DOI:
10.1039/D4SC00839A
(Edge Article)
Chem. Sci., 2024,
15, 4547-4555
AI-assisted mass spectrometry imaging with in situ image segmentation for subcellular metabolomics analysis†
Received
5th February 2024
, Accepted 20th February 2024
First published on 26th February 2024
Abstract
Subcellular metabolomics analysis is crucial for understanding intracellular heterogeneity and accurate drug–cell interactions. Unfortunately, the ultra-small size and complex microenvironment inside the cell pose a great challenge to achieving this goal. To address this challenge, we propose an artificial intelligence-assisted subcellular mass spectrometry imaging (AI-SMSI) strategy with in situ image segmentation. Based on the nanometer-resolution MSI technique, the protonated guanine and threonine ions were respectively employed as the nucleus and cytoplasmic markers to complete image segmentation at the subcellular level, avoiding mutual interference of signals from various compartments in the cell. With advanced AI models, the metabolites within the different regions could be further integrated and profiled. Through this method, we decrypted the distinct action mechanism of isomeric drugs, doxorubicin (DOX) and epirubicin (EPI), only with a stereochemical inversion at C-4′. Within the cytoplasmic region, fifteen specific metabolites were discovered as biomarkers for distinguishing the drug action difference between DOX and EPI. Moreover, we identified that the downregulations of glutamate and aspartate in the malate–aspartate shuttle pathway may contribute to the higher paratoxicity of DOX. Our current AI-SMSI approach has promising applications for subcellular metabolomics analysis and thus opens new opportunities to further explore drug–cell specific interactions for the long-term pursuit of precision medicine.
Introduction
Since the end of the 20th century, high-throughput mass spectrometry technology combined with metabolomics analysis has brought significant discoveries to the life sciences.1,2 Its application in precision medicine has made it possible to specifically identify genetic variants or molecular alterations that contribute to the occurrence of disease.3–5 To explore the complex interactions between drugs and cells or organs, advanced mass spectrometry imaging (MSI) techniques were developed to achieve a variety of cutting-edge spatial metabolomics analysis, especially spatial single-cell metabolomics.6,7 These approaches generate massive and intricate datasets, which need to be further analyzed and interpreted. Artificial intelligence (AI) provides a powerful tool to extract and integrate overwhelming data, facilitating the understanding of the data correlations or distinctions.8,9 The combination of AI and MSI technology has greatly promoted spatial metabolomics analysis for abundant scientific research.2,10
Further application of the above methods in uncovering different drug–cell interactions caused by varying drug structures may provide essential insights for the development of more accurate and personalized therapy.11–13 However, such a task is very challenging due to the complexity of microenvironments and intracellular heterogeneity. In particular, drugs may elicit different effects in other cellular compartments than the one targeted by the drug.14,15 Traditional spatial single-cell metabolomics analysis tends to overlook these variations, if a single cell is the smallest unit of analysis. Further exploring drug-induced regional metabolic abnormalities at the subcellular scale may provide valuable insights into understanding the specific drug–cell interactions.16–18 This will definitely help to reveal the origin of drug side effects and elucidate the action mechanisms of different drugs with similar structures, especially isomeric drugs.
For instance, doxorubicin (DOX) and its isomer epirubicin (EPI) are representatives of anthracycline drugs used for clinical treatment of cancer.19–21 They differ only by the stereochemical inversion at C-4′, where the hydroxyl group is axial in DOX and equatorial in EPI (Fig. 1A-I). This minor structural difference does not obviously alter their therapeutic efficacy.22 However, DOX has higher paratoxicity compared to EPI, which can cause heart failure with eventual mortality of 20–40% at comparable doses.23 This disadvantage further restricts the clinical use of DOX. In recent years, many researchers have tried to clarify the drug targets and toxic side effects of DOX and EPI.21,24 One of the major reasons for the antitumor activity of DOX and EPI is their high affinity to chromosomal DNA.25 By embedding DNA molecules, they can interfere with DNA replication and transcription, thereby inhibiting the proliferation of tumor cells. Meanwhile, growing evidence suggested that mitochondria are also one important site for DOX and EPI targeting effects.16,26 The mitochondrial DNA damage can further trigger cardiotoxicity to induce drug side effects, especially for DOX.27,28 Although numerous drug targets and action mechanisms of DOX and EPI have been proposed,29,30 the reason behind the marked difference in toxicity between the two isomeric drugs is not well clarified so far. Spatial subcellular metabolomics, which enable the capture of metabolic changes in different regions of the cell, could provide a new strategy to solve this challenge. Unfortunately, the subcellular regions with micrometer size pose difficulty in accurately identifying endogenous molecules with extremely low abundance. Advanced technologies and methods need to be employed to achieve high spatial resolution and detection sensitivity.
Recently, our group has developed an MSI technique with an optimal ∼300 × 300 × 25 nm3 spatial resolution for in-depth anatomical profiling of single cells.31,32 It revealed the action mechanisms of anticancer drugs that affect the expression level of partial endogenous metabolites in the nucleus. Moreover, the metabolomics analysis of cell membranes was successfully performed without signal interference from the cytoplasm. The ability of subcellular imaging and in situ metabolomics analysis has been confirmed for this nanometer-resolution MSI technique. To achieve the subcellular metabolomic analysis, herein we developed an AI-assisted subcellular mass spectrometry imaging (AI-SMSI) method by integrating the above-mentioned MSI technique together with in situ image segmentation and AI-assisted data mining. The endogenous metabolite guanine and threonine ions in cells could directly serve as nucleus and cytoplasmic markers to complete image segmentation at the subcellular level. Thus, regional metabolomic analysis was performed to capture specific drug–cell interactions in the nucleus and cytoplasm without signal interference between different cellular compartments. Then massive metabolic differences associated with downstream cellular responses were clustered and in-depth analyzed through the AI model. With this strategy, we found fifteen characteristic metabolites in the cytoplasm that contribute to the difference in drug action between DOX and EPI. Importantly, the malate–aspartate shuttle pathway was identified as the metabolic pathway most responsible for the higher paratoxicity of DOX compared to EPI. Our AI-SMSI strategy here shows promising potential for studying drug-induced metabolic changes at the subcellular level and elucidating action mechanisms of isomeric drugs.
Results and discussion
Workflow and feasibility of the AI-SMSI method
The whole AI-SMSI strategy includes three important steps: subcellular-resolution MSI (Fig. 1A-I), in situ image segmentation (Fig. 1A-II), and AI-assisted data analysis (Fig. 1A-III). First, subcellular-resolution MSI is the foundation and provides abundant information about exogenous drugs and endogenous metabolites within single cells. Our previous work has confirmed the nanometer resolution of the vacuum ultraviolet laser desorption/ionization reflection time-of-flight mass spectrometry (VUVDI-RTOF-MS).31 The subcellular imaging ability has been proven to map the subcellular organelles. The experimental details of MSI are presented in the ESI (Fig. S1†). Herein, to capture the m/z signals within the cytoplasm and nucleus simultaneously, the VUV laser desorption/ionization source with ∼550 nm diameter (Fig. S1D†) and ∼170 nm depth (Fig. S1E†) was integrated into this MSI technique. Second, based on the subcellular resolution of MSI, the image segmentation was performed to distinguish the cytoplasm and nucleus by identifying different characteristic metabolites. Meanwhile, the mass spectra of pixel points co-located with these regional biomarkers will be extracted for further regional data analysis. Third, AI models were employed for in-depth analysis of the metabolites at different regions of cells, to unfold the biomarkers and metabolic pathways related to drug action of DOX and EPI.
Among these steps, the accuracy of image segmentation is essential in the AI-SMSI strategy, as any error in identifying compartment boundaries will directly affect the quantification of expression levels in the cells, thereby influencing downstream analysis. Currently, most image segmentation methods rely solely on stained images, which have a blurred outline and introduce probes that are not conducive to downstream metabolite analysis. Here, we developed a simple and specific image segmentation assay that takes full advantage of the subcell-specific distribution of certain cellular endogenous metabolites without the introduction of exogenous region probes. Remarkably, hundreds of mass peak signals will be produced after each MSI experiment. All of them come from endogenous metabolites or exogenous substances located in different regions of the cell. We can screen and map the m/z signals of these endogenous metabolite ions in the cell. Among them, the ions at m/z 152.1 (Fig. 1B-a) and m/z 120.2 (Fig. 1B-b) showed a clear distribution of different compartments within the nucleus and cytoplasm, respectively. Their tentative assignments were the protonated guanine ions ([C5H5N5O + H]+, fragment peak of DNA, Fig. S4†) and protonated threonine ions ([C4H9NO3 + H]+, Fig. S4†). Thus, they can be directly employed as regional markers for the nucleus and cytoplasm. To further validate the accuracy of the selected regional markers, the fluorescence image of 4′,6-diamide-2-phenylindole (DAPI) is also presented and it showed a similar distribution feature (Fig. 1B–d) to the mass image of the signal marker of nucleus ([C5H5N5O + H]+, m/z 152.1). Importantly, the mass images of these two regional markers showed a clearer separation of nucleus and cytoplasm in single cells (Fig. 1B-c), compared with the fluorescence (Fig. 1B-d) and bright field (Fig. 1B-e) images. Additionally, rather than the external labelling, the specific distributions of these endogenous metabolites enhanced the in situ and generality of image segmentation assay. This will further facilitate the precise extraction of metabolites in different compartments.
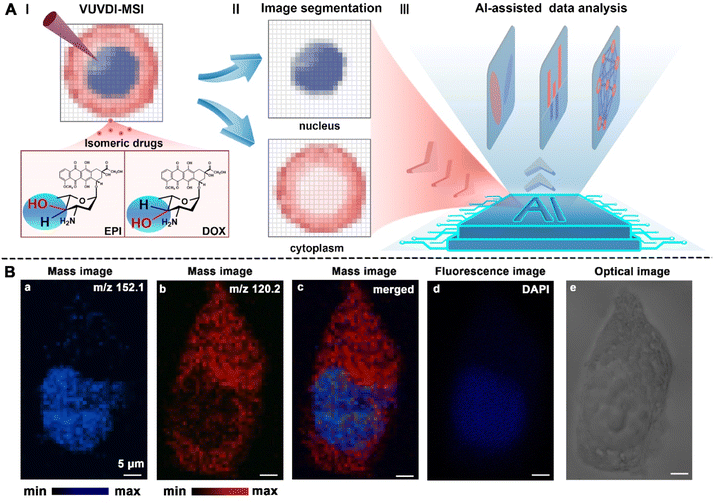 |
| Fig. 1 Schematic diagram of the AI-SMSI method and validation of the regional markers. (A) Workflow of the AI-SMSI method. (B) MS and fluorescence images of a single HeLa cell: (a) MS image of guanine ([C5H5N5O + H]+, m/z 152.1); (b) MS image of threonine ([C4H9NO3 + H]+, m/z 120.2); (c) merged image of (a) and (b); (d) fluorescence image of DAPI; (e) optical image of a single HeLa cell. | |
To evaluate the feasibility of the AI-SMSI approach, we took DOX- and EPI-cultured cells as a proof of concept, and compared the whole-cell mass spectrometry with subcellular regional mass spectrometry based on image segmentation of MSI.
For all experiments, the administration concentration and time of DOX and EPI were the same, 100 μM and 3 hours. Specifically, we first obtained the MSI of the whole cells through a pixel-by-pixel imaging scan (Fig. 2A). Unfortunately, the average mass spectra of whole cells were very similar for both DOX and EPI administration. The PCA scatter plot also failed to distinguish between the effects of the two drugs on whole cells.
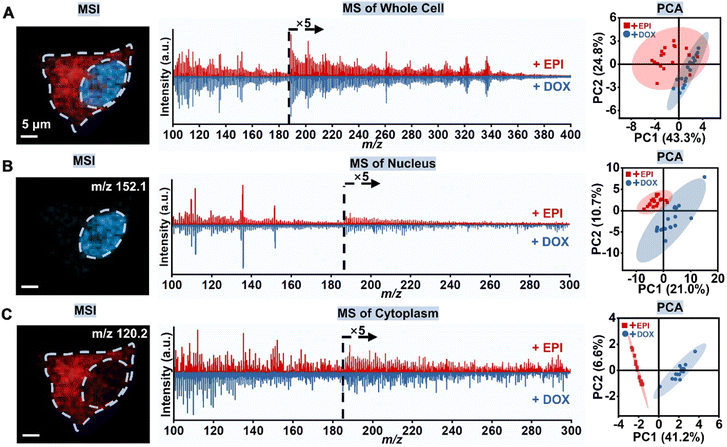 |
| Fig. 2 Evaluation of the AI-SMSI method for investigating the drug actions of the isomers DOX and EPI. MSI images, mass spectra and PCA scatter plots of the whole cell (A), nucleus (B) and cytoplasm (C). MSI images: blue, guanine ([C5H5N5O + H]+, m/z 152.1); red, threonine ([C4H9NO3 + H]+, m/z 120.2). Mass spectra and PCA scatter plots: EPI-treated cells (red), DOX-treated cells (blue). | |
Next, the nucleus and cytoplasmic region were selected by locating the pixel positions within the MS image of regional markers, protonated guanine and threonine ions. At the same time, the mass spectra within corresponding pixels were collected and the metabolite information was extracted for the next regional data analysis. As shown in Fig. 2B, the mass spectra of the nuclear region were very similar for both DOX and EPI administration. Compared to the nuclear region, the cytoplasmic regions of the two isomeric drugs exhibit greater variability in mass spectrometry (Fig. 2C). In addition, as can be seen from the score plots of PC1 and PC2, after DOX and EPI administration, datasets from the cytoplasmic region are classified as more independent clusters compared to datasets from the nuclear region. These results reveal significant metabolic differences in the cytoplasmic region. This is reasonable because both DOX and EPI have highly similar structures and comparable binding abilities with DNA, resulting in early consistency in the metabolic status within the nucleus. While DOX and EPI drugs primarily target the nucleus, subsequent cellular reactions and metabolite changes occur within the cytoplasm.33,34 Meanwhile, mitochondria in the cytoplasm are also one of the targets of the two drugs. Consequently, the distinctions between DOX and EPI administration in the cytoplasm gradually broaden, allowing for further differentiation. These results demonstrate the feasibility of the AI-SMSI method, which can avoid signal interference from different cellular compartments and is conducive to the capture of drug–cell interactions.
AI modeling and metabolic data mining
In light of the significant inter-group variation in the datasets from the cytoplasm, we then focused on analyzing the metabolic changes within this critical cell compartment. After image segmentation, a large number of metabolic molecules were obtained from each set of samples. In order to extract the most significant effective differences from the big metabolic data, we utilized an AI-assisted deep data mining method, instead of the traditional data analysis. We constructed an orthogonal partial least squares discriminant analysis (OPLS-DA) model for the dimensionality reduction and classification of high-throughput m/z signals (Fig. 3A). We first calibrated and normalized the raw mass spectrometry data, and then extracted the valid m/z peaks from the noise. The signal interference from small fragment ion peaks of drugs has been excluded (Fig. S2†). A total of 101 positive ion peaks were then put into the OPLS-DA model to further screen and evaluate differential compounds caused by the two isomeric drugs within the cytoplasm. Finally, we describe the classification and expression level of differential compounds through the OPLS-DA model, while also efficiently extracting the biomarkers of the DOX- and EPI-treated samples. The validation plot of the OPLS-DA model is shown in Fig. 3B. When the correlation coefficients between permuted (yperm in Fig. 3B) and original response variables (y in Fig. 3B) decreased, the regression lines of R2 and Q2 synchronously decreased, indicating that the OPLS-DA models did not overfit.35 Moreover, the intercept of the Q2 regression line with the Y-axis was less than 0, indicating a highly reliable model with no overfitting.36 Similar to the result of the PCA analysis, the OPLS-DA model successfully classified the DOX-treated and EPI-treated cells (Fig. 3C). The cumulative R2Y and Q2Y were very close to 1, at 0.954 and 0.907 respectively, implying the good fit and model validity.
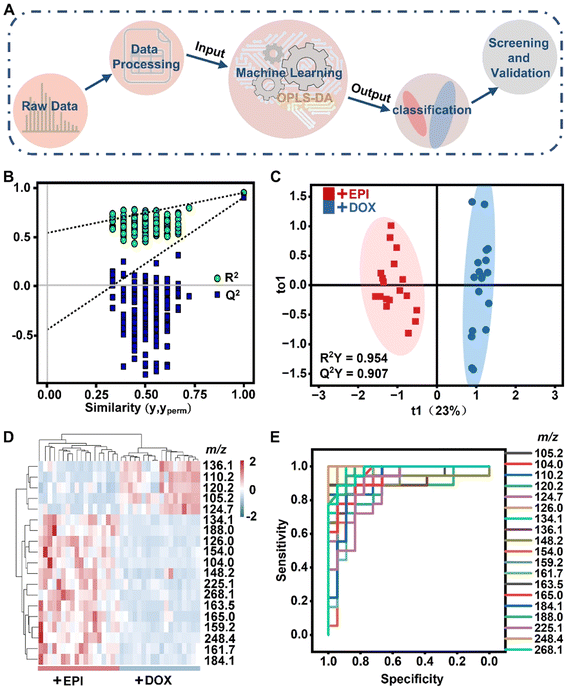 |
| Fig. 3 Identification of characteristic metabolites by artificial intelligence models. (A) Schematic diagram of the OPLS-DA model processing. (B) Displacement test plot of the OPLS-DA model. OPLS-DA scores map (C) and expression heat map (D) of metabolites derived from cytoplasm cultured with EPI (red) and DOX (blue). (E) Receiver operating characteristic (ROC) curves of the above putative differential signals in the cytoplasm. The area under the ROC curve (AUC) was the metric evaluated. AUC values for all differential signals are listed in Table S1.† | |
Additionally, the OPLS-DA model extracted 19 differential compounds with Variable Importance in Projection (VIP) ≥ 1 and p-value < 0.0001, as illustrated in Fig. 3D. The abnormal expressions of these 19 compounds (listed in Table S1†) may serve as biomarkers to identify the toxic effect of isomeric drugs. To verify the reliability of these putative differential compounds, a receptor operating characteristic (ROC) analysis was further performed (Fig. 3E). The results revealed that the area under the ROC curve (AUC) of the above-discovered compounds was in the range of 0.867–1.000 (Table S1†). All AUCs were >0.5, suggesting a high degree of confidence in the presumed differential compound. Through the above AI-assisted spatial subcellular data analysis, 19 m/z signals with significant expression differences were finally screened. All in all, the above AI model is reliable for accurate recognition of characteristic metabolites in the cytoplasm of the DOX- and EPI-treated cell groups.
Differential expressions of biomarkers for distinguishing the action of isomeric drugs
To further analyze the expression level of characteristic metabolites in the cytoplasm caused by the isomeric drugs, we drew the map of fold change (FC) values for related differential compounds screened by the OPLS-DA model (Fig. 4A). The FC values of the m/z signal that were up-regulated or down-regulated all ≥2 or ≤0.5 (i.e., log2(FC) ≥ 1 or ≤−1). Notably, the EPI drug induced more up-regulated features compared to DOX in the cytoplasmic region. According to the online databases Human Metabolome Database (HMDB) and Kyoto Encyclopedia of Genes and Genomes (KEGG), the tentative assignments of the above-extracted differential compounds have been listed in Table S1.† Finally, 15 out of 19 ions in the cytoplasm, as known endogenous biomarkers, show remarkable variances in subcellular metabolic profiles. We found that amino acids, carboxylic acids, purines, and organic bases constitute the main categories of confirmed metabolic markers. As shown in Fig. 4B and S3,† DOX reduced the abundance of 11 metabolites compared to the EPI groups, including choline, aspartate (m/z 134.1, Fig. S4†), glutamate (m/z 148.2, Fig. S4†), 5-methylcytosine, 3-hydroxyanthranilic acid (3-HAA), allantoin, 1-methylguanine, phosphorylcholine, 1-hydroxy-2-naphthoic acid (1H2N), sinapic acid (m/z 225.1, Fig. S4†) and adenosine. Additionally, DOX increased 4 metabolites in the cytoplasmic region, including hydroquinone, threonine (m/z 120.2, Fig. S4†), 3-hydroxybutanoic acid (3-HBA) and adenine.
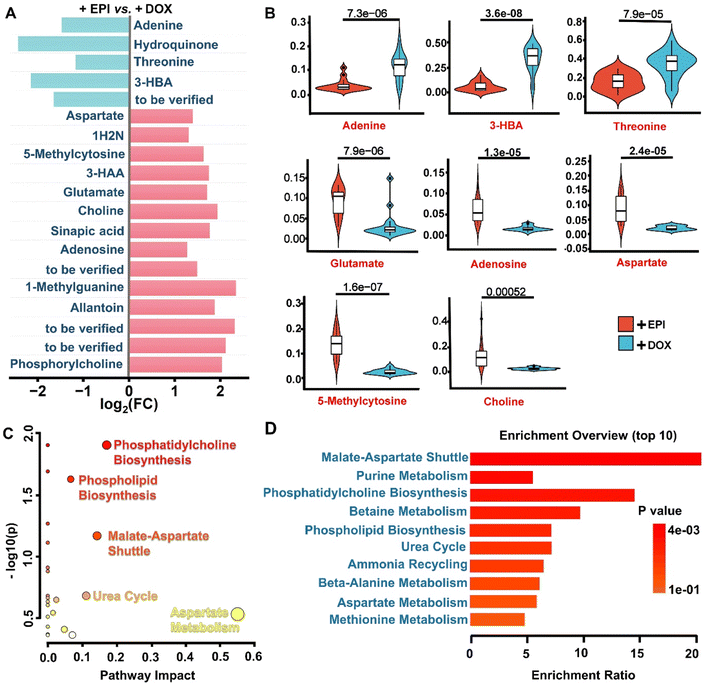 |
| Fig. 4 Differential expressions of metabolites and key metabolic pathways for distinguishing the actions of isomeric drugs. (A) Fold change (FC) values of the differential compounds screened by the OPLS-DA model. Red bar means that the FC value is greater than 2, and blue bar means that the FC value is less than 0.5. (B) Violin boxes of part metabolites from EPI-treated (red) and DOX-treated (blue) cells with the degree of differentiation. After DOX and EPI treatment, pathway analysis (C) and pathway enrichment analysis (D) of metabolites in the cytoplasm. | |
Previous work has reported some of the DOX-induced metabolic changes found by the current AI-SMSI method. For example, it has been reported that DOX can reduce the expression level of adenosine in anti-cancer therapy.37 Our findings supported this claim, showing lower adenosine expression levels within the cytoplasm induced by DOX. In addition, a high expression of 3-HBA has been shown to cause cardiac fibrosis.38 Our results indicated that DOX administration indeed upregulated the expression of 3-HBA in the cytoplasm by approximately 200% compared to the EPI group. Notably, glutamate plays an essential role in the cellular coupling of energy states within the cytosol and mitochondria, partially regulated by the malate–aspartate shuttle (MAS) pathway.39 Previous studies have reported that glutamate has many physiological functions, including cardioprotective effects.39,40 In our study, DOX-treated cells showed approximately 80% lower glutamate expressions in cytoplasm than EPI-treated groups, which may contribute to the higher toxic effects of DOX. Furthermore, DOX was shown to decrease nitric oxide levels in congestive heart failure, which is simultaneously manifested by a decrease in the expression of aspartate.41 We indeed observed a 50% reduction of aspartate levels in the cytoplasm with DOX treatment compared to EPI. Overall, the upregulation of 3-HBA and downregulation of glutamate and aspartate in response to DOX exposure align with previous studies and highlight their value as biomarkers of anticancer drug-induced organic toxicity. In addition to known markers, we discovered some new metabolic markers whose main categories are amino acids, carboxylic acids, purines, and lipid metabolites. The dysregulation of these metabolites may also serve as potential indicators for distinguishing the toxicity of the two isomeric drugs DOX and EPI. These findings suggested that the AI-SMSI effectively avoids the signal interference of different regions in the cell, and can distinguish more subtle metabolic differences. This will facilitate mechanism explanations associated with the different toxic side effect of DOX and EPI.
Action mechanism of DOX and EPI
We subsequently used MetaboAnalyst 5.0 to analyze the metabolic pathways of the above-screened biomarkers (VIP ≥ 1, FC ≥ 2 or ≤0.5 and p < 0.05). As shown in Fig. 4C and D, the main metabolic pathways affected by isomeric drugs include MAS, purine metabolism, phosphatidylcholine biosynthesis, betaine metabolism, phospholipid biosynthesis, urea cycle, etc. Eventually, the network of five metabolic pathways with significant contributions in the cytoplasm provides a comprehensive explanation of the possible molecular mechanisms underlying the differential drug actions of DOX and EPI (Fig. S5†). Myocardial ischemia profoundly affects phosphatidylcholine and phospholipid metabolism, which may lead to membrane damage.42 In the case of DOX administration, it is ultimately manifested by a decrease in choline and phosphorylcholine expression levels. In betaine metabolism, choline can be converted to adenosine, which protects the heart from hypoxia damage.43 An increase in purine metabolites is also observed in patients with cardiotoxicity.44 In purine metabolism, DOX-treated cytoplasm showed higher adenine expressions than EPI-treated cytoplasm.
In particular, the MAS pathway is a critical biological process in cells that connects mitochondrial and cytoplasmic metabolic pathways.45 Notably, these crucial metabolic pathways are functionally intertwined and interact with each other. For instance, choline not only serves as an ingredient for producing phosphorylcholine in determining the biosynthesis of phospholipids and phosphatidylcholine, but also can be converted into adenosine through betaine metabolism, thus affecting the purine metabolism process. As a result, the MAS pathway can be further disturbed. The dysregulation of these metabolic pathways is a direct feedback of different drug–cell interactions and toxic side effects. Among these pathways, the MAS pathway exhibited the most significant difference in metabolite participation, which is described in Fig. 5. Specifically, MAS can be involved in mitochondrial respiration by transporting electrons from cytosolic NADH into mitochondria NADH. A disturbance in substrate glutamate and aspartate levels can interfere with cellular energy metabolism by affecting the oxygen consumption rate (OCR) in cells, particularly for myocardial ischemia and liver disease.46,47 This may be the origin of side toxicity differences between DOX and EPI isomeric drugs. DOX treatment was found to downregulate the expression levels of glutamate and aspartate. This reduction led to a decrease in the rate of oxygen consumption, ultimately resulting in increased cellular toxicity.
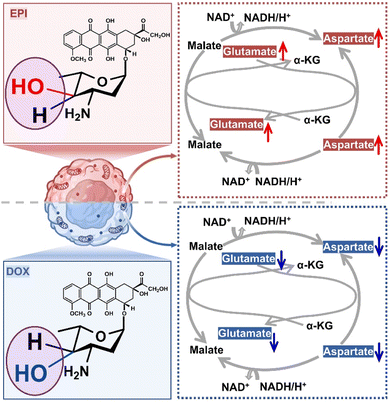 |
| Fig. 5 The most key pathway caused by metabolite participation in isomeric drug-cultured cells: malate–aspartate shuttle. The arrows pointing up and down represent the upregulation (red) and downregulation (blue) of metabolite expression levels. | |
Conclusions
We have proposed a new strategy called the artificial intelligence-assisted subcellular mass spectrometry imaging (AI-SMSI) approach to explore the underlying mechanisms of action of the isomeric drugs DOX and EPI. This approach is composed of three key parts, including subcellular-resolution MSI, in situ image segmentation and AI-assisted data analysis. Subcellular-resolution mass spectrometry imaging enables the detection of large amounts of endogenous metabolites within single cells. Based on the mass images of protonated guanine and threonine ions, in situ image segmentation was developed to perform mass spectrometry analysis on the nucleus and cytoplasm separately, thus avoiding mutual interference of signals. Furthermore, the AI model was utilized to implement cluster analysis and in-depth data mining of metabolites induced by drugs. Among them, the in situ image segmentation is the most important step in implementing this AI-SMSI strategy. It incorporates the advantages of subcellular resolution in the MSI technique and enhances the performance of AI data analysis, notably improving the identification of metabolites that differentiate between drug effects. This in turn facilitates the association of these metabolites with relevant metabolic pathways. Finally, we demonstrated the application of the AI-SMSI method for subcellular metabolomics analysis to decrypt the distinct action mechanism of the isomeric drugs DOX and EPI. We found fifteen specific metabolites as biomarkers for distinguishing the drug action difference between DOX and EPI. In particular, the malate–aspartate shuttle pathway was identified as the main cause of more significant side toxicity of DOX than EPI. This AI-SMSI method advances single-cell spatial metabolomics to subcellular spatial metabolomics, which provides tools for more precise analysis of intracellular drug effects between different cell compartments. Our results also provide valuable clues for elucidating the action mechanism of isomeric drugs and assist in the development of safer and more effective drugs.
Data availability
All data included in this study are available upon reasonable request by contact with the corresponding author.
Author contributions
Cong-Lin Zhao, Han-Zhang Mou and Lei Xing conducted all the experiments. Jian-Bin Pan and Lei Xing assembled the instrument. Cong-Lin Zhao and Lei Xing performed the data analysis. Cong-Lin Zhao, Lei Xing and Bin Kang wrote the initial manuscript. Yuxiang Mo helped design the instrument. Jing-Juan Xu conceived this study. Hong-Yuan Chen supervised the project. All authors reviewed the manuscript and provided valuable feedbacks.
Conflicts of interest
There are no conflicts to declare.
Acknowledgements
This work was supported by the National Key R&D Program of China (Grant No. 2021YFA0910003), National Natural Science Foundation of China (22034003, 22250009, 22261132510), the Excellent Research Program of Nanjing University (ZYJH004) and the China Postdoctoral Science Foundation (2019TQ0145).
Notes and references
- F. A. Rosenberger, M. Thielert, M. T. Strauss, L. Schweizer, C. Ammar, S. C. Mädler, A. Metousis, P. Skowronek, M. Wahle, K. Madden, J. Gote-Schniering, A. Semenova, H. B. Schiller, E. Rodriguez, T. M. Nordmann, A. Mund and M. Mann, Nat. Methods, 2023, 20, 1530–1536 CrossRef CAS PubMed.
- H. Shi, Y. He, Y. Zhou, J. Huang, K. Maher, B. Wang, Z. Tang, S. Luo, P. Tan, M. Wu, Z. Lin, J. Ren, Y. Thapa, X. Tang, K. Y. Chan, B. E. Deverman, H. Shen, A. Liu, J. Liu and X. Wang, Nature, 2023, 622, 552–561 CrossRef CAS PubMed.
- R. Chen, G. I. Mias, J. Li-Pook-Than, L. Jiang, H. Y. Lam, R. Chen, E. Miriami, K. J. Karczewski, M. Hariharan, F. E. Dewey, Y. Cheng, M. J. Clark, H. Im, L. Habegger, S. Balasubramanian, M. O'Huallachain, J. T. Dudley, S. Hillenmeyer, R. Haraksingh, D. Sharon, G. Euskirchen, P. Lacroute, K. Bettinger, A. P. Boyle, M. Kasowski, F. Grubert, S. Seki, M. Garcia, M. Whirl-Carrillo, M. Gallardo, M. A. Blasco, P. L. Greenberg, P. Snyder, T. E. Klein, R. B. Altman, A. J. Butte, E. A. Ashley, M. Gerstein, K. C. Nadeau, H. Tang and M. Snyder, Cell, 2012, 148, 1293–1307 CrossRef CAS PubMed.
- I. Barut, X. He, E. Sener, S. Sämfors, A. G. Ewing and J. S. Fletcher, Angew. Chem., Int. Ed., 2023, 62, e202217993 CrossRef CAS PubMed.
- M. Woolman, J. Qiu, C. M. Kuzan-Fischer, I. Ferry, D. Dara, L. Katz, F. Daud, M. Wu, M. Ventura, N. Bernards, H. Chan, I. Fricke, M. Zaidi, B. G. Wouters, J. T. Rutka, S. Das, J. Irish, R. Weersink, H. J. Ginsberg, D. A. Jaffray and A. Zarrine-Afsar, Chem. Sci., 2020, 11, 8723–8735 RSC.
- H. Zhang, D. G. Delafield and L. Li, Nat. Methods, 2023, 20, 327–330 CrossRef CAS PubMed.
- D. Unsihuay, H. Hu, J. M. Qiu, A. Latorre-Palomino, M. X. Yang, F. Yue, R. C. Yin, S. H. Kuang and J. Laskin, Chem. Sci., 2023, 14, 4070–4082 RSC.
- F. Wong, C. D. L. Fuente-Nunez and J. J. Collins, Science, 2023, 381, 164–170 CrossRef CAS PubMed.
- Y. C. Hu, Z. C. Wang, L. Liu, J. H. Zhu, D. X. Zhang, M. Y. Xu, Y. Y. Zhang, F. F. Xu and Y. Chen, Chem. Sci., 2021, 12, 7993–8009 RSC.
- Z. Y. Zhou, S. Mukherjee, S. J. Hou, W. J. Li, M. Elsner and R. A. Fischer, Angew. Chem., Int. Ed., 2021, 60, 20551–20557 CrossRef CAS PubMed.
- M. Duran-Frigola, M. Cigler and G. E. Winter, J. Am. Chem. Soc., 2023, 145, 2711–2732 CrossRef CAS PubMed.
- G. Palla, H. Spitzer, M. Klein, D. Fischer, A. C. Schaar, L. B. Kuemmerle, S. Rybakov, I. L. Ibarra, O. Holmberg, I. Virshup, M. Lotfollahi, S. Richter and F. J. Theis, Nat. Methods, 2022, 19, 171–178 CrossRef CAS PubMed.
- D. M. Camacho, K. M. Collins, R. K. Powers, J. C. Costello and J. J. Collins, Cell, 2018, 173, 1581–1592 CrossRef CAS PubMed.
- M. Luu and A. Visekruna, Signal Transduction Targeted Ther., 2022, 7, 360 CrossRef CAS PubMed.
- V. Ruiz-Rodado, A. Lita and M. Larion, Nat. Methods, 2022, 19, 1048–1063 CrossRef CAS PubMed.
- K. Jung and R. Reszka, Adv. Drug Delivery Rev., 2001, 49, 87–105 CrossRef CAS PubMed.
- H. Kitakata, J. Endo, H. Ikura, H. Moriyama, K. Shirakawa, Y. Katsumata and M. Sano, Int. J. Mol. Sci., 2022, 23, 1414 CrossRef CAS PubMed.
- K. C. Nitiss and J. L. Nitiss, Clin. Cancer Res., 2014, 20, 4737–4739 CrossRef CAS PubMed.
- D. Nielsen, P. Dombernowsky, T. Skovsgaard, J. Jensen, E. Andersen, S. A. Engelholm and M. Hansen, Ann. Oncol., 1990, 1, 275–280 CrossRef CAS PubMed.
- L. Simón-Gracia, V. Sidorenko, A. Uustare, I. Ogibalov, A. Tasa, O. Tshubrik and T. Teesalu, Angew. Chem., Int. Ed., 2021, 60, 17018–17027 CrossRef PubMed.
- A. G. Cheetham, R. W. Chakroun, W. Ma and H. G. Cui, Chem. Soc. Rev., 2017, 46, 6638–6663 RSC.
- E. I. El-Kimary and A. F. El-Yazbi, Spectrochim. Acta, Part A, 2016, 163, 145–153 CrossRef CAS PubMed.
- A. U. Buzdar, C. Marcus, T. L. Smith and G. R. Blumenschein, Cancer, 1985, 55, 2761–2765 CrossRef CAS PubMed.
- G. Minotti, P. Menna, E. Salvatorelli, G. Cairo and L. Gianni, Pharmacol. Rev., 2004, 56, 185–229 CrossRef CAS PubMed.
- G. L. Beretta and F. Zunino, Top. Curr. Chem., 2008, 283, 1–19 CAS.
- L. Wu, L. T. Wang, Y. X. Du, Y. M. Zhang and J. Ren, Trends Pharmacol. Sci., 2023, 44, 34–49 CrossRef CAS PubMed.
- X. S. Hou, H. S. Wang, B. P. Mugaka, G. J. Yang and Y. Ding, Biomater. Sci., 2018, 6, 2786–2797 RSC.
- X. J. Luo, X. Q. Gong, L. Y. Su, H. Y. Lin, Z. X. Yang, X. M. Yan and J. H. Gao, Angew. Chem., Int. Ed., 2020, 60, 1403–1410 CrossRef PubMed.
- D. Bernstein, Circ. Res., 2018, 122, 188–190 CrossRef CAS PubMed.
- D. Toroz and I. R. Gould, Sci. Rep., 2019, 9, 2155 CrossRef CAS PubMed.
- H. Z. Mou, J. B. Pan, C. L. Zhao, L. Xing, Y. X. Mo, B. Kang, H. Y. Chen and J. J. Xu, ACS Nano, 2023, 17, 10535–10545 CrossRef CAS PubMed.
- L. Xing, C.
L. Zhao, H. Z. Mou, J. B. Pan, B. Kang, H. Y. Chen and J. J. Xu, Chem. Biomed. Imaging, 2023, 1, 670–682 CrossRef CAS.
- H. T. Zhou, W. Yao, X. T. Zhou, S. Dong, R. N. Wang, Z. Y. Guo, W. H. Li, C. B. Qin, L. T. Xiao, S. T. Jia, Z. F. Wu and S. J. Li, ACS Nano, 2023, 17, 8433–8441 CrossRef CAS PubMed.
- P. K. Arnold, B. T. Jackson, K. I. Paras, J. S. Brunner, M. L. Hart, O. J. Newsom, S. P. Alibeckoff, J. Endress, E. Drill, L. B. Sullivan and L. W. S. Finley, Nature, 2022, 603, 477–481 CrossRef CAS PubMed.
- S. Zhao, H. Liu, Z. Su, C. Khoo and L. Gu, Mol. Nutr. Food Res., 2020, 64, e1901242 CrossRef PubMed.
- L. H. Nurani, A. Rohman, A. Windarsih, A. Guntarti, F. D. O. Riswanto, E. Lukitaningsih, N. A. Fadzillah and M. Rafi, Molecules, 2021, 26, 7626 CrossRef CAS PubMed.
- T. Porkka-Heiskanen and A. V. Kalinchuk, Sleep Med. Rev., 2011, 15, 123–135 CrossRef PubMed.
- S. Xu, H. Tao, W. Cao, L. Cao, Y. Lin, S. M. Zhao, W. Xu, J. Cao and J. Y. Zhao, Signal Transduction Targeted Ther., 2021, 6, 54 CrossRef CAS PubMed.
- T. T. Nielsen, N. B. Stottrup, B. Lofgren and H. E. Botker, Cardiovasc. Res., 2011, 91, 382–391 CrossRef CAS PubMed.
- D. Murashige, C. Jang, M. Neinast, J. J. Edwards, A. Cowan, M. C. Hyman, J. D. Rabinowitz, D. S. Frankel and Z. Arany, Science, 2020, 370, 364–368 CrossRef CAS PubMed.
- S. Fogli, P. Nieri and M. C. Breschi, FASEB J., 2004, 18, 664–675 CrossRef CAS PubMed.
- A. Lochner and M. Devilliers, J. Mol. Cell. Cardiol., 1989, 21, 151–163 CrossRef CAS PubMed.
- Y. Ye, Y. Cai, E. Xia, K. Shi, Z. Jin, H. Chen, F. Xia, Y. Xia, T. J. Papadimos, X. Xu, L. Liu and Q. Wang, Anesth. Analg., 2021, 133, 1048–1059 CrossRef CAS PubMed.
- A. Asnani, X. Shi, L. Farrell, R. Lall, I. A. Sebag, J. C. Plana, R. E. Gerszten and M. Scherrer-Crosbie, J. Cardiovasc. Transl. Res., 2019, 13, 349–356 CrossRef PubMed.
- K. F. Lanoue and J. R. Williamson, Metab., Clin. Exp., 1971, 20, 119 CrossRef CAS PubMed.
- T. Ackermann, H. R. Zuidhof, C. Muller, G. Kortman, M. G. S. Rutten, M. J. C. Broekhuis, M. A. Zaini, G. Hartleben and C. F. Calkhoven, Mol. Metab., 2023, 72, 101726 CrossRef CAS PubMed.
- L. Z. Agudelo, D. M. S. Ferreira, S. Dadvar, I. Cervenka, L. Ketscher, M. Izadi, L. Zhengye, R. Furrer, C. Handschin, T. Venckunas, M. Brazaitis, S. Kamandulis, J. T. Lanner and J. L. Ruas, Nat. Commun., 2019, 10, 2767 CrossRef PubMed.
Footnotes |
† Electronic supplementary information (ESI) available: Experimental sections, characterization of instrument performance, typical mass spectrum of DOX, metabolites with significant differences in the cytoplasmic region between DOX-cultured and EPI-cultured cells, mass spectra of several typical metabolites, the relational network of key metabolic pathways, and m/z signals and tentative assignments corresponding to the peaks in Fig. 2. See DOI: https://doi.org/10.1039/d4sc00839a |
‡ These authors contributed equally. |
|
This journal is © The Royal Society of Chemistry 2024 |