DOI:
10.1039/D4AY02269F
(Paper)
Anal. Methods, 2025,
17, 3183-3201
IRF4 as a molecular biomarker in pan-cancer through multiple omics integrative analysis†
Received
18th December 2024
, Accepted 28th March 2025
First published on 28th March 2025
Abstract
IRF4, characterized by its unique helix-turn-helix DNA-binding motif, is a member of the interferon regulatory factor (IRF) family. It plays a critical role in regulating host defense mechanisms, including innate and adaptive immune responses, as well as oncogenesis. However, the precise role of IRF4 in malignant tumors remains poorly understood. In this study, we first investigated IRF4 gene expression across various cancer types and its distribution within different molecular and immunological subtypes, providing a comprehensive understanding of its expression patterns in pan-cancer. We further explored the interacting proteins, diagnostic significance, molecular characteristics, prognostic relevance, and biological functions of IRF4 in diverse cancers. Focusing on colorectal cancer (CRC), we conducted a detailed analysis of IRF4, examining its associations with clinical features and outcomes across multiple clinical subgroups and databases. Additionally, we assessed IRF4 expression at both transcriptional and translational levels in CRC tumor specimens using tissue microarrays. Our findings revealed that IRF4 expression varies significantly not only across cancer types but also among molecular and immunological subtypes. In CRC, elevated IRF4 expression was associated with poorer overall survival. Notably, IRF4 was predominantly expressed in immune cells and showed a strong correlation with tumor immune regulation. Given its high predictive accuracy for cancer outcomes and robust prognostic associations, IRF4 may serve as a valuable prognostic biomarker for CRC. In conclusion, IRF4 represents a unique molecular biomarker for pan-cancer prognosis and an independent prognostic risk factor for CRC. Its critical role in immune regulation also positions IRF4 as a promising target for immunotherapeutic strategies in CRC.
Introduction
Pan-cancer biomarkers are molecular markers that are universally expressed or biologically significant across multiple cancer types. These biomarkers not only play a crucial role in cancer diagnosis and prognosis but also provide potential targets for targeted therapy and immunotherapy. Among them, EpCAM (Epithelial Cell Adhesion Molecule) and PD-L1 (Programmed Death-Ligand 1) are two extensively studied pan-cancer biomarkers.
EpCAM is a transmembrane glycoprotein widely expressed on the surface of tumor cells originating from epithelial tissues, including breast, colon, lung, and prostate cancers.1 Beyond its role in cell–cell adhesion, EpCAM promotes tumor cell proliferation and metastasis by regulating the Wnt/β-catenin signaling pathway.2 Due to its high expression in tumor cells and low expression in normal tissues, EpCAM has emerged as a critical target for liquid biopsy and circulating tumor cell (CTC) detection.3 Furthermore, EpCAM-based targeted therapies, such as antibody-drug conjugates and CAR-T cell therapies, have shown promising potential in clinical research.4 PD-L1, the ligand for the immune checkpoint molecule PD-1, is highly expressed in various tumor cells and facilitates immune evasion by suppressing T-cell activity.5 The expression of PD-L1 is closely associated with immune escape and poor prognosis in cancers such as non-small cell lung cancer, melanoma, and bladder cancer.6 In recent years, immune checkpoint inhibitors targeting the PD-1/PD-L1 pathway (e.g., pembrolizumab and atezolizumab) have demonstrated remarkable efficacy in treating multiple cancer types, marking a significant breakthrough in cancer immunotherapy.7 In summary, as pan-cancer biomarkers, EpCAM and PD-L1 not only hold significant value in basic cancer research but also exhibit broad application prospects in clinical diagnosis and treatment. Further investigation into the molecular mechanisms of these biomarkers and their roles in the tumor microenvironment will contribute to the development of more precise cancer diagnostic and therapeutic strategies.
Interferon regulatory factors (IRFs) are a family of structurally related proteins comprising nine members in mammals. Initially identified for their roles in inflammation, IRFs have since been shown to play significant functional roles in tumor biology. These proteins are critical regulators of immune responses and cancer pathogenesis, participating in a wide range of biological processes, including immune cell maturation, cell division, and apoptosis. As such, they exert substantial influence on both oncogenesis and immunity.8 In recent years, IRFs have emerged as key regulators of type I interferon signaling, which is implicated in tumor resistance to immune checkpoint blockade.9 Tumor immunology plays a pivotal role in tumorigenesis and progression. A critical aspect of the tumor microenvironment (TME) is the dynamic interplay between immune cells and tumor cells, often characterized by competition for nutrients or coordination of metabolic processes. Effective tumor elimination relies on robust adaptive immune responses.10 Notably, tertiary lymphoid structures (TLS)—ectopic lymphoid aggregates formed in various cancer types—are associated with favorable prognoses and enhanced responses to immunotherapy.11 Additionally, certain cancers produce inflammatory mediators that may serve as potential biomarkers for prognosis and diagnosis.12 The TME also plays a central role in enabling tumors to evade immune recognition and destruction.13
Interferon regulatory factor 4 (IRF4) is a transcription factor that is expressed in hematopoietic cells and plays pivotal roles in the immune response. IRF4 is critical for immunocytes' progress and activity, and autoimmune disorders.14 Originally described as a lymphocyte-specific nuclear factor, IRF4 promotes differentiation of naïve CD4+ T-cells into T helper 2 (Th2), Th9, Th17, or T follicular helper (Tfh) cells and is required for the function of effector regulatory T (eTreg) cells. Moreover, IRF4 is essential for the sustained differentiation of cytotoxic effector CD8+ T cells, for CD8+ T-cell memory formation and for differentiation of naïve CD8+ T cells into IL-9-producing (Tc9) and IL-17-producing (Tc17) CD8+ T-cell subsets.15 Furthermore, IRF4 is constitutively expressed in B-cells, and supports B-cell development. In particular, the highest level of IRF4 is observed in plasma cells.16,17
IRF4 is an oncogene that is frequently dysregulated in a wide range of adult lymphoid neoplasms. In malignant lymphoid cells, IRF4 modulates oncogenic transcriptional programs through a regulatory circuit involving its upstream component, nuclear factor kappa-light-chain-enhancer of activated B cells (NF-κB).18 Despite its established role in lymphoid malignancies, the broader implications of IRF4 in pan-cancer remain underexplored.
This study aims to provide a comprehensive and systematic understanding of IRF4's role across various cancers. To our knowledge, this is the first pan-cancer investigation to examine the expression patterns and functional dysregulation of IRF4. By evaluating its diagnostic and prognostic significance, we demonstrated that IRF4 exhibits variable expression across different molecular and immunological subtypes in multiple cancer types. Notably, IRF4 was significantly downregulated in ten distinct human malignancies. Furthermore, IRF4 showed high predictive accuracy for several cancers, including kidney clear cell carcinoma (KIRC), rectal cancer (READ), colon adenocarcinoma (COAD), esophageal carcinoma (ESCA), breast cancer (BRCA), bladder cancer (BLCA), liver cancer (LIHC), and cervical cancer (CESC). In patients with COAD, READ, and CESC, IRF4 levels were strongly correlated with both overall survival (OS) and progression-free interval (PFI). Focusing specifically on colorectal cancer (CRC), which encompasses READ and COAD, we identified IRF4 as an independent risk factor for OS using tissue microarray analysis and database mining. Our tissue microarray findings were consistent with our exploration of the relationship between IRF4 and immune cell infiltration, revealing that immune cells with high IRF4 expression were associated with improved prognosis. These results highlight IRF4 as a promising molecular target for CRC and a potential diagnostic and prognostic biomarker across multiple cancer types.
Results
Expression of IRF4 in pan-cancer
By analyzing data from the Human Protein Atlas (HPA, https://www.proteinatlas.org/) database, we systematically characterized IRF4 expression patterns in normal human tissues. The highest levels of IRF4 expression were observed in lymphoid-rich tissues, including the appendix, spleen, tonsil, and bone marrow (Fig. 1A). Comparative analysis revealed a consistent downregulation of IRF4 expression across nearly all cancer cell lines (Fig. 1B). Through integrated analysis of tumor specimens from The Cancer Genome Atlas (TCGA) and matched normal tissues from the Xiantao platform, we identified distinct patterns of IRF4 dysregulation across various malignancies. Specifically, IRF4 expression was significantly upregulated in head and neck squamous cell carcinoma (HNSC), kidney renal clear cell carcinoma (KIRC), and lung adenocarcinoma (LUAD), while demonstrating marked downregulation in bladder urothelial carcinoma (BLCA), breast invasive carcinoma (BRCA), colon adenocarcinoma (COAD), rectal adenocarcinoma (READ), liver hepatocellular carcinoma (LIHC), and kidney chromophobe (KICH) (Fig. 1C). Further validation using GTEx database controls from the Xiantao platform confirmed these findings, with TCGA data showing significant downregulation of IRF4 in BLCA, BRCA, COAD, and READ, while demonstrating elevated expression in KIRC, LIHC, and LUAD (Fig. 1D).
 |
| Fig. 1 Expression of IRF4 in both normal tissues and tumors. (A) IRF4 expression in HPA database-sourced normal tissues. (B) IRF4 expression in HPA database tumor cell lines. (C) IRF4 expression in adjoining normal tissues and TCGA tumors from the Xiantao platform. (D) IRF4 expression in normal tissues and TCGA tumors using information from the Xiantao platform's GTEx database as controls (*p < 0.05, **p < 0.01, ***p < 0.001). | |
IRF4 with molecular or immune subtypes of cancer correlations
Leveraging the TISIDB database, we conducted a comprehensive analysis of IRF4 differential expression across molecular subtypes in seven distinct cancer types. Our investigation revealed significant heterogeneity in IRF4 expression patterns among 12 molecular subgroups spanning multiple malignancies, including breast invasive carcinoma (BRCA), head and neck squamous cell carcinoma (HNSC), lung squamous cell carcinoma (LUSC), adrenocortical carcinoma (ACC), colon adenocarcinoma (COAD), lower-grade glioma (LGG), liver hepatocellular carcinoma (LIHC), esophageal carcinoma (ESCA), kidney renal papillary cell carcinoma (KIRP), ovarian serous cystadenocarcinoma (OV), and stomach adenocarcinoma (STAD). Notably, within COAD subtypes, the HM-SNV molecular subtype exhibited significantly elevated IRF4 expression compared to other molecular classifications (Fig. 2A). Further analysis identified distinct molecular subtypes with predominant IRF4 expression patterns across various cancers: CIMP-low in ACC (Fig. 2B), atypical in HNSC (Fig. 2C), iCluster:1 in LIHC (Fig. 2D), POLE in UCES (Fig. 2E), C2a in KIRP (Fig. 2F), G-CIMP-high in LGG (Fig. 2G), secretory in LUSC (Fig. 2H), EBV and GS in STAD (Fig. 2I), Her2 in BRCA (Fig. 2J), immunoreactive in OV (Fig. 2K), and CIN in ESCA (Fig. 2L).
 |
| Fig. 2 IRF4 expression and molecular subtype correlations in TCGA tumors. (A) COAD, (B) ACC, (C) HNSC, (D) LIHC, (E) UCEC, (F) KIRP, (G) LGG, (H) LUSC, (I) STAD, (J) BRCA, (K) OV, and (L) ESCA. | |
Conversely, there was a notable correlation between IRF4 expression and various immunological subtypes across 12 different cancer types (C1: wound healing, C2: IFN-gamma dominant, C3: inflammatory, C4: lymphocyte depleted, C5: immunologically silent, and C6: TGF-b dominant): COAD (Fig. 3A), ACC (Fig. 3B), PRAD (Fig. 3C), LIHC (Fig. 3D), UCEC (Fig. 3E), OV (Fig. 3F), LUAD (Fig. 3G), LUSC (Fig. 3H), BRCA (Fig. 3I), STAD (Fig. 3J), ESCA (Fig. 3K), and KIRC (Fig. 3L).
 |
| Fig. 3 IRF4 expression and immunological subtype correlations in TCGA tumors. (A) COAD, (B) ACC, (C) PRAD, (D) LIHC, (E) UCEC, (F) OV, (G) LUAD, (H) LUSC, (I) BRCA, (J) STAD, (K) ESCA, and (L) KIRC. C1, wound healing, C2, IFN-gamma dominant, C3, inflammatory, C4, lymphocyte depleted, C5, immunologically quiet, C6, TGF-β dominant. | |
Kyoto encyclopedia of genes and genomes (KEGG), gene ontology (GO), and protein–protein interaction (PPI) network enrichment analysis
Using the STRING database (Fig. 4A) and Cytoscape (Fig. 4B, displaying the top 20 interacting proteins), e successfully identified and excluded 50 potential binding proteins for IRF4. Gene Ontology (GO) enrichment analysis of the remaining 50 target proteins (Fig. 4B) revealed that the predominant biological processes (BP) were associated with antiviral defense mechanisms and symbiotic interactions. Cellular component (CC) analysis demonstrated significant enrichment in the proteasome core complex, transcription regulator complex, and RNA polymerase II transcription regulator complex. Molecular function (MF) analysis identified three primary activities: RNA polymerase II specificity, DNA-binding transcription factor binding, and DNA-binding transcription activator activity. Furthermore, KEGG pathway enrichment analysis (Fig. 4C and D) indicated significant associations with three major viral infection pathways: influenza A, hepatitis C, and measles.
 |
| Fig. 4 KEGG, GO, and protein–protein interaction (PPI) networks of 50 specific IRF4 binding proteins. (A) Analysis of the PPI network for 50 IRF4-targeted binding proteins from STRING. (B) Visual network of GeneMANIA's top 20 IRF4 targeted binding proteins. (C) Xiantao platform visual network of GO and KEGG analysis of 50 targeted binding proteins of IRF4. (D) Targeted binding proteins of IRF4: GO and KEGG study using the Xiantao platform. | |
Diagnostic value of IRF4 in patients with pan-cancer
To assess the diagnostic potential of IRF4 in pan-cancer prevention, we employed receiver operating characteristic (ROC) curve analysis. The results demonstrated that IRF4 exhibited significant predictive accuracy for multiple cancer types, including KIRC (AUC = 0.804), ESCA (AUC = 0.768), READ (AUC = 0.941), LIHC (AUC = 0.769), COAD (AUC = 0.908), BRCA (AUC = 0.775), BLCA (AUC = 0.713), and CESC (AUC = 0.861) with a significant degree of accuracy (area under the curve [AUC] > 0.7) (Fig. 5A). Notably, IRF4 showed particularly high diagnostic accuracy for COAD and READ, achieving AUC > 0.9.
 |
| Fig. 5 The predictive significance of IRF4 expression in pan-cancer and the receiver operating characteristic (ROC) curve. (A) READ, ESCA, BRCA, BLCA, KIRC, LIHC, and CESC IRF4 expression ROC curves. (B) Prognostic significance of IRF4 expression in COAD, READ, CESC, and LIHC (overall survival [OS] and progress-free interval [PFI]). | |
Prognostic of IRF4 significance in cancers
To further elucidate the prognostic significance of IRF4 expression in various cancers, we performed comprehensive survival analyses using the Kaplan–Meier method. The OS analysis (Fig. 5B) demonstrated significantly prolonged survival times in patients with elevated IRF4 expression across multiple cancer types, including CESC (p = 0.011), COAD (p = 0.033), and READ (p = 0.012). Consistent with these findings, PFI analysis revealed similar patterns, showing markedly extended PFI durations in patients with higher IRF4 expression levels for COAD (p = 0.012), LIHC (p = 0.015), and CESC (p = 0.002) compared to those with lower expression (Fig. 5B).
To further investigate the clinical relevance of IRF4 in CRC prognosis, we analyzed its correlation with OS across various CRC subgroups, including COAD and READ. Our analysis demonstrated that elevated IRF4 expression was significantly associated with improved OS in multiple clinical subgroups. Specifically, patients aged ≥65 years, those presenting with colon polyps without lymphatic invasion, and individuals with higher IRF4 expression levels showed prolonged survival. This prognostic advantage was consistently observed across several advanced disease stages, including pathologic T3 and T4, stage III and IV, as well as in patients with pathologic N0 stage, M0 stage, and CEA levels >5 ng mL−1.
IRF4 is correlated with different clinical characteristics in CRC
Building upon these findings, our analysis revealed that IRF4 exerts a significantly stronger prognostic influence in CRC than previously recognized. This compelling evidence prompted us to conduct a more in-depth investigation into its role in CRC pathogenesis. Through comprehensive clinical correlation analysis, we identified significant associations between IRF4 expression levels and multiple clinicopathological parameters, including histological type, neoplasm type, colon polyp history, and the N, M, and TNM stages. Notably, comparative analysis demonstrated markedly reduced IRF4 expression in patients with rectal adenocarcinoma at advanced stages (III/IV), nodal involvement (N1/N2), distant metastasis (M1), and absence of colon polyp history, when compared to those with mucinous adenocarcinoma (Fig. 6B) (Table 1).
 |
| Fig. 6 Relationships between OS in various clinical subgroups and CRC features and IRF4 expression. (A) Links between IRF4 expression and overall survival in several CRC clinical subgroups, such as T3 and T4 stages, III and IV stages, N0 stage, M0 stage, CEA level of >5, no history of colon polyps, colon polyps present, absence of lymphatic invasion, adenocarcinoma, and ages over 65. (B) Links between IRF4 expression and OS in various clinical CRC characteristics, including N stage, M stage, TNM stage, histological type, neoplasm type, and colon polyp history (*p < 0.05, **p < 0.01). | |
Table 1 Clinical characteristics of CRC patients
Characteristics |
Low expression of IRF4 |
High expression of IRF4 |
P value |
n
|
322 |
322 |
Age, n (%)
|
0.426
|
≤ 65 |
143 (22.2%) |
133 (20.7%) |
|
> 65 |
179 (27.8%) |
189 (29.3%) |
|
![[thin space (1/6-em)]](https://www.rsc.org/images/entities/char_2009.gif) |
Gender, n (%)
|
0.580
|
Female |
147 (22.8%) |
154 (23.9%) |
|
Male |
175 (27.2%) |
168 (26.1%) |
|
![[thin space (1/6-em)]](https://www.rsc.org/images/entities/char_2009.gif) |
Pathologic stage, n (%)
|
0.022
|
Stage I |
48 (7.7%) |
63 (10.1%) |
|
Stage II |
107 (17.2%) |
131 (21%) |
|
Stage III |
98 (15.7%) |
86 (13.8%) |
|
Stage IV |
55 (8.8%) |
35 (5.6%) |
|
![[thin space (1/6-em)]](https://www.rsc.org/images/entities/char_2009.gif) |
Histological type, n (%)
|
0.130
|
Adenocarcinoma |
281 (44.4%) |
269 (42.5%) |
|
Mucinous adenocarcinoma |
35 (5.5%) |
48 (7.6%) |
|
![[thin space (1/6-em)]](https://www.rsc.org/images/entities/char_2009.gif) |
CEA level, n (%)
|
0.211
|
≤ 5 |
119 (28.7%) |
142 (34.2%) |
|
> 5 |
80 (19.3%) |
74 (17.8%) |
|
![[thin space (1/6-em)]](https://www.rsc.org/images/entities/char_2009.gif) |
History of colon polyps, n (%)
|
0.042
|
No |
198 (35.7%) |
179 (32.3%) |
|
Yes |
77 (13.9%) |
101 (18.2%) |
|
![[thin space (1/6-em)]](https://www.rsc.org/images/entities/char_2009.gif) |
Lymphatic invasion, n (%)
|
0.112
|
No |
162 (27.8%) |
188 (32.3%) |
|
Yes |
123 (21.1%) |
109 (18.7%) |
|
Validation of IRF4 at transcription and translational level
The establishment of standardized protocols for tissue collection, staining procedures, and quantification methods is crucial for reliable detection of IRF4 mRNA and protein expression in CRC specimens using quantitative real-time polymerase chain reaction (qRT-PCR) and immunohistochemistry (IHC). Such standardization is essential to improve study reproducibility and facilitate validation of bioinformatics predictions. In this study, we quantitatively assessed IRF4 expression at both transcriptional and translational levels using complementary approaches: cDNA analysis for mRNA quantification and tissue microarray (TMA) analysis of paraffin-embedded specimens for protein detection. Our experimental results consistently demonstrated significantly elevated levels of both IRF4 mRNA and protein in normal colorectal tissues compared to their malignant counterparts (Fig. 7A and B, Table 2 and 3).
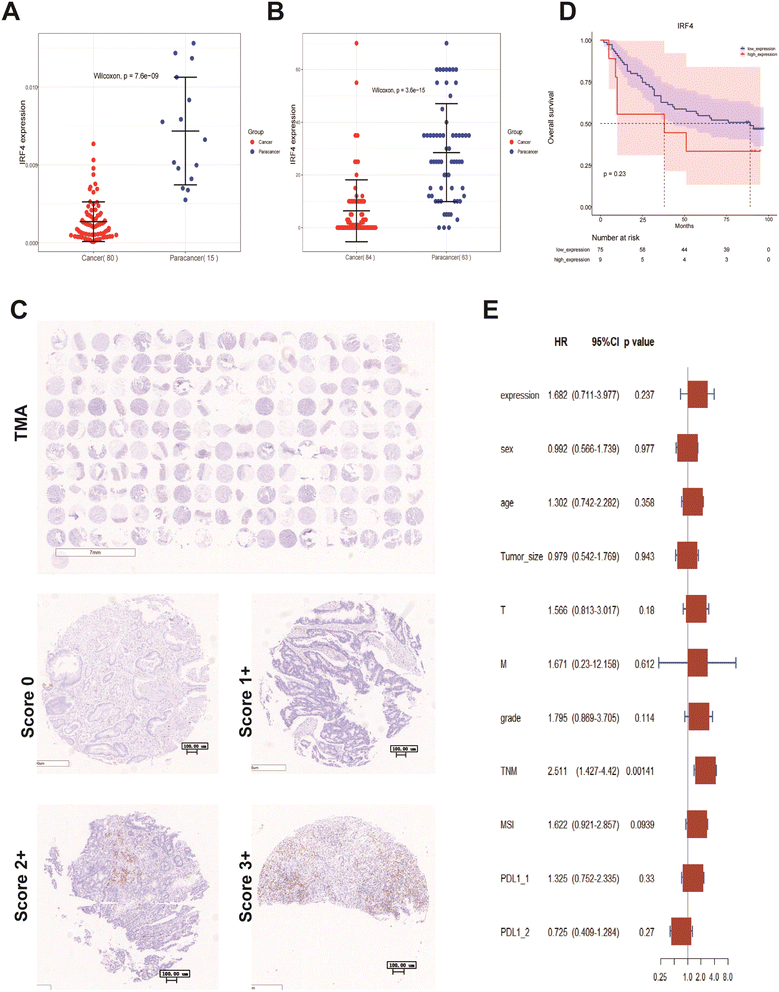 |
| Fig. 7 Validation of IRF4 at the transcriptional and translational levels by microassays. (A) IRF4 qPCR analysis in healthy colorectal and colorectal cancer tissues. (B) Using IHC, the IRF4 protein level in CRC tissues. (C) IRF4 staining samples that are representative of CRC TMA. Absent (score: 0), weak (score: 1+), moderate (score: 2+), and strong (score: 3+) IRF4-positive infiltrates in CRC. (D) Associations between IRF4 protein levels and OS in CRC. (E) Univariate survival analysis of clinicopathological variables in patients with CRC. | |
Table 2 Correlation between IRF4 expression and clinicopathological characteristics
|
Variables |
IRF4 expression |
Total |
p value |
r value |
Low |
High |
Sex |
|
|
|
|
0.33 |
0.116 |
|
Male |
12 |
21 |
33 |
|
|
|
Female |
12 |
35 |
47 |
|
|
Age |
|
|
|
|
0.465 |
0.109 |
|
≤65.5 |
14 |
26 |
40 |
|
|
|
>65.5 |
10 |
30 |
40 |
|
|
Tumor_size |
|
|
|
|
0.078 |
−0.225 |
|
≤5 cm |
21 |
55 |
76 |
|
|
|
>5 cm |
3 |
1 |
4 |
|
|
T |
|
|
|
|
1 |
0.016 |
|
II–III |
7 |
16 |
23 |
|
|
|
IV |
15 |
37 |
52 |
|
|
N |
|
|
|
|
0.317 |
−0.138 |
|
N0 |
10 |
31 |
41 |
|
|
|
N1/N2 |
13 |
22 |
35 |
|
|
M |
|
|
|
|
1 |
0.105 |
|
M0 |
24 |
54 |
78 |
|
|
|
M1 |
0 |
2 |
2 |
|
|
Grade |
|
|
|
|
0.361 |
0.122 |
|
I |
3 |
3 |
6 |
|
|
|
II–III |
21 |
52 |
73 |
|
|
TNM |
|
|
|
|
0.317 |
−0.138 |
|
I–II |
10 |
31 |
41 |
|
|
|
III–IV |
13 |
22 |
35 |
|
|
Table 3 Correlation between IRF4 expression and clinicopathological characteristics
|
Variables |
IRF4 expression |
Total |
p value |
r value |
Low |
High |
Sex |
|
|
|
|
0.73 |
0.055 |
|
Male |
40 |
4 |
44 |
|
|
|
Female |
35 |
5 |
40 |
|
|
Age |
|
|
|
|
0.307 |
−0.124 |
|
≤68 |
35 |
6 |
41 |
|
|
|
>68 |
40 |
3 |
43 |
|
|
Tumor_size |
|
|
|
|
1 |
−0.02 |
|
≤5 cm |
47 |
6 |
53 |
|
|
|
>5 cm |
27 |
3 |
30 |
|
|
T |
|
|
|
|
0.365 |
0.124 |
|
I–III |
61 |
6 |
67 |
|
|
|
IV |
13 |
3 |
16 |
|
|
N |
|
|
|
|
0.733 |
−0.05 |
|
N0 |
44 |
6 |
50 |
|
|
|
N1/N2 |
31 |
3 |
34 |
|
|
M |
|
|
|
|
1 |
−0.038 |
|
M0 |
74 |
9 |
83 |
|
|
|
M1 |
1 |
0 |
1 |
|
|
Grade |
|
|
|
|
0.207 |
−0.168 |
|
I |
16 |
4 |
20 |
|
|
|
II–III |
59 |
5 |
64 |
|
|
TNM |
|
|
|
|
0.733 |
−0.05 |
|
I–II |
44 |
6 |
50 |
|
|
|
III–IV |
31 |
3 |
34 |
|
|
MSI |
|
|
|
|
0.154 |
−0.171 |
|
MSS |
47 |
8 |
55 |
|
|
|
MSI-H |
28 |
1 |
29 |
|
|
PDL1_1 |
|
|
|
|
0.272 |
0.134 |
|
≤5 |
45 |
3 |
48 |
|
|
|
>5 |
30 |
5 |
35 |
|
|
PDL1_2 |
|
|
|
|
0.131 |
0.2 |
|
≤20 |
44 |
2 |
46 |
|
|
|
>20 |
31 |
6 |
37 |
|
|
To investigate IRF4 protein expression patterns in CRC, we constructed a TMA from paraffin-embedded samples of 101 primary tumors. The demographic characteristics of the patient cohort are detailed in Table 3. Subsequent analysis revealed no significant associations between IRF4 expression levels and clinicopathological parameters. IHC analysis demonstrated a distinct distribution pattern, with tumor-infiltrating mesenchymal lymphocytes exhibiting higher IRF4-positive plasma cell density compared to parenchymal tumor cells (ESI Fig. 2A†). Using a standardized semiquantitative scoring system, we categorized IRF4 expression levels as follows: 76.23% (77/101) of cases showed low expression (score 0–1+), while 13.86% (14/101) exhibited high expression (score 2–3+) (Fig. 7C). Ten tumor samples were excluded from evaluation due to technical limitations. Comparative survival analysis between low-IRF4 and high-IRF4 groups revealed a non-significant trend toward poorer OS in patients with elevated IRF4 expression (Fig. 7D). Univariate analysis identified pTNM stage as a significant prognostic factor for OS. This finding was further substantiated by multivariate analysis, which established pTNM stage as an independent predictor of OS (ESI Fig. 2B,†7E and Table 4). These results corroborate previous investigations and suggest that IRF4 may serve as a potential predictive biomarker in CRC management.
Immune infiltrate analysis of IRF4 in CRC
Given the established role of the IRF family in modulating inflammatory and immunological responses that influence tumor progression and prognosis, we conducted a comprehensive analysis of tumor immune infiltration patterns using the TIMER database. Tumor purity, representing the proportion of malignant cells within tumor tissue, significantly impacts immune infiltration studies in clinical tumor samples when employing genomic approaches.19 Our analysis revealed a significant inverse correlation between IRF4 expression levels and tumor purity (Fig. 8A). Furthermore, we identified positive associations (all p < 0.05) between IRF4 expression and infiltration levels of multiple immune cell populations in COAD, including CD4+ T cells, CD8+ T cells, B cells, macrophages, dendritic cells (DCs), and neutrophils. Comparable patterns were observed in READ (Fig. 8A). To further explore the relationship between tumor-infiltrating lymphocytes (TILs), copy number variation, methylation status, and IRF4 expression across various cancers, we employed the TISIDB database. While most TIL levels demonstrated positive correlations with IRF4 expression, immune infiltration in COAD and READ exhibited inverse relationships with IRF4 copy number and methylation status (Fig. 8B). Notably, IRF4 expression was associated with both immune stimulators and inhibitors across multiple cancer types, with the exception of LGG, UVM, and SKCM (Fig. 8B). Specifically, in COAD and READ, IRF4 showed strong positive correlations with key immune checkpoints CD19 and CD79A (Fig. 8C). Moreover, we identified significant associations between IRF4 copy number variation and infiltration levels of CD8+ T cells, B cells, neutrophils, DCs, and macrophages (Fig. 8D). Complementing these findings, we utilized the Human Protein Atlas (HPA) to characterize IRF4 expression across immune cell subtypes. IRF4 was predominantly expressed in naive B cells, plasmacytoid DCs, memory B cells, activated naive CD4+ T cells, myeloid DCs, and activated naive CD8+ T cells (ESI Fig. 3A–C†). Single-cell RNA expression profiling through HPA revealed significant correlations between IRF4 and specific immune cell populations, particularly T and B cell subsets (ESI Fig. 3D and E†).
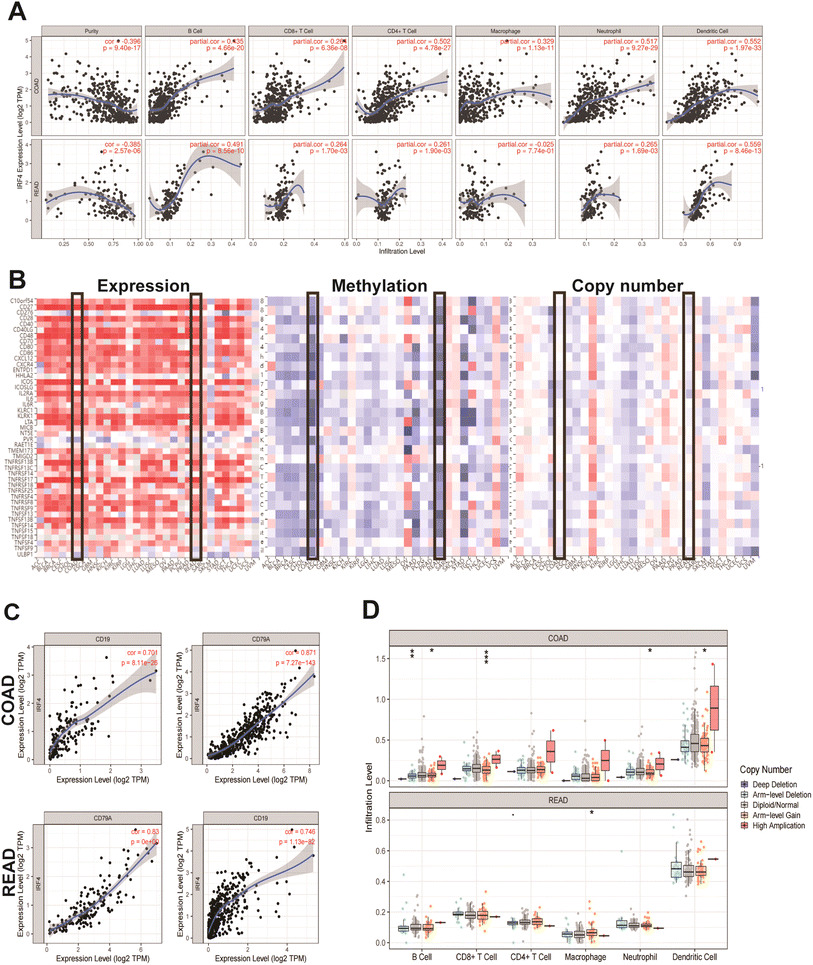 |
| Fig. 8 Immune infiltration analysis of IRF4 in CRC. (A) The link between IRF4 and various immune cells is demonstrated using Spearman's correlation test. (B) Analysis of the relationship between immune infiltration levels in TISIDB pan-cancer cells and IRF4 expression, copy number, and methylation. (C) IRF4 and representative immunological genes: a relationship examined with TIMER. (D) The TIMER database indicates the applicability of different somatic copy number alterations for CRC and IRF4 infiltration levels (*p < 0.05, **p < 0.01, ***p < 0.001). | |
Base on single-cell RNA-seq data of ten CRC patients were performed by the SMART-seq2 platform and the 10x Genomic single cell 3′ library platform, we found that higher expression of IRF4 was in plasma cells (ESI Fig. 4A and B†).20 Moreover, through analyzing the data of 600 CRC patients in the TCGA database and corresponding clinical information, we found that the positive correlation between the expression of IRF4 and the immune score themselves (ESI Fig. 4C†).21
To further elucidate the prognostic significance of immune cell infiltration and IRF4 expression in CRC, we conducted comprehensive univariate and multivariate Cox regression analyses using the TIMER database. After rigorous adjustment for potential confounding variables, our multivariate analysis revealed several independent prognostic factors in COAD patients: advanced disease stage (stage III: p = 0.006; stage IV: p < 0.001), age (p < 0.001), and CD8+ T cell infiltration (p = 0.033) were significantly associated with clinical outcomes. In READ patients, age (p = 0.002) and IRF4 expression levels (p = 0.032) emerged as significant prognostic indicators (Table 5).
Discussion
IRFs family plays a pivotal role in modulating inflammatory and immunological signaling pathways that are fundamentally involved in tumorigenesis and cancer progression. Dysregulation of IRFs has been extensively documented across various malignancies, including melanoma,19 leukemia,22 BRCA,23 esophageal squamous cell carcinoma,17 ovarian cancer,24 and hepatocellular carcinoma.25 However, to the best of our knowledge, no systematic investigation has been conducted to comprehensively evaluate the role of IRF4 across multiple cancer types.
In this study, we performed an integrative analysis of IRF4 expression patterns in pan-cancer malignancies utilizing multiple genomic databases, including TCGA, GTEx, Xiantao, and Cancer Cell Line Encyclopedia (CCLE). Our analysis revealed significant downregulation of IRF4 expression in several human cancers, particularly in BRCA, COAD, BLCA, KICH, LIHC, and READ. These findings suggest that IRF4 may exert tissue-specific functions in different cancer types, potentially mediated through tumor heterogeneity mechanisms.
Significant correlations were identified between the molecular subtypes of 12 different cancer types—COAD, ACC, HNSC, LIHC, UCEC, KIRP, LGG, LUSC, STAD, BRCA, OV, and ESCA—and the expression levels of IRF4. Notably, the HM-SNV subtype in COAD, the CIMP-low subtype in ACC, and the iCluster:1 subtype in LIHC exhibited the highest IRF4 expression levels. Furthermore, IRF4 demonstrated strong associations with various immunological subtypes across these 12 cancer types, underscoring its relevance in both molecular and immunological classification. Previous research has validated IRF4 as a potential prognostic marker and therapeutic target in a subset of esophageal squamous cell carcinoma (ESCC).17 Consequently, the research was concentrated on a specific molecular subtype or immune subtype of malignancies, potentially establishing a solid groundwork for subsequent examinations concerning the function of IRF4. To evaluate the prognostic and diagnostic potential of IRF4 in pan-cancer, Kaplan–Meier (K–M) survival curves and receiver operating characteristic (ROC) curves were employed. IRF4 exhibited moderate to high predictive accuracy (AUC > 0.7) for eight cancer types, with particularly high accuracy (AUC > 0.9) in identifying COAD and READ. Additionally, IRF4 expression was significantly correlated with OS and PFI in COAD, READ, and CESC. These results suggest that IRF4 plays a critical role in the diagnosis and prognosis of these malignancies and may serve as a valuable biomarker or therapeutic target for precision oncology. To further elucidate the functional implications of IRF4, pathway enrichment analysis was conducted using GO and KEGG on 50 IRF4-targeted binding proteins. The analysis revealed that IRF4 is involved in BP critical to viral defense responses, while its MF is associated with DNA-binding transcription factor activity. Key pathways enriched included those related to measles, hepatitis C, and influenza A. Intriguingly, beyond its role in transcriptional activation, IRF4 also plays a pivotal role in immune modulation, highlighting its multifaceted involvement in cancer biology.
The findings outlined above demonstrate that IRF4 holds significant diagnostic and prognostic value in CRC, encompassing both COAD and READ. To further explore the role of IRF4 in CRC, we examined its expression in relation to various clinical parameters, including the N, M, and TNM stages, histological type, neoplasm type, and history of colon polyps. Our analysis revealed a strong correlation between IRF4 expression and these clinical features. Subsequent evaluation of clinical subgroups within CRC indicated that elevated IRF4 expression was associated with improved OS and PFI. However, this correlation was specifically observed in subgroups characterized by the following criteria: age > 65 years, CEA levels > 5, adenocarcinoma of the colon and rectum, presence or absence of colon polyps, absence of lymphatic invasion, N0, M0, T3, T4, and stage III–IV. These findings suggest that the prognostic significance of IRF4 may be context-dependent, highlighting its potential utility in specific clinical subsets of CRC patients.
To further substantiate the potential diagnostic and prognostic significance of IRF4 in CRC, we investigated the relationships between IRF4 expression and clinical features in CRC cases using TMA and cDNA microarray analyses. Our findings revealed that IRF4 is predominantly expressed in TILs within the tumor stroma, rather than in parenchymal tumor cells. This observation aligns with our previous research findings.26 A deeper analysis of the association between IRF4 expression in TILs and patient prognosis demonstrated that CRC patients with elevated IRF4 protein levels tended to have a poorer prognosis. These results underscore the potential role of IRF4 as a prognostic marker in CRC and highlight its differential expression within the tumor microenvironment.
A major obstacle to tumor regression is the suppression of antitumor immune responses within TME. Regulatory CD4+ T cells (Tregs), an immunosuppressive cell population, play a critical role in inhibiting tumor rejection. In contrast, antitumor immune cells, such as T cells and natural killer (NK) cells, often infiltrate tumor tissues, and their presence is associated with improved prognosis in certain malignancies.24 However, Tregs, which are frequently overrepresented in cancers, actively suppress antitumor immunity. IRF4+ Tregs secrete a variety of immunosuppressive factors and are linked to the presence of multiple exhausted T cell populations.24 Furthermore, elevated IRF4 expression contributes to T cell exhaustion, including the upregulation of inhibitory receptors.27 In addition to Tregs, Tfh and B cells also infiltrate tumors. Through their interactions with Tfh cells in the TME, B cells can influence tumor progression.28–30 IRF4 is known to regulate the development and function of T and B cells, among other immune cells, highlighting its critical role in immuno-oncology.15,31–33 In this study, we identified high IRF4 expression as a negative prognostic factor for OS in CRC. IRF4 was predominantly expressed in tumor-infiltrating lymphocytes within the CRC stroma, likely in Tregs, and was implicated in signaling pathways related to tumor immunity. These findings suggest that IRF4 has strong predictive value and could serve as a valuable therapeutic biomarker for tumor-induced immune regulation in CRC. Investigating the underlying mechanisms of IRF4's role in the TME may provide new insights into potential therapeutic targets for CRC.
Our study has clinical significance, but several limitations still remain. Firstly, the sample sizes of clinical specimens and the TCGA database are in no way inadequate, so further information is required to further validate its accuracy. Secondly, although we performed IRF4 expression and survival analysis based on cDNA and paraffin-embedded TMA assays, more functional experiments are needed to determine the role and mechanism of IRF4 in the TME concerning B cells, T cells, Tregs, and other immune cells. Thirdly, in recent years, machine learning technology and deep learning have been widely used in fields of bioinformatics analysis, such as analysing single-cell multiomics data,34 computational toxicology,35–38 metabolite-disease relationships prediction,39,40 interaction prediction between miRNA and lncRNA or drugs,41–43 remote health monitoring44 and other interaction prediction problems.45 These investigations provide strong support for computational models correlations mRNA with diagnosis and prognosis. In the future, we will also learn and apply machine learning and deep learning technologies to build appropriate models to further analyze the diagnostic and immunological predict role of IRF4 in pan-cancers.
Conclusion
The identification of IRF4's prognostic and diagnostic significance across various cancers, particularly in CRC, positions it as a valuable diagnostic and immunological predictor for CRC. This insight also provides a novel perspective on potential strategies for CRC immunotherapy.
Materials and methods
Analysis of gene expression
The TCGA database was searched for RNA-seq and clinical information about 15
776 samples, an assortment of 33 tumor types and healthy tissues,27,46 the GGTEx database (https://xenabrowser.net/datapages/) at the UCSC Xena server,47–49 and the Xiantao platform (https://www.xiantaozi.com/). The CCLE database, available at https://portals.broadinstitute.org/ccle/, provided the tumor cell line data.50 For visualization purposes, the ggplot2 application was utilized, whereas R software version 4.2.1 was utilized to perform the statistical analysis. The Wilcoxon rank sum test identified two data sets, with p-values < 0.05 considered significant.51
Analysis of immunological subtypes and molecular subtypes
Using the TISIDB database (http://cis.hku.hk/TISIDB/index.php),52 which integrates diverse data sources to assess the interplay between malignancies and the immune system, we investigated the correlations between molecular or immunological subtypes and IRF4 expression in pan-cancer. Using information from the TISIDB database, we also investigated the connections between immunomodulators and IRF4 expression in pan-cancer.
Network analysis of protein–protein interactions
We retrieved 50 IRF4-binding proteins from the STRING site (https://string-db.org) by specifying the primary parameters as follows: active interaction sources (“Experiments, Text mining, and Databases”) and minimum needed interaction score (“medium confidence [0.400]”).53 Cytoscape (version 3.7.2, http://chianti.ucsd.edu/cytoscape-3.7.2/) was used after the PPI network was visualized.54
Functional annotation of differentially expressed genes
For 50 IRF4-binding proteins, KEGG and GO enrichment analyses were performed using the cluster Profiler software for statistical analysis and the ggplot2 package for visualization.55,56
Diagnostic value analysis
To evaluate the diagnostic efficacy of IRF4 in patients diagnosed with pan-cancer, the ROC curve was utilized. The AUC ranges from 0.5 to 1. The AUC is closer to 1 the better the diagnostic impact. High, certain, and low accuracy were indicated by AUC values of 0.5–0.7, 0.7–0.9, and >0.9, respectively.
Survival analysis
From the TCGA dataset, clinical and RNA expression data were extracted to perform a survival analysis. K–M plots were utilized to evaluate the relationship between IRF4 expression and prognosis in different malignancies and clinical subgroups of CRC. Visualization and statistical analysis were performed with the survminer and survival package, respectively. A p-value < 0.05 in the Cox regression hypothesis test demonstrated statistical significance.
Clinical characteristic analysis in CRC
Tables and box plots representing the IRF4 levels of patients with various CRC clinical features were displayed. The level 3 HTSeq-fragments per kilobase per million (FPKM) formats including the RNA-seq data and related clinical information were obtained by accessing the TCGA database. The data was then converted to transcripts per million reads (TPM) format for analysis following log 2 conversion. Two different sets of data were identified using the Wilcoxon rank sum test; a p-value < 0.05 denoted statistical significance (ns, p > 0.05; *, p < 0.05; **, p < 0.01; ***, p < 0.001).
Tissue microarrays
Primary CRC tumor tissues and matched tumor-margin tissues (MecDNA-HColA095Su01, 96*R100-M-20220223, Outdo biotech Co., Ltd, Shanghai, China) were used to generate paraffin-embedded TMA (HColA180Su17, Outdo biotech Co., Ltd, Shanghai, China) and cDNA microarrays to measure the IRF4 levels in CRC tissues. Between June 2007 and April 2008, tissue samples from 101 patients with stages I–IV CRC were collected for IHC from formalin-fixed paraffin-embedded tissues. Using pathological examination, the study group included patients with CRC who exhibited characteristics typical of squamous cell carcinoma. Tables 2 and 3 list the detailed clinicopathological characteristics of the patients. The term “follow-up period” refers to the period from the date of surgery to the date of death or the final follow-up. OS was the amount of time that passed between the patient's date of surgery and either their last follow-up appointment or their death from CRC. The American Joint Committee on Cancer Staging Manual, seventh edition, was used to categorize the TNM staging system. Each patient signed written informed consent, and authorized by the Ethics Committee of the First Affiliated Hospital of Chongqing Medical University (2022-X308).
Table 4 Univariate and multivariate analyses of the factors correlated with Overall survival of colorectal cancer
Variables |
Univariate analysis |
Multivariate analysis |
HR |
95% CI |
p value |
HR |
95% CI |
p value |
Lower-bound |
Upper-bound |
Lower-bound |
Upper-bound |
Expression |
1.682 |
0.711 |
3.977 |
0.237 |
|
|
|
|
Sex |
0.992 |
0.566 |
1.739 |
0.977 |
|
|
|
|
Age |
1.302 |
0.742 |
2.282 |
0.358 |
|
|
|
|
Tumor_size |
0.979 |
0.542 |
1.769 |
0.943 |
|
|
|
|
T |
1.566 |
0.813 |
3.017 |
0.18 |
|
|
|
|
M |
1.671 |
0.23 |
12.158 |
0.612 |
|
|
|
|
Grade |
1.795 |
0.869 |
3.705 |
0.114 |
|
|
|
|
TNM |
2.511 |
1.427 |
4.42 |
0.00141 |
2.51 |
1.43 |
4.42 |
0.00141 |
MSI |
1.622 |
0.921 |
2.857 |
0.0939 |
|
|
|
|
PDL1_1 |
1.325 |
0.752 |
2.335 |
0.33 |
|
|
|
|
PDL1_2 |
0.725 |
0.409 |
1.284 |
0.27 |
|
|
|
|
Table 5 Univariate and multivariate analyses of the factors correlated with overall survival of colorectal cancer
Variables |
COAD multivariate analysis |
READ multivariate analysis |
HR |
95% CI |
p
|
HR |
95% CI |
p
|
Lower-bound |
Upper-bound |
Lower-bound |
Upper-bound |
Stage2 |
1.819 |
0.687 |
4.816 |
0.228 |
0.673 |
0.121 |
3.742 |
0.651 |
Stage3 |
3.961 |
1.492 |
10.516 |
0.006** |
1.164 |
0.222 |
6.115 |
0.857 |
Stage4 |
11.192 |
4.145 |
30.215 |
0.000*** |
4.281 |
0.826 |
22.183 |
0.083 |
Age |
1.041 |
1.022 |
1.061 |
0.000 *** |
1.095 |
1.035 |
1.159 |
0.002** |
Gender male |
0.978 |
0.643 |
1.487 |
0.916 |
1.146 |
0.447 |
2.938 |
0.776 |
B cell |
13.410 |
0.100 |
1795.559 |
0.299 |
6484.640 |
0.005 |
8.653839 × 109 |
0.223 |
CD8_Tcell |
0.014 |
0.000 |
0.712 |
0.033* |
0.003 |
0.000 |
1 455 622 |
0.572 |
CD4_Tcell |
0.313 |
0.002 |
44.897 |
0.647 |
116.937 |
0.000 |
6.514602 × 1012 |
0.706 |
Macrophage |
14.735 |
0.113 |
1917.711 |
0.279 |
0.001 |
0.000 |
4665.174 |
0.375 |
Neutrophil |
0.045 |
0.000 |
81.335 |
0.419 |
1431.307 |
0.000 |
7.589990 × 1015 |
0.627 |
Dendritic |
7.042 |
0.335 |
147.842 |
0.209 |
21 822.091 |
0.631 |
7.549702 × 108 |
0.061 |
IRF4 |
0.923 |
0.641 |
1.328 |
0.665 |
0.190 |
0.042 |
86.5 |
0.032* |
IHC staining
IHC was employed to quantify the proteins produced by specific genes in formalin-fixed, paraffin-embedded samples from normal colorectal and CRC tissues. Following xylene deparaffinization, ethanol was used to dehydrate the tissue sections. Goat serum was utilized to block the area for an hour after antigen extraction using citrate buffer at pH 6 to prevent non-specific antibody binding. The samples were then incubated in solutions containing secondary antibodies after being exposed to solutions containing particular primary antibodies (IRF4, 1
:
500, Abcam, and ab133590). The sections were finally evaluated after the application of 3,3′-diaminobenzidine staining 5.57,58
Evaluation of immunostaining
Plasma cells expressed IRF4 constitutively; it was predominantly detected in stromal immune infiltration. The proportion of stromal surface area colonized by IRF4-positive plasma cells was investigated. The guidelines for the assessment of TILs developed by the International TILs Working Group state that stained sections were assessed blindly and without reference to clinical data.28,59 The following method was used to assess the IRF4-positive infiltrate's intensity: there are four levels of IRF4-positive infiltrate: 0 indicates no infiltrate; 1+ indicates a weak infiltrate (∼10–20% of IRF4-positive plasma cell-occupied stromal surface); 2+ indicates a moderate infiltration (∼20–30% of IRF4-positive plasma cell-occupied stromal surface); and 3+ indicates a strong infiltration (>30% of plasma cell-occupied stromal surface). A patient was identified with “low IRF4 expression” if their IRF4 score was 0 or 1+, and with “high IRF4 expression” if it was 2+ or 3+. When disparities were found in the individual pathologists' opinions, the cases were reevaluated in conjunction with additional pathologists to come to a consensus.
Quantitative real-time polymerase chain reaction (q-PCR)
Using Generay, we created and manufactured qPCR primers (Shanghai, China). Using Hiscript1 III RT SuperMix for qPCR (+gDNA wiper) (Vazyme, R312-01), total RNA was transformed into cDNA. The internal control GAPDH and the relative mRNA expression level were measured with an ABI PrismR 7900HT Real-Time PCR System (Applied Biosystems). Three independent experiments were performed with data analyzed using the 2−ΔΔCt technique. Table 6 displays the primer sequences used in qPCR.
Table 6 List of qPCR primers used in this study
PCR |
Primer |
Sequence (5′–3′) |
Product size (bp) |
Annealing temperature (°C) |
|
IRF4F |
AGCGAGGGCATAAATACAG |
272bp |
60 |
IRF4R |
ACAGAGGACTTGGGGAGATA |
|
|
ActinF |
GTCTTCCCCTCCATCGTG |
113bp |
55 |
ActinR |
AGGGTGAGGATGCCTCTCTT |
|
|
Tumor filtrating immune cell analysis
TIMER (https://cistrome.shinyapps.io/timer/)60 and TISIDB (http://cis.hku.hk/TISIDB/)52 were used to link tumor-infiltrating cells with IRF4 expression. Download the corresponding single-cell data in .h5 format and annotation results from TISCH. Use the R software MAESTRO and Seurat to process and analyze the single-cell data. Re-cluster the cells using the t-SNE method.20 We downloaded STAR-counts data and corresponding clinical information for XX tumors from the TCGA database (https://portal.gdc.cancer.gov). We then extracted data in TPM format and performed normalization using the log 2(TPM + 1) transformation. After retaining samples that included both RNAseq data and clinical information, we ultimately selected 600 CRC samples for further analysis. To conduct a reliable immune score assessment, we used immunedeconv, an R package that integrates six state-of-the-art algorithms, including TIMER, xCell, MCP-counter, CIBERSORT, EPIC, and quanTIseq. We used the R package ggClusterNet for analysis and visualization of the results. All the above analysis methods and R packages were performed using R software version v4.1.3 (R Foundation for Statistical Computing, 2022). p < 0.05 was considered statistically significant.
Statement
The First Affiliated Hospital of Chongqing Medical University approved the experiments, including any relevant details. And all experiments were performed in accordance with relevant guidelines and regulations.
Statistical analysis
Using GraphPad Prism 9 and R software 4.2.1, the experimental results were statistically evaluated and given as mean ± standard. On the basis of median gene expression, TCGA database samples were categorized as either high- or low-expression. For mRNAs, survival curves using the K–M approach and the log-rank test were created. A statistical significance was indicated by a p-value threshold of <0.05.
Abbreviations
TCGA | The cancer genome atlas |
GTEx | Genotype-tissue expression |
CCLE | Cancer cell line encyclopedia |
PPI | Protein–protein interaction |
GO | Gene ontology |
KEGG | Kyoto encyclopedia of genes and genomes |
ROC | Receiver operating characteristic |
BP | Biological process |
MF | Molecular function |
CC | Cellular component |
ROC | Receiver operating characteristic |
AUC | Area under the curve |
OS | Overall survival |
PFI | Progression-free interval |
TPM | Transcripts per million reads |
FPKM | Fragments per kilobase per million |
FC | Foldchange |
ACC | Adrenocortical carcinoma |
BLCA | Bladder urothelial carcinoma |
BRCA | Breast invasive carcinoma |
CESC | Cervical squamous cell carcinoma and endocervical adenocarcinoma |
CHOL | Cholangiocarcinoma |
COAD | Colon adenocarcinoma |
DLBC | Lymphoid neoplasm diffuse large B-cell lymphoma |
ESCA | Esophageal carcinoma |
GBM | Glioblastoma multiforme |
HNSC | Head and neck squamous cell carcinoma |
KICH | Kidney chromophobe |
KIRC | Kidney renal clear cell carcinoma |
KIRP | Kidney renal papillary cell carcinoma |
LAML | Acute myeloid leukemia |
LGG | Brain lower-grade glioma |
LIHC | Liver hepatocellular carcinoma |
LUAD | Lung adenocarcinoma |
LUSC | Lung squamous cell carcinoma |
OV | Ovarian serous cystadenocarcinoma |
PAAD | Pancreatic adenocarcinoma |
PRAD | Prostate adenocarcinoma |
READ | Rectum adenocarcinoma |
SARC | Sarcoma |
SKCM | Skin cutaneous melanoma |
STAD | Stomach adenocarcinoma |
TGCT | Testicular germ cell tumors |
THCA | Thyroid carcinoma |
THYM | Thymoma |
UCEC | Uterine corpus endometrial carcinoma |
UCS | Uterine carcinosarcoma |
UVM | Uveal melanoma |
PPI | Protein–protein interaction |
IHC | Immunohistochemical staining |
qPCR | Quantitative real-time PCR |
TMA | Tissue microarray |
IRF4 | Interferon regulating factor |
IRFs | Interferon regulating factors |
Th2 | T helper 2 |
Tfh | T follicular helper |
eTreg | Effector regulatory T |
Ethical statement
This study was performed in line with the principles of the Declaration of Helsinki. Approval was granted by the Ethics Committee of Shanghai Outdo Biotech Company (No. YB M-05-02).
Data availability
The datasets generated during and analysed during the current study are available from the corresponding author on reasonable request.
Author contributions
All authors contributed to the study conception and design. RS and FT contributed to the conception and design of the study. YT organized the database. YY, ZX and MZ performed the statistical analysis. YX, MD, LH, NL, YW, and YZ conducted the experiments and analyzed the data. YT, YY, ZX and MZ wrote the sections of the manuscript. YT, RS, and FT reviewed the data and finalized the manuscript. All authors commented on previous versions of the manuscript. All authors read and approved the submitted version.
Conflicts of interest
The authors have no relevant financial interests to disclose.
Acknowledgements
This work was supported by the Postdoctoral Special Foundation of Chongqing (2021XM2011), Science and Technology Planning Foundation of Jiulongpo District, Chongqing (2024-04-001-Z), and China Health Promotion Foundation (XH-B026).
References
- M. Trzpis, P. M. McLaughlin, L. M. de Leij and M. C. Harmsen, Am. J. Pathol., 2007, 171, 386–395 CrossRef PubMed.
- D. Maetzel, S. Denzel, B. Mack, M. Canis, P. Went, M. Benk, C. Kieu, P. Papior, P. A. Baeuerle, M. Munz and O. Gires, Nat. Cell Biol., 2009, 11, 162–171 Search PubMed.
- T. M. Gorges, I. Tinhofer, M. Drosch, L. Rose, T. M. Zollner, T. Krahn and O. von Ahsen, BMC Cancer, 2012, 12, 178 CrossRef PubMed.
- M. Munz, P. A. Baeuerle and O. Gires, Cancer Res., 2009, 69, 5627–5629 CrossRef PubMed.
- S. L. Topalian, F. S. Hodi, J. R. Brahmer, S. N. Gettinger, D. C. Smith, D. F. McDermott, J. D. Powderly, R. D. Carvajal, J. A. Sosman, M. B. Atkins, P. D. Leming, D. R. Spigel, S. J. Antonia, L. Horn, C. G. Drake, D. M. Pardoll, L. Chen, W. H. Sharfman, R. A. Anders, J. M. Taube, T. L. McMiller, H. Xu, A. J. Korman, M. Jure-Kunkel, S. Agrawal, D. McDonald, G. D. Kollia, A. Gupta, J. M. Wigginton and M. Sznol, N. Engl. J. Med., 2012, 366, 2443–2454 Search PubMed.
- E. B. Garon, N. A. Rizvi, R. Hui, N. Leighl, A. S. Balmanoukian, J. P. Eder, A. Patnaik, C. Aggarwal, M. Gubens, L. Horn, E. Carcereny, M. J. Ahn, E. Felip, J. S. Lee, M. D. Hellmann, O. Hamid, J. W. Goldman, J. C. Soria, M. Dolled-Filhart, R. Z. Rutledge, J. Zhang, J. K. Lunceford, R. Rangwala, G. M. Lubiniecki, C. Roach, K. Emancipator, L. Gandhi and K.-. Investigators, N. Engl. J. Med., 2015, 372, 2018–2028 CrossRef PubMed.
- M. Reck, D. Rodriguez-Abreu, A. G. Robinson, R. Hui, T. Csoszi, A. Fulop, M. Gottfried, N. Peled, A. Tafreshi, S. Cuffe, M. O'Brien, S. Rao, K. Hotta, M. A. Leiby, G. M. Lubiniecki, Y. Shentu, R. Rangwala, J. R. Brahmer and K.-. Investigators, N. Engl. J. Med., 2016, 375, 1823–1833 CrossRef PubMed.
- T. Tamura, H. Yanai, D. Savitsky and T. Taniguchi, Annu. Rev. Immunol., 2008, 26, 535–584 CrossRef PubMed.
- Y. J. Chen, J. Li, N. Lu and X. Z. Shen, Int. Immunopharmacol., 2017, 49, 1–5 Search PubMed.
- X. Jin, S. H. Kim, H. M. Jeon, S. Beck, Y. W. Sohn, J. Yin, J. K. Kim, Y. C. Lim, J. H. Lee, S. H. Kim, S. H. Kang, X. Pian, M. S. Song, J. B. Park, Y. S. Chae, Y. G. Chung, S. H. Lee, Y. J. Choi, D. H. Nam, Y. K. Choi and H. Kim, Brain, 2012, 135, 1055–1069 Search PubMed.
- L. Tarassishin and S. C. Lee, J Neurooncol., 2013, 113, 185–194 CrossRef PubMed.
- J. Liang, Y. Piao, V. Henry, N. Tiao and J. F. de Groot, Oncotarget, 2015, 6, 31479–31492 Search PubMed.
- N. Jacquelot, T. Yamazaki, M. P. Roberti, C. P. M. Duong, M. C. Andrews, L. Verlingue, G. Ferrere, S. Becharef, M. Vetizou, R. Daillere, M. Messaoudene, D. P. Enot, G. Stoll, S. Ugel, I. Marigo, S. Foong Ngiow, A. Marabelle, A. Prevost-Blondel, P. O. Gaudreau, V. Gopalakrishnan, A. M. Eggermont, P. Opolon, C. Klein, G. Madonna, P. A. Ascierto, A. Sucker, D. Schadendorf, M. J. Smyth, J. C. Soria, G. Kroemer, V. Bronte, J. Wargo and L. Zitvogel, Cell Res., 2019, 29, 846–861 CrossRef PubMed.
- J. L. Benci, B. Xu, Y. Qiu, T. J. Wu, H. Dada, C. Twyman-Saint Victor, L. Cucolo, D. S. M. Lee, K. E. Pauken, A. C. Huang, T. C. Gangadhar, R. K. Amaravadi, L. M. Schuchter, M. D. Feldman, H. Ishwaran, R. H. Vonderheide, A. Maity, E. J. Wherry and A. J. Minn, Cell, 2016, 167, 1540–1554 CrossRef PubMed.
- M. Huber and M. Lohoff, Eur. J. Immunol., 2014, 44, 1886–1895 CrossRef PubMed.
- A. Grossman, H. W. Mittrucker, J. Nicholl, A. Suzuki, S. Chung, L. Antonio, S. Suggs, G. R. Sutherland, D. P. Siderovski and T. W. Mak, Genomics, 1996, 37, 229–233 CrossRef PubMed.
- R. Sun, L. Ye, M. Zhang, Z. Qiu, T. Xiang, J. Tang, X. Wang, L. Li, J. Luo, D. Zhang and G. Ren, Biochem. Biophys. Res. Commun., 2018, 506, 685–691 CrossRef PubMed.
- M. Deng and G. Q. Daley, Blood, 2001, 97, 3491–3497 CrossRef PubMed.
- E. Fratta, L. Sigalotti, A. Covre, G. Parisi, S. Coral and M. Maio, Immunotherapy, 2013, 5, 1103–1116 CrossRef PubMed.
- Y. Han, Y. Wang, X. Dong, D. Sun, Z. Liu, J. Yue, H. Wang, T. Li and C. Wang, Nucleic Acids Res., 2023, 51, D1425–D1431 CrossRef PubMed.
- L. Xu, C. Deng, B. Pang, X. Zhang, W. Liu, G. Liao, H. Yuan, P. Cheng, F. Li, Z. Long, M. Yan, T. Zhao, Y. Xiao and X. Li, Cancer Res., 2018, 78, 6575–6580 CrossRef PubMed.
- D. Aran, M. Sirota and A. J. Butte, Nat. Commun., 2015, 6, 8971 CrossRef PubMed.
- J. M. Connett, L. Badri, T. J. Giordano, W. C. Connett and G. M. Doherty, J. Interferon Cytokine Res., 2005, 25, 587–594 CrossRef PubMed.
- A. S. Heimes, M. Schmidt, J. Jakel, K. Almstedt, S. Gebhard, V. Weyer-Eiberich, T. Elger, S. Krajnak, W. Brenner, A. Hasenburg and M. J. Battista, Arch. Gynecol. Obstet., 2019, 299, 239–246 CrossRef PubMed.
- Q. Gao, H. Zhu, L. Dong, W. Shi, R. Chen, Z. Song, C. Huang, J. Li, X. Dong, Y. Zhou, Q. Liu, L. Ma, X. Wang, J. Zhou, Y. Liu, E. Boja, A. I. Robles, W. Ma, P. Wang, Y. Li, L. Ding, B. Wen, B. Zhang, H. Rodriguez, D. Gao, H. Zhou and J. Fan, Cell, 2019, 179, 561–577 CrossRef PubMed.
- K. Yoshihara, M. Shahmoradgoli, E. Martinez, R. Vegesna, H. Kim, W. Torres-Garcia, V. Trevino, H. Shen, P. W. Laird, D. A. Levine, S. L. Carter, G. Getz, K. Stemke-Hale, G. B. Mills and R. G. Verhaak, Nat. Commun., 2013, 4, 2612 Search PubMed.
- G. F. Gao, J. S. Parker, S. M. Reynolds, T. C. Silva, L. B. Wang, W. Zhou, R. Akbani, M. Bailey, S. Balu, B. P. Berman, D. Brooks, H. Chen, A. D. Cherniack, J. A. Demchok, L. Ding, I. Felau, S. Gaheen, D. S. Gerhard, D. I. Heiman, K. M. Hernandez, K. A. Hoadley, R. Jayasinghe, A. Kemal, T. A. Knijnenburg, P. W. Laird, M. K. A. Mensah, A. J. Mungall, A. G. Robertson, H. Shen, R. Tarnuzzer, Z. Wang, M. Wyczalkowski, L. Yang, J. C. Zenklusen, Z. Zhang, N. Genomic Data Analysis, H. Liang and M. S. Noble, Cell Syst., 2019, 9, 24–34 Search PubMed.
- S. Adams, L. J. Goldstein, J. A. Sparano, S. Demaria and S. S. Badve, Oncoimmunology, 2015, 4, e985930 Search PubMed.
- G. Alvisi, J. Brummelman, S. Puccio, E. M. Mazza, E. P. Tomada, A. Losurdo, V. Zanon, C. Peano, F. S. Colombo, A. Scarpa, M. Alloisio, A. Vasanthakumar, R. Roychoudhuri, M. Kallikourdis, M. Pagani, E. Lopci, P. Novellis, J. Blume, A. Kallies, G. Veronesi and E. Lugli, J. Clin. Invest., 2020, 130, 3137–3150 Search PubMed.
- N. Gutierrez-Melo and D. Baumjohann, Trends Cancer, 2023, 9, 309–325 CrossRef PubMed.
- R. Sciammas, A. L. Shaffer, J. H. Schatz, H. Zhao, L. M. Staudt and H. Singh, Immunity, 2006, 25, 225–236 CrossRef PubMed.
- D. Finlay, Immunol. Cell Biol., 2014, 92, 6–7 CrossRef CAS PubMed.
- S. L. Cook, M. C. Franke, E. P. Sievert and R. Sciammas, Trends Immunol., 2020, 41, 614–628 Search PubMed.
- H. Hu, Z. Feng, H. Lin, J. Cheng, J. Lyu, Y. Zhang, J. Zhao, F. Xu, T. Lin, Q. Zhao and J. Shuai, Comput. Biol. Med., 2023, 157, 106733 Search PubMed.
- X. Yang, J. Sun, B. Jin, Y. Lu, J. Cheng, J. Jiang, Q. Zhao and J. Shuai, J. Adv. Res., 2025, 68, 477–489 Search PubMed.
- Z. Chen, L. Zhang, J. Sun, R. Meng, S. Yin and Q. Zhao, J. Cell. Mol. Med., 2023, 27, 3117–3126 Search PubMed.
- T. Wang, J. Sun and Q. Zhao, Comput. Biol. Med., 2023, 153, 106464 CrossRef PubMed.
- J. Wang, L. Zhang, J. Sun, X. Yang, W. Wu, W. Chen and Q. Zhao, Methods, 2024, 221, 18–26 CrossRef PubMed.
- H. Gao, J. Sun, Y. Wang, Y. Lu, L. Liu, Q. Zhao and J. Shuai, Briefings Bioinf., 2023, 24, bbad259 Search PubMed.
- F. Sun, J. Sun and Q. Zhao, Briefings Bioinf., 2022, 23, bbac266 Search PubMed.
- W. Wang, L. Zhang, J. Sun, Q. Zhao and J. Shuai, Briefings Bioinf., 2022, 23, bbac463 CrossRef PubMed.
- S. Yin, P. Xu, Y. Jiang, X. Yang, Y. Lin, M. Zheng, J. Hu and Q. Zhao, J. Cell. Mol. Med., 2024, 28, e18591 CrossRef PubMed.
- J. Xie, P. Xu, Y. Lin, M. Zheng, J. Jia, X. Tan, J. Sun and Q. Zhao, J. Cell. Mol. Med., 2024, 28, e18590 CrossRef PubMed.
- F. Zhu, Q. Niu, X. Li, Q. Zhao, H. Su and J. Shuai, Research, 2024, 7, 0361 CrossRef PubMed.
- L. Liu, Y. Wei, Q. Zhang and Q. Zhao, IEEE J. Biomed. Health Inform., 2024, 28, 1762–1772 Search PubMed.
- N. Cancer Genome Atlas Research, J. N. Weinstein, E. A. Collisson, G. B. Mills, K. R. Shaw, B. A. Ozenberger, K. Ellrott, I. Shmulevich, C. Sander and J. M. Stuart, Nat. Genet., 2013, 45, 1113–1120 Search PubMed.
- G. T. Consortium, Nat. Genet., 2013, 45, 580–585 Search PubMed.
- J. Vivian, A. A. Rao, F. A. Nothaft, C. Ketchum, J. Armstrong, A. Novak, J. Pfeil, J. Narkizian, A. D. Deran, A. Musselman-Brown, H. Schmidt, P. Amstutz, B. Craft, M. Goldman, K. Rosenbloom, M. Cline, B. O'Connor, M. Hanna, C. Birger, W. J. Kent, D. A. Patterson, A. D. Joseph, J. Zhu, S. Zaranek, G. Getz, D. Haussler and B. Paten, Nat. Biotechnol., 2017, 35, 314–316 Search PubMed.
- M. J. Goldman, B. Craft, M. Hastie, K. Repecka, F. McDade, A. Kamath, A. Banerjee, Y. Luo, D. Rogers, A. N. Brooks, J. Zhu and D. Haussler, Nat. Biotechnol., 2020, 38, 675–678 Search PubMed.
- M. Ghandi, F. W. Huang, J. Jane-Valbuena, G. V. Kryukov, C. C. Lo, E. R. McDonald III, J. Barretina, E. T. Gelfand, C. M. Bielski, H. Li, K. Hu, A. Y. Andreev-Drakhlin, J. Kim, J. M. Hess, B. J. Haas, F. Aguet, B. A. Weir, M. V. Rothberg, B. R. Paolella, M. S. Lawrence, R. Akbani, Y. Lu, H. L. Tiv, P. C. Gokhale, A. de Weck, A. A. Mansour, C. Oh, J. Shih, K. Hadi, Y. Rosen, J. Bistline, K. Venkatesan, A. Reddy, D. Sonkin, M. Liu, J. Lehar, J. M. Korn, D. A. Porter, M. D. Jones, J. Golji, G. Caponigro, J. E. Taylor, C. M. Dunning, A. L. Creech, A. C. Warren, J. M. McFarland, M. Zamanighomi, A. Kauffmann, N. Stransky, M. Imielinski, Y. E. Maruvka, A. D. Cherniack, A. Tsherniak, F. Vazquez, J. D. Jaffe, A. A. Lane, D. M. Weinstock, C. M. Johannessen, M. P. Morrissey, F. Stegmeier, R. Schlegel, W. C. Hahn, G. Getz, G. B. Mills, J. S. Boehm, T. R. Golub, L. A. Garraway and W. R. Sellers, Nature, 2019, 569, 503–508 Search PubMed.
- S. Tabaries and P. M. Siegel, Oncogene, 2017, 36, 1176–1190 Search PubMed.
- B. Ru, C. N. Wong, Y. Tong, J. Y. Zhong, S. S. W. Zhong, W. C. Wu, K. C. Chu, C. Y. Wong, C. Y. Lau, I. Chen, N. W. Chan and J. Zhang, Bioinformatics, 2019, 35, 4200–4202 CrossRef PubMed.
- D. Szklarczyk, A. L. Gable, D. Lyon, A. Junge, S. Wyder, J. Huerta-Cepas, M. Simonovic, N. T. Doncheva, J. H. Morris, P. Bork, L. J. Jensen and C. V. Mering, Nucleic Acids Res., 2019, 47, D607–D613 Search PubMed.
- P. Shannon, A. Markiel, O. Ozier, N. S. Baliga, J. T. Wang, D. Ramage, N. Amin, B. Schwikowski and T. Ideker, Genome Res., 2003, 13, 2498–2504 Search PubMed.
- A. Subramanian, P. Tamayo, V. K. Mootha, S. Mukherjee, B. L. Ebert, M. A. Gillette, A. Paulovich, S. L. Pomeroy, T. R. Golub, E. S. Lander and J. P. Mesirov, Proc. Natl. Acad. Sci. U. S. A., 2005, 102, 15545–15550 Search PubMed.
- G. Yu, L. G. Wang, Y. Han and Q. Y. He, OMICS, 2012, 16, 284–287 CrossRef PubMed.
- R. Sun, J. He, Q. Xiang, Y. Feng, Y. Gong, Y. Ning, C. Deng, K. Sun, M. Zhang, Z. Cheng, X. Le, Q. Xiong, F. Dai, Y. Wu and T. Xiang, Int. J. Biol. Sci., 2023, 19, 641–657 Search PubMed.
- R. Sun, T. Xiang, J. Tang, W. Peng, J. Luo, L. Li, Z. Qiu, Y. Tan, L. Ye, M. Zhang, G. Ren and Q. Tao, Theranostics, 2020, 10, 2243–2259 CrossRef PubMed.
- R. Salgado, C. Denkert, S. Demaria, N. Sirtaine, F. Klauschen, G. Pruneri, S. Wienert, G. Van den Eynden, F. L. Baehner, F. Penault-Llorca, E. A. Perez, E. A. Thompson, W. F. Symmans, A. L. Richardson, J. Brock, C. Criscitiello, H. Bailey, M. Ignatiadis, G. Floris, J. Sparano, Z. Kos, T. Nielsen, D. L. Rimm, K. H. Allison, J. S. Reis-Filho, S. Loibl, C. Sotiriou, G. Viale, S. Badve, S. Adams, K. Willard-Gallo, S. Loi and T. W. G. International, Ann. Oncol., 2015, 26, 259–271 CrossRef PubMed.
- T. Li, J. Fan, B. Wang, N. Traugh, Q. Chen, J. S. Liu, B. Li and X. S. Liu, Cancer Res., 2017, 77, e108–e110 Search PubMed.
|
This journal is © The Royal Society of Chemistry 2025 |
Click here to see how this site uses Cookies. View our privacy policy here.