DOI:
10.1039/D3MO00127J
(Research Article)
Mol. Omics, 2024,
20, 64-77
Long-term physical inactivity induces significant changes in biochemical pathways related to metabolism of proteins and glycerophospholipids in mice†
Received
29th June 2023
, Accepted 14th October 2023
First published on 16th October 2023
Abstract
Physical inactivity affects multiple organ systems, including the musculoskeletal system, which upsets the delicate balance of several secretory factors leading to metabolic derailment. This reduces contractile recruitment of the skeletal muscle with dampening of its oxidative capacity resulting in impaired intramuscular lipid metabolism and substrate utilization. We hypothesized that this altered phenotype would also have an indispensable effect on circulatory cytokines and the level of metabolic intermediates. In this study, comparison between sedentary (SED) and exercised (EXER) animal models showed that organismal metabolic parameters (body mass, oxygen utilization and glucose tolerance) are altered based on physical activity. Our data suggest that cytokines linked to glycemic excursions (insulin, c-peptide, glucagon) and their passive regulators (leptin, BDNF, active ghrelin, and GIP) exhibit changes in the SED group. Furthermore, some of the proinflammatory cytokines and myokines were upregulated in SED. Interestingly, serum metabolite analysis showed that the levels of glucogenic amino acids (alanine, glycine, tryptophan, proline and valine), nitrogenous amino acids (ornithine, asparagine, and glutamine) and myogenic metabolites (taurine, creatine) were altered due to the level of physical activity. A pyrimidine nucleoside (uridine), lipid metabolite (glycerol) and ketone bodies (acetoacetate and acetate) were found to be altered in SED. A Spearman rank correlation study between SED and CTRL showed that cytokines build a deformed network with metabolites in SED, indicating significant modifications in amino acids, phosphatidylinositol phosphate and glycerophospholipid metabolic pathways. Overall, long-term physical inactivity reorganizes the profile of proinflammatory cytokines, glucose sensing hormones, and protein and glycerophospholipid metabolism, which might be the initial factors of metabolic diseases due to SED.
1. Introduction
Recent studies have shown that the rapid switching of substrate pathways, also termed metabolic flexibility, is highly connected to the level of physical activity and is integrated by several metabolic intermediates and cytokines.1 Glucose and lipid metabolism are major determinants of metabolic flexibility, which are maintained by insulin signaling in organs such as skeletal muscle, adipose tissue, and the liver.2,3 The metabolic flexibility is further synchronized with many extrinsic stimuli (exercise, gut microbiota, cold, diet, stress) and intrinsic biomolecules (hormones, secretory peptides, cytokines) that in turn lead to divergent phenotypes. These intrinsic biomolecules are target specific and act in an endocrine, paracrine, and autocrine manner either independently or synergistically with other factors including metabolic pathway intermediates.4 The metabolome of tissues affects cellular physiology by modulating the genome, epigenome, transcriptome, and proteome.5 This production of metabolites shows a combination of nutrient metabolism with gut-associated hormones and peptides. Evidence shows that metabolites like leucine, taurine, carnosine, creatine, and hydroxyproline play crucial roles in protecting cells from oxidative damage, and dietary supplementation of these improves metabolic diseases.6 Many other metabolites serve as negative regulators of metabolism.7 For instance, glucose, fructose, amino acids (AAs), and lipids have a predictive association with diabetes and glutamine, glycine, and aromatic amino acids (AAAs) have even been suggested as biomarkers for diabetes.8,9 The role of the branched-chain amino acids (BCAAs) is unclear as some reported that they enhance insulin signaling while others suggested that they worsen insulin resistance (InR) in patients.10,11 There has been an exponential increase in the incidence of metabolic diseases associated with unbalanced diets and insufficient physical activity.12–14 In reverse, physical activity has a myriad of health benefits with improved heart rate, oxygen consumption, cardiac output, and cellular homeostasis.14,15 Interestingly, the grade of exercise swiftly switches substrate metabolism between the anaerobic and aerobic state and simultaneously provides nutrient supply for other vital organs.16 In this aspect of physiological homeostasis during exercise, we cannot underestimate the temporal role of hormones and cytokines in physiology and disease. Secretion of these during or after exercise depends on the duration of activity and the site of secretion to play inflammatory and anti-inflammatory roles.17–19 These changes in metabolism are closely associated with the molecular adaptation of organs to the molecules in the systemic circulation (energy-sensing metabolites and cytokines).
Despite existing literature on metabolism, the alteration of metabolites and secretory peptides in the circulatory system in a modulated activity state is unexplored. Furthermore, many cytokines have a network of physiological effects on the function of these metabolites that has not beeninvestigated properly. This triggered our interest to build a hypothesis that these cytokines are altered when body activity is minimized by physical restriction or increased by exercise using mice as model organisms. Simultaneously, we are enthusiastic to discover the effect of activity modulation on circulating metabolites. Furthermore, we are trying to establish a correlation between circulatory cytokines and metabolites through metabolic network study. Our data presented here shows that long-term exercise increases metabolic rate providing protection against weight gain. Furthermore, change in physical activity has a significant effect on the level of circulatory metabolites and cytokines and biochemical networks thereof.
2. Materials and methods
2.1 Ethical approval
All the animal experiments were approved by the Institutional Animal Ethics Committee (IAEC) at the School of Biotechnology, KIIT University, Bhubaneswar Odisha, India 751024. The experiments were performed in compliance with the guidelines provided by the above-mentioned committee at the animal housing facility of the School of Biotechnology, KIIT University.
2.2 Animal models
C57BL/6J male mice were obtained from the National Institution of Nutrition (Hyderabad) for this study. We started with a total of 47 (10–12 weeks old, weighing 23–35 g) mice for the experiment. These animals were randomly divided into three groups: physically inactive sedentary (SED, n = 16; initial weight 30 ± 1), control (CTRL, n = 16 initial weight 28 ± 0.9), and more active exercise (EXER, n = 15 initial weight 29 ± 0.8) models and housed for 12 weeks in separate cages (11.41′′ × 8.66′′ × 5.51′′) with free access to sterilized water and standard rodent pellets (nitrogen-free extract 60%, protein 14%, and fat 4%, calcium 1%, phosphorus 0.4%, fiber 5%, moisture 10%, and ash 8% from VRK Nutritional Solutions, Hyderabad) in the animal house of the School of Biotechnology, KIIT. The whole-body weight and food intake were measured on alternate days. The SED mice were kept isolated in self-designed (5′′ × 2′′ × 3′′) rectangular boxes with separate compartments for food supply on the lid. Before the onset of the experiment, EXER mice were first acclimatized to the treadmill for 15 min per day for one week. The EXER group animals were trained using a treadmill with a designed protocol (Table 1). The CTRL and EXER animals were kept in the standard cages up to 5 animals/cage supplied by the Animal house facility throughout the experiments. The animal room was kept hygienic and air-conditioned to maintain the temperature of 23 ± 3 °C under a 12 h light–12 h dark cycle.
Table 1 Treadmill running protocol for the endurance physical activity group
Design of the treadmill exercise protocol |
Week |
Time (min) |
Inclination (in degrees) |
Speed (m min−1) |
Sessions in a week |
1 |
15 |
0 |
5 |
3 |
2 |
15 |
0 |
8 |
3 |
3 |
20 |
5 |
8 |
5 |
4 |
30 |
10 |
10 |
5 |
5 |
40 |
10 |
12 |
5 |
6 |
40 |
10 |
14 |
5 |
7 |
40 |
10 |
16 |
5 |
8 |
40 |
10 |
18 |
5 |
9 |
40 |
10 |
20 |
5 |
10 |
40 |
10 |
22 |
5 |
11 |
40 |
10 |
24 |
5 |
12 |
40 |
10 |
26 |
5 |
Throughout the experiment specific exclusion criteria were implemented, in addition to unexpected death. The exclusion criteria are: (1) drastic reduction in body weight (∼5 g per week); (2) body injuries; (3) aggressive behaviours that make handling the animal impossible. In addition to these we also removed certain animals from the EXER group who were unwilling to run and made contact with the shock grid for more than 10 s. Therefore, after the completion of the experiments, the total number of mice taken for final analysis was 10, 13, and 9 for SED, CTRL, and EXER respectively.
2.3 Intraperitoneal glucose tolerance test (GTT) and insulin tolerance test (ITT)
After 6 weeks and at the end of 12 weeks, animals of each group were randomly separated for intraperitoneal GTT and ITT. An intraperitoneal GTT was performed after a 6 h fasting (n = 6 for each group) to test the level of insulin action to utilize glucose in the body. Following an intraperitoneal glucose injection (2 g kg−1 glucose dissolved in saline), glucose levels were measured at 0, 15, 30, 60, 120 min, and 4 h from tail-snip blood samples using a glucometer (Dr Morepen Glucose Monitor (GlucoOne BG-03), Solan, Himachal Pradesh, India). An ITT (n = 6 per group) was similarly performed after a 5 h fast to observe the whole-body sensitivity of insulin receptors by measuring blood glucose levels at 0, 15, 30, 60, and 120 min after injecting 0.8 IU per kg of insulin (Huminsulin R, 40 IU per mL, Eli Lilly, India) according to the previous protocol.20
2.4 Metabolic measurements using indirect calorimetry
The whole-body oxygen consumption, respiratory exchange ratio, and heat production were measured for each animal using an Oxymax Comprehensive Lab Animal Monitoring System (CLAMS, Columbus Instruments, Columbus, OH) towards the end of the experiment as described earlier.21 The system records the oxygen input at the interval of 30 s using paramagnetic sensors.
2.5 Serum sample collection
On the final day of the experiment (week 12), all C57BL/6J mice were sacrificed in accordance with the guidelines of IAEC. Blood collection was done by cardiac puncture, then left undisturbed for 4 hours at room temperature and centrifuged at 5000 rpm for 5 min. The serum samples were then aliquoted and stored at −80 °C for cytokine analysis, and 125 μL serum was lyophilized for metabolomics study in the Center of Biomedical Research (CBMR), Lucknow, India.
2.6 Cytokine expression profile analysis
The concentration of gut-associated hormones, peptides, myokines, and adipokines in the blood serum samples (n ≥ 9) was measured using MMYOMAG-74K, MMHMAG-44K, MADKMAG-71K, and MADPNMAG-70K KITs in the MAGPX system (DiaSorin Corporate, Italy) with the detection of the fluorescent signal by MagPlex®-C microspheres (fluorescent-coded magnetic beads). These experiments were conducted in Merck Innovation Center Bangalore, India, and the acquired data were further analyzed by Belysa 1.1.0 software.
2.7 Nuclear magnetic resonance measurements
The lyophilized serum samples were mixed with 100% deuterium oxide and analysed using 800 MHz 1H NMR. They were then further processed for multivariate study in Metaboanalyst v4.0. (The details procedure is provided in the ESI.†)
2.8 Statistical analysis
Statistical analyses were performed by either unpaired two-tailed t-tests or one-way analysis of variance (ANOVA) or two-way ANOVA with Tukey posthoc tests (p < 0.05) for body weight, feed weight, metabolic studies and cytokine concentration using the GraphPad Prism 8 statistical software programme. Furthermore, univariate analysis was performed by applying the independent samples T-test and non-parametric Wilcoxon Mann Whitney test to metabolites of interest (identified by the multivariate analysis).
2.9 Metabolomics and cytokine data integration
Prior to data integration, the correlation of selected metabolites and cytokines was carried out by Spearman correlation coefficient using R software. The integration of cytokine data with metabolites was carried out using the metscape interface in cytoscape 3.9.1 software with a cut off of correlation value −0.5 to 0.5, respectively. For the distinct visualization of the data, we used the yFiles organic layout and yFiles remove overlap layout.
3. Results
3.1 Physical activity restriction imbalances the whole-body energy homeostasis and leads to weight gain with a higher fasting glucose load
To understand the effect of physical activity on phenotypic changes of SED and EXER, we initially monitored their basic physiological and metabolic indices using indirect calorimetry. As expected, the SED exhibited drastic weight gain, with a feed intake exciding 2-fold by the end of the experiment. In contrast, the EXER group showed less weight gain than CTRL even though it consumed double the quantity of feed during the later stage of the experiment (Fig. 1A and B). Our studies support the originally published analysis that physical activity is associated with oxygen consumption.22 The rate of oxygen utilization not only varies with degree of physical activity but also varies with a short period of physical activity. After long term sedentariness the animals show reduced oxygen utilization even with shorter duration of activity. Approving this, VO2 data revealed that the EXER group has higher oxygen intake than the SED and control with the highest in the awakened state than the resting state, while the SED group has significantly less oxygen consumption up to 2/3rd of EXER in the awakened and resting state (Fig. 1C and D). After validating the well-known efficacy of physical activity in energy utilization and weight gain status, we tested whether the effect of sedentariness has different effects than reported studies.22,23 As expected, the GTT and ITT after 6 weeks and 12 weeks of the experiment match with previous work on physical activity. The EXER group displayed greater insulin sensitivity throughout the intervention, which was effective from 15 min of the onset of the test (Fig. 1E and F). Whereas the SED group had gradually increasing InR, milder in 6 weeks and became severe towards the end of the 12 weeks of the experiment (Fig. 1G and H).
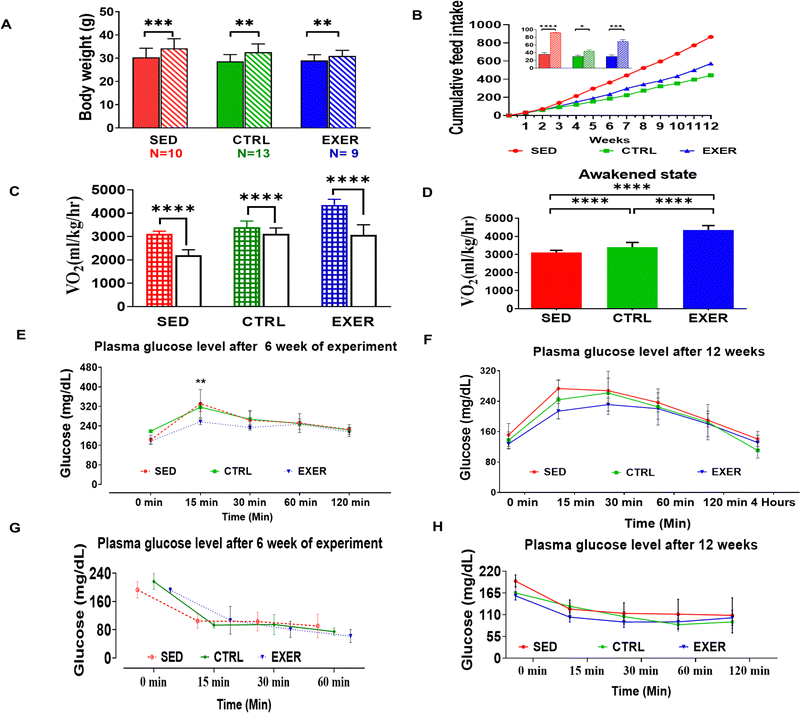 |
| Fig. 1 Change in whole body physiological parameters due to modulation in activity of mice. (A) Comparison of body weight (g) between initial state and final state in different groups of the experiment. (B) Cumulative feed intake (g) with embedded histogram for initial and final stage of feed intake of a 12 week experiment (n = 6). Solid bars represent initial state of feed intake and bars with oblique lines represent final state. Comparison of oxygen intake in all groups (C) in the awakened (most active) and resting state (n = 5) and (D) for four hours. Solid bars represent resting phase and bars with squares represent active awakened state of calorimetric analysis. (‘****’, ‘***’, ‘**’, ‘*’) represent statistical significance (p < 0.0001, p = 0.0001 and p < 0.001 and p < 0.05, respectively). (E) and (F) Glucose tolerance test (GTT) to assess the body's response to glucose in animals of different groups at different GTT time courses. Panels (G and H) show the effect of physical activity in whole-body insulin action and clearance from blood monitored by insulin tolerance test (ITT) in all three models. (SED; red lines with symbols), (CTRL; green line with symbols) and (EXER; blue line with symbols). Data are shown as mean ± SEM, SED groups n = 6, EXER groups n = 5–6, *p < 0.05. Groups in BAR colour coded: sedentary (SED) in red, control (CTRL) in green and exercise (EXER) in blue. | |
3.2 Exercise induces insulin sensitivity, while sedentariness promotes InR by secretory products
To further validate the importance of sedentariness and active lifestyle on the body's glucose homeostasis with crucial synergistic or antagonistic regulating factors of glucose homeostasis like insulin, glucagon, leptin, adiponectin, and resistin, we have conducted cytokine microarray. The insulin shows a highly positive correlation with glucose in EXER in contrast to the other two groups (Fig. S1, ESI†). We have examined the rate of insulin action and its clearance from the body with C-peptide level. The plasma insulin was much higher in SED with lower C-peptide level (Fig. 2A and B). Further, the ratio of C-peptide to insulin shows a significantly lower value in SED (p = 0.001) in comparison to EXER associated with less insulin clearance (Fig. 2C). In addition, SED has higher amounts of glucagon, leptin, and resistin in contrast to adiponectin, which work against effective insulin signaling (Fig. 2D–G). Mostly known as adipokines, resistin levels in the blood rose by nearly 2-fold in SED contributing to inflammation along with InR and less muscle mass than the other groups (Fig. 2G). As evident from previous research,24,25 BDNF is elevated in the EXER groups. It is worth mentioning that the concentration of BDNF was also significantly elevated in SED (Fig. 2H). Another aspect of glucose metabolism is the biological role of incretins and GI tract peptides mediated via hypothalamic responses. The circulatory orexigenic peptide (PP, activated ghrelin) and anorexigenic peptide PYY did not exhibit significant variation in SED compared to the control, but activated ghrelin showed a significant upregulation compared to the exercise group (p < 0.05). While considering the anorexigenic peptide PYY peptides and incretin effect in energy homeostasis, GIP showed less secretion to serum in SED than other groups and a significant increase in exercise (p < 0.001).
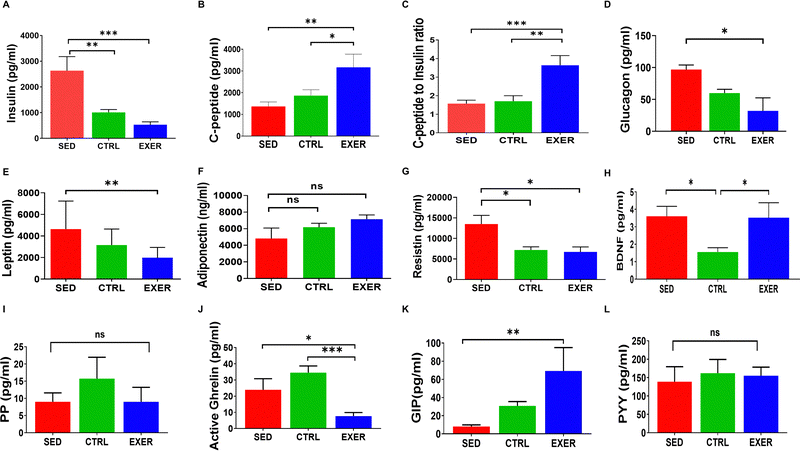 |
| Fig. 2 Influence of activity of hormones and peptides regulating metabolic fuel cycling in the circulation of mice. Bar graph representing the concentration of insulin (A), C-peptide (B), C-peptide to insulin ratio (C), glucagon (D), leptin (E), adiponectin (F), resistin (G), BDNF (H), PP (I), active ghrelin (J), GIP (K) and PYY (L) in the serum of the mentioned groups. Data are presented as mean ± SEM, n = 16–20 mice per group. (‘***’, ‘**’, ‘*’ represent statistical significance p = 0.0001 and p < 0.001 and p < 0.05, respectively). And ns represents statistically nonsignificant. Groups in BAR colour coded: sedentary (SED) in red, control (CTRL) in green and exercise (EXER) in blue. | |
3.3 Modulation in physical activity alters circulatory cytokines affecting muscle metabolism
Recent discoveries on the role of cytokines in various metabolic processes motivated us to investigate immunomodulators and myokines' circulatory level in physical constraint models as the level of physical activity predominantly recruits the skeletal muscle. According to Fig. 3A and B, muscle-derived cytokines show a variable trend in the serum of all the groups. Commonly known for limiting muscle growth, the myostatin level is higher in SED. On the other hand, musclin is significantly greater in both the experimental groups with EXER somewhat higher than SED associated with the remodelling of skeletal muscle. In line with the expression of myokines (musclin, myostatin), immunomodulatory cytokines increased in SED correlated to InR, tissue injury, and muscle atrophy with reduced lipolysis. As said above, cytokines well known for inducing an inflammatory response (TNFα, IL-6, and MCP-1) show higher levels in the SED group than the other two models (Fig. 3C–F). Considering the level of immune modulators, TNF α is more than 2-fold higher in SED (p < 0.001) than EXER and CTRL. IL-6, MCP-1, and PAI-1 descend on the same pathway as TNF α, and follow a similar pattern of about 2-fold higher level in the SED group than the other groups (p < 0.05).
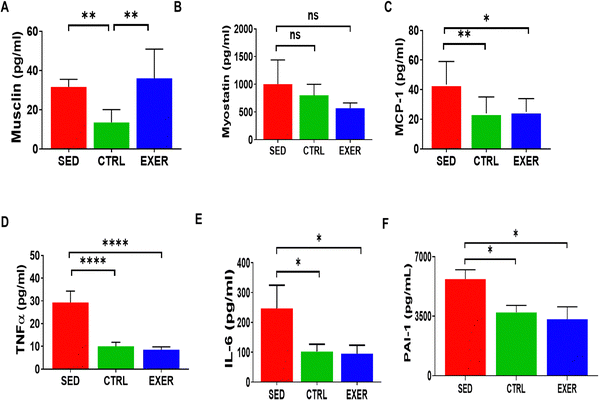 |
| Fig. 3 Sedentariness leads to increased cytokine level in the blood. (A) and (B) Represent the concentration of musclin and myostatin in the serum of the mentioned groups, respectively. (C)–(F) Represent MCP-1, TNFα, IL-6 and PAI-1. Data shown as mean ± SEM, n = 6–12 mice per group, (‘****’, ‘**’, ‘*’ represent statistical significance p < 0.0001 and p < 0.001 and p < 0.05, respectively). And ns represents nonsignificant. Groups in BAR colour coded: sedentary (SED) in red, control (CTRL) in green and exercise (EXER) in blue. | |
3.4 Metabolomics study displays that circulatory asparagine, uridine, and malonate levels are deviated in the SED group
As evident from GTT and cytokine analysis, both groups show intriguing results in serum glucose load, while having a different phenotype and insulin sensitivity. So we further concentrated on profiling of other metabolic intermediates in serum for an activity-modulated response using 1H NMR, and the identified metabolites are represented in Table S1 (ESI†). The metabolite concentrations were measured using formate as an internal reference to reduce experimental deviations (explicit details were described in the ESI,† of materials and method). The PLS-DA data show no distinct group separation in SED, CTRL, and EXER, which suggests few significant metabolite variations between the 3 groups (Fig. S2A–C, ESI†). The hierarchical analysis and metabolic pathway in Fig. 4 give us an overview of significant metabolic disturbances due to long-term modulation of physical activity. In the heat map, out of 45 metabolites, most of the glucogenic and ketogenic AAs (excluding glutamine and tryptophan, threonine) clustered together with much higher concentrations in EXER. Both anaerobic and aerobic glycolysis metabolites and myogenic metabolites show higher concentrations in EXER while thirteen metabolites from the second cluster have much lower values in EXER. On the other hand, out of 43 altered metabolites in SED, 8 metabolites have higher value, especially tryptophan, malonate, taurine, creatine, and acetate, but TCA cycle metabolites (succinate, fumarate, citrate, methylmalonate), ketone bodies (3-HB, acetone), and BCAAs and phosphocreatine show lower values in SED in contrast to the CTRL (Fig. 4A).
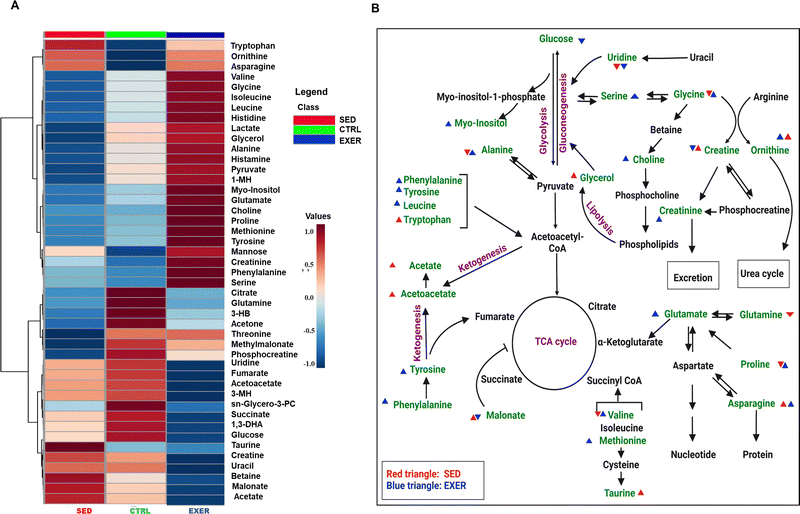 |
| Fig. 4 Exercise challenges cause metabolite profile changes in the serum correlated with altered metabolic pathway. (A) Hierarchical heatmap showing discrimination of EXER and SED groups than CTRL with two clusters of metabolite profile. The bar (red-blue) on the right shows the concentration of each metabolite. Metabolite–cytokine correlation in serum samples from physical inactivity in mice. (B) Schematic diagram depicting key disturbed metabolic pathways in response to level of physical activity. Green colored metabolites were altered in the SED and EXER groups. Purple color represents pathways. Red line indicates an inhibitor of the TCA cycle. Upward triangle signifies increased concentration, downward triangle denotes decreased concentration. 3-HB: 3-hydroxybutyrate, 1,3-dihydroxyacetone: 1,3-DHA. | |
It is observed that the SED has significantly higher malonate (79.78 ± 45.03) in contrast to EXER (30.19 ± 12.78). Furthermore, the circulatory asparagine level is increased in SED as high as EXER in contrast to CTRL. But the concentration of uridine is significantly lower in EXER (3.66 ± 2.63) with no significant variation between SED and CTRL. The correlation studies of these 3 metabolites with the most bonafide top 24 show that there is a positive link between asparagine and 11 metabolites, including AAs (specifically AAAs), while the remaining metabolites, including the glucose and pyrimidine metabolic intermediates, interact with it in the reverse way. Malonate and uridine behave differently than asparagine with the selected metabolites (Fig. S3, ESI†). The pyrimidine metabolism, ketogenic route, and TCA cycle intermediate are all positively related to malonate and uridine, but BCAA shows a negative trend. It is noteworthy that myogenic intermediates and pyruvate exhibit the inverse correlation with the levels of asparagine, malonate, and uridine. Certain metabolites were chosen to depict the metabolite alteration in each group while considering the VIP Score and those have been displayed here (Fig. S2D, ESI† and Table 2). In our study, the VIP score plot showed higher metabolic redundancy with a dominating resonance of glucogenic AAs, glucose and myogenic metabolites. To avoid these redundancies and for screening of other relevant circulatory metabolites, the concentration values were estimated. We observed that other than malonate and uridine, acetate (67.13 ± 13.62), acetoacetate (12.30 ± 1.93), taurine (110.11 ± 27.68), and betaine showed a similar trend of upregulation in SED while most of the non-polar AAs (leucine, 39.758 ± 9.596; tyrosine, 23.80 ± 4.66; valine, 44.64 ± 9.27; methionine 21.699 ± 5.456, alanine, 66.64 ± 15.81 and proline, 36.89 ± 10.41) and glycerol, myoinositol, and choline are lower in the SED than the EXER group. Citrate, glutamate, glycine, serine and BCAAs are higher in the EXER group. Of note, the altered glucogenic AAs, BCAAs, TCA intermediates and glycerol in SED indicate a dysregulation of the metabolic microenvironment due to less energy expenditure with higher energy conservation.
Table 2 The mean (±standard deviation; SD) values of significant serum metabolite concentration in different experimental groups
Metabolites |
Mean ± SD |
SED (N = 10) |
CTRL (N = 13) |
EXER (N = 9) |
Values followed by ‘*’, ‘**’ and ‘***’ are significantly different from CTRL at p < 0.05, p < 0.001 and p < 0.0001. Values followed by ‘#’ and ‘##’ are significantly different from EXER at p < 0.05 and p <0.01. p-Value with (W) is calculated by the Wilcoxon Mann-Whitney test. |
3-MH |
15.735 ± 9.805 |
16.654 ± 8.606 |
11.160 ± 6.834 (*, W) |
Acetate |
67.13 ± 13.62 (#) |
62.34 ± 11.93 |
51.96 ± 5.82 (*) |
Acetoacetate |
12.30 ± 1.93 (#) |
12.87 ± 5.19 |
9.69 ± 2.04 |
Alanine |
66.64 ± 15.81 (#, W) |
76.54 ± 18.11 |
86.26 ± 15.43 |
Asparagine |
23.97 ± 8.07 (**) |
15.49 ± 2.51 |
23.71 ± 7.33 (**) |
Choline |
47.24959 ± 17.24 (##) |
49.27 ± 22.58 |
75.91 ± 19.66 (*) |
Citrate |
37.136 ± 13.453 |
45.713 ± 9.662 |
37.837 ± 13.067 |
Creatine |
54.912 ± 23.086 |
52.806 ± 16.208 |
41.977 ± 11.908 |
Creatinine |
16.778 ± 8.871 |
14.585 ± 7.534 |
22.240 ± 8.069 (*, W) |
Glucose |
219.368 ± 69.935 |
239.325 ± 74.955 |
190.479 ± 65.054 |
Glutamate |
43.627 ± 10.341 |
46.790 ± 19.847 |
55.026 ± 17.660 |
Glutamine |
49.30 ± 11.08 (**) |
65.70 ± 9.54 |
50.10 ± 20.77 |
Glycerol |
78.378 ± 31.232 (#, W) |
100.735 ± 40.971 |
113.942 ± 35.311 |
Histamine |
5.824 ± 5.975 |
8.584 ± 6.055 |
11.373 ± 10.891 |
histidine |
25.278 ± 5.683 |
27.153 ± 4.566 |
30.349 ± 17.757 |
Isoleucine |
34.393 ± 4.286 |
36.393 ± 4.971 |
38.980 ± 9.767 |
Lactate |
12.849 ± 2.458 |
13.689 ± 1.850 |
14.221 ± 2.695 |
Leucine |
39.758 ± 9.596 (*) |
46.599 ± 6.773 |
57.140 ± 22.346 |
Malonate |
79.78 ± 45.03 (#) |
61.93 ± 24.14 |
30.19 ± 12.78 (**) |
Methionine |
21.699 ± 5.456 |
22.479 ± 6.058 |
26.198 ± 5.864 |
Methylmalonate |
31.332 ± 5.881 |
34.870 ± 11.600 |
33.999 ± 7.358 |
myo-Inositol |
23.94 ± 6.94 |
26.11 ± 14.21 |
32.86 ± 15.93 |
Ornithine |
13.29 ± 11.48 |
8.84 ± 6.13 |
12.91 ± 7.51 |
Phenylalanine |
27.28 ± 3.35 |
26.37 ± 4.19 |
32.87 ± 11.96 |
Phosphocreatine |
20.292 ± 2.323 |
26.139 ± 16.039 |
23.580 ± 7.539 |
Proline |
36.89 ± 10.41 (#) |
38.67 ± 11.72 |
50.10 ± 17.32 |
Pyruvate |
17.85 ± 7.05 (#, W) |
22.82 ± 13.29 |
26.69 ± 11.94 |
Serine |
32.39 ± 11.13 |
30.31 ± 13.00 |
46.01 ± 19.74 |
sn-glycero-3-PC |
29.45 ± 10.42 |
35.01 ± 13.54 |
27.21 ± 18.67 |
Succinate |
72.32 ± 20.27 |
74.96 ± 21.95 |
67.53 ± 15.58 |
Taurine |
110.11 ± 27.68 (#) |
88.73 ± 35.54 |
84.75 ± 21.98 |
Tryptophan |
7.96 ± 4.50 (*, W) |
4.30 ± 2.36 |
6.78 ± 5.73 |
Tyrosine |
23.80 ± 4.66 |
25.50 ± 4.71 |
33.01 ± 13.46 |
Uracil |
12.62 ± 2.23 |
12.58 ± 3.87 |
11.65 ± 2.36 |
Uridine |
8.39 ± 4.89 (#) |
9.87 ± 2.99 |
3.66 ± 2.63 (***) |
Valine |
44.64 ± 9.27 |
50.79 ± 7.17 |
59.02 ± 17.78 |
3.5 Metabolite-cytokine correlation study shows that sedentariness alters amino acid, phosphatidylinositol phosphate, and glycerophospholipid metabolism pathways
The correlation analysis in our work indicated that the selected cytokines have various degrees of association within each group (Fig. 5A–C). The CTRL and SED groups demonstrated a higher degree of dependency among cytokines than the EXER group. In particular, the correlation between leptin and insulin was more strongly positive in the EXER group, showing a significant decrease in the levels of both cytokines with the presence of BDNF (−0.9). Furthermore, glucose-sensing cytokines and myostatin interact positively but in the opposite direction to inflammatory cytokines. The SED group exhibits more highly converted cytokine correlation values than the CTRL group in serum. Specifically, the correlation profiles of TNFα, resistin, MCP-1, adiponectin, GIP and insulin were altered in the serum in SED with TNFα more positively connected with IL-6 in the SED group compared to the CTRL group. While IL-6 showed minimized opposing interaction with GIP and C-peptide in this SED group, suggesting the cooccurrence of inflammatory cytokines. Musclin showed an increased positive relationship with insulin (0.98) and C-peptide (0.90) and GIP (0.85) in SED. Remarkably, the significant negative interaction of PP with MCP-1 is altered in SED, with MCP-1 exhibiting a stronger negative value with musclin (−0.8) and insulin (−0.85).
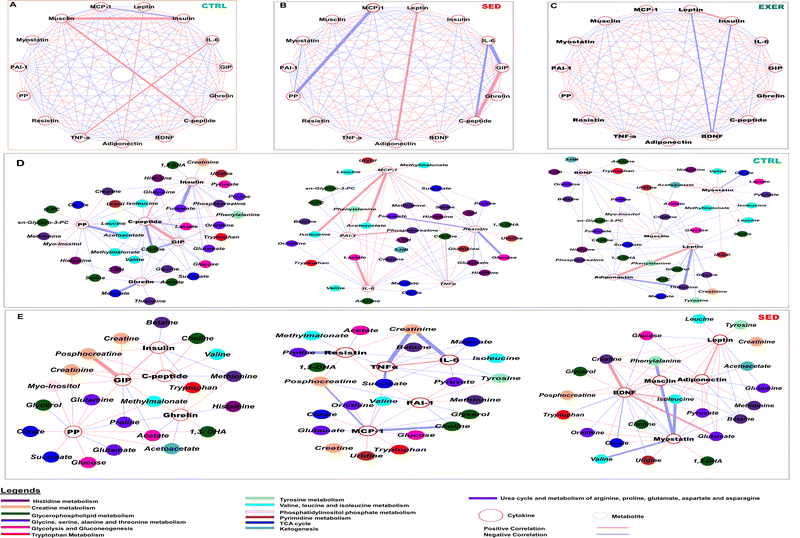 |
| Fig. 5 Correlation network of key cytokines and metabolites showing significant differences in the SED group. (A)–(C) Cytokines showing various degrees of correlation in SED, CTRL and EXER, respectively. (D) and (E) Interaction of metabolites to cytokines represents connection with various biochemical pathways in the CTRL and SED groups. The integrative network was generated using the MetScape plugin available in Cytoscape 3.9.1. software. The color of the nodes represents different metabolic pathways. The color of the edges indicates positive (red) or negative (purple) correlation. The width of the edges is proportional to the strength of the correlation. The position of the nodes was manually adjusted to improve the clarity of the network. All pairs were plotted using a threshold of a Spearman correlation coefficient >0.99 and p value <0.01 (check the range). Metabolite–cytokine correlation in serum samples from exercise challenge mice. O-Phosphocholine: O-PC. 1-Methylhistidine: 1-MH, 3-methylhistidine: 3-MH. | |
Considering the discovery of a significant difference in cytokine interaction, we conducted an independent correlation analysis between cytokines and metabolites involved in dysregulated metabolic pathways in SED versus CTRL. Leptin and adiponectin were found to be associated with elevated levels of ketogenic AAs (tryptophan, isoleucine, phenylalanine and leucine) in the circulation of the body in the SED group (Fig. 5D and E). In the SED group, circulating insulin and C-peptide were favourably linked with creatine, choline, and phosphocholine (PC) and lactate represented altered creatinine, glycerophospholipid metabolism, and glycolysis. PP positively interacts with ketogenesis metabolites (acetoacetate, 3-HB) and TCA cycle metabolites (citrate, succinate) with a simultaneous negative impact on glutamate in SED contrasting to CTRL mice. Although GIP expressed a similar trend with PP in CTRL, it has a positive interaction to creatine, phosphocreatine and uracil in SED associated with reduced creatine metabolism and pyrimidine metabolism. Serum ghrelin shows minute positive values for essential AAs (tryptophan, and methionine) in the SED group.
With the above changed orientation in the correlation networks, inflammatory cytokines showed an effect on inositol phosphate metabolism, creatine metabolism, and mitochondrial metabolism with variable interaction value. In the sedentary group, the elevated resistin is associated with increased levels of myoinositol, phosphocreatine, succinate, and 1,3-DHA while showing negative interaction with glycine and proline and methyl malonate. (Fig. 5E). MCP-1 exhibited a positive interaction with BCAA, acetoacetate, and creatine metabolites, but negative associations with creatine and glycerophospholipid metabolic intermediates. It also interacted with choline, ornithine, and creatinine. The strong positive interaction of MCP-1 with BCAA, acetoacetate, and creatine metabolites, fully vanished in SED with showing negative associations to creatine and glycerophospholipid metabolic intermediates. It also interacts with choline, ornithine and creatinine. The positive interactions of IL-6 with BCAA, glycolytic metabolites, choline, and glutamate were altered with an extremely negative interaction with creatinine in the SED group. TNFα showed transformed negative interactions with pyruvate, malonate, and succinate, and increasing degrees of negative interaction with tyrosine and creatinine in the SED group compared to the CTRL group. PAI-1 showed highly negative interactions with fumarate and glycerophospholipid metabolites but enhanced interactions with asparagine, tyrosine, methionine, pyruvate, and succinate in the SED group compared to the CTRL group.
Additionally, circulatory myostatin showed negative associations with ornithine and uridine but negative correlation with most of the essential AAs (phenylalanine, isoleucine, valine, threonine and tryptophan, histidine) suggesting altered AA (BCAA, histidine, and tyrosine) metabolism to urea cycle and pyrimidine metabolism in SED contrasting to the CTRL group. Despite their elevated level, SED affected the association of metabolites with BDNF and musclin and showed an opposite pattern among them. BDNF had positive interactions with uridine, tryptophan, BCAAs and glutamate that were altered in SED in contrast to musclin perhaps directed towards the leucine–glutamate cycle. In SED, the positive interaction of inflammatory cytokines (PAI-1, MCP-1) and the negative connection of IL-6, TNFα, adipokines (leptin and adiponectin), and GIP with phosphocreatine were altered, indicating creatine metabolism disturbance. In the sedentary group, the elevated resistin is associated with increased levels of myoinositol, creatinine, phosphocreatine, succinate, and 1,3-DHA (Fig. 5E).
4. Discussion
Regular exercise is beneficial for bodyweight management by influencing the energy equilibrium of the whole body regulating substrate utilization that has the potential to ameliorate neurodegenerative conditions in mice as well as in humans.26,27 In our study, we found that mice adapted for a sedentary lifestyle have low oxygen consumption during resting and are unable to upregulate their VO2. On the other hand, those trained for exercise maintain a higher VO2 and are able to increase their VO2 upon exercise test. Moreover, data indicate that the SED group has impaired GTT and ITT while this is improved in the EXER group, indicating that the level of physical activity programs the bodily metabolism to enhance flexibility in substrate usage. These data corroborate with published literature showing that metabolic flexibility is closely associated with the level of skeletal muscle recruitment.28 It is suggested that physical activity modulates the circulatory levels of some hormones and cytokines (like insulin, glucagon, leptin, adiponectin), thereby regulating muscle metabolic flexibility. While some of them work synergistically, some counteract the insulin signaling leading to their effects on substrate utilisation and storage. Data shows that the C-peptide
:
insulin ratio is higher in the exercise group while lower in the sedentary group, which might indicate an equilibrium between insulin secretion and utilization as proposed earlier. Studies in rodents and horses show a reduced level of C-peptide associated with InR and reduced hepatic insulin clearance.29,30 The other prominent one is glucagon that is known to oppose insulin stimulated hepatic glycogenesis and antilipolytic effect to maintain optimal glucose availability to the brain.28,29,31 During exercise, glucagon is chiefly supplied to the liver with minimal concentration in systemic circulation,32,33 whereas higher circulatory glucagon in long-term physical inactivity is associated with ketogenesis due to oxidation of lipids.34 Our finding showing higher serum glucagon in the sedentary group indicates that 12 weeks of low activity is enough to induce InR in mice. Two major adipokines (leptin and adiponectin) work antagonistically to each other modulating hypothalamic insulin signaling.35 Data showing reduced leptin and increased adiponectin in exercising animals (and reverse in sedentary) suggest that adaptation to higher physical activity enables a competent insulin response.
Circulatory levels of many inflammatory cytokines (TNFα, Il-6, resistin) show a linear relationship with skeletal muscle InR and atrophy.36–38 Exercise activity reduced their proinflammatory activity with the concomitant secretion of anti-inflammatory cytokines (IL-1RA, IL-10).39 Data in our study revealed that long-term physical inactivity associated with persistent elevated resistin and IL-6 led to accelerated InR and loss of muscle protein thereby initiating muscle degeneration.40,41 Physical activity is also suggested to modulate the secretion of many contraction-associated myokines.18 One of these, myostatin accumulation, is another plausible cause for InR-induced skeletal muscle wasting and fat accumulation in mice.42 In our study, an increased circulatory concentration of this in SED is associated with loss of muscle protein due to fat storage. Another myokine, musclin, is known to be secreted post exercise to enhance physical endurance and is associated with InR-induced ER stress in diabetic and obese patients and rats.43,44 In our study, its upregulation might signify efficient insulin signalling associated adaptation to endurance training in EXER; on the other hand, in SED this corroborates the link of InR to blunted mitochondrial oxidativeness in muscle. Notably, BDNF helps in synaptic and muscular plasticity with maintaining glucose metabolism and fat oxidation showing sceptical findings during exercise intervention.45,46 Many studies in rat and human subjects reported increased concentration of BDNF secretion from skeletal muscle associated with fat oxidation, but this is not reflected in circulation in exercise.25 In contrast to these results, our study is the first one to show that a higher concentration in circulation in both distinct features of physical activity may provide an adaptive response against oxidative stress in the brain and periphery.
The serum metabolite concentration depends primarily on three factors i.e. secretion as metabolic byproduct, efficiency of tissue uptake and rate of utilization. Studies have highlighted that the differential abundance of AAs in the serum (which is associated with protein turnover) is modulated by the level of physical activity. Long-term inactivity induces muscle protein break down releasing AAs into circulation leading to greater AA transport and utilization, impairing the body's ability to effectively utilize dietary AAs.47 Interestingly, out of the metabolite AAs, BCAAs act as intermediates for lipid synthesis. In our study, lower concentration of these metabolites might associate with phospholipids biogenesis. Glycine (a glucogenic non-essential AA) participates in the biosynthesis of nucleic acids, proteins and lipids, while on the other hand serving as a precursor for glycogen, urea, and creatine. In addition, glycine is linked with cellular glutathione (GSH, a major oxidative stress defenser) level; consequently, lowered glycine concentration results in downregulated mitochondrial GSH (mGSH) leading to mitochondrial dysfunction.48–53 Our study shows that low plasma glycine levels were associated with high body fat and metabolic complications, which was partially corrected by exercise. Next, a prominent AA metabolite is taurine that was found to be lower in EXER and higher in the SED group. Taurine is a β-amino sulfonic acid that is produced from cysteine via GSH and is abundant in the skeletal muscles. Our observation that SEDs have higher taurine may impair GSH biosynthesis causing mitochondrial dysfunction contributing to their compromised metabolic health.
Another metabolite whose serum level is greatly influenced by the level of physical activity is creatine and is closely associated with the energy demand. Creatine is synthesized in the liver, and converted to phosphocreatine in muscle for instant ATP generation during exercise and forming creatinine. Previous studies reported that long-term exercise decreases total creatine along with enhanced phosphocreatine and creatinine in plasma.54,55 In our experiment, sedentariness accumulated serum creatine due to lowered reliance on this energy buffering pathway. One of the significant dicarboxylic acids in serum is malonate, which modulates mitochondrial function and fatty acid synthesis.56,57 In our study, the higher malonate level in the SED group might promote fatty acid synthesis by inhibiting oxidative phosphorylation causing reduced oxygen consumption, as suggested by others.58,59 Higher malonate level is reported to be associated with increased succinate concentration, while we did not observe any significant alteration in serum succinate level. From our studies we found alteration in other physiologically active metabolites that facilitate muscle performance, namely glycerophospholipids, generated primarily from choline and PCs. Our data showing a lowered level of choline and PCs in SED might suggest compromised ability to boost muscle performance upon challenge.54,60 This might indicate that long-duration physical inactivity reprograms muscle metabolism towards storage of glycerophospholipids, which lead to inefficient muscle functionality causing InR.19,61
Studies have speculated the immune modulatory role of BBCA, accumulated lactate and oxidized phospholipids in cytokine secretion62,63 and provided clues for the reciprocal regulatory circuits between metabolic alterations and cytokine release. Intriguingly, the correlation network analysis in our study revealed that metabolite-cytokine correlations were in SED perturbated compared to the CTRL group. Studies identify the important role of insulin in the incorporation of AAs into protein, inhibition of proteolysis and simultaneous stimulated lipogenesis with suppressing lipolysis.64–66 In our correlation study in the SED group, impaired insulin signalling and level of associated GI peptides intricately playing a critical role in altered phospholipid synthesis may lead to ceramide formation. Furthermore, the high correlation of insulin to musclin may be associated with an impaired condition of proline, asparagine and urea metabolism with disturbed metabolic state due to InR.
Interestingly, inflammatory cytokines (TNF-α, IL-6) affect the phospholipid metabolism and subsequent ceramide, and ROS.67 In particular, the circulating inflammatory cytokine levels were highly correlated with metabolites constituting glycerophospholipid, urea metabolism and creatine metabolism in SED. Published literature reported that the reduced availability of BBCA was coordinated downregulation of the entire metabolic pathway in response to TNFα.68 In agreement with this, the profile of BBCA amino acids was reprogrammed with elevated levels of other inflammatory cytokines (MCP1 and IL-6), leptin and myostatin. Moreover, BCAA metabolism serves as a source of anaplerotic replenishment for the TCA cycle. The TNFα treatment revealed changes in the level of TCA cycle intermediates with impaired BCAA metabolism with ER stress.68 The altered TCA cycle intermediates with higher level of circulatory metabolites delineate the crosstalk between metabolism and inflammatory cytokine secretion leading to mitochondrial dysfunction due to long-term physical inactivity. Some other studies speculated the effect of IL-6 and TNFα in tryptophan metabolism.69–71 Perhaps, the MCP1 (downstream product of TNFα signaling) cooperates with myostatin and antagonizes insulin, c-peptide and BDNF to alter tryptophan metabolism and facilitate tryptophan secretion to serum. Furthermore, TNFα and IL6 negatively affect creatinine, but the higher correlation of creatine to adipokines, insulin and BDNF is corroborated with higher serum creatine level suggesting a disturbed creatine metabolism in SED animals due to less energy demand.
5. Conclusion
In summary, our study showed that long-term inactivity reprogrammed the metabolic status, tightly accompanied with proinflammatory cytokines. In addition to providing a resource of metabolism, our data support the involvement of creatine metabolism with the level of physical activity. Our study also uncovered new insight related to the tight correlation of glycerophospholipid and protein metabolism to InR and secretory peptide release, thereby providing further information on tissue-specific utilization of substrates and metabolic intermediates and will be a valuable therapeutic strategy for manipulating InR-induced complications.
Abbreviations
VO2 | Velocity of oxygen consumption |
GIP | Gastric inhibitory factor |
3-HB | 3-Hydroxybutyrate |
1,3-DHA | 1,3-Dihydroxyacetone |
1D | One dimensional |
1-MH | 1-Methylhistidine |
3-MH | 3-Methylhistidine |
AA | Amino acid |
AAA | Aromatic amino acid |
CV-ANOVA | Analysis of variance testing of cross-validated predictive residuals |
BDNF | Brain-derived neurotrophic factor |
BCAA | Branched-chain amino acid |
CPMG | Carr–Purcell–Meiboom–Gill |
CLAMS | Comprehensive lab animal monitoring system |
CTRL | Control |
EXER | Exercise |
GTT | Glucose tolerance test |
GSH | Glutathione |
IAEC | Institutional animal ethics committee |
InR | Insulin resistance |
ITT | insulin tolerance test |
IL-6 | Interleukin 6 |
MCP-1 | Monocyte chemoattractant protein-1 |
NMR | Nuclear magnetic resonance |
PP | Pancreatic peptide |
PYY | Peptide YY |
PC | Phosphocholine |
PAI-1 | Plasminogen activator inhibitor-1 |
PCA | Principal component analysis |
PLS-DA | Projection to least-squares discriminant analysis |
RER | Respiratory exchange ratio |
SED | Sedentary |
TCA | Tri carboxylic acid |
TNFα | Tumour necrosis factor-alpha |
VIP | variable importance on projection |
Animal ethical statement
The animal experiments were approved by the Institutional Animal Ethics Committee (IAEC) with approval no. KSBT/IAEC/2017/MEET-1/A11.
Data availability
All the NMR spectra, chenomx files and source data needed to evaluate the results are provided as ESI.† R scripts for metaboanalyst and templates of cytoscape files were added for a thorough analysis.
Author contributions
Conceptualization: BS and NCB; methodology: BS, SP, GS, RR, DK, and NCB; formal analysis: BS, SP, RR, RT, NCB, and DK; investigation: BS and NCB; resources: NCB. data curation: BS, RR, SD, and RT; writing-original draft preparation: BS and NCB. writing-review and editing: BS, BP, US, PP, SR, and NCB, visualization: BS; supervision: NCB; project administration: NCB. All authors have read and agreed to the published version of the manuscript.
Conflicts of interest
The authors declare no conflict of interest.
Acknowledgements
This work was supported by the Council of Scientific and Industrial Research (CSIR), India [File no. 09/1035 (0011)/2017-EMR-I] to BS, the Science and Engineering Research Board (SERB), DST, India [Grant number ECR/2016/001247]; and DBT, India [Grant Number BT/RLF/Re-entry/41/2014 and BT/PR28935/MED/30/2035/2018] to NCB. G. S. S. P. U. S. and B. P. are recipients of senior research fellowships from the Indian Council of Medical Research (ICMR) vide grant no. 45/9/2020-PHY/BMS, 45/3/2019/PHY/BMS, 45/02/2022-PHY/BMS, and 45/17/2022-PHA/BMS, respectively. P. P. is a recipient of a JRF from DST, Ministry of Science & technology, INDIA with grant no. DST/INSPIRE Fellowship/2018/IF180892. We thank Akhilesh Srivastava, Arunkumar Padmanaban, Aparna M. S., Ankur Gautam, and Rekha Jain for their assistance at the Merck Innovation Center for cytokine multiplex assays. Additionally, we would like to express our gratitude to Dr Bikash Baishya, Dr Umesh Kumar, Rajeev Verma, and Sachin Yadav for their constructive suggestions and discussions that enabled us to interpret the metabolomics data in CBMR.
References
- F. Grün and B. Blumberg, Minireview: The Case for Obesogens, Mol. Endocrinol., 2009, 23(8), 1127–1134 CrossRef PubMed.
- N. Abedpoor, F. Taghian and F. Hajibabaie, Physical activity ameliorates the function of organs via adipose tissue in metabolic diseases, Acta Histochem., 2022, 124(2), 151844 CrossRef CAS PubMed.
- N. Abedpoor, F. Taghian, K. Ghaedi, I. Niktab, Z. Safaeinejad and F. Rabiee,
et al., PPARγ/Pgc-1α-Fndc5 pathway up-regulation in gastrocnemius and heart muscle of exercised, branched chain amino acid diet fed mice, Nutr. Metab., 2018, 15(1), 59 CrossRef PubMed.
- T. Andus, J. Bauer and W. Gerok, Effects of cytokines on the liver, Hepatology, 1991, 13(2), 364–375 CrossRef CAS PubMed.
- M. M. Rinschen, J. Ivanisevic, M. Giera and G. Siuzdak, Identification of bioactive metabolites using activity metabolomics, Nat. Rev. Mol. Cell Biol., 2019, 20(6), 353–367 CrossRef CAS PubMed.
- N. Abedpoor, F. Taghian and F. Hajibabaie, Cross Brain–Gut Analysis Highlighted Hub Genes and LncRNA Networks Differentially Modified During Leucine Consumption and Endurance Exercise in Mice with Depression-Like Behaviors, Mol. Neurobiol., 2022, 59(7), 4106–4123 CrossRef CAS.
- O. Savolainen, B. Fagerberg, M. Vendelbo Lind, A. S. Sandberg, A. B. Ross and G. Bergström, Biomarkers for predicting type 2 diabetes development—Can metabolomics improve on existing biomarkers?, PLoS One, 2017, 12(7), e0177738 CrossRef.
- M. Diniz, F. H. S. de, A. M. R. Beleigoli, M. I. Schmidt, B. B. Duncan, A. L. P. Ribeiro and P. G. Vidigal,
et al., Homeostasis model assessment of insulin resistance (HOMA-IR) and metabolic syndrome at baseline of a multicentric Brazilian cohort: ELSA-Brasil study, Cad. Saude Publica, 2020, 36, e00072120 CrossRef.
- B. Arneth, R. Arneth and M. Shams, Metabolomics of type 1 and type 2 diabetes, Int. J. Mol. Sci., 2019, 20(10), 2467 CrossRef PubMed.
- C. B. Newgard, Interplay between Lipids and Branched-Chain Amino Acids in Development of Insulin Resistance, Cell Metab., 2012, 15(5), 606–614 CrossRef CAS PubMed.
- S. H. Shah, D. R. Crosslin, C. S. Haynes, S. Nelson, C. B. Turer and R. D. Stevens,
et al., Branched-chain amino acid levels are associated with improvement in insulin resistance with weight loss, Diabetologia, 2012, 55(2), 321–330 CrossRef CAS PubMed.
- G. V. Denis and M. S. Obin, ‘Metabolically healthy obesity’: origins and implications, Mol. Aspects Med., 2013, 34(1), 59–70 CrossRef CAS.
- World Health Organization, Global Strategy on Diet, Physical Activity and Health: Diet and Physical Activity-A Public Health Priority, 2016 Search PubMed.
- F. W. Booth, C. K. Roberts and M. J. Laye, Lack of Exercise Is a Major Cause of Chronic Diseases, Compr. Phys., 2023, 1143–1211, DOI:10.1002/cphy.c110025.
- P. D. Neufer, M. M. Bamman, D. M. Muoio, C. Bouchard, D. M. Cooper and B. H. Goodpaster,
et al., Understanding the Cellular and Molecular Mechanisms of Physical Activity-Induced Health Benefits, Cell Metab., 2015, 22(1), 4–11 CrossRef CAS.
- R. M. Murphy, M. J. Watt and M. A. Febbraio, Metabolic communication during exercise, Nat. Metab., 2020, 2(9), 805–816 CrossRef.
- B. Sahu and N. C. Bal, Adipokines from white adipose tissue in regulation of whole body energy homeostasis, Biochimie, 2022, 204, 92–107 CrossRef PubMed.
- B. Sahu, O. Tikoo, B. Pati, U. Senapati and N. C. Bal, Role of Distinct Fat Depots in Metabolic Regulation and Pathological Implications, Reviews of Physiology, Biochemistry and Pharmacology, 2022, 186, 135–176 Search PubMed.
- H. Yaribeygi, M. Maleki, T. Sathyapalan, T. Jamialahmadi and A. Sahebkar, Pathophysiology of Physical Inactivity-Dependent Insulin Resistance: A Theoretical Mechanistic Review Emphasizing Clinical Evidence. Papanas N, editor, J. Diabetes Res., 2021, 2021, 7796727 Search PubMed.
- E. Kokkotou, J. Y. Jeon, X. Wang, F. E. Marino, M. Carlson and D. J. Trombly,
et al., Mice with MCH ablation resist diet-induced obesity through strain-specific mechanisms, Am. J. Physiol.: Regul., Integr. Comp. Physiol., 2005, 289(1), R117–R124 CrossRef CAS.
- N. C. Bal, S. Singh, F. C. Reis, S. K. Maurya, S. Pani and L. A. Rowland,
et al., Both brown adipose tissue and skeletal muscle thermogenesis processes are activated during mild to severe cold adaptation in mice, J. Biol. Chem., 2017, 292(40), 16616–16625 CrossRef CAS.
- N. M. Hamburg, C. J. McMackin, A. L. Huang, S. M. Shenouda, M. E. Widlansky and E. Schulz,
et al., Physical inactivity rapidly induces insulin resistance and microvascular dysfunction in healthy volunteers, Arterioscler., Thromb., Vasc. Biol., 2007, 27(12), 2650–2656 CrossRef CAS PubMed.
- R. L. Bradley, J. Y. Jeon, F. F. Liu and E. Maratos-Flier, Voluntary exercise improves insulin sensitivity and adipose tissue inflammation in diet-induced obese mice, Am. J. Physiol.: Endocrinol. Metab., 2008, 295(3), E586–E594 CrossRef CAS PubMed.
- A. Araya, X. Orellana, D. Godoy, L. Soto and J. Fiedler, Effect of exercise on circulating levels of brain-derived neurotrophic factor (BDNF) in overweight and obese subjects, Horm. Metab. Res., 2013, 45(07), 541–544 CrossRef CAS PubMed.
- Y. Nofuji, M. Suwa, H. Sasaki, A. Ichimiya, R. Nishichi and S. Kumagai, Different circulating brain-derived neurotrophic factor responses to acute exercise between physically active and sedentary subjects, J. Sports Sci. Med., 2012, 11(1), 83 Search PubMed.
- F. Hajibabaie, N. Abedpoor, F. Taghian and K. Safavi, A Cocktail of Polyherbal Bioactive Compounds and Regular Mobility Training as Senolytic Approaches in Age-dependent Alzheimer's: the In Silico Analysis, Lifestyle Intervention in Old Age, J. Mol. Neurosci., 2023, 73(2), 171–184 CrossRef CAS PubMed.
- C. E. Cox, Role of Physical Activity for Weight Loss and Weight Maintenance, Diabetes Spectr., 2017, 30(3), 157–160 CrossRef PubMed.
- B. Egan and J. R. Zierath, Exercise Metabolism and the Molecular Regulation of Skeletal Muscle Adaptation, Cell Metab., 2013, 17(2), 162–184 CrossRef CAS PubMed.
- F. Tóth, N. Frank, T. Martin-Jiménez, S. B. Elliott, R. J. Geor and R. C. Boston, Measurement of C-peptide concentrations and responses to somatostatin, glucose infusion, and insulin resistance in horses, Equine Vet. J., 2010, 42(2), 149–155 CrossRef PubMed.
- C. Marmentini, G. M. Soares, G. A. Bronczek, S. Piovan, C. E. Mareze-Costa and E. M. Carneiro,
et al., Aging Reduces Insulin Clearance in Mice, Front. Endocrinol., 2021, 12, 679492 CrossRef PubMed.
- M. M. Adeva-Andany, R. Funcasta-Calderón, C. Fernández-Fernández, E. Castro-Quintela and N. Carneiro-Freire, Metabolic effects of glucagon in humans, J. Clin. Transl. Endocrinol., 2019, 15, 45–53 Search PubMed.
- E. Trefts, A. S. Williams and D. H. Wasserman, Exercise and the regulation of hepatic metabolism, Prog. Mol. Biol. Transl. Sci., 2015, 135, 203–225 Search PubMed.
- B. Ahrén, Glucagon secretion in relation to insulin sensitivity in healthy subjects, Diabetologia, 2006, 49(1), 117–122 CrossRef.
- F. Gyntelberg, M. J. Rennie, R. C. Hickson and J. O. Holloszy, Eff Train Response Plasma Glucagon Exerc, J. Appl. Physiol., 1977, 43, 302–305 CrossRef CAS.
- L. Forny-Germano, F. G. De Felice and M. N. do N. Vieira, The Role of Leptin and Adiponectin in Obesity-Associated Cognitive Decline and Alzheimer's Disease, Front. Neurosci., 2019, 12, 1027 CrossRef.
- R. H. Coker and R. R. Wolfe, Weight loss strategies in the elderly: a clinical conundrum, Obesity, 2018, 26(1), 22–28 CrossRef PubMed.
- J. M. Webster, L. J. Kempen, R. S. Hardy and R. C. Langen, Inflammation and skeletal muscle wasting during cachexia, Front. Physiol., 2020, 11, 597675 CrossRef.
- J. Gao, M. Deng, Y. Li, Y. Yin, X. Zhou and Q. Zhang,
et al., Resistin as a systemic inflammation-related biomarker for sarcopenia in patients with chronic obstructive pulmonary disease, Front. Nutr., 2022, 9, 921399 CrossRef.
- S. Docherty, R. Harley, J. J. McAuley, L. A. N. Crowe, C. Pedret and P. D. Kirwan,
et al., The effect of exercise on cytokines: implications for musculoskeletal health: a narrative review, BMC Sports Sci. Med. Rehabil., 2022, 14(1), 5 CrossRef PubMed.
- S. R. Hennigar, J. P. McClung and S. M. Pasiakos, Nutritional interventions and the IL-6 response to exercise, FASEB J., 2017, 31(9), 3719–3728 CrossRef CAS PubMed.
- É. Cerqueira, D. A. Marinho, H. P. Neiva and O. Lourenço, Inflammatory Effects of High and Moderate Intensity Exercise—A Systematic Review, Front. Physiol., 2020, 10, 1550 CrossRef PubMed.
- H. Kumagai, A. R. Coelho, J. Wan, H. H. Mehta, K. Yen and A. Huang,
et al., MOTS-c reduces myostatin and muscle atrophy signaling, Am. J. Physiol.: Endocrinol. Metab., 2021, 320(4), E680–E690 CrossRef CAS.
- W. J. Chen, Y. Liu, Y. B. Sui, H. T. Yang, J. R. Chang and C. S. Tang,
et al., Positive association between musclin and insulin resistance in obesity: evidence of a human study and an animal experiment, Nutr. Metab., 2017, 14(1), 46 CrossRef.
- H. Nishizawa, M. Matsuda, Y. Yamada, K. Kawai, E. Suzuki and M. Makishima,
et al., Musclin, a Novel Skeletal Muscle-derived Secretory Factor, J. Biol. Chem., 2004, 279(19), 19391–19395 CrossRef CAS PubMed.
- D. Máderová, P. Krumpolec, L. Slobodová, M. Schön, V. Tirpáková and Z. Kovaničová,
et al., Acute and regular exercise distinctly modulate serum, plasma and skeletal muscle BDNF in the elderly, Neuropeptides, 2019, 78, 101961 CrossRef.
- C. Clow and B. J. Jasmin, Brain-derived Neurotrophic Factor Regulates Satellite Cell Differentiation and Skeltal Muscle Regeneration, Mol. Biol. Cell, 2010, 21(13), 2182–2190 CrossRef CAS PubMed.
- R. Fitts, S. Trappe, D. Costill, P. M. Gallagher, A. C. Creer and P. Colloton,
et al., Prolonged space flight-induced alterations in the structure and function of human skeletal muscle fibres, J. Physiol., 2010, 588(18), 3567–3592 CrossRef CAS PubMed.
- I. Amelio, F. Cutruzzolá, A. Antonov, M. Agostini and G. Melino, Serine and glycine metabolism in cancer, Trends Biochem. Sci., 2014, 39(4), 191–198 CrossRef CAS PubMed.
- R. Heidari, V. Ghanbarinejad, H. Mohammadi, A. Ahmadi, M. M. Ommati and N. Abdoli,
et al., Mitochondria protection as a mechanism underlying the hepatoprotective effects of glycine in cholestatic mice, Biomed. Pharmacother., 2018, 97, 1086–1095 CrossRef CAS PubMed.
- J. C. Fernández-Checa, A. Colell and C. García-Ruiz,
S-Adenosyl-L-methionine and mitochondrial reduced glutathione depletion in alcoholic liver disease, Alcohol., 2002, 27(3), 179–183 CrossRef PubMed.
- M. A. Razak, P. S. Begum, B. Viswanath and S. Rajagopal, Multifarious Beneficial Effect of Nonessential Amino Acid, Glycine: A Review. Essa MM, editor, Oxid. Med. Cell. Longevity, 2017, 2017, 1716701 Search PubMed.
- M. Marí, A. Morales, A. Colell, C. García-Ruiz and J. C. Fernández-Checa, Mitochondrial glutathione, a key survival antioxidant, Antioxid. Redox Signaling, 2009, 11(11), 2685–2700 CrossRef.
- A. Alves and B. Morio, Alterations in glycine metabolism in obesity and chronic metabolic diseases–an update on new advances, Curr. Opin. Clin. Nutr. Metab. Care, 2023, 26(1), 50–54 CrossRef.
- D. Monleon, R. Garcia-Valles, J. M. Morales, T. Brioche, G. Olaso-Gonzalez and R. Lopez-Grueso,
et al., Metabolomic analysis of long-term spontaneous exercise in mice suggests increased lipolysis and altered glucose metabolism when animals are at rest, J. Appl. Physiol., 2014, 117(10), 1110–1119 CrossRef CAS.
- L. Guimarães-Ferreira, Role of the phosphocreatine system on energetic homeostasis in skeletal and cardiac muscles, Einstein Sao Paulo, 2014, 12, 126–131 CrossRef.
- F. J. Fernandez-Gomez, M. F. Galindo, M. Gómez-Lázaro, V. J. Yuste, J. X. Comella and N. Aguirre,
et al., Malonate induces cell death via mitochondrial potential collapse and delayed swelling through an ROS-dependent pathway, Br. J. Pharmacol., 2005, 144(4), 528 CrossRef CAS.
- I. Choi, H. Son and J. H. Baek, Tricarboxylic acid (Tca) cycle intermediates: Regulators of immune responses, Life, 2021, 11(1), 69 CrossRef CAS PubMed.
- A. Andersson, A. Sjödin, R. Olsson and B. Vessby, Effects of physical exercise on phospholipid fatty acid composition in skeletal muscle, Am. J. Physiol.: Endocrinol. Metab., 1998, 274(3), E432–E438 CrossRef CAS PubMed.
- Y. C. Koay, K. Stanton, V. Kienzle, M. Li, J. Yang and D. S. Celermajer,
et al., Effect of chronic exercise in healthy young male adults: a metabolomic analysis, Cardiovasc. Res., 2021, 117(2), 613–622 CrossRef CAS PubMed.
- J. P. Warber, J. F. Patton, W. J. Tharion, S. H. Zeisel, R. P. Mello and C. P. Kemnitz,
et al., The effects of choline supplementation on physical performance, Int. J. Sport Nutr. Exercise Metab., 2000, 10(2), 170–181 CAS.
- S. B. Eaton and S. B. Eaton, Physical inactivity, obesity, and type 2 diabetes: an evolutionary perspective, Res. Q. Exerc. Sport, 2017, 88(1), 1–8 CrossRef.
- P. Hojman, C. Brolin, N. Nørgaard-Christensen, C. Dethlefsen, B. Lauenborg and C. K. Olsen,
et al., IL-6 release from muscles during exercise is stimulated by lactate-dependent protease activity, Am. J. Physiol.: Endocrinol. Metab., 2019, 316(5), E940–E947 CrossRef CAS.
- Z. Ye, S. Wang, C. Zhang and Y. Zhao, Coordinated Modulation of Energy Metabolism and Inflammation by Branched-Chain Amino Acids and Fatty Acids, Front. Endocrinol., 2020, 11, 617 CrossRef PubMed.
- M. M. Qaid and M. M. Abdelrahman, Role of insulin and other related hormones in energy metabolism—A review. González-Redondo P, editor, Cogent Food Agric., 2016, 2(1), 1267691 Search PubMed.
-
J. Bassett, Dietary and gastro-intestinal control of hormones regulating carbohydrate metabolism in ruminants. Dig. Metab. Rumin., 1975 Search PubMed.
- K. D. Galsgaard, M. Winther-Sørensen, J. Pedersen, S. A. S. Kjeldsen, M. M. Rosenkilde and N. J. Wewer Albrechtsen,
et al., Glucose and amino acid metabolism in mice depend mutually on glucagon and insulin receptor signaling, Am. J. Physiol.: Endocrinol. Metab., 2019, 316(4), E660–E673 CrossRef CAS.
- W. L. Holland, B. T. Bikman, L. P. Wang, G. Yuguang, K. M. Sargent and S. Bulchand,
et al., Lipid-induced insulin resistance mediated by the proinflammatory receptor TLR4 requires saturated fatty acid–induced ceramide biosynthesis in mice, J. Clin. Invest., 2011, 121(5), 1858–1870 CrossRef CAS PubMed.
- J. S. Burrill, E. K. Long, B. Reilly, Y. Deng, I. M. Armitage and P. E. Scherer,
et al., Inflammation and ER Stress Regulate Branched-Chain Amino Acid Uptake and Metabolism in Adipocytes, Mol. Endocrinol., 2015, 29(3), 411–420 CrossRef CAS.
- M. Sakurai, Y. Yamamoto, N. Kanayama, M. Hasegawa, A. Mouri and M. Takemura,
et al., Serum Metabolic Profiles of the Tryptophan-Kynurenine Pathway in the high risk subjects of major depressive disorder, Sci. Rep., 2020, 10(1), 1961 CrossRef CAS PubMed.
- G. L. Haverkamp, W. L. Loosman, C. F. Franssen, I. P. Kema, M. van Diepen and F. W. Dekker,
et al., The role of tryptophan degradation in the association between inflammatory markers and depressive symptoms in chronic dialysis patients, Nephrol., Dial., Transplant., 2017, 32(6), 1040–1047 CAS.
- D. Enko, S. Zelzer, J. Wenninger, S. Holasek, W. J. Schnedl and A. Baranyi,
et al., Interleukin-6 is associated with tryptophan metabolism and signs of depression in individuals with carbohydrate malabsorption, EXCLI J., 2020, 19, 1414 Search PubMed.
|
This journal is © The Royal Society of Chemistry 2024 |