DOI:
10.1039/D5MH00236B
(Review Article)
Mater. Horiz., 2025, Advance Article
Empowering artificial muscles with intelligence: recent advancements in materials, designs, and manufacturing
Received
7th February 2025
, Accepted 4th April 2025
First published on 7th April 2025
Abstract
Drawing on foundational knowledge of the structure and function of biological muscles, artificial muscles have made remarkable strides over the past decade, achieving performance levels comparable to those of their natural counterparts. However, they still fall short in their lack of inherent intelligence to autonomously adapt to complex and dynamic environments. Consequently, the next frontier for artificial muscles lies in endowing them with advanced intelligence. Herein, recent works aimed at augmenting intelligence in artificial muscles are summarized, focusing on advancements in functional materials, structural designs, and manufacturing techniques. This review emphasizes memory-based intelligence, enabling artificial muscles to execute a range of pre-programmed movements and refresh stored actuation states in response to changing conditions, as well as sensory-based intelligence, which allows them to perceive and respond to environmental changes through sensory feedback. Furthermore, recent applications benefiting from intelligent artificial muscles, including adaptable robotics, biomedical devices, and wearables, are discussed. Finally, we address the remaining challenges in scalability, dynamic reprogramming, and the integration of multi-functional capabilities and discuss future perspectives of augmented intelligent artificial muscles to support further advancements in the field.
Wider impact
This review highlights recent advancements in artificial muscles, focusing on integrating intelligence for adaptive, multifunctional behavior. It explores programming methodologies, from single-mode to multi-mode actuation, and reprogramming capabilities for entirely new states. Additionally, research outcomes in self-sensing and neuromorphic feedback systems that enable artificial muscles to perceive and respond to conditions are summarized. This field bridges materials science, bioengineering, and robotics, driving advancements in healthcare, robotics, and wearable technologies. Future efforts will focus on materials with enhanced programmability, energy efficiency, and functional integration. This review identifies critical challenges and opportunities guiding the development of high-performance artificial muscles for transformative applications.
|
Introduction
The wisdom of nature has long served as an invaluable guide across a broad range of disciplines, inspiring advancements in bio-inspired material synthesis,1–4 structural design,5–8 mechanistic principles,9–11 and energy-efficient functionalities.12–14 These natural inspirations provide reference points for most technologies, offering solutions and blueprints to emulate. In particular, the intricate and elegant evolution of nature has inspired us to incorporate flexibility,15–17 physical compliance,18–21 dexterity,22–24 and optimized design in many applications.25–28 Consequently, biomimicry has shown significant utility across diverse fields—including sensors,29–33 actuators,34–38 energy systems,39–41 structural engineering,42–44 and environmental sustainability45–48—demonstrating nature's power to inspire practical, high-performance solutions. Specifically, when it comes to designing force-generating and locomotion-driving systems, researchers have actively drawn inspiration from the structure, design, and activation of biological motor units.49–51 This biological framework has thus become instrumental in guiding the development of artificial muscles, which serve as fundamental components in replicating the capacities of natural motors.
Early research focused on understanding and mimicking the structure and function of skeletal muscle, which is composed of muscle fibers (myofibers) organized into bundles and connected by myofibrils.52 Each myofiber contains sarcomeres, the basic contractile units, which operate through the sliding filament theory.53 According to this principle, actin and myosin filaments within sarcomeres slide past each other in response to stimuli, primarily electrical signals from motor neurons, leading to muscle contraction and relaxation.54 Inspired by these biological principles, early developments in artificial muscles emulated muscle structure and function by embedding responsive, functional materials into soft, flexible matrices. These materials respond to various stimuli, such as electric fields, magnetic fields, light, and thermal changes, allowing artificial muscles to generate force and undergo contraction and expansion, similar to biological muscles. Another approach involved creating artificial muscles using responsive materials in distinct structural forms—such as films,55–57 tubes,58,59 wires,60,61 and coils62,63—to mimic biological muscle contraction and force generation. As a result, various types of artificial muscles have been developed based on pneumatic/hydraulic systems,64–68 magneto-responsive materials,69–73 tendon-driven wires,74–77 electroactive elastomers,78–82 hydrogels,83–85 shape memory polymers,86–90 and shape memory alloys.91–95 Each of these artificial muscles has unique advantages and limitations, which have been systematically reviewed in numerous studies, providing a foundation for ongoing innovations in artificial muscle technology.
The field of artificial muscles is now advancing toward diversifying and enhancing their functional capabilities. This progress goes beyond simple contraction and relaxation, striving to mimic the advanced properties and performance of biological muscles. As illustrated in Fig. 1, these developments enable artificial muscles to achieve complex motions that conventional artificial muscles show difficulty in replicating. For example, by designing artificial muscles with multi-directional alignments, akin to shoulder muscles in the human body, simultaneous contraction, extension, and rotational movements can be implemented, resulting in multi-actuation modes. Moreover, artificial muscles can be reprogrammed for entirely new roles, moving beyond their originally intended applications. This reprogramming is analogous to modifying biological tissues through stem cell cultivation to repurpose skeletal muscles for organ functionality. Similarly, artificial muscles could be adapted with high degrees of freedom to perform diverse functions, significantly enhancing their versatility. Additionally, as biological muscles utilize neuromorphic configurations to facilitate rapid cognition and action, advanced artificial muscles are being developed with self-sensing capabilities to detect and respond swiftly and appropriately to changing environments. These advancements position artificial muscles as key enablers of intelligent, adaptive systems capable of mimicking and surpassing the functional complexity of their biological counterparts. However, while the principles, performance, and optimization of artificial muscles have been extensively reviewed in previous studies, there remains a gap in the literature summarizing the types of intelligence that could be embedded within artificial muscles and enhance their responsiveness and adaptability.
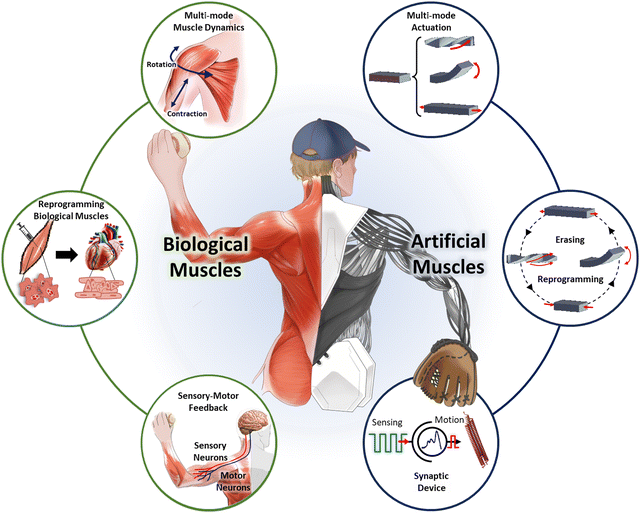 |
| Fig. 1 Comparison between biological muscles and intelligent artificial muscles, highlighting multi-mode dynamics, reprogrammability, and integrated sensory feedback. | |
This review aims to provide a comprehensive overview of how intelligence is imbued into artificial muscles, enabling them to respond actively to complex and diverse environments. It begins by examining how conventional artificial muscles are programmed to actuate, focusing on the diversity of materials, mechanisms, and fabrication processes used to encode and retain motion patterns. Then, the review systematically explores advanced methodologies, materials, and synthesis processes developed to endow artificial muscles with enhanced intelligence, enabling them to store not only single motion types but also various behavioral characteristics. This includes approaches allowing artificial muscles to adapt by reprogramming new memories in response to unforeseen changes. In addition to exploring intelligence through memory-based behavioral characteristics, it also covers research on self-sensing capabilities, providing insights into the ways artificial muscles can be self-aware and autonomous. The review further examines the current state of applications in fields such as intelligent robots, biomedical robotics, and wearable robotics, with a focus on the principles and mechanisms of how artificial muscles fulfill complex, task-agnostic roles through embedded intelligence in each field. Finally, the review proposes key aspects and research directions essential for advancing the intelligence of artificial muscles, outlining pathways for further enhancing their capabilities to meet future demands.
Intelligence imbued by programming of artificial muscles
Single programming-single actuation mode (SP-SAM)
In the initial stages of artificial muscle development, single programming-single actuation mode (SP-SAM) designs focused on creating muscles that respond to specific stimuli with a single, defined actuation mode, such as linear (tensile, contractile) movement, bending, rotation, or twisting. Each muscle is programmed to perform a distinct motion in response to targeted stimuli, ensuring consistent actuation in its programmed mode. SP-SAM programming typically relies on materials and structures that react predictably to specific stimuli, achieved by directly using responsive materials, embedding stimuli-reactive elements into flexible matrices, or designing tunable structures that deform under controlled conditions. These materials and structures respond to various stimuli, including air pressure, electric fields, light, chemical, magnetic fields, and thermal changes (Fig. 2). The development of SP-SAM designs has provided essential building blocks for understanding and controlling artificial muscle responses, forming the foundation for more complex actuation modes in advanced artificial muscles. However, as the environments in which artificial muscles are used and the situations they need to respond to have become increasingly complex, SP-SAM artificial muscles programmed with a single actuation mode have shown limitations in adaptability and compliance. To address the increasing diversity of requirements, research into intelligent artificial muscles has evolved to enable a single artificial muscle to implement multiple actuation modes.
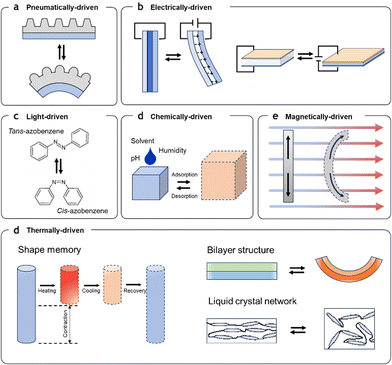 |
| Fig. 2 Schematic illustration of artificial muscles with different actuation mechanisms. Single programming-single actuation mode artificial muscles are designed with pre-memorized states based on the following principles: (a) pneumatically-driven, (b) electrically-driven, (c) light-driven, (d) chemically-driven, (e) magnetically-driven, and (f) thermally-driven mechanisms. | |
Single programming-multiple actuation mode (SP-MAM)
Single programming-multiple actuation mode (SP-MAM) in artificial muscles involves programming a single artificial muscle to remember distinct states, enabling it to exhibit multiple actuation modes in response to external stimuli (Fig. 3(a)). This allows a single artificial muscle to perform a variety of movements—such as tensile/contraction, bending, twisting, and folding—based on the pre-memorized states. Realizing SP-MAM reduces the need for the use of multiple actuators by allowing a single muscle to perform complex and varied tasks, making it particularly advantageous for applications where space and weight constraints are critical.
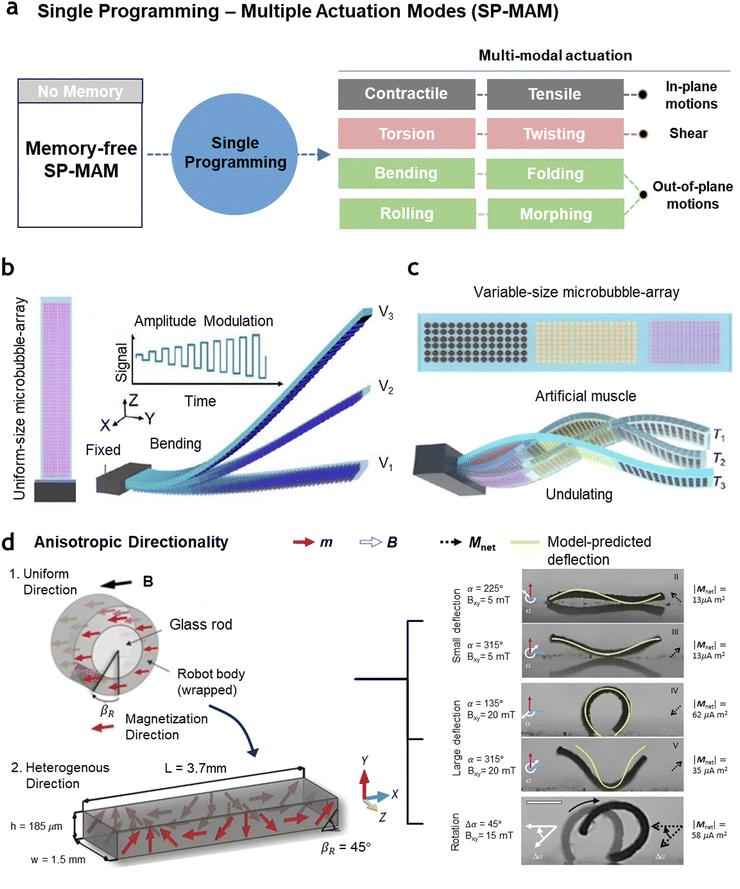 |
| Fig. 3 Artificial muscles with single programming-multiple actuation modes. (a) Conceptual diagram of SP-MAM artificial muscles showing their ability to be programmed with multiple states and exhibit multiple actuation modes. (b) Ultrasound-actuated microbubble-array artificial muscle with a uniform-size array consisting of tens of thousands of microbubbles. Under varying ultrasound frequencies, the muscle can only demonstrate bending deformation with different bending angles. (c) Ultrasound-actuated microbubble-array artificial muscle with a variable-size microbubble-array featuring different microbubble diameters, each corresponding to distinct natural frequencies. The muscle displays multi-mode deformation under varying frequencies. (b) and (c) Reproduced under the terms of the Creative Commons CC-BY-NC-ND license.96 (d) Rectangular-sheet-shaped magnetically responsive artificial muscle created by wrapping the sheet around a cylindrical rod, magnetizing it uniformly in one direction, and subsequently unwrapping it to form a spatially heterogeneous magnetization profile m. The muscle exhibits multi-mode actuation under varying magnetic field directions and magnitudes. Reproduced with permission from Macmillan Publishers Limited, part of Springer Nature, Copyright 2018.97 | |
Multi-mode actuation via anisotropic design. One approach to implementing multiple actuation modes is by dividing subdomains within the artificial muscle, enabling distinct responses to the same stimulus in different areas. For example, artificial muscles having magnetic powders embedded in a polymer matrix and magnetized in different directions perform not only simple 1D tensile/contractile movements but also 3D actuation modes.98–100 Additionally, the anisotropic crystallization of shape-memory polymers and electroactive polymers follows a similar mechanism.101–103 However, these approaches require spatially differentiated memorization, and if a new actuation mode is needed, the internal alignment must be reconfigured to shift the muscle from its previously memorized shape in response to a single type of external stimulus. In contrast, Shi et al. proposed a synthetic muscle that includes a thin and flexible beam containing over 80
000 microcavities arranged in discrete arrays, each designed to confine microbubbles of various sizes.96 As shown in Fig. 3(b), in the case of a uniform-size distribution, the muscle demonstrates a single bending mode, where the degree of bending varies depending on the input level. However, as illustrated in Fig. 3(c), when a variable-size microbubble array is incorporated within a single film, sweep-frequency ultrasound excitation results in multi-mode deformation. This muscle, measuring 3 cm in length, 0.5 cm in width, and 80 μm in thickness, incorporates three distinct arrays of microbubbles with dimensions of 12 μm × 50 μm, 16 μm × 50 μm, and 66 μm × 50 μm. When stimulated at its natural frequency, the 12 μm × 50 μm microbubble array, covering an area of 0.5 cm2, induced a downward deformation in the corresponding region of the muscle. By applying a sweeping-frequency ultrasound excitation from 30 to 90 kHz, an undulatory sinusoidal deformation pattern was generated, creating a continuous, time-dependent motion driven by the periodic reverse thrust across different regions of the muscle. Analogous to creating multiple domains with varying arrays, seamless mechanical integration of multi-material components can also be used to form structural anisotropy and enable multi-mode actuation. For example, copolymerization of stimuli-responsive polymer networks can connect two or more types of materials that exhibit distinct behaviors or activate under different conditions, thereby enabling localized, stimulus-dependent responses.104,105 Zhang et al. proposed a seamless, multi-material 3D liquid crystal elastomer (LCE) by welding and aligning LCE materials with different compositions and physical properties.106 Mechanical connections were achieved by polymerizing LCE films containing reactive acrylate groups. By simply overlapping and pressing the films, followed by thermal polymerization, the acrylate-rich LCE films were welded without the need for adhesives. This method enabled the creation of a multi-material LCE with three distinct parts, each having a different transition temperature for multi-stage reversible actuation. Additionally, by tuning polydopamine doping within the LCE, the artificial muscle exhibited two different actuation modes within a single unit.When creating anisotropic domains by dividing regions within a single structure or by physically combining materials, the resulting actuation mode and performance highly depend on the degree of separation, the precision with which regions are differentiated, and the ability to introduce diversity by creating varied patterns within a limited area.107 Additionally, since different properties are integrated within the same material, it is crucial to ensure homogeneous bonding of the components, as well as to consider the stability of the combined structure and its ability to maintain that stability over time.108 To bypass these complexities, alternative approaches involve using materials that are initially uniform but transform into heterogeneous alignments.109 Hu et al. proposed a straightforward method to create a sheet of silicone elastomer embedded with hard-magnetic microparticles in a spatially heterogeneous manner (Fig. 3(d)).97 First, the sheet, containing the magnetic microparticles, is wrapped around a cylindrical rod and magnetized along a uniform direction. Once unwrapped, this process generates a spatially heterogeneous magnetic alignment within the elastomer sheet. The resulting magnetic profile on the soft material creates regions with differing magnetic orientations, allowing for complex deformations when subjected to external magnetic fields. By applying a time-varying magnetic field and magnetic torque, the artificial muscle can dynamically alter its shape, which enables various soft-bodied locomotion modes. The shape of the robot responds to field strength: low-magnitude fields induce a sine or cosine shape, while higher fields produce U- or V-shaped deflections. This versatile design supports multiple locomotion modes, including swimming, meniscus climbing, landing, immersion, rolling, walking, crawling, and jumping.
Multi-mode actuation via multi-directional alignment. In biological systems, skeletal muscles exhibit multi-directional alignment of muscle fibers, allowing them to produce a wide range of forces and displacements depending on the direction of the structural alignment.110 The unique architectural design of muscle fascicles, combined with intramuscular connective tissues, contributes to the muscles’ capacity for exerting force in diverse directions, enhancing both flexibility and functional adaptability. For instance, the multi-level alignment within skeletal muscle structures, such as pennate and fusiform architectures, provides an optimal blend of strength and range of motion, essential for performing complex, multi-directional tasks.49 Another clear example of multi-directional force generation in humans is the first dorsal interosseous muscle, which achieves this through systematic recruitment of motor units.111 This muscle can contract in different directions, such as abduction and flexion, with distinct recruitment patterns that reflect its adaptability to a variety of tasks. Likewise, convergent muscles, like the pectoralis major, further illustrate this adaptability. Although categorized as parallel muscles, the fibers of convergent muscles spread across a broad area but converge at a common attachment point.112 This design allows a single pull on the tendon to create tension in multiple directions, contributing to the muscle's versatility. In larger organisms, such as elephants, natural tubular actuators like elephant trunks display intricate 3D fibrous architectures.113 As shown in Fig. 4(a)–(c), elephant trunks consist of closely packed, directionally aligned muscle fibers arranged around the trunk's long axis, forming a tubular “muscle hydrostat.” Multiple muscle layers with varied orientations contribute to this 3D structure, enabling a wide range of controlled shape transformations, from simple motions like shortening, elongation, bending, and twisting, to complex compound movements that combine multiple deformations.
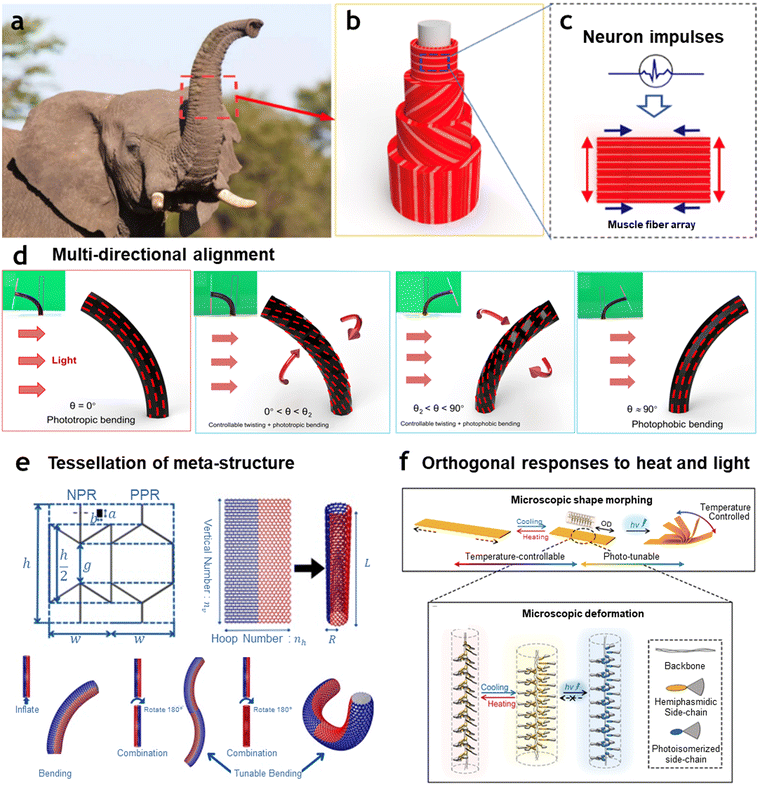 |
| Fig. 4 Artificial muscles with single programming-multiple actuation modes achieved through multi-directional alignment and multi-material conjugation. (a) 3D fibrous architecture inspired by the trunks of elephants and muscular hydrostats, which achieve flexible movement without bones or joints. Photo credit: elephant by Villiers Steyn via Shutterstock. (b) Bioinspired tubular artificial muscle composed of directionally aligned microfiber arrays. (c) Mechanism of actuation showing how each layer of the microfiber array deforms upon excitation. (d) Demonstration of different morphing modes exhibited by helical artificial fibrous muscle-structured tubular soft actuators. (a)–(d) Reproduced under the terms of the Creative Commons CC BY 4.0 license.114 (e) Artificial muscles inspired by auxetic metamaterials designed with repeating negative Poisson's ratio unit cells, enabling programmable bending deformations. Reprinted with permission from Elsevier, copyright 2024.115 (f) Hemiphasmidic side-chain liquid crystal polymer-based artificial muscle showing orthogonal responses to thermal and photo stimuli. Reproduced with permission from WILEY-VCH GmbH, Copyright 2023.116 | |
Similar to the fibrous alignment seen in muscle tissues, several approaches have been developed to introduce alignment in artificial muscles.117 One such method is polymer matrix crystalline multi-alignment, where the crystalline structure within polymer matrices is oriented to achieve directional mechanical properties.118 In particular, research conducted by Hu et al. demonstrated how fiber-reinforced soft tubular structures with helically aligned polymer matrices exhibited sophisticated deformations with well-defined degrees of freedom.114 The authors showed that precise control of fiber winding angles within a liquid crystal elastomer matrix, defined by critical angles θ1 and θ2, enabled the muscle to morph into distinct shapes depending on the fiber alignment angle. For instance, a 0° angle results in simple axial shortening and radial expansion, while angles between θ1 and θ2 produce combined twisting and contraction modes. Here, the alignment was achieved through a two-stage fabrication process: first, mechanical stretching aligned the liquid crystal mesogens along the fiber axis; second, the winding angle controlled the overall 3D orientation and anisotropy. This configuration allowed for diverse shape transformations by adjusting the winding angle θ, resulting in seven morphing modes under symmetrical stimuli and four morphing modes under asymmetrical stimuli, yielding a total of 11 distinct actuation modes. Among these, the seven morphing modes exhibit in-plane deformations (axial or radial), similar to those observed in conventional tubular-type artificial muscles. Notably, they incorporate cases where the Poisson's ratio (ν) is both greater than and less than zero. Particularly in Fig. 4(d), unlike conventional tubular-type artificial muscles that exhibit only unidirectional axial or radial motion, this system demonstrates four different modes of directional bending, enabling multi-mode actuation with 3D deformation.
Another approach is, inspired by the sarcomere structure in biological muscles, arranging motor unit arrays with directions. For example, Xie et al. proposed the “ExoMuscle”, which mimics the directional alignment of sarcomeres in skeletal muscle by contracting “myofilaments” to generate multi-directional force.119 This bio-inspired structure allows the artificial muscle to emulate various muscle architectures, including parallel, fusiform, convergent, and pennate arrangements. The authors demonstrated that two ExoMuscles aligned at different angles and converging on the arm could assist in shoulder flexion and abduction—movements requiring force generation across multiple axes. In addition, Connolly et al. proposed a design strategy that incorporates complex kinematic trajectories by analytically modeling nonlinear elasticity and optimizing fiber-oriented design parameters.120 The authors first established a relationship between fiber orientation and each resultant motion type, and then developed an analytical model to determine optimal segment lengths and fiber angles for replicating complex motions. Furthermore, fabric-making techniques—such as weaving, braiding, knitting, knotting, and coiling—are fundamentally based on the concept of fiber alignment and offer an intuitive way to arrange fibers in specific patterns. Through these techniques, fibers are intricately aligned, with simulated and experimentally confirmed responses that depend on the precise orientation and arrangement of fibers within the artificial muscle.
Recently, in the pursuit of implementing multi-mode actuation through structural and arrangement design, research has advanced toward using meta-structures or metamaterials to enhance the versatility of artificial muscles.121–123 Metamaterials—engineered materials with properties not found in nature, typically achieved through unique structural designs—enable the controlled actuation modes of artificial muscles by leveraging these structural characteristics.124 Additionally, metamaterials can be seamlessly fabricated through tessellation of unit structures, allowing for the integration of different meta-structures with distinct properties into a single assembly.125 This approach uses the structural differences induced by external stimuli to produce varying actuation modes within a single framework. As illustrated in Fig. 4(e), the asymmetric deformation resulting from the tessellation of metamaterials with diverse design parameters enables complex, programmable bending behaviors that are highly dependent on both the design parameters and the positioning of each unit.115 Similarly, Khan et al. utilized the unique properties of metamaterials to enable orthogonal actuation along two perpendicular axes.126 By knotting shape memory alloy wires into an auxetic unit with orthogonal characteristics, the authors demonstrated that the resulting artificial muscle could independently contract and expand along both the y- and x-axes. This structural separation enables a high degree of decoupling between actuation modes, allowing for complex bending transformations with multi-angled deformations or enabling actuation in specific directions. Such designs enhance the versatility and control of artificial muscles, making them well-suited for applications requiring intricate, direction-specific movements.
Multi-mode actuation via conjugation of multi-materials
By integrating materials that respond differently to multiple stimuli, a single artificial muscle can exhibit orthogonal responses to two or more distinct external inputs.127 For instance, a stimuli-responsive phase-changing polymer matrix combined with magnetic microparticles enables the artificial muscle to respond independently to heat and magnetic fields.128,129 In this configuration, mode alteration involves a sequential process: first, the phase-changing matrix adjusts its stiffness upon heating, effectively locking its mechanical state, followed by motion or force generation triggered by an external magnetic field. This design harnesses the benefits of the phase-changing matrix in tuning mechanical properties significantly, while leveraging the rapid response of magnetic actuation for swift transformation. Furthermore, when magnetic particles are embedded, the system also offers wireless heating capabilities through inductive heating, whereby an alternating external magnetic field generates heat within the structure.128 However, there remains room for improvement, as these types of multi-mode actuation tend to operate sequentially rather than simultaneously—requiring the stiffness change in the polymer matrix before motion or force can be generated by magnetic input. This stepwise process limits the immediate co-activation of responses to different stimuli, presenting a potential area for future optimization in simultaneous, multi-stimuli actuation. Recently, to achieve simultaneous yet differentiated responses to different stimuli, Yang et al. proposed a temperature-controllable and photo-tunable liquid crystal polymer that exhibits distinct behaviors in response to temperature and light stimuli within a single composite.116 By combining azobenzene-based photo-responsive molecules with a liquid crystal polymer backbone, this system allows for dual functionality: the azobenzene groups undergo trans-to-cis photoisomerization in response to light, inducing bending through photo-stimulation, while temperature changes cause phase transitions within the liquid crystal structure, leading to contraction or expansion of the material (Fig. 4(f)). By combining dual actuation modes, the authors successfully demonstrated two distinct conditions with a single material: bulk deformation through heat stimulation and precise, directional adjustments through light stimulation. As summarized in Table 1, significant efforts have been made to enhance the degrees of freedom in intelligent artificial muscles. Broadly, two primary approaches have been employed: structural design strategies and multi–stimuli responsive materials. These approaches have enabled multi-mode actuation, expanding the behavioral diversity of artificial muscles and allowing them to adapt more effectively to varied environments in practical applications. Structural design-based strategies leverage material alignments, multi-domain patterning, and composite integrations to achieve diverse actuation modes. For instance, the use of magnetically programmable composites, pneumatic fiber alignments, and photothermal programming of hydrogels has enabled various forms of shape morphing, contraction, bending, twisting, and even complex out-of-plane deformations. These allow artificial muscles to exhibit tunable and dynamic responses that enhance their adaptability in real-world applications. On the other hand, multi–stimuli responsive materials integrate external stimuli such as light, humidity, thermal, electrical, and magnetic fields to enable more versatile actuation. These materials allow artificial muscles to undergo sequential reconfiguration, multi-temperature shape memory programming, and dynamic stiffness tuning, further increasing their functional diversity. Despite these advancements, there remain inherent limitations in the degree of freedom achievable through pre-programmed states. While these strategies have proven effective in expanding actuation capabilities, they still struggle with real-time adaptability to unanticipated external conditions.
Table 1 Summary of single programming-multiple actuation mode artificial muscles
Source of multi-mode actuation |
Materials |
Programming method |
Actuation stimulus |
Actuation response |
Key features for multi-mode actuation |
Ref. |
Structural design |
Liquid crystal elastomer |
Thermal + multi-domain placement |
Thermal |
Contraction, bending, twisting |
Segmented actuation domains |
130 |
Local heating of LCE tube |
Electro-thermal |
Multi-directional bending |
Segmented by local UV irradiation and local heating |
131 |
Magnetic composite |
Magnetization with multi-domain |
Magnetic |
Shape-morphing |
Magnetization of multi-domain |
99 |
Silicone |
Multilamellar fiber alignment |
Pneumatic |
Contraction, bending, twisting |
Multiple alignment designs |
118 |
Locally aligned tensile jamming fibers |
Pneumatic |
Contraction, bending, shape-morphing |
Tunable local jamming and inflation |
132 |
Hydrogel |
Mechanical shear-induced alignment with photopolymerization |
Photothermal |
Shape-morphing, bending, rotation, rolling |
Control of symmetry and asymmetry |
133 |
Two-photon polymerization process |
Thermal |
Shape-morphing |
Pixel-wise varying laser power and scanning speed |
134 |
Multi-stimuli responsive materials |
Hybrid polymers |
Light, humid, thermal |
Light, Humid, Thermal |
Folding, bending, shape-morphing |
Requires sequential process for reconfiguration |
135 |
Physical Patterning |
Light, Humid |
Shape-morphing |
Tunable actuation mode via oriental printing |
136 |
Magnetization |
Magnetic, Thermal, Light |
Extension, contraction, bending, twisting |
Stiffness tunable (thermal), dynamic actuation (magnetic) |
128 |
Thermal + mechanical |
Electrical, Thermal |
Bending, stretching |
Multi-temperature shape memory programming |
137 |
Multiple programming-multiple actuation mode (MP-MAM)
When an artificial muscle encounters an unexpected situation that requires a different actuation mode, the most intuitive approach is to erase the pre-memorized state and reprogramming a new one to fit the situation—a concept known as “erasing and reprogramming”. Unlike traditional artificial muscles with a single pre-set actuation, erasing and reprogramming would allow for autonomous adaptation and reduce the need for predictive algorithms. The core principle involves a memory reset that returns the muscle to its initial state, enabling it to acquire new actuation modes. As shown in Fig. 5(a), an artificial muscle can be programmed to retain state 1 with a bending motion. After the task, the memory is erased, and the muscle is reprogrammed with state 2 to exhibit a twisting motion. This cycle of erasing and re-learning provides versatility, allowing artificial muscles to expand their functional range beyond static actuation patterns. This transformable adaptability is crucial in applications requiring continuous modulation, such as robotics in unpredictable environments or wearable devices responding to users’ evolving needs. Just as programming artificial muscles, erasing processes can also be initiated through external stimuli.88,138 Photo-initiated erasure offers the unique advantages of both speed and selectivity. This approach utilizes the photo-responsive properties of polymers, which adjust their molecular shape and structural configuration in response to specific light wavelengths. As illustrated in Fig. 5(b), Lahikainen et al.139 used azobenzene groups that respond to distinct wavelengths of light: UV light (365 nm) for programming, blue light (455 nm) for erasure, and red light (625 nm) for actuation. In this process, the azobenzene molecules toggle between trans (a linear, extended form) and cis (a bent, shorter form) configurations in response to light, enabling the muscle to store or erase particular actuation states. Additionally, as shown in Fig. 5(c), specific regions of the artificial muscle are selectively exposed to UV light, altering actuation characteristics in targeted areas to increase the diversity of actuation modes. This allowed a single artificial muscle to be programmed into a variety of distinct shape morphing, followed by an erasure process using blue light to reset the material to its unprogrammed state. This cycle can be repeated, allowing for continuous adaptation to new configurations. Notably, the trans-to-cis and cis-to-trans transitions occur rapidly, completing the reset within approximately 10 seconds. Erasing and reprogramming the magnetic alignment is achieved by applying a changing magnetic field while simultaneously softening the polymer matrix that holds the magnetic particles in place. For example, in a composite material made from a thermoplastic polymer and magnetic powder (e.g., NdFeB), the polymer matrix fixes or releases the magnetic alignment based on its temperature-altered molecular state. Deng et al.140 utilized laser-assisted heating to locally soften the polycaprolactone (PCL) matrix, a thermoplastic polymer, and reprogramming of the magnetic anisotropy in soft composites (Fig. 5(d)). This allows rapid, localized adjustments, and the artificial muscle can be programmed to execute complex 3D movements, such as bending, twisting, and folding.
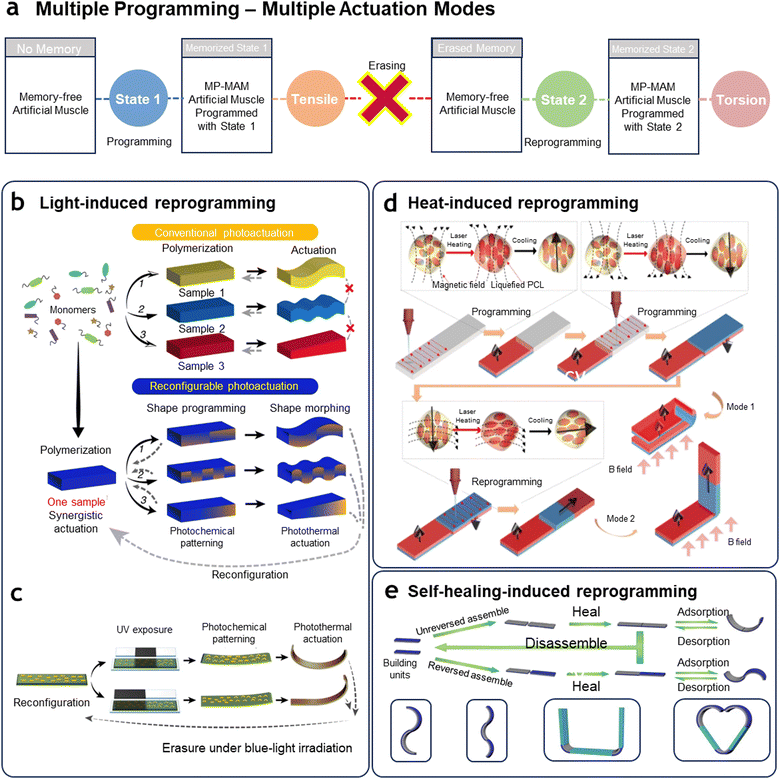 |
| Fig. 5 Artificial muscles with multiple programming-multiple actuation modes (MP-MAM). (a) Conceptual diagram of MP-MAM artificial muscles showing their ability to be programmed, erased, and reprogrammed into entirely different states, enabling multiple actuation modes. (b) Comparison of conventional stimuli-responsive actuators that require distinct programming processes during fabrication for each actuation mode, versus a reconfigurable and reprogrammable artificial muscle capable of multiple shape changes from a single sample through programming, erasing, and reprogramming cycles. (c) Reconfiguration strategy using photochemical and photothermal materials, where pre-memorized states are erased under blue-light irradiation, enabling new configurations. (b) and (c) Reproduced under the terms of the Creative Commons CC BY 4.0 license.139 (d) Reprogramming magnetic anisotropy in magnetically responsive soft materials via direct laser writing. Localized laser heating melts the polycaprolactone encapsulating NdFeB microparticles, allowing the magnetic particles to realign in the direction of the applied programming magnetic field. Reproduced under the terms of the Creative Commons CC BY 4.0 license.140 (e) Macroscopically discretionary healing-assembly strategy for reconfigurable artificial muscles. Mechanical assembly and disassembly of building units allow diverse morphing modes, such as “S,” “wave,” “U,” and “heart” shapes, which are achieved through solvent-induced self-healing. Reproduced with permission from WILEY-VCH GmbH, Copyright 2021.141 | |
Mechanical re-assembly of building blocks can serve as a method for erasing and reprogramming artificial muscles. Here, unit blocks are physically separated and recombined through mechanical assembly, much like configuring with toy bricks. To facilitate this, the composite materials are designed with polymers that possess both actuation capabilities and self-recovery properties. These polymers allow the unit blocks to be repeatedly assembled, disassembled, and reassembled into new configurations, facilitating versatile actuation modes and adaptability in response to various tasks. Polymers with self-recovery properties are often held together through reversible bonding mechanisms, which allow for mechanical reconnection.142 These polymers can be combined with stimuli-responsive polymers to form a double-layer composite, enabling the development of self-healing, reprogrammable artificial muscles.143 Alternatively, building blocks can be composed entirely of a single type of polymer that both actuates in response to stimuli and possesses self-healing properties. Lou et al.141 demonstrated this approach using a macroscopically discretionary healing-assembly strategy to fabricate reprogrammable artificial muscles based on an intrinsically self-healing poly(dimethylglyoxime-urethane) (PDOU) elastomer (Fig. 5(e)). The PDOU elastomers achieved self-healing due to the dynamic dimethylglyoxime-urethane (DOU) bonds, which enabled reconfiguration upon damage. The material's response to solvent (chloroform) stimuli varied with different crosslinking densities, allowing tailored actuation characteristics. The combination of dynamic DOU bonds and reversible hydrogen bonds (H-bonds) in PDOU allowed building units with diverse crosslinking levels and shapes to heal and assemble seamlessly, forming flexible actuators capable of varied actuation responses. Through this modular approach, the units could be separated and reassembled to exhibit multiple actuation modes and morph into desired shapes by simply tailoring and reassembling—without requiring any external stimuli. Table 2 summarizes the research on reprogrammable artificial muscles, those capable of completely erasing their initially memorized state and reprogramming into a different state. These studies encompass a variety of actuation mechanisms, with reprogramming times ranging from a few seconds to several hours, depending on the specific approach used. The capability for reprogramming also allows for actuation responses to exceed the initially defined modes, demonstrating the versatility of these systems. Despite the advancements in reprogrammable artificial muscles, several challenges remain. Most of the current research has focused on film-based polymer structures, which limits their ability to generate significant forces. As a result, many studies primarily demonstrate shape morphing capabilities rather than achieving sufficient mechanical output to perform functional tasks. Expanding reprogrammability beyond film-based structures to more bulk or fiber-based architectures could be a crucial step toward developing artificial muscles that can adaptively generate force for practical applications. Additionally, long-term cycle stability remains an unresolved issue. Many reported studies either lack systematic evaluations of repeated reprogramming cycles or demonstrate stability only over a limited number of cycles (often fewer than 10 iterations). For reprogrammable artificial muscles to be viable for real-world applications, achieving stable performance over long cycles will be essential. Future efforts should focus on developing materials and mechanisms that retain their functional integrity through repeated reprogramming and actuation cycles. To further advance the field, exploring new material systems beyond traditional polymers—such as metallic or hybrid composites—could open pathways toward higher-force generation and improved durability.
Table 2 Summary of multiple programming – multiple actuation mode artificial muscles
Materials |
Programming method |
Erasing method |
Reprogramming time |
Actuation stimulus |
Actuation response |
Ref. |
Liquid crystal elastomer |
Photochemical |
Blue-light irradiation |
30 s |
Photothermal |
Reconfigurable shape morphing (>6 geometries) |
139 |
Thermal and mechanical excitation |
Heating at 140 °C |
10 s |
Thermal |
Reconfigurable shapes (>4 geometries) |
144 |
Swelling in solution |
48 hours |
Thermal |
Elongation/contraction, twisting, reconfigurable shapes |
145 |
Mechanical deformation |
Heating at 180 °C |
20 min |
Joule heating |
Deformation along X, Y axis |
146 |
Chemical |
Heating at 140 °C |
2 hours |
Thermal |
Twisting, stretching, reconfigurable shape |
147 |
Magnetic composite |
Heating and magnetization |
Localized laser heating |
— |
Magnetic field |
Reconfigurable shape morphing (>3 geometries) |
140 |
NIR-II triggered photothermal shape locking |
30 s |
Magnetic field |
Stretching, bending, reconfigurable shapes |
148 |
Shape memory polymer |
Chemical |
Heating, mechanical reassembly |
30 min |
NIR light irradiation |
Reconfigurable shapes (>5 geometries) |
143 |
Mechanical assembly |
Mechanical disassembling and reassembling |
4 days |
Solvent adsorption–desorption |
Reconfigurable shape (>4 geometries) |
141 |
Hydrogels |
Electrical |
Left in water |
— |
Electric field |
Reconfigurable shape morphing |
149 |
Meanwhile, erasing and re-programming in artificial muscles can be carried out through either comprehensive full-muscle reprogramming or localized memory refresh. Full-muscle reprogramming provides a uniform response across the muscle, making it ideal for tasks requiring synchronized or large-scale actuation without regional variations. Consequently, it is suitable for general-purpose actuation in situations where uniform motion is advantageous. Chen et al. demonstrated full-muscle reprogramming, enabling the complete transformation of one memorized state into another, as shown in Fig. 6(a).144 The authors developed a dual-phase liquid crystal elastomer, where the crystalline phase acts as an “alignment frame” to lock mechanical deformations via a shape-memory mechanism. This alignment can be erased through melting, providing a starting point for reprogramming. Using this approach, the artificial muscle was initially programmed to perform a flower-like opening and closing motion. Subsequently, by heating the muscle to 140 °C to completely erase its memory, the same artificial muscle was reprogrammed to perform an entirely different motion, mimicking the flapping of an origami crane. On the other hand, localized memory refresh targets specific sections of the muscle for selective reprogramming, allowing for precise control over different regions within the same muscle. Prior to selectively refreshing the memory, studies have focused on achieving locally selective actuation of artificial muscles by exciting specific regions. For example, rather than heating the entire area of shape-memory polymers using conventional thermal methods, local heating was achieved through composites combining photothermal-responsive materials with shape-memory polymers. Wang et al.150 developed a method for direct ink writing to fabricate a 3D-printed structure using a photoresponsive composite ink composed of gold nanorods (AuNR) and liquid crystal elastomers. Upon irradiation of near-infrared (NIR) light at 808 nm and 1.4 W cm−2 (corresponding to approximately 160 °C), the resulting structure exhibited a photothermal response with a 27% actuation strain that could be controlled either globally or locally. Additionally, conductive pathways have been incorporated within shape-memory polymers through printing, enabling localized heating via Joule heating. In particular, in this process, flexible conductive paths are used to ensure that the overall stiffness of the printed conductive network does not affect actuation characteristics or lead to performance degradation. Along with the material used to alter control scheme, structural design can be considered to induce localized actuation. Oh et al.151 addressed the challenges of achieving localized control in artificial muscles made from traditional NiTi shape memory alloys by configuring pairs of NiTi wires knotted together into tessellated auxetic form. Then, by applying a Parylene coating to alter the surface conductivity, each pair of NiTi wires was independently actuated through Joule heating, allowing localized control within a single artificial muscle. With advancements in research enabling localized control by regions, studies are moving forward to implement localized memory refresh. The process of localized reprogramming for selective actuation involves erasing and reprogramming specific regions within artificial muscles, with a focus on fine control over resolution in localized areas to enhance precision in memory refresh. High-resolution, focused light stimulation is often used for targeted activation, enabling advanced, real-time reprogramming methods to modify actuation states accurately and quickly. Recently, Miao et al.148 developed intelligent 4D-printed soft actuators composed of elements that can reversibly switch between transforming and locking states (Fig. 6(b)), acting as physical binary elements (m-bits) encoded as “0” and “1.” These elements enable complex spatial deformations by controlling each state in real-time according to a binary encoding mechanism. Using high-resolution digital light processing-based 4D printing, researchers created intricate 3D structures from a UV-curable magSMP composite composed of shape memory polymer resin and NdFeB magnetic particles. This magSMP composite offers high photothermal conversion efficiency and magnetic responsiveness, allowing precise, remote, and real-time reprogramming of multi-spatial shape deformations and shape locking via the combined use of NIR-II light and a magnetic field. Through digital mask projection with NIR-II light, which triggers localized photothermal conversion of NdFeB particles at a conversion efficiency of 42%, the magSMP composite achieves reversible shape locking and unlocking in designated regions. Following the binary encoding mechanism, the 4D-printed magSMP composite can achieve both global and localized reversible deformations. Under a specific magnetic field, elements in the glassy state without NIR-II light show negligible deformation (code “0”), while NIR-II heated elements reach a rubbery state and undergo significant deformation (code “1”). Using this principle, the authors successfully demonstrated localized memory refresh in a 3D structure with 12 selective sites.
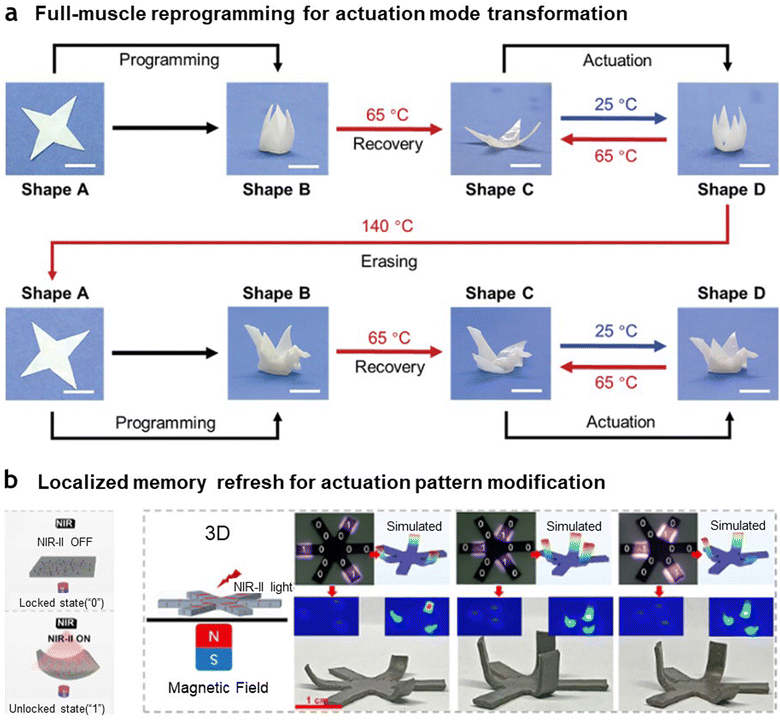 |
| Fig. 6 Full structure versus localized reprogramming in artificial muscles. (a) Full-muscle reprogramming using a dual-phase liquid crystal elastomer (LCE) network. The entire artificial muscle is initially programmed to exhibit a flower-like opening and closing motion. This behavior is fully erased by melting the crystalline phase, and the entire structure is reprogrammed to perform a completely different behavior: a reversible flapping motion resembling an origami crane. Reproduced with permission from WILEY-VCH GmbH, Copyright 2022.144 (b) Localized reprogramming using targeted NIR-II light irradiation. Specific regions of the artificial muscle, composed of a magnetic shape memory polymer (magSMP) composite, are selectively reprogrammed. By applying NIR-II light patterns, deformation states of individual elements are programmed using machine language (e.g., binary code), enabling independent and localized actuation within a single structure. Reproduced with permission from Elsevier, Copyright 2024.148 | |
Despite the significant advantages that erasing and reprogramming methods offer in terms of versatility and adaptability to unforeseen environments, several limitations remain. A key challenge is the need for external systems to control reprogramming stimuli. For instance, magnetic actuation requires a field-control system, while thermal reprogramming needs a heat source, meaning these processes rely on external, often stationary setups. Consequently, achieving in situ reprogramming in mobile or dynamic environments is currently difficult, restricting applications to specific controlled settings. Additionally, fine-tuning the resolution and precision of localized reprogramming remains technically complex, especially for intricate geometries, necessitating further advancements in high-resolution stimulus delivery. Durability and reprogramming speed also present concerns, as repeated cycles can lead to material degradation and increased energy demands. Future research could expand to focus on improving scalability, efficiency, and multi–stimuli integration, reducing dependence on external systems and enabling more adaptable, resilient artificial muscles capable of real-time reprogramming in diverse environments. Addressing these challenges could bring erasing and reprogramming techniques closer to achieving the robust adaptability observed in biological systems. Nevertheless, it is essential to recognize that the ability to move beyond simple memory processes and reprogram artificial muscles to retain new states represents a significant advancement in imbuing artificial muscles with intelligence.
Intelligence imbued by self-sensing capabilities
Imbuing artificial muscles with intelligence involves programming and endowing them with sensing capabilities to detect and interpret environmental cues before reacting.152–154 This closely reflects the structure and function of biological sensory and feedback systems. In biological systems, sensory mechanisms convert external and internal stimuli into neural signals, which are then processed to help understand the surroundings.155,156 For example, as shown in Fig. 7(a), the human somatosensory feedback pathway operates as a closed-loop system, involving sensory receptors, the nervous system, and effectors.157 When a stimulus is detected, the pathway transmits sensory feedback that leads to a coordinated response, enabling precise control of muscle movement in changing environments. Unlike traditional artificial sensory systems that typically rely on microprocessors and external circuits, recent advancements in flexible and neuromorphic electronics have opened up new possibilities for creating artificial perception systems that replicate the closed-loop functionality found in biological feedback mechanisms.158–160 To achieve such biological-inspired closed-loop feedback, an intelligent artificial muscle requires integration with two essential components: sensors and neuromorphic devices. In a recent study, Sun et al.161 proposed an artificial neuromorphic somatosensory system capable of spatio-temporal tactile perception and feedback functions, as shown in Fig. 7(b). This system integrates flexible tactile sensors, multi-gate synaptic transistors, coupling circuits, and artificial muscles to mimic the human somatosensory feedback pathway. The flexible tactile sensor, made of carbon nanotubes/polyimide (CNT/PI) with a pyramidal microstructure, detects pressure based on resistance changes. This information is transformed into a frequency signal by an oscillator and then transmitted to an artificial synapse. The synapse is realized with an In2O3 and sodium alginate (SA) channel and dielectric gate layer, which processes the signal and triggers an ionic polymer–metal composite (IPMC) artificial muscle for feedback actuation when the threshold pressure is reached. The synaptic transistor in the system plays a crucial role in achieving parallel information processing, allowing the device to handle signals from multiple tactile sensors simultaneously. Additionally, because the system mimics synaptic plasticity, it can distinguish between varying pressure intensities and durations, thus enhancing its perceptual accuracy and efficiency. This research demonstrates the potential for artificial somatosensory systems in applications requiring real-time feedback, such as e-skin and advanced bio-robots, without the need for extensive traditional circuitry. However, implementing sensing in artificial muscles through neuromorphic configurations still requires separate sensors and synaptic devices, along with conversion stages to transfer outputs from sensors to inputs in the synaptic device.
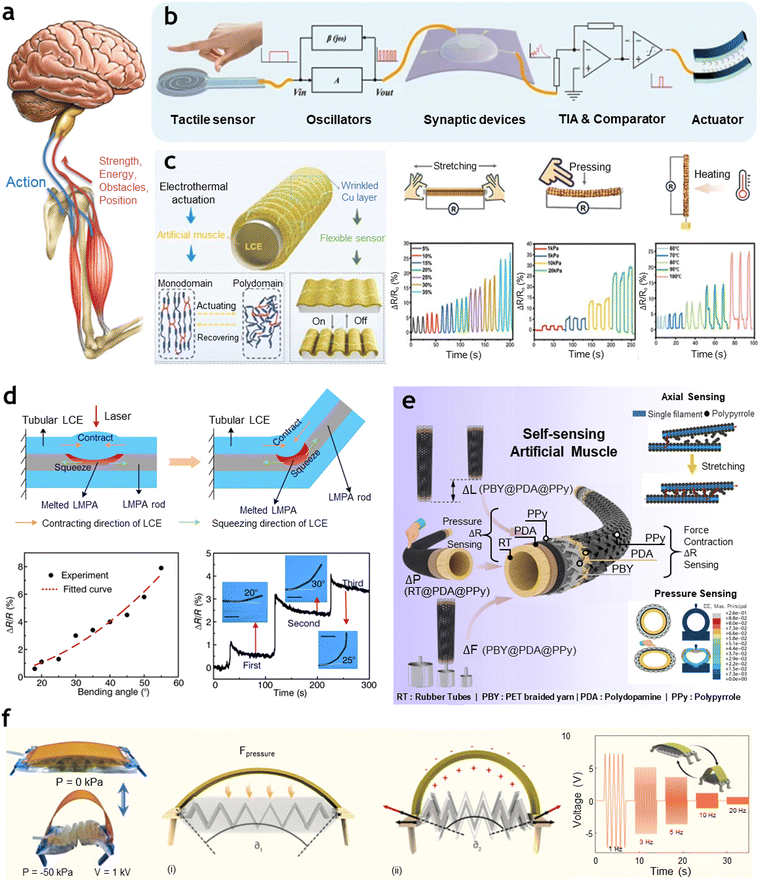 |
| Fig. 7 Intelligence imbued into artificial muscles by incorporating self-sensing capabilities. (a) Schematic of the natural muscular system showing the configuration of the brain/motor neurons (blue) and sensory neurons/muscles (red). Motor neurons deliver actuation signals from the brain to the muscles, while sensory neurons send feedback to the brain regarding mechanical, chemical, and thermal conditions. Reproduced with permission from Elsevier, Copyright 2021.157 (b) The schematic of the artificial neuromorphic somatosensory system for spatio-temporal tactile perception and feedback functions. Reproduced under the terms of the Creative Commons CC BY 4.0 license.161 (c) Liquid crystal elastomer (LCE)-based artificial neuromuscular system with integrated actuation-sensing-perception functions. The wrinkled Cu layer on the LCE surface enables self-sensing of strain, pressure, and heat by detecting resistance changes under varying environmental conditions. Reproduced with permission from WILEY-VCH GmbH, Copyright 2024.162 (d) Tubular LCE-based artificial muscle with a polydopamine coating encapsulating a low-melting-point alloy core. Localized laser-induced heating causes the LCE to contract, bending the muscle. The bending state is self-sensed via changes in electrical resistance. Reproduced under the terms of the Creative Commons CC BY 4.0 license.163 (e) Multi-sensorized pneumatic artificial muscle yarns designed to sense displacement, pressure, and force. These hierarchical, stimuli-responsive structures integrate multiple sensing functions into a single fiber. Reproduced with permission from Elsevier, Copyright 2022.164 (f) Contraction and recovery of the artificial muscle under vacuum pressure, elastic forces, release forces, and auxiliary forces generated by the muscle and a bionic spine. The muscle demonstrates environmental awareness and recognition through voltage signals generated by the bionic spine's reversible piezoelectric effect. Reproduced under the terms of the Creative Commons CC-BY-NC-ND license.165 | |
In contrast, self-sensing allows the muscle to simultaneously perform sensing and actuation without relying on external components.161,166–168 This approach simplifies the system, reduces the need for additional circuitry, and enables the muscle to autonomously detect and respond to environmental changes in real time. To implement this, the materials used in the artificial muscle must include a functional material that can move in response to stimuli, as well as materials and structures that exhibit changes in properties—such as resistance, capacitance, or voltage—when exposed to stimuli. As shown in Fig. 7(c), Zhu et al.162 developed a self-sensing artificial muscle by using a liquid crystal elastomer (LCE) core for actuation and a copper (Cu) sheath for sensing. The LCE serves as the actuating material, taking advantage of its temperature-responsive properties that allow it to contract and expand, thereby mimicking biological muscle functions. Meanwhile, the copper layer provides sensing capabilities, changing its electrical resistance in response to mechanical deformations such as stretching, pressure, and temperature fluctuations. To integrate the actuating LCE and sensing Cu layer seamlessly, they applied a copolymer layer composed of levodopa/polyethyleneimine (L-DOPA/PEI). This interface bridges the LCE core and Cu sheath, enhancing adhesion and allowing the Cu layer to remain stable and functional during the repeated deformations of the LCE. This structural combination enables the artificial muscle to reliably detect strain, pressure, and temperature, as well as track its own actuation path by monitoring resistance changes in real-time. The study demonstrated the capabilities of this self-sensing artificial muscle with an application in Chinese shadow puppetry. By embedding these fibers in the puppet system and using a machine learning model, the setup could not only actuate the puppet's movements but also recognize and classify the puppet's actions based on resistance feedback. In similar vein, Liu et al.163 developed a multifunctional artificial muscle capable of programmable deformation, self-sensing, and shape-locking, utilizing a tubular liquid crystal elastomer (LCE) as the actuating material and a low-melting-point alloy (LMPA) rod as the core for sensing and locking functions (Fig. 7(d)). The LCE responds to laser-induced heating, contracting and bending in a controlled manner. To achieve self-sensing and shape retention, they incorporated an LMPA rod within the LCE tube. This alloy rod remains solid at room temperature, providing locking deformations in place without continuous energy input. However, when exposed to a laser, the LMPA transitions to a liquid state, allowing the muscle to bend freely and monitor its deformation. For seamless integration and effective photothermal conversion, a polydopamine (PDA) coating was applied to the LCE, enhancing its response to laser stimulation. This setup allows the LCE to control bending angles accurately, while the LMPA rod, due to its resistance changes, enables real-time feedback on the bending angle and deformation extent. The resistance variation in response to the bending angles provides direct feedback on the artificial muscle's position and orientation, enabling it to self-monitor without additional sensors.
Beyond the integration of distinct functional materials within a composite structure, self-sensing capabilities in artificial muscles can also be achieved through leveraging architectural features. As shown in Fig. 7(e), Fu et al.164 developed a multi-sensorized pneumatic artificial muscle yarn (mPAMy) that combines actuation and proprioceptive sensing capabilities. The mPAMy design integrates a rubber tube core, which is coated with polydopamine (PDA) and polypyrrole (PPy) to enable sensing functions. Surrounding this core is a braided layer of polyethylene terephthalate (PET) yarn, also treated with PDA and PPy, which provides structural support and additional sensing functionality. This hierarchical arrangement facilitates independent measurements across different layers: the rubber core provides actuation while the braided PET layer allows for pressure and strain sensing. Functionally, the mPAMy operates as a McKibben actuator, where the rubber tube inflates when pressurized, causing radial expansion and axial contraction. Self-sensing abilities stem from the PPy-coated layers, which undergo resistance changes in response to mechanical deformations. The braided PET sheath senses axial strain, while the rubber core detects vertical pressure. As the muscle contracts, the distinct resistance variations in the PPy coatings provide real-time feedback on force, strain, and pressure, enabling simultaneous actuation and sensing. The multi-layered structure offers distinct advantages. By separating the sensing and actuation functions into different layers, the design minimizes electrical interference and reduces the need for external sensing equipment, thereby simplifying the system. In addition, as shown in Fig. 7(f), Gong et al.165 implemented a self-sensing approach that utilizes structural adaptation to achieve self-sensing based on the dynamic characteristics of the structure during motion induced by the artificial muscle. Here, a pneumatically driven artificial muscle provides the primary contractile force. Concurrently, a bionic spine composed of piezoelectric macro-fiber composites serves dual functions of actuation and sensing. The piezoelectric properties of the spine generate real-time feedback by detecting changes in deformation and environmental resistance, thus allowing the system to sense environmental interactions autonomously. The piezoelectric spine not only enhances movement through auxiliary actuation but also functions as a self-monitoring mechanism. This dual functionality is made possible by the structural design, which decouples the piezoelectric feedback signal into distinct components for sensing and actuation. The robot made of this self-sensing setup was tested across various terrains, autonomously adjusting its gait and speed based on real-time feedback from the piezoelectric spine. This feedback-driven control allowed it to navigate transitions between rough and smooth surfaces effectively and even adapt its movement in aquatic environments. As such, endowing artificial muscles with sensing capabilities to enhance their intelligence can be achieved through various approaches. However, a common feature across all methods is that these sensing capabilities enable intelligent artificial muscles to apply their multi-actuation modes and tunable response modes more effectively in response to environmental conditions. This approach ultimately elevates the intelligence of artificial muscles, significantly advancing their functional potential significantly.
Table 3 summarizes the overall performance of various artificial muscle types, highlighting their specific power, strain, response time, stability, and the advanced intelligence functionalities integrated into each type. Over the years, significant advancements have been made in optimizing the performance of artificial muscles, with the goal of achieving functionalities comparable to or even surpassing those of biological muscles. As this review focuses on empowering intelligence in artificial muscles, we have additionally summarized research efforts on integrating multi-mode actuation, self-sensing, and reprogramming across different artificial muscle types. Notably, polymer-based artificial muscles (e.g., magnetoactive elastomers, hydrogels, liquid crystal elastomers) have demonstrated the most progress in incorporating diverse intelligence-enhancing functionalities. Their tunable mechanical properties and ease of fabrication have made them promising candidates for reconfigurable and adaptive artificial muscles. However, while polymer-based systems have excelled in embedding intelligence, they still face limitations in terms of power output. Conversely, other artificial muscle types, such as shape memory alloys and pneumatics, exhibit superior output power but have yet to fully explore the integration of intelligence-driven functionalities. These findings highlight the remaining opportunities for further innovation, particularly in developing high-power, long-lasting artificial muscles with enhanced adaptability. Future research directions should focus on combining high-performance actuation with intelligence, enabling artificial muscles to not only replicate biological functions but also adapt and learn in real-time environments. This will be crucial for advancing their applications in soft robotics, wearable technologies, and biomedical devices.
Table 3 Summary of artificial muscle types, their key performance metrics, and empowered advanced intelligence types
Artificial muscles |
Specific power (W kg−1) |
Strain (%) |
Response time (mSec) |
Stability (cycles) |
Empowered advanced intelligence ypes |
Reference |
Skeletal muscle |
200 |
∼40 |
10–100 |
1 000 000 000 |
– Multi-mode |
169 |
– Reprogramming |
– Self-sensing |
Pneumatic |
5700 |
∼100 |
1–100 |
100 000 |
– Multi-mode actuation |
170–173 |
– Self-sensing |
Dielectric elastomer actuator (DEA) |
3600 |
∼380 |
2–100 |
600 000 |
– Multi-mode actuation |
79 and 174–176 |
– Self-sensing |
HASEL |
614 |
∼124 |
8–20 |
100 000 |
– Self-sensing |
177 and 178 |
Magnetoactive elastomers |
— |
∼90 |
10–250 |
2 000 000 |
– Multi-mode actuation |
128, 179 and 180 |
– Reprogramming |
– Self-sensing |
Hydrogels |
— |
∼90 |
300 |
1000 |
– Multi-mode actuation |
181–184 |
– Reprogramming |
– Self-sensing |
Ionic polymer composite (IPMC) |
244 |
∼40 |
10–1000 |
1 000 000 |
– Self-sensing |
185–187 |
Liquid crystal elastomer |
1 360 |
∼50 |
200–1000 |
1 000 000 |
– Multi-mode actuation |
188–190 |
– Reprogramming |
– Self-sensing |
Shape memory alloy |
10 000 |
∼60 |
0.62–5000 |
100 000 |
– Multi-mode actuation |
91, 191 and 192 |
– Self-sensing |
Applications for intelligent artificial muscles
As the fundamental units capable of generating force and initiating movement, artificial muscles serve a wide range of applications in both standalone and integrated forms. They can be deployed individually,193 bundled together,194 or arranged in modular systems that can be assembled and disassembled based on functional needs.195 In terms of application fields, artificial muscles are being widely utilized in exoskeleton muscles,196–198 wearable suits,199–201 and soft robotics,202–208 where flexibility and adaptability are crucial. As this review centers on the imbuing intelligence in artificial muscles, we aim to explore applications that highlight how intelligence enables artificial muscles to exhibit spontaneous responsiveness, task-agnostic adaptability, autonomous operation, and energy-efficient performance across diverse applications.
Adaptive robotics with intelligent artificial muscles
One of the key advantages of applying intelligent artificial muscles is their ability to enable soft robots to exhibit spontaneous responses to environmental changes. Robots designed to perform specific tasks may encounter a range of environments. For instance, locomotion or exploration robots may traverse varied terrain, transitioning from flat to inclined surfaces or moving from dry land to wet, amphibious areas.209,210 They may also need to pass through wide, open spaces as well as narrow passages.58 When applied as such adaptive, environment-responsive robotics, artificial muscles equipped with single programming-multiple actuation mode or multiple programming-multiple actuation mode capabilities can enhance multifunctionality and enable robots to respond dynamically to changing conditions. As shown in Fig. 8(a), Wang et al.211 demonstrate how intelligent artificial muscles can overcome physical constraints through a stepwise process enabled by their programmable and reprogrammable features. The robot, composed of ferromagnetic neodymium-iron-boron (NdFeB) microparticles embedded within a low-melting-point LM51 matrix (e.g., Galinstan, −19 °C; EGaIn, 15.7 °C; gallium, 29.8 °C; Bi45Sn23In19Sn8Cd5, 47 °C), is capable of phase transitions facilitated by inductive heating via an alternating magnetic field (AMF) and ambient cooling at room temperature. When encountering confined spaces in its solid state, which restrict movement, the robot not only utilizes its magnetically aligned properties but also transitions to a liquid state. This liquid phase, achieved through magnetic field-induced heating, allows it to adopt a slime-like form and navigate constrained environments. Once it has exited the restricted space, the robot solidifies again, reverting to its original structure, effectively demonstrating the morphological adaptability and versatility of intelligent artificial muscles in overcoming environmental challenges.
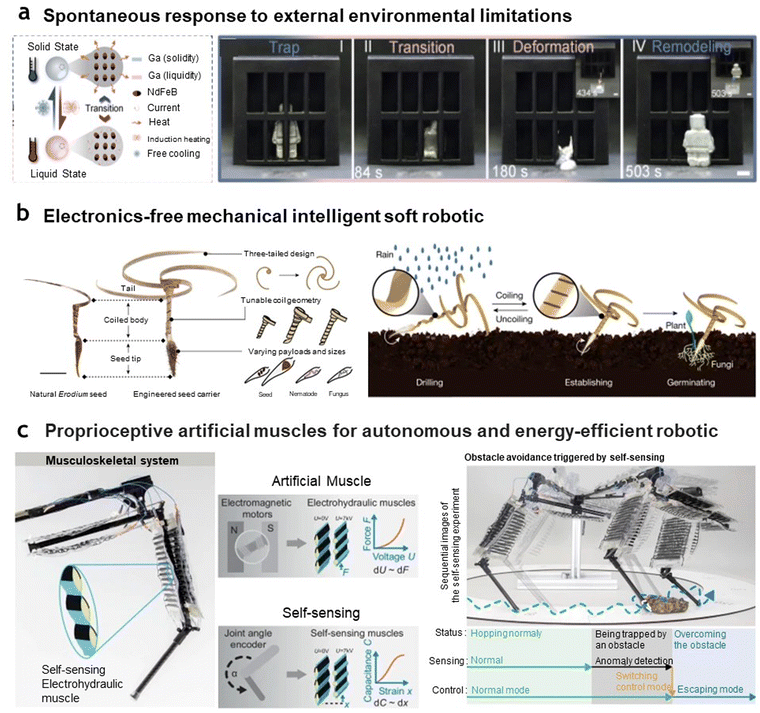 |
| Fig. 8 Applications of intelligent artificial muscles for adaptable, task-agnostic, and energy-efficient robotics. (a) Soft robot made of magnetoactive phase transitional matter (MPTM). The robot demonstrates the ability to freely transition between solid and liquid phases to overcome physical constraints and subsequently restore its original shape. This capability allows rapid adaptation to unexpected environmental challenges. Reproduced with permission from Elsevier, Copyright 2023.211 (b) Autonomous self-drilling and self-burying seed carriers. Inspired by Erodium seeds, the carriers are made of stiffness tuning wood veneer with hygromorphic bending or coiling properties. The three-tailed carrier autonomously delivers vegetable seeds alongside mycorrhizal fungi, acting as symbiotic biofertilizers. Reproduced with permission from Springer Nature, Copyright 2023.212 (c) Electrohydraulic musculoskeletal robotic leg for agile, adaptive, and energy-efficient locomotion. The system integrates electrohydraulic artificial muscles with self-sensing capabilities by monitoring capacitance changes during deformation. Obstacle avoidance is autonomously triggered through self-sensing mechanisms, enabling seamless locomotion mode transitions. Reproduced under the terms of the Creative Commons CC BY 4.0 license.213 | |
Another major advantage of using intelligent artificial muscles is their potential to create electronics-free, mechanically intelligent soft robots. Typically, enhancing a robot's intelligence for effective task performance involves integrating sensors and controllers. However, the addition of each component increases weight, bulk, and energy consumption, ultimately limiting efficiency. Instead, by utilizing intelligent artificial muscles, particularly those that can adapt to the environment without external sensors or controllers, it becomes possible to develop electronics-free robots. Recently, Luo et al.212 developed an autonomous self-burying seed carrier, a soft robotic system capable of high-efficiency self-drilling for seeding after areal dispersal (Fig. 8(b)). Inspired by the self-burying mechanism of Erodium seeds, the researchers crafted the seed carrier using wood veneer, leveraging its hygromorphic properties. The wood veneer exhibits a significant stiffness contrast between dry (about 4.9 GPa) and wet (about 1.3 GPa) states, enabling bending and coiling actuation with a high bending curvature (up to 1854 m−1). The design meticulously incorporated not only the inherent properties of wood but also structural elements—such as the awn topology and the parametric geometries of the tail and coils—while carefully aligning the wood fibers through mechanical molding. These design considerations allow the device to anchor, drill, establish, and facilitate germination autonomously, achieving all steps without the need for electronics. This innovative approach well demonstrates the potential for energy-free, autonomous, and mechanically intelligent soft robotics applications.
Intelligent artificial muscles with self-sensing capabilities have shown significant potential in developing proprioceptive robots.214–216 These robots, through enhanced compliance, achieve agility and environmental responsiveness comparable to biological systems, which are often challenging to replicate with rigid robotic designs. This approach contrasts with the AI-driven control models commonly used in rigid robots, where extensive case learning is needed to develop control schemes. For instance, as demonstrated by Buchner et al.213 (Fig. 8(c)), the team developed an electrohydraulic artificial muscle capable of terrain-adaptive transitions on varying surfaces such as pebbles, sand, and gravel using solely open-loop muscle force control. This setup enabled the muscle to exhibit agile responses to diverse terrains. The electrohydraulic muscle's design incorporates dielectric elastomers, which enable intrinsic strain-sensing through electrostatic properties. When external forces or deformations are applied, the dielectric elastomers experience changes in capacitance, allowing the muscle to detect and measure strain autonomously. This built-in strain-sensing capability allows the muscle to recognize obstacles in real-time, dynamically adjusting control modes based on environmental conditions, ensuring optimal performance in real-time and enhancing adaptability without the need for additional sensors. This streamlined design minimizes the system's weight and energy requirements, making it highly suitable for autonomous robotics operating in unpredictable environments where agility, efficiency, and real-time adaptability are essential.
Biomedical and organ simulation applications
Using artificial muscles as artificial organs, particularly in soft, bio-integrative forms, provides promising possibilities for replacing dysfunctional organs or serving as wearable or implantable devices.217–220 Given their soft mechanical properties, artificial muscles can closely match the softness of biological tissues, minimizing mechanical stress mismatches and reducing impact on surrounding tissues. Additionally, actuation in artificial muscles can be driven by biocompatible stimuli sources such as magnetic fields, fluidics, humidity, and temperature—all of which are generally safer and more compatible with the body than high electrical currents traditionally used to power electric motors.221 However, a crucial consideration in designing artificial organs is the ability to precisely and realistically replicate complex biological motions. In this regard, developing intelligent artificial muscles with anisotropic properties or specialized alignments proves especially advantageous for emulating the multi-directional arrangement of muscle fibers found in biological organs. This capability has had a significant impact on advancing organ simulators, which require intricate architectures to achieve realistic, functional mimicry. For instance, the heart's complex myocardial structure—often referred to as the “Gordian knot of anatomy” due to its intricate, interwoven fibers—drives cardiac motions that are challenging to replicate.222 Davis et al.223 developed a bio-inspired soft robotic left ventricle simulator that closely mimics cardiac motion while generating physiological pressures (Fig. 9(a)). This device employs thin-filament artificial muscles that emulate the multi-layered structure of the myocardium. By using canine myocardial strain data as input signals, the artificial myocardial layers are driven to simulate realistic cardiac motions. The system can replicate physiological volumes and pressures in both healthy and heart failure conditions, and it effectively demonstrated the simulation of a cardiac support device within a left-sided mock circulation loop. Here, instead of relying on pneumatic artificial muscles, which have previously struggled to reproduce biomimetic motion, authors proposed using hydraulic filament artificial muscles (HFAMs). HFAMs are highly scalable with high aspect ratios and high elongation capacity, allowing for the precise tuning of fiber density needed to capture the nuanced motions of cardiac muscle. The device's three layers of differently angled artificial muscle fibers are stabilized using a removable 3D pinboard, where pins link and fix the fiber angles—analogous to the extracellular matrix networks in the native heart. Through this multi-layered alignment and tunable hydraulic input, the artificial muscle layers are calibrated to reflect patient-specific cardiac functions, underscoring the potential of intelligent artificial muscles in personalized organ simulation and replacement applications.
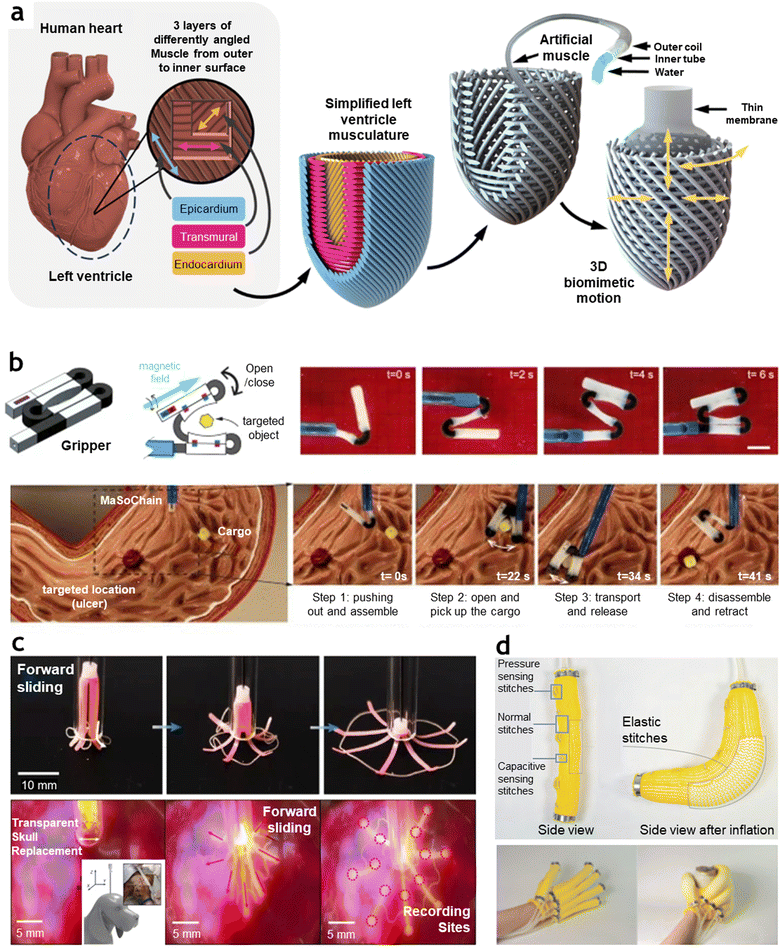 |
| Fig. 9 Applications of intelligent artificial muscles for biomedical and wearable robotics. (a) Soft artificial left ventricle simulator designed to replicate myocardial biomechanics. Three layers of thin-filament artificial muscles with varying fiber angles mimic the multilayered myocardial architecture. The device reproduces physiological volume and pressure under both healthy and heart failure conditions and effectively simulates cardiac support devices. Reproduced with permission from The American Association for the Advancement of Science, Copyright 2024.223 (b) Magnetic continuum soft robots with self-folding chains featuring reconfigurable shapes. The magnetic soft robotic chains exhibit automatic folding and disassembly via mechanical pushing and pulling, enabling cargo grasping and transport within organs without the need for electrical components. Reproduced under the terms of the Creative Commons CC BY 4.0 license.224 (c) Biodegradable and self-deployable electronic tent structure that automatically deploys after injection through a narrow tube into the brain via the skull, demonstrating its suitability for minimally invasive biomedical applications. Reproduced with permission from Springer Nature, Copyright 2024.225 (d) Capacitive self-sensing machine-knitted pneumatic wearable actuators designed for assistive wearables, integrating self-sensing capabilities for real-time performance monitoring and adaptability. Reproduced under the terms of the Creative Commons CC BY 4.0 license.226 | |
Wearable Robots and human augmentation
Biomedical applications of artificial muscles benefit significantly from their soft, conformable properties, allowing them to be inserted and guided within the body to perform tasks in complex in vivo environments.227–230 Their flexibility enables functionality within the narrow passages of organs, where a lower system complexity is often necessary. However, simplifying the system can reduce responsiveness to environmental changes during navigation. In these cases, the advanced intelligence of artificial muscles enables task performance with a simple system setup by leveraging the inherent intelligence of the muscle itself. In a notable example, Gu et al.224 proposed self-folding soft-robotic chains composed of units made from soft segments and embedded magnetic materials (Fig. 9(b)). The chain is designed by repeating these units, allowing it to be pushed and pulled relative to its catheter sheath. This structure enables repeated assembly and disassembly, with programmable shapes and functions, facilitating effective navigation in complex in vivo environments and supporting minimally invasive interventions. In even more delicate and confined environments, such as brain interfaces, artificial muscles offer potential for innovative applications. Brain–machine interfaces (BMIs) require sensors to make broad, stable contact with the brain to capture and transmit data effectively, often involving strategically placed electronics. However, the insertion of such large devices into the brain in vivo presents significant challenges, necessitating deployable structures that can be inserted compactly and then expand into the desired configuration. For example, Bae et al.225 recently developed a self-deployable electronic tent electrode for interfacing with the brain cortex (Fig. 9(c)). While this device does not use artificial muscle, it functions similarly by expanding to establish close contact with the brain once inserted. Importantly, just as intelligent artificial muscles are engineered through precise material and structural design, this self-deployable structure is designed and simulated to achieve close brain contact. Building on this approach, incorporating intelligent artificial muscles in place of purely passive deployable structures could enhance functionality.231,232 Intelligent artificial muscles would still fulfill the core function of expanding within confined spaces, but could also enable additional capabilities. For example, using artificial muscles would allow dynamic adaptability in response to brain movements or minor shifts, helping to maintain stable sensor contact for more accurate data collection. Moreover, artificial muscles could offer controlled shape adjustments to optimize the contact area with specific brain regions, enhance positioning precision, or even provide a gentle retraction mechanism to minimize tissue strain during removal. This added versatility could elevate brain-interface devices by integrating responsive, adaptable interactions in in vivo settings. Last but not least, intelligent artificial muscles are beneficial for soft wearable robots, offering lightweight, safe, and unobtrusive support to assist human movement.233 Initially, research in wearable soft robots focused on simple assistive contractions, but it has evolved to address more complex human movements. The shoulder joint, for instance, is a prime example of this complexity, featuring multiple degrees of freedom (DOFs). The glenohumeral joint—a ball-and-socket joint—facilitates three-dimensional movement, while the acromioclavicular joint provides an additional two DOFs.234–236
The elbow, with its hinge joint, enables bending in a single plane, contributing to the arm's overall flexibility.237 For artificial muscles to effectively assist these complex joints, they must be meticulously designed with well-aligned, clearly defined zones to match the intricacies of human biomechanics. Proper alignment and separation of regions within the muscle enable precise, controlled motion that supports natural movements, as illustrated in Fig. 9(d).226 This allows wearable artificial muscles to be applied in augmenting motions and enhancing human strength.
Conclusions and perspectives
This review summarizes the transformative advancements in artificial muscles, focusing on programming, reprogramming after complete erasing, and self-sensing capabilities that enable adaptive and intelligent functionality. The progress of memory-based programming has allowed artificial muscles to perform diverse tasks, especially in terms of exhibiting adaptive behaviour suited for dealing with dynamic environments. Similarly, the incorporation of self-sensing materials and neuromorphic feedback systems has paved the way for artificial muscles that can autonomously sense and respond to environmental changes. These advances have positioned artificial muscles as promising candidates for diverse applications such as soft robotics, wearable devices, and biomedical technologies.
Despite these remarkable developments, several limitations must be addressed to fully realize the potential of intelligent artificial muscles. First, while reprogramming allows artificial muscles to adapt to unforeseen conditions, the time required for reprogramming must be reduced. More critically, current methods lack the capability for dynamic reprogramming, as memory refresh and state adjustments must be performed within stationary setups. This limitation restricts true adaptive intelligence and mobile functionality. A potential solution involves leveraging self-healing polymers to create modular block units that can be assembled or disassembled like toy bricks, allowing for immediate reconfiguration. Recent advancements in rapidly self-healing polymers provide a foundation for such developments, facilitating fast recovery and dynamic adaptability in artificial muscles.238 Second, enhancing the structural complexity and scalability of artificial muscles is essential. Most existing reprogrammable artificial muscles are fabricated as films, which limits their functional diversity and alignment precision. Integrating multi-material 3D printing and 4D printing technology could overcome this limitation, enabling the creation of highly scalable, intricate bionic muscle structures with precise and diverse fiber alignments.239–241 Such innovations would allow artificial muscles to closely mimic the complex architecture of biological systems while maintaining reprogrammability. Lastly, achieving high-resolution localized reprogramming for complex geometries remains technically challenging. Advanced precision engineering and materials science are required to enable the precise activation and control of specific regions within artificial muscles.
Addressing these challenges will pave the way for the next generation of intelligent artificial muscles, capable of functioning effectively in diverse, dynamic, and unpredictable environments, thereby advancing their adoption in real-world applications.
Author contributions
Conceptualization, S. O. and W.-H. Y.; writing – original draft preparation, S. O. and W.-H. Y.; writing – review and editing, S. O., D. C. and W.-H. Y.; illustrations, S. O. and Y. H.; supervision, W.-H. Y.; project administration, W.-H. Y.; funding acquisition, W.-H. Y. All authors have read and agreed to the published version of the manuscript.
Data availability
No primary research results, software or code have been included and no new data were generated or analysed as part of this review.
Conflicts of interest
The authors have no relevant financial or non-financial interests to disclose.
Acknowledgements
The authors acknowledge the support of the Imlay Foundation – Innovation Fund and the WISH Center at the Institute for Matter and Systems at Georgia Tech.
Notes and references
- J. Song, C. Chen, S. Zhu, M. Zhu, J. Dai, U. Ray, Y. Li, Y. Kuang, Y. Li, N. Quispe, Y. Yao, A. Gong, U. H. Leiste, H. A. Bruck, J. Y. Zhu, A. Vellore, H. Li, M. L. Minus, Z. Jia, A. Martini, T. Li and L. Hu, Nature, 2018, 554, 224–228 CrossRef CAS PubMed.
- H. A. Lowenstam, Science, 1981, 211, 1126–1131 CrossRef CAS.
- U. G. K. Wegst, H. Bai, E. Saiz, A. P. Tomsia and R. O. Ritchie, Nat. Mater., 2015, 14, 23–36 CrossRef CAS.
- J. Aizenberg and P. Fratzl, Adv. Mater., 2009, 21, 387–388 CrossRef CAS.
- R. Elbaum, L. Zaltzman, I. Burgert and P. Fratzl, Science, 2007, 316, 884–886 CrossRef CAS.
- W. Huang, D. Restrepo, J.-Y. Jung, F. Y. Su, Z. Liu, R. O. Ritchie, J. McKittrick, P. Zavattieri and D. Kisailus, Adv. Mater., 2019, 31, 1901561 CrossRef CAS.
- M. A. Meyers, J. McKittrick and P.-Y. Chen, Science, 2013, 339, 773–779 CrossRef CAS PubMed.
- R. O. Ritchie, Nat. Mater., 2011, 10, 817–822 CrossRef CAS.
- T. J. Roberts and E. Azizi, J. Exp. Biol., 2011, 214, 353–361 CrossRef PubMed.
- Y. Forterre, J. M. Skotheim, J. Dumais and L. Mahadevan, Nature, 2005, 433, 421–425 CrossRef CAS PubMed.
- C. Dawson, J. F. V. Vincent and A.-M. Rocca, Nature, 1997, 390, 668 CrossRef CAS.
- M. Imani, M. Donn and Z. Balador, Handbook of Ecomaterials, Springer International Publishing, Cham, 2019, pp. 2213–2236 Search PubMed.
- R. Mc. N. Alexander and H. C. Bennet-Clark, Nature, 1977, 265, 114–117 CrossRef CAS PubMed.
- H. Cruse, Ch Bartling, M. Dreifert, J. Schmitz, D. E. Brunn, J. Dean and T. Kindermann, Adapt. Behav., 1995, 3, 385–418 CrossRef.
- A. Chortos, J. Liu and Z. Bao, Nat. Mater., 2016, 15, 937–950 CrossRef PubMed.
- X. Cheng, Z. Shen and Y. Zhang, Natl. Sci. Rev., 2024, 11, nwad314 CrossRef.
- B. Nie, S. Liu, Q. Qu, Y. Zhang, M. Zhao and J. Liu, Acta Biomater., 2022, 139, 280–295 CrossRef.
- E. Munch, M. E. Launey, D. H. Alsem, E. Saiz, A. P. Tomsia and R. O. Ritchie, Science, 2008, 322, 1516–1520 CrossRef.
- A. R. Studart, Adv. Funct. Mater., 2013, 23, 4423–4436 CrossRef.
- L. Beker, N. Matsuhisa, I. You, S. R. A. Ruth, S. Niu, A. Foudeh, J. B.-H. Tok, X. Chen and Z. Bao, Proc. Natl. Acad. Sci. U. S. A., 2020, 117, 11314–11320 CrossRef.
- B. Shin, Y. Kwon, M. Mittaz, H. Kim, X. Xu, E. Kim, Y. J. Lee, J. Lee, W.-H. Yeo and H. J. Choo, Nat. Commun., 2024, 15, 6803 CrossRef PubMed.
- C. De Pascali, G. A. Naselli, S. Palagi, R. B. N. Scharff and B. Mazzolai, Sci. Robot., 2022, 7, eabn4155 CrossRef PubMed.
- S. I. Rich, R. J. Wood and C. Majidi, Nat. Electron., 2018, 1, 102–112 CrossRef.
- C. Laschi, B. Mazzolai and M. Cianchetti, Sci. Robot., 2016, 1, eaah3690 CrossRef.
- D. Rus and M. T. Tolley, Nature, 2015, 521, 467–475 CrossRef PubMed.
- J. S. Mohammed and W. L. Murphy, Adv. Mater., 2009, 21, 2361–2374 Search PubMed.
- X. Liu, J. Fang, S. Huang, X. Wu, X. Xie, J. Wang, F. Liu, M. Zhang, Z. Peng and N. Hu, Microsyst. Nanoeng., 2021, 7, 1–23 CrossRef.
- J. Kim, A. S. Campbell, B. E.-F. de Ávila and J. Wang, Nat. Biotechnol., 2019, 37, 389–406 CrossRef CAS PubMed.
- D. Sengupta and A. G. P. Kottapalli, Adv. Electron. Mater., 2024, 10, 2300436 CrossRef CAS.
- M. L. Hammock, A. Chortos, B. C.-K. Tee, J. B.-H. Tok and Z. Bao, Adv. Mater., 2013, 25, 5997–6038 CrossRef CAS PubMed.
- Y. Kim, A. Chortos, W. Xu, Y. Liu, J. Y. Oh, D. Son, J. Kang, A. M. Foudeh, C. Zhu, Y. Lee, S. Niu, J. Liu, R. Pfattner, Z. Bao and T.-W. Lee, Science, 2018, 360, 998–1003 CrossRef CAS.
- J. Son, G. Y. Bae, S. Lee, G. Lee, S. W. Kim, D. Kim, S. Chung and K. Cho, Adv. Mater., 2021, 33, 2102740 CrossRef CAS.
- K. R. Pyun, K. Kwon, M. J. Yoo, K. K. Kim, D. Gong, W.-H. Yeo, S. Han and S. H. Ko, Natl. Sci. Rev., 2024, 11, nwad298 CrossRef CAS PubMed.
- M. Wehner, R. L. Truby, D. J. Fitzgerald, B. Mosadegh, G. M. Whitesides, J. A. Lewis and R. J. Wood, Nature, 2016, 536, 451–455 CrossRef CAS PubMed.
- A. K. Taseer, S. Oh, J.-S. Kim, M. Garai, H. Yoo, V. H. Nguyen, Y. Yang, M. Khan, M. Mahato and I. Oh, Adv. Mater., 2024, 36, 2312340 CrossRef CAS.
- V. H. Nguyen, S. Oh, M. Mahato, R. Tabassian, H. Yoo, S.-G. Lee, M. Garai, K. J. Kim and I.-K. Oh, Nat. Commun., 2024, 15, 435 CrossRef CAS PubMed.
- B. Shin, J. Ha, M. Lee, K. Park, G. H. Park, T. H. Choi, K.-J. Cho and H.-Y. Kim, Sci. Robot., 2018, 3, eaar2629 CrossRef PubMed.
- Y. Chen, Y. Zhang, H. Li, J. Shen, F. Zhang, J. He, J. Lin, B. Wang, S. Niu, Z. Han and Z. Guo, Nano Today, 2023, 49, 101764 CrossRef CAS.
- W. Li, Y. Pei, C. Zhang and A. G. P. Kottapalli, Nano Energy, 2021, 84, 105865 CrossRef CAS.
- G. Qian, B. Zhu, X. Liao, H. Zhai, A. Srinivasan, N. J. Fritz, Q. Cheng, M. Ning, B. Qie, Y. Li, S. Yuan, J. Zhu, X. Chen and Y. Yang, Adv. Mater., 2018, 30, 1704947 CrossRef PubMed.
- M.-H. Kim, S. Nam, M. Oh, H.-J. Lee, B. Jang and S. Hyun, Soft Robot., 2022, 9, 486–496 CrossRef.
- S.-M. Wen, S.-M. Chen, W. Gao, Z. Zheng, J.-Z. Bao, C. Cui, S. Liu, H.-L. Gao and S.-H. Yu, Adv. Mater., 2023, 35, 2211175 CrossRef CAS PubMed.
- M. K. Islam, P. J. Hazell, J. P. Escobedo and H. Wang, Mater. Des., 2021, 205, 109730 CrossRef.
- Z. Jia and L. Wang, Acta Mater., 2019, 173, 61–73 CrossRef CAS.
- H. Lund, Energy, 2007, 32, 912–919 CrossRef.
- I. Gomez and E. Lizundia, Adv. Sustainable Syst., 2021, 5, 2100236 CrossRef CAS.
- D. Saatchi, S. Oh, H. Yoo, J.-S. Kim, M.-J. Lee, M. Khan, B. Wicklein, M. Mahato and I.-K. Oh, Adv. Sci., 2024, 11, 2402872 CrossRef.
- D. Saatchi, S. Oh and I.-K. Oh, Adv. Funct. Mater., 2023, 33, 2214580 CrossRef CAS.
- Z. Zhakypov, K. Mori, K. Hosoda and J. Paik, Nature, 2019, 571, 381–386 CrossRef CAS.
- Y. Wu, J. K. Yim, J. Liang, Z. Shao, M. Qi, J. Zhong, Z. Luo, X. Yan, M. Zhang, X. Wang, R. S. Fearing, R. J. Full and L. Lin, Sci. Robot., 2019, 4, eaax1594 CrossRef.
- E. Chang, L. Y. Matloff, A. K. Stowers and D. Lentink, Sci. Robot., 2020, 5, eaay1246 CrossRef PubMed.
- R. L. Lieber and J. Fridén, Muscle Nerve, 2000, 23, 1647–1666 CrossRef CAS.
- A. F. Huxley and R. Niedergerke, Nature, 1954, 173, 971–973 CrossRef CAS PubMed.
- H. Huxley and J. Hanson, Nature, 1954, 173, 973–976 CrossRef CAS PubMed.
- M. Garai, M. Mahato, S. Nam, E. Kim, D. Seo, Y. Lee, V. H. Nguyen, S. Oh, P. Sambyal, H. Yoo, A. K. Taseer, S. A. Syed, H. Han, C. W. Ahn, J. Kim and I.-K. Oh, Adv. Funct. Mater., 2023, 33, 2212252 CrossRef CAS.
- M. Mahato, M. Garai, V. H. Nguyen, S. Oh, S. Nam, X. Zeng, H. Yoo, R. Tabassian and I.-K. Oh, Sci. Adv., 2023, 9, eadk9752 CrossRef CAS.
- M. Garai, V. H. Nguyen, M. Mahato, S. Oh, D. Saatchi, H. Yoo, S. S. Ali, D. Van Lam, A. K. Taseer and I.-K. Oh, Adv. Funct. Mater., 2024, 34, 2406603 CrossRef CAS.
- E. W. Hawkes, L. H. Blumenschein, J. D. Greer and A. M. Okamura, Sci. Robot., 2017, 2, eaan3028 CrossRef PubMed.
- P. T. Phan, T. T. Hoang, M. T. Thai, H. Low, N. H. Lovell and T. N. Do, Soft Robot., 2022, 9, 820–836 CrossRef PubMed.
- A. Maziz, A. Concas, A. Khaldi, J. Stålhand, N.-K. Persson and E. W. H. Jager, Sci. Adv., 2017, 3, e1600327 CrossRef PubMed.
- L. Wu, M. J. de Andrade, L. K. Saharan, R. S. Rome, R. H. Baughman and Y. Tadesse, Bioinspiration Biomimetics, 2017, 12, 026004 CrossRef PubMed.
- C. S. Haines, M. D. Lima, N. Li, G. M. Spinks, J. Foroughi, J. D. W. Madden, S. H. Kim, S. Fang, M. Jung de Andrade, F. Göktepe, Ö. Göktepe, S. M. Mirvakili, S. Naficy, X. Lepró, J. Oh, M. E. Kozlov, S. J. Kim, X. Xu, B. J. Swedlove, G. G. Wallace and R. H. Baughman, Science, 2014, 343, 868–872 CrossRef CAS PubMed.
- J. Foroughi, G. M. Spinks, G. G. Wallace, J. Oh, M. E. Kozlov, S. Fang, T. Mirfakhrai, J. D. W. Madden, M. K. Shin, S. J. Kim and R. H. Baughman, Science, 2011, 334, 494–497 CrossRef CAS.
- J. Zou, M. Feng, N. Ding, P. Yan, H. Xu, D. Yang, N. X. Fang, G. Gu and X. Zhu, Natl. Sci. Rev., 2021, 8, nwab048 CrossRef.
- S. M. Mirvakili, D. Sim, I. W. Hunter and R. Langer, Sci. Robot., 2020, 5, eaaz4239 CrossRef PubMed.
- R. S. Diteesawat, T. Helps, M. Taghavi and J. Rossiter, Sci. Robot., 2021, 6, eabc3721 CrossRef PubMed.
- E. Siéfert, E. Reyssat, J. Bico and B. Roman, Nat. Mater., 2019, 18, 24–28 CrossRef PubMed.
- B. Mosadegh, P. Polygerinos, C. Keplinger, S. Wennstedt, R. F. Shepherd, U. Gupta, J. Shim, K. Bertoldi, C. J. Walsh and G. M. Whitesides, Adv. Funct. Mater., 2014, 24, 2163–2170 CrossRef CAS.
- Y. Wu, S. Zhang, Y. Yang, Z. Li, Y. Wei and Y. Ji, Sci. Adv., 2022, 8, eabo6021 CrossRef CAS PubMed.
- M. Mahato, W.-J. Hwang, R. Tabassian, S. Oh, V. H. Nguyen, S. Nam, J.-S. Kim, H. Yoo, A. K. Taseer, M.-J. Lee, H. Zhang, T.-E. Song and I.-K. Oh, Adv. Mater., 2022, 34, 2203613 CrossRef CAS PubMed.
- G. Mao, M. Drack, M. Karami-Mosammam, D. Wirthl, T. Stockinger, R. Schwödiauer and M. Kaltenbrunner, Sci. Adv., 2020, 6, eabc0251 CrossRef CAS PubMed.
- S. Sundaram, M. Skouras, D. S. Kim, L. van den Heuvel and W. Matusik, Sci. Adv., 2019, 5, eaaw1160 CrossRef CAS.
- Y. Alapan, A. C. Karacakol, S. N. Guzelhan, I. Isik and M. Sitti, Sci. Adv., 2020, 6, eabc6414 CrossRef CAS PubMed.
- N. Sholl and K. Mohseni, Commun. Eng., 2024, 3, 1–10 CrossRef.
- A. Shiva, A. Stilli, Y. Noh, A. Faragasso, I. D. Falco, G. Gerboni, M. Cianchetti, A. Menciassi, K. Althoefer and H. A. Wurdemann, IEEE Robot. Autom. Lett., 2016, 1, 632–637 Search PubMed.
- T. Ren, Y. Li, M. Xu, Y. Li, C. Xiong and Y. Chen, Soft Robot., 2020, 7, 130–139 CrossRef PubMed.
- U. Jeong, K. Kim, S.-H. Kim, H. Choi, B. D. Youn and K.-J. Cho, Int. J. Robot. Res., 2021, 40, 494–511 CrossRef.
- C.-H. Li, C. Wang, C. Keplinger, J.-L. Zuo, L. Jin, Y. Sun, P. Zheng, Y. Cao, F. Lissel, C. Linder, X.-Z. You and Z. Bao, Nat. Chem., 2016, 8, 618–624 CrossRef PubMed.
- X. Ji, X. Liu, V. Cacucciolo, M. Imboden, Y. Civet, A. El Haitami, S. Cantin, Y. Perriard and H. Shea, Sci. Robot., 2019, 4, eaaz6451 CrossRef PubMed.
- O. Kim, H. Kim, U. H. Choi and M. J. Park, Nat. Commun., 2016, 7, 13576 CrossRef PubMed.
- S. Umrao, R. Tabassian, J. Kim, V. H. Nguyen, Q. Zhou, S. Nam and I.-K. Oh, Sci. Robot., 2019, 4, eaaw7797 CrossRef PubMed.
- S. Shian, K. Bertoldi and D. R. Clarke, Adv. Mater., 2015, 27, 6814–6819 CrossRef CAS PubMed.
- Y. S. Kim, M. Liu, Y. Ishida, Y. Ebina, M. Osada, T. Sasaki, T. Hikima, M. Takata and T. Aida, Nat. Mater., 2015, 14, 1002–1007 CrossRef CAS PubMed.
- Y. Osada and S. B. Ross-Murphy, Sci. Am., 1993, 268, 82–87 CrossRef CAS.
- D. Jiao, Q. L. Zhu, C. Y. Li, Q. Zheng and Z. L. Wu, Acc. Chem. Res., 2022, 55, 1533–1545 CrossRef CAS PubMed.
- A. Lendlein and S. Kelch, Angew. Chem., Int. Ed., 2002, 41, 2034–2057 CrossRef CAS.
- B. Jin, H. Song, R. Jiang, J. Song, Q. Zhao and T. Xie, Sci. Adv., 2018, 4, eaao3865 CrossRef PubMed.
- A. Lendlein and O. E. C. Gould, Nat. Rev. Mater., 2019, 4, 116–133 CrossRef.
- M. Zadan, D. K. Patel, A. P. Sabelhaus, J. Liao, A. Wertz, L. Yao and C. Majidi, Adv. Mater., 2022, 34, 2200857 CrossRef CAS PubMed.
- M. R. Vinciguerra, D. K. Patel, W. Zu, M. Tavakoli, C. Majidi and L. Yao, ACS Appl. Mater. Interfaces, 2023, 15, 24777–24787 CrossRef CAS PubMed.
- H.-T. Lee, M.-S. Kim, G.-Y. Lee, C.-S. Kim and S.-H. Ahn, Small, 2018, 14, 1801023 CrossRef PubMed.
- M.-S. Kim, J.-K. Heo, H. Rodrigue, H.-T. Lee, S. Pané, M.-W. Han and S.-H. Ahn, Adv. Mater., 2023, 35, 2208517 CrossRef CAS.
- X. Huang, K. Kumar, M. K. Jawed, A. M. Nasab, Z. Ye, W. Shan and C. Majidi, Sci. Robot., 2018, 3, eaau7557 CrossRef PubMed.
- W. Wang and S.-H. Ahn, Soft Robot., 2017, 4, 379–389 CrossRef PubMed.
- H. Zhang, S. Oh, M. Mahato, H. Yoo and I.-K. Oh, Adv. Funct. Mater., 2022, 32, 2205732 CrossRef CAS.
- Z. Shi, Z. Zhang and D. Ahmed, bioRxiv, 2024, preprint DOI:10.1101/2024.01.08.574699.
- W. Hu, G. Z. Lum, M. Mastrangeli and M. Sitti, Nature, 2018, 554, 81–85 CrossRef CAS PubMed.
- J. Cui, T.-Y. Huang, Z. Luo, P. Testa, H. Gu, X.-Z. Chen, B. J. Nelson and L. J. Heyderman, Nature, 2019, 575, 164–168 CrossRef CAS PubMed.
- Y. Kim, H. Yuk, R. Zhao, S. A. Chester and X. Zhao, Nature, 2018, 558, 274–279 CrossRef CAS PubMed.
- J. Rahmer, C. Stehning and B. Gleich, Sci. Robot., 2017, 2, eaal2845 CrossRef PubMed.
- T. Sauter, K. Kratz, S. Madbouly, F. Klein, M. Heuchel and A. Lendlein, Macromol. Mater. Eng., 2021, 306, 2000730 CrossRef CAS.
- E. Ahmed, D. P. Karothu, M. Warren and P. Naumov, Nat. Commun., 2019, 10, 3723 CrossRef PubMed.
- X. Liu, A. A. L. Michalchuk, B. Bhattacharya, N. Yasuda, F. Emmerling and C. R. Pulham, Nat. Commun., 2021, 12, 3871 CrossRef CAS PubMed.
- P. Xue, H. K. Bisoyi, Y. Chen, H. Zeng, J. Yang, X. Yang, P. Lv, X. Zhang, A. Priimagi, L. Wang, X. Xu and Q. Li, Angew. Chem., Int. Ed., 2021, 60, 3390–3396 CrossRef CAS PubMed.
- A. Toncheva, F. Khelifa, Y. Paint, M. Voué, P. Lambert, P. Dubois and J.-M. Raquez, ACS Appl. Mater. Interfaces, 2018, 10, 29933–29942 CrossRef CAS PubMed.
- Y. Zhang, Z. Wang, Y. Yang, Q. Chen, X. Qian, Y. Wu, H. Liang, Y. Xu, Y. Wei and Y. Ji, Sci. Adv., 2020, 6, eaay8606 CrossRef CAS PubMed.
- S. Z. Sharabani, E. Livnat, M. Abuchalja, N. Haphiloni, N. Edelstein-Pardo, T. Reuveni, M. Molco and A. Sitt, Soft Matter, 2024, 20, 2301–2309 RSC.
- Q. Chen, J. Mater. Res., 2024, 39, 2349–2368 CAS.
- S. Qi, H. Guo, J. Fu, Y. Xie, M. Zhu and M. Yu, Compos. Sci. Technol., 2020, 188, 107973 CrossRef CAS.
- A. Jafari, E. Behjat, H. Malektaj and F. Mobini, Wiley Interdiscip. Rev.: Mech. Dis., 2023, 15, e1620 Search PubMed.
- Y. Lei, N. L. Suresh, W. Z. Rymer and X. Hu, Muscle Nerve, 2018, 57, E85–E93 CrossRef PubMed.
- T. J. Roberts, C. M. Eng, D. A. Sleboda, N. C. Holt, E. L. Brainerd, K. K. Stover, R. L. Marsh and E. Azizi, Physiol., 2019, 34, 402–408 CrossRef PubMed.
- W. M. Kier and K. K. Smith, Zool. J. Linn. Soc., 1985, 83, 307–324 CrossRef.
- Z. Hu, Y. Zhang, H. Jiang and J. Lv, Sci. Adv., 2023, 9, eadh3350 CrossRef PubMed.
- Y. Pu, S. Zheng, X. Hu, S. Tang and N. An, Mater. Des., 2024, 246, 113334 Search PubMed.
- Z. Yang, J. Li, X. Chen, Y. Fan, J. Huang, H. Yu, S. Yang and E.-Q. Chen, Adv. Mater., 2023, 35, 2211648 Search PubMed.
- A. Baranwal and P. K. Agnihotri, Compos. Sci. Technol., 2022, 230, 109724 CrossRef.
- M. Schaffner, J. A. Faber, L. Pianegonda, P. A. Rühs, F. Coulter and A. R. Studart, Nat. Commun., 2018, 9, 878 CrossRef PubMed.
- D. Xie, Y. Su, X. Li, J. Chen, X. Shi, D. Liang, J. Yip, J. Liu, Z. Li and R. K. Tong, Adv. Intell. Syst., 2023, 5, 2200370 CrossRef.
- F. Connolly, C. J. Walsh and K. Bertoldi, Proc. Natl. Acad. Sci. U. S. A., 2017, 114, 51–56 CrossRef PubMed.
- A. Sedal, M. Fisher, J. Bishop-Moser, A. Wineman and S. Kota, in 2018 IEEE/RSJ International Conference on Intelligent Robots and Systems (IROS), 2018, pp. 464–471.
- A. G. Mark, S. Palagi, T. Qiu and P. Fischer, in 2016 IEEE International Conference on Robotics and Automation (ICRA), 2016, pp. 4951–4956.
- J. I. Lipton, R. MacCurdy, Z. Manchester, L. Chin, D. Cellucci and D. Rus, Science, 2018, 360, 632–635 CrossRef CAS PubMed.
- R. S. Kshetrimayum, IEEE Potentials, 2005, 23, 44–46 Search PubMed.
- K. Bertoldi, V. Vitelli, J. Christensen and M. van Hecke, Nat. Rev. Mater., 2017, 2, 1–11 Search PubMed.
- M. Khan, S. Oh, T.-E. Song, W. Ji, M. Mahato, Y. Yang, D. Saatchi, S. S. Ali, J. Roh, D. Yun, J.-H. Ryu and I.-K. Oh, Adv. Mater., 2025, 37, 2411353 CrossRef CAS PubMed.
- S. Zhuo, Z. Zhao, Z. Xie, Y. Hao, Y. Xu, T. Zhao, H. Li, E. M. Knubben, L. Wen, L. Jiang and M. Liu, Sci. Adv., 2020, 6, eaax1464 CrossRef CAS PubMed.
- M. Seong, K. Sun, S. Kim, H. Kwon, S.-W. Lee, S. C. Veerla, D. K. Kang, J. Kim, S. Kondaveeti, S. M. Tawfik, H. W. Park and H. E. Jeong, Nat. Commun., 2024, 15, 7929 CrossRef CAS PubMed.
- Q. Ze, X. Kuang, S. Wu, J. Wong, S. M. Montgomery, R. Zhang, J. M. Kovitz, F. Yang, H. J. Qi and R. Zhao, Adv. Mater., 2020, 32, 1906657 CrossRef CAS PubMed.
- H. Yang, C. Zhang, B. Chen, Z. Wang, Y. Xu and R. Xiao, Smart Mater. Struct., 2023, 32, 085023 CrossRef.
- Q. He, Z. Wang, Y. Wang, A. Minori, M. T. Tolley and S. Cai, Sci. Adv., 2019, 5, eaax5746 CrossRef CAS PubMed.
- B. Yang, R. Baines, D. Shah, S. Patiballa, E. Thomas, M. Venkadesan and R. Kramer-Bottiglio, Sci. Adv., 2021, 7, eabh2073 CrossRef PubMed.
- Q. L. Zhu, W. Liu, O. Khoruzhenko, J. Breu, W. Hong, Q. Zheng and Z. L. Wu, Nat. Commun., 2024, 15, 300 CrossRef CAS PubMed.
- M. Zhang, A. Pal, Z. Zheng, G. Gardi, E. Yildiz and M. Sitti, Nat. Mater., 2023, 22, 1243–1252 CrossRef CAS PubMed.
- T. Zhao, W. Dou, Z. Hu, W. Hou, Y. Sun and J. Lv, Macromol. Rapid Commun., 2020, 41, 2000313 CrossRef CAS PubMed.
- Y. Dong, L. Wang, N. Xia, Y. Wang, S. Wang, Z. Yang, D. Jin, X. Du, E. Yu, C. Pan, B.-F. Liu and L. Zhang, Nano Energy, 2021, 88, 106254 CrossRef CAS.
- A. Khaldi, J. A. Elliott and S. K. Smoukov, J. Mater. Chem. C, 2014, 2, 8029–8034 RSC.
- Y. He, H. Liu, J. Luo, N. Li, L. Li, P. Xiong, J. Gan and Z. Yang, npj Flexible Electron., 2023, 7, 1–9 CrossRef CAS.
- M. Lahikainen, H. Zeng and A. Priimagi, Nat. Commun., 2018, 9, 4148 CrossRef.
- H. Deng, K. Sattari, Y. Xie, P. Liao, Z. Yan and J. Lin, Nat. Commun., 2020, 11, 6325 CrossRef CAS.
- J. Lou, Z. Liu, L. Yang, Y. Guo, D. Lei and Z. You, Adv. Funct. Mater., 2021, 31, 2008328 CrossRef CAS.
- Y. Chen, A. M. Kushner, G. A. Williams and Z. Guan, Nat. Chem., 2012, 4, 467–472 CrossRef CAS PubMed.
- J. Lin, P. Zhou, Q. Chen, W. Zhang, Z. Luo and L. Chen, Sens. Actuators, B, 2022, 362, 131776 CrossRef CAS.
- G. Chen, B. Jin, Y. Shi, Q. Zhao, Y. Shen and T. Xie, Adv. Mater., 2022, 34, 2201679 CrossRef CAS PubMed.
- H. Xu, H. Liang, Y. Yang, Y. Liu, E. He, Z. Yang, Y. Wang, Y. Wei and Y. Ji, Nat. Commun., 2024, 15, 7381 CrossRef CAS PubMed.
- Y. Wang, Z. Wang, Q. He, P. Iyer and S. Cai, Adv. Intell. Syst., 2020, 2, 1900177 CrossRef.
- J.-H. Lee, J. Bae, J. H. Hwang, M.-Y. Choi, Y. S. Kim, S. Park, J.-H. Na, D.-G. Kim and S. Ahn, Adv. Funct. Mater., 2022, 32, 2110360 CrossRef CAS.
- S. Miao, Y. Xing, X. Li, B. Sun, Z. Du, H. Cao, P. Guo, Y. Chang, Y. Tian, M. Yao, K. Chen, D. Xiao, X. Zhang, B. Zhao, K. Pan, J. Sun and X. Liang, Appl. Mater. Today, 2024, 40, 102413 CrossRef.
- Y. Wang, L. Li, Y.-E. Ji, T. Wang, Y. Fu, X. Li, G. Li, T. Zheng, L. Wu, Q. Han, Y. Zhang, Y. Wang, D. L. Kaplan and Y. Lu, Proc. Natl. Acad. Sci. U. S. A., 2023, 120, e2305704120 CrossRef CAS PubMed.
- Y. Wang, R. Yin, L. Jin, M. Liu, Y. Gao, J. Raney and S. Yang, Adv. Funct. Mater., 2023, 33, 2210614 CrossRef CAS.
- S. Oh, T.-E. Song, M. Mahato, J.-S. Kim, H. Yoo, M.-J. Lee, M. Khan, W.-H. Yeo and I.-K. Oh, Adv. Mater., 2023, 35, 2304442 CrossRef CAS PubMed.
- L. Dong, M. Ren, X. Yuan, G. Yang, J. Di and Q. Li, Acc. Mater. Res., 2024, 5, 479–491 CrossRef CAS.
- Z. Lin, Z. Wang, W. Zhao, Y. Xu, X. Wang, T. Zhang, Z. Sun, L. Lin and Z. Peng, Adv. Intell. Syst., 2023, 5, 2200329 CrossRef.
- H. Wang, M. Totaro and L. Beccai, Adv. Sci., 2018, 5, 1800541 CrossRef PubMed.
- L. A. Jones, Can. J. Physiol. Pharmacol., 1994, 72, 484–487 CrossRef CAS PubMed.
- U. Proske and S. C. Gandevia, Physiol. Rev., 2012, 92, 1651–1697 CrossRef CAS PubMed.
- T. F. Otero, Electrochim. Acta, 2021, 368, 137576 CrossRef CAS.
- Y. Lee, Y. Liu, D.-G. Seo, J. Y. Oh, Y. Kim, J. Li, J. Kang, J. Kim, J. Mun, A. M. Foudeh, Z. Bao and T.-W. Lee, Nat. Biomed. Eng., 2023, 7, 511–519 CrossRef PubMed.
- J. Zhu, X. Zhang, R. Wang, M. Wang, P. Chen, L. Cheng, Z. Wu, Y. Wang, Q. Liu and M. Liu, Adv. Mater., 2022, 34, 2200481 CrossRef CAS PubMed.
- J. Yu, Y. Wang, S. Qin, G. Gao, C. Xu, Z. Lin Wang and Q. Sun, Mater. Today, 2022, 60, 158–182 CrossRef CAS.
- F. Sun, Q. Lu, M. Hao, Y. Wu, Y. Li, L. Liu, L. Li, Y. Wang and T. Zhang, npj Flexible Electron., 2022, 6, 1–8 CrossRef.
- C. Zhu, Y. Zhang, G. He, Y. Shi, Y. Wu, Y. Yu and X. Liu, Adv. Funct. Mater., 2025, 35, 2413845 CrossRef CAS.
- H. Liu, H. Tian, X. Li, X. Chen, K. Zhang, H. Shi, C. Wang and J. Shao, Sci. Adv., 2022, 8, eabn5722 CrossRef CAS PubMed.
- C. Fu, K. Wang, W. Tang, A. Nilghaz, C. Hurren, X. Wang, W. Xu, B. Su and Z. Xia, Chem. Eng. J., 2022, 446, 137241 CrossRef CAS.
- S. Gong, F. Fang, Z. Yi, B. Feng, A. Li, W. Li, L. Shao and W. Zhang, Innovation, 2024, 5, 100640 Search PubMed.
- R. Wang, Y. Shen, D. Qian, J. Sun, X. Zhou, W. Wang and Z. Liu, Mater. Horiz., 2020, 7, 3305–3315 RSC.
- L. Dong, M. Ren, Y. Wang, J. Qiao, Y. Wu, J. He, X. Wei, J. Di and Q. Li, Mater. Horiz., 2021, 8, 2541–2552 RSC.
- H. C. Lee, N. Elder, M. Leal, S. Stantial, E. Vergara Martinez, S. Jos, H. Cho and S. Russo, Nat. Commun., 2024, 15, 8456 CrossRef CAS PubMed.
- R. H. Baughman, Science, 2005, 308, 63–65 CrossRef CAS PubMed.
- S. M. Mirvakili and I. W. Hunter, Adv. Mater., 2018, 30, 1704407 CrossRef PubMed.
- M. Feng, D. Yang, L. Ren, G. Wei and G. Gu, Sci. Adv., 2023, 9, eadi7133 CrossRef PubMed.
- Z. Wang, H. Cui, M. Liu, S. L. Grage, M. Hoffmann, E. Sedghamiz, W. Wenzel and P. A. Levkin, Adv. Mater., 2022, 34, 2107791 CrossRef CAS PubMed.
- S. Terryn, J. Brancart, D. Lefeber, G. Van Assche and B. Vanderborght, Sci. Robot., 2017, 2, eaan4268 CrossRef PubMed.
- Y. Guo, L. Liu, Y. Liu and J. Leng, Adv. Intell. Syst., 2021, 3, 2000282 CrossRef.
- Y. Chen, H. Zhao, J. Mao, P. Chirarattananon, E. F. Helbling, N. P. Hyun, D. R. Clarke and R. J. Wood, Nature, 2019, 575, 324–329 CrossRef CAS PubMed.
- M. W. M. Tan, H. Bark, G. Thangavel, X. Gong and P. S. Lee, Nat. Commun., 2022, 13, 6769 CrossRef CAS PubMed.
- P. Rothemund, N. Kellaris, S. K. Mitchell, E. Acome and C. Keplinger, Adv. Mater., 2021, 33, 2003375 CrossRef CAS PubMed.
- E. Acome, S. K. Mitchell, T. G. Morrissey, M. B. Emmett, C. Benjamin, M. King, M. Radakovitz and C. Keplinger, Science, 2018, 359, 61–65 CrossRef CAS PubMed.
- X. Wang, G. Mao, J. Ge, M. Drack, G. S. Cañón Bermúdez, D. Wirthl, R. Illing, T. Kosub, L. Bischoff, C. Wang, J. Fassbender, M. Kaltenbrunner and D. Makarov, Commun. Mater., 2020, 1, 1–10 CrossRef.
- V. Q. Nguyen, A. S. Ahmed and R. V. Ramanujan, Adv. Mater., 2012, 24, 4041–4054 CrossRef CAS PubMed.
- Hydrogel Sensors and Actuators: Engineering and Technology, ed. G. Gerlach and K.-F. Arndt, Springer Berlin Heidelberg, Berlin, Heidelberg, 2010, vol. 6 Search PubMed.
- J. Du, Q. Ma, B. Wang, L. Sun and L. Liu, iScience, 2023, 26, 106796 CrossRef CAS PubMed.
- M. Shang, S. Ma, J. Ma, L. Guo, C. Liu and X. Xu, Chem. Eng. J., 2024, 496, 153895 CrossRef CAS.
- C. Y. Li, S. Y. Zheng, X. P. Hao, W. Hong, Q. Zheng and Z. L. Wu, Sci. Adv., 2022, 8, eabm9608 CrossRef CAS PubMed.
- J. Li, W. Ma, L. Song, Z. Niu, L. Cai, Q. Zeng, X. Zhang, H. Dong, D. Zhao, W. Zhou and S. Xie, Nano Lett., 2011, 11, 4636–4641 CrossRef CAS.
- S. Liu, Y. Liu, H. Cebeci, R. G. de Villoria, J.-H. Lin, B. L. Wardle and Q. M. Zhang, Adv. Funct. Mater., 2010, 20, 3266–3271 CrossRef CAS PubMed.
- L. Lu, J. Liu, Y. Hu, Y. Zhang, H. Randriamahazaka and W. Chen, Adv. Mater., 2012, 24, 4317–4321 CrossRef CAS PubMed.
- Y. Wang, Q. He, Z. Wang, S. Zhang, C. Li, Z. Wang, Y.-L. Park and S. Cai, Adv. Mater., 2023, 35, 2211283 CrossRef CAS.
- Q. He, Z. Wang, Y. Wang, Z. Wang, C. Li, R. Annapooranan, J. Zeng, R. Chen and S. Cai, Sci. Robot., 2021, 6, eabi9704 CrossRef PubMed.
- I. H. Kim, S. Choi, J. Lee, J. Jung, J. Yeo, J. T. Kim, S. Ryu, S. Ahn, J. Kang, P. Poulin and S. O. Kim, Nat. Nanotechnol., 2022, 17, 1198–1205 CrossRef CAS.
- J. Mohd Jani, M. Leary, A. Subic and M. A. Gibson, Mater. Des., 2014, 56, 1078–1113 CrossRef CAS.
- A. Weirich and B. Kuhlenkötter, Actuators, 2019, 8, 61 CrossRef.
- W. Liang, H. Liu, K. Wang, Z. Qian, L. Ren and L. Ren, Adv. Mech. Eng., 2020, 12, 1687814020933409 CrossRef.
- S. Y. Yang, K. Kim, J. U. Ko, S. Seo, S. T. Hwang, J. H. Park, H. S. Jung, Y. J. Gong, J. W. Suk, H. Rodrigue, H. Moon, J. C. Koo, J. Nam and H. R. Choi, Soft Robot., 2023, 10, 17–29 CrossRef PubMed.
- Z. Yoder, E. H. Rumley, I. Schmidt, P. Rothemund and C. Keplinger, Sci. Robot., 2024, 9, eadl3546 CrossRef PubMed.
- Y.-L. Park, B. Chen, N. O. Pérez-Arancibia, D. Young, L. Stirling, R. J. Wood, E. C. Goldfield and R. Nagpal, Bioinspir. Biomim., 2014, 9, 016007 CrossRef PubMed.
- S. Kurumaya, K. Suzumori, H. Nabae and S. Wakimoto, ROBOMECH J., 2016, 3, 18 CrossRef.
- A.-M. Georgarakis, M. Xiloyannis, P. Wolf and R. Riener, Nat. Mach. Intell., 2022, 4, 574–582 CrossRef.
- S. J. Park, K. Choi, H. Rodrigue and C. H. Park, Sci. Rep., 2022, 12, 11398 CrossRef CAS PubMed.
- S. J. Park and C. H. Park, Sci. Rep., 2019, 9, 9157 CrossRef.
- J. Piao, M. Kim, J. Kim, C. Kim, S. Han, I. Back, J. Koh and S. Koo, Sci. Rep., 2023, 13, 4869 CrossRef CAS PubMed.
- M. Mahato, R. Tabassian, V. H. Nguyen, S. Oh, S. Nam, K. J. Kim and I.-K. Oh, Adv. Funct. Mater., 2020, 30, 2003863 CrossRef CAS.
- M. Mahato, R. Tabassian, V. H. Nguyen, S. Oh, S. Nam, W.-J. Hwang and I.-K. Oh, Nat. Commun., 2020, 11, 5358 CrossRef CAS.
- M. Kotal, R. Tabassian, S. Roy, S. Oh and I.-K. Oh, Adv. Funct. Mater., 2020, 30, 1910326 CrossRef CAS.
- Y. Yang, D. Li, Y. Sun, M. Wu, J. Su, Y. Li, X. Yu, L. Li and J. Yu, Microsyst. Nanoeng., 2023, 9, 1–10 CrossRef.
- X. Li, D. Rao, M. Zhang, Y. Xue, X. Cao, S. Yin, J.-W. Wong, F. Zhou, T.-W. Wong, X. Yang and T. Li, Cell Rep. Phys. Sci., 2024, 5, 101957 CrossRef CAS.
- J. Lee, Y. Yoon, H. Park, J. Choi, Y. Jung, S. H. Ko and W.-H. Yeo, Adv. Intell. Syst., 2022, 4, 2100271 CrossRef.
- R. Goldoni, Y. Ozkan-Aydin, Y.-S. Kim, J. Kim, N. Zavanelli, M. Mahmood, B. Liu, F. L. I. Hammond, D. I. Goldman and W.-H. Yeo, ACS Appl. Mater. Interfaces, 2020, 12, 43388–43397 CrossRef CAS PubMed.
- D. K. Patel, X. Huang, Y. Luo, M. Mungekar, M. K. Jawed, L. Yao and C. Majidi, Adv. Mater. Technol., 2023, 8, 2201259 CrossRef.
- R. Baines, S. K. Patiballa, J. Booth, L. Ramirez, T. Sipple, A. Garcia, F. Fish and R. Kramer-Bottiglio, Nature, 2022, 610, 283–289 CrossRef CAS PubMed.
- Q. Wang, C. Pan, Y. Zhang, L. Peng, Z. Chen, C. Majidi and L. Jiang, Matter, 2023, 6, 855–872 CrossRef CAS.
- D. Luo, A. Maheshwari, A. Danielescu, J. Li, Y. Yang, Y. Tao, L. Sun, D. K. Patel, G. Wang, S. Yang, T. Zhang and L. Yao, Nature, 2023, 614, 463–470 CrossRef CAS.
- T. J. K. Buchner, T. Fukushima, A. Kazemipour, S.-D. Gravert, M. Prairie, P. Romanescu, P. Arm, Y. Zhang, X. Wang, S. L. Zhang, J. Walter, C. Keplinger and R. K. Katzschmann, Nat. Commun., 2024, 15, 7634 CrossRef CAS PubMed.
- L. Dong, M. Ren, Y. Wang, G. Wang, S. Zhang, X. Wei, J. He, B. Cui, Y. Zhao, P. Xu, X. Wang, J. Di and Q. Li, Sci. Adv., 2022, 8, eabq7703 CrossRef CAS PubMed.
- R. Tabassian, V. H. Nguyen, S. Umrao, M. Mahato, J. Kim, M. Porfiri and I.-K. Oh, Adv. Sci., 2019, 6, 1901711 CrossRef CAS PubMed.
- H. Zhao, K. O’Brien, S. Li and R. F. Shepherd, Sci. Robot., 2016, 1, eaai7529 Search PubMed.
- D. Zrinscak, L. Lorenzon, M. Maselli and M. Cianchetti, Prog. Biomed. Eng., 2023, 5, 012002 CrossRef.
- E. T. Roche, R. Wohlfarth, J. T. B. Overvelde, N. V. Vasilyev, F. A. Pigula, D. J. Mooney, K. Bertoldi and C. J. Walsh, Adv. Mater., 2014, 26, 1200–1206 CrossRef CAS PubMed.
- C. J. Payne, I. Wamala, C. Abah, T. Thalhofer, M. Saeed, D. Bautista-Salinas, M. A. Horvath, N. V. Vasilyev, E. T. Roche, F. A. Pigula and C. J. Walsh, Soft Robot., 2017, 4, 241–250 CrossRef PubMed.
- J. H. Lim, W. B. Han, T.-M. Jang, G.-J. Ko, J.-W. Shin, S. Han, H. Kang, C.-H. Eom, S. J. Choi, K. Rajaram, A. J. Bandodkar, W.-H. Yeo and S.-W. Hwang, Biosens. Bioelectron., 2024, 254, 116222 CrossRef CAS PubMed.
- L. Coles, P. W. Oluwasanya, N. Karam and C. M. Proctor, J. Mater. Chem. B, 2022, 10, 7122–7131 RSC.
- J. B. Pettigrew, Proc. R. Soc. London, 1997, 10, 433–440 Search PubMed.
- J. Davies, M. T. Thai, B. Sharma, T. T. Hoang, C. C. Nguyen, P. T. Phan, T. N. A. M. Vuong, A. Ji, K. Zhu, E. Nicotra, Y.-C. Toh, M. Stevens, C. Hayward, H.-P. Phan, N. H. Lovell and T. N. Do, Sci. Robot., 2024, 9, eado4553 CrossRef PubMed.
- H. Gu, M. Möckli, C. Ehmke, M. Kim, M. Wieland, S. Moser, C. Bechinger, Q. Boehler and B. J. Nelson, Nat. Commun., 2023, 14, 1263 CrossRef CAS PubMed.
- J.-Y. Bae, G.-S. Hwang, Y.-S. Kim, J. Jeon, M. Chae, J.-W. Kim, S. Lee, S. Kim, S.-H. Lee, S.-G. Choi, J.-Y. Lee, J.-H. Lee, K.-S. Kim, J.-H. Park, W.-J. Lee, Y.-C. Kim, K.-S. Lee, J. Kim, H. Lee, J. K. Hyun, J.-Y. Kim and S.-K. Kang, Nat. Electron., 2024, 7, 815–828 CrossRef.
- Y. Luo, K. Wu, A. Spielberg, M. Foshey, D. Rus, T. Palacios and W. Matusik, in CHI Conference on Human Factors in Computing Systems, ACM, New Orleans LA USA, 2022, pp. 1–13.
- M. Cianchetti, C. Laschi, A. Menciassi and P. Dario, Nat. Rev. Mater., 2018, 3, 143–153 CrossRef.
- M. Guess, I. Soltis, B. Rigo, N. Zavanelli, S. Kapasi, H. Kim and W.-H. Yeo, Soft Sci., 2023, 3, 24 CrossRef.
- M. Sang, M. Cho, S. Lim, I. S. Min, Y. Han, C. Lee, J. Shin, K. Yoon, W.-H. Yeo, T. Lee, S. M. Won, Y. Jung, Y. J. Heo and K. J. Yu, Sci. Adv., 2023, 9, eadh1765 CrossRef CAS PubMed.
- R. Herbert, M. Elsisy, B. Rigo, H.-R. Lim, H. Kim, C. Choi, S. Kim, S.-H. Ye, W. R. Wagner, Y. Chun and W.-H. Yeo, Nano Today, 2022, 46, 101557 CrossRef CAS PubMed.
- S. Song, F. Fallegger, A. Trouillet, K. Kim and S. P. Lacour, Sci. Robot., 2023, 8, eadd1002 CrossRef PubMed.
- T. Amadeo, D. Van Lewen, T. Janke, T. Ranzani, A. Devaiah, U. Upadhyay and S. Russo, Front. Robot. AI, 2022, 8, 731010 CrossRef PubMed.
- G. Bao, H. Fang, L. Chen, Y. Wan, F. Xu, Q. Yang and L. Zhang, Soft Robot., 2018, 5, 229–241 CrossRef PubMed.
- T. Proietti, C. O’Neill, L. Gerez, T. Cole, S. Mendelowitz, K. Nuckols, C. Hohimer, D. Lin, S. Paganoni and C. Walsh, Sci. Transl. Med., 2023, 15, eadd1504 CrossRef PubMed.
- W. B. Kibler, J. McMullen and T. Uhl, Oper. Tech. Sports Med., 2012, 20, 103–112 CrossRef.
- J. Lee, K. Kwon, I. Soltis, J. Matthews, Y. J. Lee, H. Kim, L. Romero, N. Zavanelli, Y. Kwon, S. Kwon, J. Lee, Y. Na, S. H. Lee, K. J. Yu, M. Shinohara, F. L. Hammond and W.-H. Yeo, npj Flexible Electron., 2024, 8, 1–13 CrossRef.
- S. Fornalski, R. Gupta and T. Q. Lee, Tech. Hand Up. Extrem. Surg., 2003, 7, 168–178 CrossRef PubMed.
- S. Wang and M. W. Urban, Nat. Rev. Mater., 2020, 5, 562–583 CrossRef CAS.
- T. J. K. Buchner, S. Rogler, S. Weirich, Y. Armati, B. G. Cangan, J. Ramos, S. T. Twiddy, D. M. Marini, A. Weber, D. Chen, G. Ellson, J. Jacob, W. Zengerle, D. Katalichenko, C. Keny, W. Matusik and R. K. Katzschmann, Nature, 2023, 623, 522–530 CrossRef CAS PubMed.
- Y. Wang, H. Cui, T. Esworthy, D. Mei, Y. Wang and L. G. Zhang, Adv. Mater., 2022, 34, 2109198 CrossRef CAS PubMed.
- E. Yarali, M. J. Mirzaali, A. Ghalayaniesfahani, A. Accardo, P. J. Diaz-Payno and A. A. Zadpoor, Adv. Mater., 2024, 36, 2402301 CrossRef CAS PubMed.
|
This journal is © The Royal Society of Chemistry 2025 |
Click here to see how this site uses Cookies. View our privacy policy here.