009, 927(1–3), 88–90, DOI:10.1016/j.molstruc.2009.02.019
.
- C. A. Lieber and A. Mahadevan-Jansen, Automated Method for Subtraction of Fluorescence from Biological Raman Spectra, Appl. Spectrosc., 2003, 57(11), 1363–1367, DOI:10.1366/000370203322554518
.
- F. Bonnier, A. Mehmood, P. Knief, A. D. Meade, W. Hornebeck and H. Lambkin,
et al., In vitro analysis of immersed human tissues by Raman microspectroscopy, J. Raman Spectrosc., 2011, 42(5), 888–896 CrossRef CAS
.
- B. Hoang, M. J. Ernsting, A. Roy, M. Murakami, E. Undzys and S. D. Li, Docetaxel-carboxymethylcellulose nanoparticles target cells via a SPARC and albumin dependent mechanism, Biomaterials, 2015, 59, 66–76, DOI:10.1016/j.biomaterials.2015.04.032
.
- I. J. Mackie, S. Kitchen, S. J. Machin and G. D. O. Lowe, Guidelines on fibrinogen assays, Br. J. Haematol., 2003, 121(3), 396–404 CrossRef PubMed
.
- G. D. Lowe and A. Rumley, Use of fibrinogen and fibrin D-dimer in prediction of arterial thrombotic events, Thromb. Haemostasis, 1999, 82(2), 667–672 CAS
.
- S. Akman, I. Kurt, M. Gultepe, I. Dibirdik, C. Kilinc and T. Kutluay,
et al., The development and validation of a competitive, microtiter plate enzymeimmunoassay for human albumin in urine, J. Immunoassay, 1995, 16(3), 279–296 CrossRef CAS PubMed
.
-
T. Peters, All About Albumin: Biochemistry, Genetics, and Medical Applications, Elsevier Science, 1995. Available from: https://books.google.ie/books?id=i1DC3KlTAB8C Search PubMed
.
- Thermoscientific. Human Albumin (ALB) ELISA Kit, 2017, (April), pp. 1–8.
- K. Zhang, C. Song, Q. Li, Y. Li, Y. Sun and K. Yang,
et al., The establishment of a highly sensitive ELISA for detecting bovine serum albumin (BSA) based on a specific pair of monoclonal antibodies (mAb) and its application in vaccine quality control, Hum. Vaccines, 2010, 6(8), 652–658 CrossRef CAS
.
- F. Bonnier, H. Blasco, C. Wasselet, G. Brachet, R. Respaud and L. F. C. S. Carvalho,
et al., Ultra-filtration of human serum for improved quantitative analysis of low molecular weight biomarkers using ATR-IR spectroscopy, Analyst, 2017, 142(8), 1285–1298 RSC
. F. Bonnier, et al., 2017. Ultra–filtratio. Available from: http://xlink.rsc.org/?DOI=C6AN01888B.
- W. Miesbach, J. Schenk, S. Alesci and E. Lindhoff-Last, Comparison of the fibrinogen Clauss assay and the fibrinogen PT derived method in patients with dysfibrinogenemia, Thromb. Res., 2010, 126(6), e428–e433, DOI:10.1016/j.thromres.2010.09.004
.
Footnote |
† Electronic supplementary information (ESI) available. See DOI: 10.1039/c8an01701h |
|
This journal is © The Royal Society of Chemistry 2018 |
Click here to see how this site uses Cookies. View our privacy policy here.