DOI:
10.1039/D4RA03638G
(Paper)
RSC Adv., 2024,
14, 19362-19380
Exploring beetroot (Beta vulgaris L.) for diabetes mellitus and Alzheimer's disease dual therapy: in vitro and computational studies
Received
17th May 2024
, Accepted 11th June 2024
First published on 17th June 2024
Abstract
This study explored the flavonoid-rich extract of beetroot (Beta vulgaris L.) for type 2 diabetes mellitus (T2D) and Alzheimer's disease (AD) dual therapy by using in vitro and molecular simulation studies. Flavonoid-rich extracts of B. vulgaris fruit were evaluated for their antidiabetic and anti-alzheimic activities. Molecular docking and dynamic simulation were performed to identify potential bioactive flavonoids with dual therapeutic effects on T2D and AD. Flavonoid-rich extracts of B. vulgaris fruit (IC50 = 73.062 ± 0.480 μg mL−1) had moderate activity against α-amylase compared to the standard acarbose (IC50 = 27.104 ± 0.270 μg mL−1). Compared with acarbose, flavonoid-rich extracts of B. vulgaris fruit had appreciable activity against α-glucosidase (IC50 = 17.389 ± 0.436 μg mL−1) (IC50 = 37.564 ± 0.620 μg mL−1). For AChE inhibition, flavonoid-rich extracts of B. vulgaris fruit exhibited (p < 0.0001) inhibitory activity (IC50 = 723.260 ± 5.466 μg mL−1), albeit weaker than that of the standard control, galantamine (IC50 = 27.950 ± 0.122 μg mL−1). Similarly, flavonoid-rich extracts of B. vulgaris fruit showed considerable (p < 0.0001) inhibitory effects on BChE (IC50 = 649.112 ± 0.683 μg mL−1). In contrast, galantamine (IC50 = 23.126 ± 0.683 μg mL−1) is more potent than the extracts of B. vulgaris fruit. Monoamine oxidase (MAO) activity increased in FeSO4-induced brain damage. In contrast, flavonoid-rich extracts of B. vulgaris fruit protected against Fe2+-mediated brain damage by suppressing MAO activity in a concentration-dependent manner. HPLC-DAD profiling of the extracts identified quercetrin, apigenin, rutin, myricetin, iso-quercetrin, p-coumaric acid, ferulic acid, caffeic acid, and gallic acid. Molecular docking studies revealed quercetrin, apigenin, rutin, iso-queretrin, and myricetin were the top docked bioactive flavonoids against the five top target proteins (α-amylase, α-glucosidase AchE, BchE, and MAO). Molecular dynamic simulations revealed that the complexes formed remained stable over the course of the simulation. Collectively, the findings support the prospect of flavonoid-rich extracts of B. vulgaris root functioning as a dual therapy for T2D and AD.
Introduction
In contemporary times, diabetes mellitus (DM) and Alzheimer's disease (AD) present formidable challenges to public health.1 DM, characterized by elevated blood sugar levels resulting from insulin deficiency or resistance, affects millions of people worldwide, leading to complications such as cardiovascular diseases, neuropathy, and nephropathy. By 2019, there were approximately 463 million confirmed and undiagnosed cases of diabetes globally, with projections to reach 700 million by 2045.2 DM is classified into two primary types, type 1 (T1D) and type 2 (T2D), with T2D accounting for up to 95% of cases. T2D is characterized by elevated blood sugar levels due to insufficient insulin production by pancreatic cells and insulin resistance.3 One therapeutic avenue for mitigating postprandial hyperglycemia involves inhibiting carbohydrate-hydrolyzing enzymes, α- and β-glucosidases (α- and β-Glu), to decelerate glucose digestion in the digestive system.4
Alzheimer's disease (AD), a progressive neurodegenerative disorder, precipitates cognitive decline, memory impairment, and behavioral changes, imposing significant burdens on patients, caregivers, and healthcare systems globally. It was estimated that approximately 57.4 million individuals were affected by the disease in 2019, with projections to hit 152.8 million by 2050.5 The degeneration of cholinergic neuron structure and function is a hallmark of AD. The brain's vulnerability to damage due to its limited antioxidant capacity is recognized as a major contributing factor to AD pathogenesis.6 The “cholinergic hypothesis” posits that acetylcholinesterase (AChE) serves as a pivotal regulatory enzyme at cholinergic synapses, while butyrylcholinesterase (BuChE), a closely related enzyme, acts as a coregulator of cholinergic neurotransmission by breaking down acetylcholine (ACh). The inhibition of both AChE and BuChE has been identified as a critical target for effectively managing Alzheimer's disease, as it enhances the availability of ACh in brain regions.7
The utilization of plants in traditional medicine is deeply rooted in the customs and heritage of a significant portion of the global population. Beta vulgaris L. (chenopodiaceae), commonly referred to as ‘beetroot’ or ‘chukandar’, has been recognized and cultivated since ancient times and is found in both white and red varieties.8 Beta maritima, the precursor of all modern beet cultivars, including beetroots, is believed to have originated in the Mediterranean region. This sea beet species has been found along the coastlines of Europe, North Africa, the Middle East, and parts of Asia since ancient times.9 As a nutrient-dense source, beetroot is believed to possess health-enhancing properties in addition to antioxidant, anti-inflammatory, anticarcinogenic, antidiabetic, hepatoprotective, hypotensive, and wound-healing properties.10
Beetroot contains an array of bioactive compounds, including betalains, polyphenols, flavonoids, vitamins, minerals, and dietary fibers. Betalains, comprising betacyanins and betaxanthins, confer the red and yellow hues of beetroot and exhibit antioxidant, anti-inflammatory, and cytoprotective properties.11 Polyphenols, another crucial group of phytochemicals in beetroot, exert various biological effects, such as antioxidant, anti-inflammatory, antidiabetic, and neuroprotective effects. These bioactive constituents play a pivotal role in the potential therapeutic benefits of beetroot in managing the processes associated with diabetes and Alzheimer's disease.12 Meanwhile, high-performance liquid chromatography (HPLC) profiling of bioactive compounds in beetroot extracts, offering valuable insights for further research.13
Molecular docking simulations provide a computational framework for predicting the interactions between beetroot constituents and target proteins associated with the pathogenesis of diabetes mellitus and Alzheimer's disease. Through the analysis of binding affinity and mode of interaction, it becomes feasible to rationalize the design of novel therapeutic agents.14 Ex vivo studies involving animal tissue samples furnish valuable preclinical data on the pharmacokinetic and pharmacodynamic properties of beetroot. By scrutinizing its impact on cellular signaling pathways, gene expression profiles, and tissue morphology, the translational potential of beetroot for therapeutic interventions substantiated.15 This study investigated the therapeutic potential of beetroot as a dual therapy and elucidated its mechanisms of action to devise innovative strategies for managing AD and T2D conditions, thereby offering hope for improved patient outcomes and quality of life.
Materials and methods
Plant materials
B. vulgaris fruit (beetroot) was purchased from the Jos Terminal Market, Plateau State, Nigeria. The plant was verified at the Forestry Research Institute of Nigeria in Ibadan with herbarium number FHI 114105.
Flavonoid-rich extract preparation
Fifty (50) gram of powdered B. vulgaris fruit was macerated for 72 hours in 80% methanol to obtain a crude methanolic extract. Twenty (20) gram of the crude methanolic extract was then dissolved in 200 millilitres of 10% H2SO4 and heated to 100 degrees Celsius in a water bath for 30 minutes to initiate hydrolysis. The mixture was left on ice for fifteen minutes to allow the flavonoid aglycones to precipitate. After dissolving the flavonoid aglycone in 50 mL of warm 95% ethanol, the mixture was filtered into a 100 mL volumetric flask that had been filled to the top with 95% ethanol. A rotary evaporator was used to concentrate the solution. The filtrate was then precipitated using concentrated ammonium hydroxide. To extract the flavonoid extracts, the entire solution was allowed to settle, the precipitate was collected, and then it was washed with diluted ammonium hydroxide.
High-performance liquid chromatography (HPLC–DAD) analysis
An HPLC system (Agilent Technologies 1100-series, Agilent, San Jose, CA, USA) with a quaternary pump and a UV-DAD detector equipped with a C18 column (250 mm × 4.6 mm, internal diameter 5 μm, Zorbax Eclipse Plus, Agilent, USA) was used. Following a previously established protocol (Araujo-León et al., 2019), chromatography was performed under gradient conditions with H2O:MeOH:THF. The water contained 1% H3PO4, and the flow rate of the mobile phase was 1.5 mL min−1. A total of 20 μm of the sample was injected. The column was purged with the mobile phase for 10 minutes, followed by equilibration for 10 minutes, after which 35 minutes was required for sample analysis. Spectral data were collected at a detection wavelength of 220 nm for analysis.16
Enzyme inhibitory studies
α-Amylase inhibition. The inhibitory effect of the flavonoid-rich extract of B. vulgaris roots on α-amylase was assessed using the methodology described elsewhere.17 The sample, consisting of 250 μL at concentrations ranging from 7.81 to 1000 μg mL−1, was incubated with approximately 500 μL of porcine pancreatic amylase at a concentration of 2 U m L−1. The mixture was incubated in phosphate buffer at a pH of 6.8 and a concentration of 100 mmol L−1. The mixture was incubated for 20 minutes at 37 °C. Next, 250 microliters of a 1% starch solution mixed in phosphate buffer with a pH of 6.8 (100 millimoles per liter) was added to the mixture and incubated at 37 °C for 1 hour. A solution of 1 mL of dinitrosalicylic acid was prepared, which included 3,5-dinitrosalicylic acid (1%), phenol (approximately 0.2%), Na2SO3 (0.05%), and sodium hydroxide (1%). This solution was then heated at 100 °C for 10 minutes. Subsequently, the mixture was cooled to approximately 25 °C using a cold-water bath, after which the absorbance of the resultant mixture was measured at 540 nm using a spectrophotometer. The research used acarbose as the standard. The outcome was computed and reported as percentage inhibition.
α-Glucosidase inhibitory activity. The inhibitory effect of the flavonoid-rich extract of B. vulgaris roots on α-glucosidase was assessed using a protocol described elsewhere.17 One milligram of α-glucosidase was dissolved in a phosphate buffer solution of 100 millilitres, with a pH of approximately 6.8. The buffer solution also included 200 milligrams of bovine serum albumin (BSA). The solution consisted of 10 μL of the sample at different concentrations ranging from 15–240 μg mL−1 combined with 490 μL of phosphate buffer at a pH of approximately 6.8 and 250 μL of 5 mM p-nitrophenyl α-D-glucopyranoside. The sample was preincubated at 37 °C for 15 minutes. Next, 2000 μL of Na2CO3 (200 mM) was added to halt the process. The α-glucosidase activity was measured using a spectrophotometer by recording the absorbance at a wavelength of 400 nm. The positive control used was acarbose, an inhibitor of α-glucosidase.
Determination of acetylcholinesterase (AChE) and butyrylcholinesterase (BChE) enzyme inhibition. The inhibitory effects of flavonoid-rich extracts of B. vulgaris roots on AChE and BChE were determined. The enzyme activity experiments used acetylthiocholine iodide (AChI) and butyrylcholine iodide (BChI) as substrates.18 The activities of BChE and AChE were determined using 5,5′-dithio-bis(2-nitrobenzoic) acid (DTNB). A total of 7.81–1000 μg mL−1 flavonoid-rich extract of B. vulgaris roots and buffer solution (100 μL, pH 8.0, Tris–HCl, 1.0 M) were added to 50 μL of BChE and AChE solutions (5.32 × 10−3 enzyme units). Subsequently, the products were incubated for 10 minutes at a temperature of 20 degrees Celsius. Following the completion of the aforementioned procedures, 50 μL of DTNB (0.5 mM and 25 mL) and BChI/AChI (50 μL) were introduced into the solutions. Furthermore, the reaction was triggered by adding 50 μL of BChI/AChI. The effectiveness of both enzymes was assessed at a wavelength of 412 nm. An AChE unit catalyzes the hydrolysis of 1.0 mol of AChI to produce choline and acetate. Similarly, a BChE unit refers to the quantity of enzyme that hydrolyzes 1.0 mol of BChI to produce choline and butyrate per minute at 37 °C (pH 8.0). The enzymes, including AChE from electric electrophorus electricus, and BChE from horse serum, were obtained from Sigma. Galanthamine was used as the positive control for AChE and BChE assays.
Ex vivo experiment
Experimental rats and organ preparation. Healthy male Wistar rats weighing 150–200 g each were purchased from the Department of Biochemistry, Bowen University, Nigeria. The rats were euthanized with halothane after being fasted overnight, and the brain was removed and homogenized in 1% Triton X-100 in 50 mM phosphate buffer. The homogenate was centrifuged at 3000 rpm and 40 °C. For ex vivo studies, the supernatants were collected in simple plain tubes. The rats were in agreement with the approved policies of The Department of Biochemistry, Bowen University Research Ethics Committee, and the study was approved (approval number: BUI/BCH/2024/0002) and reported in accordance with the ARRIVE guidelines.
Induction of brain injury ex vivo. Brain damage was induced ex vivo using Fe2+ following the methods described by Erukainure et al.19 (2020). In brief, 100 μL of 0.1 mM FeSO4 was mixed with 200 μL of the tissue lysate containing varying concentrations (31.25–1000 μg mL−1) of the flavonoid-rich extract. The samples were then incubated for 30 minutes at 37 °C before being used for biochemical evaluations. Reaction mixtures containing only the tissue homogenate served as the normal control, while a mixture of only the tissue homogenate and FeSO4 served as the negative control.
Determination of monoamine oxidase (MAO) activity. The inhibitory effect of flavonoid-rich extract of B. vulgaris roots on monoamine oxidase activity was assessed by using the methodology outlined by Green and Haughton in 1961. The orange–yellow color was quantified at a wavelength of 450 nm using a UV spectrophotometer and determined using the specified formula.20 Safinamide was used as the positive control for MAO activity.
% inhibition = abscontrol − abstest sample/abscontrol × 100 |
Computational studies
Protein structure preparation. Protein structures were retrieved from the Protein Data Bank (http://www.rcsb.org/) for the three-dimensional structures of human α-glucosidase complexed with acarbose (PDB ID: 3TOP). α-Amylase was complexed with acarbose (PDBID: 1B2Y), butyrylcholinesterase (hBChE) was complexed with decamethonium (PDBID: 6EP4), monoamine oxide B was complexed with safinamide (PDBID: 2V5Z), and acetylcholinesterase (hAChE) was complexed with donepezil (PDBID: 4EY7). MGL-AutoDockTools (ADT, v1.5.6) was used to add missing hydrogen atoms to all crystal structures while removing the current ligands and water molecules.21
Ligand preparation. The structures of reference inhibitors (donepezil, decamethonium acarbose and safinamide) and the HPLC-identified phytocompounds from flavonoid-rich extracts of B. vulgaris roots (beetroot) were retrieved from the PubChem database (http://www.pubchem.ncbi.nlm.nih.gov/). The compounds were further converted to the pdb chemical format by means of Open Babel. Nonpolar hydrogen molecules were merged with the carbons, while the polar hydrogen charges of the Gasteiger type were assigned to atoms. Furthermore, ligand molecules were converted to the dockable PDBQT format with the help of AutoDock Tools.
Validation of the molecular docking protocol. The extracted cocrystallized ligand from both proteins was aligned with the docked poses of the natural ligands (acarbose and donepezil) with the least binding affinity from the initial docking to validate the docking technology to be used for virtual screening. Using Discovery Studio Visualizer (BIOVIA, 2020), the RMSD was computed.
Molecular docking of phytochemicals with targeted active sites. The methods employed for molecular docking were reported elsewhere.22,23 Using AutoDock Vina in PyRx 0.8, the reference inhibitors and the HPMS-identified compounds were molecularly docked against the five protein targets in an active site-directed manner.24 The ligands were imported for the docking study, and PyRx 0.8's Open Babel25 was used to minimize energy. The optimization algorithm and universal force field (UFF) were utilized as the energy minimization parameter and conjugate gradient descent, respectively. The binding site coordinates of the target enzymes are shown in Table S1, and the molecular interactions were determined using Discovery Studio Visualizer version 16—although additional parameters not listed were set to default values.
Molecular dynamics. The complexes of the top two docked phytochemicals with AChE (4ey7) and α-amylase (1b2y) were further selected for a 100 ns molecular dynamics simulation. The study was conducted using the GROMACS 2019.2 and GROMOS96 43a1 force fields. The protein and ligand topology files were generated using Charmm GUI.26,27 Similar to our earlier work, the simulation's solvation system, periodic boundary conditions, physiological circumstances, system minimization, equilibration in a constant number of atoms, constant pressure, and constant temperature (NPT) were all employed.28–30 The velocity rescales and Parrinello–Rahman barostat were used to maintain the temperature and pressure at 310 K and 1 atm, respectively. A 2 femtosecond time step was used with a Leap-Frog integrator. Each system underwent a 100 ns simulation, with snapshots being taken every 0.1 ns for a total of 1000 frames for each system. From the MD trajectories, the RMSD and RMSF, ROG, SASA and H-bonds were determined.
Binding free energy calculation using MM-GBSA
To determine the binding free energy of the two top docked phytochemicals from the initial docking analysis, the Molecular Mechanics Generalized Born Surface Area (MM-GBSA) method and decomposition analysis using the gmx MMPBSA package were utilized to obtain the binding energies of amino acids within 0.5 nm of the ligand.31,32 The methods used were the same as those published in our previous manuscripts.29,30 The procedures used were the same as those detailed in our earlier articles.29,30
Data analysis
We performed the in vitro experiments three times. For the in vivo analysis, we calculated the mean and standard error of the mean (SEM) from six measurements taken across the groups. The data from this study were then analyzed using one-way analysis of variance (ANOVA). Tukey's post hoc comparison test was conducted using GraphPad Prism version 9, with a significance level set at P < 0.05.
Results
HPLC analysis of the flavonoid-rich extract of B. vulgaris (FREBV) roots indicated the presence of various phytochemicals that are well known to be very valuable in the management of different diseases. These phytochemicals include gallic acid, caffeic acid, rutin, p-coumaric acid, ferulic acid, myricetin, apigenin, isoquercetrin, and quercetrin. Among the identified phytochemicals, caffeic acid exhibited the highest peak (Fig. 1) in comparison to the other phytochemicals.
 |
| Fig. 1 Chromatogram of flavonoid-rich extract of B. vulgaris root extract. | |
The α-amylase inhibitory activity of the flavonoid-rich extract of B. vulgaris (FREBV) fruit (Fig. 2) increased in a dose-dependent manner compared to that of the reference (acarbose). However, acarbose showed better inhibitory activity (IC50 = 27.104 ± 0.270 μg mL−1) than the flavonoid-rich extract of B. vulgaris roots (IC50 = 73.062 ± 0.480 μg mL−1).
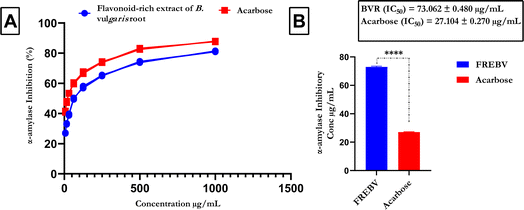 |
| Fig. 2 α-Amylase inhibitory activities of the flavonoid-rich extract of B. vulgaris fruit (A) α-amylase inhibitory activity; (B) IC50 values of the flavonoir-rich extract and standard acarbose. Data are represented as the mean ± SD (n = 3); p < 0.0001 according to t tests. BVR: flavonoid-rich extract of Beta vulgaris fruit; acarbose: standard drug. | |
The α-glucosidase inhibitory assay results showed a dose-dependent increase in FREBV activity (Fig. 3A) compared to that of the reference (acarbose). In contrast, acarbose had a lower IC50 value (IC50 = 17.389 ± 0.436 μg mL−1) than the flavonoid-rich extract of B. vulgaris (FREBV) roots (IC50 = 37.564 ± 0.620 μg mL−1), indicating that acarbose possesses better inhibitory activity than the extract (Fig. 3B).
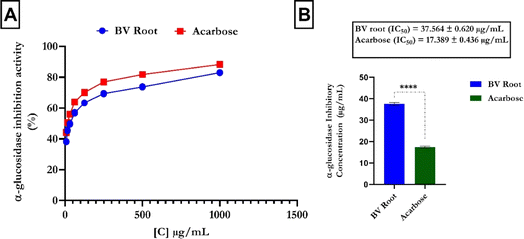 |
| Fig. 3 α-Glucosidase inhibitory activities of the flavonoid-rich extract of B. vulgaris fruit. (A) α-Glucosidase inhibitory activity; (B) IC50. Data are represented as the mean ± SD (n = 3); p < 0.0001 according to t tests. FREBV: flavonoid-rich extract of Beta vulgaris fruit; acarbose: standard drug. | |
Fig. 4 shows that compared with the reference (galanthamine), FREBV significantly increased AchE activity in a concentration-dependent manner. However, galanthamine possesses better inhibitory activity due to its lower IC50 value (IC50 = 27.950 ± 0.122 μg mL−1) than FREBV (IC50 = 723.260 ± 5.466 μg mL−1).
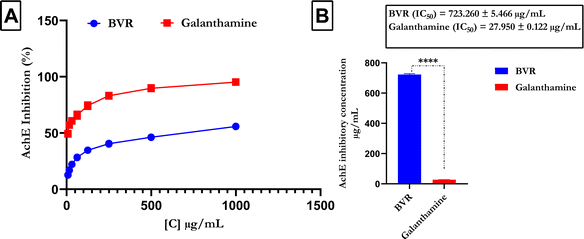 |
| Fig. 4 Acetylcholinesterase activity of the flavonoid-rich extract of B. vulgaris leaf (A) AchE inhibitory activity; (B) IC50 graph of flavonoid-rich extract of B. vulgaris root and standard galanthamine. Data are represented as the mean ± SD (n = 3); p < 0.0001 according to t tests. BVR: flavonoid-rich extract of Beta vulgaris fruit; galanthamine: standard drug. | |
Fig. 5 shows that compared with that of the reference (galanthamine), the FREBV activity significantly increased the activity of BchE in a concentration-dependent manner. In contrast, galanthamine had a lower IC50 value (IC50 = 23.126 ± 0.683 μg mL−1) than the flavonoid-rich extract of B. vulgaris (FREBV) roots (IC50 = 649.112 ± 3.407 μg mL−1), indicating that galanthamine possesses better inhibitory activity than the extract (Fig. 5B).
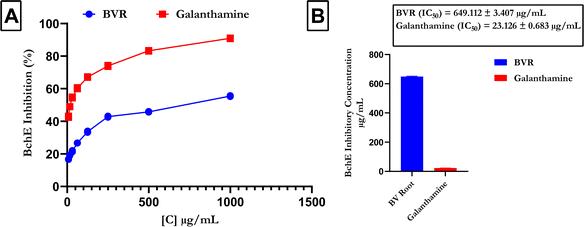 |
| Fig. 5 Butyrylcholinesterase activity of the flavonoid-rich extract of B. vulgaris leaves (A) AchE inhibitory activity; (B) IC50 of flavonoid-rich extract of B. vulgaris root and standard galanthamine. Data are represented as the mean ± SD (n = 3); p < 0.0001 according to t tests. FREBV: flavonoid-rich extract of Beta vulgaris fruit; galanthamine: standard drug. | |
Compared with those in the groups treated with FREBV, the amount of monoamine oxidase (MAO) in the group not treated with FREBV significantly (p < 0.05) increased in a dose-dependent manner. Among the treated groups, those with a more significant reduction were those treated with 250 μg mL−1, 500 μg mL−1, and 1000 μg mL−1, which were the lowest and most effective, according to the results (Fig. 6).
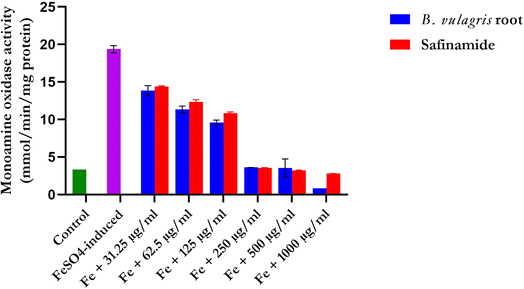 |
| Fig. 6 Impact of varying concentrations of flavonoid-rich extracts of Beta vulgaris roots on monoamine oxidase (MAO) activity in ex vivo brain samples. Compared to the group induced solely with FeSO4, a notable reduction in MAO activity was observed in the range of flavonoid-rich extracts of Beta vulgaris fruit. Safinamide was used as a standard drug. Data are represented as the mean ± SD (n = 3); p < 0.0001 according to ANOVA. | |
Molecular docking results
Molecular docking of HPLC-identified compounds from Beta vulgaris root against target proteins. Table 1 displays the binding affinities obtained from the docking study of the compounds discovered by HPLC against the five protein targets. The top-two scoring phytochemicals from the docking analysis for each enzyme were chosen for interactive analysis based on their catalytic site interaction, minimal binding energies, and binding poses in the binding sites. The binding energies for the phytochemicals were ranked, and the binding energies of the top-two compounds docked to the five targets were close to those of the reference inhibitors (Table 1). Donepezil and acarbose, two co-crystallized reference compounds, were docked into the binding sites of the co-crystallized proteins with binding energies of −12.2 and −12.5 kcal mol−1 according to the validation of the docking procedure (Fig. S1). The two-top scoring phytochemicals for the five top target proteins were quercetrin and apigenin, with binding energies of −9.2 and −9.1 kcal mol−1, α-glucosidase (3TOP) respectively; MAO (2V5Z), with binding energies of apigenin (−9.4 kcal mol−1) and myricetin (−9.3 kcal mol−1); α-amylase (1B2Y), with binding energies of apigenin (−9.0 kcal mol−1) and myricetin (−9.1 kcal mol−1); BChE (6EP4), with binding energies of rutin (−10.6 kcal mol−1) and iso-quercetrin (−10.6 kcal mol−1); and AChE (4EY7), with binding energies of apigenin (−10.2 kcal mol−1) and myricetin (−10.1 kcal mol−1) (Table 2). Among the top scoring compounds, apigenin demonstrated the greatest tendency to dock to four of the five protein targets, while myricetin demonstrated the same tendency to dock to three of the protein targets.
Table 1 The names and structures of the top scoring phytochemicals from flavonoid-rich extracts of B. vulgaris roots according to docking analysis of the five target proteins
S. No. |
Name |
Structure |
1 |
Apigenin |
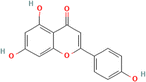 |
2 |
Myricetin |
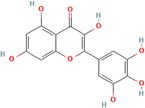 |
3 |
Quercitrin |
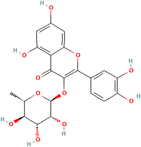 |
4 |
Isoquercetrin |
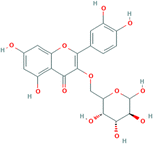 |
5 |
Rutin |
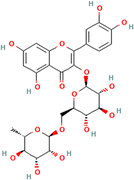 |
Table 2 Binding energies of HPLC-identified compounds from flavonoid-rich extracts of B. vulgaris roots against target proteinsa
Ligand |
Binding affinity |
3TOP |
2V5Z |
1B2Y |
6EP4 |
4EY7 |
3TOP: α-glucosidase; 2V5Z: monoamine oxidase (MAO); 1B2Y: α-amylase; 6EP4:BChE:4EY7:AChE. |
Safinamide (E = 122.72) |
|
−9.8 |
|
|
|
Decamethonium (DME) (E = 261.56) |
|
|
|
−5.4 |
−6.8 |
Donepezil (ED) (E = 306.68) |
|
|
|
−9.6 |
−12.2 |
Acarbose (E = 372.76) |
−14.2 |
|
−12.5 |
|
|
Quercetrin (E = 588.43) |
−9.2 |
−6.8 |
−8.5 |
−9.8 |
−8.1 |
Apigenin (E = 233.26) |
−9.1 |
−9.4 |
−9 |
−9.3 |
−10.2 |
Myricetin (E = 388.24) |
−8.8 |
−9.3 |
−9.1 |
−9.5 |
−10.1 |
Rutin (E = 751.29) |
−8.8 |
−7.5 |
−8.9 |
−10.6 |
−9.4 |
Iso-quercetrin (E = 610.55) |
−8.2 |
−7.1 |
−8.5 |
−10.6 |
−9.2 |
p-Coumaric_acid (E = 90.82) |
−7.3 |
−7 |
−6.2 |
−6.6 |
−7.6 |
Ferulic_acid (E = 171.00) |
−7 |
−7.3 |
−6.5 |
−6.8 |
−7.8 |
Caffeic_acid (E = 98.70) |
−6.9 |
−7.3 |
−6.6 |
−6.8 |
−7.7 |
Gallic_acid (E = 77.81) |
−6.4 |
−6.3 |
−6.2 |
−6 |
−6.6 |
Amino acid interactions of the top two docked HPLC-identified phytochemicals from flavonoid-rich extracts of B. vulgaris roots and reference compounds with the five protein targets. Table S2 shows the interactions between the reference molecule and two top-docked HPLC-identified phytochemicals with target protein binding site residues. With the exception of a few H-bonds below 3.40 Å, most of the interactions between the ligand groups and the enzyme residues were hydrophobic. According to the validation study, donepezil was stretched in the long, narrow, hydrophobic gorge of AChE (4ey7) in a binding conformation akin to that of the natural ligand. There was only one hydrogen bond between Phe295 and the carbonyl oxygen of the indenone ring. Two Pi-alkyl interactions were established between Tyr337 and Tyr341 of AChE (4ey7) and the donepezil piperidine ring. Trp86 and His447 interact with the 1-benzyl unit of donepezil via aromatic pi–pi stacking. It was discovered that there are two pi-sigma bonds between the 5-methoxy unit of inden-1-one and the piperidine rings Trp286 and Phe338. Myricetin and apigenin, the phytochemical most closely related to AChE (4ey7), were docked in a similar fashion in the active site of the gorge, where they formed several hydrogen bonds and hydrophobic contacts, as presented in Table S2 and Fig. 7. In the case of BChE (6ep4), the best conformation of decamethonium was docked deeply into the active site gorge and aligned similarly to the co-crystallized inhibitor. While the reference compound did not form any hydrogen bonds with BChE (6ep4), the top docked phytochemicals (rutin and myricetin) formed several hydrogen bonds with the catalytic residues and interacted with all the residues that formed bonds with decamethonium (Fig. 8). Although the orientation of acarbose in the binding site of α-amylase (1B2Y) was stretched into the five subsites, the top-scoring phytocompounds (apigenin and myricetin) docked into the −3 and −1 subsets of α-amylase (1B2Y) (Fig. 9). Both apigenin and myricetin formed hydrogen bonds and hydrophobic contacts with the catalytic residues at the hydrophobic gate of α-amylase, which is composed of residues Trp-59, Tyr62, and His299 and other catalytic residues, such as Asp197, His305, Glu233, Arg197, and Ala198. The top-scoring phytochemicals (apigenin and myricetin) docked into the active sites of α-glucosidase (3TOP) in a similar fashion to that of the reference compound acarbose, and they interacted with the catalytic residues of acarbose (Fig. 10). While safinamide, the reference compound for monoamine oxidase (MAO) (2V5Z), interacted with catalytic residues with just one hydrogen bond with Gln206 and several hydrophobic interactions, the two-top scoring phytochemicals formed more hydrogen bonds with the catalytic residues in addition to several hydrophobic interactions (Fig. 11).
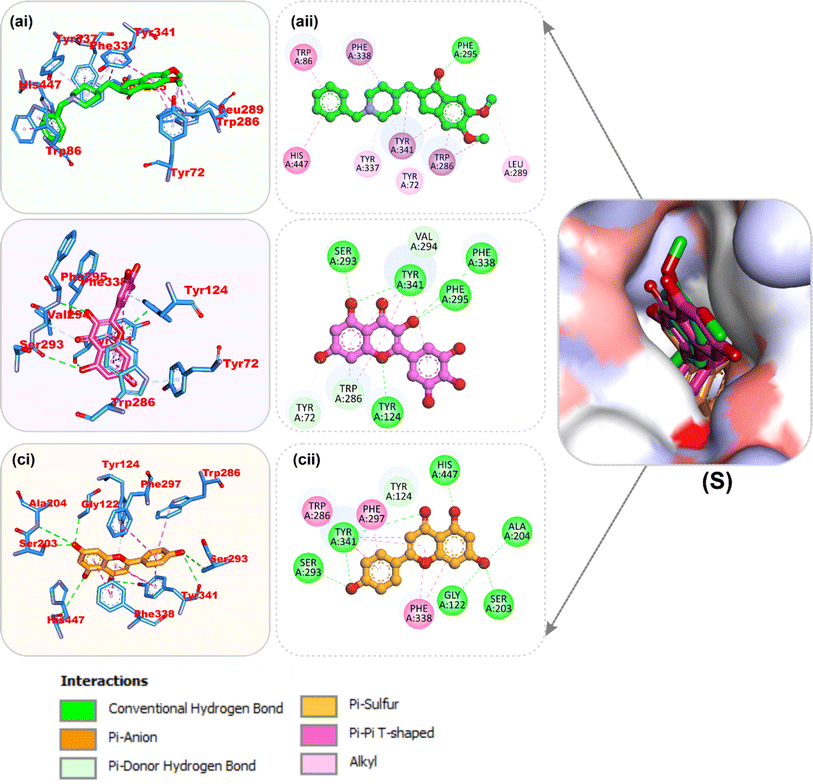 |
| Fig. 7 Top docked phytochemicals and reference inhibitor (donepezil) from the docking analysis of HPLC-identified phytochemicals from flavonoid-rich extracts of B. vulgaris roots that interact with amino acids in the active site of AChE (4EY7). The ligands are displayed as colored sticks: green: donepezil, pink: apigenin, and yellow: myricetin. (i) 3D and (ii) 2D interactions. | |
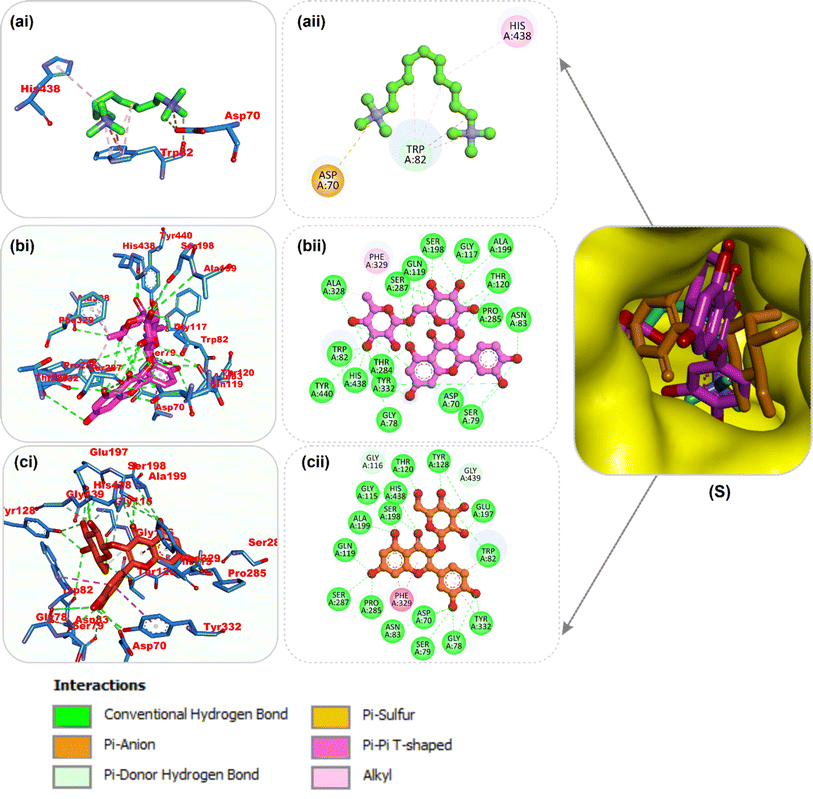 |
| Fig. 8 Top docked phytochemicals and reference inhibitor (decamethonium) from the docking analysis of HPLC-identified phytochemicals from flavonoid-rich extracts of B. vulgaris roots that interact with amino acids in the active site of BChE (6EP4). (S) Surface representation of ligands in binding sites of target proteins. The ligands are displayed as sticks (a) decamethonium (b) rutin (c) isoquercetrin. (i) 3D and (ii) 2D interactions. | |
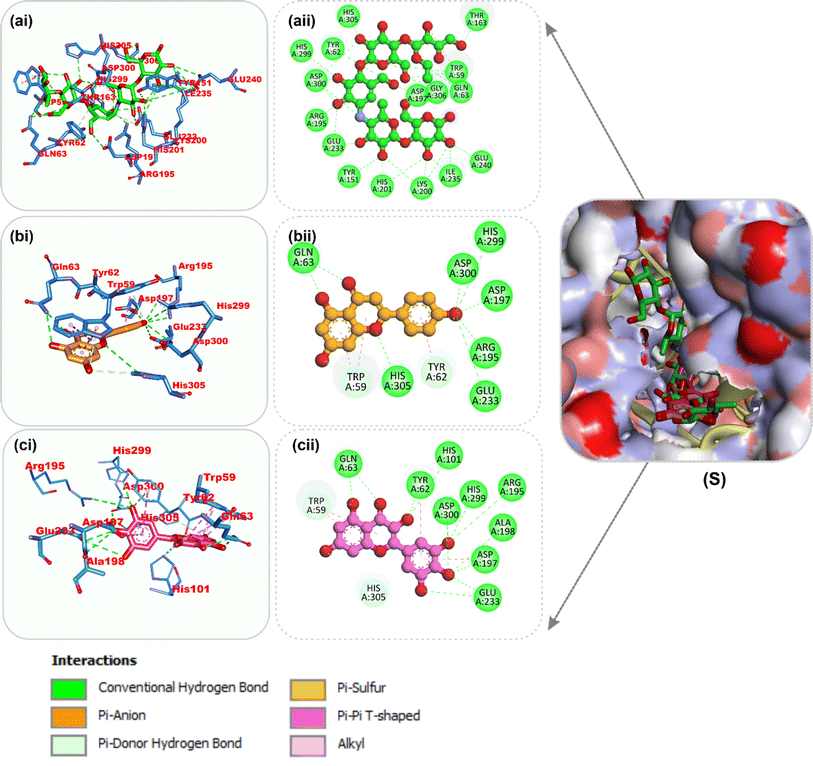 |
| Fig. 9 Top docked phytochemicals and reference inhibitor (acarbose) from the docking analysis of HPLC-identified phytochemicals from flavonoid-rich extracts of B. vulgaris roots that interact with amino acids in the active site of α-amylase (1B2Y). (S) Surface representation of ligands in binding sites of target proteins. The ligands are displayed as sticks: (a) acarbose, (b) myricetin, and (c) apigenin. (i) 3D and (ii) 2D interactions. | |
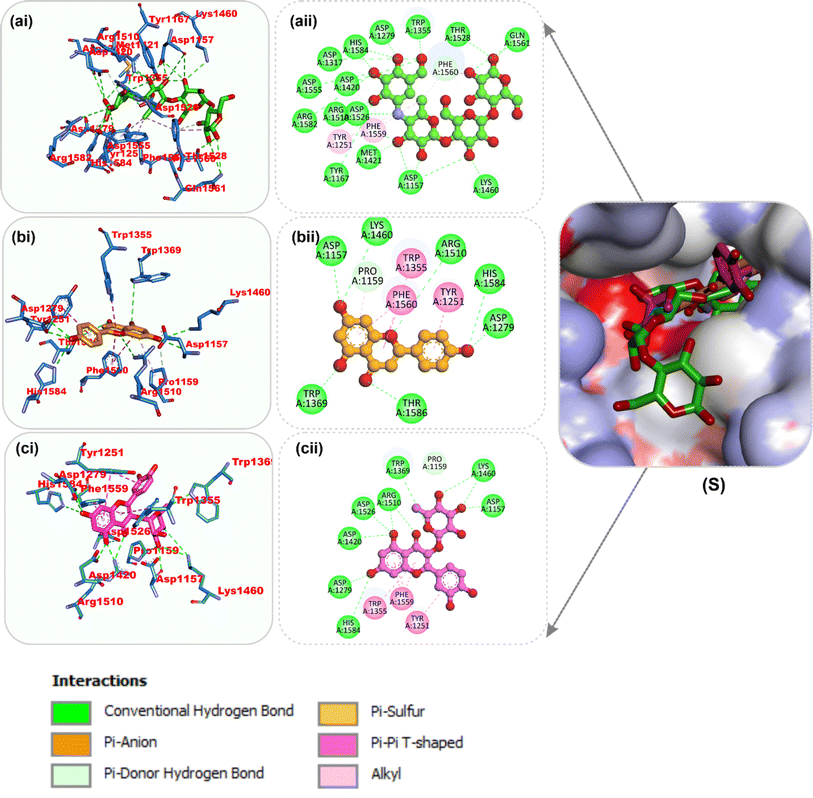 |
| Fig. 10 Top docked phytochemicals and reference inhibitor (acarbose) from the docking analysis of HPLC-identified phytochemicals from flavonoid-rich extracts of B. vulgaris roots that interact with amino acids in the active site of α-glucosidase (3TOP). (S) Surface representation of ligands in binding sites of target proteins. The ligands are displayed as sticks (a) acarbose (b) apigenin (c) quercetrin (i) 3D and (ii) 2D interactions. | |
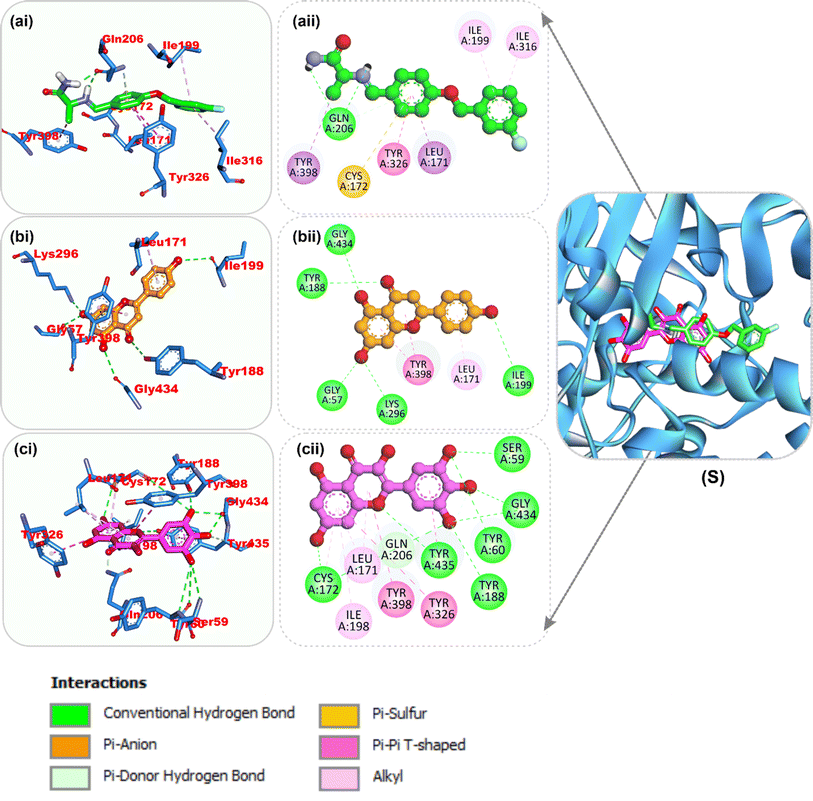 |
| Fig. 11 Top docked phytochemicals and reference inhibitor (safinamide) from the docking analysis of HPLC-identified phytochemicals from flavonoid-rich extracts of B. vulgaris roots that interact with amino acids in the active site of monoamine oxidase (MAO) (2V5Z). (S) Surface representation of ligands in binding sites of target proteins. The ligands are displayed as sticks (a) safinamide (b) apigenin (c) myricetin(I) 3D and (ii) 2D interactions. | |
Molecular dynamics (MD) simulation
Using Tk console scripts, the stabilities of the complexes containing representative proteins and the reference inhibitors (donepezil and acarbose) were investigated during the molecular phase. The MD trajectories obtained throughout the simulation were examined using the RMSD, RMSF, RoG, SASA, and quantity of H-bonds. The measured parameters' descriptive statistics are shown in Table S3, and the complex spectrum maps are shown in Fig. 12–16. All of the RMSD graphs for the AChE (4EY7) and α-amylase (1B2Y) complexes showed equilibrium before 10 ns, and the system showed very little fluctuation for the remainder of the run. According to the mean RMSD values, the greatest fluctuations were observed for 1.70 ± 0.22 and AChE_apigenin. The three systems for the AChE (4EY7) systems showed relatively similar mean RMSF values. Additionally, all of the α-amylase complex systems showed similar mean RMSF values (Fig. 13). The RoG plots demonstrate that during the course of the simulation, the AChE and α-amylase complexes were also equilibrated at approximately 10 ns with minimal fluctuation (Fig. 14). The mean RoG values of the reference compounds and those of the apigenin and myricetin systems were similar. The SASA plots of the AChE and α-amylase complexes demonstrated that there was very little volatility over the simulation period. Additionally, this finding supported the extremely close mean SASA values (Fig. 15). Throughout the simulations, only a few changes in the average number of H-bonds were noticed in the entire molecule. The ligand–bound complexes presented a close number of hydrogen bonds (Fig. 16).
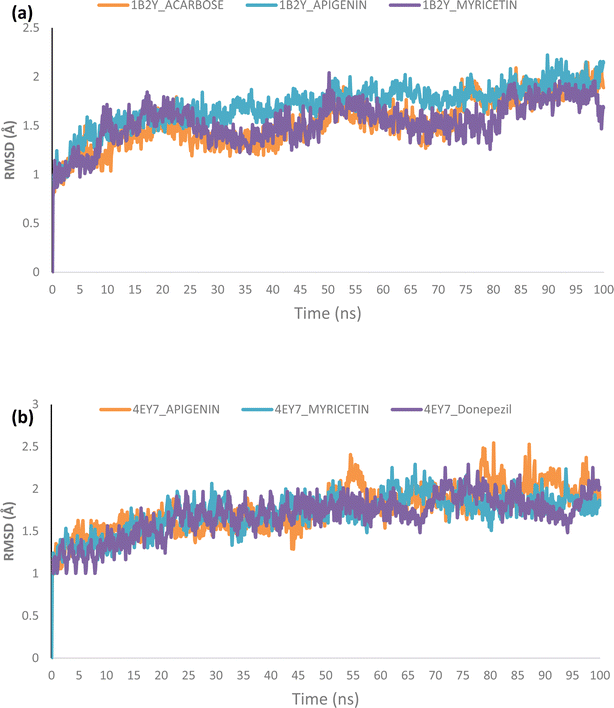 |
| Fig. 12 Backbone-root mean square deviation (RMSD) plots of the MD simulations of the top docked HPLC-identified phytochemicals and reference compounds complexed to (a) human α-amylase and (b) human acetylcholinesterase. | |
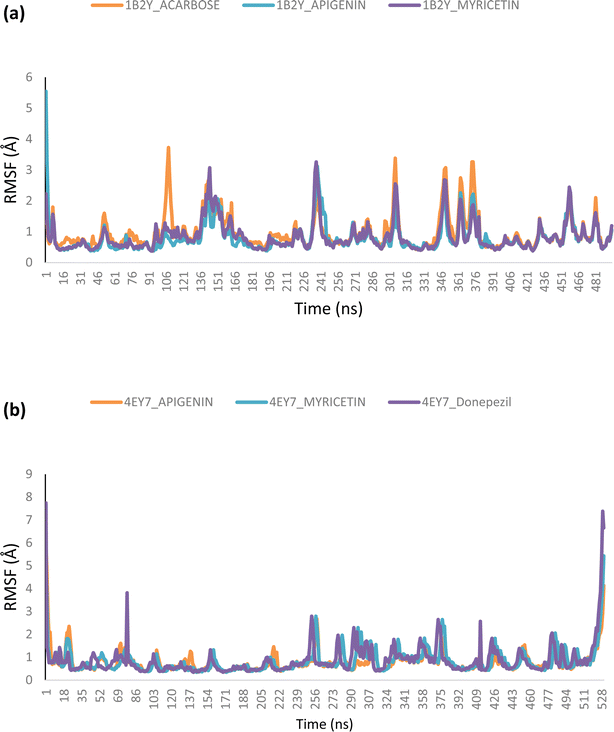 |
| Fig. 13 Per residue root mean square fluctuation (RMSF) plots of the MD simulations of the top docked HPLC-identified phytochemicals and reference compounds complexed to (a) human α-amylase and (b) human acetylcholinesterase. | |
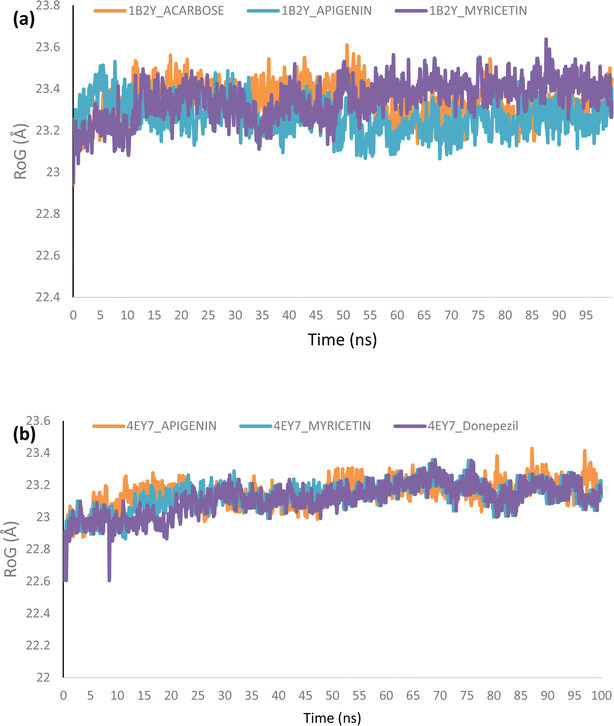 |
| Fig. 14 Radius of gyration (RoG) plots of MD simulations of top docked HPLC-identified phytochemicals and reference compounds complexed to (a) human α-amylase and (b) human acetylcholinesterase. | |
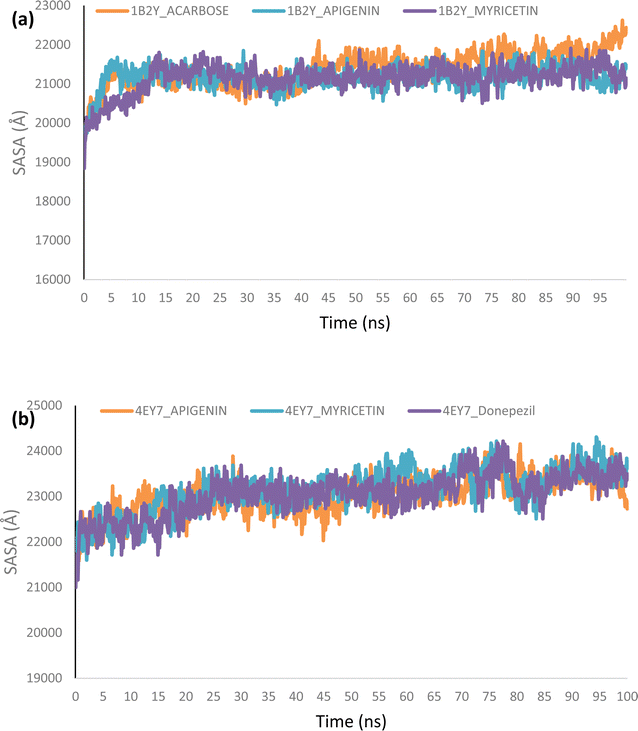 |
| Fig. 15 Surface accessible surface area (SASA) plots of MD simulations of top docked HPLC-identified phytochemicals and reference compounds complexed to (a) human α-amylase and (b) human acetylcholinesterase. | |
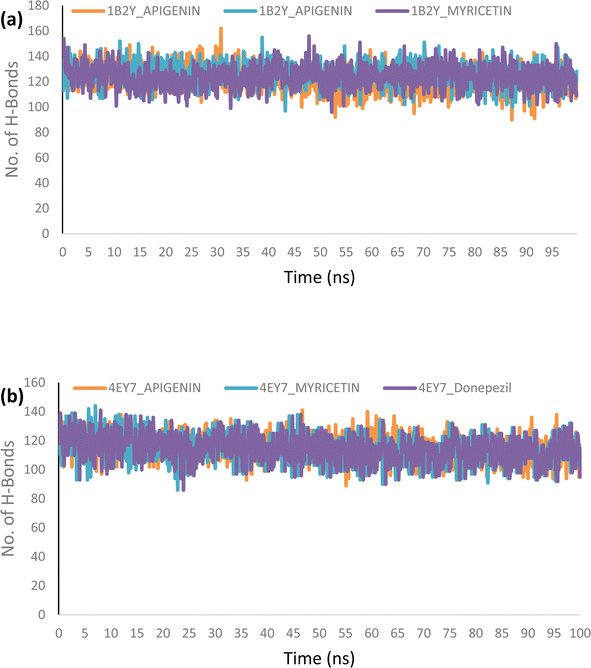 |
| Fig. 16 The top docked HPLC-identified phytochemicals and reference compounds complexed to (a) human α-amylase and (b) human acetylcholinesterase. | |
Molecular mechanics generalized born surface area (MMGBSA) analysis
Binding free energy estimates provide comprehensive information on the binding mechanisms of the best docked compounds throughout the early stages of drug discovery and development (Kollman et al., 2000). Using the MMGBSA method, the binding free energy of the two top scoring phytochemicals to the proteins AChE and α-amylase was ascertained. According to the calculated binding free energy, myricetin had the greatest binding free energy to AChE, whereas apigenin had the highest binding free energy to α-amylase. Interestingly, the binding free energy of both phytocompounds was greater than that of the reference compounds. The various components that make up the total binding free energy are presented in Table S4. The contributing amino acids that make up the total binding energy were analyzed using decomposition analysis and are presented in Fig. S2 and S3. It was observed that the interacting residues during the static docking were primarily involved in the contribution to the total binding free energy.
Discussion
Medicinal plants have been used for their curative properties. They possess bioactive chemicals that may have several health advantages, including anti-inflammatory, antioxidant, and antibacterial properties. These plants are often used in traditional medicine and are now undergoing extensive research for their potential applications in contemporary healthcare. Beetroot, a subterranean vegetable, is renowned for its remarkable therapeutic qualities.22 It has a high concentration of vital elements such as vitamins, minerals, and antioxidants. Beetroot is highly esteemed for its capacity to reduce blood pressure, boost physical performance, and promote cardiovascular well-being. Moreover, beetroot contains nitrates that can enhance cerebral blood flow, possibly enhancing cognitive function. The inclusion of this substance in a nutritious diet is very beneficial due to its ability to reduce inflammation and eliminate toxins, thereby promoting general health and wellness. Integrating beetroot into dishes or drinking beetroot juice may serve as a straightforward method because of its therapeutic advantages.33
Elevated blood glucose levels in diabetes mellitus patients have the potential to impair blood vessel and neuron function, affecting the well-being of the brain. Insulin resistance, a characteristic feature of diabetes, may influence brain function contributing to the development of Alzheimer's disease. Moreover, diseases associated with diabetes, such as obesity and hypertension, might worsen cognitive deterioration.34 Alzheimer's disease is characterized by abnormal protein accumulation in the brain, which leads to nerve cell deterioration and cognitive decline. Genetic factors, lifestyle decisions, and environmental influences are also contributing factors to both illnesses. Implementing lifestyle alterations, medication, and consistent monitoring might mitigate the likelihood of acquiring Alzheimer's disease, underscoring the need for comprehensive health strategies to avoid neurodegenerative conditions.35
Several plants rich in flavonoids, such as gallic acid, caffeic acid, rutin, p-coumaric acid, ferulic acid, myricetin, apigenin, iso-quercetrin, and quercetrin, have shown great promise in treating diabetes and Alzheimer's disease. These phytochemicals possess antioxidant capabilities and are essential for countering oxidative stress, a common component in both illnesses.36 Gallic acid and caffeic acid exhibit antidiabetic properties by facilitating glucose metabolism and enhancing insulin sensitivity.37 Rutin and quercetrin have shown potential in the management of Alzheimer's disease by decreasing neuroinflammation and providing protection against neurodegeneration.38 Moreover, p-coumaric acid, ferulic acid, myricetin, apigenin, and isoquercetrin have shown neuroprotective effects against Alzheimer's disease.39 These bioactive chemicals have the ability to inhibit essential enzymes such as acetylcholinesterase, which is associated with a decline in cognitive function in Alzheimer's disease patients.40 The synergistic effect of these phytochemicals not only addresses the metabolic components of diabetes but also focuses on the neuroprotective processes essential for controlling Alzheimer's disease. The wide range of phytochemicals found in plant extracts high in flavonoids offers a great opportunity for the development of natural treatments that might help patients suffering from both diabetes and Alzheimer's disease.41
FREBV contains many flavonoids, such as gallic acid, caffeic acid, rutin, p-coumaric acid, ferulic acid, myricetin, apigenin, isoquercetrin, and quercetrin, and effectively inhibits the activity of two important carbohydrate enzymes, α-amylase and α-glucosidase. These enzymes play a critical role in carbohydrate metabolism and glucose regulation.42 The potent inhibitory effect of FREBV may be attributed to the combined effects of its several phytochemical ingredients. Gallic acid, caffeic acid, and ferulic acid have strong inhibitory effects on α-amylase and α-glucosidase enzymes.43 The inhibition of the enzymes slows the breakdown and absorption of carbohydrates. Flavonoids such as myricetin, rutin, and quercetrin improve the inhibitory function by changing how quickly enzyme reactions occur and by attaching to the active parts of these enzymes. Moreover, flavonoid extracts possess antioxidant characteristics that are essential for the management of metabolic illnesses such as diabetes.44 Apigenin and isoquercetrin have shown the capacity to eliminate free radicals, decrease oxidative stress, and enhance insulin sensitivity, all of which are crucial for preventing and managing diabetes.45 The FREBV, which is high in flavonoids, has great potential as a natural treatment for metabolic diseases such as diabetes. It has the ability to block α-amylase and α-glucosidase and provides antioxidant benefits. These properties make it a good option for developing multitargeted treatments to address diabetes and its consequences. Our findings correlates with a previous report by46 who documented that rutin was found to be a potent α-glucosidase inhibitor, which was isolated from a vegetable, Coccinia grandis.
Flavonoids, a group of plantchemicals, have shown significant promise in regulating the levels of monoamine oxidase (MAO), which is an essential enzyme involved in the metabolism of neurotransmitters.47 FREBV, which contains flavonoids, such as gallic acid, caffeic acid, rutin, p-coumaric acid, ferulic acid, myricetin, apigenin, iso-quercetrin, and quercetrin, may be very effective at lowering MAO levels. This can influence the levels of neurotransmitters and potentially provide therapeutic advantages.48 Gallic acid, renowned for its antioxidant characteristics, has been associated with neuroprotective benefits and has the potential to contribute to the suppression of MAO.49 Caffeic acid, a very effective antioxidant, has shown potential for regulating enzyme function. These three chemicals—rutin, p-coumaric acid, and ferulic acid—are often found in different plants. These compounds have many biological functions and might work together to help control MAO. Research has emphasized myricetin, a flavonoid that has shown inhibitory effects on MAO, indicating its ability to decrease MAO levels.50 Apigenin, isoquercetrin, and quercetrin are well-recognized flavonoids that have shown neuroprotective and anti-inflammatory properties.51 These flavonoids have the potential to increase the capacity of the extract to regulate MAO concentrations. This flavonoid-rich extract may help to maintain neurotransmitter balance, specifically dopamine balance, by targeting MAO. This is particularly important in conditions such as Parkinson's disease. The ability of chemicals to inhibit MAO may result in elevated dopamine levels, thereby enhancing motor performance and neural well-being.52 Additionally, the large number of flavonoids in FREBV extract suggests a way to target many aspects of MAO regulation. This could make the extract more effective while reducing the negative effects normally associated with MAO inhibitors.
Furthermore, HPLC-identified phytochemicals were docked against human α-amylase, human α-glucosidase, acetylcholinesterase, butyrylcholinesterase, and monoamine oxidase using both molecular docking and dynamic simulation protocols. Quercetrin, apigenin, rutin, iso-quercetrin, and myricetin were shown to be the top-docked substances against the five protein targets. Molecular dynamics simulations at 100 ns were utilized to examine the conformational stability of the bound complexes. All of the RMSD graphs for the 4EY7 and 1B2Y complexes showed equilibrium before 10 ns, and the system showed very little fluctuation for the remainder of the run. According to the mean RMSD values, the greatest fluctuations were observed for 1.70 ± 0.22 and 4EY7_apigenin. Additionally, all of the 1B2Y complex systems showed similar mean RMSF values The RoG plots demonstrate that during the course of the simulation, the 4EY7 and 1B2Y complexes were also equilibrated at approximately 10 ns with minimal fluctuation. The mean RoG values of the reference compounds and those of the apigenin and myricetin systems were similar. The SASA plots of the 4EY7 and 1B2Y complexes demonstrated that there was very little volatility over the simulation period. Additionally, this finding supported the extremely close mean SASA values. Throughout the simulations, only a few changes in the average number of H-bonds were noticed in the entire molecule. The ligand–bound complexes presented a close number of hydrogen bonds.
The lead bioactive flavonoids did not cause the protein's structural conformation to change; instead, a more compact structure was created, as seen by the flexibility of the amino acid residues of the protein targets that were accessible using the RMSF plots.53,54 When comparing the lead bioactive flavonoid–bound complexes to the unbound protein, the close mean values for the different thermodynamic parameters show that the structural integrity of the enzymes was not compromised by bioactive flavonoid binding,53,55 allowing for additional research on the complexes.
In the early phases of drug design and development, the ΔGbind calculations offer detailed information regarding the binding mechanisms of the best docked compounds.56 The binding free energy of the two top phytochemicals docked to the 4ey7 and 1B2Y proteins was determined using the MMGBSA technique.
Conclusion
Overall, this study provides valuable insights into the dual therapeutic ability of flavonoid-rich extracts of B. vulgaris roots. The FREBV extract showed a promising ability to inhibit key metabolizing enzymes involved in glucose metabolism and neurotransmission. The inhibitory effects are attributed to the bioactive compounds identified HPLC. Additionally, computational screening of these HPLC-identified compounds, namely, quercetrin, iso-quercetrin, rutin, apigenin, and myricetin, demonstrated promising interactions with specific protein targets implicated in T2D and AD.
Data availability
Data are available on reasonable request from the corresponding author.
Conflicts of interest
Not applicable.
Author contributions
OAO conceptualized and designed the study; GAG, MI, EHE, and OAO wrote the first draft; DAE, OAA, and FEO, performed the experiment; ABO, OAO, MI, TEO, DEB, FEO, GAG, analyzed and interpreted the data; OAO supervised the experiment. EHE, DAE, GAG, OAA, FEO, TEO, DEB, ABO, OAO, and OSA review the final draft of the manuscript. All authors approved the final version of the manuscript.
References
- E. Richter, T. Geetha, D. Burnett, T. L. Broderick and J. R. Babu, Int. J. Mol. Sci., 2023, 24, 4643 CrossRef CAS PubMed.
- P. Aschner, S. Karuranga, S. James, D. Simmons, A. Basit, J. E. Shaw, S. H. Wild, K. Ogurtsova and P. Saeedi, Diabetes Res. Clin. Pract., 2021, 172, 108630 CrossRef PubMed.
- H. Xu, X. Du, J. Xu, Y. Zhang, Y. Tian, G. Liu, X. Wang, M. Ma, W. Du, Y. Liu, L. Dai, W. Huang, N. Tong, Y. Wei and X. Fu, PLoS Biol., 2020, 18, e3000603 CrossRef CAS PubMed.
- E. A. Nyenwe, T. W. Jerkins, G. E. Umpierrez and A. E. Kitabchi, Metabolism, 2011, 60, 1 CrossRef CAS PubMed.
- GBD 2019 Dementia Forecasting Collaborators, The Lancet Public Health, DOI:10.1016/s2468-2667(21)00249-8.
- M. A. Akanji, D. E. Rotimi, T. C. Elebiyo, O. J. Awakan and O. S. Adeyemi, Oxid. Med. Cell. Longevity, 2021, 2021, 1–14 CrossRef PubMed.
- M. Penumala, R. B. Zinka, J. B. Shaik, S. K. R. Mallepalli, R. Vadde and D. G. Amooru, BMC Compl. Alternative Med., 2018, 18, 77 CrossRef PubMed.
- S. Rehman, U. Ali Ashfaq, M. Sufyan, I. Shahid, B. Ijaz and M. Hussain, PLoS One, 2022, 17, e0264074 CrossRef CAS PubMed.
- D. Székely, B. Illés, M. Stéger-Máté and J. Monspart-Sényi, Acta Univ. Sapientiae, Aliment., 2016, 9, 60–68 Search PubMed.
- R. Domínguez, E. Cuenca, J. Maté-Muñoz, P. García-Fernández, N. Serra-Paya, M. Estevan, P. Herreros and M. Garnacho-Castaño, Nutrients, 2017, 9, 43 CrossRef PubMed.
- A. Paulauskienė, D. Šileikienė, R. Karklelienė, Ž. Tarasevičienė and L. Česonienė, Sustainability, 2023, 15, 7102 CrossRef.
- J. Szymański, D. Szwajgier and E. Baranowska-Wójcik, Appl. Sci., 2023, 13, 1044 CrossRef.
- H. S. El-Beltagi, M. M. El-Mogy, A. Parmar, A. T. Mansour, T. A. Shalaby and M. R. Ali, Antioxidants, 2022, 11, 906 CrossRef CAS PubMed.
- S. A. Ashraf, A. E. O. Elkhalifa, K. Mehmood, M. Adnan, M. A. Khan, N. E. Eltoum, A. Krishnan and M. S. Baig, Molecules, 2021, 26, 5957 CrossRef CAS PubMed.
- F. Saad, T. M. Al-Shaikh, F. Zouidi, M. A. Taher, S. Abdelkader Saidi and K. Hamden, J. Food Process. Preserv., 2023, 2023, 1–11 CrossRef.
- J. A. Araujo-León, Z. Cantillo-Ciau, D. V. Ruiz-Ciau and T. I. Coral-Martínez, Rev. Bras. Farmacogn., 2019, 29, 171–176 CrossRef.
- O. A. Ojo, J. C. Amanze, A. I. Oni, S. Grant, M. Iyobhebhe, T. C. Elebiyo, D. Rotimi, N. T. Asogwa, B. E. Oyinloye, B. O. Ajiboye and A. B. Ojo, Sci. Rep., 2022, 12, 2919 CrossRef CAS PubMed.
- E. Bursal, A. Aras, Ö. Kılıç, P. Taslimi, A. C. Gören and İ. Gülçin, J. Food Biochem., 2019, 43, e12776 CrossRef PubMed.
- O. L. Erukainure, C. I. Chukwuma, M. G. Matsabisa, V. F. Salau, N. A. Koorbanally and M. S. Islam, J. Ethnopharmacol., 2020, 248, 112358 CrossRef CAS PubMed.
- B. O. Ajiboye, M. C. Akalabu, O. A. Ojo, O. B. Afolabi, M. A. Okesola, I. Olayide and B. E. Oyinloye, J. Food Biochem., 2018, 42, e12643 CrossRef.
- G. M. Morris, R. Huey, W. Lindstrom, M. F. Sanner, R. K. Belew, D. S. Goodsell and A. J. Olson, J. Comput. Chem., 2009, 30, 2785–2791 CrossRef CAS PubMed.
- O. A. Ojo, A. D. Ogunlakin, M. Iyobhebhe, C. B. Olowosoke, O. A. Taiwo, A. Akinola, D. Fadiora, A. I. Odugbemi, G. A. Gyebi, C. O. Nwonuma, A. B. Ojo and O. O. Ojo, Inform. Med. Unlocked, 2022, 35, 101137 CrossRef.
- B. J. Okoli, W. A. Eltayb, G. A. Gyebi, A. R. Ghanam, Z. Ladan, J. C. Oguegbulu and M. Abdalla, Appl. Sci., 2022, 12, 7500 CrossRef CAS.
- O. Trott and A. J. Olson, J. Comput. Chem., 2009, 31, NA Search PubMed.
- N. M. O’Boyle, M. Banck, C. A. James, C. Morley, T. Vandermeersch and G. R. Hutchison, J. Cheminf., 2011, 3, 33 Search PubMed.
- J. Lee, X. Cheng, J. M. Swails, M. S. Yeom, P. K. Eastman, J. A. Lemkul, S. Wei, J. Buckner, J. C. Jeong, Y. Qi, S. Jo, V. S. Pande, D. A. Case, C. L. Brooks, A. D. MacKerell, J. B. Klauda and W. Im, J. Chem. Theory Comput., 2015, 12, 405–413 CrossRef PubMed.
- J. Lee, M. Hitzenberger, M. Rieger, N. R. Kern, M. Zacharias and W. Im, J. Chem. Phys., 2020, 153(3), 035103 CrossRef CAS PubMed.
- O. M. Ogunyemi, G. A. Gyebi, I. M. Ibrahim, A. M. Esan, C. O. Olaiya, M. M. Soliman and G. E.-S. Batiha, Mol. Diversity, 2023, 27(1), 1–25 CrossRef CAS PubMed.
- G. A. Gyebi, O. M. Ogunyemi, I. M. Ibrahim, S. O. Afolabi and J. O. Adebayo, Comput. Biol. Med., 2021, 134, 104406 CrossRef CAS PubMed.
- O. M. Ogunyemi, G. A. Gyebi, I. M. Ibrahim, C. O. Olaiya, J. O. Ocheje, M. M. Fabusiwa and J. O. Adebayo, RSC Adv., 2021, 11, 33380–33398 RSC.
- M. S. Valdés-Tresanco, M. E. Valdés-Tresanco, P. A. Valiente and E. Moreno, J. Chem. Theory Comput., 2021, 17, 6281–6291 CrossRef PubMed.
- B. R. Miller, T. D. McGee, J. M. Swails, N. Homeyer, H. Gohlke and A. E. Roitberg, J. Chem. Theory Comput., 2012, 8, 3314–3321 CrossRef CAS PubMed.
- O. Adeleke Ojo, A. Oluyemi Agboola, O. Bukunmi Ogunro, M. Iyobhebhe, T. Christiana Elebiyo, D. Rotimi, J. Folashade Ayeni, A. Busola Ojo, A. Isaiah Odugbemi, S. Egieyeh and O. M. Oluba, Heliyon, 2023, 9, e17700 CrossRef PubMed.
- A. Vinuesa, C. Pomilio, A. Gregosa, M. Bentivegna, J. Presa, M. Bellotto, F. Saravia and J. Beauquis, Front. Neurosci., 2021, 15, 653651 CrossRef PubMed.
- M. T. Heneka, M. J. Carson, J. El Khoury, G. E. Landreth, F. Brosseron, D. L. Feinstein, A. H. Jacobs, T. Wyss-Coray, J. Vitorica, R. M. Ransohoff, K. Herrup, S. A. Frautschy, B. Finsen, G. C. Brown, A. Verkhratsky, K. Yamanaka, J. Koistinaho, E. Latz, A. Halle and G. C. Petzold, Lancet Neurol., 2015, 14, 388–405 CrossRef CAS PubMed.
- S. Chikara, L. D. Nagaprashantha, J. Singhal, D. Horne, S. Awasthi and S. S. Singhal, Cancer Lett., 2018, 413, 122–134 CrossRef CAS PubMed.
- Y. Xu, G. Tang, C. Zhang, N. Wang and Y. Feng, Molecules, 2021, 26(23), 7115 CrossRef CAS PubMed.
- B. Salehi, A. Venditti, M. Sharifi-Rad, D. Kręgiel, J. Sharifi-Rad, A. Durazzo, M. Lucarini, A. Santini, E. Souto, E. Novellino, H. Antolak, E. Azzini, W. Setzer and N. Martins, Int. J. Mol. Sci., 2019, 20, 1305 CrossRef CAS PubMed.
- A. Rojas-García, Á. Fernández-Ochoa, M. Cádiz-Gurrea, D. Arráez-Román and A. Segura-Carretero, Nutrients, 2023, 15, 449 CrossRef PubMed.
- M. Saxena and R. Dubey, Curr. Top. Med. Chem., 2019, 19, 264–275 CrossRef CAS PubMed.
- U. Anand, N. Jacobo-Herrera, A. Altemimi and N. Lakhssassi, Metabolites, 2019, 9, 258 CrossRef CAS PubMed.
- G. O. Aspinall, Carbohydr. Res., 1985, 135, C23–C24 CrossRef.
- I. Ćorković, D. Gašo-Sokač, A. Pichler, J. Šimunović and M. Kopjar, Life, 2022, 12, 1692 CrossRef PubMed.
- K. Herget, H. Frerichs, F. Pfitzner, M. N. Tahir and W. Tremel, Adv. Mater., 2018, 30, 1707073 CrossRef PubMed.
- W. Alam, C. Rocca, H. Khan, Y. Hussain, M. Aschner, A. De Bartolo, N. Amodio, T. Angelone and W. S. Cheang, Antioxidants, 2021, 10(10), 1643 CrossRef CAS PubMed.
- M. A. Astiti, A. Jittmittraphap, P. Leaungwutiwong, N. Chutiwitoonchai, P. Pripdeevech, C. Mahidol, S. Ruchirawat and P. Kittakoop, Foods, 2021, 10(12), 3041 CrossRef CAS PubMed.
- M. C. Gidaro, L. Astorino, A. Petzer, F. Mannina, G. Alcaro, A. Costa, A. Artese, G. Rafele, F. M. Russo, J. P. Petzer and S. Alcaro, J. Agric. Food Chem., 2016, 64, 1394–1400 CrossRef CAS PubMed.
- S.-H. Jiang, L.-P. Hu, X. Wang, J. Li and Z.-G. Zhang, Oncogene, 2019, 39, 503–515 CrossRef PubMed.
- R. D. Gupta, S. A. Mahant, P. R. Wankhade, A. T. Hemke, K. J. Wadher and M. J. Umekar, Int. J. Pharmacognosy Life Sci., 2021, 2, 49–56 CrossRef.
- C. Banerjee, S. Nandy, J. Chakraborty and D. Kumar, Food Funct., 2022, 13(12), 6545–6559 RSC.
- A. Altemimi, N. Lakhssassi, A. Baharlouei, D. Watson and D. Lightfoot, Plants, 2017, 6, 42 CrossRef PubMed.
- T. Hu, R. Ou, H. Liu, Y. Hou, Q. Wei, W. Song, B. Cao, Y. Chen, X. Yuan and H. Shang, Clin. Neurol. Neurosurg., 2018, 175, 124–129 CrossRef PubMed.
- Y. W. Dong, M. L. Liao, X. L. Meng and G. N. Somero, Proc. Natl. Acad. Sci. U. S. A., 2018, 115(6), 1274–1279 CrossRef CAS PubMed.
- A. B. Ojo, G. Gyebi, O. Alabi, M. Iyobhebhe, C. O. Nwonuma and O. A. Ojo, J. Mol. Struct., 2022, 1268, 133675 CrossRef CAS.
- G. A. Gyebi, O. M. Ogunyemi, A. A. Adefolalu, A. Rodríguez-Martínez, J. F. López-Pastor, A. J. Banegas-Luna, H. Pérez-Sánchez, A. P. Adegunloye, O. B. Ogunro and S. O. Afolabi, J. Mol. Struct., 2022, 1262, 133019 CrossRef CAS PubMed.
- P. A. Kollman, I. Massova, C. Reyes, B. Kuhn, S. Huo, L. Chong, M. Lee, T. Lee, Y. Duan and W. Wang, Accounts Chem. Res., 2000, 33(12), 889–897 CrossRef CAS PubMed.
|
This journal is © The Royal Society of Chemistry 2024 |
Click here to see how this site uses Cookies. View our privacy policy here.