DOI:
10.1039/D3SC06920F
(Edge Article)
Chem. Sci., 2024,
15, 7219-7228
Polymer design via SHAP and Bayesian machine learning optimizes pDNA and CRISPR ribonucleoprotein delivery†
Received
23rd December 2023
, Accepted 25th March 2024
First published on 22nd April 2024
Abstract
We present the facile synthesis of a clickable polymer library with systematic variations in length, binary composition, pKa, and hydrophobicity (clog
P) to optimize intracellular pDNA and CRISPR-Cas9 ribonucleoprotein (RNP) performance. We couple physicochemical characterization and machine learning to interpret quantitative structure–property relationships within the combinatorial design space. For the first time, we reveal unexpected disparate design parameters for nucleic acid carriers; via explainable machine learning on 432 formulations, we discover that lower polymer pKa and higher percentages of benzimidazole ethanethiol enhance pDNA delivery, yet polymer length and captamine cation identity improve RNP delivery. Closed-loop Bayesian optimization of 552 formulation ratios further enhances in vitro performance. The top three polymers yield a higher signal and stable transgene expression over 20 days in vivo, and a 1.7-fold enhancement over controls. Our facile coupling of synthesis, characterization, and machine analysis provides powerful tools to quantitate performance parameters accelerating next-generation vehicles for nucleic acid medicines.
Introduction
Nucleic acids are important therapeutics, yet issues with delivery efficiency continue to hinder widespread advancement in the clinic. Delivery systems are crucial to encapsulate and protect these large and highly sensitive payloads and improve tissue internalization ensuring efficacy.1,2 Current viral delivery methods have struggled to overcome obstacles including limited cargo capacity,3 manufacturing costs,4 and immunogenicity.5,6 Nonviral delivery methods have been proven in commercial formulations and offer facile, tunable, and inexpensive vehicles for exogenous nucleic acid medicines. Polymers are established pharmaceutical formulation agents but have been under-utilized for carrying nucleic acids in vivo due to low performance.7 However, there exists limitless potential for polymer delivery vehicles due to ease of chemical and physical modulation along with affordable and scaled manufacturing.7,8 Controlled radical polymerization,9–15 post-polymerization modification,16–18 and parallel synthetic techniques19–21 have rapidly advanced and offer powerful tools to accelerate the next generation of bioactive polymer libraries.17,22–24 To this end, the field of machine learning25–27 coupled with parallel experimentation is aiding analysis and understanding of data sets in identifying the chemical, physical, and biological factors involved in the performance enhancement of polymers.23,24,28–33 However, we are limited by the vast chemical space and prediction of formulation chemistries indicating discrete selection and optimization of next-generation systems. Indeed, new machine learning models aimed at selecting and predicting discrete parameters influencing biological efficacy are needed to advance the next frontier of personalized medicine.
To accelerate the advancement of next-generation nucleic acid medicines, we present a facile method to generate cationic polymers coupled with a machine learning workflow as a powerful tool to tailor nucleic acid delivery vehicles (Fig. 1). Herein, we probe the effect of physicochemical properties on in vitro delivery of plasmid DNA (pDNA) and CRISPR-Cas9 ribonucleoprotein (RNP) payloads through SHapley Additive exPlanations (SHAP) analysis. We also utilize machine learning through Bayesian Optimization (BO) to identify formulation prediction for optimizing in vitro delivery. We present three scaffold lengths to produce 36 copolymers containing systematic binary compositions of a hydrophobic cation benzimidazole ethanethiol (BET), along with the co-cations with cysteamine (Cys), captamine (Cap), or 2-(diethylamino) ethanethiol (DiE), to examine their effect on performance. Our model allows rapid comparison of 552 formulations across numerous chemical and physical characteristics of the polymer scaffolds: repeat units (RU), incorporation of BET, type of co-cation, polymer pKa, polymer clog
P, polyplex size, formulation ratio, and relative binding, to identify overarching structure–property relationships benefitting cellular delivery (transfection) efficiency and cellular viability. We show that the chemical parameters that dictate delivery performance differ as a function of payload identity. Our predictive pipeline identifies three top performers in vivo, displaying higher and longer-term transgene expression in the mouse liver compared to a well-studied commercial control (JetPEI). Our powerful methods presented herein provide advanced understanding of quantitative structure–activity relationships important for rapid preclinical development of next-generation nucleic acid medicines.
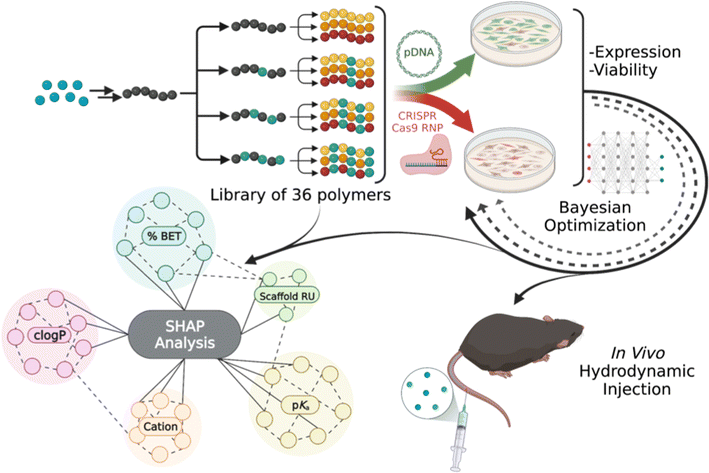 |
| Fig. 1 A tunable polymer scaffold for facile optimization of pDNA and RNP delivery performance. The polymers are modified with chemical functionality to modulate binding, delivery, and release. SHAP analysis, a machine learning technique, aids in understanding the structure–activity relationships and identifying the polymer feature importance in expression and viability. A closed-loop Bayesian optimization further improves performance. Polymers of interest were evaluated for pDNA delivery to the mouse liver. | |
Results and discussion
Polymer scaffold synthesis
Pentafluorophenyl methacrylate (PFPMA) is known to polymerize under the reversible addition–fragmentation chain transfer (RAFT) mechanism to control the degree of polymerization and dispersity of the backbone.16,34 PFPMA allows efficient post-polymerization modification due to the labile nature of the pentafluoro group via amidation.16,17,34–36 We performed synthesis to create three well-defined pPFPMA scaffold lengths yielding short (Sh), medium (Md) and long (Lg) (N = 90, 190, 250) variants. Through amidation with allylamine, we obtain poly(allylmethacrylamide) (pAMAm), a polymer backbone decorated with pendent alkenes and responsive to the highly efficient thiol–ene click chemistry.37 We create the library of copolymers in a stepwise conjugation of functional groups. First, four compositions of BET, ranging in incorporation from 0–45%, are obtained, and then each sample is split into three and further saturated with either Cys, Cap, or DiE cations. Our modular process allows for consistent BET incorporation (high log
P, possibly intercalating) and co-cation (promotes electrostatic binding) composition to be consistent across each sample ensuring structural uniformity. Full characterization of the polymer systems through 1H NMR, 19F NMR, ATR-FTIR, and SEC-MALS is shown in Fig. S1–S4 and S7–S12.† Polymers are named according to polymer RU_Cation_%BET (i.e., Sh_DiE_40).
Altering the protonation state and hydrophobicity of the polymer systems has been shown to affect the ability of polymers to bind and release nucleic acids, interact with cell membranes, promote endosomal escape, and enable beneficial aggregation in vitro promoting particle settling onto cells.24,38–44 Using an autotitrator, we measured the pKa values (Fig. S13†) of the small molecule thiols. The values for Cys, Cap, DiE, and BET, respectively, were 8.10, 7.74, 7.68, and 5.90 (Fig. 2). It should be noted that upon polymerization, neighboring group effects suppress amine ionization resulting in a common decrease of one pKa unit comparing monomer to polymer, which is found after measuring the pKa of all polymers.45,46 We also calculated the octanol to water partition coefficient (clog
P) values of the monomers to be −2.70, −2.50, 1.74, and 2.40, respectively, for Cys, Cap, DiE, and BET (the more negative the number, the higher the water solubility). The molar averages of pKa and clog
P of the copolymer systems based on the repeat units and percent incorporation of the functional moieties are shown in Fig. 2 and eqn (S2) and (S3).†
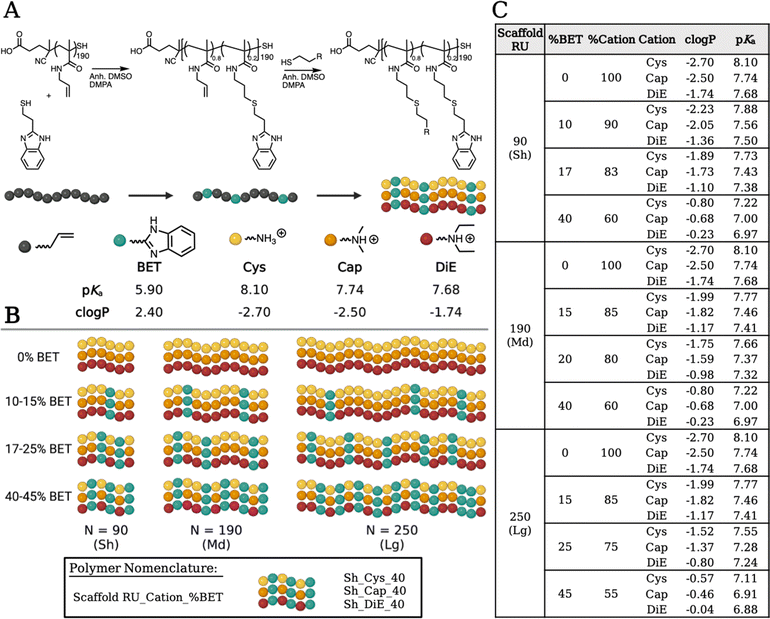 |
| Fig. 2 (A) Schematic of the poly(allylmethacrylamide) (pAMAm) polymer scaffold undergoing a stepwise thiol–ene post-polymerization modification of the medium backbone with 20% BET and then further split into three to be saturated with the remaining cations (Cys, Cap, DiE). The functional amines have a range of charge state (pKa) and hydrophobicity (clog P) characteristics. (B) A visual representation of the 36 polymers in the library showing the repeat units in the parent backbone (N = 90, 190, 250) and the range in BET incorporation within each polymer set. (C) Table of data displaying the chemical characterization of all 36 polymers. | |
Polyplex physical characterization
For physical characterization and analysis of the polymer–payload complexes (polyplexes), we used dynamic light scattering (DLS) to determine the hydrodynamic radius (Rh) and a dye exclusion assay to establish the relative binding affinity of each polymer with the pDNA or RNP (Fig. 3B). We mixed polymers with either payload in phosphate buffered saline (PBS, pH 7.4) at a given nitrogen (N, on polymers) to phosphate (P, on nucleotides) (N/P) ratio (from either pDNA or guide RNA (gRNA)). We observed that pDNA polyplexes formed with the Md and Lg backbones were around 50 nm in size, while the Sh backbone polyplexes ranged in size from 50 to 250 nm (Fig. S14–S16†). When the polymers were bound with RNP, the polyplex sizes were considerably larger with all of the polyplex systems being larger than 100 nm, ranging to 1000 nm (Fig. S17–S19†). We also found that the RNP aggregates alone, which could be the cause for these larger aggregates.
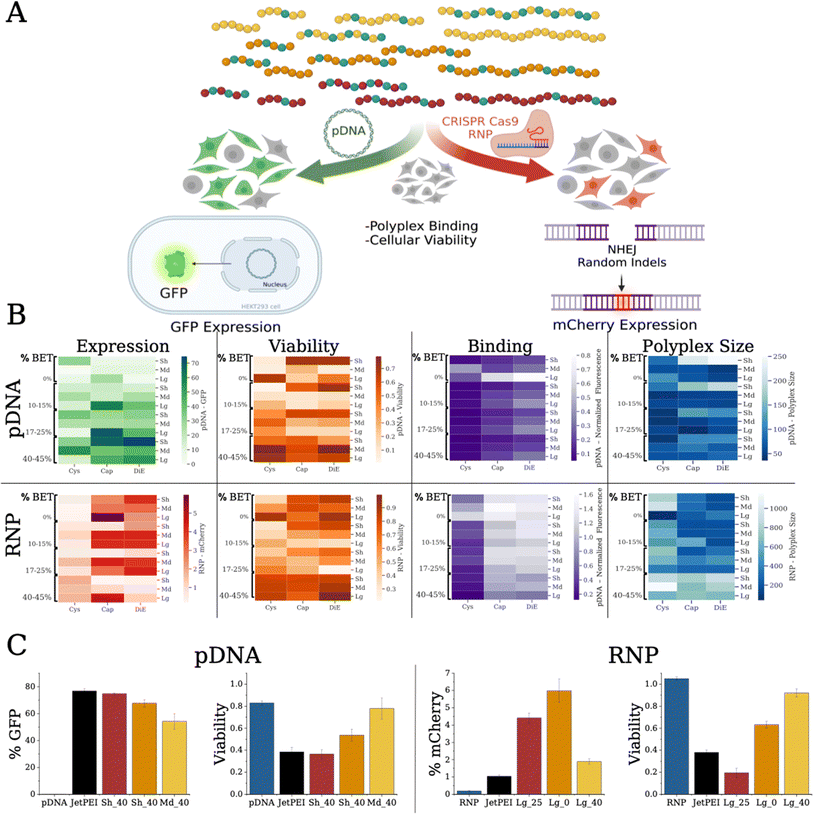 |
| Fig. 3 (A) Data schematic showing the parallel characterization workflow on polymer formulations with pDNA and CRISPR RNP displaying relative polymer–payload binding affinity, polyplex size (Rh), and in vitro assays with HEK293T cells monitoring GFP or mCherry expression. (B) Heat maps of compiled data to visualize binding, polyplex size, % cells with GFP (pDNA) or mCherry (RNP) expression, and toxicity reported as % cell viability. Diagrams show the polymer library with pDNA (top row, N/P 10) and RNP (bottom row, N/P 5). (C) Top expressing polymer of each cation (DiE – red, Cap – orange, Cys – yellow) in comparison to the payload pDNA or RNP only (blue) and JetPEI polyplex (black) controls. Data also shows the respective viability of each system. | |
To compare the relative binding affinity of the polymers to the payloads, we mixed a fluorescent dye, PicoGreen, with pDNA prior to forming polyplexes at various N/P ratios (Fig. S20–S22†). Lower fluorescence is linked to more dye excluded from the pDNA, indicative of higher relative binding affinity.47 Similar to previous reports, we found that higher steric bulk displayed lower relative binding (bulk increases from Cys to Cap to DiE).17 Additionally, we show that stronger binding correlates with BET composition, indicating possible intercalation of the pDNA.40,41 The Sh polymer backbones resulted in tighter binding compared to the Md and Lg scaffolds. For RNP binding (Fig. S23†), we used an OliGreen dye for RNP polyplexes. We found similar trends with regard to steric bulk, i.e., Cys functionalized polymers showed the tightest binding17 and that higher BET incorporation results in higher dye exclusion from the gRNA. We notice an opposite trend for polymer size however, where Lg scaffold variants promote stronger RNP binding. While efficient binding is important for payload encapsulation and protection, strong binding is not always positively correlated with biological delivery as payload release is important for performance.48 Overall, polyplex size and binding appeared to be correlated with BET composition, cation bulk, and scaffold length.
Transfection performance and toxicity
Reporter gene assays, via fluorescence output, are a facile tool to assess the delivery of biological payloads. We employ two transfection assays (Fig. 3A): (i) delivery of pDNA to HEK293T cells exhibiting expression of a green fluorescent protein (GFP) and (ii) delivery of RNP that upregulates mCherry expression in a modified HEK293T cell49 type, where the expression within can be measured on a per cell count through flow cytometry. We monitor cell health and metabolism through a cell counting kit (CCK-8) viability assay. As an initial screen we transfect pDNA at N/P ratios of 10 and 20 with the entire polymer library, and GFP expression was probed forty-eight hours post transfection (Fig. 3B and S24–S26†). We find that increasing BET in the Sh and Md scaffolds enhances transfection efficiency. The most effective polymer is Sh_DiE_40 (polymer RU_Cation_%BET) yielding 75% +GFP cells (Fig. 3C, left), similar to the JetPEI control (77% +GFP cells). We notice that at a formulation of N/P = 10, the Sh backbone exhibits higher viability, yet at N/P = 20, higher cell death occurs (Fig. S28–S30†).
An RNP is a complex formed with gRNA and CRISPR-Cas9 protein, here also containing 2 nuclear localization signals (NLS) to assist in nuclear translocation, that targets a precise genome cut in HEK293T cells engineered with a traffic light reporter (TLR) gene, reporting nonhomologous enjoining (NHEJ) via an indel frameshift upregulating mCherry expression.49 We show RNP transfection and toxicity at N/P ratios of 2.5 and 5 in Fig. 3B, S31, and S33–S35.† Formulation ratios were picked based on preliminary studies and standardized as a basic screening level to create a standard for the Bayesian optimization model to evaluate and optimize therefrom. We notice that while the Cys polymers have the strongest RNP binding, the Cap and DiE systems show significantly higher mCherry expression. Interestingly, Lg_Cap_0 at N/P 5 displays the highest mCherry expression (Fig. 3C, right) with ∼6% +mCherry. We note that only 1/3 of the indels cause the +mCherry frameshift and expression with this assay, but its facile application here allowed for rapid screening of the polymer library and recognition of its limitations.49 The commercial control, JetPEI, exhibits only 1% +mCherry cells, representing a six-fold decrease from the highest performing polymer in our library. Unlike our findings for pDNA, BET did not improve binding to RNP nor did it correlate with improving mCherry expression (denoting possible intercalation with only DNA). We discover a further deviating trend that higher editing is found with the longest polymers. Further, we notice that cell viability was much higher in this assay due to the lower N/P ratios. Overall, our screening protocols identify promising formulations that are highly effective at delivering pDNA and RNP while balancing toxicity. Collectively, we show that polymer chemistry, physical properties, and payload identity significantly affect performance; indeed, quantitative discernment of the physicochemical drivers of performance is complex and difficult, supporting the need for quantitative multifactorial analysis techniques to enable optimization more rapidly.
Polymer feature attribution through SHAP analysis
SHapley Additive exPlanations (SHAP),50 a machine learning technique, is used to extrapolate predictive importance on a given model variable (or feature) on a particular output. A high and positive SHAP value correlates with a high impact and a positive effect on the output variable. We start with our previous approach23,24 and then fit a machine learning model (details in the ESI†). To identify polymer and polyplex characteristics, our independent variables of scaffold RU, cation type (encoded as a continuous variable based on chemical fingerprints, as detailed in the ESI,† with Cys, Cap, and DiE values of 0.42, 0.74 and 0.82 respectively), % BET, pKa, clog
P, polyplex size (Rh), formulation (N/P) ratio, and binding strength are modeled to their effect on the dependent variables of expression and viability. Based on our cross-validated model, we analyze SHAP values for both pDNA (Fig. 4A) and RNP (Fig. 4B) payloads. We find that for pDNA, polymer pKa, BET incorporation, and scaffold RU have the highest absolute SHAP values across samples, which translate into a positive impact on GFP expression. We compare the average SHAP values in a spider plot (Fig. 4A) and show the level of impact each polymer feature has on GFP expression and cell viability for pDNA polyplexes. We show that lowering the pKa has a positive impact on GFP expression, likely due to a higher buffering capacity promoting endosomal escape.43,44 It should be noted that the decrease in the polymer pKa is a result of an increase in BET incorporation, where the co-cation aids in the initial binding at physiological pH. The SHAP for cell viability delivering pDNA (Fig. 4A) shows that lower formulation ratios and Md scaffold size, and higher % BET incorporation have the highest impact. We plot the SHAP values as a function of a given feature (SHAP dependency plots) for pDNA expression and find a linear dependence correlating lower pKa, increasing BET incorporation (Fig. 4C: left), and increasing clog
P values (Fig. 4C: middle) to higher SHAP values. Interestingly, when we compare the polymer length, the Md length was the least effective. Higher percentages of BET incorporation positively correlated with GFP expression and cell viability. Often, higher incorporation of hydrophobic units can contribute to increased cellular internalization through membrane disruption but this often causes higher toxicity,23,38,39,51 which is not found with our system. For pDNA delivery, we correlate the BET incorporation and improved performance with lowering polymer pKa.
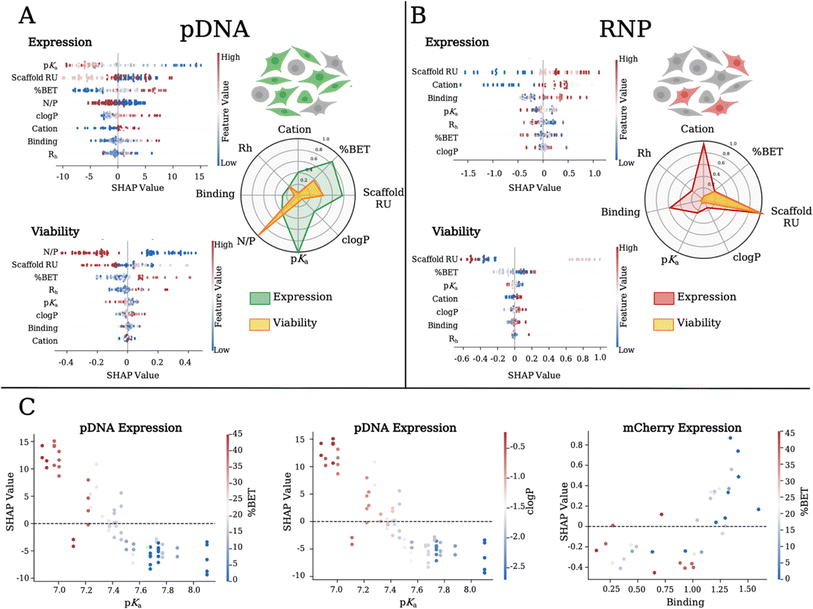 |
| Fig. 4 SHAP values for physicochemical features related to expression and cell viability when delivering (A) pDNA or (B) RNP. Higher SHAP values correlate with higher impact on the output variable. The feature value color bar corresponds to the normalized value of the feature of interest (where low = blue; moderate = white; high = red). Each dot represents a polymer formulation. (A) Overlay spider plot showing the average impact of individual polymer variables on expression and viability when delivering (A) pDNA and (B) RNP. The spider web plot is constructed by taking the mean SHAP value for a given feature across all samples and normalizing to the maximum SHAP value for each output variable. (C) SHAP dependency plot values across two variables relating to expression. | |
For RNP delivery, the mean SHAP values are shown in a spider plot (Fig. 4B), detailing the impact of each polymer feature on mCherry expression and cell viability. We find a positive correlation between the polymer length and increase in mCherry expression. We find that the Cys cation has the least importance for both pDNA and RNP delivery, while RNP delivery favors the Cap cation in the copolymer composition. Interestingly, with RNP we find that weaker binding polymers yield higher correlations with mCherry expression. SHAP values related to cell viability when delivering RNP (Fig. 4B) indicate that again Md scaffold size and higher % BET incorporation played the largest role in achieving higher cell viability. We notice on the SHAP dependency plot that RNP delivery efficiency is negatively correlated with binding and % BET, an opposite trend to that found with pDNA (Fig. 4C: left), demonstrating the intricacies of tuning polymer chemistry for specific biological payloads. Overall, we find that SHAP analysis quantifies and identifies predictive correlations and fundamental insight into the physicochemical components most influential for delivery of pDNA versus RNP. We show that lower pKa in conjunction with higher BET incorporation in the Sh and Lg scaffold variants are important for pDNA delivery. However, for gene editing with RNP, the Lg polymers along with Cap and lower BET incorporation are most influential.
Batch Bayesian optimization
To efficiently explore the combinatorial design space and its impact on expression performance outputs, we use batch BO. The total design space with discrete choices of N/P, cation, scaffold repeating units and BET incorporation spans over 5790 combinations, making manual exploration impractical. Using BO, we learn a probabilistic model, “the optimization model” (Fig. 5A, a Gaussian process model), which relates design variables to our target delivery efficacy and predicts outcomes of discrete polymer formulations. Then, based on the predicted mean expression and variability of the target variable in the design space, we select a new batch, which we call a BO round, of promising polymers to formulate and measure transfection efficacy. Our round 1 dataset is defined by sampling the entire polymer library at two N/P ratios, in triplicate, for each of the pDNA and RNP payloads, resulting in sampling 432 formulations (216 per payload). We complete two additional rounds of transfections with the most promising polymer formulation subsets defined by the batch BO model. Rather than uniform sampling across the entire library for optimization, BO beneficially limits the number of experimental sample conditions required by predicting the most promising polymer formulations to sample for round 2. This consists of 72 formulation ratios (36 per payload) for the model to improve predictive sampling. Round 3 predicts 48 more discrete sample formulations (24 per payload) to improve the mean expression output, while hovering above a certain viability threshold (viability ≥ 0.30). After three optimization rounds and sampling less than 10% of the design space, we did not observe further improvement in the expression while at the same time the BO proposals occurred frequently within the experimental resolution of the N/P variable (∼1%), and thus we deemed our sequential optimization concluded. The “Analysis by machine learning” section in the ESI† contains more information on the model and sampling algorithms used for BO.
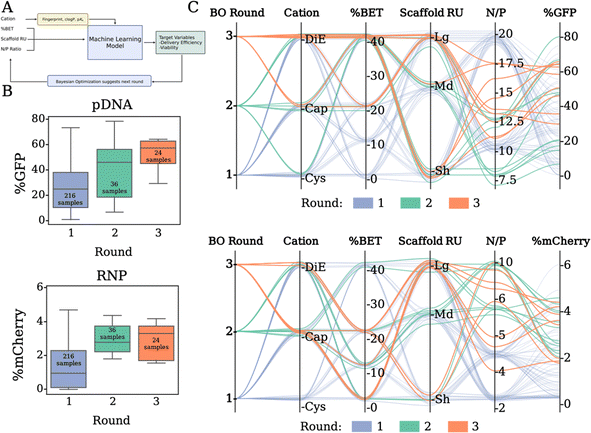 |
| Fig. 5 (A) Looped machine learning approach by tuning the N/P ratio based on polymer characteristics to effect delivery efficiency and cell viability through Bayesian optimization. (B) Sequential optimization data from the 3 rounds of experimental expression of GFP (top) and mCherry (bottom). (C) Parallel coordinate plots showing the copolymer compositions selected in rounds 2 and 3 and how this relates to the effective expression of GFP (top) and mCherry (bottom). | |
The progression of Fig. 5B presents our expression outputs for pDNA and RNP cargos respectively. Each box plot corresponds to the measured outputs after transfection. For pDNA (Fig. 5B, top), our model suggests a subset of polymer formulations that results in the overall best polymer for the dataset in round 2. The subset of polymer formulations chosen in round 3 was able to increase in overall expression, however no new optimal formulations were found. For RNP, our model explores a subset of promising polymers in rounds 2 and 3, which outperform most of the initial polymers, but was similar to the performance found in round 1. This is likely due to the degree of error in the mCherry expression to noise ratio in the assay making it slightly more difficult to refine. The parallel coordinate plots (Fig. 5C) summarize the progression of the sequential optimization to identify subsets of polymers that result in the highest expression. For pDNA (Fig. 5C, top), our model directs experimental sampling towards the cations of Cap and DiE, higher BET incorporation, and intermediate N/P ratios than the initial screen of 10 and 20, with the top three performing polymers being Sh_DiE_40 (N/P 12.5), Sh_Cap_40 (N/P 10), and Lg_Cap_25 (N/P 12.5). For RNP (Fig. 5C, bottom), our model suggests sampling cations with Cap and DiE, lower BET incorporation, and polymers derived from the larger scaffolds, with the top three performing polymers being Lg_Cap_0 (N/P 5), Lg_Cap_15 (N/P 8.6), and Sh_DiE_0 (N/P 10). The trends we outline from the parallel coordinate plots align well with the SHAP analysis of the most distinct correlative features, displaying that our model is properly identifying the most important physicochemical variables for prediction of future polymer formulations. Overall, we reveal a two-fold benefit of our customized probabilistic BO model: (i) BO allows for narrowing the amount of sampling and experimental time/expense from vast possibilities, and (ii) BO identifies optimal chemical configurations and formulation ratios for given constructs unique to a biological payload of choice.
In vivo delivery of pDNA
While polymeric vehicles are effective in vitro, the largest hurdle is in vivo performance. It is well known that cellular responses to various nanomedicines are not directly translatable and reflective through in vivo outcomes. This interplay often involves slow trial-and-error endeavors with exhaustive screenings between polymer chemistry, cellular transfection/viability assays, and ultimately, in vivo administration and therapeutic quantification. In terms of in vivo delivery and human therapy, the optimization process presented herein seeks to accelerate the pain points of polyplex formulation and bioevaluation by leveraging growing datasets of in vitro and in vivo response outputs to monomer, polymer, and polyplex descriptor inputs. The current work focuses on the systematic binary compositions of a hydrophobic cation BET with three other cations Cys, Cap, and DiE; the ease and versatility of this platform enable researchers to examine hydrophobic effects, stimuli responsivity, charge patterning, and other known design factors in an agile and generalizable manner for delivering any nucleic acid or protein of interest.
Predicted by SHAP and BO models, we selected our three top performers, Sh_DiE_40, Sh_Cap_40, and Md_Cys_40, for pDNA delivery in vivo (we selected N/P = 5 to minimize toxicity, although the optimal N/P in vitro occurs at different N/Ps). We chose these polymers, based on BO and diversity considerations, as Sh_DiE_40 and Sh_Cap_40 are the best-performing for each cation after optimization and Md_Cys_40 has the highest ratio of performance and uncertainty as estimated by BO. Our polyplexes and controls (pDNA only and JetPEI) were administered to mice via hydrodynamic tail vein injections in triplicate and the expression was compared over a 20 day span (Fig. 6A–C). The pDNA only control commonly promotes luciferase expression through hydrodynamic injections due to the large injection volume (5–10 wt%) over a small time period (4–8 s), inducing a pressure plug localizing to the liver (but generally diminishes rapidly).52–54 While these injections can induce stress to the mice, we find that all mice survive the injections with stable body weight over the 20 day span, indicating formulation tolerance (Fig. 6D). We show that mice injected with JetPEI polyplexes have the greatest loss of weight but recover after ∼6 days. The highest performing polymers yield a smaller dip in weight over the first 48 h, and an increase in weight is found prior to injection at 96 h, which remain stable over the 20 day span. While pDNA initially shows luciferase expression it rapidly decays, 1.7-fold faster than Md_Cys_40 and 1.6-fold faster than Sh_DiE_40 over a 6 day period (Fig. 6B). All three of our optimized polymers show a slower decay rate and outperform both controls. Polyplex formulations with Sh_DiE_40 and Sh_Cap_40 outperform the controls at all timepoints, however, Md_Cys_40 polyplexes decay in expression at a faster rate between days 10 and 20, dipping below JetPEI. Sh_DiE_40 shows the highest radiance throughout, outperforming all other formulations. Our data reveal the discovery of polymer formulations that can bind, protect, and deliver pDNA in vivo that shows stable and long-term expression and stability over excretion over 20 days in the mouse liver. In vivo studies with RNP formulations are forthcoming and will be reported in a future follow-up study as they require specifically engineered reporter animal models.
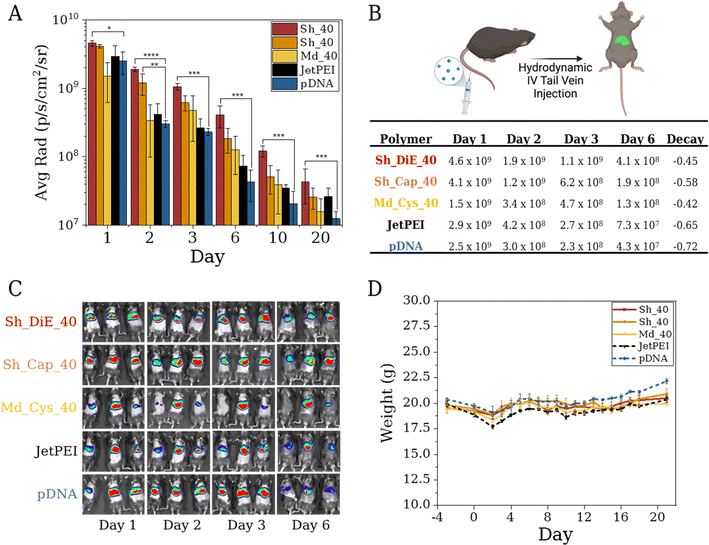 |
| Fig. 6 (A) Kinetic hydrodynamic tail vein study showing the average radiance (p per s per cm2 per sr) emitted after polyplex administration to mice over a 20 day study after delivery of a luciferase expressing pDNA (n = 3). (B) Table displaying average radiance values (p per s per cm2 per sr) and a decay rate that displayed first-order kinetics of triplicate mice on days 1–6. (C) Images of mice in triplicate showing the heat map expression on days 1, 2, 3, and 6 post injection. (D) Average weight of mice per sample group over 22 days. Day 0 is injection day. Statistical analysis was conducted via one-way ANOVA followed by a post hoc Tukey test (ns: p > 0.05, *: p ≤ 0.05, **: p ≤ 0.01, ***: p ≤ 0.001). | |
Conclusions
Here, we demonstrate a streamlined synthetic method via a facile post-polymerization modification yielding a polymer library systematically exploring chemical composition and physical parameters. Parallel assays combined with SHAP analysis and BO through progressive sampling allow modeling and quantitative understanding of features important to increasing the effective transient expression of pDNA as well as gene editing though the delivery of CRISPR-Cas9 RNP. Features of lower polymer pKa and higher % BET increase pDNA delivery, while polymer length and Cap cation identity are more effective for RNP delivery. Additionally, our three top performing copolymers selected by SHAP and BO display higher expression in vivo with a 1.7-fold kinetic enhancement of transgene expression over controls. Overall, facile tunable synthesis combined with screening and machine learning are powerful tools toward a data-driven materials discovery platform to identify candidates for in vivo screening and will aid the selection for clinical nucleic acid therapeutic delivery.
Data availability
In addition to the methods section, materials, characterization through NMR, SEC-MALLS, pKa titrations, DLS, dye exclusion, viability, supplemental data from flow cytometry, machine learning, and the in vivo experimental setup are available in the ESI.† Training data is proprietary and available under request and after author and company approval.
Author contributions
Rishad J. Dalal designed, performed and analyzed experiments along with writing the manuscript. Felipe Oviedo performed the machine learning analysis of SHAP and Bayesian optimization. Michael C. Leyden performed DLS studies on the RNP complexes and assisted in assembling the manuscript. Theresa M. Reineke helped design and supervised the research and helped write the manuscript.
Conflicts of interest
The authors declare the following competing financial interest(s): Theresa M. Reineke is one of the founders of Nanite, Inc. and has an equity interest. Nanite, Inc. is one of the sponsors of this research. This interest has been reviewed and managed by the University of Minnesota in accordance with its conflict-of-interest policy. Felipe Oviedo consults for Nanite, Inc. and has an equity interest.
Acknowledgements
We acknowledge Nanite, Inc. for funding this work. The resources and staff (Dr Guillermo Marques) at the University of Minnesota University Imaging Centers (UIC, SCR_020997) supported this work. We would also like to acknowledge Craig Flory for aid in the in vivo transfections and the technical assistance of Brenda Koniar, Joshua McCarra, Lia Coicou, Victoria Hoehn, and Kira Rolf from the Center for Translational Medicine at the University of Minnesota. We acknowledge Ramya Kumar, Leon Lillie, Derek Saxon and Cristiam Santa Chalarca for technical advice. Figures were partially made on https://Biorender.com. All in vivo hydrodynamic tail vein experiments were performed in compliance with the relevant guidelines from the IACUC committee at the University of Minnesota under an approved protocol.
References
- M. Ramamoorth and A. Narvekar, J. Clin. Diagn. Res., 2015, 9, GE01–GE06 Search PubMed.
- S. D. Li and L. Huang, J. Controlled Release, 2007, 123, 181–183 CrossRef CAS PubMed.
- I. Trapani, P. Tornabene and A. Auricchio, Gene Ther., 2021, 28, 220–222 CrossRef CAS PubMed.
- M. May, Genet. Eng. Biotechnol. News, 2020, 40, 42–44 Search PubMed.
- S. Nayak and R. W. Herzog, Gene Ther., 2010, 17, 295–304 CrossRef CAS PubMed.
- C. Liu, L. Zhang, H. Liu and K. Cheng, J. Controlled Release, 2017, 266, 17–26 CrossRef CAS PubMed.
- R. Kumar, C. F. Santa Chalarca, M. R. Bockman, C. Van Bruggen, C. J. Grimme, R. J. Dalal, M. G. Hanson, J. K. Hexum and T. M. Reineke, Chem. Rev., 2021, 121, 11527–11652 CrossRef CAS PubMed.
- C. Van Bruggen, J. K. Hexum, Z. Tan, R. J. Dalal and T. M. Reineke, Acc. Chem. Res., 2019, 52, 1347–1358 CrossRef CAS PubMed.
- S. Perrier, Macromolecules, 2017, 50, 7433–7447 CrossRef CAS.
- J. Chiefari, Y. K. B. Chong, F. Ercole, J. Krstina, J. Jeffery, T. P. T. Le, R. T. A. Mayadunne, G. F. Meijs, C. L. Moad, G. Moad, E. Rizzardo, S. H. Thang and C. South, Macromolecules, 1998, 9297, 5559–5562 CrossRef.
-
G. Moad, RAFT Polymerization – Then and Now, 2015 Search PubMed.
- R. B. Grubbs, Polym. Rev., 2011, 51, 104–137 CrossRef CAS.
- C. J. Hawker, A. W. Bosman and E. Harth, Chem. Rev., 2001, 101, 3661–3688 CrossRef CAS PubMed.
- K. Matyjaszewski, Macromolecules, 2012, 45, 4015–4039 CrossRef CAS.
- K. Matyjaszewski and J. Xia, Chem. Rev., 2001, 101, 2921–2990 CrossRef CAS PubMed.
- M. I. Gibson, E. F. Hlich and H.-A. Klok, American Chemical Society, Polymer Preprints, Division of Polymer Chemistry, 2008, 49, 511–512 Search PubMed.
- C. F. Santa Chalarca, R. J. Dalal, A. Chapa, M. G. Hanson and T. M. Reineke, ACS Macro Lett., 2022, 588–594 CrossRef CAS PubMed.
- M. A. Gauthier, M. I. Gibson and H.-A. Klok, Angew. Chem., Int. Ed., 2009, 48, 48–58 CrossRef CAS PubMed.
- A. Akinc, D. M. Lynn, D. G. Anderson and R. Langer, J. Am. Chem. Soc., 2003, 125, 5316–5323 CrossRef CAS PubMed.
- S. Barua, A. Joshi, A. Banerjee, D. Matthews, S. T. Sharfstein, S. M. Cramer, R. S. Kane and K. Rege, Mol. Pharm., 2009, 6, 86–97 CrossRef CAS PubMed.
- M. Goldberg, K. Mahon and D. Anderson, Adv. Drug Delivery Rev., 2008, 60, 971–978 CrossRef CAS PubMed.
- D. G. Anderson, A. Akinc, N. Hossain and R. Langer, Mol. Ther., 2005, 11, 426–434 CrossRef CAS PubMed.
- R. Kumar, N. Le, F. Oviedo, M. E. Brown and T. M. Reineke, JACS Au, 2022, 2, 428–442 CrossRef CAS PubMed.
- R. Kumar, N. Le, Z. Tan, M. E. Brown, S. Jiang and T. M. Reineke, ACS Nano, 2020, 14, 17626–17639 CrossRef CAS PubMed.
- T. K. Patra, ACS Polym. Au, 2022, 2, 8–26 CrossRef CAS PubMed.
- R. Batra, L. Song and R. Ramprasad, Nat. Rev. Mater., 2021, 6, 655–678 CrossRef.
- R. Upadhya, S. Kosuri, M. Tamasi, T. A. Meyer, S. Atta, M. A. Webb and A. J. Gormley, Adv. Drug Delivery Rev., 2021, 171, 1–28 CrossRef CAS PubMed.
- A. J. Gormley and M. A. Webb, Nat. Rev. Mater., 2021, 6, 642–644 CrossRef CAS PubMed.
- R. Ramprasad, R. Batra, G. Pilania, A. Mannodi-Kanakkithodi and C. Kim, npj Comput. Mater., 2017, 3, 54 CrossRef.
- R. Gómez-Bombarelli, J. Aguilera-Iparraguirre, T. D. Hirzel, D. Duvenaud, D. Maclaurin, M. A. Blood-Forsythe, H. S. Chae, M. Einzinger, D.-G. Ha, T. Wu, G. Markopoulos, S. Jeon, H. Kang, H. Miyazaki, M. Numata, S. Kim, W. Huang, S. I. Hong, M. Baldo, R. P. Adams and A. Aspuru-Guzik, Nat. Mater., 2016, 15, 1120–1127 CrossRef PubMed.
- J. N. Kumar, Q. Li, K. Y. T. Tang, T. Buonassisi, A. L. Gonzalez-Oyarce and J. Ye, npj Comput. Mater., 2019, 5, 73 CrossRef.
- Y. Wang, T. Xie, A. France-Lanord, A. Berkley, J. A. Johnson, Y. Shao-Horn and J. C. Grossman, Chem. Mater., 2020, 32, 4144–4151 CrossRef CAS.
- M. J. Tamasi, R. A. Patel, C. H. Borca, S. Kosuri, H. Mugnier, R. Upadhya, N. S. Murthy, M. A. Webb and A. J. Gormley, Adv. Mater., 2022, e2201809 CrossRef PubMed.
- A. Das and P. Theato, Macromolecules, 2015, 48, 8695–8707 CrossRef CAS.
- K. A. Günay, N. Schüwer and H. A. Klok, Polym. Chem., 2012, 3, 2186–2192 RSC.
- E. Blasco, M. B. Sims, A. S. Goldmann, B. S. Sumerlin and C. Barner-Kowollik, Macromolecules, 2017, 50, 5215–5252 CrossRef CAS.
- C. E. Hoyle and C. N. Bowman, Angew. Chem., Int. Ed., 2010, 49, 1540–1573 CrossRef CAS PubMed.
- C. Van Bruggen, D. Punihaole, A. R. Keith, A. J. Schmitz, J. Tolar, R. R. Frontiera and T. M. Reineke, Proc. Natl. Acad. Sci. U. S. A., 2020, 117, 32919–32928 CrossRef CAS PubMed.
- D. Pezzoli, E. Giupponi, D. Mantovani and G. Candiani, Sci. Rep., 2017, 7, 1–11 CrossRef PubMed.
- S. Bhattacharya and P. Chaudhuri, Curr. Med. Chem., 2008, 15, 1762–1777 CrossRef CAS PubMed.
- Y. Kubota, T. Iwamoto and T. Seki, Nucleic Acids Symp. Ser., 1999, 42, 53–54 CrossRef CAS PubMed.
- J. Shi, J. G. Schellinger, R. N. Johnson, J. L. Choi, B. Chou, E. L. Anghel and S. H. Pun, Biomacromolecules, 2013, 14, 1961–1970 CrossRef CAS PubMed.
- A. M. Nelson, A. M. Pekkanen, N. L. Forsythe, J. H. Herlihy, M. Zhang and T. E. Long, Biomacromolecules, 2017, 18, 68–76 CrossRef CAS PubMed.
- K. Miyata, M. Oba, M. Nakanishi, S. Fukushima, Y. Yamasaki, H. Koyama, N. Nishiyama and K. Kataoka, J. Am. Chem. Soc., 2008, 130, 16287–16294 CrossRef CAS PubMed.
- D. Sprouse and T. M. Reineke, Biomacromolecules, 2014, 15, 2616–2628 CrossRef CAS PubMed.
- R. J. Dalal, R. Kumar, M. Ohnsorg, M. Brown and T. M. Reineke, ACS Macro Lett., 2021, 10, 886–893 CrossRef CAS PubMed.
- P. M. McLendon, K. M. Fichter and T. M. Reineke, Mol. Pharm., 2010, 7, 738–750 CrossRef CAS PubMed.
- D. V. Schaffer, N. A. Fidelman, N. Dan and D. A. Lauffenburger, Biotechnol. Bioeng., 2000, 67, 598–606 CrossRef CAS PubMed.
- M. T. Certo, B. Y. Ryu, J. E. Annis, M. Garibov, J. Jarjour, D. J. Rawlings and A. M. Scharenberg, Nat. Methods, 2011, 8, 671–676 CrossRef CAS PubMed.
-
R. Sayres, A. Taly, E. Rahimy, K. Blumer, D. Coz, N. Hammel, J. Krause, A. Narayanaswamy, Z. Rastegar, D. Wu, S. Xu, S. Barb, A. Joseph, M. Shumski, J. Smith, A. B. Sood, G. S. Corrado, L. Peng and D. R. Webster, Proc. 31st Int. Conf. Neural Inf. Process. Syst., 2017, pp. 4768–4777 Search PubMed.
- Z. Tan, Y. Jiang, M. S. Ganewatta, R. Kumar, A. Keith, K. Twaroski, T. Pengo, J. Tolar, T. P. Lodge and T. M. Reineke, Macromolecules, 2019, 52, 8197–8206 CrossRef CAS.
- Z. P. Tolstyka, H. Phillips, M. Cortez, Y. Wu, N. Ingle, J. B. Bell, P. B. Hackett and T. M. Reineke, ACS Biomater. Sci. Eng., 2016, 2, 43–55 CrossRef CAS PubMed.
- K. M. Podetz-Pedersen, J. B. Bell, T. W. J. Steele, A. Wilber, W. T. Shier, L. R. Belur, R. S. McIvor and P. B. Hackett, Hum. Gene Ther., 2010, 21, 210–220 CrossRef CAS PubMed.
- J. B. Bell, K. M. Podetz-Pedersen, E. L. Aronovich, L. R. Belur, R. S. McIvor and P. B. Hackett, Nat. Protoc., 2007, 2, 3153–3165 CrossRef CAS PubMed.
|
This journal is © The Royal Society of Chemistry 2024 |
Click here to see how this site uses Cookies. View our privacy policy here.