DOI:
10.1039/D4AN00967C
(Paper)
Analyst, 2025,
150, 353-361
Reproducible protein quantitation of 270 human proteins at increased depth using nanoparticle-based fractionation and multiple reaction monitoring mass spectrometry with stable isotope-labelled internal standards†
Received
10th July 2024
, Accepted 9th November 2024
First published on 13th December 2024
Abstract
Here we show that when using a mix of 274 light synthetic peptide standards (NAT) as surrogates for 270 human plasma proteins, as well as stable isotope-labelled standards (SIS) as normalizers (both from MRM Proteomics Inc.) for targeted quantitative analysis by liquid chromatography multiple reaction monitoring mass spectrometry (LC/MRM-MS), the Seer Proteograph™ platform allowed for the enrichment and absolute quantitation of up to an additional 62 targets (median) compared to two standard proteomic workflows without enrichment, representing an increase of 44%. The nanoparticle-based fractionation workflow resulted in improved reproducibility compared to a traditional proteomic workflow with no fractionation (median 8.3% vs. 13.1% CV). As expected, the protein concentrations in nanoparticle coronas were higher and had more compressed dynamic range in comparison to the concentrations determined either by a 3-hour Trypsin/LysC or overnight tryptic digestion methods. As the nanoparticle-based fractionation technology gains popularity, additional steps such as establishing technique-specific protein reference ranges across plasma samples and comparisons to well-established protein quantitation methods like enzyme-linked immunosorbent assay (ELISA) and LC/MRM-MS may be explored to enable absolute quantification of plasma proteins based on nanoparticle-based fractionation data.
Introduction
Quantitation of plasma proteins by liquid chromatography multiple reaction monitoring mass spectrometry (LC/MRM-MS) based on proteotypic peptides as protein surrogates is a popular approach for preclinical and clinical researchers.1–5 This is due to its high multiplexity, specificity, precision, and dynamic range, as well as its sensitivity in the low ng mL−1 range and its absolute quantitation capability when using synthetic stable isotope-labelled standard (SIS) peptides as internal standards.6 Although plasma is the most readily used biofluid for diagnostics in proteomics,2,7 many protein targets are routinely below the lower limit of quantitation by LC/MRM-MS and even undetectable due to the natural complexity and wide dynamic range of protein concentrations in plasma.8,9
The Seer Proteograph™ nanoparticle-based platform (NP-based platform) has been used primarily for untargeted shotgun proteomics experiments sampling at the intact and native protein level (proteoforms) across the dynamic range of the plasma proteome measuring the relative abundance of proteins between different plasma samples by LC tandem mass spectrometry (MS/MS).10,11 Compared to conventional in-solution digestion protocols, the NP-based platform assay separates plasma proteins into different fractions using distinct types of functionalized nanoparticles (NPs)12 that form specific protein coronas – at NP surfaces driven by specific nano-bio interactions. At the time the study presented in this publication was performed, the NP-based platform made use of 5 NPs (NP1 to NP5), whereas the most recent iteration requires only two NP suspensions per sample.12 This fractionation reduces the wide dynamic range of plasma,13 resulting in deeper proteomic coverage of plasma proteins.
For this study, we focused on determining the reproducibility of protein quantitation from the NP-based platform multi-nanoparticle-processed samples using a validated MRM panel for absolute quantitation of 270 human plasma proteins (from MRM Proteomics, Inc.). The NP-based platform approach was compared with two standard approaches involving no protein fractionation, to determine whether the NP-based platform led to improved sensitivity and precision for absolute plasma protein quantitation compared to traditional methods (Fig. 1).
 |
| Fig. 1 Study workflow. Human plasma was treated in three ways: (1) separated into five nanoparticle-derived fractions using the NP-based platform and then digested with Trypsin/LysC, (2) digested as neat plasma using an overnight (ON) trypsin-only digestion protocol, and (3) digested as neat plasma using a 3-hour Trypsin/LysC protocol. All resulting sample digests were spiked with a mix of 274 stable isotope-labelled internal standard (SIS) peptides acting as surrogates for 270 human plasma proteins, followed by LC/MRM-MS analysis. | |
Experimental
Materials
Reagents and labware.
Phosphate buffered saline (PBS) tablets, Trizma pre-set crystals (pH 8.0), urea, dithiothreitol (DTT), and iodoacetamide (IAA) were purchased from Sigma Aldrich. Deep-well plates (1.1 mL) from AXYGEN were used for digestion. Protein LoBind tubes and LoBind 96-well PCR plates were purchased from Eppendorf. Oasis HLB μElution plates (2 mg sorbent per well, 30 μm particle size, Waters) were used for sample cleanup. Ultrapure water was generated with a Milli-Q Direct 8 water purification system. Formic acid (FA), methanol (MeOH) and acetonitrile (ACN), all LC-MS grade, were purchased from Fisher Scientific. Eppendorf protein LoBind tubes were used for preparing dilutions of the light (natural abundance, NAT) and stable isotope-labelled standard (SIS) peptide mixtures, which had been synthesized in-house.
Peptide mixtures.
The NAT and SIS peptide mixtures used were from MRM Proteomics’ PeptiQuant™ 270-protein human plasma MRM assay kits. The NAT peptide mixture was used for standard curve generation, and the SIS peptide mixture as the normalizer. The synthetic tryptic peptides contained in the two mixtures act as molecular surrogates for 270 human proteins and cover six orders of magnitude in protein concentration.6 The peptides had been selected as described previously6 and their purity and amount were determined by capillary zone electrophoresis (CZE) and amino acid analysis (AAA).14 The detectability for each peptide when spiked into human plasma had previously been established, and the ionization conditions had been optimized empirically. Additionally, the peptide assays had been validated following the Clinical Proteomic Tumor Analysis Consortium (CPTAC) guidelines for assay development15 which are available on the CPTAC Assay portal.16 Thus, the limit of detection (LOD) and linear range (lower limit of quantitation, LLOQ; upper limit of quantitation, ULOQ) had been previously established, along with interference screening for each peptide. The concentrations of the light peptide mixtures were balanced to the LLOQs that had been established for each peptide.
Human plasma digestion.
A commercially available human plasma sample PC5 (prepared by pooling four plasma pools purchased from ProMedDx, LLC) was stored at −80 °C before being processed in one of three ways: (1) NP-based automated sample enrichment/fractionation and digestion, for 16 replicates performed on the Proteograph SP100 Automation Instrument, (2) overnight (ON) digestion of neat plasma with trypsin-only done in triplicate at MRM Proteomics, (3) a 3-hour Trypsin/LysC digestion of neat plasma done once at Seer Inc.
Note that the term “neat” as used throughout this manuscript refers to plasma digests that did not undergo any NP-based fractionation (i.e., plasma digestion approaches 2 and 3).
Approach 1: enrichment and digestion on a NP-based platform.
Sixteen 250 μL aliquots of PC5 plasma were transferred to sample tubes for processing with the Proteograph Assay kit (S55R1100). Tubes were loaded onto the SP100 Automation Instrument for sample preparation to generate purified peptides for LC-MS analysis. Briefly, plasma was incubated with proprietary, physicochemically distinct nanoparticles for protein corona formation. After incubation, nanoparticles along with bound proteins were isolated using a magnet. A series of washes followed by reduction, alkylation, protein digestion with Trypsin/LysC, and peptide purification were performed prior to peptide elution with 142 μL of high-organic elution buffer.17,18 The purified peptide mix concentrations were determined using the Pierce Quantitative Fluorometric Peptide Assay (Thermo Fisher Scientific, catalog # 23290), a modified bicinchoninic acid (BCA) assay. Peptides were purified using solid phase extraction and the positive pressure (MPE2) system on the SP100 Automation Instrument. Peptides were dried down overnight with a vacuum concentrator. Dried peptides consisting of approximately 1–2 μg mixed peptide per fraction were stored at −80 °C until further processing. Of the 16 process replicates, five were randomly selected for further processing.
Approach 2: overnight digestion of neat plasma with trypsin only.
Three 10 μL aliquots of PC5 neat plasma were digested as described previously.6 Briefly, 10 μL of plasma was denatured and reduced with final concentrations of 6 M urea, 13 mM DTT and 200 mM TrisHCl (pH 8.0) at 37 °C for 30 minutes. Next, proteins were alkylated with 40 mM iodoacetamide (IAA) in the dark at room temperature for 30 minutes. The samples were diluted with 100 mM TrisHCl (pH 8.0) to <1 M urea, followed by overnight tryptic digestion at 37 °C using L-1-tosylamido-2-phenylethyl chloromethyl ketone (TPCK)-treated trypsin (Worthington) at a protein to enzyme ratio of 20
:
1 (w/w). The digestion reaction was quenched by adding FA to produce a final concentration of 1%.
Approach 3: 3-hour trypsin/LysC digestion of neat plasma.
An aliquot of PC5 neat plasma was denatured, reduced, alkylated, and subjected to proteolytic Trypsin/LysC digestion for 3 hours at 37 °C using the NP-based platform, but omitting the nanoparticle reagent.17,18 The resulting peptides were purified by solid phase extraction and yields were determined (Thermo Fisher Scientific catalog # 23290). A 2 μg mass aliquot was used for further processing (see below).
Reference standard and quality-control (QC) sample preparation.
A digested surrogate matrix (10 mg mL−1 bovine serum albumin (BSA) in PBS) was used to prepare calibration-curve standards and quality control (QC) samples. A lyophilized NAT peptide mixture standard stock was reconstituted in 60 μL of 30% ACN/0.1% FA to a final concentration of 400× LLOQ per μL. The NAT stock was serially diluted with 30% ACN/0.1% FA to 0.1×, 0.25×, 0.5×, 2×, 4×, 16×, 40×, and 100× LLOQ per μL to prepare eight calibration standard levels. The 400× LLOQ per μL NAT peptide stock was diluted to the final concentrations of 0.4×, 3×, and 30× LLOQ per μL for QC samples A, B, and C, respectively. Three replicates of each QC concentration were prepared and analyzed along with the plasma digest samples.
SIS addition and solid-phase extraction.
The SIS–peptide mixture was rehydrated in 450 μL of 30% ACN/0.1% FA to a final concentration of 40× LLOQ per μL and spiked into the plasma digest samples and BSA digests as internal standards at a concentration of 10× LLOQ per μg digest.
The final eight-point calibration curves and three QC samples were prepared by combining the SIS peptide-spiked digested surrogate matrix (BSA) mixtures with level-specific amounts of the light peptide mixture corresponding to each calibration-curve standard and QC level.
All plasma samples (NP-based-fractionated or not), calibration-curve standards, and QC samples were desalted by solid phase extraction (SPE) using a Waters Oasis HLB μElution plate and dried using a Speed Vacuum concentrator as previously described, in accordance with the manufacturer's instructions.6 Briefly, the wells of the μElution plate were conditioned with MeOH, equilibrated with 0.1% FA/dH2O, then washed with water following sample loading, and bound peptides eluted with 50% acidic ACN.
LC separation and MS analysis.
The dried plasma digests, calibration curve standards, and QC samples were solubilized with aqueous 0.1% FA and injected for LC/MRM-MS analysis on an Agilent 1290 Infinity UPLC system interfaced to an Agilent triple quadrupole mass spectrometer 6495C. Column loading amounts were 10 μg for the overnight trypsin-only digests of neat plasma, 2 μg for the 3-hour Trypsin/LysC digest of neat plasma, and 2 μg (estimated) for each of the digests prepared via the NP-based platform.
The LC mobile phases were 0.1% FA in LC-MS grade water (A) and 0.1% FA in LC-MS grade ACN (B). Plasma digest samples, calibration-curve standards and QC samples were injected onto a Zorbax Eclipse Plus RP-UHPLC column (2.1 × 150 mm, 1.8 μm particle diameter; Agilent) maintained at 50 °C. Peptide separation was achieved at a flow rate of 0.4 mL min−1 over a 52.6 min run with a multi-step LC gradient. The gradient started at 2% B, increased to 7% at 2 min, then to 30% at 50 min, 80% at 50.1 min, and was held at 80% until 52.5 min, and was then reset back to 2% at 52.6 min. The column was re-equilibrated by a 4 min post-gradient run.
The Agilent 6495C triple quadrupole instrument was operated in the positive ion mode with capillary voltage and nozzle voltages at 3.5 kV and 300 V, respectively. The sheath gas temperature was 250 °C with the flow set to 11 L min−1, and the drying gas flow to 15 L min−1 at a temperature of 150 °C, with the nebulizer gas pressure at 30 psi. The collision cell accelerator voltage was 5 V, the high energy dynode (HED) multiplier was set to −20 kV, and unit mass resolution was used in the first and third quadrupole mass analyzers. For this study, each calibration curve was made with a single tryptic peptide per protein, and that same peptide was used to quantify the protein in each plasma sample. A single transition per peptide target was monitored over 700 ms cycles and detection windows between 90 and 120 s were used for the quantitative analysis.
Data analysis.
Visual examination of the LC/MRM-MS data obtained was performed using Skyline Quantitative Analysis software (version 22.2.0.351, University of Washington).19 The chromatographic peaks for the NAT and SIS peptides in the plasma samples, calibration curves, and QC samples were assessed manually for peak shape and accurate integration. Calibration curves were generated using 1/x2-weighted linear regression and were used to calculate the final protein concentrations in the samples as fmol per μL of plasma. Note that for the digests prepared on the NP-based platform, 2 μg of digest (based on BCA quantitation), is equal to 28.6 nL of plasma, assuming an initial plasma protein concentration of 70 mg mL−1. Additional data analysis and visualization were performed using Microsoft Excel and the R package UpSetR20 (v 1.4.0) with RStudio21 (v 2023.06.1 Build 524) employing R (v 3.4.3). The violin plot in Fig. 5A was generated with Statistics Kingdom's Violin Plot Maker.22
Standard and QC sample acceptance criteria.
For the calibration curve standards and QC samples to pass the acceptance criteria, the quantitative results were required to fall within ±20% of their theoretical concentrations, otherwise they were rejected. All calibration curves were required to contain at least 5 points with concentration accuracies within ±20%. A minimum of 90% of all individual calibration-curve standards and 66% of all QC samples were required to pass these criteria for the experiment to be considered successful.
NP-based platform and neat plasma concentration value acceptance criteria (average, median, CV).
The concentration values for each peptide were obtained for five PC5 plasma replicates processed with the NP-based platform which utilizes a 5-nanoparticle panel (NP1 to NP5) for each plasma processing replicate, resulting in a total of 25 samples (5 processing replicates × 5 NPs). For each peptide target, the average and median concentration values, as well as coefficients of variation (CVs), were calculated for each nanoparticle type only when three or more NP replicates had concentration values above the LLOQ. Similarly, peptide concentration averages, median values, and CVs were calculated for the overnight, trypsin-only digest of neat plasma only when three of three replicates showed quantifiable concentrations.
Results and discussion
NP-based-platform fractionation increases the number of quantifiable targets by up to 44%
The three neat-plasma replicates that were digested overnight with trypsin resulted in 142–151 of the 274 targets (median = 142) being quantifiable (i.e., the endogenous concentrations were within the linear region of each peptide's standard curve) (Fig. 2). The single neat-plasma aliquot that was digested with a 3-hour Trypsin/LysC digestion resulted in 164 quantifiable peptides. These results covered a concentration range of approximately 0.6 fmol μL−1 to 200 pmol μL−1 and are comparable to data published previously.6
 |
| Fig. 2 Number of quantifiable peptides (i.e., within the linear range of the assay) for overnight trypsin-based neat-plasma digest, a 3-hour Trypsin/LysC neat-plasma digest, and fractionated and digested plasma prepared on a NP-based platform, both at the individual NP and 5-NP panel levels. ON = overnight; NP = nanoparticle. | |
The number of quantifiable peptides per individual nanoparticle fraction ranged between 132 and 216 (median = 164) while – across the panel of five-nanoparticles – it ranged from 197 to 241 (median = 204). This represents a median increase of 44% compared to the analysis of neat-plasma digests generated by overnight tryptic digestion, and an increase of 24% compared to the analysis of the neat-plasma digest generated by the 3-hour Trypsin/LysC digestion. The increased coverage of quantifiable peptides (164) with the 3-hour Trypsin/LysC digestion can be explained by the addition of LysC to this version of the digestion protocol, rather than trypsin alone, which yielded 142 peptides, despite the longer incubation time (overnight). Indeed, it has been shown that the addition of LysC to trypsin essentially generates an “improved” trypsin and enhances the number of identified peptides and proteins.23
It is important to note, however, that analyzing the 5-NP panel requires 5 times as much instrument time as it takes to analyze a single non-fractionated neat-plasma digest sample. However, with continuously improving MS instrumentation and increasingly shorter dwell times (which lead to shorter gradients), we believe that coupling the NP-based platform 5-NP approach with targeted LC/MRM-MS is feasible. Moreover, recently a new panel of 2 NP suspensions (instead of 5) has been released and shows similar or slightly increased ID performance.12 Alternatively, for routine targeted workflows a single optimal NP fraction can be selected for each peptide and targeted LC/MRM-MS can be performed on a reduced set of NP injections rather than 5 injections per sample.
A summary of the quantifiable peptides in each workflow can be found in ESI Table 1.†
Concentration distribution amongst the five nanoparticle types
The NP-based platform assay used for this study utilizes a panel of five distinct nanoparticles (the 5-NP panel). Each type of functionalized nanoparticle exhibits unique physicochemical properties, driving protein corona formation and compression of dynamic range of protein concentrations. It is possible, however, that the same protein could be present in the protein corona of multiple NPs, but its absolute concentrations might differ due to the distinct patterns of enrichment by each NP.
For the 274 peptide targets, we determined the average concentrations whenever at least 3 of 5 replicates of the same NP type (e.g., NP1, NP2, NP3, NP4 or NP5) were within a peptide's linear range. Fig. 3A shows the distribution of the peptides for which average concentrations were determined according to the NP type. A total of 151 (55%) peptides were quantifiable on all five NP types, and 93 peptides (34%) on either one, two, three, or four NP types. Thirty peptides (11%) were not quantified on any of the five NP types.
 |
| Fig. 3 NP-based platform protein fractionation specificities. (A) UpSet plot highlighting the overlap of peptides/proteins quantified on the 5 different nanoparticle types. (B) NP-based platform peptide concentration variation between the nanoparticles (n = 219). Calculated as the fold-change (conc_max/conc_min), where conc_max and conc_min are the average peptide concentrations on the NPs with the highest and the lowest concentrations of a given peptide, respectively. | |
To further investigate the specificities of the different types of nanoparticles, for each peptide quantified by at least two nanoparticle types, we have taken the pair of NPs with the highest and the lowest concentration of this peptide and calculated the corresponding fold-change (Fig. 3B). Of the 219 such peptides, 56 (25.6%) showed fold-changes between 1× and 2×, 91 peptides (41.6%) showed fold-changes of 2–10×, and the remainder (72 peptides, 32.9%) showed fold-changes of >10×. The maximum fold-change observed was 2803-fold for the prothrombin-derived peptide, ELLESYIDGR.
Overall, these data suggest that, while there is overlap amongst the five NPs to enrich for the same proteins, most of the targets exhibit a large concentration difference when enriched by the different NP types, which may allow screening for an optimal NP to achieve maximum sensitivity and thereby precision for each individual protein target.
Precision of NP-based platform digestion vs. neat-plasma digest (Tryp, ON)
We assessed the precision of the concentrations determined with the NP-based platform (5 nanoparticle types, each with n = 5 replicates) and the approach using overnight trypsin-only digestion of neat plasma (n = 3 replicates).
Because of the significant differences in average peptide concentrations, depending on which nanoparticle type was used for the NP-based enrichment, we also established criteria to select a single nanoparticle type (i.e., the “best NP”) per peptide for establishing the average peptide concentration to use for comparing the CV performances of the two workflows. The selection criteria to define the “best NP” were three-fold: (1) ≥3 out of 5 replicate measurements per NP above the LLOQ to allow calculation of an average concentration, (2) the average concentration needed to be the largest among the different nanoparticle types, and (3) the corresponding CV needed to be less than 20%. Note that peptide CVs calculated for the neat-plasma trypsin-only digests required that 3 of 3 replicates had to be above the LLOQ. The CVs were calculated by dividing the standard deviations by the corresponding average protein concentrations. To ensure statistically justified CVs, at least 3 replicates with quantified protein concentrations were required for each calculation.
The CV distributions for the Trypsin/LysC approach using the NP-based platform and the overnight neat plasma digestion using trypsin are shown in Fig. 4A and B, respectively. The NP-based approach resulted in a median CV of 8.3%, while the neat-plasma digestion resulted in a median CV of 13.1%. While the neat plasma digest approach resulted in 137 peptides quantified across three replicates (Fig. 4B), the “best NP” from the NP-based platform approach allowed quantitation of 199 peptides in at least 3 of 5 replicates (Fig. 4A), suggesting that an additional 62 peptides could be quantified, equivalent to a 45% increase.
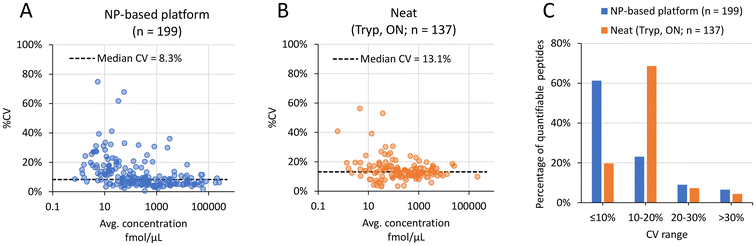 |
| Fig. 4 Distribution of CVs of the process replicates for (A) NP-based-fractionated plasma digests for best NP (based on ≥3 concentration values above LLOQ, highest average concentration amongst NPs, and CV <20%), and (B) overnight, trypsin-based neat plasma digests. (C) Histogram of CV ranges. | |
Moreover, most of the CV values calculated for the NP-based platform approach (∼60%) were ≤10%, and between 10–20% (∼70% of CV values) for the neat-plasma digestion approach (Fig. 4C).
Notably, three peptides had CVs of >60% when using the NP-based platform approach, and four peptides had CVs of ≥39% with the neat plasma digest approach. None of these high-CV peptides overlapped between the two approaches. The high CVs could be attributed to one of two factors: (1) slight interferences in the light signals when using the neat approach for peptides EATLELLGR and VSQYIEWLQK, representing Tumor necrosis factor receptor superfamily member 1A and Coagulation factor VII, which were overcome by using the NP-based platform approach, and (2) extremely low signals for either light signal alone, or for both light and heavy signals, either consistently across the various injections, or for only some of the injections.
The data indicate that the NP-based fractionation approach, using the best NP (based on ≥3 concentration values above LLOQ, highest average concentration amongst NPs, and a CV of <20%), is highly reproducible, and showed better CVs than the standard, neat-plasma digestion.
Distribution and correlation of neat-plasma digests with concentrations determined by the NP-based platform
We then compared the protein concentrations determined from the neat plasma digests and the NP-based platform workflow. Note that for the 3-hour Trypsin/LysC neat-plasma digest only a single replicate was available. For the overnight neat-plasma digest using trypsin only, the concentration values used were the average concentrations when all three replicates had peptide quantities within the linear range of the assay. For the NP-based platform data, average concentrations were calculated for the “best NP” (see criteria for “best NP” above).
We compared the protein concentrations of the proteins quantified across all three approaches (n = 107). As expected, the highest determined concentration for both the neat Trypsin/LysC and the neat trypsin-only plasma digests was serum albumin, shown in Fig. 5A, the most abundant protein in human plasma.24 The dynamic range compression of the NP-based platform workflow, however, was observed as a shift toward higher protein concentrations compared to the neat-plasma digestion workflows, as evidenced by the significantly elevated 1st quartile, median and 3rd quartile concentrations shown in Table 1, and which is also visualized in Fig. 5A.
 |
| Fig. 5 Protein concentration comparison between neat plasma digests and NP-based platform approach. (A) Concentration distribution for the 107 proteins quantified across all three approaches. (B) 3-hour Trypsin/LysC digestion of neat plasma vs. overnight trypsin-only digestion of neat plasma. (C) NP-based platform digestion vs. neat-plasma overnight tryptic digestion. (D) NP-based platform digestion vs. the 3-hour Trypsin/LysC digestion of neat plasma. The dashed lines in panels B–D indicate the lines of identity. | |
Table 1 Protein concentration distribution of peptides quantified with all three approaches: neat plasma digest done with trypsin only, neat plasma digest done with Trypsin/LysC, and the NP-based platform (n = 107). Unit in fmol μL−1 plasma
|
Neat trypsin |
Neat trypsin/LysC |
NP-based platform |
Min |
1.37 |
1.45 |
4.33 |
1st quartile |
38.8 |
55.6 |
218.3 |
Median |
170.2 |
355.0 |
2599 |
3rd quartile |
844.8 |
1338 |
20 071 |
Max |
217 940 |
408 440 |
446 326 |
The neat-plasma digests showed excellent correlation with an R2 of 0.97 (Fig. 5B). The slope of 1.86 suggests systematically higher concentrations were determined using the neat-plasma digestion done with Trypsin/LysC. This can be explained by the fact that the plasma peptide amounts used for this digest were determined by BCA assay, while the plasma digest prepared for the trypsin-only digest assumed an original 70 μg μL−1 plasma protein concentration and a final peptide concentration of 1 μg μL−1 after digestion. Thus, the 1.86 slope could result from a combination of inherent measurement errors from the BCA assay, and/or deviations of the actual plasma concentration from the expected 70 μg μL−1. Moreover, minor non-systematic variations between the neat-plasma digestion results done by two separate, different laboratory sites can be explained by differences in the two digestion protocols, which use different enzyme combinations (trypsin by MRM Proteomics, Trypsin/LysC by Seer Inc.) and digestion lengths (overnight vs. 3 hours, respectively). As has been shown in previous publications, variations in digestion conditions such as duration can result in differences in the protein amount quantified.25
Comparing the average protein concentrations determined from the NP-based platform fractionated plasma digests with the trypsin-only (Fig. 5C) and Trypsin/LysC non-fractionated neat-plasma digests (Fig. 5D) resulted in negligible R2 values, suggesting no correlation between the NP-based-derived and neat-plasma digests. This is expected, since the NP-based platform approach enriches at the protein level and the composition of the protein corona differs depending on the physicochemical properties of the five nanoparticle types, as was evidenced by the distribution of calculated protein concentration from the different nanoparticle types (Fig. 3B). This enrichment enables accurate measurement of relative abundances for a wider set of proteins.26 In other words, while the NP-based-platform protein concentrations determined by MRM were normalized as fmol per amount of digest, the NP-based platform digests were composed of protein concentrations that differed from neat plasma due to the enrichment step.
Despite non-correlating protein concentrations between neat-plasma digests and NP-based-fractionated plasma digests, the concentrations that were determined from the NP-based platform approach are highly precise. As demonstrated, the combination of NP-based plasma processing, followed by LC/MRM-MS using internal standard peptides for protein quantitation would represent an important tool for obtaining concentration values for plasma protein targets that are commonly below their respective LLOQs by conventional, neat-plasma in-solution digestion followed by MRM analysis. As a next step, to address the protein concentration differences between the NP-based platform and neat plasma LC/MRM-MS approaches and related, potential accuracy concerns, NP-based-specific protein reference ranges should be determined using plasma samples with known concentrations, established with protein quantitation techniques such as ELISA or fractionation-free LC/MRM-MS.
Conclusion
In this study, we coupled the fractionation done on the Proteograph NP-based platform with absolute protein quantitation by MRM using stable isotope-labelled standard (SIS) peptides to establish whether the NP-based platform method provided improvements in sensitivity and precision over conventional plasma protein quantitation for a panel of 270 human plasma proteins.
The results showed a significant increase in the number of proteins quantified when using the NP-based separation approach (up to 44% more proteins), and increased precision (8.3% vs. 13.1% CV).
By absolute quantification of proteins using both standard and NP-based platform LC/MRM-MS workflows, this study potentially enables inferring absolute plasma protein concentrations from the NP-based platform data. Looking ahead, next steps to validate this technique would be to establish NP-based LC/MRM-MS workflow-specific protein reference ranges, as well as determining protein concentrations for a larger sample cohort and comparing them to established protein quantitation methodologies such as ELISA or fractionation-free LC/MRM-MS.
Author contributions
CHB: funding acquisition, conceptualization, manuscript review and editing, project administration. CG: methodology, sample preparation, formal analysis, writing original draft, visualization, writing review and editing. RP: methodology, sample preparation, formal analysis, writing original draft, visualization, writing review and editing, project management and coordination. ASG: sample preparation, review, edit, methodology, data analysis guidance.
Data availability
The data has been deposited to Panorama with the permanent link (https://panoramaweb.org/1aidn3.url). The reviewer account details are: E-mail: panorama+reviewer275@proteinms.net; password: CTU4%muAG7?k5f.
The ProteomeXchange ID reserved for the data is: PXD053704 (https://proteomecentral.proteomexchange.org/cgi/GetDataset?ID=PXD053704), and the DOI for the data is: https://doi.org/10.6069/2a58-q964.
Conflicts of interest
CHB is a Scientific Advisor of MRM Proteomics, Inc. and the VP of Proteomics at Molecular You; ASG is an employee of Seer Inc.
Acknowledgements
MRM Proteomics Inc. is grateful to the University of Victoria - Genome BC Proteomics Centre, for their assistance in designing and validating the 274 human surrogate peptides.
CHB is grateful for support from the Segal McGill Chair in Molecular Oncology at McGill University (Montréal, Quebec, Canada). CHB is also grateful for support from the Warren Y. Soper Charitable Trust for the Warren Y. Soper Clinical Proteomics Centre at the Jewish General Hospital (Montréal, Quebec, Canada), from Genome Canada and Genome Quebec via the MutaQuant GAPP (#6567 Borchers_Zahedi_APF), and for support from the Terry Fox Research Institute and the Alvin Segal Family Foundation for the Segal Cancer Proteomics Centre at the Jewish General Hospital (Montréal, Quebec, Canada).
This work was done under the auspices of a Memorandum of Understanding between McGill and the U.S. National Cancer Institute's International Cancer Proteogenome Consortium (ICPC). ICPC encourages international cooperation among institutions and nations in proteogenomic cancer research in which proteogenomic datasets are made available to the public. This work was also done in collaboration with the U.S. National Cancer Institute's Clinical Proteomic Tumor Analysis Consortium (CPTAC).
References
- M. A. Kuzyk, C. E. Parker, D. Domanski and C. H. Borchers, Methods Mol. Biol., 2013, 1023, 53–82 CrossRef CAS.
- C. Gaither, R. Popp, R. P. Zahedi and C. H. Borchers, Mol. Cell. Proteomics, 2022, 21, 100212 CrossRef CAS.
- A. J. Percy, J. Yang, D. B. Hardie, A. G. Chambers, J. Tamura-Wells and C. H. Borchers, Methods, 2015, 81, 24–33 CrossRef CAS.
- E. S. Boja and H. Rodriguez, Proteomics, 2012, 12, 1093–1110 CrossRef CAS.
- M. A. Gillette and S. A. Carr, Nat. Methods, 2013, 10, 28–34 CrossRef CAS PubMed.
- C. Gaither, R. Popp, Y. Mohammed and C. H. Borchers, Analyst, 2020, 145, 3634–3644 RSC.
- V. Ignjatovic, P. E. Geyer, K. K. Palaniappan, J. E. Chaaban, G. S. Omenn, M. S. Baker, E. W. Deutsch and J. M. Schwenk, J. Proteome Res., 2019, 18, 4085–4097 CrossRef CAS.
- D. R. Barnidge, M. K. Goodmanson, G. G. Klee and D. C. Muddiman, J. Proteome Res., 2004, 3, 644–652 CrossRef CAS.
- L. Anderson and C. L. Hunter, Mol. Cell. Proteomics, 2006, 5, 573–588 CrossRef CAS PubMed.
- S. Ferdosi, B. Tangeysh, T. R. Brown, P. A. Everley, M. Figa, M. McLean, E. M. Elgierari, X. Zhao, V. J. Garcia, T. Wang, M. E. K. Chang, K. Riedesel, J. Chu, M. Mahoney, H. Xia, E. S. O'Brien, C. Stolarczyk, D. Harris, T. L. Platt, P. Ma, M. Goldberg, R. Langer, M. R. Flory, R. Benz, W. Tao, J. C. Cuevas, S. Batzoglou, J. E. Blume, A. Siddiqui, D. Hornburg and O. C. Farokhzad, Proc. Natl. Acad. Sci. U. S. A., 2022, 119, e2106053119 CrossRef CAS.
- J. E. Blume, W. C. Manning, G. Troiano, D. Hornburg, M. Figa, L. Hesterberg, T. L. Platt, X. Zhao, R. A. Cuaresma, P. A. Everley, M. Ko, H. Liou, M. Mahoney, S. Ferdosi, E. M. Elgierari, C. Stolarczyk, B. Tangeysh, H. Xia, R. Benz, A. Siddiqui, S. A. Carr, P. Ma, R. Langer, V. Farias and O. C. Farokhzad, Nat. Commun., 2020, 11, 3662 CrossRef CAS PubMed.
-
B. Lacar, S. Ferdosi, A. Alavi, A. Stukalov, G. R. Venkataraman, M. de Geus, H. Dodge, C. Y. Wu, P. Kivisakk, S. Das, H. Guturu, B. Hyman, S. Batzoglou, S. E. Arnold and A. Siddiqui, bioRxiv: the preprint server for biology, 2024, 2024.01.05.574446.
- S. Ferdosi, A. Stukalov, M. Hasan, B. Tangeysh, T. R. Brown, T. Wang, E. M. Elgierari, X. Zhao, Y. Huang, A. Alavi, B. Lee-McMullen, J. Chu, M. Figa, W. Tao, J. Wang, M. Goldberg, E. S. O'Brien, H. Xia, C. Stolarczyk, R. Weissleder, V. Farias, S. Batzoglou, A. Siddiqui, O. C. Farokhzad and D. Hornburg, Adv. Mater., 2022, 34, e2206008 CrossRef PubMed.
- M. A. Kuzyk, D. Smith, J. Yang, T. J. Cross, A. M. Jackson, D. B. Hardie, N. L. Anderson and C. H. Borchers, Mol. Cell. Proteomics, 2009, 8, 1860–1877 CrossRef CAS.
- CPTAC, Assay Characterization Guidance Document, (accessed Nov. 4, 2015, 2015).
- CPTAC_Assay_Portal, Assay Portal, (accessed Nov. 14, 2015, 2015).
- K. Suhre, G. R. Venkataraman, H. Guturu, A. Halama, N. Stephan, G. Thareja, H. Sarwath, K. Motamedchaboki, M. K. R. Donovan, A. Siddiqui, S. Batzoglou and F. Schmidt, Nat. Commun., 2024, 15, 989 CrossRef CAS PubMed.
-
M. D. Roberts, B. A. Ruple, J. S. Godwin, M. C. McIntosh, S. Y. Chen, N. J. Kontos, A. Agyin-Birikorang, J. Max Michel, D. L. Plotkin, M. L. Mattingly, C. Brooks Mobley, T. N. Ziegenfuss, A. D. Fruge and A. N. Kavazis, bioRxiv: the preprint server for biology, 2023, 2023.06.02.543459.
- B. MacLean, D. M. Tomazela, N. Shulman, M. Chambers, G. L. Finney, B. Frewen, R. Kern, D. L. Tabb, D. C. Liebler and M. J. MacCoss, Bioinformatics, 2010, 26, 966–968 CrossRef CAS.
- RStudio_Team, RStudio Team (2020). RStudio: Integrated Development for R. RStudio, (accessed Dec. 21, 2023, 2023).
- J. R. Conway, A. Lex and N. Gehlenborg, Bioinformatics, 2017, 33, 2938–2940 CrossRef CAS.
- Statistics Kingdom, Violin Plot Maker, https://www.statskingdom.com/violin-plot-maker.html, (accessed Dec. 21, 2023, 2024).
- S. Saveliev, M. Bratz, M. Urh, R. Zubarev, H. Budamgunta and M. Szapacs, Nat. Methods, 2013, 10, i–ii CrossRef.
- E. J. Cohn, J. L. Oncley, L. E. Strong, W. L. Hughes and S. H. Armstrong, J. Clin. Invest., 1944, 23, 417–432 CrossRef CAS.
- J. L. Proc, M. A. Kuzyk, D. B. Hardie, J. Yang, D. S. Smith, A. M. Jackson, C. E. Parker and C. H. Borchers, J. Proteome Res., 2010, 9, 5422–5437 CrossRef CAS PubMed.
-
T. Huang, J. Wang, A. Stukalov, M. K. R. Donovan, S. Ferdosi, L. Williamson, S. Just, G. Castro, L. S. Cantrell, E. Elgierari, R. W. Benz, Y. Huang, K. Motamedchaboki, A. Hakimi, T. Arrey, E. Damoc, S. Kreimer, O. C. Farokhzad, S. Batzoglou, A. Siddiqui, J. E. Van Eyk and D. Hornburg, BioRxiv: the preprint server for biology, 2023, DOI:10.1101/2023.08.28.555225.
|
This journal is © The Royal Society of Chemistry 2025 |
Click here to see how this site uses Cookies. View our privacy policy here.