DOI:
10.1039/D3SD00286A
(Critical Review)
Sens. Diagn., 2024,
3, 933-945
Application of breathomics in pediatric asthma: a review
Received
26th October 2023
, Accepted 26th April 2024
First published on 9th May 2024
Abstract
Breathomics involves the use of non-invasive methods for diagnosing asthma by analyzing exhaled breath. While significant progress has been made in applying this approach to adult asthma, extending its application to pediatric asthma is crucial due to the increasing concern in this population. This review delineates five potential clinical applications: asthma diagnosis, differential diagnosis of asthma, assessment of asthma control levels, prediction of asthma exacerbation, and asthma phenotyping. Additionally, it highlights the moderate to reasonable predictive accuracy of exhaled breath volatile organic compounds (VOCs) breathomics in childhood asthma. However, it acknowledges that this field is still in its nascent stage of development, with particularly limited data available for Asian populations. Moreover, the identification of VOC biomarkers in pediatric asthma patients remains inconclusive, with varying reports. Therefore, large-scale data collection and standardization are imperative. Refinement and methodological improvements are necessary before integrating breathomics into clinical practice. This article provides clear directions for future research to optimize the clinical applicability of breathomics in evaluating asthma in children.
1. Introduction
Asthma has emerged as the predominant chronic respiratory ailment, exhibiting a rising prevalence over recent decades among both pediatric and adolescent populations. As of 2019, 262 million people suffered from asthma worldwide, with an overall prevalence of 9.1% in children and 11.0% in adolescents.1 The prevalence of asthma among Chinese children aged 0–14 years is increasing at a rate of 50% per decade, and more than 7.5% of children diagnosed with asthma exhibit severe symptoms.2 Asthma imposes a significant burden, quantified by disability-adjusted life years (DALYs), and reaches one of its peak magnitudes within the demographic of 10 to 14-year-old children, thereby emphasizing its profound influence on the quality of life experienced by this age group.1 The escalating prevalence and concomitant medical expenditures linked with pediatric asthma have culminated in its classification as a pronounced public health concern.3 Many patients exhibit lifelong symptoms and require treatment throughout their lives.4 Not surprisingly, this results in a significant economic burden to the families, and eventually for the nation5 (Fig. 1).
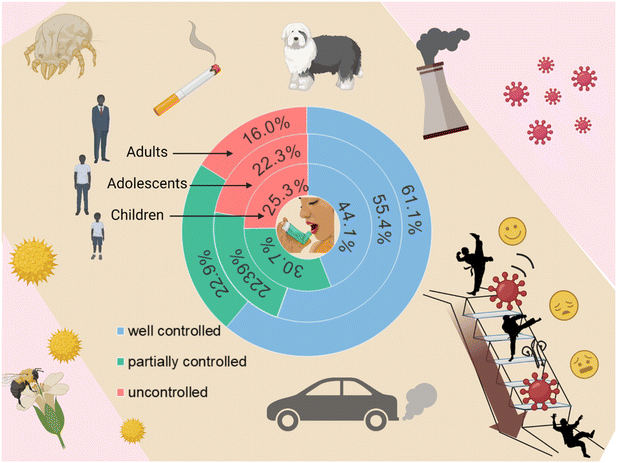 |
| Fig. 1 Global asthma control across age groups: children, adolescents, and adults. Asthma is susceptible to numerous triggers, including pet hair, dust mites, air pollution, and pollen particles. Regrettably, adherence to treatment guidelines leaves roughly half of individuals with asthma experiencing poorly controlled symptoms. Alarmingly, one in five children and adolescents grapple with uncontrolled symptoms, imposing unwarranted suffering on patients and their families, and placing an avoidable burden on healthcare systems. | |
The main pathophysiological features of asthma are chronic respiratory inflammation, respiratory hyper-responsiveness and recurrent reversible airflow obstruction.6 The underlying pathogenic mechanisms have not yet been fully elucidated. However, these mechanisms are known to be highly heterogeneous. The causal factors include genetic susceptibility, nutritional factors, and environmental factors, such as exposure to infections and tobacco smoke.7 The interplay between the host and the environment gives rise to distinct clinical phenotypes among the affected individuals.8 This includes differences in (i) the type of respiratory inflammation (eosinophilic, neutrophilic, multicellular), (ii) wheezing patterns (early, transient, persistent, late), (iii) degrees of the cough reflex and mucus hypersecretion,9 and (iv) different responses to treatment.10 For this highly heterogeneous disease, precision medicine would require personalized diagnosis and tailored treatment.11 For example, a clear comprehension of a patient's molecular phenotypes and endophenotype, quantification of asthma severity, and stratification of asthma subtypes will facilitate more effective diagnosis and evaluation. Additionally, it will aid in the development of targeted therapies tailored to specific asthma subtypes, thereby enhancing treatment guidance. Regrettably, the diagnosis of asthma continues to rely on clinical assessment, recollection of data, identification of airflow constriction, and assessment of bronchial reactivity through pulmonary function tests. Although respiratory inflammation is a key feature of the disease, it does not establish a direct correlation with clinical data or pulmonary function tests.9 Hence, these approaches cannot offer a means to assess a true response to treatment. In addition, asthma diagnosis in preschool children is particularly problematic because there are no tools to distinguish true asthma from asthma-like symptoms at a young age, let alone predict good or bad treatment outcomes.
To optimize the diagnosis and treatment of asthma, biomarkers that directly detect respiratory inflammation are needed to reflect the different pathological mechanisms of the inflammatory processes. These approaches can guide clinical treatment, determine prognosis, and successfully distinguish different phenotypes12 (Fig. 2). Established invasive “gold standard” techniques for assessing respiratory inflammation and remodeling encompass bronchoalveolar lavage (BAL) and bronchoscopic mucosal biopsy.13 However, non-invasive methods like induced sputum and exhaled breath temperature (EBT) are encumbered by limitations, including substantial cost, time-intensive procedures, deficiency in sensitivity and specificity, and an inability to effectively distinguish phenotypes.12,14 These challenges impede their widespread adoption as inflammation detection methods.15 Furthermore, the assessment of respiratory inflammation in preschool children with asthma is more difficult because it requires non-invasive, low-demand operations to obtain samples. Thus, the optimal inflammatory biomarker should possess attributes of easy accessibility, simplicity, rapidity, accuracy, and reproducibility. Moreover, the envisioned result should encompass heightened clinical applicability and cost-effectiveness spanning diagnostic, prognostic, monitoring, and treatment guidance spheres.
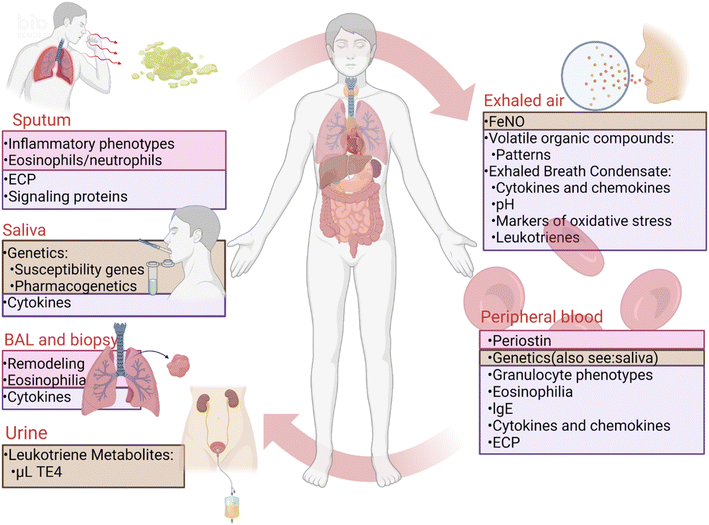 |
| Fig. 2 Asthma biomarkers for diagnosis, phenotyping, and efficacy. The provided image offers an overview of established asthma biomarkers in clinical practice as well as promising biomarkers currently under development. Clinically available biomarkers currently include bronchoalveolar lavage (BAL), endobronchial biopsy and fractional exhaled nitric oxide (FeNO), the first two of which have proven value in clinical practice, while FeNO is disputed. Several biomarkers under development are still at the research level. The pink areas represent proven clinical value, the purple areas represent controversial clinical value, and the brown areas represent the current level of research. ECP, eosinophil cationic protein; lgE, immunoglobulin E; uLTE4, urinary leukotriene E4. Figure adapted from ref. 14, Dove Medical Press. | |
Over the past decades, progress in molecular technology has spurred the emergence of “omics” research, which entails the high-throughput analysis of biological information across various levels.16 The amalgamation of histological data with clinical features and laboratory parameters facilitates a more profound exploration of disease mechanisms, permits the delineation of disease phenotypes, and facilitates the optimal selection of treatment modalities.16 Two typical examples are to explore the association of volatile organic compounds (VOCs) in exhaled breath or non-volatile compounds in exhaled breath condensate (EBC) with target pathophysiological processes for elucidating the heterogeneity of chronic respiratory diseases and monitoring treatment efficacy, which is known as breathomics. Breathomics is a specific branch of metabolomics that quantifies unstable, semi and non-volatile molecules in exhaled breath samples.17 Diagnosis of diseases by the amount of compounds in the exhaled breath is a reliable and non-invasive method. Especially for the assessment of asthma in preschool children, breathomics can provide a non-invasive, low-demand operation plan, which is of great significance for predicting the effectiveness of treatment. Such breathomics could be a key step towards disease stratification and personalized medicine.18
The composition of EBC reflects that of the respiratory lining fluid, facilitating noninvasive studies of inflammatory processes in respiration.19 EBC collection entails cooling exhaled air through contact with a cold surface or condenser, yielding a diluted fluid primarily composed of water. Consequently, the analysis of EBC necessitates highly sensitive methodologies to accurately assess solute species.19 Numerous biomarkers indicative of respiratory inflammation and oxidative stress have been identified and quantified in the EBC of pediatric asthma patients.20–23 Despite the promise of EBC biomarkers as a noninvasive means of assessing asthma, their clinical utility is impeded primarily by the absence of a standardized and rigorous protocol governing collection, preservation, and analysis. Therefore, this review offers a concise introduction to VOCs breathomics and its analytical tools. Meanwhile, the application of VOCs in pediatric asthma is discussed and concludes with an analysis of current trends and the challenges it encounters.
2. Fundamentals of breathomics
Exhaled human breath primarily comprises water vapor, nitrogen (N2), oxygen (O2), carbon dioxide (CO2), nitric oxide (NO), ammonium, and rare gases.24 Furthermore, it contains thousands of VOCs present in exceedingly low concentrations.24 VOCs, which are generated through physiological and pathological processes in humans, predominantly consist of hydrocarbons, such as ethanol, acetone, isoprene, and benzene.25 The origins of VOCs can be categorized into two groups: compounds acquired from the environment through inhalation (exogenous VOCs) and compounds synthesized by the host or internal microorganisms through biological metabolism (endogenous VOCs). The majority of respiratory investigations primarily concentrate on endogenous VOCs. For instance, acetone exhibits a strong association with ketoacidosis, while hydrocarbons are closely linked to the endogenous process of lipid peroxidation.26 Furthermore, cyclic compounds, including aromatic compounds, cyclic hydrocarbons, and furans, have demonstrated significant utility in differentiating lung cancer from other diseases in various studies. The quantification of exogenous VOCs present in the environment represents a potent diagnostic approach. For example, the assessment of the body's metabolism of limonene, commonly found in air fresheners and plants, can offer insights into liver function.27 Similarly, the detection of labeled urea in exhaled breath serves as an indicator of H. pylori infection.28 However, it is essential to note that exposure to exogenous chemicals such as benzene, commonly found in cigarettes, may lead to false-positive results.
Respiratory VOCs are derived from not only the upper and lower respiratory but compounds transferred from other parts of the body to the lungs through the circulatory system at capillary beds near the alveoli.29 Because of the close contact with the respiratory tract, specific differences in exhaled breath VOC hold potential significance in appraising pulmonary conditions like asthma. Furthermore, they play a pivotal role in advancing the creation of diagnostic instruments, a subject of global scholarly interest. To effectively diagnose and monitor complex and diverse ailments like asthma, it is essential to utilize a composite set of multiple VOCs rather than relying on a single VOC as the disease biomarkers. This amalgamation of VOCs can be conceptualized as a distinctive “molecular fingerprint” of breath.30 By extracting comprehensive information from composite multidimensional biological samples and employing pattern recognition algorithms and artificial intelligence, it becomes feasible to accurately capture nonlinear relationships both in health and disease states. This approach further enables the derivation of numerical probabilities indicating the presence or absence of specific clinical conditions.31 Ultimately each patient's respiratory characteristics can be identified to optimize treatment, which is the value of “breathomics”. Employing advanced analytical chemistry techniques such as gas chromatography and mass spectrometry, combining with cross-reactive sensor arrays like electronic noses (eNoses), enables highly sensitive detection of VOCs. These tools can be harnessed for precise clinical diagnosis.32 For pediatrics, breathomics is an attractive approach because it enables the safe, easy, and noninvasive collection and analysis of gaseous VOC molecules acquired in exhaled breath. Such noninvasive methods could be developed for determining the phenotype associated with asthma.33 Breathomics holds promising application potential due to its flexibility and user-friendly nature.
3. Available technologies in breathomics
Exhaled breath analysis can be broadly divided into two mainstream methodologies based on analytical techniques and sensor technologies.29
3.1 Chemical analysis methods
Mass spectrometry (MS), frequently integrated with separation methodologies such as gas chromatography (GC), is employed to discern biomarker compounds linked to distinct disease states and their accompanying pathophysiological mechanisms.34 Mass spectrometers utilize a mass analyzer to ascertain the mass, elemental composition, or chemical structure of an analyte. In a standard procedure, the analyte undergoes initial ionization. Subsequently, owing to variations in mass-to-charge ratio (m/z), the mass analyzer separates the charged particles within an electromagnetic field. These partitioned particles are then recorded by a detector and converted into a mass spectrogram. The mass spectral data is subsequently compared to a spectral library to extract detailed information about the analyte.30 The commonly used methods include gas chromatography-mass spectrometer (GC-MS), two-dimensional gas chromatography-mass spectrometry (GC × GC-MS, gas chromatography-flame ionization detection (GC-FID)) etc.35–37 GC-MS stands as the foremost approach for VOC analysis, offering elevated sensitivity and selectivity.38 Moreover, GC-MS excels in discerning the molecular identities of distinct components, rendering it well-suited for unearthing disease-specific biomarkers in scientific investigations. Nonetheless, it lacks the capability for real-time breath measurements.34 Furthermore, GC-MS does not provide access to real-time (online) data, necessitates proficient technicians and costly equipment, and imposes constraints on its utilization within clinical testing contexts.30
3.2 Gas sensor technology in breathomics
Cross-reactive sensor technologies employed for intricate mixture pattern recognition encompass diverse methods like gas sensors (electronic noses, eNose), colorimetric sensor arrays, and optoelectronic sensors. These techniques utilize specialized sensors founded upon optical, chemical, or electronic attributes to scrutinize VOCs within exhaled breath.39 Among them, gas sensors have been widely studied for their advantages such as portability, rapid detection, suitability for clinical practice, and affordability.40 In the standard procedure, participants were instructed to wear a nose-clip and breathe normally, while EBC samples were collected in a pre-cooled condenser system and subsequently transferred to a glass analysis tube. Following a 2-minute heating interval within a dry bath set to 37 °C to enhance gas phase concentration, the analysis tube was connected to the eNose via needle insertion, establishing direct contact with the gaseous EBC. A computer interface was employed to capture the electrical signal data generated by the eNose, facilitating comprehensive respiratory chart analysis.36 The testing procedure was conducted within a laminar flow hood to maintain the integrity and precision of the experimental environment (Fig. 3). Optimization of algorithms enables fast and intelligent pattern recognition of complex components of endogenous exhaled breath for probabilistic prediction related to health and disease.41 In recent times, numerous studies have showcased the clinical utility of these instruments in the context of respiratory diseases.42,43 Nevertheless, the advancement of breathomics remains within the realm of ongoing research, and establishing breathomics as a clinically pertinent approach necessitates a comprehensive sequence of actions, including the standardization of procedures, longitudinal studies, exploration of inflammatory sub-phenotypes, external validation, and field testing involving children with asthma.
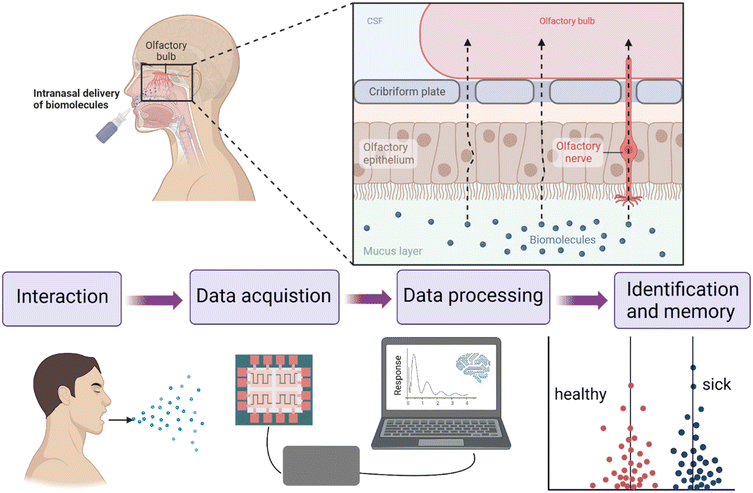 |
| Fig. 3 Schematic comparison of the working principle of the human nose and electronic nose (eNose). The eNose is designed to mimic the olfactory-sensitive human system. The detection of gas molecules in the human nose is accomplished through specialized receptors in the nasal cavity. These receptors interact with gas molecules, generating an electrochemical potential that is subsequently transmitted to the brain center via nerve axons. After learning and training, the human brain can recognize and memorize different odors. Figure adapted from ref. 44, Springer Nature. | |
4. Breathomics in pediatric asthma diagnosis
Utilizing variable sampling methods and comprehensive chemical analysis techniques, coupled with appropriate data processing and analysis, provides powerful and user-friendly tools for distinguishing between asthmatic and healthy children. Breathomics offers insights into metabolic activity, with VOCs serving as the molecular fingerprint of breathomics. This facilitates the correlation of clinical condition phenotypes and endotypes. An optimal breath test designed for diagnosing and monitoring pediatric asthma ought to possess attributes of compactness, rapidity, reliability, and clinical convenience. Potential clinical goals include asthma prediction, diagnosis, acute exacerbation prediction, phenotype prediction, and treatment response prediction. Only if breath testing meets one of these goals and contributes to the understanding of breathing problems will breathomics develop into a valuable diagnostic tool for the clinical management of pediatric asthma.
In the past decade, limited studies have reported VOCs in exhaled breath from children with wheezing or asthma.17 Overall, the collection of exhaled breath from children is deemed acceptable and feasible. The most commonly adopted sampling procedure involves instructing subjects to inhale, hold their breath for 5 seconds, and then exhale fully into a Tedlar bag (as recommended) until the bag is filled. Subsequently, the VOCs in the bag are transferred to a Tenax tube using a peristaltic pump. Molecular recognition or gas sensing techniques (as outlined in section 3) are then utilized to obtain omics data. Hence, breathomics is ideal for monitoring respiratory inflammation in asthma since it is non-invasive and can be repeated frequently, even in preschool children. Depending on the clinical objectives, studies have been categorized into various directions. Table 1 presents a summary of the research on breathomics in pediatric asthma.
Table 1 Summary of the research about breathomics in pediatric asthma
Research directions in breathomics |
Authors (year) |
Sample size |
Methodology |
VOCs |
Ref. |
GC-TOFMS, gas chromatography-time of flight mass spectrometry.
GC × GC-qMS, two-dimensional gas chromatography with quadrupole mass spectrometry.
GC × GC-TOFMS, two-dimensional gas chromatography-time of flight mass spectrometry.
GC-MS, gas chromatography-mass spectrometer.
|
Distinguish asthma in children |
J. W. Dallinga (2010) |
Children with asthma |
GC-TOFMSa |
(Branched) hydrocarbon (C13H28, C11H24) |
45
|
(n = 63) |
Unsaturated hydrocarbon (C15H26) |
Healthy controls |
Carbon disulphide (CS2) |
(n = 57) |
1-Penten-2-on |
Butanoic acid |
3-(1-Methylethyl)-benzene |
Benzoic acid |
p-Xylene |
M. Caldeira (2011) |
Children with asthma |
GC × GC-qMSb |
2-Methyl-1,3-butadiene |
46
|
(n = 35) |
2,4-Dimethyloctane |
Healthy controls |
Dodecane |
(n = 15) |
Tetradecane |
3-Ethyl-3-methylheptane |
3,9-Dimethylundecane |
pentadecane |
2,3,7-Trimethylundecane |
3,6-Dimethyl decane |
Hexadecane |
Undecane |
Tridecane |
2-Methyltridecane |
Decane |
2,3-Dimethyl decane |
2,3,6-Trimethyldodecane |
2-Methylpentadecane |
3,3-Dimethylheptane |
Acetone |
Nonanal |
Benzaldehyde |
M. Caldeira (2012) |
Children with asthma |
GC × GC-TOFMSc |
Decane |
47
|
(n = 32) |
Tetradecane |
Healthy controls |
Nonane |
(n = 27) |
Dodecane |
2,2,4,6,6-pentamethylheptane |
3,6-Dimethyldecane |
F. Gahleitner, C. Guallar-Hoyas (2013) |
Children with asthma |
GC-MSd |
1-(Methylsulfonyl)-propane |
48
|
(n = 11) |
1,4-Dichlorobenzene |
Healthy controls |
Dioctanol |
(n = 12) |
1-Isopropyl-3-methylbenzene |
Ethylbenzene |
3-Isopropyl-1-methylcyclohexene |
Octadecane |
1,7-Dimethylnaphthalene |
Discriminate children with asthma from early-life-transitory wheezing |
A. Smolinska (2014) |
Children with transient wheezers |
GC-TOFMS |
Acetone |
49
|
(n = 202) |
Octane |
Healthy controls |
Biphenyl |
(n = 50) |
1-Methyl-4-(1-methylethenyl) cyclohexene |
2-Vinyl-naphthalene |
2-Methylpentane |
2,4-Dimethylpentane |
2,3,6-Trimethyloctane |
2,6,10-Trimethyldodecane |
2,4-Dimethylheptane |
2-Undecenal |
2-Methylhexane |
2,2,4-Trimethylheptane |
E. M. Klaassen (2015) |
Children with asthma |
GC-TOFMS |
Acetone |
50
|
(n = 76) |
Octane |
Children with transient wheezers |
2-Methylhexane |
(n = 122) |
2,3,6-Trimethyloctane |
2,6,10-Trimethyldodecane |
2,4-Dimethylpentane |
2.4-Dimethylheptane |
2-Undecenal |
2-Methylpentane |
M. P. Van Der Schee (2015) |
Asymptomatic children |
Cyranose 320 |
Six main components |
42
|
(n = 97, of which rhinovirus positive n = 26, rhinovirus negative = 71) |
eNose |
Wheezy children |
(n = 81, of which rhinovirus positive n = 37, rhinovirus negative = 44) |
Discriminate uncontrolled children from well-controlled children with asthma |
S. J. Vijverberg (2013) |
Long-term uncontrolled asthma |
Cyranose 320 |
— |
43
|
(n = 33) |
eNose |
Long-term controlled asthma |
(n = 33) |
D. Van Vliet (2016) |
Children with asthma |
GC-TOFMS |
Sulphurdioxide dimethylsulfone |
51
|
(n = 96) |
Butanoic acid |
3-Methylfuran |
2-Methylfuran |
2,4-Hexadiene |
1,2-Dimethylcyclohexane |
Tetrachloroethylene |
m-Cymene (isopropyltoluene) |
Propylcyclohexane |
C10H16 unknown monoterpene |
Branched C14H30 |
M. A. Bannier (2022) |
Children with asthma |
GC-TOFMS |
Undecane |
52
|
(n = 89) |
Butanal |
Octanol |
Undecane |
Butanal |
Octanol |
Acetic acid (ester) |
Methylated pentane |
Two terpenes |
Seven alkanes |
Four branched chain alkanes |
Predict the development and worsening of asthma |
C. M. Robroeks (2013) |
Children with asthma |
GC-TOFMS |
p-Xylene |
53
|
(n = 40) |
3-Methylpentane |
2-Ethyl-4-methyl-1-pentanol |
1-Phenyl-1-butene |
4,6,9-Nonadecatriene |
D. Van Vliet (2017) |
Children with asthma |
GC-TOFMS |
1,2-Dimethylcyclohexane |
54
|
(n = 96) |
2-Ethylhexanal |
Octanal |
Nonanal |
6,10-Dimethyl-5,9-undecadien-2-one |
2-Methylfuran |
3-Methylfuran |
4.1 Distinguishing asthma in children
Differences between the VOCs of children with asthma and normal controls are highly sensitive and specific. Dallinga et al.45 reported a classification in distinguishing asthmatic and healthy children based on eight gas components measured by GC-MS with an accuracy of 92%. Caldeira et al.46,48 performed two studies that identified 88% and 96% of patients using 6 and 28 VOCs in GC-MS, respectively. This allows distinguishing children with asthma from healthy controls with high accuracy. Gahleitner et al.48 reported classification with 100% accuracy involving 11 children with asthmatic and 12 healthy control children. However, these studies with the same clinical objectives reported different VOCs. Table 1 presents recent examples of VOCs used to distinguish between asthmatic and non-asthmatic children. Only a few VOCs were repeated 1–2 times, such as dodecane and tetradecane.46,47 The poor reproducibility might stem from real disparities among populations and/or the constrained standardization of analytical chemistry methodologies across different laboratories. Furthermore, variance may be expected due to the differences in external validation, and different breath collection and analysis techniques.45–48
4.2 Separating asthmatic children from transitory wheezing
Transient wheezing and asthma share clinically similar symptoms, leading to overtreatment of children with transient wheezing and undertreatment of children with asthma. Smolinska et al.49 followed up 252 children between 2–6 years of age, who were at risk of asthma in a 6-year study “Asthma Detection and Monitoring” (ADEM). They studied the predictive value of VOCs in exhaled breath in the development stage of the disease and concluded that a set of 17 VOCs could distinguish children with preschool asthma from transient wheezers with a predictive accuracy of 80%. Respiratory inflammation is a potential pathological state of asthma. E. M. Klaassen et al. included the expression genes of respiratory inflammation and inflammatory markers in exhaled breath into the asthma prediction index (API), which improved the accuracy of asthma diagnosis in preschool children.50 Evaluation of model performance is quantified by the area under the receiver operating curve (AUC).55
In breathomics, there is a growing interest in breath analysis through the use of eNose. An eNose56 is an array of multiple gas sensors that interact with several VOCs simultaneously in a competitive manner, generating unique breathprints through changes in the resistance of the sensors to create an algorithm designed to discriminate between mixtures of VOCs. Such an eNose can provide inexpensive, in situ, and immediate discrimination of disease processes. Various types of eNose devices are employed for distinct pulmonary diseases and can be categorized broadly into four classes: electro-acoustic sensors, field-effect transistor (FET) sensors, electrochemical sensors, and chemiresistive sensors.57 Sometimes the choice of the eNose depends on the samples, for example, the BIONOTE,58 Cyranose 320,59 PEN3, or Tor Vergata eNose60 can collect and store samples on site for later analysis. In some occasions, easy portability is more important. Among eNoses, the SpiroNose stands alone as the sole device endowed with the capability to account for ambient air disruptions through the integration of external sensors.
M. P. Van Der Schee et al.42 assessed whether acute preschool wheezing was associated with a unique VOC characteristic in the Early Unbiased Risk Assessment of Pediatric Asthma (EUROPA). The breathomic characteristics in 178 wheezing and non-wheezing children were investigated by using an eNose. Compared with non-asthmatic children, the VOC profiles of rhinovirus-infected wheezing (AUC 0.77, 95% CI 0.07) and non-rhinovirus-infected wheezing (AUC 0.81, 95% CI 0.05) were different. Even after recovery, different breathomic characteristics can distinguish rhinovirus-infected wheezing. Given the linkage between rhinovirus-induced wheezing and an elevated risk of later school-age asthma diagnosis, these discoveries potentially indicate the feasibility of monitoring subtle asthma-related lung inflammation through respiratory analysis. This, in turn, enables the early detection of nascent asthma symptoms. Table 1 provides a summary of the findings in this area.
4.3 Identifying asthma control levels in children
Vijverberg et al.43 measured exhaled breath in children enrolled in the ongoing pharmacy-based “Pharmacogenetics of Asthma medication in Children: Medication with Anti-inflammatory effects” (PACMAN) study by eNose. Respiratory analysis was shown to successfully differentiate long-term controlled asthma from uncontrolled asthma (accuracy: 87.5%, AUC: 0.97, 95% CI: 0.89–1.00). The team also separated out currently controlled from uncontrolled asthma (accuracy: 75.8, AUC: 0.71, 95% CI: 0.52–0.90). A 1-year longitudinal observational study involving 96 asthma children was undertaken by Van Vliet et al.54 Within this study, assessments were conducted every 2 months to evaluate asthma control, lung function, fraction of exhaled nitric oxide (FeNO), VOCs, as well as cytokines and chemokines, a collection of 15 exhaled VOCs could discriminate between subgroups of children with persistently controlled and uncontrolled asthma during all clinical visits (with an area under the ROC curve of 86%). An 8-week crossover trial involving 89 children, conducted by M. A. Bannier et al.,52 aimed to explore the impact of Inhaled CVOC-sorticosteroids (ICS) on exhaled VOCs among preschool wheezing children. Notably, a collection of 20 VOCs exhibited the capacity to differentiate between ICS responders and non-responders both before and after treatment, achieving a sensitivity of 73% and a specificity of 67% (with an area under the ROC curve of 0.72). Among these top 5 discriminating VOCs, predominantly alkanes demonstrated significant discriminatory power. The aforementioned findings demonstrate the capacity of VOCs to discriminate between uncontrolled pediatric asthma and well-controlled pediatric asthma.
4.4 Tracking asthma development
Given the adverse impact of exacerbations on the quality of life for children with asthma, the potential clinical value lies in the ability of exhaled breath VOCs to forecast exacerbation occurrences in this group. Robroeks et al.53 observed 40 asthmatic children for one year, measured the distribution of FeNO and VOCs in exhaled breath, and conducted pulmonary function tests every 2 months. At the one-year mark, 16 children experienced exacerbations, aligning with the projections made by a model incorporating 6 VOCs, achieving 96% accurate classification, 100% sensitivity, and 93% specificity. Conversely, FeNO levels and lung function testing were unable to anticipate asthma exacerbations. By matching mass spectrometry maps to a spectral library, five compounds were identified among six groups. In a one-year prospective observational study of 96 children in a larger population, Van Vliet et al.54 demonstrated that seven VOCs in exhaled breath predicted asthma exacerbations within 14 days (sensitivity 88%, specificity 75%) and 21 days (sensitivity 63%) after sampling. Six compounds among seven VOCs were identified. Despite both studies identifying predictive VOCs for asthma exacerbation, the specific compounds differed, reflecting a recurrent challenge in pediatric asthma breathomics. When assimilating findings into a clinical context, comprehensive investigations necessitate large subject cohorts, environmental impact assessment on breath samples, implementation of reproducible and transparent data pre-processing workflows, and rigorous data analysis protocols.61 The identification of VOCs as potential disease biomarkers necessitates comprehensive data analysis for the development of predictive models using clinical data. However, many of the results reported in the existing literature have not been validated in an independent data pool to check the interpretability and generalizability of the models.62,63
4.5 Uncovering asthma phenotypes through breathomics
Asthma is highly heterogeneous with different pathogenesis, and different patients reflect various disease phenotypes. The imperative to achieve precision therapy is to identify the different phenotypes of asthma and discover diagnostic biomarkers to characterize the phenotypes. It would be valuable to obtain information about the inflammatory phenotypes of pediatric asthma through breath VOC analysis. Children with asthma could be offered targeted therapy, and their progress could be tracked through breathomics. This could improve the quality of life of the children while also reducing overall healthcare costs. Research conducted in adults has revealed associations between VOCs and differentials in inflammatory cell counts.64–66 Employing analysis of covariance (ANCOVA), the study identified acetonitrile and bicyclooctan-1-ol, 4-methyl as significantly linked to heightened sputum eosinophilia.64
However, there is very limited data on children, as obtaining induced sputum in children is more invasive than bronchoscopy.67 Sputum analysis is technically complex, and there is significant variability in routine clinical,15 so the research in this field is blank.
5. Clinical applications and challenges of breathomics in pediatric asthma
5.1 Strengths/limitations
Breathomics, as a branch of metabolomics, is being developed in various fields for early prediction and non-invasive monitoring of diseases.17 To date, studies of breathomics in pediatric asthma are limited, providing moderate to reasonable predictive accuracy in children, while identifying VOC biomarkers in pediatric asthma is inconclusive, with discrepancies in reporting and experimental shortcomings (e.g., separating low abundance compounds from noise) often resulting in irreproducible results.68 Determining whether non-replicated results signify spurious findings or reflect study-to-study heterogeneity defies assessment solely through the lens of clinical application as the ultimate objective. This challenge underscores the importance of considering a multitude of factors. A pivotal consideration is the establishment of specificity: biomarkers should exhibit specificity towards distinct asthma phenotypes, rather than depicting a generalized profile of a biological system in a perturbed physiological state. Numerous asthma-related metabolites, especially those linked to amino acid and choline metabolism, demonstrate connections with various chronic conditions, encompassing multiple malignancies.69 Take for instance, hydrocarbons like ethane and pentane, which result from the oxidative processes of cellular lipid constituents.70 In cases where lipid component metabolism derails, these hydrocarbons emerge, and their presence has been correlated with various respiratory obstructive disorders attributed to inflammation. Such conditions encompass asthma, chronic obstructive pulmonary disease (COPD), obstructive sleep apnea, and acute respiratory distress syndrome (ARDS).71 Although the above discussion does not negate their possible involvement in the pathogenesis of asthma, they have indeed been questioned as independent biomarkers of their utility.
The second key consideration is the standardization of sample sampling procedures and data pre-processing. It is worth noting that during the sampling process, environmental factors affect the sampling results to varying degrees. It is known that the Tedlar bag will release phenol and N,N-dimethylacetamide, and show changes in VOC levels over time.72 The water vapor in breath, on the one hand, will condense in the inner wall of the air bag to form a water film, and that in turn dissolves some of the VOCs affecting their detection rate. On the other hand, the uncondensed water vapor will occupy the adsorption site of the sampling tube, affecting the qualitative and quantitative results of VOCs.72 Human exhaled breath is divided into two parts, a part of dead space gas (not involved in gas exchange in the alveoli) and a part of alveolar gas from the lower respiratory, and it has been demonstrated that the contribution of dead space air to the overall total breath does not cause sensitivity problems for VOCs measured by gas chromatography time-of-flight mass spectrometry (GC-TOF-MS).73 Whether to incorporate this breath component hinges on the specific disease under investigation. To elucidate the origin of VOCs, it is advisable to undertake a comparative assessment of the two approaches. Data pre-processing commonly presents several challenges, including dealing with substantial volumes of raw data, notably in non-targeted analysis. Other hurdles involve mitigating background noise, addressing variances in VOC retention times and mass spectra across samples, and managing instances where VOCs overlap due to similar retention times.74 Consequently, ongoing refinement and methodological enhancements are prerequisite prerequisites before the integration of breathomics into clinical practice can be envisioned. A recent milestone in this domain was achieved with the release of a technical standard for exhaled biomarkers of lung disease by the ERS (European Respiratory Society) team.75 These standards include sampling methods, conditioning VOC samples, breathing pattern, subject/patient status, identification of VOC and their concentrations, pattern recognition of VOCs, calibration, processing, statistical analysis, internal validation and external validation, and so on.75 Future respiratory studies should follow these recommendations to ensure high quality respiratory studies.
5.2 Future implications
Indeed, omics technologies have earnestly strived to infiltrate clinical domains, offering vast potential benefits. Cost remains a pivotal consideration, spanning the realms of mechanistic study development, analytical technique refinement, and the employment of high-throughput methodologies for biomarker screening.76 Surprisingly, despite the substantial initial investments, omics, with a particular focus on breathomics, bear the potential to usher in more cost-effective healthcare solutions. In regions where access to skilled clinicians is constrained or in the context of early asthma prevention and management, portable devices like eNose play a pivotal role in alleviating the healthcare system's burden in extending care to a wider community. A prevalent research avenue involves the identification of asthma biomarker gases through chemical methodologies grounded in molecular recognition, often referred to as molecular fingerprinting. Subsequently, specialized gas sensors responsive to distinct VOCs are developed, and eNose devices are tailored with diagnostic algorithms designed specifically for asthma and its sub-phenotypes.77 In the realm of detection and diagnosis, pinpointing individual VOCs is not essential. What holds significance is the comprehensive VOC spectrum recorded by the eNose, which furnishes adequate accuracy founded upon probabilistic indications. Moreover, anticipations regarding treatment responses can be made through composite biomarkers, such as the respiratory fingerprint. Subsequently, eNose uploads singular measurements to the cloud, thereby constructing a personalized database that can be further augmented upon the confirmation of a diagnosis.
Significantly, there has been a notable upsurge in the count of pharmacogenomics, epigenomics, and metabolomics studies encompassing ethnically diverse minority groups like African Americans and Hispanics/Latinos, particularly in recent times as opposed to the past. Nevertheless, studies focused on Asian populations continue to be scarce.78 To realize the vision of precision medicine, the research community must intensify endeavors to foster international collaborations that emphasize racial and ethnic inclusivity. In fact, cross-ethnic studies have contributed to the advancement of histological technologies, expanding the breadth and accuracy of studies by comparing different ethnic populations to obtain targeted treatment options. Also, high-throughput technologies are expected to expand our understanding of the molecular mechanisms underpinning the pathophysiology of asthma and may help in the selection of targeted therapeutic strategies. Notably, rapid advancements in bioinformatics have unlocked the potential for simultaneous exploration across various histological layers.79,80 Numerous studies have unveiled correlations among diverse histological markers, including epigenomics, transcriptomics, breathomics, and asthma.30 A highly promising step forward would involve merging breathomics data with clinical inputs to create personalized risk prediction models. However, integrating multi-omics and clinical data requires large-scale databases, powerful computational capabilities, and close collaboration between clinicians and bioinformaticists with expertise in asthma.
Biobank serves as a specialized repository for biological samples, including the long-term storage of collected samples alongside clinical, epidemiological, and research data. In the realm of breathomics, a biobank provides comprehensive information about each breath sample, ensuring that sample data is meticulously processed, primarily analyzed, and fully preserved. This facilitates researchers in developing new scientific collaborations and enables access to the data and samples for sharing with the broader scientific community.81 Implementing a biobank in a research center could provide a solution to the standardization and validation problems described above. Biobanks can improve the reproducibility of research by developing and validating robust protocols to collect, store, distribute, and organize samples and data.82 Thus, biobanks shoulder the responsibility of managing data-intensive research and function as gatekeepers within the realm of integrated biological exploration.
6. Conclusion
The objective of the healthcare system is to diagnose diseases using cost-effective, non-invasive, straightforward, and dependable techniques. Breathomics, as a non-invasive diagnostic tool, can significantly assist physicians in early screening, improving diagnostic accuracy, and preventing diseases in children with asthma. This review provides an overview of the fundamentals of breathomics, the various techniques at disposal, methods for data collection and analysis, as well as the applications and challenges specific to children asthma. The emphasis placed on breathomics techniques in this review lays a crucial groundwork for future research in the realm of childhood asthma, both in terms of disease understanding and clinical applications.
Author contributions
There are no conflicts to declare.
Conflicts of interest
There are no conflicts to declare.
Acknowledgements
This work was supported by the National Natural Science Foundation of China (Grant No. 61971405), the Fundamental Research Funds for the Central Universities (Grant No. DUT22RC(3)050, DUT23YG135, DUT22QN252).
Notes and references
-
G. Asthma, The global asthma report, 2022, vol. 26 Search PubMed.
- C. K. Lai, R. Beasley, J. Crane, S. Foliaki, J. Shah, S. Weiland and I. P. T. S. Group, Thorax, 2009, 64, 476–483 CrossRef CAS PubMed.
-
D. T. Capriotti, N. Dawn and T. J. C. A. Ciocco, Update on Asthma Management: the 2022 GINA Report, Clinical Advisor, 2023, https://link.gale.com/apps/doc/A738448832/HRCA?u=anon~8a7af5ce&sid=googleScholar&xid=b487534e Search PubMed.
- A. Tai, H. Tran, M. Roberts, N. Clarke, A.-M. Gibson, S. Vidmar, J. Wilson and C. F. Robertson, J. Allergy Clin. Immunol., 2014, 133, 1572–1578.e1573 CrossRef PubMed.
- C. Nunes, A. M. Pereira and M. Morais-Almeida, Asthma Res. Pract., 2017, 3, 1–11 CrossRef PubMed.
- N. Mthembu, P. Ikwegbue, F. Brombacher and S. Hadebe, Front. Allergy, 2021, 2, 692841 CrossRef PubMed.
- H. T. Mocelin, G. B. Fischer and A. Bush, J. Pediatr., 2022, 98, 86–95 CrossRef PubMed.
- D. Hamilton and H. Lehman, Clin. Rev. Allergy Immunol., 2020, 59, 160–174 CrossRef CAS PubMed.
- I. D. Pavord, R. Beasley, A. Agusti, G. P. Anderson, E. Bel, G. Brusselle, P. Cullinan, A. Custovic, F. M. Ducharme and J. V. Fahy, Lancet, 2018, 391, 350–400 CrossRef PubMed.
- M. Iordanidou, S. Loukides and E. Paraskakis, Expert Rev. Clin. Pharmacol., 2017, 10, 293–303 CAS.
- A. Muraro, R. F. Lemanske Jr, P. W. Hellings, C. A. Akdis, T. Bieber, T. B. Casale, M. Jutel, P. Y. Ong, L. K. Poulsen and P. Schmid-Grendelmeier, J. Allergy Clin. Immunol., 2016, 137, 1347–1358 CrossRef PubMed.
- K. F. Chung, J. Intern. Med., 2016, 279, 192–204 CrossRef CAS PubMed.
- T. F. Leung, F. W. S. Ko and G. W. K. Wong, Ther. Adv. Respir. Dis., 2013, 7, 297–308 CrossRef CAS PubMed.
- S. J. Vijverberg, B. Hilvering, J. A. Raaijmakers, J.-W. J. Lammers, A.-H. Maitland-van der Zee and L. Koenderman, Biol.: Targets Ther., 2013, 7, 199–210 CAS.
- L. Tenero, M. Zaffanello, M. Piazza and G. Piacentini, Front. Pediatr., 2018, 6, 196 CrossRef PubMed.
- S. R. Tyler and S. Bunyavanich, J. Allergy Clin. Immunol., 2019, 144, 13–23 CrossRef PubMed.
- A. H. Neerincx, S. J. H. Vijverberg, L. D. J. Bos, P. Brinkman, M. P. van der Schee, R. de Vries, P. J. Sterk and A.-H. Maitland-van der Zee, Pediatr. Pulmonol., 2017, 52, 1616–1627 CrossRef PubMed.
- W. Ibrahim, L. Carr, R. Cordell, M. J. Wilde, D. Salman, P. S. Monks, P. Thomas, C. E. Brightling, S. Siddiqui and N. J. Greening, Thorax, 2021, 76, 514–521 CrossRef PubMed.
- E. Rahimpour, M. Khoubnasabjafari and V. Jouyban-Gharamaleki,
et al., Non-volatile compounds in exhaled breath condensate: review of methodological aspects, Anal. Bioanal. Chem., 2018, 410, 6411–6440 CrossRef CAS PubMed.
- S. Carraro, G. Giordano and F. Reniero,
et al., Asthma severity in childhood and metabolomic profiling of breath condensate, Allergy, 2013, 68, 110–117 CrossRef CAS PubMed.
- J. Cavaleiro Rufo, I. Paciência and F. C. Mendes,
et al., Exhaled breath condensate volatilome allows sensitive diagnosis of persistent asthma, Allergy, 2019, 74, 527–534 CrossRef CAS PubMed.
- V. A. Ferraro, S. Carraro and P. Pirillo,
et al., Breathomics in asthmatic children treated with inhaled corticosteroids, Metabolites, 2020, 10, 390 CrossRef CAS PubMed.
- J. Chang-Chien, H. Y. Huang and H. J. Tsai,
et al., Metabolomic differences of exhaled breath condensate among children with and without asthma, Pediatr. Allergy Immunol., 2021, 32, 264–272 CrossRef CAS PubMed.
- M. Phillips, J. Herrera, S. Krishnan, M. Zain, J. Greenberg and R. N. Cataneo, J. Chromatogr. B: Biomed. Sci. Appl., 1999, 729, 75–88 CrossRef CAS PubMed.
- N. Drabińska, C. Flynn, N. Ratcliffe, I. Belluomo, A. Myridakis, O. Gould, M. Fois, A. Smart, T. Devine and B. D. L. Costello, J. Breath Res., 2021, 15, 034001 CrossRef PubMed.
- E. Gaude, M. K. Nakhleh, S. Patassini, J. Boschmans, M. Allsworth, B. Boyle and M. P. van der Schee, J. Breath Res., 2019, 13, 032001 CrossRef CAS PubMed.
- M. E. O'Hara, R. Fernández del Río, A. Holt, P. Pemberton, T. Shah, T. Whitehouse and C. A. Mayhew, J. Breath Res., 2016, 10, 046010 CrossRef PubMed.
- F. Bazzoli, M. Zagari, S. Fossi, P. Pozzato, L. Ricciardiello, C. Mwangemi, A. Roda and E. Roda, Helicobacter, 1997, 2, 34–37 CrossRef PubMed.
- M. P. Van Der Schee, T. Paff, P. Brinkman, W. M. C. Van Aalderen, E. G. Haarman and P. J. Sterk, Chest, 2015, 147, 224–231 CrossRef PubMed.
- C. E. Wheelock, V. M. Goss, D. Balgoma, B. Nicholas, J. Brandsma, P. J. Skipp, S. Snowden, D. Burg, A. D'Amico and I. Horvath, Eur. Respir. J., 2013, 42, 802–825 CrossRef CAS PubMed.
- M. V. Farraia, J. C. Rufo, I. Paciência, F. Mendes, L. Delgado and A. Moreira, Porto Biomed. J., 2019, 4, e42 CrossRef PubMed.
- A. W. Boots, L. D. Bos, M. P. van der Schee, F.-J. van Schooten and P. J. Sterk, Trends Mol. Med., 2015, 21, 633–644 CrossRef CAS PubMed.
- V. de Rianne, W. F. D. Yennece, S. Pien, S. Erik, M. C. J. Patrick, B. Paul, D. Erica, K. B. Simon, E. Fred, H. C. D. J. Frans, G. H. Eric, C. C. M. I. T. V. Johannes, Z. Anke-Hilse Maitland-van der and J. S. Peter, Eur. Respir. J., 2018, 51, 1701817 CrossRef PubMed.
- O. Lawal, W. M. Ahmed, T. M. E. Nijsen, R. Goodacre and S. J. Fowler, Metabolomics, 2017, 13, 110 CrossRef PubMed.
- M. Caldeira, R. Perestrelo, A. S. Barros, M. J. Bilelo, A. Morête, J. S. Câmara and S. M. Rocha, J. Chromatogr. A, 2012, 1254, 87–97 CrossRef CAS PubMed.
- M. Phillips, R. N. Cataneo, A. Chaturvedi, P. D. Kaplan, M. Libardoni, M. Mundada, U. Patel and X. Zhang, PLoS One, 2013, 8, e75274 CrossRef CAS PubMed.
- C. M. Frank Kneepkens, G. Lepage and C. C. Roy, Free Radical Biol. Med., 1994, 17, 127–160 CrossRef PubMed.
- L. Moschino, S. Zanconato, S. Bozzetto, E. Baraldi and S. Carraro, Paediatr. Respir. Rev., 2015, 16, 205–212 Search PubMed.
- E. Van Mastrigt, J. De Jongste and M. Pijnenburg, Clin. Exp. Allergy, 2015, 45, 1170–1188 CrossRef CAS PubMed.
- N. Fens, M. Van der Schee, P. Brinkman and P. Sterk, Clin. Exp. Allergy, 2013, 43, 705–715 CrossRef CAS PubMed.
- M. V. Farraia, J. Cavaleiro Rufo, I. Paciência, F. Mendes, L. Delgado and A. Moreira, Porto Biomed. J., 2019, 4, e42 CrossRef PubMed.
- M. P. Van Der Schee, S. Hashimoto, A. C. Schuurman, J. S. R. van Driel, N. Adriaens, R. M. van Amelsfoort, T. Snoeren, M. Regenboog, A. B. Sprikkelman and E. G. Haarman, Eur. Respir. J., 2015, 45, 440–448 CrossRef PubMed.
- S. J. Vijverberg, L. Koenderman, F. C. van Erp, C. K. van der Ent, D. S. Postma, P. Brinkman, P. J. Sterk, J. A. Raaijmakers and A.-H. Maitland-van der Zee, BMC Pediatr., 2013, 13, 1–11 CrossRef PubMed.
- K. Hashimoto, Mol. Psychiatry, 2023, 28, 3625–3637 CrossRef PubMed.
- J. Dallinga, C. Robroeks, J. Van Berkel, E. Moonen, R. Godschalk, Q. Jöbsis, E. Dompeling, E. Wouters and F. Van Schooten, Clin. Exp. Allergy, 2010, 40, 68–76 CrossRef CAS PubMed.
- M. Caldeira, A. S. Barros, M. Bilelo, A. Parada, J. Câmara and S. M. Rocha, J. Chromatogr. A, 2011, 1218, 3771–3780 CrossRef CAS PubMed.
- M. Caldeira, R. Perestrelo, A. S. Barros, M. Bilelo, A. Morete, J. Câmara and S. M. Rocha, J. Chromatogr. A, 2012, 1254, 87–97 CrossRef CAS PubMed.
- F. Gahleitner, C. Guallar-Hoyas, C. S. Beardsmore, H. C. Pandya and C. P. Thomas, Bioanalysis, 2013, 5, 2239–2247 CrossRef CAS PubMed.
- A. Smolinska, E. M. Klaassen, J. W. Dallinga, K. D. Van De Kant, Q. Jobsis, E. J. Moonen, O. C. Van Schayck, E. Dompeling and F. J. Van Schooten, PLoS One, 2014, 9, e95668 CrossRef PubMed.
- E. M. Klaassen, K. D. Van De Kant, Q. Jöbsis, O. C. Van Schayck, A. Smolinska, J. W. Dallinga, F. J. van Schooten, G. J. Den Hartog, J. C. de Jongste and G. T. Rijkers, Am. J. Respir. Crit. Care Med., 2015, 191, 201–207 CrossRef PubMed.
- D. Van Vliet, A. Smolinska, Q. Jöbsis, P. Rosias, J. Muris, J. Dallinga, F. Van Schooten and E. Dompeling, J. Breath Res., 2016, 10, 016014 CrossRef CAS PubMed.
- M. A. Bannier, S. Kienhorst, Q. Jöbsis, K. D. van de Kant, F.-J. Van Schooten, A. Smolinska and E. Dompeling, J. Clin. Med., 2022, 11, 5160 CrossRef CAS PubMed.
- C. M. Robroeks, J. J. Van Berkel, Q. Jöbsis, F.-J. van Schooten, J. W. Dallinga, E. F. Wouters and E. Dompeling, Eur. Respir. J., 2013, 42, 98–106 CrossRef CAS PubMed.
- D. Van Vliet, A. Smolinska, Q. Jöbsis, P. Rosias, J. Muris, J. Dallinga, E. Dompeling and F.-J. Van Schooten, J. Breath Res., 2017, 11, 016016 CrossRef PubMed.
- G. M. M. Karel, K. Andre Pascal, W. Mark, R. Patrick, V. Yvonne, G. A. Douglas and E. G. Diederick, Heart, 2012, 98, 683 CrossRef PubMed.
- M. Van der Schee, N. Fens, P. Brinkman, L. Bos, M. Angelo, T. Nijsen, R. Raabe, H. Knobel, T. Vink and P. Sterk, J. Breath Res., 2012, 7, 016002 CrossRef PubMed.
- A. T. John, K. Murugappan, D. R. Nisbet and A. Tricoli, Sensors, 2021, 21, 2271 CrossRef CAS PubMed.
- R. Rocco, R. A. Incalzi, G. Pennazza, M. Santonico, C. Pedone, I. R. Bartoli, C. Vernile, G. Mangiameli, A. La Rocca and G. De Luca, Eur. J. Cardiothorac. Surg., 2016, 49, 1112–1117 CrossRef PubMed.
- S. Dragonieri, R. Schot, B. J. Mertens, S. Le Cessie, S. A. Gauw, A. Spanevello, O. Resta, N. P. Willard, T. J. Vink and K. F. Rabe, J. Allergy Clin. Immunol., 2007, 120, 856–862 CrossRef PubMed.
- I. Van der Sar, N. Wijbenga, G. Nakshbandi, J. Aerts, O. Manintveld, M. Wijsenbeek, M. Hellemons and C. Moor, Respir. Res., 2021, 22, 1–22 CrossRef PubMed.
- R. A. Sola-Martínez, G. Lozano-Terol, J. Gallego-Jara, E. Morales, E. Cantero-Cano, M. Sanchez-Solis, L. García-Marcos, P. Jiménez-Guerrero, J. A. Noguera-Velasco and M. Cánovas Díaz, Sci. Rep., 2021, 11, 13823 CrossRef PubMed.
- A. Amann, B. de Lacy Costello, W. Miekisch, J. Schubert, B. Buszewski, J. Pleil, N. Ratcliffe and T. Risby, J. Breath Res., 2014, 8, 034001 CrossRef CAS PubMed.
- R. S. Kelly, A. Dahlin, M. J. McGeachie, W. Qiu, J. Sordillo, E. S. Wan, A. C. Wu and J. Lasky-Su, Chest, 2017, 151, 262–277 CrossRef PubMed.
- P. Brinkman, M. A. van de Pol, M. G. Gerritsen, L. D. Bos, T. Dekker, B. S. Smids, A. Sinha, C. J. Majoor, M. M. Sneeboer and H. H. Knobel, Clin. Exp. Allergy, 2017, 47, 1159–1169 CrossRef CAS PubMed.
- F. N. Schleich, D. Zanella, P.-H. Stefanuto, K. Bessonov, A. Smolinska, J. W. Dallinga, M. Henket, V. Paulus, F. Guissard and S. Graff, Am. J. Respir. Crit. Care Med., 2019, 200, 444–453 CrossRef CAS PubMed.
- I. Baharudin, B. Maria, C. Paul, S. Dave, D. David, W. Ashley and J. F. Stephen, Thorax, 2011, 66, 804 CrossRef PubMed.
- L. R. Grant, L. L. Hammitt, D. R. Murdoch, K. L. O'Brien and J. A. Scott, Clin. Infect. Dis., 2012, 54, S140–S145 CrossRef PubMed.
- O. Ivanova, L. B. Richards, S. J. Vijverberg, A. H. Neerincx, A. Sinha, P. J. Sterk and A. H. Maitland-van der Zee, Allergy, 2019, 74, 2129–2145 CrossRef PubMed.
- I. A. Ratiu, T. Ligor, V. Bocos-Bintintan, C. A. Mayhew and B. Buszewski, J. Clin. Med., 2021, 10, 32 CrossRef PubMed.
- P. Paredi, S. A. Kharitonov, D. Leak, S. Ward, D. Cramer and P. J. Barnes, Am. J. Respir. Crit. Care Med., 2000, 162, 369–373 CrossRef CAS PubMed.
- T. L. Mathew, P. Pownraj, S. Abdulla and B. Pullithadathil, Diagnostics, 2015, 5, 27–60 CrossRef CAS PubMed.
- M. M. L. Steeghs, S. M. Cristescu, P. Munnik, P. Zanen and F. J. M. Harren, Physiol. Meas., 2007, 28, 503 CrossRef CAS PubMed.
- J. J. B. N. Van Berkel, J. W. Dallinga, G. M. Möller, R. W. L. Godschalk, E. Moonen, E. F. M. Wouters and F. J. Van Schooten, J. Chromatogr., B, 2008, 861, 101–107 CrossRef CAS PubMed.
- R. A. Sola Martínez, J. M. Pastor Hernández, G. Lozano Terol, J. Gallego-Jara, L. García-Marcos, M. Cánovas Díaz and T. de Diego Puente, Sci. Rep., 2020, 10, 22008 CrossRef PubMed.
- I. Horváth, P. J. Barnes, S. Loukides, P. J. Sterk, M. Högman, A.-C. Olin, A. Amann, B. Antus, E. Baraldi, A. Bikov, A. W. Boots, L. D. Bos, P. Brinkman, C. Bucca, G. E. Carpagnano, M. Corradi, S. Cristescu, J. C. D. Jongste, A.-T. Dinh-Xuan, E. Dompeling, N. Fens, S. Fowler, J. M. Hohlfeld, O. Holz, Q. Jöbsis, K. V. D. Kant, H. H. Knobel, K. Kostikas, L. Lehtimäki, J. O. Lundberg, P. Montuschi, A. V. Muylem, G. Pennazza, P. Reinhold, F. L. M. Ricciardolo, P. Rosias, M. Santonico, M. P. van d. Schee, F.-J. V. Schooten, A. Spanevello, T. Tonia and T. J. Vink, Eur. Respir. J., 2017, 49, 1600965 CrossRef PubMed.
- K. J. Karczewski and M. P. Snyder, Nat. Rev. Genet., 2018, 19, 299–310 CrossRef CAS PubMed.
- T. Saidi, O. Zaim, M. Moufid, N. El Bari, R. Ionescu and B. Bouchikhi, Sens. Actuators, B, 2018, 257, 178–188 CrossRef CAS.
- M. M. Papamichael, C. Katsardis, E. Sarandi, S. Georgaki, E.-S. Frima, A. Varvarigou and D. Tsoukalas, Metabolites, 2021, 11, 251 CrossRef CAS PubMed.
- S. T. Vernon, T. Hansen, K. A. Kott, J. Y. Yang, J. F. O'Sullivan and G. A. Figtree, Microcirculation, 2019, 26, e12488 CrossRef PubMed.
-
V. Chavda, R. Bezbaruah, D. Valu, S. Desai, N. Chauhan, S. Marwadi, G. Deka and Z. Ding, in Bioinformatics Tools for Pharmaceutical Drug Product Development, 2023, DOI:10.1002/9781119865728.ch7.
- R. Nadif, E. Bouzigon, N. Le Moual and V. Siroux, Open J. Bioresour., 2017, 4, 5 CrossRef.
- B. Zhao, L. Bryant, R. Cordell, M. Wilde, D. Salman, D. Ruszkiewicz, W. Ibrahim, A. Singapuri, T. Coats, E. Gaillard, C. Beardsmore, T. Suzuki, L. Ng, N. Greening, P. Thomas, P. Monks, C. Brightling, S. Siddiqui and R. C. Free, BMC Bioinf., 2020, 21, 556 CrossRef PubMed.
Footnote |
† These authors contributed equally to this work. |
|
This journal is © The Royal Society of Chemistry 2024 |