DOI:
10.1039/D4RA04364B
(Paper)
RSC Adv., 2024,
14, 26747-26759
A new non-destructive method to decipher the origin of organic matter in fossils using Raman spectroscopy†
Received
14th June 2024
, Accepted 13th August 2024
First published on 23rd August 2024
Abstract
Ancient biomolecules provide a unique perspective on the past but are underutilized in paleontology because of challenges in interpreting the chemistry of fossils. Most organically preserved soft tissues in fossils have been altered by thermal maturation during the fossilization process, obscuring original chemistry. Here, we use a comprehensive program of thermal maturation experiments on soft tissues from diverse extant organisms to systematically test whether thermally altered biosignatures can be discriminated using Raman spectroscopy. All experimentally matured samples show chemical signatures that are superficially similar. Comparative analysis of Raman spectra following peak deconvolution, however, reveals strong tissue-specific signals. Application of this approach to fossils from the Bolca (49 Ma) and Libros (10 Ma) Konservat-Lagerstätten successfully discriminates fossil vertebrate soft tissue from that of fossil plants. Critically, our data confirm that a robust interrogation of Raman spectra coupled with multivariate analysis is a powerful tool to shed light on the taxonomic origins of thermally matured fossil soft tissues.
Introduction
The resolution of fossil biomolecular signatures is a major research frontier in paleontology. Macrofossils preserve molecular archives that can yield biological and ecological information on extinct organisms, including metabolic strategies,1 diet,2,3 palaeogeographical dispersion and niche partitioning4 and phylogenetic affinities.5–7 Organically preserved soft tissues in fossils are potentially a rich source of information on ancient biomolecules, but the nature of the tissue is not always known. This is especially likely where the soft tissues are preserved as an amorphous carbonaceous film.
Our ability to discriminate different fossil tissues based on preserved chemical data is constrained by two key factors. First, the ability of the fossil record to preserve biomolecular signals for different tissue types is presumed to be limited to fossils that are thermally immature.8–10 Fossil tissues that show evidence of more extensive thermal maturation – i.e., those with a kerogen-like chemistry – are relatively abundant in the fossil record but are understudied at the molecular level because considered to be relatively uninformative and to have lost diagnostic chemical markers (but see ref. 7 and 9). Second, many of the chemical analytical techniques used for detailed characterization of fossil chemistry are destructive (e.g., GC-MS,11–15 HPLC16–18), but not all fossils are available for destructive chemical analysis. Enhancing the diagnostic power of non-destructive analytical techniques is therefore of particular interest.
Raman spectroscopy (RS) is a non-destructive technique for the analysis of the vibrational fingerprint of organic and inorganic molecules, with broad applications in biological and medical research,19,20 materials science21 and geoscience.22–24 RS has been used to investigate molecular fingerprints of organic matter associated with fossil biomineralized tissues,25–30 fossil soft tissues5,31–33 and coprolites.3 The application of RS to thermally matured soft tissues is, however, limited by the nature of data collection, data processing and the original chemistry of the fossil soft tissues.
First, RS data are sensitive to the specific data collection/processing protocols used. Analysis of samples using different microscopes and/or laser wavelengths can yield spectra that differ in shape and/or band position.34–36 This can hinder comparative analysis of data from different studies (e.g., compare spectra of fossil melanin using Renishaw,33 HORIBA37 and custom-built microscopes38). Further, there are no standardized protocols for processing of RS data (indeed, data processing steps are not always reported), even though processing can substantially impact the final shape and interpretation of Raman spectra.39–42 An additional, critical, issue in applying RS to paleontological samples relates specifically to thermally matured soft tissues: Raman spectra from such fossils are superficially similar, if not identical, to that of kerogen (i.e., sedimentary insoluble organic matter)29,31,34 and eumelanin.43 Kerogen and eumelanin have similar vibrational modes for bonds in the indole ring and other key functional groups (e.g., C–C, C
C, C–N, C–O40,42), yielding Raman spectra with two prominent broad bands. The G (graphite) band is centered at ca. 1582 cm−1 and the D (disordered) band, at circa 1350 cm−1 (these wavenumber values correspond to analysis with a 532 nm laser). The G band relates to the E2g symmetric in-plane vibration of carbon in graphene-like sheets.42,44 The origin of the D band is not fully resolved, but may relate to double-resonant Raman scattering45–47 or A1g symmetric vibration in the graphite-like subunit and/or in polycyclic aromatic compounds (PAHs48–50). This broad spectral similarity between thermally matured soft tissues, kerogens and eumelanin potentially limits the applications of RS in fossils. Broader applications of RS to more matured (and often older) fossils therefore require a method to discriminate among kerogen and kerogen-like spectra.
Recent studies22,51 report that RS can discriminate different disordered aromatic carbonaceous solids, including different types of kerogen, using specific parameters that characterize secondary peaks that are derived from the deconvolution of the G and D bands (see Fig. S1†). The typical secondary peaks are as follows (reviewed in ref. 42). The G and D secondary peaks (not to be confused with the broad G and D bands, which are major spectral features; note that these peaks are, in some studies,42 referred to as the G1 and D1 peaks) are centered at ca. 1580 cm−1 and 1350 cm−1, respectively, and are assigned to the vibrational modes of the indole ring. The D2 and D6 peaks occur at ca. 1620 cm−1 and 1500 cm−1, respectively42,50,52 and are usually attributed to lattice-induced defects or out-of-plane tetrahedral carbon, respectively. The D5 peak is centered at ca. 1200 cm−1 and derives from sp3–sp2 bonds or C–C and C
C stretching vibrations of polyene-like structures.53,54 The D3 band is centered at 1400 cm−1 and the D4 bands occur primarily in the region between 1150 cm−1 and 1250 cm−1; both relate to C–H species in aliphatic hydrocarbon chains55 or to polyacetylene-like structures.56 Minor variations in the position, shape and intensity of the above secondary peaks reflect differences in the local biomolecular environment (i.e., the bonding environment immediately surrounding the molecule19,35) and/or molecular architecture (i.e., the three-dimensional structure and orientation of the molecule57). Peak deconvolution followed by extraction of secondary peak parameters, has the potential to discriminate among kerogen-like spectra, but has not yet been applied to thermally matured fossils.
Here, we resolve this issue by using RS to investigate the molecular alteration of diverse biological tissues (Fig. S2†) during thermal maturation experiments. Peak deconvolution coupled with multivariate analysis of extracted values for Raman parameters reveals that different tissues retain distinct chemical signatures after maturation. Analysis of fossil soft tissues from the Konservat-Lagerstätten of Bolca (49 Ma, Italy) and Libros (10 Ma, Spain) confirms that this approach can discriminate fossil tissues from different sources by revealing the preservation of cryptic tissue-specific Raman signatures. Critically, we show that RS can be used as a non-destructive, standalone, technique to decipher the chemistry of soft tissues in carbonaceous fossils in deep time.
Results
Molecular fingerprints of diverse thermally matured soft tissues
Untreated samples display Raman spectra that are consistent with their expected chemistry, based on key Raman-active molecules (Fig. 1; Datasets S1 and S2†). The cyanobacterial film (Fig. 1A), gymnosperm leaves, green algal film (Fig. S3†) and red insect cuticles exhibit a similar Raman signature comprising three strong, sharp peaks at 1005–1008 cm−1, 1156–1158 cm−1 and 1521–1523 cm−1. These peaks are assigned to CH3 coupled with C–C bonds in polyene chains (1008 cm−1), C–C bonds (1156–1158 cm−1) and C
C bonds (1521–1523 cm−1), respectively and are typical of carotenoids.58 Transparent shrimp cuticles (Fig. 1B) show strong peaks at 1200–1500 cm−1, 1630 cm−1 and 1665 cm−1. These peaks are assigned respectively to CH2 wagging and bending vibrational modes (1200–1500 cm−1), amide (1630 cm−1) and C
O bonds (1665 cm−1) and are typical of chitin. The medium-sized peak at 1007 cm−1 can be assigned to CH3 bonds typical of a carotenoid pigment dispersed in the cuticle.58 Weak peaks at 910 cm−1, 1045 cm−1 and 1150 cm−1 are assigned respectively to amidic (C–N vibration) bonds, C–O vibration and C–C antisymmetric ring vibrations; all are typical of chitin.59 White feathers (Fig. 1C) show a Raman signature dominated by strong peaks at ca.1003 cm−1, 1245 cm−1, 1455 cm−1, 1615 cm−1 and 1665 cm−1, assigned respectively to vibration of the aromatic ring (phenylalanine), amide III, CH2 and amide I;60 minor peaks at 500 cm−1 and 850 cm−1 are assigned to S–S and H-bonding groups.60 All of these peaks are typical of keratin.60 Spectra for melanin-rich samples (Fig. 1E–G), i.e., black insect cuticles, melanotic fungi and black feathers, are dominated by D and G bands. Despite this broad spectral similarity, there are subtle differences in spectral shape among these samples. For instance, the depth of the saddle between the D and G bands is narrower and deeper in black insect cuticles and melanotic fungi than in black feathers; further, the D band is narrower and sharper in melanotic fungi than in black feathers and insects.
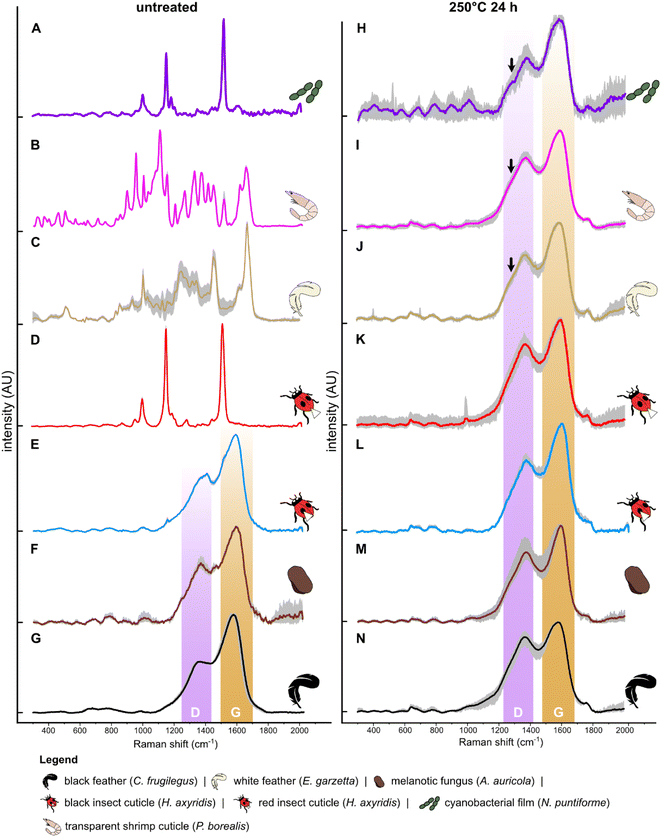 |
| Fig. 1 Mean (n = 9) Raman spectra for selected untreated and experimentally matured biological samples. Spectra for all samples are shown in Fig. S5.† Grey area represents the standard deviation. Arrow indicates shoulder. White arrowhead on beetle cuticle indicates sampling location. | |
After thermal maturation (Fig. S4†), all samples exhibit Raman spectra dominated by D and G bands, centered at ca. 1350 cm−1 and 1580 cm−1, respectively (Fig. 1H–N and S2†); an additional minor peak at ca. 1780 cm−1 is assigned to C
O bonds61 reflecting oxidation of the samples during maturation. Raman spectra for each set of samples differ in three ways. Spectra for cyanobacterial films (Fig. 1A), green algal films (Fig. S3†) and gymnosperm leaves (Fig. S5†) show sharper D and G bands than in spectra for other samples. In addition, spectra for these samples show a prominent shoulder centered at ca. 1270 cm−1 that is weak or absent in spectra for other samples. Finally, the saddle between the D and G bands is especially pronounced in spectra for matured gymnosperm leaves (Fig. S3†).
Linear Discriminant Analysis (LDA) of the dataset successfully discriminates samples based on their Raman spectra (LD1 = 87%; LD2 = 7%; Fig. 2A and S5, Dataset S3†), revealing four discrete chemical groups in the data. Group 1, in the center right of the chemospace, comprises all untreated melanin-rich samples and all matured samples (Fig. 2A). Group 2, in the lower left of the chemospace, comprises untreated samples rich in non-melanin pigments (i.e., carotenoids). Untreated samples rich in keratin (white feathers) and chitin (transparent shrimp cuticles) plot separately to groups 1 and 2, forming groups 3 and 4, respectively. The separation among groups is controlled by the variation in intensity of the wavenumbers that form peaks and bands in the Raman spectra (see Fig. S5; see ESI Text†). LD1 loadings discriminate between groups 1 and 3 (both characterized by positive LD1 loadings) and groups 2 and 4 (both characterized by negative LD1 loadings). Positive LD2 loadings characterize groups 3 and 4, whereas negative LD2 loadings characterize groups 1 and 2.
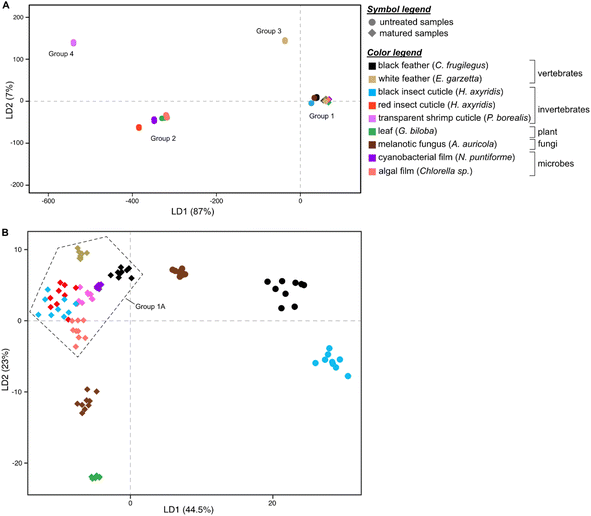 |
| Fig. 2 Linear Discriminant Analysis (LDA) chemospace plots showing variation in Raman parameters in the dataset. (A) Chemospace for the entire dataset (all untreated and matured samples). (B) Chemospace for the melanin-rich untreated samples and all matured samples. | |
To further explore chemical variation in group 1 (melanin-rich and matured samples), we performed LDA on this subset of the dataset. The resulting LDA chemospace plot (LD1 = 44.5%; LD2 = 23%; Fig. 2B and S6, Dataset S4†) successfully reveals chemical variation between untreated melanin-rich samples and matured samples (of all biological materials analyzed). LD1 loadings are positive for untreated melanin-rich samples and negative for matured samples. LD2 primarily explains the variation among matured samples; negative loadings identify (in ascending order), matured gymnosperm leaf, melanotic fungi and algal film samples. Positive LD2 loadings characterize matured insect (both red and black) and shrimp cuticles, matured white feather and matured black feather samples.
Untreated samples of melanin-rich feathers, fungi and black insect cuticles plot in the upper right quadrant of the chemospace and are chemically distinct from each other and from the other samples (Fig. 2B and S6†). Matured samples plot in the lower and left-hand regions of the chemospace. More specifically, matured gymnosperm leaves and melanotic fungi plot separately to a cluster of datapoints (group 1A) located close to the center of the chemospace (that includes all other matured samples). Within group 1A, the data for black and white feathers and cyanobacterial films are relatively distinct, but there is considerable overlap of the data for matured (amelanotic) shrimp cuticles and matured insect cuticles (both red and black) and matured algal film.
To discriminate the samples in this cluster, a further LDA was performed on this subset of the dataset using calculated Raman parameters (Dataset S5†). The results show that all groups plot separately in the chemospace (Fig. 3; LD1 = 89%; LD2 = 4%; Dataset S6†). The major discriminants controlling the separation are R1 and G frequency and, to a lesser extent, RBS, D frequency, wD/wG and Aratio. The data form three clusters that correspond to broad taxonomic groupings: vertebrate tissues plot on the left of the chemospace, invertebrate tissues in the center, and microbial samples on the right. Vertebrate tissues (represented by feather samples) show high values for Aratio and the frequency of the G peak relative to all other samples. Photosynthetic microbes (i.e., green algal films and cyanobacterial films) have the highest values for both RBS and D frequency. Arthropod cuticles (both red- and black insect cuticles and shrimp cuticles) show intermediate values for all of these parameters.
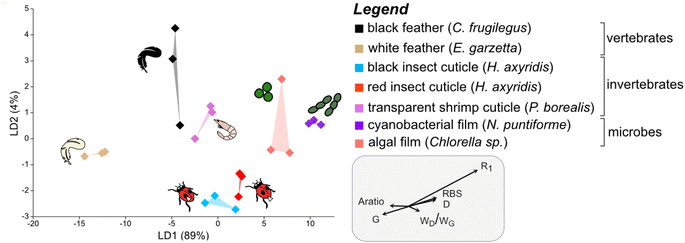 |
| Fig. 3 Linear Discriminant Analysis (LDA) chemospace plot showing variation in Raman parameters for the matured samples in group 1A, i.e., the plot in the top left quadrant of Fig. 2B. R1, ID/IG (where I is the intensity of the peak); RBS, Raman Band Separation; wD/wG (w denotes the Full Width Half Maximum (FWHM)); G, G peak frequency; D, D peak frequency; Aratio, areaD/areaG. | |
We interrogated the dataset using several statistical tests to assess the significance of the LDA groupings. The Wilks' lambda test results confirm that the separation between sample groups is statistically significant. The MANOVA test does not, however, recover statistically significant differences among all groups in the dataset (Wilks' lambda = 0.005; df = 6; F = 1.5; pMANOVA = 0.08). These apparently incongruous results may reflect, in part, the relatively small size of the dataset and important differences in the sensitivities of the two tests to different parameters of the transformed data. The Wilks' lambda test examines variation in within-class eigenvectors in the entire dataset, whereas MANOVA examines variation in within-class versus between-class scatter in both eigenvector and eigenvalues. Finally, ANOVA test results show that differences among sample groups are significant for the following Raman parameters (Dataset S7†): G frequency, R1 and RBS.
Discriminating between melanin-rich and amelanotic soft tissues in fossils
Raman spectra of all fossil samples (i.e., melanosome films and plants) exhibit D and G bands (Fig. 4). LDA of the total dataset (including untreated matured and fossil samples) resolves four distinct groups in the chemospace (Fig. S7, Dataset S8†): (A) melanin-rich, matured and fossil samples, (B) untreated (transparent) shrimp cuticles, (C) white feathers and (D) untreated leaves, green algal films, cyanobacterial films and red insect cuticles. Subsequent LDA (LD1 = 35%; LD2 = 20%; LD3 = 16%; Fig. 5A and S8, S9, Dataset S9†) of the data in group A resolves differences in chemistry among untreated melanin-rich, matured samples and fossils. Positive LD1 loadings are characteristic of fossil samples and matured leaves, plotting in the bottom right quadrant of the chemospace; negative LD1 and LD2 loadings are representative of matured samples, irrespective of their biochemistry. These samples plot on the lower left quadrant of the chemospace. Untreated melanins are characterized by positive LD2 loadings on the top right quadrant. Among the fossil samples, the plants from Libros plot separately to all other fossils.
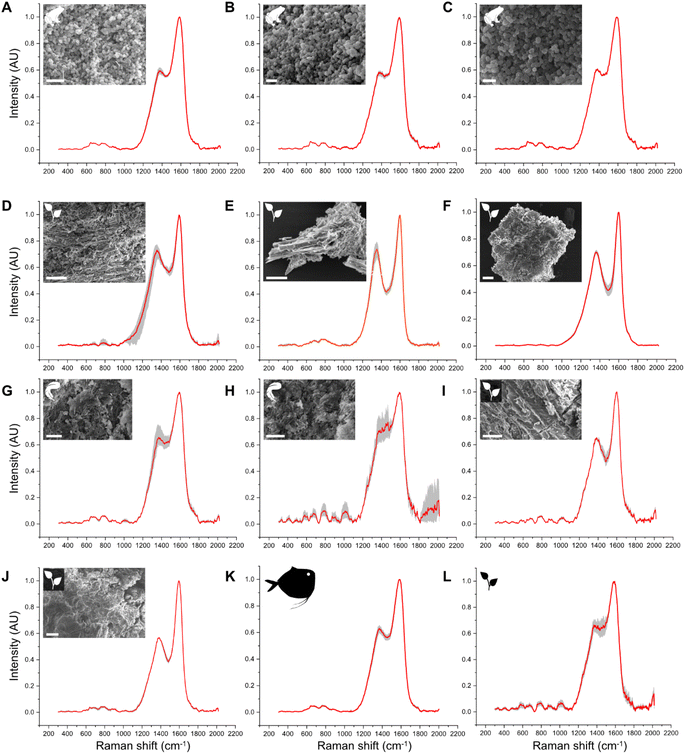 |
| Fig. 4 Raman spectra for fossil samples. Red: averaged spectra. Grey: replicate spectra (n = 3). (A–C) Pelophylax pueyoi (Anura, Libros biota); (D and E) plant fragments found on the same lamina as the fossil frog specimen in (A). (G and H) Anatidae indet. (Aves, Libros biota). (K and J) Plant fragments found on the same layer as the fossil bird. (I) Mene rhombea (Actinopterygii, Bolca biota). (L) Plant fragment (Bolca biota). Scale bars: 1 μm (A–C), 4 μm (G and H), 20 μm (D, F, I and J), 25 μm (E). | |
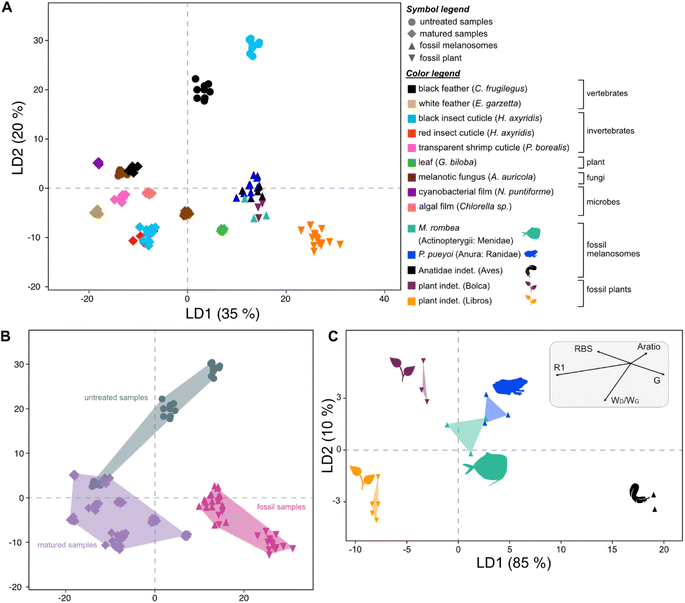 |
| Fig. 5 Linear Discriminant Analysis (LDA) including fossil data. (A) Chemospace for melanin-rich, experimentally matured and fossil samples. (B) Simplified representation of the space occupied by untreated, matured and fossil samples. The indicated groups were not constrained a priori, but were resolved by the analysis. (C) Chemospace for all fossil samples. R1, ID/IG (where I is the intensity of the peak); RBS, Raman Band Separation; wD/wG (w denotes the Full Width Half Maximum (FWHM)); G, G peak frequency; D, D peak frequency; Aratio, areaD/areaG. | |
A subsequent LDA using Raman parameters (Dataset S10†) resolves the origins of the chemical variation among fossils (LD1 = 85%; LD2 = 10%; Fig. 5C, Dataset S11†). Fossilized melanosome films from the frog (Libros) and the moonfish (Bolca) cluster in the center of the chemospace, whereas feather melanosomes (Libros) plot separately in the bottom right corner of the chemospace. The data from the fossil plants plot as two distinct groups in the left side of the plot; plant fragments from Bolca plot in the top left quadrant of the chemospace, whereas plant fragments from Libros plot in the bottom left quadrant. This separation is controlled by the following Raman parameters, in descending order of importance: R1 and G frequency, Aratio, wD/wG, RBS and D frequency. Fossil feather melanosomes show lower values for RBS and high values for G frequency compared to samples of internal melanosomes and plants. Fossil plant samples show higher values for RBS and R1 compared to melanosome samples. Libros plant samples show higher values for wD/wG and lower values for RBS relative to plants from Bolca.
MANOVA test results confirm that the separation between sample groups as visualized in the LDA chemospace is statistically significant. Similar to the results for the experimental dataset, this test does not recover significant differences among groups (Pillai test = 1.9; df = 4: F = 1; pMANOVA = 0.4). The ANOVA test, however, recovers significant differences among groups for the following Raman parameters (Dataset S7†): G-FWHM, R1 and RBS.
Discussion
Our results support the hypothesis that Raman spectroscopy can discriminate different tissues in both experimentally matured and fossil samples. Our experiments show that after maturation, all samples converge toward a common Raman signal dominated by D and G bands. These spectra cannot be readily discriminated without further interrogation of the data. Subtle differences in this kerogen-like Raman signal can be probed using LDA on spectral data, successfully discriminating between untreated melanin-rich samples and matured samples of different tissue types, i.e. leaves, fungi, cuticles and feathers. This preliminary screening, however, cannot discriminate all matured samples, or between these and fossils, as many matured and fossil samples possess similar spectra despite different original chemistries. Deeper interrogation of the data requires peak deconvolution and subsequent calculation of Raman parameters;22 collectively, these analytical procedures can successfully discriminate diverse tissue types in datasets that include thermally matured melanin-rich and amelanotic materials.
To date, Raman spectroscopy has been under-utilized in paleontology, especially on thermally matured fossils, due (at least in part) to the presumed inability of the technique to discriminate different kerogen-like molecules. Interrogation of spectral data via deconvolution into secondary peaks can clearly reveal important chemical variation that has important applications in understanding the original chemistry and, by extension, taxonomy of fossils. In recent studies, Raman spectra dominated by D and G bands have been used as non-diagnostic evidence for fossil melanin, especially to support other (usually morphological) evidence, e.g., preserved melanosomes, in fossil vertebrate soft tissues.31,33 RS has also been used to support evidence for melanin derived from other chemical data (e.g., AHPO-HPLC and/or FTIR32). The ultrastructural evidence of melanosomes, however, is applicable only to fossil vertebrates: invertebrates and other organisms (e.g., fungi and plants) that produce melanins in vivo62,63 lack melanosomes. Our approach to the analysis of Raman spectra will therefore be particularly useful for confident identification of fossil examples of melanin-rich tissues. Correct analysis and interpretation of key spectral features is contingent upon the appropriate acquisition of the data and application of certain processing steps, e.g. baseline correction and smoothing. In non-corrected spectra (see ref. 31 and 64), background fluorescence may limit or prevent correct interpretation of the saddle between the D and G bands, the presence/absence of peak shoulders and the degree of symmetry of the D and G bands.
As with other spectroscopic techniques (e.g., FTIR), deconvolution of broad Raman bands is typically used to characterize the chemistry of unknown materials, but the methodology varies. In studies on melanin in extant frogs and humans,43,65 deconvoluted secondary peaks are typically assigned to specific chemical bonds (reviewed in ref. 40) of indole, carboxylic and proteinaceous moieties. These secondary peaks have subsequently been used to characterize melanin types (e.g., synthetic versus natural,40 chemical changes due to photobleaching65 and metal loading66,67). In contrast, studies on kerogen usually use secondary peaks to extract Raman parameters, whereby the latter are then used to discriminate kerogens and enhance models of kerogen formation.22 In the absence of a standardized deconvolution protocol (including reporting of processing steps), comparative analysis of published data is not possible. Previous work has proposed68 that Raman parameters could be calculated from non-deconvoluted spectra, but peak deconvolution is essential to identify the vibrational mode of certain bonds when the latter overlap and form broad bands (e.g., D and G bands in melanin and amide I in proteinaceous compounds). Our data support other studies40–42,69 that strongly recommend the adoption of a standard protocol in the use and reporting of peak deconvolution of Raman spectra. Future studies on fossil tissues should report, in detail, all post-acquisition spectral processing and peak fitting steps (e.g. including baseline correction, smoothing, type of fitting function and number of peaks) in order to facilitate reproducibility and comparison with other data. Here, we present a novel and comprehensive approach for the analysis of Raman data from fossil materials, combining peak deconvolution with the use of Raman parameters. In particular, we demonstrate that the inclusion of Raman parameters in multivariate statistical analysis is a potent tool to successfully discriminate different thermally matured tissue types despite their superficially similar Raman signal.
Our study confirms that diverse melanin-rich tissues can be discriminated using their Raman signals and parameters. Application of our new approach to the analysis of Raman spectra of fossil samples confirms that fossil tissues originally rich in melanin can be successfully discriminated from other tissue types using Raman spectra. Indeed, our data suggest that degraded melanin may retain a diagnostic Raman signature in fossils, even if melanosomes are not preserved. Our data also reveal that fossilized amphibian melanosomes (Libros, 10 Ma) are more similar to fish melanosomes (Bolca, 48 Ma) than feather melanosomes (Libros, 10 Ma) despite differences in age and diagenetic history. This feature requires further investigation but may reflect broad differences in melanin composition between ectotherms and endotherms.70 Alternatively, chemical differences among fossils (as reflected in the distribution of specimens in Raman parameter chemospace) may reflect shifts in the position and shape of the D and G bands due to differences in the melanin metallome in different tissues and/or species.66,67 Fossilized melanosomes17,71,72 and leaves73 are associated with various metals that may be biological17,74 and/or diagenetic75–77 in origin. Future studies will assess the extent to which diagenetically incorporated metals can impact the Raman signal of thermally matured organic materials.
In conclusion, our findings support the feasibility of using RS to discriminate diverse thermally matured and fossilized soft tissues. Our approach expands the applications of RS in paleontology and provides a rigorous platform for future studies investigating the fossil record and evolution of melanins and other biomolecules in deep time.
Experimental
Experimental justification
The goal of the experiments was to determine whether tissues of different composition can be discriminated following thermal maturation using Raman spectroscopy. Diverse extant organisms were therefore selected that represent important fossil groups, i.e., vertebrates, invertebrates, plants, fungi, single-celled microalgae (i.e., green algae) and cyanobacteria; groups were also selected that contain important biomolecules, i.e., eumelanin, keratin, chitin and other pigments (i.e., carotenoids; Fig. S2†).
The tissues selected for analysis (Fig. S2†) are classified as follows. Class 1 (melanin-rich samples): black feather,18 black ladybird cuticle,78 melanotic fungi;79 class 2 (amelanotic samples): red ladybird cuticle, transparent shrimp cuticle, white feather,80 gymnosperm leaf, cyanobacterial film and green algal film. The specific parameters used in the maturation experiments were chosen in order to ensure the survival of melanosomes81,82 and the transformation of other tissue molecular components to low-grade organic matter (i.e., kerogen68).
Experimental set-up
Biological materials were procured as follows. Black and white feathers were collected from carcasses of a rook (Corvus frugilegus; Aves) and an egret (Egretta garzetta; Aves), respectively, each recovered in Cork city. Shrimp (Pandalus borealis; Malacostraca) and melanotic fungi (Auricularia auricola; Agaricomycetes) were purchased from a local market. Note that the use of “melanotic” is restricted to the fungal samples in accordance with convention. Gingko leaves (Gingko biloba; Ginkgoopsida) and ladybird (Harmonia axyridis; Insecta) carcasses were collected on UCC campus. A culture of green algae (Chlorella sp.; Trebouxiophyceae) was provided by Dr Raghuram Badmi, University College Cork and a culture of cyanobacteria (Nostoc punctiforme; Cyanophyceae) was provided by Prof. Carl Ng, University College Dublin.
The impact of maturation on the morphology and ultrastructure of the experimental samples was not investigated here because it is not relevant to this study; further, for many of these tissues, maturation-induced changes to tissue structure have been investigated previously.12,78,81–84
Samples of each biological material were prepared in triplicates (total n = 27). All samples (ca. 10 mm2 for feathers, leaf, fungus and shrimp cuticle; ca. 2 mm2 for ladybird elytron) were dissected with sterile tools and placed in sterile glass vials. Small aliquots (0.25 ml) of algal and cyanobacterial cultures, respectively, were placed in glass vials and dried in a fume hood prior to maturation. Vials were sealed loosely with aluminum foil to prevent cross-contamination during the experiment. Untreated samples were stored at −80 °C prior to Raman analysis. Samples for maturation experiments were placed in a standard laboratory oven at 250 °C for 24 hours, under atmospheric pressure, with oxygenated, dry conditions (no liquid medium). After the experiment, samples were allowed to cool to room temperature, photographed using a Leica EZ4W microscope (Fig. S4†) and stored at −80 °C prior to further analysis. Samples were defrosted and air-dried prior to Raman analysis.
Fossil samples
Fossil specimens (Fig. S10†) were selected from the Libros and Bolca Lagerstätten as these biotas include vertebrate specimens with melanosome films and abundant plant fragments on fossil-bearing slabs. For the Libros biota, one specimen of Pelophylax pueyoi (Anura) and one specimen of Anatidae indet. (Aves) were analyzed. Small (ca. 1 mm2) samples of the melanosome film were dissected from the torso of the frog (n = 3) and from the plumage of the duck (n = 2) using sterile tools. Three plant fragments were analyzed from the rock slab hosting the fossil frog, and two fragments from the slab hosting the fossil duck. The precise stratigraphic position of the fossils is unknown, but all come from a single interval of laminated mudstone < 120 m thick85 and presumably experienced a similar diagenetic history (e.g., burial pressure and temperature). For the Bolca biota, one specimen of the moonfish (Mene rhombea33) and one plant fragment were analyzed. Samples were dissected as described in ref. 33. All Bolca specimens are from a single outcrop and presumably experienced a similar diagenetic history.
Scanning electron microscopy
Samples of fossil soft tissues were placed on carbon tape on aluminum stubs, sputter coated with Au and screened for the presence of melanosomes and plant diagnostic features using a JEOL IT100 VP-SEM at an accelerating voltage of 10 kV and working distance of 10 mm. SEM analysis confirms (Fig. 4) that the samples from frog and feather soft tissues comprise exclusively melanosomes.
Raman spectroscopy
Three points were analyzed per replicate. Spectra were collected with a Renishaw inVia Qontor Raman Microscope System using a 532 nm 50 mW laser. The collected polychromatic light is diffracted by a grating with 1800 lines/mm and captured on a Peltier-cooled (−70 °C) near-infrared-enhanced, deep depletion CCD (Charge-Coupled Device; 1024 × 256 pixels). The instrument was calibrated to the 520.5 cm−1 line using an integrated silicon standard. Individual spectra were obtained using a 50× objective and, for almost all samples, a laser residence time of 10 s, laser power of 0.05–1% and ten accumulations. For white feather (keratin) and shrimp cuticle (chitin) samples, we increased laser power to 10% (but retained the same residence time and number of accumulations as the other samples) to obtain strong diagnostic peaks. A laser power of 0.05% was used for samples with high background fluorescence in order to avoid saturation of the detector. Post-analysis visual assessment of each analyzed point using the inbuilt light microscope confirmed no evidence of laser damage. All spectra were processed in WiRE 5.6 as follows. Background fluorescence was removed via intelligent fitting (type: intelligent polynomial, polynomial function order 9). Spectra were smoothed (Savitzky–Golay type, smooth window 9, polynomial 2) and then normalized with respect to the height of the highest peak. These data (hereafter referred to as spectral data) were exported as text and imported into OriginPro (v. 2023b) for further statistical analysis. Spectra (Dataset S2†) were analyzed using Principal Component Analysis (PCA) in OriginPro and using Linear Discriminant Analysis (LDA) in R (2023.06.0+421). During this analysis, each wavenumber was treated as a variable (nvar = 672). Data clusters in LDA plots were investigated further using Gaussian function deconvolution using the built-in deconvolution app in OriginPro (Dataset S12†). In these cases, three spectra from each point per sample replicate were averaged to obtain three spectra per sample (i.e. one per replicate). Averaged spectra from each replicate were truncated to fit peaks between 1813 cm−1 and 900 cm−1.
Peak deconvolution
For peak deconvolution, the baseline was set as constant, hidden peaks were added in line with the second derivative and the best fit (R2 ≥ 0.998) was achieved with 400 iterations per fit using a Gaussian function. The Gaussian function yields a better fit than other functions (e.g., Lorentzian, Voight) and is also widely used in biological and geological studies.40,41,69 All spectra were fitted using this approach. Following deconvolution, secondary peaks were identified and labelled following the literature on the Raman signature of kerogen;22,68 conventional peak labels and positions are reported in Fig. S1.†
Values for Raman parameters (sensu ref. 22) were defined as follows: D and G frequencies (i.e., the wavenumber defining the center of a peak), D-FWHM (full width at half maximum height), G-FWHM, R1(ID/IG; I denotes intensity of the peak), Aratio (areaD/areaG; note that this parameter is labelled “area: aD/aG” in ref. 22 and 42), RBS (Raman Band Separation: Dfrequency − Gfrequency), wD/wG (where w denotes FWHM; note that this parameter is referred to as FWHM-D/FWHM-G in ref. 22 and 42), D2-FWHM (D2-full width at half maximum height), D4-FWHM (D4-full width at half maximum height) and D6-FWHM (D6-full width at half maximum height).
Statistical analysis
Multivariate statistical techniques are widely used to analyze and classify Raman spectra for different biological samples25,86,87 and kerogens.42,88 We used Principal Component Analysis (PCA) and Linear Discriminant Analysis (LDA) to analyze the data. Here we provide a comprehensive explanation on the general use of PCA and LDA to the study of spectral data, with a final focus on the results presented here.
PCA and LDA are linear transformation techniques that are commonly used for reducing the dimensionality of a multivariate dataset, in order to readily visualize variation and/or as classification methods. In this study, we used PCA and LDA to visualize subtle, yet significant, differences in Raman spectral data among the samples in our dataset. Analysis of entire spectral datasets is commonly performed using PCA in isolation.89–91 In contrast, LDA is rarely used in isolation.92 Instead, it is usually used in combination with PCA as a classification method, i.e., PCA-LDA. This approach is often used for large datasets with hundreds of replicates per sample.93–95 Both PCA and LDA can be used on datasets comprising only selected wavenumbers that relate to specific peaks and/or bands.25 Here we discuss the application of PCA and LDA to data from the entire spectrum, whereby wavenumbers are used as variables.
PCA uses an “unsupervised” algorithm that ignores group labels (if provided a priori) and aims to find the directions (i.e., principal components) that maximize the variance in a dataset.96 LDA, on the other hand, is a “supervised” algorithm that maximizes the variance between known groups while minimizing the variance within each group. LDA computes the directions (i.e., linear discriminants) that represent the axes that maximize the between-group separation. Both PCA and LDA provide a scatterplot (also referred to as a score plot) which may assist in the identification of differences and/or similarities among groups. Although LDA maximizes among-group separation, the relative separation between different pairs of groups is indicative of similarity. The primary variables responsible for the separation of groups are shown in a loadings plot. The loading values can be visualized as vectors, histograms or line plots; the latter style is preferred for spectral data,89–92 and thus we use line plots to report the loadings in our analysis.
In general, PC loadings identify variations in peak position and intensity that can differentiate various chemical moieties present in a spectral dataset.94 Differences in the loading values, however, may not be reflected in well-resolved groups in the chemospace (see Fig. 3 in ref. 92). In fact, LD loadings do not define precise peaks and are usually noisy (e.g., see Fig. S5 and S6†), hindering direct interpretation of Raman spectra (see also ref. 92 and 97). This likely reflects how the LDA algorithm transforms the original spectral dataset and determines the linear discriminant axis that maximises between-group variation. This, coupled with the complexity of the dataset, which includes hundreds of variables (i.e., wavenumbers) and many groups (in this study, our dataset comprises 24 known groups), may deteriorate the loadings signal, yielding a plot dominated by analytical noise. Despite this, in general LDA is more successful than PCA in discriminating several groups as shown in the chemospace in Fig. 2 and 5A.
In our study the spectral data were first investigated using PCA; this failed to discriminate between melanin-rich, matured and fossil samples (see ESI Text; Fig. S11–S13†). For this reason, the spectral data were then analyzed using LDA to better visualize differences among known groups defined a priori.17,92 LDA on spectral data was performed in R as it can treat large datasets that include more variables than samples. The dataset for the Raman parameters includes cells with a value of N/A (as not all secondary peaks are present in all spectra); the LDA algorithm in R cannot process such datasets. Instead, LDA of the data for Raman parameters was performed in PAST as the software can analyze datasets that include cells with a value of N/A. MANOVA test was used to test the significance of the grouping of the data in the LDA chemospace: a Wilks' lambda test was used for the experimental dataset (balanced dataset) and the Pillai test was used for the dataset including the fossils (which is an unbalanced dataset). An ANOVA-type test was used to test the significance of single Raman parameters (i.e., variables) in controlling the differences among groups.
Data availability
The data supporting this article have been included as part of the ESI† and Datasets.
Author contributions
Valentina Rossi: conceptualization, methodology, investigation, formal analyses, data curation, visualization, writing – original draft preparation, project administration. Richard Unitt: methodology, investigation, visualization, data curation, writing – reviewing and editing. Maria McNamara: supervision, funding acquisition, resources, validation, writing – reviewing and editing.
Conflicts of interest
There are no conflicts to declare.
Acknowledgements
We thank T. Slater for assistance during the experiments. This project was funded by an ERC Consolidator grant H2020-ERC-CoG-1010003293-Palaeochem awarded to MMN.
References
- J. Wiemann, I. Menéndez, J. M. Crawford, M. Fabbri, J. A. Gauthier, P. M. Hull, M. A. Norell and D. E. Briggs, Fossil biomolecules reveal an avian metabolism in the ancestral dinosaur, Nature, 2022, 606, 522–526 CrossRef CAS PubMed.
- J. V. Tejada, J. J. Flynn, R. MacPhee, T. C. O'Connell, T. E. Cerling, L. Bermudez, C. Capuñay, N. Wallsgrove and B. N. Popp, Isotope data from amino acids indicate Darwin's ground sloth was not an herbivore, Sci. Rep., 2021, 11, 18944 CrossRef CAS PubMed.
- M. Tripp, J. Wiemann, J. Brocks, P. Mayer, L. Schwark and K. Grice, Fossil biomarkers and biosignatures preserved in coprolites reveal carnivorous diets in the Carboniferous Mazon Creek ecosystem, Biology, 2022, 11, 1289 CrossRef CAS PubMed.
- T. van der Valk, P. Pečnerová, D. Díez-del-Molino, A. Bergström, J. Oppenheimer, S. Hartmann, G. Xenikoudakis, J. A. Thomas, M. Dehasque and E. Sağlıcan, Million-year-old DNA sheds light on the genomic history of mammoths, Nature, 2021, 591, 265–269 CrossRef CAS PubMed.
- V. E. McCoy, J. Wiemann, J. C. Lamsdell, C. D. Whalen, S. Lidgard, P. Mayer, H. Petermann and D. E. G. Briggs, Chemical signatures of soft tissues distinguish between vertebrates and invertebrates from the Carboniferous Mazon Creek Lagerstätte of Illinois, Geobiology, 2020, 18, 560–565 CrossRef PubMed.
- C. S. Rogers, T. I. Astrop, S. M. Webb, S. Ito, K. Wakamatsu and M. E. McNamara, Synchrotron X-ray absorption spectroscopy of melanosomes in vertebrates and cephalopods: implications for the affinity of Tullimonstrum, Proc. R. Soc. B, 2019, 286, 20191649 CrossRef CAS PubMed.
- C. Loron, M. Sforna, F. Borondics, C. Sandt and E. Javaux, Synchrotron FTIR investigations of kerogen from Proterozoic organic-walled eukaryotic microfossils, Vib. Spectrosc., 2022, 123, 103476 CrossRef CAS.
- D. E. Briggs, Molecular taphonomy of animal and plant cuticles: selective preservation and diagenesis, Philos. Trans. R. Soc. London, Ser. B, 1999, 354, 7–17 CrossRef CAS.
- J. Alleon, S. Bernard, C. Le Guillou, D. Daval, F. Skouri-Panet, M. Kuga and F. Robert, Organic molecular heterogeneities can withstand diagenesis, Sci. Rep., 2017, 7, 1508 CrossRef.
- C. Colleary, H. M. Lamadrid, S. S. O'Reilly, A. Dolocan and S. J. Nesbitt, Molecular preservation in mammoth bone and variation based on burial environment, Sci. Rep., 2021, 11, 2662 CrossRef CAS PubMed.
- N. S. Gupta, R. Michels, D. E. Briggs, R. P. Evershed and R. D. Pancost, The organic preservation of fossil arthropods: an experimental study, Proc. R. Soc. B, 2006, 273, 2777–2783 CrossRef CAS PubMed.
- N. S. Gupta, G. D. Cody, O. E. Tetlie, D. E. Briggs and R. E. Summons, Rapid incorporation of lipids into macromolecules during experimental decay of invertebrates: initiation of geopolymer formation, Org. Geochem., 2009, 40, 589–594 CrossRef CAS.
- M. E. McNamara, B. E. Van Dongen, N. P. Lockyer, I. D. Bull and P. J. Orr, Fossilization of melanosomes via sulfurization, Palaeontology, 2016, 59, 337–350 CrossRef PubMed.
- I. Bobrovskiy, J. M. Hope, A. Ivantsov, B. J. Nettersheim, C. Hallmann and J. J. Brocks, Ancient steroids establish the Ediacaran fossil Dickinsonia as one of the earliest animals, Science, 2018, 361, 1246–1249 CrossRef CAS PubMed.
- A. Cincotta, T. T. Nguyen Tu, J. L. Colaux, G. Terwagne, S. Derenne, P. Godefroit, R. Carleer, C. Anquetil and J. Yans, Chemical preservation of tail feathers from Anchiornis huxleyi, a theropod dinosaur from the Tiaojishan Formation (Upper Jurassic, China), Palaeontology, 2020, 63, 841–863 CrossRef.
- K. Glass, S. Ito, P. R. Wilby, T. Sota, A. Nakamura, C. R. Bowers, J. Vinther, S. Dutta, R. Summons and D. E. Briggs, Direct chemical evidence for eumelanin pigment from the Jurassic period, Proc. Natl. Acad. Sci. U. S. A., 2012, 109, 10218–10223 CrossRef CAS PubMed.
- V. Rossi, M. E. McNamara, S. M. Webb, S. Ito and K. Wakamatsu, Tissue-specific geometry and chemistry of modern and fossilized melanosomes reveal internal anatomy of extinct vertebrates, Proc. Natl. Acad. Sci. U. S. A., 2019, 116, 17880–17889 CrossRef CAS PubMed.
- T. S. Slater, S. Ito, K. Wakamatsu, F. Zhang, P. Sjövall, M. Jarenmark, J. Lindgren and M. E. McNamara, Taphonomic experiments reveal authentic molecular signals for fossil melanins and verify preservation of phaeomelanin in fossils, Nat. Commun., 2023, 14, 5651 CrossRef CAS PubMed.
- J. J. Ruiz, M. Marro, I. Galván, J. Bernabeu-Wittel, J. Conejo-Mir, T. Zulueta-Dorado, A. B. Guisado-Gil and P. Loza-Álvarez, Novel non-invasive quantification and imaging of eumelanin and DHICA subunit in skin lesions by Raman spectroscopy and MCR algorithm: improving dysplastic nevi diagnosis, Cancers, 2022, 14, 1056 CrossRef CAS PubMed.
- I. P. Santos, R. van Doorn, P. J. Caspers, T. C. Bakker Schut, E. M. Barroso, T. E. Nijsten, V. Noordhoek Hegt, S. Koljenović and G. J. Puppels, Improving clinical diagnosis of early-stage cutaneous melanoma based on Raman spectroscopy, Br. J. Cancer, 2018, 119, 1339–1346 CrossRef PubMed.
- D. R. Neuville, D. De Ligny and G. S. Henderson, Advances in Raman spectroscopy applied to earth and material sciences, Rev. Mineral. Geochem., 2014, 78, 509–541 CrossRef CAS.
- A. Schito, D. Muirhead and J. Parnell, Towards a kerogen-to-graphite kinetic model by means of Raman spectroscopy, Earth-Sci. Rev., 2023, 237, 104292 CrossRef CAS.
- F. Foucher, G. Guimbretière, N. Bost, F. Westall and K. Maaz, Petrographical and mineralogical applications of Raman mapping, Raman Spectrosc. Appl., 2017, 163–180 CAS.
- N. V. Chukanov, M. F. Vigasina, N. V. Chukanov and M. F. Vigasina, Raman Spectra of Minerals, Springer, New York, 2020 Search PubMed.
- J. Wiemann, M. Fabbri, T.-R. Yang, K. Stein, P. M. Sander, M. A. Norell and D. E. Briggs, Fossilization transforms vertebrate hard tissue proteins into N-heterocyclic polymers, Nat. Commun., 2018, 9, 4741 CrossRef PubMed.
- J. Wiemann, J. M. Crawford and D. E. Briggs, Phylogenetic and physiological signals in metazoan fossil biomolecules, Sci. Adv., 2020, 6, eaba6883 CrossRef CAS PubMed.
- J. Wiemann and D. E. Briggs, Raman spectroscopy is a powerful tool in molecular paleobiology: an analytical response to Alleon et al, BioEssays, 2022, 44, 2100070, DOI:10.1002/bies.202000295.
- J. Wiemann and D. E. Briggs, Validation of biosignatures confirms the informative nature of fossil organic Raman spectra, bioRxiv, 2021, DOI:10.1101/2021.02.07.430162.
- J. Alleon, G. Montagnac, B. Reynard, T. Brulé, M. Thoury and P. Gueriau, Pushing Raman spectroscopy over the edge: purported signatures of organic molecules in fossil animals are instrumental artefacts, BioEssays, 2021, 43, 2000295 CrossRef CAS PubMed.
- E. T. Saitta, J. Vinther, M. K. Crisp, G. D. Abbott, L. Wheeler, S. Presslee, T. G. Kaye, I. Bull, I. Fletcher and X. Chen, Non-avian dinosaur eggshell calcite can contain ancient, endogenous amino acids, Geochim. Cosmochim. Acta, 2024, 365, 1–20 CrossRef CAS.
- J. A. Peteya, J. A. Clarke, Q. Li, K. Q. Gao and M. D. Shawkey, The plumage and colouration of an enantiornithine bird from the Early Cretaceous of China, Palaeontology, 2017, 60, 55–71 CrossRef.
- F. L. Pinheiro, G. Prado, S. Ito, J. D. Simon, K. Wakamatsu, L. E. Anelli, J. A. Andrade and K. Glass, Chemical characterization of pterosaur melanin challenges color inferences in extinct animals, Sci. Rep., 2019, 9, 15947 CrossRef PubMed.
- V. Rossi, R. Unitt, M. McNamara, R. Zorzin and G. Carnevale, Skin patterning and internal anatomy in a fossil moonfish from the Eocene Bolca Lagerstätte illuminate the ecology of ancient reef fish communities, Palaeontology, 2022, 65, e12600 CrossRef PubMed.
- D. G. Henry, I. Jarvis, G. Gillmore and M. Stephenson, Raman spectroscopy as a tool to determine the thermal maturity of organic matter: application to sedimentary, metamorphic and structural geology, Earth-Sci. Rev., 2019, 198, 102936 CrossRef CAS.
- Z. Huang, H. Lui, X. Chen, A. Alajlan, D. I. McLean and H. Zeng, Raman spectroscopy of in vivo cutaneous melanin, J. Biomed. Opt., 2004, 9, 1198–1205 CrossRef CAS PubMed.
- B. Thomas and S. Taylor, Proteomes of the past: the pursuit of proteins in paleontology, Expert Rev. Proteomics, 2019, 16, 881–895 CrossRef CAS PubMed.
- M. Fabbri, J. Wiemann, F. Manucci and D. E. Briggs, Three-dimensional soft tissue preservation revealed in the skin of a non-avian dinosaur, Palaeontology, 2020, 63, 185–193 CrossRef.
- I. Galván and A. Jorge, Dispersive Raman spectroscopy allows the identification and quantification of melanin types, Ecol. Evol., 2015, 5, 1425–1431 CrossRef PubMed.
- H. J. Butler, L. Ashton, B. Bird, G. Cinque, K. Curtis, J. Dorney, K. Esmonde-White, N. J. Fullwood, B. Gardner and P. L. Martin-Hirsch, Using Raman spectroscopy to characterize biological materials, Nat. Protoc., 2016, 11, 664–687 CrossRef CAS PubMed.
- G. Perna, M. Lasalvia and V. Capozzi, Vibrational spectroscopy of synthetic and natural eumelanin, Polym. Int., 2016, 65, 1323–1330 CrossRef CAS.
- M. Unal, R. Ahmed, A. Mahadevan-Jansen and J. S. Nyman, Compositional assessment of bone by Raman spectroscopy, Analyst, 2021, 146, 7464–7490 RSC.
- D. Henry, I. Jarvis, G. Gillmore and M. Stephenson, A rapid method for determining organic matter maturity using Raman spectroscopy: application to Carboniferous organic-rich mudstones and coals, Int. J. Coal Geol., 2019, 203, 87–98 CrossRef CAS.
- V. Capozzi, G. Perna, A. Gallone, P. Biagi, P. Carmone, A. Fratello, G. Guida, P. Zanna and R. Cicero, Raman and optical spectroscopy of eumelanin films, J. Mol. Struct., 2005, 744, 717–721 CrossRef.
- F. Tuinstra and J. L. Koenig, Raman spectrum of graphite, J. Chem. Phys., 1970, 53, 1126–1130 CrossRef CAS.
- I. Pócsik, M. Hundhausen, M. Koós and L. Ley, Origin of the D peak in the Raman spectrum of microcrystalline graphite, J. Non-Cryst. Solids, 1998, 227, 1083–1086 CrossRef.
- S. Reich and C. Thomsen, Raman spectroscopy of graphite, Philos. Trans. R. Soc. London, Ser. A, 2004, 362, 2271–2288 CrossRef CAS PubMed.
- M. Pimenta, G. Dresselhaus, M. S. Dresselhaus, L. Cancado, A. Jorio and R. Saito, Studying disorder in graphite-based systems by Raman spectroscopy, Phys. Chem. Chem. Phys., 2007, 9, 1276–1290 RSC.
- F. Negri, E. di Donato, M. Tommasini, C. Castiglioni, G. Zerbi and K. Müllen, Resonance Raman contribution to the D band of carbon materials: modeling defects with quantum chemistry, J. Chem. Phys., 2004, 120, 11889–11900 CrossRef CAS PubMed.
- C. Castiglioni, M. Tommasini and G. Zerbi, Raman spectroscopy of polyconjugated molecules and materials: confinement effect in one and two dimensions, Philos. Trans. R. Soc. London, Ser. A, 2004, 362, 2425–2459 CrossRef CAS PubMed.
- N. K. Lünsdorf, Raman spectroscopy of dispersed vitrinite—Methodical aspects and correlation with reflectance, Int. J. Coal Geol., 2016, 153, 75–86 CrossRef.
- A. Merlen, J. G. Buijnsters and C. Pardanaud, A guide to and review of the use of multiwavelength Raman spectroscopy for characterizing defective aromatic carbon solids: from graphene to amorphous carbons, Coatings, 2017, 7, 153 CrossRef.
- O. Beyssac, B. Goffé, C. Chopin and J. Rouzaud, Raman spectra of carbonaceous material in metasediments: a new geothermometer, J. Metamorph. Geol., 2002, 20, 859–871 CrossRef CAS.
- A. Sadezky, H. Muckenhuber, H. Grothe, R. Niessner and U. Pöschl, Raman microspectroscopy of soot and related carbonaceous materials: spectral analysis and structural information, Carbon, 2005, 43, 1731–1742 CrossRef CAS.
- A. Lahfid, O. Beyssac, E. Deville, F. Negro, C. Chopin and B. Goffé, Evolution of the Raman spectrum of carbonaceous material in low-grade metasediments of the Glarus Alps (Switzerland), Terra Nova, 2010, 22, 354–360 CrossRef CAS.
- N. Ferralis, E. D. Matys, A. H. Knoll, C. Hallmann and R. E. Summons, Rapid, direct and non-destructive assessment of fossil organic matter via microRaman spectroscopy, Carbon, 2016, 108, 440–449 CrossRef CAS.
- S. L. Rebelo, A. Guedes, M. E. Szefczyk, A. M. Pereira, J. P. Araújo and C. Freire, Progress in the Raman spectra analysis of covalently functionalized multiwalled carbon nanotubes: unraveling disorder in graphitic materials, Phys. Chem. Chem. Phys., 2016, 18, 12784–12796 RSC.
- Z. Li, L. Deng, I. A. Kinloch and R. J. Young, Raman spectroscopy of carbon materials and their composites: graphene, nanotubes and fibres, Prog. Mater. Sci., 2023, 101089 CrossRef CAS.
- P. M. Badgujar, Y. C. Wang and C. L. Cheng, A light-mediated study of carotenoids in carrots (Daucus carota) using resonance Raman spectroscopy, J. Raman Spectrosc., 2021, 52, 2609–2620 CrossRef CAS.
- N. Gierlinger, C. Reisecker, S. Hild and S. Gamsjaeger, Raman microscopy: insights into the chemistry and structure of biological materials, in Materials Design Inspired ByNnature: Function through Inner Architecture, The Royal Society of Chemistry, London, 2013, pp. 151–179 Search PubMed.
- S. Mattiello, A. Guzzini, A. Del Giudice, C. Santulli, M. Antonini, G. Lupidi and R. Gunnella, Physico-chemical characterization of keratin from wool and chicken feathers extracted using refined chemical methods, Polymers, 2022, 15, 181 CrossRef.
- D. Zahn, Raman studies of molecular thin films, Phys. Status Solidi A, 2001, 184, 41–50 CrossRef CAS.
- R. J. Cordero and A. Casadevall, Melanin, Curr. Biol., 2020, 30, R142–R143 CrossRef CAS PubMed.
- A. Y. Glagoleva, O. Y. Shoeva and E. K. Khlestkina, Melanin pigment in plants: current knowledge and future perspectives, Front. Plant Sci., 2020, 11, 770 CrossRef PubMed.
- Q. Li, J. A. Clarke, K.-Q. Gao, J. A. Peteya and M. D. Shawkey, Elaborate plumage patterning in a Cretaceous bird, PeerJ, 2018, 6, e5831 CrossRef PubMed.
- A. Saha, R. Arora, V. V. Yakovlev and J. M. Burke, Raman microspectroscopy of melanosomes: the effect of long term light irradiation, J. Biophot., 2011, 4, 805–813 CrossRef CAS PubMed.
- A. Samokhvalov, Y. Liu and J. D. Simon, Characterization of the Fe (III)-binding site in sepia eumelanin by resonance raman confocal microspectroscopy, Photochem. Photobiol., 2004, 80, 84–88 CAS.
- Y. Liu, L. Hong, V. R. Kempf, K. Wakamatsu, S. Ito and J. D. Simon, Ion-exchange and adsorption of Fe (III) by Sepia melanin, Pigm. Cell Res., 2004, 17, 262–269 CrossRef CAS.
- D. G. Henry, I. Jarvis, G. Gillmore, M. Stephenson and J. F. Emmings, Assessing low-maturity organic matter in shales using Raman spectroscopy: effects of sample preparation and operating procedure, Int. J. Coal Geol., 2018, 191, 135–151 CrossRef CAS.
- O. Beyssac, B. Goffé, J.-P. Petitet, E. Froigneux, M. Moreau and J.-N. Rouzaud, On the characterization of disordered and heterogeneous carbonaceous materials by Raman spectroscopy, Spectrochim. Acta, Part A, 2003, 59, 2267–2276 CrossRef.
- M. E. McNamara, V. Rossi, T. S. Slater, C. S. Rogers, A.-L. Ducrest, S. Dubey and A. Roulin, Decoding the evolution of melanin in vertebrates, Trends Ecol. Evol., 2021, 36, 430–443 CrossRef CAS.
- R. A. Wogelius, P. L. Manning, H. Barden, N. Edwards, S. Webb, W. Sellers, K. Taylor, P. Larson, P. Dodson and H. You, Trace metals as biomarkers for eumelanin pigment in the fossil record, Science, 2011, 333, 1622–1626 CrossRef CAS PubMed.
- P. L. Manning, N. P. Edwards, U. Bergmann, J. Anné, W. I. Sellers, A. van Veelen, D. Sokaras, V. M. Egerton, R. Alonso-Mori and K. Ignatyev, Pheomelanin pigment remnants mapped in fossils of an extinct mammal, Nat. Commun., 2019, 10, 2250 CrossRef PubMed.
- N. Edwards, P. Manning, U. Bergmann, P. Larson, B. Van Dongen, W. Sellers, S. Webb, D. Sokaras, R. Alonso-Mori and K. Ignatyev, Leaf metallome preserved over 50 million years, Metallomics, 2014, 6, 774–782 CrossRef CAS PubMed.
- N. P. Edwards, A. Van Veelen, J. Anné, P. L. Manning, U. Bergmann, W. I. Sellers, V. M. Egerton, D. Sokaras, R. Alonso-Mori and K. Wakamatsu, Elemental characterisation of melanin in feathers via synchrotron X-ray imaging and absorption spectroscopy, Sci. Rep., 2016, 6, 1–10 CrossRef PubMed.
- V. Rossi, S. M. Webb and M. E. McNamara, Hierarchical biota-level and taxonomic controls on the chemistry of fossil melanosomes revealed using synchrotron X-ray fluorescence, Sci. Rep., 2020, 10, 8970 CrossRef CAS PubMed.
- V. Rossi, S. M. Webb and M. McNamara, Maturation experiments reveal bias in the chemistry of fossil melanosomes, Geology, 2021, 49, 784–788 CrossRef CAS.
- C. S. Rogers, S. M. Webb and M. E. McNamara, Synchrotron X-ray fluorescence analysis reveals diagenetic alteration of fossil melanosome trace metal chemistry, Palaeontology, 2021, 64, 63–73 CrossRef.
- S. Wang, M. E. McNamara, B. Wang, H. Hui and B. Jiang, The origins of colour patterns in fossil insects revealed by maturation experiments, Proc. R. Soc. B, 2023, 290, 20231333 CrossRef CAS PubMed.
- Z. Qiu, S. Wang, J. Zhao, L. Cui, X. Wang, N. Cai, H. Li, S. Ren, T. Li and L. Shu, Synthesis and structural characteristics analysis of melanin pigments induced by blue light in Morchella sextelata, Front. Microbiol., 2023, 14, 1276457 CrossRef PubMed.
- T. S. Slater, N. P. Edwards, S. M. Webb, F. Zhang and M. E. McNamara, Preservation of corneous β-proteins in Mesozoic feathers, Nat. Ecol. Evol., 2023, 7, 1706–1713 CrossRef PubMed.
- M. E. McNamara, D. E. Briggs, P. J. Orr, D. J. Field and Z. Wang, Experimental maturation of feathers: implications for reconstructions of fossil feather colour, Biol. Lett., 2013, 9, 20130184 CrossRef PubMed.
- T. S. Slater, M. E. McNamara, P. J. Orr, T. B. Foley, S. Ito and K. Wakamatsu, Taphonomic experiments resolve controls on the preservation of melanosomes and keratinous tissues in feathers, Palaeontology, 2020, 63, 103–115 CrossRef PubMed.
- B. Stankiewicz, D. Briggs, R. Michels, M. Collinson, M. Flannery and R. Evershed, Alternative origin of aliphatic polymer in kerogen, Geology, 2000, 28, 559–562 CrossRef CAS.
- N. S. Gupta, O. E. Tetlie, D. E. Briggs and R. D. Pancost, The fossilization of eurypterids: a result of molecular transformation, Palaios, 2007, 22, 439–447 Search PubMed.
- M. E. McNamara, P. J. Orr, L. Alcalá, P. Anadón and E. Peñalver, What controls the taphonomy of exceptionally preserved taxa—environment or biology? A case study using frogs from the Miocene Libros Konservat-Lagerstätte (Teruel, Spain), Palaios, 2012, 27, 63–77 Search PubMed.
- J. Chen, M. Huang, Y. Zou, B. Song, Y. Wang, K. Wang, X. Li, X. Liu, X. Chen and F. Li, Multiple myeloma detection based on blood plasma surface-enhanced Raman spectroscopy using a portable Raman spectrometer, Laser Phys. Lett., 2016, 13, 105601 Search PubMed.
- E. Brauchle, S. Noor, E. Holtorf, C. Garbe, K. Schenke-Layland and C. Busch, Raman spectroscopy as an analytical tool for melanoma research, Clin. Exp. Dermatol., 2014, 39, 636–645 CrossRef CAS PubMed.
- E. Quirico, G. Montagnac, J.-N. Rouzaud, L. Bonal, M. Bourot-Denise, S. Duber and B. Reynard, Precursor and metamorphic condition effects on Raman spectra of poorly ordered carbonaceous matter in chondrites and coals, Earth Planet. Sci. Lett., 2009, 287, 185–193 CrossRef CAS.
- A. Ditta, H. Nawaz, T. Mahmood, M. Majeed, M. Tahir, N. Rashid, M. Muddassar, A. Al-Saadi and H. Byrne, Principal components analysis of Raman spectral data for screening of Hepatitis C infection, Spectrochim. Acta, Part A, 2019, 221, 117173 CrossRef CAS PubMed.
- S. Guo, P. Rösch, J. Popp and T. Bocklitz, Modified PCA and PLS: Towards a better classification in Raman spectroscopy–based biological applications, J. Chemom., 2020, 34, e3202 CrossRef CAS.
- X. He, Y. Liu, S. Huang, Y. Liu, X. Pu and T. Xu, Raman spectroscopy coupled with principal component analysis to quantitatively analyze four crystallographic phases of explosive CL-20, RSC Adv., 2018, 8, 23348–23352 RSC.
- L. Munyendo, M. Babor, Y. Zhang and B. Hitzmann, Chemometrics using near-infrared spectra for the quantification of robusta coffee and chicory added as adulterants in roasted arabica coffee, J. Food Meas. Charact., 2023, 1–14 Search PubMed.
- P. Buzzini, J. Curran and C. Polston, Comparison between visual assessments and different variants of linear discriminant analysis to the classification of Raman patterns of inkjet printer inks, Forensic Chem., 2021, 24, 100336 CrossRef CAS.
- H. Li, Y. Ren, F. Yu, D. Song, L. Zhu, S. Yu, S. Jiang and S. Wang, Raman microspectral study and classification of the pathological evolution of breast cancer using both principal component analysis-linear discriminant analysis and principal component analysis-support vector machine, J. Spectrosc., 2021, 2021, 1–11 Search PubMed.
- M. Lasalvia, V. Capozzi and G. Perna, A comparison of PCA-LDA and PLS-DA techniques for classification of vibrational spectra, Appl. Sci., 2022, 12, 5345 CrossRef CAS.
- I. T. Jolliffe, Principal component analysis for special types of data, in Principal Component Analysis, Springer, New York, 2002, 2nd edn, pp. 338–372 Search PubMed.
- N. Gerhardt, M. Birkenmeier, T. Kuballa, M. Ohmenhaeuser, S. Rohn and P. Wellen, Differentiation of the botanical origin of honeys by fast, non-targeted 1H-NMR profiling and chemometric tools as alternative authenticity screening tool, Proceedings of the XIII International Conference on the Applications of Magnetic Resonance in Food Science, 2016, pp. 7–10 Search PubMed.
|
This journal is © The Royal Society of Chemistry 2024 |
Click here to see how this site uses Cookies. View our privacy policy here.