DOI:
10.1039/D4MA00152D
(Paper)
Mater. Adv., 2024,
5, 3921-3928
Interpretable-machine-learning-guided discovery of dominant intrinsic factors of sensitivity of high explosives†
Received
16th February 2024
, Accepted 5th March 2024
First published on 28th March 2024
Abstract
Determination of sensitivity of high explosives (HEs) to different external loadings in a safe and rapid way is of great concern throughout the entire lifetime of a high explosive. However, sensitivity related research is still challenging due to the complexity of numerous physical–chemical processes that coexist under a transient external loading. Laser-induced plasma spectroscopy (LIPS) has emerged as a promising tool for detecting and characterizing explosives. Herein, we present an interpretable-machine-learning analytical approach for probing the potential intrinsic factors of sensitivity at the atomic and molecular levels using LIPS spectra and custom descriptors. The intrinsic natures of sensitivity are revealed as an attempt, which promotes a tight correlation between measured sensitivity and molecular structure, atomic proportions and electron transfer through statistical methods. Several factors, such as multi-core oil–water partition coefficient (Mlog
P), oxygen balance (OB), minimum value of partial charge (MinPC), aromatic index (AI), and emission intensity of CN radicals and C2 dimers, are found to be intimately related to sensitivity. By considering these factors, we can easily differentiate between sensitive and insensitive explosives. These findings highlight the significance of combining observed LIPS spectra and calculated atomic and molecular descriptors with machine learning to enhance the future analytical workflow for studying the sensitivity of explosives.
1. Introduction
As a special group of high-power energy release materials, high explosives (HEs) play an irreplaceable role in promoting the development of science, technology, and society. Significant progress has been achieved in developing novel high energy density materials, such as energetic extended solids,1 energetic ionic liquids,2 energetic metal organic frameworks,3 energetic co-crystals4 and energetic perovskites.5 However, HEs can be easily detonated under very low external stimuli, which has greatly hindered their application and development. Therefore, the accurate measurement, internal mechanism and influencing factors of different sensitivities are the leading scientific issues in the field of energetic materials. In terms of experimental aspect, the measurement of sensitivity is usually based on common standard methods established by countries or organizations, for instance, drop weight impact test6 for impact sensitivity and pendulum tribometer for friction sensitivity.7 Obviously, every measure of sensitivity requires specialized equipment and lengthy testing. With regard to the theoretical aspect, with the application of molecular simulation and the corresponding theoretical methods, it has been proved that the factors related to sensitivity include, but are not limited to, molecular and crystal level parameters, such as composition, geometric structure, electronic structure, molecular packing pattern, intermolecular interaction, crystal morphology, crystal size and so on. Delpuech et al.8,9 investigate the effect of molecular electronic structure on sensitivity. Zeman et al.10 found a linear relationship between the nuclear magnetic resonance (NMR) chemical shift and impact sensitivity as well as electric spark sensitivity. Zhang et al.11,12 elaborate on how an intrinsic structure like the crystal packing structure and its substructure affect sensitivity. Of course, non-intrinsic structures for example crystal defects are also associated with sensitivity.13 Notwithstanding all these attempts, there is still a scientific gap to build a bridge between those meaningful intrinsic material parameters and sensitivity obtained by measurement.
Nowadays, laser-induced micro-explosion technology14,15 including laser-induced plasma spectroscopy (LIPS)16–19 and laser-induced air shock from energetic materials (LASEM)20 has been considered as a promising high-throughput microscale experimental method for detonation study because the conditions in the laser-induced plasma are very similar to those in the chemical reaction zone behind the detonation wave of a detonating bulk explosive.21 The past few years have witnessed the unprecedented rapid development of fast performance prediction and chemical reaction analysis by the interaction of nanosecond pulsed laser interaction with small dose energetic materials on the microgram to milligram scale. Gottfried et al. came up with a laboratory-scale method for estimating the detonation velocity of explosives22,23 and the release of chemical energy in spatially programmed ferroelectrics24 from laser-induced shock waves. Our prior work25,26 has proved that effective radiation of laser-induced plasma is closely related to the sensitivity of HEs, and then naturally proposes a quantitative analysis of sensitivity based on LIPS spectra. Nevertheless, the relatively high accuracy with the sacrifice of interpretability makes the nature of the physical association between radiation spectra and sensitivity remain elusive.
As the extension of our previous work, a timesaving and resource-intensive approach based on collaborative analysis of LIPS spectral variables and theoretical descriptor variables by using interpretive machine learning (ML) models is discussed and represented by HEs, in particular, CL-20, HMX and so on. The current study focuses on the discovery of dominant intrinsic differences of explosive sensitivity by using SHapley Additive exPlanations (SHAP) and feature importance derived for the random forest (RF) classifier. We present a framework for quickly establishing a classification model of different sensitivities, including impact sensitivity, friction sensitivity, electrostatic sensitivity and laser sensitivity. It consists of three components: (1) feature extraction from observed LIPS spectra and calculated atomic and molecular descriptors, (2) ML model building, and (3) feature interpretation. With only 9 kinds of HEs measured and 16 kinds of structurally alike compounds used for comparison, we demonstrate several sensitivity classification tasks with an accuracy of R2 = 100%. The framework, together with feature importance analysis and domain expertise, could serve as a foundation for the accelerated development and understanding of sensitivity.
2. Materials and methods
2.1. Preparation and characterization of HEs
Nine kinds of single-compound HEs (particle size <500 μm, purity >99.5%) referred to as E1 to E9 were investigated in an open air environment. The impact sensitivity (IS) and friction sensitivity (FS) of HEs were measured by using a BAM fall hammer BFH-10 (Germany) and FSKM-10 BAM (Germany) friction apparatus, respectively. Electrostatic sensitivity (ES) was obtained by using a charge capacitance of 500 pF and an electrode gap length of 0.12 mm. The measurement of laser sensitivity (LS) was through our homemade laser-loaded micro-explosion setup.25 Sensitivity test results are given in Table 1. Note that when the explosives still fail to detonate under the maximum load of the instrument, their sensitivity values are marked as “>”. For instance, “>40” in Table 1 means that TNT, TATB, and DNAN are very insensitive to external impacts compared to other samples. The LIPS spectra are collected by a self-assembled device, which was described in our previous studies.27,28 In order to eliminate the influence of carbon ring bodies on molecular properties and expand the size of data set, two different types of organic ring compounds including eight kinds of energetic tetrazole ring-based organic high-nitrogen compounds marked as S1 to S8 and eight kinds of benzene ring organics marked as B1 to B8 were also investigated (Table S1, ESI†). 10–15 mg of fine pulverized compound was firmly pressed and evenly pasted on a double-sided tape (18 mm × 20 mm), and a spatula was used to press tightly and scrape off excess powders. LIPS acquisition parameters were optimized to obtain a high signal-to-noise ratio (SNR) with an excitation of 30 mJ, a time delay of 0.5 μs and an acquisition time of 30 μs. For each compound, totally 100 optical emission spectra are collected.
Table 1 A list of the HEs studied and their sensitivities
Sample |
Abbreviation |
Chemical name |
Impact sensitivity (IS, J) |
Friction sensitivity (FS, N) |
Electrostatic sensitivity (ES, mJ) |
Laser sensitivity (LS, J cm−2) |
E1 |
CL-20 |
Hexanitrohexaazaisowurtzitane |
2.0 |
64 |
601 |
17.02 |
E2 |
HMX |
Cyclotetramethylene tetranitramine |
4.5 |
80 |
763 |
33.89 |
E3 |
RDX |
Cyclotrimethylenetrinitramine |
6.5 |
112 |
760 |
29.68 |
E4 |
TNT |
Trinitrotoluene |
>40 |
355 |
2770 |
10.59 |
E5 |
FOX-7 |
1,1-Diamino-2,2-dinitroethylene |
11.0 |
360 |
1380 |
28.28 |
E6 |
TATB |
1,3,5-Triamino-2,4,6-trinitrobenzene |
>40 |
>360 |
1690 |
13.67 |
E7 |
LLM-105 |
2,6-Diamino-3,5-dinitropyrazine-1-oxide |
8.0 |
>360 |
1260 |
22.78 |
E8 |
DNAN |
2,4-Dinitroanisole |
>40 |
>360 |
1950 |
5.54 |
E9 |
NTO |
3-Nitro-1,2,4-triazole-5-one |
15 |
>360 |
1130 |
>70 |
2.2. Descriptors computational details
The calculation of atomic and molecular custom descriptors was accomplished by RDkit library29 based on simplified molecular input line entry specification (SMILES),30 including molecular weight (MolWt), number of hydrogen bond acceptor (NumHBA), number of hydrogen bond donor (NumHBA), multi-core oil–water partition coefficient (Mlog
P),31 Aromatic Index (AI) and so on. The crystal density (density) was obtained from the Cambridge Crystallographic Data Centre (CCDC).
2.3. Forming the data set
For spectroscopic data, the dimensionality of the feature space regularly outweighs the number of samples available for training, which requires feature reduction to avoid later misclassifications after the removal of abnormal spectra based on a 2σ-criterion.32 Therefore, 10 main spectral lines from the LIPS spectra resorting to domain spectral knowledge and 13 atomic and molecular custom descriptors were selected as features to feed into the subsequent model (Table S2, ESI†). These selected features can be divided into four major categories: atomic spectral features, molecular spectral features, atomic custom descriptors and molecular custom descriptors as shown in Table S3 (ESI†). Atomic spectral features are the emission intensities from atoms, such as carbon atoms, hydrogen atoms and so on, while molecular spectral features are the emission intensities from CN and C2 molecules. Atomic custom descriptors, including maximum value of partial charge (MaxPC) and minimum value of partial charge (MinPC), are often used for a qualitative understanding of the structure and reactivity of molecules.33 Molecular custom descriptors indicate molecular composition, topological shape and structure, such as oxygen balance (OB), BalabanJ, AI and so on. According to the responses of the HEs to different external stimuli, multiple binary classification tasks were constructed, including impact sensitive (impact sensitivity ≤40 J) vs. impact insensitive (impact sensitivity >40 J), friction sensitive (friction sensitivity ≤360 N) vs. friction insensitive (friction sensitivity >360 N), and laser sensitive (laser sensitivity ≤70 J cm−2) vs. laser insensitive (laser sensitivity >70 J cm−2). For electrostatic sensitivity, all the measure values are within the range. LLM-105 is one of the well-known electrostatic insensitive explosives,34 as well as FOX-7, TATB, TNT and so on. So a relatively reasonable value (1200 mJ) is considered to be the threshold for the division of sensitivity and insensitivity, ensuring that the above samples are classified as electrostatic insensitive. Subsequently, random sampling and averaging strategy was adopted to balance the number of samples of different categories and facilitate data augmentation (Fig. S1, ESI†). For the data matrix of each sample spectral, four spectra are randomly selected and averaged as a final spectrum. And then the above step was repeated until a sufficient amount of the desired spectra data matrix is obtained. The amount of final spectra is determined by the number of repeats. The sample sets of different categories and the final spectral quantities are shown in Table S4 (ESI†). Eventually, the training set, validation set and the prediction set were divided in a ratio of 3
:
1
:
1.
2.4. Machine learning models and feature interpretation
Sensitivity classification models were trained by random forest (RF) classifier implemented in the Scikit-learn package.35 The training data were fed to ML models, and the validation data needed to be well split by tuning multiple model parameters. And then, the prediction data were used to test the accuracy and applicability of the trained ML models. In order to further explore the interpretation of features, the feature importance directly derived from RF feature importance ranking and deduced from SHAP analysis by using the SHAP Python package36,37 was considered comprehensively. Prior to the analysis, Spearman rank correlation matrices38 of features were calculated to confirm that the features did not have very high correlations with each other, which could distort the analysis reliability (Fig. S2, ESI†). T-distributed stochastic neighbor embedding (t-SNE) was introduced for better visualization of feature space, which was a nonlinear dimensionality reduction technique proposed in 2008.39
3. Results and discussion
The overall framework and procedures for interpretable-ML-assisted analysis of the dominant factors of sensitivities are illustrated in Fig. 1. Initially, LIPS spectra collected from a homemade experimental device and atomic and molecular custom descriptors calculated by RDkit library are obtained (Fig. 1a). For lifting the “curse of dimensionality”, 10 spectral variables are carefully selected from more than ten thousand spectral variables as the effective spectral features, which are derived from the main particles of laser-induced plasma, such as carbon atoms, hydrogen atoms, and carbon nitrogen radicals, to name a few. Then, multiple sensitivity classification models are built through an RF classifier (Fig. 1b). Finally the ML model is further explained based on the organic fusion of SHAP framework, feature importance and feature visualization (Fig. 1c).
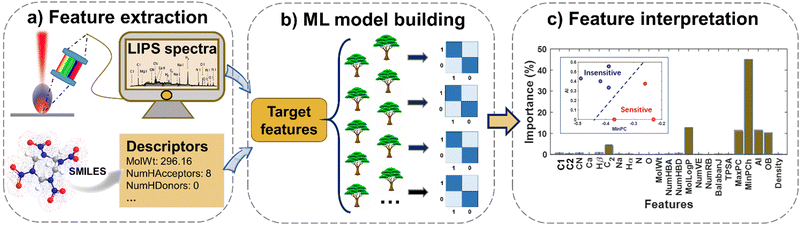 |
| Fig. 1 Overall framework for interpretable-ML-assisted analysis of sensitivities in this work. (a) Feature extraction from LIPS and atomic and molecular custom descriptors. (b) RF ML model building. (c) Feature interpretation from feature importance and SHAP analysis. | |
3.1. Feature extraction and visualization
Prototypical original LIPS spectra and molecular structures of HEs are shown in Fig. 2. According to the spectral database of the National Institute of Standards and Technology (NIST), the well-isolated characteristic spectral lines can be determined as shown in Fig. S3 (ESI†), including (i) the atomic emission from main elements, namely, carbon, hydrogen, oxygen, and nitrogen, (ii) the atomic or ionic emission from small amounts of impurity elements, such as magnesium, calcium and sodium, and (iii) the molecular emission from diatomic molecules for examples, CN violet system and C2 swan system. It can be seen that the emission lines from the LIPS spectra of different kinds of HEs are basically at the identical wavelength due to the same element composition, while the emission intensity varies greatly. This means that laser-induced plasmas of different HEs contain the same kind of particles, whereas the number of particles is very different. It has been proved that the LIPS spectra of explosives induced by pulsed laser reflect the number of different types of atoms, ions and other particles in the laser-induced plasma which is considered as “micro explosion”, and these particles are intrinsic to the electron transfer mechanism, elemental composition, oxygen balance, thermal properties and other factors of explosive. Coincidentally, these factors happen to be the key indicators affecting the sensitivity of explosives. Therefore, LIPS spectra may contain immense hidden information of sensitivity.25 10 spectral lines from the main emission particles of the laser-induced plasma are selected from 12
288 spectral variables as part of the target features. To study the effects of benzene and tetrazole rings, LIPS spectra of eight kinds of energetic tetrazole ring-based organic high-nitrogen compounds and eight kinds of benzene ring organics are also collected (Fig. S4, ESI†). Theoretically, since sensitivity and the intrinsic structure of atoms and molecules are inseparable, 13 calculated atomic and molecular custom descriptors by virtue of RDkit library are chosen. Histograms and probability density distribution of target features of all data sets are shown in Fig. S5 (ESI†). It reflects the great differences that exist between LIPS spectral features and descriptor features of different samples, and the correlation between the above features is not significant (Fig. S2, ESI†).
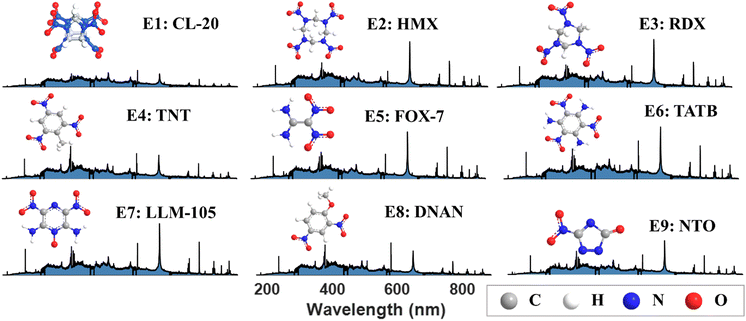 |
| Fig. 2 LIPS spectra and molecular structures of HEs utilized in this work. | |
Data visualization is often essential to design a good machine learning model. Due to nonlinear correlation between target features, a nonlinear dimensionality reduction algorithm named t-SNE is adopted. Fig. 3 shows the t-SNE plots of targets features of different sensitivity categories in the order of impact sensitivity, friction sensitivity, electrostatic sensitivity and laser sensitivity. In this figure, red clouds root in the HEs that are sensitive to certain external stimuli, on the contrary, blue clouds represent samples that are insensitive to certain external stimuli. The first point to note is that data points of each sample are gathered in a specific position in the low-dimensional space, but the samples with high sensitivity and low sensitivity cannot be simply separated linearly. Therefore, RF algorithm, which is a commonly used nonlinear algorithm, is employed to construct classification tasks.
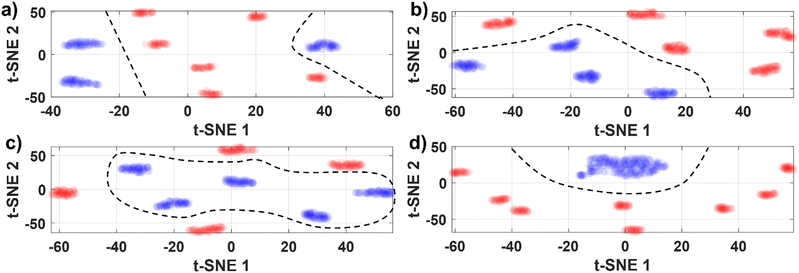 |
| Fig. 3 T-SNE plots of target features of different sensitivity categories. (a) Red clouds: impact sensitive (impact sensitivity ≤40 J) and blue clouds: impact insensitive (impact sensitivity >40 J). (b) Red clouds: friction sensitive (friction sensitivity ≤360 N) and blue clouds: friction insensitive (friction sensitivity >360 N). (c) Red clouds: electrostatic sensitive (electrostatic sensitivity ≤1200 mJ) and blue clouds: electrostatic insensitive (electrostatic sensitivity >1200 mJ). (d) Red clouds: laser sensitive (laser sensitivity ≤70 J cm−2) and blue clouds: laser insensitive. | |
3.2. Machine learning classification
Since its development by Breiman, RF has become a well-established supervised machine learning technique and is known to perform well for medium to large size data sets, while having only minimal requirements on data type and feature correlation.40 In this work, four sensitivities related binary classification tasks are established by RF, which are impact sensitive samples (samples with high sensitivity) vs. impact insensitive samples (samples with low sensitivity), friction sensitive samples (samples with high friction sensitivity) vs. friction insensitive samples (samples with low friction sensitivity), electrostatic sensitive samples (samples with high electrostatic sensitivity) vs. electrostatic insensitive samples (samples with low electrostatic sensitivity), and laser sensitive samples (samples with high laser sensitivity) vs. laser insensitive samples (samples with low laser sensitivity). To address the issue of uneven sample quantity in a binary classification task, a data extraction strategy is cleverly proposed as described in the Materials and methods section and Fig. S1 (ESI†). The RF models are trained using 23 target features as input with the dominating model parameters n_estimators = 201 and max_features = 10. It means that a small segment of features (≤10) is randomly selected to build a decision tree. After bootstrap sampling of the original training set, 201 decision trees are constructed. The final RF model and prediction results are obtained by voting or averaging. And then randomly divided validation set data are applied to check the accuracy of the training model. Following this, the prediction data could be recognized quickly through using the final RF model. As can be seen from the confusion matrix figures of the prediction results by using the well-trained classification models of impact sensitive, friction sensitivity, electrostatic sensitivity and laser sensitivity (Fig. 4), all of the predicted samples are accurately identified into their respective sensitivity categories with the classification accuracy of 100% as we expected.
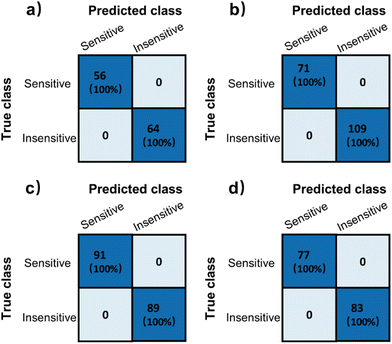 |
| Fig. 4 Confusion matrix figures of prediction results. (a) Impact sensitivity. (b) Friction sensitivity. (c) Electrostatic sensitivity. (d) Laser sensitivity. | |
3.3. Machine learning interpretation
For deeply explaining the ML model and analyzing the key features that influence different sensitivities, multiple strategies are adopted comprehensively, including SHAP framework, feature importance and feature visualization. Considering the impact sensitivity model as an example, the overall feature importance analysis is exhibited in Fig. 5. From the mean SHAP values of different classes shown in Fig. 5a, it can be concluded that Mlog
P, OB and density are the most influential factors in impact sensitivity. Moreover, the spectral intensities of C2 and CN occupy a large percentage as well. Fig. 5b shows the definite SHAP value of the top 10 influential features. It can be seen that impact sensitivity is inversely proportional to Mlog
P, C2 and CN. However, it is positively correlated with OB. To further intuitively investigate the reliability of feature analysis, feature importance is derived from the RF model as shown in Fig. 5c. The results of the interpretation of the RF model by the two methods are basically the same, only a little difference exists in the descriptor of density. Ultimately, Mlog
P, OB, C2 and CN are considered as the most important features. Sample data distribution in the feature space of Mlog
P and OB, C2 and CN are arranged in Fig. 5d and e, respectively. There is sufficient evidence to show that HEs with high (sensitive) or low (insensitive) impact sensitivity can be easily distinguished in the feature space of Mlog
P and OB, whereas there is some overlap in the spectral space of C2 and CN. It's worth noting that NTO mixed with insensitive materials has a relatively lower impact sensitivity in comparison with other sensitive HEs, such as CL-20 and HMX. All the findings clearly prove the fact that the impact insensitive HEs tend to have a higher level of Mlog
P, a lower level of OB, and more intense emission intensities of C2 and CN in the laser-induced plasmas compared to those with high impact sensitivity. The above results can be interpreted as follows: (i) the laser-induced plasma radiation intensity, especially the molecular emission bands like C2 and CN, is usually weaker in samples with higher impact sensitivity as reported before.21 This is because HEs with higher sensitivity are more likely to undergo chemical reactions under external impact stimuli, that is, the molecular dissociation in the laser-induced plasma is more thorough, and a large number of particles participate in non-radiative chemical reactions rather than radiative transitions, so weaker radiation intensity is observed. Nevertheless, a large number of excited particles in the laser-induced plasma of insensitive explosives are likely to return to the ground state and radiate photons instead of attending to chemical exothermic reactions. On the other hand, the excitation temperature of laser-induced plasma of insensitive explosives is lower, which will be in favor of the molecular formation.41 (ii) The amino, nitro and other explosive groups of sensitive explosives are conducive to hydrogen bond formation and the degree of functional group ionization is large, which are beneficial to their hydrophilicity, so Mlog
P is negative and the liposolubility is poor. In addition, chemical compounds with benzene rings as the basic skeleton are usually more liposoluble and have a lower proportion of oxygen, i.e. high Mlog
P and lower OB. It is not difficult to find that the impact insensitive HEs always contain benzene rings such as TNT, TATB and DNAN, as is demonstrated in Table 1 and Fig. 2. Therefore, we infer that the molecular structure–benzene ring—may have explained why some HEs studied in this work are insensitive to impact. It is assumed that external impact stimulus may be transformed into electron delocalization inside the benzene ring owing to the existence of a π bond, which plays a role in buffering the external energy injection, improving the molecular stability and reducing the molecular impact sensitivity.
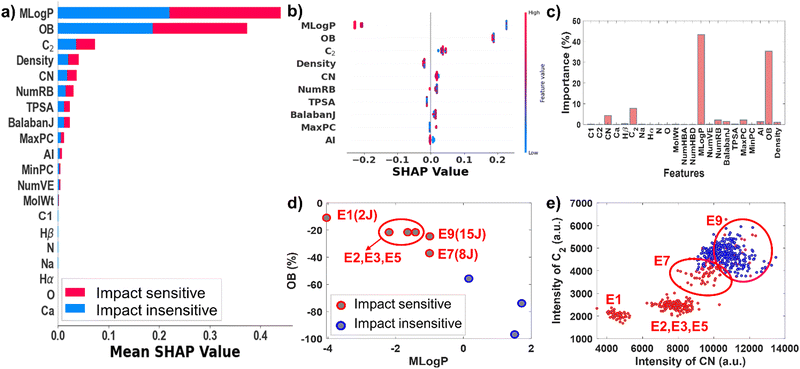 |
| Fig. 5 Feature importance analysis of impact sensitivity classification model. (a) Mean SHAP value analysis of the training data set samples and the RF model. (b) SHAP analysis of the training data set samples and the RF model. (c) Feature importance directly reduced from the RF model. (d) Sample data distribution in the custom operator feature space of Mlog P and OB. (e) Sample data distribution in the spectral feature space of C2 and CN. | |
For the purpose of proving the influence of the benzene ring structure on impact sensitivity, eight kinds of energetic tetrazole ring-based organic high-nitrogen compounds and eight kinds of benzene ring organics are added to HEs, and two additional classification tasks are constructed after expanding the sample size, that is, with benzene rings vs. without benzene rings and with tetrazole rings vs. without tetrazole rings (Table S5, ESI†). The results of their model predictions and interpretations are shown in Fig. S6 and S7 (ESI†). Evidently, Mlog
P and OB are key features for distinguishing whether the sample contains a benzene ring, while OB, MolWt and NumVE are pivotal features in determining tetrazole rings. It reflects that the benzene ring structure may contribute to reduce the sensitivity of the explosives to external impact loading. Feature importance analysis of other sensitivity classification models such as friction sensitivity, electrostatic sensitivity and laser sensitivity are shown in Fig. S8–S10 (ESI†). These figures lead us to the conclusion that MinPC, AI, emission intensities of C2 and CN are dominant features for identifying the friction sensitivity level of HEs. Partial atomic charges can be used to quantify the degree of ionic versus covalent bonding. The strength of chemical bond is closely related to the activity of chemical reaction. The weaker the bond, the stronger the chemical reaction. Therefore, MinPC is the minimum value of an atomic charge, which is related to chemical reactivity and electrostatic interaction between atoms. A higher value (lower negative charge) of MinPC means lower chemical reactivity, so external electrostatic application may lead to a concentration of injected energy or stress, making the material easy to be ignited or detonated. AI mentioned in this paper is denoted as the ratio of the number of aromatic atoms to the total number of atoms. As one of the evaluation indicators of aromaticity, the AI ranges between 0 and 1.42 The closer the aromatic index is to 1, the more aromatic and stable the molecule is, and the more insensitive to friction loading on the compound. Besides, there is a gradual decline of electrostatic sensitivity with the increase in OB and decrease in BalabanJ, which is a “topological shape” of molecules or of molecular fragments J to be known as a useful tool for quantitative structure–activity relationships proposed by Balaban.43 The negative association between electrostatic sensitivity and OB is consistent with our previous findings.25 Furthermore, NTO, which is the only laser-insensitive, high-energy material observed in our experiments has the lowest number of valence electrons, the lowest amount of rotatable chemical bonds and the highest aroma index. The related intrinsic mechanism still needs further research because of the limited number of HEs in this work.
For clarity, all the above results are summarized in Table 2. We found that molecular dependent features play a more important role in sensitivity analysis than atomic dependent features. It is noticeable that the emission intensities of C2 and CN only have a significant effect on mechanical sensitivity, i.e. impact sensitivity and friction sensitivity. It is reasonable to speculate that the degree of molecular dissociation and electron distribution has a great influence on the mechanical sensitivity of energetic molecules when they suffer from external impact or friction. It is also worth to point out that some of the atomic and molecular descriptors depended on the atomic and molecular structure combined with LIPS spectra may be a critical means to study the sensitivity mechanism and promote the quantitative analysis of sensitivity.
Table 2 Summary of the most important influencing factors of different sensitivities
Factors (features) |
Impact sensitivity |
Friction sensitivity |
Electrostatic sensitivity |
Laser sensitivity |
Mlog P ↑ |
Low (insensitive) |
— |
— |
— |
OB ↑ |
High (sensitive) |
— |
High (sensitive) |
— |
C2 ↑ |
Low (insensitive) |
Low (insensitive) |
— |
— |
CN ↑ |
Low (insensitive) |
Low (insensitive) |
— |
— |
MinPC ↑ |
— |
High (sensitive) |
— |
— |
AI ↑ |
— |
Low (insensitive) |
|
Low (insensitive) |
BalabanJ ↑ |
— |
— |
Low (insensitive) |
— |
NumVE ↑ |
— |
— |
— |
High (sensitive) |
NumRB ↑ |
— |
— |
— |
High (sensitive) |
4. Conclusions
In this work, an interpretable-machine-learning frame was developed and applied to guide the discovery of dominant intrinsic factors of sensitivity of HEs. First, LIPS spectra were recorded using our homebuilt apparatus, and atomic and molecular custom descriptors were calculated based on the molecular structure. Then, all the atomic and molecular custom descriptors and main intensities of main atomic and molecular radiation lines were picked out and used in RF classification models to predict the class of different sensitivities, including impact sensitive vs. impact insensitive, friction sensitive vs. friction insensitive, electrostatic sensitive vs. electrostatic insensitive, and laser sensitive vs. laser insensitive. Finally and foremost, SHAP framework and feature importance analysis from RF model were considered comprehensively for feature importance analysis to explore the dominant intrinsic factors of sensitivity of HEs. The primary conclusions of this work are summarized as follows:
(i) When the molecule contains benzene rings, it is easily soluble in non-polar solvents with high Mlog
P, and the OB is very negative, which is beneficial in reducing the impact sensitivity.
(ii) HEs with a dull sense of friction typically have a lower MinPC (more negative charge) and high aromaticity, which help convert external friction into intramolecular charge transfer and alleviate sensitivity.
(iii) For HEs that are not sensitive to mechanical stimulations including impact and friction, particles in their laser-induced plasma are prone to participate in radiative transitions instead of exothermic chemical reactions, resulting in brighter plasmas and higher radiation intensity, especially molecular emission.
(iv) Electrostatic sensitivity is found to be highly correlated with BalabanJ and OB. This indicates that the different reactions of HEs to electrostatic sparks seem to be closely tied to their topological structure and atomic composition.
(v) NTO, the only sample that is insensitive to 1064 nm laser pulses among the 9 types of HEs studied in this paper, has the lowest NumVE and NumRB, as well as the highest AI.
It should be noted that any kind of sensitivity is the result of a combination of indexes, including but not limited to the indicators investigated in this work. But all the above findings involved herein are expected to in principle facilitate the understanding of sensitivity and richen the insight into the nature of sensitivity with little sample consumption and low computational complexity. The analysis framework proposed here does not rely too much on prior domain knowledge and is therefore compatible with the study of the properties of other materials.
Author contributions
Xianshuang Wang: conceptualization, methodology, investigation, data curation, and writing – original draft. Yage He: data curation, investigation, and validation. Xinyu Zhang: formal analysis and validation. Maoxin Hu: investigation and validation. Wanzhu Zhao: data curation. Haohan Sun: investigation. Xiaoning Yang: investigation. Xiaodong Liu: validation. Ruibin Liu: writing – review & editing and project administration.
Conflicts of interest
There are no conflicts to declare.
Acknowledgements
The authors thank Key Lab of advanced optoelectronic quantum architecture and measurement (Ministry of Education), Beijing Key Lab of Nanophotonics & Ultrafine Optoelectronic Systems, State Key Laboratory of Explosion Science and Technology, Frontiers Science Center for High Energy Material (MOE), and School of Physics and School of Materials Science & Engineering for their support. The authors are very grateful for the support by National Key Research and Development Project (2018YFC2001100).
Notes and references
- M. J. Lipp, W. J. Evans, B. J. Baer and C.-S. Yoo, Nat. Mater., 2005, 4, 211–215 CrossRef CAS PubMed.
- Q. Zhang and J. n M. Shreeve, Chem. Rev., 2014, 114, 10527–10574 CrossRef CAS PubMed.
- S. Zhang, Q. Yang, X. Liu, X. Qu, Q. Wei, G. Xie, S. Chen and S. Gao, Coord. Chem. Rev., 2016, 307, 292–312 CrossRef CAS.
- J. C. Bennion and A. J. Matzger, Acc. Chem. Res., 2021, 54, 1699–1710 CrossRef CAS PubMed.
- S.-L. Chen, Z.-R. Yang, B.-J. Wang, Y. Shang, L.-Y. Sun, C.-T. He, H.-L. Zhou, W.-X. Zhang and X.-M. Chen, Sci. China Mater., 2018, 61, 1123–1128 CrossRef CAS.
-
J. C. Oxley, Explosive effects and applications, Springer, 1998, pp. 137–172 Search PubMed.
- R. Matyas, J. Selesovsky and T. Musil, J. Hazard. Mater., 2012, 213, 236–241 CrossRef PubMed.
- A. Delpuech and J. Cherville, Propellants, Explos., Pyrotech., 1978, 3, 169–175 CrossRef CAS.
- A. Delpuech and J. Cherville, Propellants, Explos., Pyrotech., 1979, 4, 121–128 CrossRef CAS.
- M. Jungova, S. Zeman and Q.-L. Yan, Cent. Eur. J. Energ. Mater., 2014, 11, 383–393 Search PubMed.
- G. Li and C. Zhang, J. Hazard. Mater., 2020, 398, 122910 CrossRef CAS PubMed.
-
C. Zhang, J. Huang and R. Bu, Intrinsic Structures and Properties of Energetic Materials, Springer Nature, 2023 Search PubMed.
- K. Zhong, R. Bu, F. Jiao, G. Liu and C. Zhang, Chem. Eng. J., 2022, 429, 132310 CrossRef CAS.
- X. Wang and R. Liu, Adv. Mater. Lett., 2023, 14, 2303 Search PubMed.
- X. Wang, Y. He, W. Cao, W. Guo, T. Zhang, J. Zhang, Q. Shu, X. Guo, R. Liu and Y. Yao, J. Mater. Chem. A, 2022, 10, 13114–13123 RSC.
- D. Rusak, B. Castle, B. Smith and J. Winefordner, TrAC, Trends Anal. Chem., 1998, 17, 453–461 CrossRef CAS.
- X. Wang, A. Li, N. Wazir, S. Huang, S. Guo, L. Liang, M. Zhang, B. Zou, Y. Hao and F. He, Opt. Express, 2018, 26, 13973–13984 CrossRef CAS PubMed.
- X. Wang, A. Li, X. Xu, Y. He, S. Qiu, X. Ma and R. Liu, Spectrochim. Acta, Part B, 2020, 174, 105996 CrossRef CAS.
- Y. He, X. Wang, Y. Ren, P. Chen, Y. Yao, R. Liu and R. Liu, Combust. Flame, 2023, 254, 112838 CrossRef CAS.
- J. L. Gottfried, AIP Conf. Proc., 2018, 1979, 100014 CrossRef.
- J. L. Gottfried, Phys. Chem. Chem. Phys., 2014, 16, 21452–21466 RSC.
- J. L. Gottfried, Propellants, Explos., Pyrotech., 2015, 40, 674–681 CrossRef CAS.
- J. L. Gottfried and E. J. Bukowski, Appl. Opt., 2017, 56, B47–B57 CrossRef CAS PubMed.
- Y. Hu, J. L. Gottfried, R. Pesce-Rodriguez, C.-C. Wu, S. D. Walck, Z. Liu, S. Balakrishnan, S. Broderick, Z. Guo and Q. Zhang, Nat. Commun., 2022, 13, 6959 CrossRef CAS PubMed.
- X. Wang, J. Wang, Y. Fu, R. Liu, Y. He, A. Li, D. Kong, W. Guo, Q. Shu and Y. Yao, J. Anal. At. Spectrom., 2021, 36, 2603–2611 RSC.
- X. Wang, R. Liu, Y. He, Y. Fu, J. Wang, A. Li, X. Guo, M. Wang, W. Guo and T. Zhang, Opt. Express, 2022, 30, 4718–4736 CrossRef CAS PubMed.
- Y. He, X. Wang, S. Guo, A. Li, X. Xu, N. Wazir, C. Ding, T. Lu, L. Xie and M. Zhang, Appl. Opt., 2019, 58, 422–427 CrossRef CAS PubMed.
- X. Wang, S. Wan, Y. He, S. Qiu, X. Ma, N. Wazir, R. Liu and Y. Tian, Spectrochim. Acta, Part B, 2021, 178, 106123 CrossRef CAS.
- A. P. C. Bento, A. Hersey, E. Flix, G. Landrum, A. Gaulton, F. Atkinson, L. J. Bellis, M. De Veij and A. R. Leach, J. Cheminf., 2020, 12, 1–16 Search PubMed.
- J. Arus-Pous, S. V. Johansson, O. Prykhodko, E. J. Bjerrum, C. Tyrchan, J.-L. Reymond, H. Chen and O. Engkvist, J. Cheminf., 2019, 11, 1–13 Search PubMed.
- R. Mannhold and H. Van de Waterbeemd, J. Comput.-Aided Mol. Des., 2001, 15, 337–354 CrossRef CAS PubMed.
- H. Cao, K. Zhou, X. Chen and X. Zhang, Int. J. Adv. Des. Manuf. Technol., 2017, 92, 4387–4397 CrossRef.
- J. Meister and W. Schwarz, J. Phys. Chem., 1994, 98, 8245–8252 CrossRef CAS.
-
B. Li, S. Li, Y. Zhao and Z. Li, The Performance Test and Application in Shaped Charge of Heat Resistance Explosive LLM-105, Atlantis Press, 2015, pp. 366–370 DOI:10.2991/meic-15.2015.85.
- F. Pedregosa, G. l Varoquaux, A. Gramfort, V. Michel, B. Thirion, O. Grisel, M. Blondel, P. Prettenhofer, R. Weiss and V. Dubourg, J. Mach. Learn. Res., 2011, 12, 2825–2830 Search PubMed.
- S. M. Lundberg, G. Erion, H. Chen, A. DeGrave, J. M. Prutkin, B. Nair, R. Katz, J. Himmelfarb, N. Bansal and S.-I. Lee, Nat. Mach. Intell., 2020, 2, 56–67 CrossRef PubMed.
- S. M. Lundberg and S.-I. Lee, Adv. neural inf. process. syst., 2017, 30 Search PubMed.
- F.-J. Lapointe and P. Legendre, Syst. Biol., 1992, 41, 378–384 CrossRef.
- L. Van der Maaten and G. Hinton, J. Mach. Learn. Res., 2008, 9, 2579–2605 Search PubMed.
- L. Breiman, Mach. Learn., 2001, 45, 5–32 CrossRef.
- F. Xu, S. Ma, C. Zhao and D. Dong, Front. Phys., 2022, 10, 7 Search PubMed.
- X. Wang, Y. He, Y. Zhang, A. Li, X. Zhang, X. Guo, T. Zhang, W. Guo, R. Liu and Y. Yao, J. Anal. At. Spectrom., 2023, 38, 2405–2413 RSC.
- A. T. Balaban, Chem. Phys. Lett., 1982, 89, 399–404 CrossRef CAS.
|
This journal is © The Royal Society of Chemistry 2024 |
Click here to see how this site uses Cookies. View our privacy policy here.