DOI:
10.1039/D4SD00085D
(Critical Review)
Sens. Diagn., 2024,
3, 914-932
Fluorescence coding techniques for RNA detection
Received
19th March 2024
, Accepted 14th April 2024
First published on 16th April 2024
Abstract
Fluorescence imaging along with a wide range of fluorescence detection methodologies enables the visualization of macromolecular dynamics in clinical diagnostics and fundamental biomedical research. However, multiplexed fluorescence-based detection has hitherto been largely limited by the overlap of the emission spectra of fluorophores, which only with four or five fluorochrome dyes enable simultaneous use. Fluorescent barcodes with high resolution and high throughput have emerged as a versatile biosensor platform for multianalyte analysis via fine control of fluorescence proportional types, spatial geometries, specific fluorescence properties, and time dimensions. Fluorescence imaging of RNA enables the visualization of physiological and pathological processes at the subcellular level, which is highly important for biomedical diagnostics and therapies and has made tremendous progress toward understanding the complexity of biological systems. In this review, we provide the design and development of fluorescence coding techniques for multiplexed bioanalysis detection and then discuss their applications in RNA biosensors. Additionally, associated challenges in the field and potential solutions will be discussed. Overall, we hope this review will inspire researchers and pave the way for the future design of fluorescent biosensors.
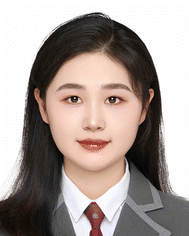 Junren Wang | Junren Wang earned her bachelor's degree from Anhui Science and Technology University in 2021 and is currently studying for her master's degree in biomedical engineering at Shenzhen University under the supervision of Prof. Dong Haifeng. Her research interest focuses on multiple detection of tumor biomarkers using fluorescence coding technology. |
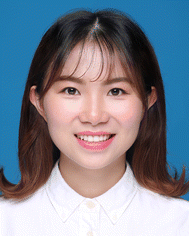 Qin Xiang | Qin Xiang received her PhD degree from Chongqing University in 2019. She pursued her research as a Postdoctoral researcher at Shenzhen University from 2020 to 2022. Afterward, she joined Shenzhen University as an Associate Researcher. She is primarily engaged in precise bioanalysis research at the cellular and in vivo levels as well as molecular probes and biosensors. |
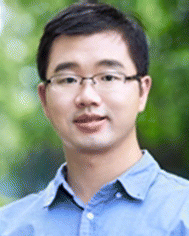 Haifeng Dong | Haifeng Dong received his PhD degree from Nanjing University in 2011. He then joined the USTB in the same year. He pursued his research as a Postdoctoral researcher at the University of Pittsburgh from 2012 to 2013. Afterward, he joined USTB as a Professor. As a Visiting Professor, he used to work at Kyoto University in 2015. He moved to Shenzhen University as a Professor in September 2020. His research interests include the application of functional nano-probes in nucleic acid biosensors and imaging analysis of intracellular biomolecules as well as nanomedicine. |
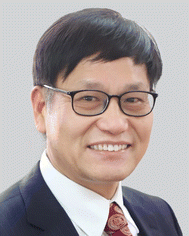 Xueji Zhang | Xueji Zhang is the Vice President of Shenzhen University. He received his PhD degree from Wuhan University in 1994 and was a postdoctoral scholar at the National Institute of Chemistry Slovenia from 1995 to 1997, the Swiss Federal Institute of Technology from 1997 to 1998, and New Mexico State University from 1998 to 1999. He joined the World Precision Instruments Inc. as a Sr. Scientist, Head of the Chemistry Department in 1999, and then became the Senior Vice President in 2004. His current research focuses on bioanalysis, electrochemical sensors, micro/nanosensors, and biomedical instrumentation. |
1. Introduction
Conducting numerous high-throughput studies in intricate biological systems is crucial for biological research and medical diagnostics.1 Fluorescence imaging is the predominant technique for the in situ detection of cells and intracellular compounds.2,3 Fluorescence imaging is a method of visualizing and examining organisms or materials using fluorescent markers. It has several benefits including exceptional sensitivity, precise resolution, and non-destructive properties. This approach finds extensive application in several areas such as biomedical research, cell biology, and molecular biology.4–6 As technology advances, fluorescence imaging techniques such as multiphoton fluorescence imaging7 and super-resolution fluorescence imaging8,9 are continuously being enhanced and refined. These advancements offer greater potential for scientific inquiry and medical diagnostics. However, the presence of spectral overlap among fluorescent dyes can make the differentiation of fluorescence signals of distinct dyes difficult when many dyes are used to identify various molecules or cells. This can lead to the mixing of information or incorrect classification.10
In order to address this issue, the concept of fluorescence coding has been suggested as a means of encoding various chemicals or organisms by utilizing different fluorescence colors or intensities. This approach has the potential to greatly enhance the ability to detect or image many targets simultaneously. Fluorescence coding is a versatile technique that can be employed to mark proteins for monitoring their whereabouts and mobility within cells or tissues.11 Additionally, it can be used to construct fluorescent probes capable of identifying the existence and function of biomolecules.12 Moreover, fluorescence coding enables the tracking and examination of cellular behaviors and interactions.13 In summary, fluorescence coding has an extensive array of applications in biological detection. The present fluorescence codes used for multiple detections mostly consist of ratio-type codes, spatial geometry codes, fluorescence property codes, and time dimension codes.
Ribonucleic acid (RNA) is a crucial molecule involved in the process of gene expression. It serves as a transporter of genetic information during transcription and translation. RNA has been identified as a potential biomarker for several illnesses, including early cancer identification and screening.14 RNA can be classified into several categories including mRNA,15 tRNA,16 microRNA (miRNA),17 and small-molecule RNA. Messenger RNA (mRNA), a type of RNA that is not double-stranded, regulates protein production and carries genetic information.18 Tumor-associated mRNAs are frequently used as specific indicators to assess the local or systemic migration of tumor cells, and any change in their expression levels is directly associated with the onset and progression of tumors.19,20 Approximately 30% of genes are directly regulated by miRNAs, which are a group of small, non-coding RNAs. They inhibit the production of proteins via regulating the post-transcriptional stage of mRNA degradation.21 Research has demonstrated that miRNAs play a significant role in a wide range of biological processes such as apoptosis, migration, aging, differentiation, and proliferation. Additionally, they have been demonstrated to contribute to the development of several illnesses such as diabetes,22 leukemia,23 and cancer.24
RNA biomarkers25,26 have higher sensitivity and specificity, and RNA detection holds significant therapeutic promise. However, several challenges need to be overcome before its application to real samples. To effectively detect RNA, it is necessary to employ techniques with high specificity. This is because RNA has a low molecular weight and a high degree of similarity within the same family. Furthermore, the assay must have sensitivity and accuracy as RNA is found in both healthy and malignant cells at low levels, with the primary distinction being the varying amounts produced. Furthermore, it is evident that multiplexed assays are essential, as relying solely on the dysregulation of a single RNA for illness diagnosis is not appropriate. This is due to the high expression of certain RNAs in various types of disease-causing cells.27,28
This article provides an overview of the current research on fluorescence coding for multiplexed detection. It discusses the application of fluorescence coding for multiplexed detection, in particular RNA detection. Additionally, it evaluates the obstacles and opportunities associated with this technique.
2. Fluorescence coding technique
Fluorescent markers,29 compounds that absorb light from the surrounding environment and emit fluorescence at specific wavelengths, are the main components used in fluorescence imaging. Fluorescent dyes,30 fluorescent proteins,31,32 and similar substances are commonly employed as fluorescent markers. In the 1960s, researchers began investigating the application of fluorescent materials for interpreting biological data.33 Fluorescent materials have the ability to stimulate and emit energy, resulting in the production of visible light due to their luminescence properties. Fluorescent materials are ideal for labeling purposes. Fluorescence imaging can be used to conduct studies on the distribution and metabolism of fluorescent markers in animals. This technique allows for the tracking of tumor growth and metastasis in live mice.34,35 Additionally, it can be utilized to observe the localization and distribution of particular proteins within cells36 and explore the physiological processes of cells.
Fluorescence imaging serves as the fundamental basis for fluorescence encoding technology.37–39 Spectral overlap is a phenomenon that occurs when numerous fluorescent dyes are employed simultaneously. This poses a challenge in precisely measuring the matching fluorescence intensity, thereby affecting the precision of detection outcomes. Consequently, the utilization of fluorescence imaging technology is limited to the simultaneous employment of just four to five distinct types of fluorescent dyes. In addition, varying excitation spectra require the use of separate exciters, resulting in increased costs and complexity of the apparatus. Fluorescence coding technology has been developed to solve these problems. It is the most commonly used coding technology because it enables easy visualization of barcodes, detection signal localization, and reading. This technology primarily utilizes the distinct characteristics of the coding original, such as fluorescence intensity, spatial geometry, and temporal lifetime, to fulfill the requirement of multiple detection and achieve various coding methods. The fluorescence coding approach is extensively employed in biomedical research to achieve more precise labeling of cells,40 proteins41 and DNA,42 among other substances. As technology has progressed, the use of fluorescence coding has gradually increased. In the 1980s, researchers initiated an inquiry into the application of fluorescent particles for coding. Fluorescent particles have the ability to mark and monitor items, such as identifying tumor markers in biomedical research.43,44 With the advancement of nanotechnology, fluorescence coding has shown significant improvement in recent years. Researchers investigated the application of nanoparticles for fluorescence coding.45 Nanoparticles possess reduced dimensions and enhanced fluorescence characteristics, facilitating the utilization of more intricate and accurate encoding techniques. The nano-fluorescence coding technology has diverse applications in the domains of biology, materials science, and information storage.46–48 Fluorescence coding primarily emerged from the investigation and utilization of fluorescent materials. Due to technological advancements, fluorescence coding technology has undergone constant development and improvement, offering new prospects for research and application in diverse domains.
3. Classification of fluorescence codes
3.1 Fluorescence coding based on proportion
Proportional fluorescence coding is a strategy that was discovered early on and is now frequently employed. This technique employs various proportions of fluorescent dyes to represent distinct biomolecules or cells. By measuring the intensity of different fluorescence signals, it can identify and quantify the presence and concentration of target biomolecules or cells. This enables the simultaneous detection of multiple biomolecules or cells, enhancing the efficiency and throughput of the assay.49 Proportional fluorescence coding has numerous benefits in the field of biological detection. First, it has the capability to simultaneously detect multiple target biomolecules or cells, enabling efficient high-throughput detection. Second, it can achieve various encodings by modifying the concentration of different ratios of fluorescent dyes, offering great flexibility and adaptability.50–52
3.1.1 Fluorescence coding based on DNA nanotechnology.
During the 1980s, Seeman suggested using the idea of base complementary pairing to create DNA nanostructures with specific morphologies.53 DNA nanostructures have significant advantages over conventional materials as they continue to develop. First, based on the Watson–Crick base pairing principle,54 DNA nanostructures possess a high level of programmability and can be designed in different spatial configurations and sizes, ranging from one-dimensional to three-dimensional.55 Second, it is possible to design various functional groups including small molecules, macromolecules, or nanoparticles to be securely attached to specific positions within DNA nanostructures. This feature allows for precise addressing of these functional groups.56–58 Furthermore, due to the predetermined and extremely small dimensions of the DNA bases, it is possible to engineer DNA backbones with a level of accuracy that approaches atomic precision.59 In addition, DNA nanoparticles have excellent biocompatibility.60
In 2005, Luo's team used Y-DNA to construct dendritic DNA (DL-DNA) nanostructures, which demonstrated the superiority of DNA nanostructures. The DL-DNA was employed as a carrier for fluorescent dyes in Alexa Fluor 488 (G) and BODIPY 630/650 (R), to create fluorescence intensity-encoded nano-barcodes. This allowed for the precise control of the ratio between the two types of dyes (Fig. 1A).61 Initially, Y-DNA was synthesized and labeled with fluorescent markers. Then, using sticky ends, five different combinations of fluorescent barcodes were created: 4G1R, 2G1R, 1G1R, 1G2R, and 1G4R. These barcodes were used to detect various pathogen DNAs through fluorescence microscopy and spot blotting. This technique enables the simultaneous detection of multiple pathogens using flow cytometers, which are typically limited to detecting only two colors. In 2006, the main focus was on preparing DL-DNA by solid-phase and liquid-phase methods. Additionally, the multivalent anisotropy of DL-DNA was used to create fluorescence coding approaches for detecting various diseases simultaneously and quickly.62 Subsequently, three Y-DNA donor molecules and one X-DNA acceptor molecule were employed to amplify and identify the signals of various diseases by encoding three fluorescence ratios, namely 1G1R, 1G3R, and 4G, utilizing quantum dots (QDs) of red (R) and green (G) hues.63 Nevertheless, the aforementioned approaches are susceptible to aggregation-induced fluorescence bursts and have limited encoding capability.
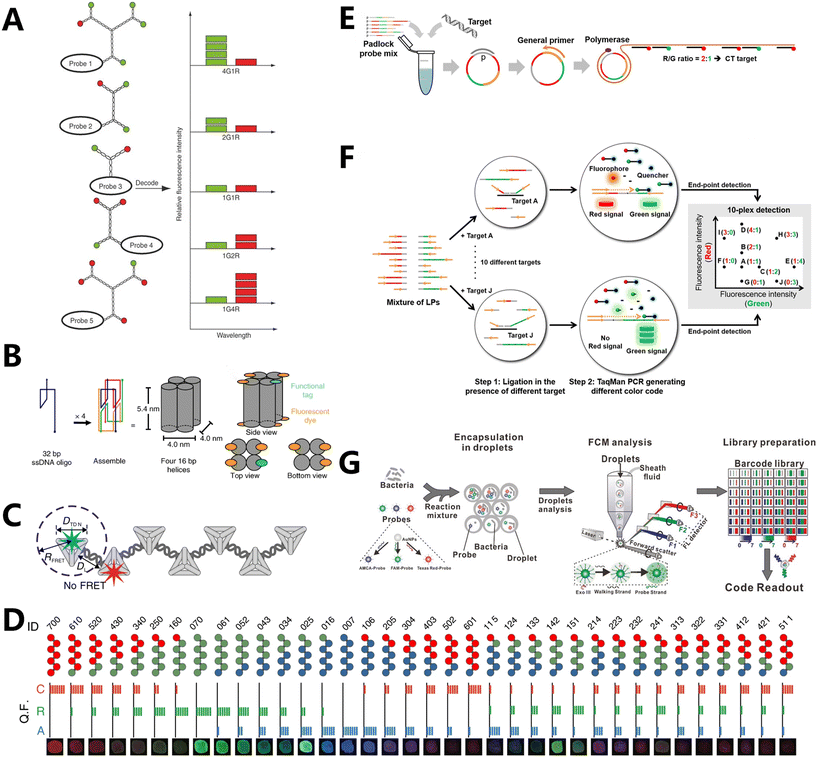 |
| Fig. 1 Fluorescence coding based on proportion. (A) Schematic illustration of barcode decoding. The nanobarcodes 4G1R, 2G1R, 1G1R, 1G2R and 1G4R were decoded based on the ratio of fluorescence intensity.61 (B) Design and assembly of DNA FluoroCubes.71 (C) Schematic illustration of the FDF structure with spatial control of fluorophores.72 (D) Thirty-six FDF barcodes encoded with different fluorophore combinations.72 (E) Schematic illustration of sequence-encoded amplicon (SeqEA) for multiplexed imaging of individual RNAs in single cells.74 (F) LiNC PCR is performed using two steps.76 (G) Schematic of the SDwalker-Drop platform for the super-multiplex bacterial phenotype analysis.79 | |
In 2006, Rothemund showcased innovative research in the field of DNA origami technology.64 DNA origami technology is a method that harnesses the inherent self-assembly capability of DNA molecules to construct structures and devices at the nanoscale. The process relies on the fundamental concept of base complementary pairing between DNA molecules, allowing for the spontaneous formation of desired structures when specified conditions are met through the design of suitable DNA sequences.65,66 The technology of DNA origami is characterized by its exceptional accuracy and controllability, enabling meticulous assembly at the nanoscale.67 Additionally, it possesses a high degree of self-assembly efficacy and rapid self-assembly velocity, enabling the formation of intricate structures within a short timeframe.68 Furthermore, the DNA origami technology has superior biocompatibility and biostability, which make it suitable for implementation in live organisms.69 When comparing small Y-DNA molecules to DNA origami technology, it is observed that DNA origami technology prevents fluorescence resonance energy transfer (FRET) due to its larger size. Additionally, it allows for the precise placement of fluorophores at specific positions and their assembly into predetermined fluorescence-aligned structures. This significantly enhances the capacity for fluorescence encoding. Jungmann and his colleagues used DNA origami to construct fluorescent probes smaller than 100 nm in size. These probes have adjustable optical properties, and were constructed by combining 24 DNA double-helix structures into a two-dimensional (2D) rectangular DNA origami with a dimension of 90 × 60 nm2. The origami structures were loaded with varying amounts of three different fluorescent dyes (ATTO 647N, Cy3, and ATTO 488).70 By employing three distinct fluorescent dyes and conducting trials at five varying intensity levels, researchers obtained a total of 53 − 1 = 124 fluorescent probes. While the encoding capacity is significantly enhanced, the large-scale production of DNA origami measuring around 100 nm often necessitates numerous single-stranded DNAs. The complexity of the strand design and synthesis process imposes limitations on its practical use. Larger DNA nanoparticles are not suitable for detecting and imaging at the cellular level.
FluoroCubes, which are only 6 nm in size, have been recently prepared by DNA engineering, and can be customized with six fluorescent moieties (Fig. 1B).71 This method enables the efficient imaging of individual molecules, along with a significant 54-fold extension in fluorescence duration and a substantial 43-fold enhancement in photon emission. Fan et al. have used Cayley dendritic fractal DNA frameworks (FDFs) to develop fluorescence intensity-encoded barcodes (Fig. 1C).72 The Cayley dendritic framework structure was initially fabricated by building DNA tetrahedra with a side length of 20 bp. Subsequently, the n-node structure (n = 53) could be achieved using only 16 strands. By accurately attaching three fluorescent motifs (Alexa 488, Cy5, and ROX) to the FDFs to create the F2,3 structure, they not only prevented fluorescence energy resonance transfer, which reduces fluorescence crosstalk, but also produced 36 coding barcodes with distinct fluorescence colors and intensities (Fig. 1D). This significantly reduces the quantity of DNA strands and diminishes the complexity and workload associated with coding, in contrast to the prior method of using large-sized DNA origami. At the same time, it enhances the capabilities of recognizing and imaging many functions in living cells.
3.1.2 Fluorescence coding based on nucleic acid amplification technology.
In addition to proportionate fluorescence coding, nucleic acid amplification technology, which relies on the performance of DNA sequence hybridization via base complementary pairing, can be used for coding purposes. This coding technique can be accomplished via a polymerase chain reaction (PCR), rolling circle amplification (RCA), and other methods. Alternatively, it can include amplifying a much small amount of the target to be detected in order to generate a significant number of duplicates. Not only will this decrease the limit at which the test can detect something, but it will also significantly enhance the sensitivity and precision of the test.
Lee et al. applied RCA to produce biotin surface-modified nanospheres with many functionalities to generate barcodes for cancer cell detection.73 The nanospheres were subsequently linked to different proportions of red and green QDs via biotin–streptavidin interactions. Wang et al. used lock-in probes to accomplish proportionate fluorescence encoding with RCA (Fig. 1E).74 In particular, lock-in probes were used to attach two probes with different fluorophores by incorporating a specific number of binding sites to target nucleic acids. The study describes the design of six lock-in probes that can be recycled. These probes were created using two separate fluorescent dyes, namely red (R) and green (G), and each probe has a unique R/G ratio. After performing nucleic acid amplification, these hybridized probes were used to identify the gene sequences of six infectious diseases, thereby expanding the method's potential to simultaneously detect several diseases.
Furthermore, the PCR has been utilized for encoding, in addition to the RCA approach. The reaction is a molecular biology technique used to amplify specific DNA fragments in a controlled laboratory setting. Due to its exceptional specificity and sensitivity, it is widely utilized in various domains such as gene amplification and medical diagnostics. This technique allows for the amplification of several target fragments from minuscule DNA samples.75 Wang et al. developed a novel fluorescence-based scheme called Ligation-eNabled fluorescence-Coding PCR (LiNC PCR) using PCR technology. This scheme significantly enhances the multiplexing capability of standard PCR analysis (Fig. 1F).76 In this approach, TaqMan probes were inserted using probe locations that corresponded to red (R) and green (G) fluorescence signals. The probes were built with ten R/G ratios, namely, 1/1, 2/1, 1/2, 4/1, 1/4, 1/0, 0/1, 3/3, 3/0, and 0/3. The binding sites provide distinctive fluorescence signals during the PCR procedure, enabling clear and unequivocal identification of the amplified targets. Consequently, the detection performance and ability to simultaneously identify several ovarian cancer epigenetic indicators were considerably enhanced by using only two universal TaqMan probes.
3.1.3 Fluorescent microsphere coding with different proportions.
Microspheres surpass planar arrays in terms of both production and use. Microspheres show the ability to be linked to a wide range of probe molecules, while planar arrays have a limited number of predetermined attachment sites for probe molecules. The Bio-plex Suspension Microarray system utilizes proportional fluorescent dye microspheres, and has demonstrated successful application in several fields. The fundamental method entails coloring the microspheres with different fluorescent hues by precisely combining two separate red fluorescent dyes. The microspheres can be classified into 100 distinct varieties based on various ratios, with each type assigned a number ranging from 1 to 100. Subsequently, protein or DNA probes that match different detectors can be chemically linked to the microspheres in different quantities, enabling the simultaneous identification of 100 specific target molecules in a single sample and the collection of numerous detection data. The interconnections and interactions between biological molecules can be elucidated by concurrently identifying 100 different target molecules in a sample and combining results from various experiments.
For example, Nie's team developed a technique to combine several fluorescent colors by incorporating red, green, and blue QDs into polymer microspheres at varying levels of fluorescence intensity.77 Similarly, Xu's team used mesoporous beads of similar sizes to microcarriers in order to develop a barcode library with a large encoding capacity.78 Subsequently, they combined different proportions of red QDs in the outermost layer and enclosed varying proportions of fluorescein isothiocyanate (FITC) isomer I green dye within the mesopores. Ultimately, they employed ten specific tumor markers as exemplar targets to demonstrate the suitability of this multiplexed bioassay platform. Based on a similar idea, Pei et al. developed a detection method called SDwalker-Drop, which utilizes stochastic DNA walkers in droplets. This technique combines DNA walker technology with coded combinations of fluorescent dyes to enable simultaneous identification and multiplex detection of pathogens (Fig. 1G).79 The paper utilized a mixture of three dyes (AMCA, FAM, and TR) with distinct spectral properties, along with eight intensity levels ranging from 0 to 7. These dyes were immobilized in thiol-labeled oligonucleotide gold nanoparticles, which functioned as spherical nucleic acid probes (SNPs). Theoretically, this system could achieve a coding capacity of 83 − 1 = 511. Through experimentation, researchers successfully obtained 20 patterns for the detection and identification of bacterial phenotypes. The process of enclosing bacteria and probes within droplets that are 50 picometers in size, and the enzymatic cascade reaction of SDwalker for signal transduction and amplification caused by bacteria, significantly enhances the potential of droplet-based microfluidics to detect numerous bacterial species simultaneously. Nevertheless, this method remains unsuitable for identifying and seeing chemicals within living cells.
Klymchenko et al. addressed this issue by creating fluorescent polymer nanoparticle barcodes that are suitable for both in vitro and in vivo cellular imaging.80 Researchers used a biodegradable poly(lactic-co-glycolic acid) polymer mixed with three colors of cyanine dye in different ratios to form homogeneous 40 nm-sized RGB (red, green, and blue) barcodes. These barcodes serve the dual purpose of distinguishing between 13 different cell populations, and can be passed down through multiple cell generations. Consequently, they enable the tracking of tumor cells in live animals and facilitate cell tracing in developing embryos. Hu's team used fluorescent proteins conjugated to magnetic beads to generate encoded microspheres. These microspheres were subsequently deployed to immobilize capture probes on their surface, enabling the detection of target oligonucleotides within the pathogen.81 Recently, the technique of DNA-induced fusions has been employed to carry out numerous randomized fusions of six different populations of cargo liposomes. A total of 566 distinct fusion sequences were detected using various ratios of chromophore-encoded liposome populations, as viewed by total internal reflection microscopy. Machine learning enables the categorization of enormous amounts of data, which make it a valuable tool for high-throughput drug screening, biosynthesis, and epitope mapping.82
3.2 Fluorescence coding based on spatial geometry
The use of fluorescence emission color-based coding is often problematic in detection devices due to its susceptibility to photobleaching and the need for several lasers to match different dyes with varying excitation spectra, which adds to the complexity. However, spatial geometry-based fluorescence coding allows for the identification of many targets utilizing a reduced number of fluorescent dyes. Spatial geometric fluorescence coding is a scalable technique that allows for the adjustment of the number of tiny areas to accommodate various detection samples. The fundamental technique entails partitioning the surface of an item into several small regions and applying fluorescent dyes, either of the same or different hues, to each individual region. Subsequently, the dyes are encoded based on their placements and colors, resulting in a system that is exceptionally resilient to errors due to the ability to selectively stimulate and detect fluorescent dyes' hues.
3.2.1 Geometry-based fluorescence coding.
Geometric barcodes not only use optical features, but also function as distinct signals based on recognizable shape structures, which enables the encoding capacity to increase exponentially. Dejneka et al. employed these data to fabricate silicate glass barcodes with stripes, including rare-earth ions as dopants.83 These barcodes enable the combining of up to CN permutations of N individuals and C distinct fluorescence colors on a single strip. Their effectiveness in fluorescence determination was verified using DNA hybridization tests. However, the synthesis procedure is more difficult. Consequently, Liu's team utilized a more efficient bottom-up technique to fabricate lanthanide-doped upconversion materials on a larger scale.84 A study documented the creation of a luminous microrod using a hexagonal NaYF4 upconversion material. Additionally, researchers successfully manufactured upconversion nanocrystals with different lanthanide activators on their surface. By altering the composition of the microrods and their terminal activators, it is possible to simply control the colors of the microrods and create barcodes for use in anti-counterfeiting applications. They have recently detailed a photolithography-based technique for mass-producing small color graphic barcodes.85 In the first stage of preparation, lanthanide-doped upconversion nanoparticles (UCNPs) were synthesized to produce three main colors: red (R), green (G), and blue (B). Subsequently, the RGB nanoparticles can be mixed in varying proportions to provide an optically encoded spectrum of luminous colors, ranging from purple to red. Furthermore, a demonstration of DNA detection can be achieved by storing the information in a specific sequence of colors and making use of the uneven distribution of colors induced by the varying thickness of the inner layers of the microbarcode, resulting in distinct geometric imaging.
The improved capacity to precisely locate and adjust DNA origami technology has opened up new possibilities for using fluorescence coding methods. Yin's team utilized DNA origami techniques to create submicron fluorescent barcodes with geometric encoding. The encoding mostly involves accurately positioning fluorescent dyes on the surface in a specific spatial geometry (Fig. 2A).86 Fluorescent labeling was applied to three specific sections of a six-helix bundle of DNA nanorods, which had a length of roughly 800 nm. The labeled portions were spaced at intervals of 450 nm and 270 nm, respectively. This facilitated the differentiation of the asymmetrical patterns during the process of decoding using fluorescence microscopy. The three sections were identified using the fluorophores “blue” (B, Alexa Fluor 488), “red” (R, Alexa Fluor 647), and “green” (G, Cy3). The BRG barcode obtained is distinct from the GRB barcode because of the fluorophore labeling of the three areas. Consequently, the three fluorophores that have different spectrum properties can be utilized to generate a total of 33 = 27 unique barcodes. Subsequently, advancements were made in total internal reflection fluorescence microscopy, resulting in the ability to accurately identify 216 different barcodes (Fig. 2B). This breakthrough showcased barcodes with increased spatial information density and achieved a super-resolution of 40 nm. Wei et al. constructed DNA origami nanorods consisting of five 10-helix bundles. Each bundle had a length of approximately 250 nm and contained both fluorescent and non-fluorescent groups (Fig. 2C).87 The five monomers unite to create a 1.25 μm DNA barcode. Each 10-helix bundle can exist in two states: “on” (1) or “off” (0). When there are two or more “on” monomers in a row in the barcode, they cannot be told apart and appear as a longer “on” monomer. However, the presence of “off” monomers creates a distinction between them. This study demonstrates the utilization of these barcodes for both multiplexed single-molecule analysis and the identification of synthetic single-stranded DNA target sequences. The spatial geometric encoding capability of barcodes is improved by including fluorescent moieties of different colors. This enables the barcodes to be encoded in a spatially geometric manner, even in scenarios where there is only one color of fluorescence, as well as in cases where there are fluctuations in the intensity of fluorescence in individual units.
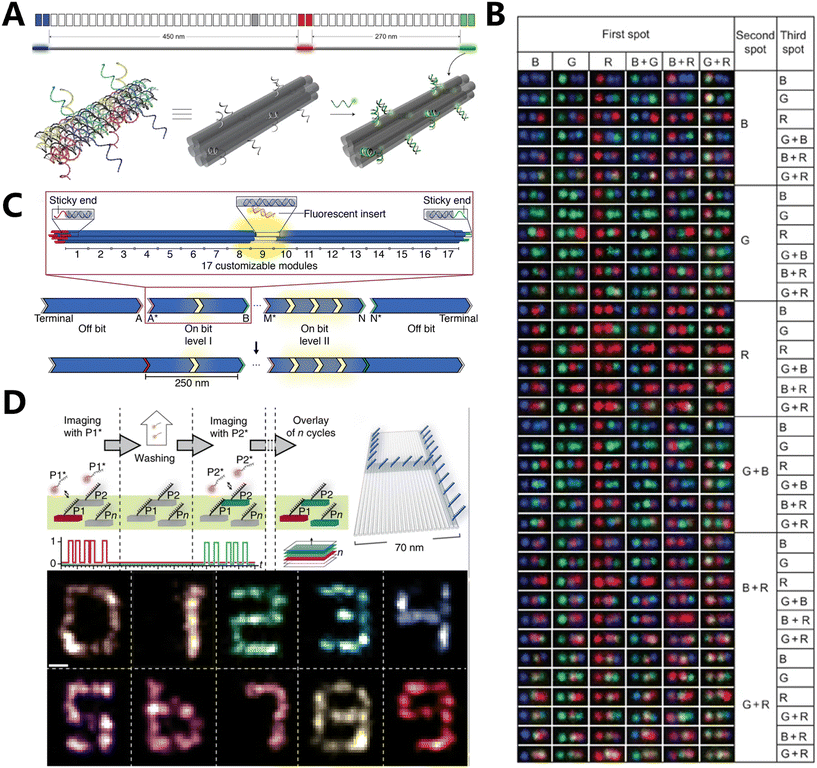 |
| Fig. 2 Fluorescence coding based on spatial geometry. (A) Three-dimensional illustrations to show the details of one fluorescently labelled zone.86 (B) Representative TIRF for 216 barcode species.86 (C) Design schematic for monochromatic fluorescent barcodes hierarchically assembled from modular DNA origami nanorods.87 (D) Exchange-PAINT schematic showing sequential imaging of multiple targets using imager strands labeled with the same fluorophore.88 | |
3.2.2 Spatial-based fluorescence coding.
In addition, Yin's group introduced a method dubbed Exchange-Paint for multiplexed 3D cellular super-resolution imaging. This technology is based on the DNA point accumulation for imaging in nanoscale topography (DNA-PAINT) method (Fig. 2D).88 DNA-PAINT is an advanced microscopy technology that utilizes DNA molecules as markers to provide exceptionally high-resolution imaging. This approach allows for imaging with a resolution of up to 25 nm by precisely manipulating the binding and dissociation of DNA.89,90 The study presents ten unique rectangular DNA origami structures that closely resemble the numerical digits from 0 to 9. The fluorescence switching between on and off states is achieved by two complementary DNA molecules: the target DNA, which is complementary to the DNA sequence of the chemical being tested, and the probe DNA, which has a fluorescent dye attached. The fluorescent dye emits a fluorescence signal to show the position of the target molecule when the target DNA and probe DNA form a complementary pairing. Using specifically designed fluidic chambers, 10 high-resolution color photographs of different digital patterns were acquired by fixing each pattern on origami structures and repeating the washing and imaging procedures. The imaging strands used were tagged with Cy3b. The capacity to control variables such as temperature and salt content enables the achievement of high-resolution imaging by influencing the rate at which DNA binds and dissociates. The position and amount of target molecules can be determined by monitoring the duration and intensity of the fluorescence signal, which is generated by the probe DNA's constant binding and dissociation during the imaging process. In the same year, Yin et al. employed the DNA origami approach to fabricate diverse three-dimensional polyhedral structures and tweaked fluorescent groups at specific locations.91 Subsequently, they employed transmission electron microscopy and three-dimensional DNA-PAINT super-resolution fluorescence microscopy to examine the configuration of individual molecules in a liquid medium.
Ganji et al. have recently employed DNA-PAINT along with the DNA nanostructure design to simultaneously detect and analyze individual dinucleotides using DNA nanotechnology. This allowed them to evaluate the energy associated with the stacking of bases at the single-molecule level.92 DNA-PAINT enables the direct measurement of the binding kinetics between fluorophore-labeled oligonucleotides and their corresponding strands of DNA origami nanostructures. The imager strands' binding kinetics are compared to the stacked and unstacked conformations of their terminal nucleotides in a single experiment using multiplexed imaging of patterned origami structures. The variation in individual base stacking energies resulted in a much wide range of stability in the residence duration during the examination of single-molecule data. Based on the observed stability of dwell duration, a distinctive grid pattern was created using five rectangular DNA origami structures: box, L, U, C, and H shapes. As a result, the structures were able to be easily differentiated in DNA-PAINT imaging, and an experiment was conducted to show that they are suitable for simultaneous super-resolution imaging. In addition to this, Jungmann et al. used a modified version of DNA-PAINT called Exchange-PAINT, which was combined with orthogonal DNA barcode imaging and wash cycles. This enabled them to achieve sequential target multiplexing and imaging through sequences, resulting in improved resolution. They referred to this technique as resolution enhancement by sequential imaging (RESI).93 This study confirms that RESI is capable of conducting intramolecular imaging in intact cells, thereby connecting the realms of super-resolution microscopy and structural biology research.
3.3 Fluorescence coding based on fluorescence properties
Although spatial geometric fluorescence encoding can enhance detection resolution and enable numerous detections using a reduced number of fluorescent moieties, it necessitates the fixing of fluorescent dyes in specific spatial places, which increases the complexity of design and synthesis. Furthermore, the encoding capacity remains restricted as a result of the object's dimensions, rendering it inappropriate for detection settings that need encoding with high capacity. It is also unsuitable for analyzing single-cell samples if the object's size is too large. Xie et al. employed the inherent characteristics of fluorescent groups, in particular fluorescence anisotropy (FA) and fluorescence polarization (FP),94 to encode and address these issues (Fig. 3A).95 The purpose of FA-based encoding in polystyrene microspheres was to encode and multiplex five DNAs by converting them into encoding signals. This was achieved by utilizing FRET, which causes a decrease in steady-state FA as the dye loading increases. Additionally, a combination of dyes with varying intrinsic FA values leads to different FA values at the same fluorescence intensity level. Meanwhile, Zhang's team has created a new type of biosensor called fluorescence anisotropy reporter (FLARE), which uses a technique called homochromatic FRET to measure the interaction between two fluorescent proteins. The biosensor detects changes in the conformation of molecular switches by analyzing the loss of polarization of emitted light by fluorescence polarization microscopy to observe the FA of the sensor. The biosensor is used to monitor specific biochemical activities (Fig. 3B).96 Research uses the monochromatic sensor's ability to integrate multiple signals and provides quantitative measurements utilizing various color variations. This enables the simultaneous imaging of three active reporter genes in a single cell, demonstrating multiplexed capabilities.
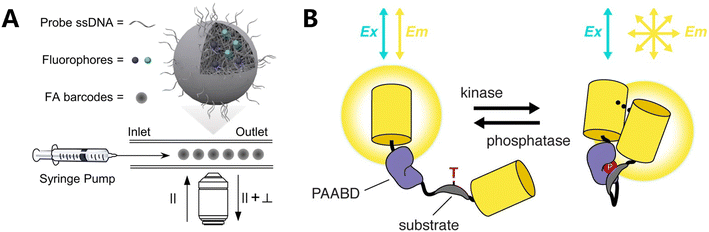 |
| Fig. 3 Fluorescence coding based on fluorescence properties. (A) Illustration of FA-encoded microspheres encapsulating lipophilic fluorescent labels inside PS microspheres for multiplexed biochemical detection on an FA imaging platform. The surface of the microspheres was covalently modified with DNA probes.95 (B) Schematic of a kinase activity FLARE.96 | |
3.4 Fluorescence coding based on the time dimension
Although fluorescence-based encoding enables cell detection and precise size control, it is limited by issues such as excessive background signals, intensity-signal artifacts, and the possibility of false-positive results. Therefore, the advancement of technologies that allow for the regulation of luminescence sequences and the use of micro- and nanomaterials with extended fluorescence lifetimes has led to the emergence of new possibilities for high-throughput detection. Additionally, these materials enhance the detecting signal range.
3.4.1 Fluorescence coding based on chronological order.
The optical resolution has achieved nanometer precision that was previously unseen, reaching levels as low as a few nanometers. This advancement has been made possible through the development of super-resolution optical techniques such as stochastic optical reconstruction microscopy (STORM)97,98 and time-dependent single-photon microscopy.99 Additionally, the time dimension can also be utilized for encoding purposes.
Reif et al. created a temporal reporter framework comprising fluorescent moiety reporter strands and DNA devices.100 Upon attaching the reporter strand to the DNA device, a momentary fluorescent spot becomes visible when observed using a microscope. In the study, the fluorescent intensity traces that are captured over a sufficient duration of time are referred to as temporal DNA barcodes. This is because the random intensity trajectories vary for each DNA device, enabling the distinction of individual molecules. Meanwhile, they introduce a method for barcoding single molecules that does not require amplification and relies on time-based signals (Fig. 4A).101 This is achieved by using reporter gene strands colored with dyes, which temporarily connect to specific nucleic acid strands and produce signals with different intensities over time. The DNA strands are designed to produce distinct temporal signals that can be used for molecular-scale identification.
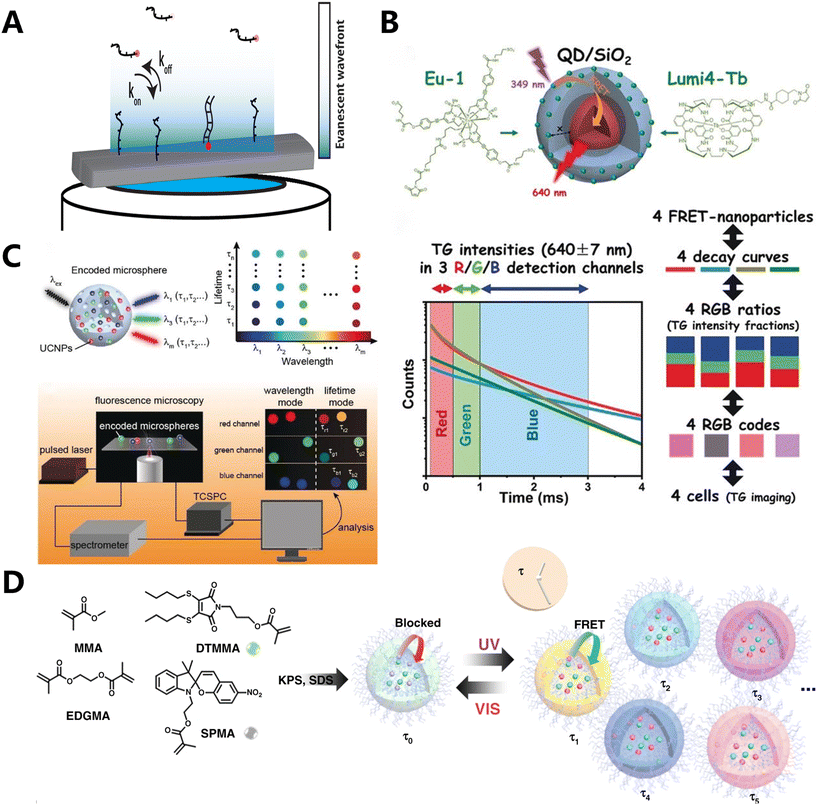 |
| Fig. 4 Fluorescence coding based on the time dimension. (A) Overview of the temporal barcoding framework.101 (B) A prototypical system for FRET lifetime encoding with individual nanoparticles.104 (C) Manipulating the luminescence emission color and decay lifetimes of UCNPs (designated as τλ-UCNPs) to create 2D barcodes.107 (D) Fabrication of the photo-switchable polymeric nanogels.108 | |
3.4.2 Fluorescence coding based on fluorescence lifetime.
In addition to encoding signals based on the frequency and duration of fluorescence flicker, fluorescence lifetime was used for detecting encoded signals,102,103 which is not influenced by either wavelength or intensity. This effectively removes the possibility of any interference caused by other encoded signals. Furthermore, the advancement of time-resolved techniques has enabled the differentiation of luminescence lifetimes with identical emission wavelengths. Therefore, in the context of multiplexed bioanalysis, it provides an opportunity to enhance the capacity for encoding when integrated with other optical dimensions.
Based on the aforementioned fluorescence characteristics, Hildebrandt et al. identified and characterized two distinct types of QDs coated with a layer of SiO2 and encased by two different rare earth complexes (Tb and Eu). The thickness of the SiO2 shell layer varied between 6 nm and 12 nm (Fig. 4B).104 Conventional organic dyes are often used because they are affordable, come in a wide range of options, and have a high quantum yield. However, their application is hampered by their broad luminescence spectrum and less persistent fluorescence, which makes them prone to photobleaching. QDs105,106 outperform traditional fluorescent dyes in several aspects, such as enhanced photostability, increased fluorescence intensity, and the ability to generate various fluorescence hues using the same wavelength by adjusting their size or composition. The text witnessed four separate photoluminescence (PL) decays due to FRET from Tb or Eu donors to QD acceptors. The discovery of these decays occurred in three distinct time-detection windows employing time-gated (TG) luminescence intensity monitoring. Consequently, the attachment of Eu or Tb complexes to QDs with two different SiO2 coating thicknesses resulted in the production of four distinct QD luminescence decays within three detection windows labeled as red (R), green (G), and blue (B). Each of these decays exhibited distinct variations in light intensity. Consequently, unique RGB codes were created for each of the four nanomaterials, taking into account the different levels of intensity seen in these three detection windows. The ability to identify and track several cells was demonstrated in this manner.
Despite the advancements made in utilizing QDs for fluorescence lifetime encoding, these materials still exhibit very brief lives ranging from 10 to 100 nanoseconds, which endow them with the potential for heightened signal interference. Consequently, there is a preference for rare earth metal compounds that exhibit luminescence durations ranging from microseconds to milliseconds and are encoded utilizing FRET based on UCNP. Zhang's team systematically design a methodology for manipulating the luminescence emission color and decay lifetimes of UCNPs (referred to as τλ-UCNPs) (Fig. 4C).107 By adjusting the shell thickness and controlling the FRET, the luminescence intensity of these particles can be modified. This allows for the encoding of the τλ-UCNPs based on their emission color and luminescence decay lifetime. Subsequently, they generated microspheres encoded with lifespan wavelength binary information using τλ-UCNPs and porous polystyrene (PS) microspheres. This unique color-lifetime encoding approach was used to evaluate nine subtypes of human papillomavirus (HPV) in patient samples. The encoding capacity of this strategy is improved by three orders of magnitude compared to the standard strategy. This demonstrates the strategy's capacity for efficient and rapid identification of biological samples.
Irrespective of the concentration of the material and the technique used for measurement, employing fluorescence lifetimes as readouts produces more precise and consistent results compared to conventional fluorescent barcodes. Nevertheless, the absence of methods to enable temporal and spatial control over the encoding process has limited the application of fluorescence lifetimes in this domain. Although there has been significant advancement in using semiconductor QDs and lanthanide complexes for encoding, achieving real-time adjustment of fluorescence lifetimes remains a challenge due to the poisonous nature of QDs.
O'Reilly et al. synthesized a two-component switching nanogel by utilizing spiropyran (SP) monomers and methacrylate maleimide dithiomaleimides (DTMs) (Fig. 4D).108 DTMs, being non-toxic and capable of modifying substituents, can be utilized to alter the fluorescence properties. By combining methyl methacrylate (MMA) as the hydrophobic matrix and ethylene glycol dimethacrylate (EGDMA) as the cross-linking agent, crosslinked nanogels are synthesized. These nanogels have different ratios of DTMMA and SPMA. Upon exposure to light, a photoisomerization-induced FRET process occurs. This enables the real-time adjustment of fluorescence lifespan by the manipulation of light. A variety of nanogels with a similar surface chemistry but wider dynamic lifetimes were used for encoding and counting different systems, after the successful implementation of dynamic photocontrol of fluorescence durations.
4. Application of fluorescence coding technology in RNA detection
RNA molecules have a low molecular weight, exhibit large variability in their abundance and play a crucial role in guiding protein synthesis within cells. Recent studies have shown that RNA expression differs between normal cells and those cells affected by various diseases, which makes it a valuable biomarker for diagnosing disorders. Studying RNA expression and control can contribute to tumor diagnosis and treatment by improving the understanding of different tumor mechanisms.
4.1 Proportional type-based fluorescence coding in RNA detection
4.1.1 Fluorescence coding based on DNA nanostructures in RNA detection.
DNA nanostructures as coding carriers provide a high level of programmability and hold significant promise for the advancement of fluorescence intensity coding with increased capacity. This is achieved by randomly altering the number of ratios between the dyes. Mi et al. developed a fluorescence intensity coding approach using DNA tetrahedra as a carrier to detect three cancer-associated miRNAs (Fig. 5A).109 This method involves using different ratios of Cy3 and Cy5 dyes and taking use of the FRET effect. By altering the quantity and positioning of Cy3 and Cy5 labels on the four corners of the DNA tetrahedron, a total of 26 samples were generated. A detection method based on a barcode probe was implemented to create an in vitro sensing platform for identifying three specific miRNA targets: miRNA-210, miRNA-199a, and miRNA-21. A code library for fluorescence intensity was created using a range of FRET intensities. The high sensitivity and specificity of the barcode probe were confirmed using miRNA-210 as a target model.
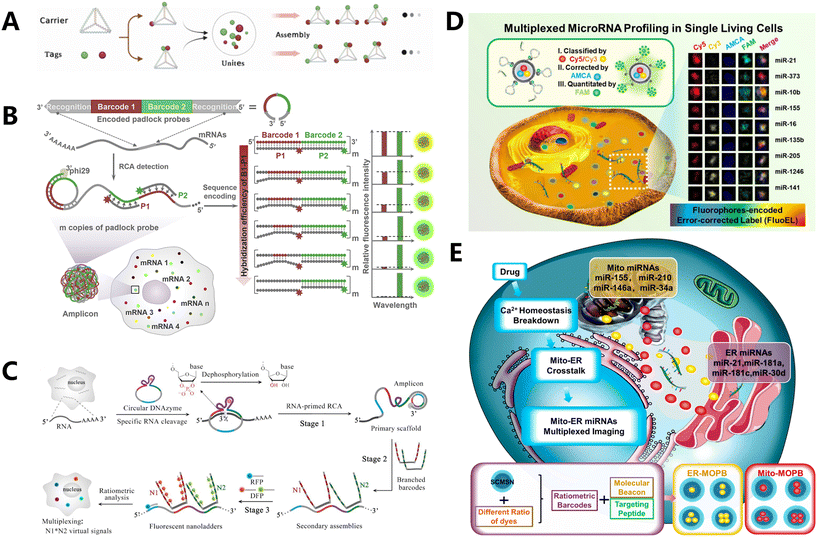 |
| Fig. 5 Proportional type-based fluorescence coding in RNA detection. (A) Construction procedure and in vitro multiplexed sensing application of Tetra-FICT.109 (B) Schematic illustration of sequence-encoded amplicon (SeqEA) for multiplexed imaging of individual RNAs in single cells.110 (C) Overview of hierarchical DNA branch assembly-encoded fluorescent nanoladders for single-cell transcript imaging.111 (D) Schematic of FluoELs for multiplexed miRNA detection.113 (E) Scheme of subcellular miRNAs' multiplexed detection using ratiometric barcodes in living cells.114 | |
4.1.2 Fluorescence coding based on nucleic acid amplification technology in RNA detection.
RNA segments with low expression levels can be enhanced by amplifying them utilizing nucleic acid amplification technology to increase their output signal. Li's team introduced a DNA sequence-encoded fluorescent barcode called sequence-encoded amplicon (SeqEA). This barcode utilizes controlled DNA hybridization to accurately encode barcodes with different fluorescence intensities. These barcodes can be rolled over and amplified, allowing for the simultaneous imaging of multiple single-molecule RNAs in individual cells (Fig. 5B).110 Their lock-in probes utilize barcode (B1 and B2) and recognition (R) modules for encoding. Hybridizing the target mRNA to the R module initiates the RCA process, leading to the creation of nanoparticles consisting of lengthy DNA amplicons including several lock-in probe repetitions. The visualization of these nanoparticles is achieved by hybridizing them with fluorescently tagged detection probes (P1 and P2). The quantity of detection probe P1 that attaches to the RCA amplicon and marks certain mRNAs can be accurately regulated by altering the sequence of the B1 barcode module. This enables the exhibition of multiplexed detection of RNAs at the individual molecule level. This technique can be utilized for studying the regulation of gene expression and analyzing the behavioral changes of mRNAs at the single-cell level. However, it necessitates cell fixation and permeabilization processes to introduce probes, which implies that it cannot be used for imaging multiple living cells simultaneously. According to this, Zhao's group implemented the use of branching DNA for encoding (Fig. 5C).111 The method employs three consecutive DNA assemblies to enhance the accuracy of its detection. First, it utilizes deoxyribozyme-incorporated circular probe-mediated RNA self-initiated RCA. Second, it incorporates branching DNA barcodes that are linked to the corresponding RNA-primed amplicons. By repeating these sequences, the number of detecting fluorescent probes (DFPs) that are stably bound to the barcodes can be determined. Here, ten branching barcodes were used to broaden the possibilities for designing branching DNA barcodes with distinct fluorescence characteristics. The range of DFPs spans from one to ten, hence expanding the assortment of DNA barcode patterns that exhibit diverse fluorescence characteristics. In conclusion, the arrangement of fluorescent probes on branched DNA enables the representation of fluorescence intensities and spectra. This report showcases the findings of a study that involved the simultaneous imaging of nine RNA samples utilizing two different detection spectral channels and three repeated sequences.
4.1.3 Fluorescent microsphere coding with different proportions in RNA detection.
The simultaneous employment of numerous unique fluorophores and intensity levels dramatically increases the number of targets that fluorescent microspheres can detect. Sun et al. developed fluorescence-encoded microspheres by attaching custom-designed stem-loop structured probes to silica cores and shells in three distinct proportions of two-color QDs. These microspheres can be used for precise target detection and amplification.112 The presence of a miRNA target leads to the generation of a significant quantity of stem-G-quadruplexes through target-triggered exponential amplification. The fluorescent G-quadruplex intercalator can selectively signal them, resulting in a change in the color of the microsphere emission. Consequently, changes in emission color that depend on the abundance of the target can be utilized to visually and directly identify specific miRNA targets.
Most fluorescence-encoded microspheres currently available in the market range in size from 2 to 100 micrometers, which poses challenges in their detection within living cells. Furthermore, biomolecules are arranged in a random manner within the internal space of the cell. The complex environment within the living cell can easily lead to uneven refraction of fluorescence, resulting in a divergence of the detection signal. Our team developed a technique called fluorophore-encoded error-corrected labels (FluoELs), which is a form of fluorescence labeling coding with error correction. This technology allows for accurate examination of marker molecules in living cells (Fig. 5D).113 Living cellular miRNAs can be identified using FluoELs, which consist of cell-safe silica nanoparticles (MSN) as the structural framework and varying proportions of coding bi-fluorescent fluorophores (Cy5 and Cy3) contained in the core. In this study, a fixed amount of AMCA fluorescence was used to correct the quantitative bias caused by refractive fluctuations in living cells. After fabricating the fluorescent coding spheres, a 20 nm silica shell layer was added to the outer surface. This layer allowed for the loading of more molecular beacon (MB) detection probes with the right size and low cytotoxicity. Additionally, the structure prevented FRET between the signal recognition component of MB probes and the coding dye, enabling specific detection of miRNAs. This method allows for accurate quantification and in situ detection of nine specific miRNAs in breast cancer cells. It also permits the examination of variations within individual cells and the quantitative assessment of miRNA levels in living cells. Using this information, we proceeded to develop multiplexed organelles portrait barcodes (MOPB), an innovative fluorescence coding method that utilizes heterochromatic dye-encoded barcodes to identify miRNAs in different locations of organelles within living cells (Fig. 5E).114 The shell–core mesoporous silica nanoparticle (SFMSN) was loaded with varying proportions of AMCA, Cy3, and Cy5 dyes, ensuring good biosafety. The surface of these nanoparticles was then modified with organelle targeting peptide (TP) and MB detection probes, resulting in the formation of MOPBs. The paper presented a model of the endoplasmic reticulum (ER) and mitochondria (Mito). Four distinct ratios of Cy3/AMCA dyes were employed to create SFMSN-encoding spheres. These spheres were then utilized to generate ER-MOPB, which targets the ER, and Mito-MOPB, which targets the Mito. ER-TP and Mito-TP were combined with the corresponding MOPBs with MB probes that covalently bind and react with target miRNA. Intracellular miRNAs were quantified by measuring the fluorescence intensity of FAM on MB. This allows for the accurate identification of miRNAs from eight distinct organelles, facilitating the discovery of biomarkers within the subcellular milieu. Similarly, He et al. suggested utilizing apolipoproteins as carriers to create fluorescence-encoded microspheres. These microspheres were loaded with two different fluorophores (fluorescein and rhodamine B) in seven different amount ratios. The purpose was to detect and visualize seven specific mRNA targets in situ.115 Chen's team constructed the core of the sensor using UCNPs, which are nanoparticles that obtained three fluorescence-encoded probes by embedding Cy3 and Cy5 in a proportional manner into poly-L-lysine-packaged UCNPs (PLL-UCNPs). Each barcode in the sensor simultaneously packaged the same number of AMCA fluorophores for error correction.116 Finally, they acquired in situ pictures of the three separate miRNAs.
4.2 Fluorescence coding based on spatial geometry in RNA detection
4.2.1 Geometry-based fluorescence coding in RNA detection.
The coding theme, which is based on geometries, exhibits exponential scaling for asymmetric particles in the form of CS and for symmetric particles in the form of CS/2. Here, C represents the number of distinguishable spectral features (‘colors’) and S represents the number of spatial features (‘stripes’). Hence, by combining a limited palette of colors and a correspondingly limited number of stripes, one can harness a substantial coding potential that rapidly amplifies with minor modifications to either parameter. Doyle et al. utilized a combination of geometric patterning, rare-earth upconversion nanocrystals, single-wavelength near-infrared excitation, and portable charge-coupled device (CCD)-based decoding to create a set of encoded barcodes that can differentiate between particles synthesized by flow lithography (Fig. 6A).117 This design exhibited an extremely low rate of false positives in decoding (<10−9). It also had a huge encoding capacity that could scale exponentially (>106 particles). Additionally, it has the capability to detect miRNA-210 and miRNA-221. Kim's group proposes utilizing primer immobilization network particles as independent reactors to investigate the expression of several miRNA targets (Fig. 6B).118 Particles ranging in size from 200 to 500 μm were recognized using two-dimensional codes engraved on the particles. This advanced method of analysis allowed for the simultaneous analysis of 10 miRNAs from extracellular vesicles (EVs) in a single chamber, greatly increasing the efficiency and multiplicity of the study. Based on this, Doyle's group conducted a multiplexed detection assay of miRNAs in colon tumor tissue utilizing a biocompatible hydrogel that is more biocompatible, non-contaminating for graphic coding, and assessable using a basic mobile phone camera.119
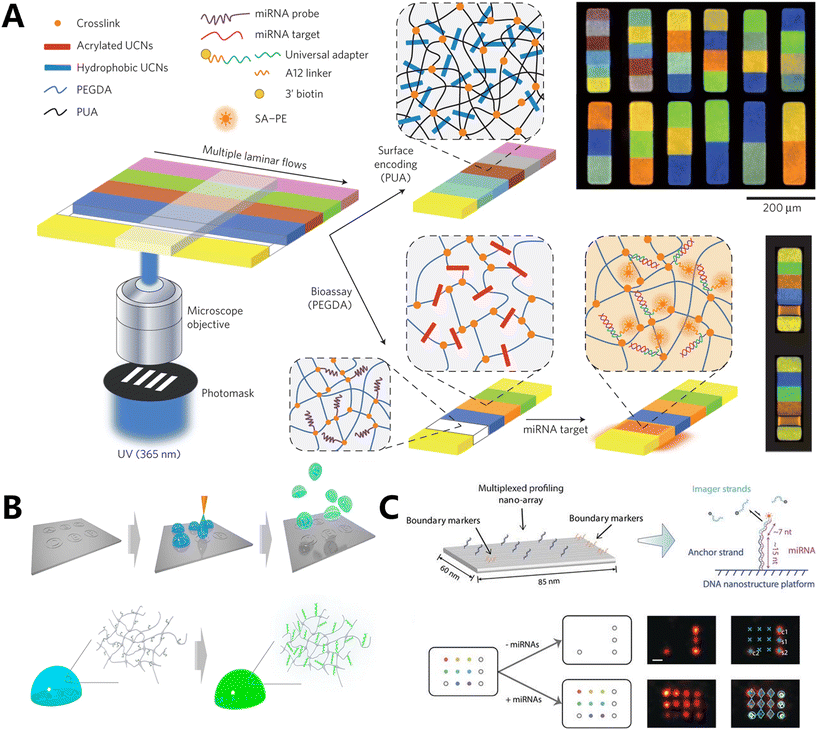 |
| Fig. 6 Fluorescence coding based on spatial geometry in RNA detection. (A) Synthesis of encoded particles by stop-flow lithography.117 (B) Lithographically encoded microparticle-primer immobilized network (LEM-PIN).118 (C) Principle of super-resolution geometric barcoding.120 | |
4.2.2 Spatial encoding-based fluorescence coding in RNA detection.
The spatial encoding-based approach offers several advantages including high sensitivity and specific single-molecule assays. It also has a high degree of multiplexing capability, indicating that it can detect multiple targets simultaneously. Unlike methods that rely on spectrally separable fluorophores or plasma interactions, the spatial encoding-based approach is not limited by the number of probe species that can be distinguished by their spectral properties.
Dai's group created a method for miRNA profiling that is extremely specific to sequences and allows for multiplexing (Fig. 6C).120 This method utilizes DNA nanostructures and repeating probe binding, which enables super-resolution geometric barcoding. In order to trap and render the relevant miRNAs immobile, they initially functionalized origami structures that were only partially complementary to the target miRNA sequences (13–17 nT) on a glass surface. The unpaired region that miRNAs capture functions as a binding site for DNA-PAINT technology imaging. This binding is detected and identified through the temporary pairing of complementary imaging strands that are labeled with fluorescent markers. The work employed a square lattice configuration, with a 20 nm gap between each of the four convenient markers and eight anchoring strands, to attain a high level of multiplexing capability. This configuration enabled the simultaneous identification and quantification of eight different miRNAs.
4.3 Fluorescence coding based on fluorescence properties in RNA detection
FA has great potential for imaging biomolecules and performing multiplexed analysis since it can efficiently distinguish fluorophores that have similar emission spectra. Regrettably, the extensive use of this substance in the field of biology and biotechnology has been impeded by its susceptibility to alterations in the environment.
Li et al. achieved precise control over the FA level and stability of a fluorophore in complex situations by attaching fluorescent motifs at various locations on the DNA tetrahedron (Fig. 7A).121 First, the researchers created a DNA tetrahedral structure to control the FA properties of the fluorophore, known as FA DNA frameworks (FAFs). They devised three methods to position the fluorophore Alexa 647: first, by attaching it to the edge of the structure, second, by attaching it to the 4th or 11th nucleotide protruding from the 13th nucleotide on the structure, and third, by placing it at the 11th nucleotide on the structure, extending inward from the 8th nucleotide (Fig. 7B). They discovered that the shorter overhanging structure resulted in higher FA. Furthermore, the fluorophore positioned within the structure exhibited higher FA compared to when it was positioned outside the structure. This suggests that different positions on the structure can influence the movement of the fluorophore, leading to variations in FA. By merging alterations in FA with two distinct fluorescent hues of fluorophores, they achieved barcodes including eight unique pseudo-colors that can be used for miRNA analysis and cell labeling.
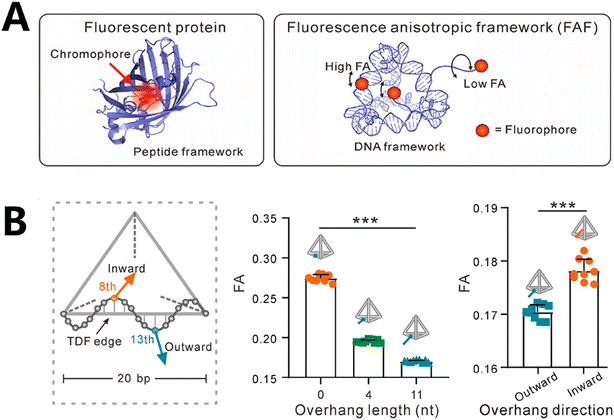 |
| Fig. 7 Fluorescence coding based on fluorescence properties in RNA detection. (A) Schematic of the peptide-like framework structure accommodating fluorophores with controllable FA features.121 (B) Regulation of FA by adjusting the fluorophores' placement on FAFs.121 | |
4.4 Fluorescence coding based on the time dimension in RNA detection
4.4.1 Fluorescence coding based on chronological order in RNA detection.
Super-resolution imaging has led to the emergence of a new category of passive imaging techniques known as DNA-PAINT. By transferring the programming of reporter genes from dye color to DNA sequence, they can achieve the multiplexing of highly monochromatic optics. Jungmann's team showcased a novel technique in super-resolution microscopy that allows for the simultaneous detection and imaging of RNA and proteins in cells (Fig. 8A).122 This was achieved by manipulating the flicker frequency and duration of the target fluorescence using the DNA-PAINT technique. By combining different fluorescence spectra, the team was able to achieve 124 different types of super-resolution imaging in just a few minutes. Two methods were employed to control the scintillation frequency of the target. First, the scintillation frequency was observed to increase linearly with the number of binding sites, as demonstrated by comparing samples labeled with one binding site and three binding sites. Second, the duration of the scintillation was altered by adjusting the length of the connecting strand. It was found that the longer the strand, the longer the duration of the scintillation. Using this approach, DNA origami structures were created with three distinct binding sites at positions 0, 3, 9, 22, and 44. These structures were then labeled with fluorescent dyes in three different colors: red, green, and blue (Fig. 8B). This resulted in a total of 53 − 1 = 124 target structures, which could effectively differentiate between TFRC and MKI67 mRNA molecules. This demonstrates the capability of RNA imaging detection and highlights its potential for use in cellular multiplex detection.
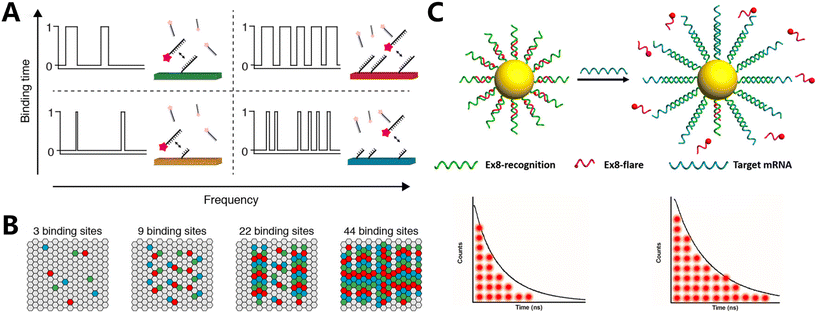 |
| Fig. 8 Fluorescence coding based on the time dimension in RNA detection. (A) Simultaneous multiplexed super-resolution imaging by engineering blinking kinetics.122 (B) Frequency-based 124-plex super-resolution imaging.122 (C) Nanofilm synthesis and experimental schematic.123 | |
4.4.2 Fluorescence coding based on fluorescence lifetime in RNA detection.
Fluctuations in fluorophore concentration and light can sometimes affect fluorescence intensity measurements. A highly effective alternative, which is not affected by variations in fluorescence intensity or initial excitation circumstances (such as wavelength), is the assessment of fluorescence lifetime. This method effectively eliminates any interference caused by background materials and fluctuations in excitation.
Sun et al. employed fluorescent lifetime imaging of nanoflares to specifically detect endogenous mRNAs (Fig. 8C).123 The researchers generated nanoflares by forming AuNP–DNA complexes and attaching Cy5-modified oligonucleotides to gold nanoparticles, they observed a significant decrease in fluorescence lifespan. In the presence of the target mRNA, the recognition sequence on the AuNP would form a hybrid with the target, resulting in an extension of the fluorescence lifetime. The suitability of this approach for identifying endogenous mRNAs was assessed using MDA-MB-231 cells. Fluorescence lifetimes can be accurately determined by studying the fluorescence decay trails in cells, which allows for the detection of target mRNAs in cells. This presents novel possibilities for the detection of a wide range of supplementary biomolecules.
5. Summary and outlook
Fluorescence coding has emerged as a crucially pivotal technique in biomedical detection due to its versatility, sensitivity, and multiplexing capabilities. This methodology involves tagging molecules with fluorescent labels based on the fine control of fluorescence scaling type, spatial geometry, specific fluorescence properties, and time dimensions, allowing for their detection and quantification by fluorescence microscopy or spectroscopy. Based on its high throughput, high spatiotemporal resolution, and real-time monitoring, fluorescence coding has shown great potential in the tracing of pathophysiological microenvironment variations. Developing a fluorescence coding technique for RNA detection in anomalous microenvironments offers numerous advantages in the diagnosis and tracing of diverse pathophysiological processes that have revolutionized the field of molecular biology and biomedicine.
Fluorescence coding allows for the simultaneous detection of multiple RNA targets within the same sample, attributing to encoding fluorescent motifs in multiple ways, the multiplexing capability of which is invaluable for studying complex biological processes. By employing different fluorescent labels with distinct emission spectra, different proportions, and spatial geometry, and using nanomaterials, researchers can detect multiple RNAs simultaneously, distinguish between various RNA species, and monitor their dynamics in real time, providing insights into cellular responses to stimuli or disease states.
However, there are several challenges in RNA detection by fluorescence coding techniques. Low signal intensity and high background noise in physiological environment can obscure the detection of low-abundance RNA targets, limiting the utility of fluorescence coding techniques for sensitive biomarker detection and quantification. To solve the problem, multiplexed fluorescence imaging strategy based on multicolor encoded hybridization chain reaction amplifiers can improve the sensitivity of fluorescence coding techniques, enabling the detection of low-abundance RNA targets with improved signal-to-noise ratios. Furthermore, fluorescence coding can be combined with advanced imaging techniques such as fluorescence in situ hybridization (FISH) or single-molecule imaging, to visualize RNA molecules with high spatial resolution. It allows researchers to study RNA localization, trafficking, and dynamics within subcellular compartments or complex tissue environments, providing mechanistic insights into RNA-mediated biological processes.
In summary, fluorescence coding has become an indispensable tool for RNA detection in biomedical research, offering sensitivity, multiplexing capabilities, and compatibility with high-throughput screening platforms. By harnessing the power of fluorescence coding technology, researchers can elucidate the roles of RNA molecules in health and disease conditions, paving the way for the development of novel diagnostics and therapeutic interventions.
Author contributions
Junren Wang: investigation, and writing – original draft, review and editing. Qin Xiang: writing – review and editing, and supervision. Haifeng Dong: project administration, funding acquisition, and writing – review and editing. Xueji Zhang: resources, project administration, and supervision.
Conflicts of interest
The authors have no conflicts of interest to declare.
Acknowledgements
The work was supported by Special Foundation for State Major Research Program of China (Grant 2022YFB3207202), National Natural Science Foundation of China (Grant 22374102), Shenzhen Medical Research Fund (Grant D2301011), Guangdong Province Pearl River Team (2021ZT09C289), Shenzhen Key Laboratory for Nano-Biosensing Technology (ZDSYS20210112161400001). We thank Instrumental Analysis Center of Shenzhen University for the assistance with the Electron Microscope/Nuclear Magnetic Resonance spectroscope/Mass Spectrometer/Confocal Microscope/Small Animal Diagnostics Imaging/Material Characterization technical support.
References
- R. Ren, S. L. Cai, X. N. Fang, X. Y. Wang, Z. Zhang, M. Damiani, C. Hudlerova, A. Rosa, J. Hope, N. J. Cook, P. Gorelkin, A. Erofeev, P. Novak, A. Badhan, M. Crone, P. Freemont, G. P. Taylor, L. H. Tang, C. Edwards, A. Shevchuk, P. Cherepanov, Z. F. Luo, W. H. Tan, Y. Korchev, A. P. Ivanov and J. B. Edel, Nat. Commun., 2023, 14, 7362 CrossRef CAS PubMed.
- T. R. Mempel, M. J. Pittet, K. Khazaie, W. Weninger, R. Weissleder, H. von Boehmer and U. H. von Andrian, Immunity, 2006, 25, 129–141 CrossRef CAS PubMed.
- A. Raj, P. van den Bogaard, S. A. Rifkin, A. van Oudenaarden and S. Tyagi, Nat. Methods, 2008, 5, 877–879 CrossRef CAS PubMed.
- W. Q. Zhang, B. J. Du, M. H. Gao and C. H. Tung, ACS Nano, 2021, 15, 16442–16451 CrossRef CAS PubMed.
- S. Wang, W. X. Ren, J. T. Hou, M. Won, J. An, X. Y. Chen, J. Shu and J. S. Kim, Chem. Soc. Rev., 2021, 50, 8887–8902 RSC.
- D. H. Li, R. S. Gamage, A. G. Oliver, N. L. Patel, S. Muhammad Usama, J. D. Kalen, M. J. Schnermann and B. D. Smith, Angew. Chem., Int. Ed., 2023, 62, e202305062 CrossRef CAS PubMed.
- D. Li, X. Q. Deng, Z. R. Xu, D. L. Wang, G. X. Xu, P. Y. Zhang, P. Qiu, W. X. Xie, D. Wang, B. Z. Tang and K. Wang, Adv. Funct. Mater., 2023, 33, 2303967 CrossRef CAS.
- B. Huang, M. Bates and X. W. Zhuang, Annu. Rev. Biochem., 2009, 78, 993–1016 CrossRef CAS PubMed.
- L. Wei, Z. Chen, L. Shi, R. Long, A. V. Anzalone, L. Zhang, F. Hu, R. Yuste, V. W. Cornish and W. Min, Nature, 2017, 544, 465–470 CrossRef CAS PubMed.
- F. H. Hu, C. Zeng, R. Long, Y. P. Miao, L. Wei, Q. Z. Xu and W. Min, Nat. Methods, 2018, 15, 194–200 CrossRef CAS PubMed.
- T. Konry, R. B. Hayman and D. R. Walt, Anal. Chem., 2009, 81, 5777–5782 CrossRef CAS PubMed.
- C. C. Li, H. Y. Chen, X. Luo, J. Hu and C. Y. Zhang, Chem. Sci., 2021, 12, 12407–12418 RSC.
- Z. Cao, L. Wang, R. Liu, S. Lin, F. Wu and J. Liu, Mater. Today Bio, 2022, 15, 100311 CrossRef CAS PubMed.
- G. J. Goodall and V. O. Wickramasinghe, Nat. Rev. Cancer, 2020, 21, 22–36 CrossRef PubMed.
- C. Buccitelli and M. Selbach, Nat. Rev. Genet., 2020, 21, 630–644 CrossRef CAS PubMed.
- S. Q. Huang, B. Sun, Z. P. Xiong, Y. Shu, H. H. Zhou, W. Zhang, J. Xiong and Q. Li, J. Exp. Clin. Cancer Res., 2018, 37, 1–11 CrossRef CAS PubMed.
- H. F. Dong, J. P. Lei, L. Ding, Y. Q. Wen, H. X. Ju and X. J. Zhang, Chem. Rev., 2013, 113, 6207–6233 CrossRef CAS PubMed.
- G. Faure, A. Y. Ogurtsov, S. A. Shabalina and E. V. Koonin, Nucleic Acids Res., 2016, 44, 10898–10911 CrossRef CAS PubMed.
- F. Q. Li, X. Q. Zhang, W. Ho, M. P. Tang, Z. Y. Li, L. Bu and X. Y. Xu, Nat. Commun., 2023, 14, 4223 CrossRef CAS PubMed.
- S. L. Cao, J. R. Wang, S. X. Ji, P. Yang, Y. Y. Dai, S. Guo, M. D. Montierth, J. P. Shen, X. Zhao, J. X. Chen, J. J. Lee, P. A. Guerrero, N. Spetsieris, N. Engedal, S. Taavitsainen, K. X. Yu, J. Livingstone, V. Bhandari, S. M. Hubert, N. C. Daw, P. A. Futreal, E. Efstathiou, B. Lim, A. Viale, J. J. Zhang, M. Nykter, B. A. Czerniak, P. H. Brown, C. Swanton, P. Msaouel, A. Maitra, S. Kopetz, P. Campbell, T. P. Speed, P. C. Boutros, H. T. Zhu, A. Urbanucci, J. Demeulemeester, P. Van Loo and W. Y. Wang, Nat. Biotechnol., 2022, 40, 1624–1633 CrossRef CAS PubMed.
- N. Y. Wang, J. Zheng, Z. Chen, Y. Liu, B. Dura, M. Kwak, J. Xavier Ferrucio, Y. C. Lu, M. M. Zhang, C. Roden, J. J. Cheng, D. S. Krause, Y. Ding, R. Fan and J. Lu, Nat. Commun., 2019, 10, 95 CrossRef PubMed.
- R. L. Liu, C. L. Liu, X. Z. He, P. Sun, B. Zhang, H. R. Yang, W. Y. Shi and Q. G. Ruan, Nat. Commun., 2022, 13, 3545 CrossRef CAS PubMed.
- R. Bhayadia, K. Krowiorz, N. Haetscher, R. Jammal, S. Emmrich, A. Obulkasim, J. Fiedler, A. Schwarzer, A. Rouhi, M. Heuser, S. Wingert, S. Bothur, K. Döhner, T. Mätzig, M. Ng, D. Reinhardt, H. Döhner, C. M. Zwaan, M. V. D. H. Eibrink, D. Heckl, M. Fornerod, T. Thum, R. K. Humphries, M. A. Rieger, F. Kuchenbauer and J.-H. Klusmann, J. Clin. Oncol., 2018, 36, 1007–1016 CrossRef CAS PubMed.
- A. Dhawan, J. G. Scott, A. L. Harris and F. M. Buffa, Nat. Commun., 2018, 9, 5228 CrossRef CAS PubMed.
- M. N. Islam, M. K. Masud, M. H. Haque, M. S. A. Hossain, Y. Yamauchi, N. T. Nguyen and M. J. A. Shiddiky, Small Methods, 2017, 1, 1700131 CrossRef.
- C. G. Sheng, J. Zhao, Z. H. Di, Y. Y. Huang, Y. L. Zhao and L. L. Li, Nat. Biomed. Eng., 2022, 6, 1074–1084 CrossRef CAS PubMed.
- X. C. Lu, K. Y. S. Kong and P. J. Unrau, Chem. Soc. Rev., 2023, 52, 4071–4098 RSC.
- T. Jet, G. Gines, Y. Rondelez and V. Taly, Chem. Soc. Rev., 2021, 50, 4141–4161 RSC.
- P. H. Ma, X. X. Jia, Y. Y. He, J. H. Tao, Q. Wang and C.-I. Wei, Trends Food Sci. Technol., 2024, 143, 104310 CrossRef CAS.
- S. Zeng, X. S. Liu, Y. S. Kafuti, H. Kim, J. Y. Wang, X. J. Peng, H. D. Li and J. Yoon, Chem. Soc. Rev., 2023, 52, 5607–5651 RSC.
- M. Hirano, R. Ando, S. Shimozono, M. Sugiyama, N. Takeda, H. Kurokawa, R. Deguchi, K. Endo, K. Haga, R. Takai-Todaka, S. Inaura, Y. Matsumura, H. Hama, Y. Okada, T. Fujiwara, T. Morimoto, K. Katayama and A. Miyawaki, Nat. Biotechnol., 2022, 40, 1132–1142 CrossRef CAS PubMed.
- B. C. Campbell, M. G. Paez-Segala, L. L. Looger, G. A. Petsko and C. F. Liu, Nat. Methods, 2022, 19, 1612–1621 CrossRef CAS PubMed.
- W. E. Yasso, Sedimentology, 1966, 6, 287–301 CrossRef.
- Y. Zhang, Q. S. Wang, T. Ma, D. W. Zhu, T. J. Liu and F. Lv, J. Controlled Release, 2020, 328, 127–140 CrossRef CAS PubMed.
- X. Gao, Y. Cui, R. M. Levenson, L. W. Chung and S. Nie, Nat. Biotechnol., 2004, 22, 969–976 CrossRef CAS PubMed.
- S. Shikha, X. Zheng and Y. Zhang, Nano-Micro Lett., 2018, 10, 31 CrossRef PubMed.
- A. M. Milosevic, L. Rodriguez-Lorenzo, S. Balog, C. A. Monnier, A. Petri-Fink and B. Rothen-Rutishauser, Angew. Chem., Int. Ed., 2017, 56, 13382–13386 CrossRef CAS PubMed.
- Y. J. Xie, Z. Z. Tong, T. L. Xia, J. C. Worch, J. Y. Rho, A. P. Dove and R. K. O'Reilly, Adv. Mater., 2023, 36, 2308154 CrossRef PubMed.
- Z. C. Liang, C. L. Hao, C. Chen, W. Ma, M. Z. Sun, L. G. Xu, C. L. Xu and H. Kuang, Adv. Mater., 2021, 34, 2107449 CrossRef PubMed.
- L. Rodriguez Lorenzo, K. Fytianos, F. Blank, C. von Garnier, B. Rothen Rutishauser and A. Petri Fink, Small, 2014, 10, 1341–1350 CrossRef CAS PubMed.
- T. Konry, R. B. Hayman and D. R. Walt, Anal. Chem., 2009, 81, 5777–5782 CrossRef CAS PubMed.
- J. Z. Song, Q. Yang, F. T. Lv, L. B. Liu and S. Wang, ACS Appl. Mater. Interfaces, 2012, 4, 2885–2890 CrossRef CAS PubMed.
- Y. K. Leng, W. J. Wu, L. Li, K. Lin, K. Sun, X. Y. Chen and W. W. Li, Adv. Funct. Mater., 2016, 26, 7581–7589 CrossRef CAS.
- L. Martín Banderas, A. Rodríguez Gil, Á. Cebolla, S. Chávez, T. Berdún Álvarez, J. M. Fernandez Garcia, M. Flores Mosquera and A. M. Gañán Calvo, Adv. Mater., 2006, 18, 559–564 CrossRef.
- M. Liong, J. Lu, M. Kovochich, T. Xia, S. G. Ruehm, A. E. Nel, F. Tamanoi and J. I. Zink, ACS Nano, 2008, 2, 889–896 CrossRef CAS PubMed.
- H. Y. Xia, Y. G. Ding, J. J. Gong, A. Lilienkampf, K. Xie and M. Bradley, ACS Appl. Mater. Interfaces, 2022, 14, 27107–27117 CrossRef CAS PubMed.
- J. Y. Zhang, S. Y. Qin, S. N. Zhang, C. Sun, Y. X. Ren, L. Y. Zhang, J. L. Liu, J. M. Xiao, W. Hu, H. Yang and D. K. Yang, Adv. Mater., 2023, 36, 2305872 CrossRef PubMed.
- H. W. Ji, H. H. Chen, T. Z. Mao, L. F. Zhao, K. Zhang, M. J. Yang, X. B. Zhou, Q. Wang, L. Wu and Y. L. Qin, Adv. Opt. Mater., 2023, 11, 2300991 CrossRef CAS.
- M. H. Lee, J. S. Kim and J. L. Sessler, Chem. Soc. Rev., 2015, 44, 4185–4191 RSC.
- S.-H. Park, N. Kwon, J.-H. Lee, J. Yoon and I. Shin, Chem. Soc. Rev., 2020, 49, 143–179 RSC.
- J. Q. Chang, Y. Zhang, Y. Li, Z. W. Han, F. Tian, C. Liu, Q. Feng, Y. G. Wang, J. S. Sun and L. Zhang, Small Methods, 2020, 5, 2001047 CrossRef PubMed.
- N. Makukhin, V. Tretyachenko, J. Moskovitz and J. Míšek, Angew. Chem., Int. Ed., 2016, 55, 12727–12730 CrossRef CAS PubMed.
- N. C. Seeman, J. Theor. Biol., 1982, 99, 237–247 CrossRef CAS PubMed.
- J. D. Watson and F. H. C. Crick, Nature, 1953, 171, 737–738 CrossRef CAS PubMed.
- Y. Hu and C. M. Niemeyer, Adv. Mater., 2019, 31, e1806294 CrossRef PubMed.
- C. M. Niemeyer, Curr. Opin. Chem. Biol., 2000, 4, 609–618 CrossRef CAS PubMed.
- R. Meyer, S. Giselbrecht, B. E. Rapp, M. Hirtz and C. M. Niemeyer, Curr. Opin. Chem. Biol., 2014, 18, 8–15 CrossRef CAS PubMed.
- H. Pei, R. Sha, X. Wang, M. Zheng, C. Fan, J. W. Canary and N. C. Seeman, J. Am. Chem. Soc., 2019, 141, 11923–11928 CrossRef CAS PubMed.
- D. Huang, K. Patel, S. Perez-Garrido, J. F. Marshall and M. Palma, ACS Nano, 2019, 13, 728–736 CrossRef CAS PubMed.
- Q. Hu, H. Li, L. Wang, H. Gu and C. Fan, Chem. Rev., 2019, 119, 6459–6506 CrossRef CAS PubMed.
- Y. Li, Y. T. Cu and D. Luo, Nat. Biotechnol., 2005, 23, 885–889 CrossRef CAS PubMed.
- S. H. Um, J. B. Lee, S. Y. Kwon, Y. Li and D. Luo, Nat. Protoc., 2006, 1, 995–1000 CrossRef CAS PubMed.
- J. B. Lee, Y. H. Roh, S. H. Um, H. Funabashi, W. Cheng, J. J. Cha, P. Kiatwuthinon, D. A. Muller and D. Luo, Nat. Nanotechnol., 2009, 4, 430–436 CrossRef CAS PubMed.
- P. W. K. Rothemund, Nature, 2006, 440, 297–302 CrossRef CAS PubMed.
- L. M. Smith, Nature, 2006, 440, 283–284 CrossRef CAS PubMed.
- A. V. Pinheiro, D. Han, W. M. Shih and H. Yan, Nat. Nanotechnol., 2011, 6, 763–772 CrossRef CAS PubMed.
- Y. Ke, L. L. Ong, W. M. Shih and P. Yin, Science, 2012, 338, 1177–1183 CrossRef CAS PubMed.
- B. Wei, M. Dai and P. Yin, Nature, 2012, 485, 623–626 CrossRef CAS PubMed.
- S. Li, Q. Jiang, S. Liu, Y. Zhang, Y. Tian, C. Song, J. Wang, Y. Zou, G. J. Anderson, J. Y. Han, Y. Chang, Y. Liu, C. Zhang, L. Chen, G. Zhou, G. Nie, H. Yan, B. Ding and Y. Zhao, Nat. Biotechnol., 2018, 36, 258–264 CrossRef CAS.
- J. B. Woehrstein, M. T. Strauss, L. L. Ong, B. Wei, D. Y. Zhang, R. Jungmann and P. Yin, Sci. Adv., 2017, 3, e1602128 CrossRef PubMed.
- S. Niekamp, N. Stuurman and R. D. Vale, Nat. Methods, 2020, 17, 437–441 CrossRef CAS PubMed.
- J. Li, J. Dai, S. Jiang, M. Xie, T. Zhai, L. Guo, S. Cao, S. Xing, Z. Qu, Y. Zhao, F. Wang, Y. Yang, L. Liu, X. Zuo, L. Wang, H. Yan and C. Fan, Nat. Commun., 2020, 11, 2185 CrossRef CAS PubMed.
- S. Han, J. S. Lee and J. B. Lee, Nanoscale, 2017, 9, 14094–14102 RSC.
- Y. Zhang, L. Chen, K. Hsieh and T. H. Wang, Anal. Chem., 2018, 90, 12180–12186 CrossRef CAS PubMed.
- C. Ramakers, J. M. Ruijter, R. H. Deprez and A. F. Moorman, Neurosci. Lett., 2003, 339, 62–66 CrossRef CAS PubMed.
- J. S. Park, T. Pisanic, Y. Zhang and T. H. Wang, Anal. Chem., 2021, 93, 2351–2358 CrossRef CAS PubMed.
- M. Y. Han, X. H. Gao, J. Z. Su and S. M. Nie, Nat. Biotechnol., 2001, 19, 631–635 CrossRef CAS PubMed.
- Y. Wang, C. Chen, J. He, Y. M. Cao, X. X. Fang, X. M. Chi, J. W. Yi, J. C. Wu, Q. S. Guo, H. Masoomi, C. Z. Wu, J. Ye, H. C. Gu and H. Xu, Small, 2021, 17, 2100315 CrossRef CAS PubMed.
- M. Xiao, K. Zou, L. Li, L. Wang, Y. Tian, C. Fan and H. Pei, Angew. Chem., Int. Ed., 2019, 58, 15448–15454 CrossRef CAS PubMed.
- B. Andreiuk, A. Reisch, M. Lindecker, G. Follain, N. Peyriéras, J. G. Goetz and A. S. Klymchenko, Small, 2017, 13, 1701582 CrossRef PubMed.
- B. J. Zheng, Y. Q. Qin, Y. Q. Xiang, A. Guo, Y. Y. Chen and Y. G. Hu, Anal. Chem., 2023, 95, 6542–6549 CrossRef CAS PubMed.
- M. G. Malle, P. M. G. Loffler, S. S. Bohr, M. B. Sletfjerding, N. A. Risgaard, S. B. Jensen, M. Zhang, P. Hedegard, S. Vogel and N. S. Hatzakis, Nat. Chem., 2022, 14, 558–565 CrossRef CAS PubMed.
- M. J. Dejneka, A. Streltsov, S. Pal, A. G. Frutos, C. L. Powell, K. Yost, P. K. Yuen, U. Müller and J. Lahiri, Proc. Natl. Acad. Sci. U. S. A., 2003, 100, 389–393 CrossRef CAS.
- Y. H. Zhang, L. X. Zhang, R. R. Deng, J. Tian, Y. Zong, D. Y. Jin and X. G. Liu, J. Am. Chem. Soc., 2014, 136, 4893–4896 CrossRef CAS PubMed.
- X. Zeng, S. K. Vanga, E. T. Poh, Y. Shi, C. H. Sow, A. A. Bettiol and X. G. Liu, Adv. Opt. Mater., 2020, 8, 2001168 CrossRef CAS.
- C. Lin, R. Jungmann, A. M. Leifer, C. Li, D. Levner, G. M. Church, W. M. Shih and P. Yin, Nat. Chem., 2012, 4, 832–839 CrossRef CAS PubMed.
- V. Pan, W. Wang, I. Heaven, T. Bai, Y. Cheng, C. Chen, Y. Ke and B. Wei, ACS Nano, 2021, 15, 15892–15901 CrossRef CAS PubMed.
- R. Jungmann, M. S. Avendano, J. B. Woehrstein, M. Dai, W. M. Shih and P. Yin, Nat. Methods, 2014, 11, 313–318 CrossRef CAS PubMed.
- D. A. Helmerich, M. Budiarta, D. Taban, S. Doose, G. Beliu and M. Sauer, Adv. Mater., 2023, 36, 2310104 CrossRef PubMed.
- F. Schueder, J. Lara-Gutierrez, B. J. Beliveau, S. K. Saka, H. M. Sasaki, J. B. Woehrstein, M. T. Strauss, H. Grabmayr, P. Yin and R. Jungmann, Nat. Commun., 2017, 8, 2090 CrossRef PubMed.
- R. Iinuma, Y. Ke, R. Jungmann, T. Schlichthaerle, J. B. Woehrstein and P. Yin, Science, 2014, 344, 65–69 CrossRef CAS PubMed.
- A. Banerjee, M. Anand, S. Kalita and M. Ganji, Nat. Nanotechnol., 2023, 18, 1474–1482 CrossRef CAS PubMed.
- S. C. M. Reinhardt, L. A. Masullo, I. Baudrexel, P. R. Steen, R. Kowalewski, A. S. Eklund, S. Strauss, E. M. Unterauer, T. Schlichthaerle, M. T. Strauss, C. Klein and R. Jungmann, Nature, 2023, 617, 711–716 CrossRef CAS PubMed.
- C. Vinegoni, P. F. Feruglio, I. Gryczynski, R. Mazitschek and R. Weissleder, Adv. Drug Delivery Rev., 2019, 151, 262–288 CrossRef PubMed.
- W. Y. Huang, Y. Cheng, J. Y. Zhai, Y. M. Qin, W. A. Zhang and X. J. Xie, Analyst, 2023, 148, 4406–4413 RSC.
- B. L. Ross, B. Tenner, M. L. Markwardt, A. Zviman, G. Shi, J. P. Kerr, N. E. Snell, J. J. McFarland, J. R. Mauban, C. W. Ward, M. A. Rizzo and J. Zhang, eLife, 2018, 7, e35458 CrossRef PubMed.
- X. Shaulli, R. Rivas-Barbosa, M. J. Bergman, C. Zhang, N. Gnan, F. Scheffold and E. Zaccarelli, ACS Nano, 2023, 17, 2067–2078 CrossRef CAS PubMed.
- W. Y. Wu, S. H. Luo, C. Y. Fan, T. J. Yang, S. W. Zhang, W. X. Meng, T. Xu, W. Ji and L. S. Gu, Light: Sci. Appl., 2023, 12, 9 CrossRef CAS PubMed.
- E. Perego, S. Zappone, F. Castagnetti, D. Mariani, E. Vitiello, J. Rupert, E. Zacco, G. G. Tartaglia, I. Bozzoni, E. Slenders and G. Vicidomini, Nat. Commun., 2023, 14, 8224 CrossRef CAS PubMed.
- S. Shah, A. K. Dubey and J. Reif, ACS Synth. Biol., 2019, 8, 1100–1111 CrossRef CAS PubMed.
- S. Shah, A. K. Dubey and J. Reif, Nano Lett., 2019, 19, 2668–2673 CrossRef CAS PubMed.
- F. Cole, J. Zähringer, J. Bohlen, T. Schröder, F. Steiner, M. Pfeiffer, P. Schüler, F. D. Stefani and P. Tinnefeld, Nat. Photonics, 2024, 1–7, DOI:10.1038/s41566-024-01384-4.
- A. Martin and P. Rivera-Fuentes, Nat. Chem., 2023, 16, 28–35 CrossRef PubMed.
- C. Chen, L. Ao, Y. T. Wu, V. Cifliku, M. Cardoso Dos Santos, E. Bourrier, M. Delbianco, D. Parker, J. M. Zwier, L. Huang and N. Hildebrandt, Angew. Chem., Int. Ed., 2018, 57, 13686–13690 CrossRef CAS PubMed.
- C. M. Green, J. Spangler, K. Susumu, D. A. Stenger, I. L. Medintz and S. A. Díaz, ACS Nano, 2022, 16, 20693–20704 CrossRef CAS PubMed.
- Y. X. Ma, G. B. Mao, W. R. Huang, G. Q. Wu, W. Yin, X. H. Ji, Z. S. Deng, Z. M. Cai, X.-E. Zhang, Z. He and Z. Q. Cui, J. Am. Chem. Soc., 2019, 141, 13454–13458 CrossRef CAS PubMed.
- L. Zhou, Y. Fan, R. Wang, X. Li, L. Fan and F. Zhang, Angew. Chem., Int. Ed., 2018, 57, 12824–12829 CrossRef CAS PubMed.
- Y. Xie, M. C. Arno, J. T. Husband, M. Torrent-Sucarrat and R. K. O'Reilly, Nat. Commun., 2020, 11, 2460 CrossRef CAS PubMed.
- X. S. Zhao, Y. Xu, Z. T. Chen, C. R. Tang and X. Q. Mi, Biosens. Bioelectron., 2024, 248, 115994 CrossRef CAS PubMed.
- R. J. Deng, K. X. Zhang, L. D. Wang, X. J. Ren, Y. P. Sun and J. H. Li, Chem, 2018, 4, 1373–1386 CAS.
- X. Cao, F. Chen, J. Xue, Y. Zhao, M. Bai and Y. Zhao, Nucleic Acids Res., 2023, 51, e13 CrossRef CAS PubMed.
- X. J. Qu, H. J. Jin, Y. Q. Liu and Q. J. Sun, Anal. Chem., 2018, 90, 3482–3489 CrossRef CAS PubMed.
- W. Wei, W. Dai, F. Yang, H. Lu, K. Zhang, Y. Xing, X. Meng, L. Zhou, Y. Zhang, Q. Yang, Y. Cheng and H. Dong, Angew. Chem., Int. Ed., 2022, 61, e202116909 CrossRef CAS PubMed.
- W. Wei, H. Lu, W. Dai, X. Zheng and H. Dong, ACS Nano, 2022, 16, 20329–20339 CrossRef CAS PubMed.
- Y. Wang, Y. Xiong, Y. Duan, K. Shi, C. Su, L. Ding, J. Wang and L. He, ACS Sens., 2023, 8, 1918–1928 CrossRef CAS PubMed.
- Y. Wen, W. Y. Liu, J. H. Wang, Y. L. Yu and S. Chen, Anal. Chem., 2023, 95, 12152–12160 CrossRef CAS PubMed.
- J. Lee, P. W. Bisso, R. L. Srinivas, J. J. Kim, A. J. Swiston and P. S. Doyle, Nat. Methods, 2014, 13, 524–529 CrossRef CAS PubMed.
- S. Jung, J. Kim, D. J. Lee, E. H. Oh, H. Lim, K. P. Kim, N. Choi, T. S. Kim and S. K. Kim, Sci. Rep., 2016, 6, 22975 CrossRef CAS PubMed.
- N. Juthani and P. S. Doyle, Analyst, 2020, 145, 5134–5140 RSC.
- W. Xu, P. Yin and M. Dai, Angew. Chem., Int. Ed., 2018, 57, 14075–14079 CrossRef CAS PubMed.
- Q. Huang, B. Chen, J. Shen, L. Liu, J. Li, J. Shi, Q. Li, X. Zuo, L. Wang, C. Fan and J. Li, J. Am. Chem. Soc., 2021, 143, 10735–10742 CrossRef CAS PubMed.
- O. K. Wade, J. B. Woehrstein, P. C. Nickels, S. Strauss, F. Stehr, J. Stein, F. Schueder, M. T. Strauss, M. Ganji, J. Schnitzbauer, H. Grabmayr, P. Yin, P. Schwille and R. Jungmann, Nano Lett., 2019, 19, 2641–2646 CrossRef CAS PubMed.
- J. Shi, M. Zhou, A. H. Gong, Q. J. Li, Q. Wu, G. J. Cheng, M. Y. Yang and Y. C. Sun, Anal. Chem., 2016, 88, 1979–1983 CrossRef CAS PubMed.
|
This journal is © The Royal Society of Chemistry 2024 |
Click here to see how this site uses Cookies. View our privacy policy here.