DOI:
10.1039/D4LC00230J
(Paper)
Lab Chip, 2024,
24, 3183-3190
Non-invasive hERG channel screening based on electrical impedance tomography and extracellular voltage activation (EIT–EVA)†
Received
14th March 2024
, Accepted 22nd May 2024
First published on 23rd May 2024
Abstract
hERG channel screening has been achieved based on electrical impedance tomography and extracellular voltage activation (EIT–EVA) to improve the non-invasive aspect of drug discovery. EIT–EVA screens hERG channels by considering the change in extracellular ion concentration which modifies the extracellular resistance in cell suspension. The rate of ion passing in cell suspension is calculated from the extracellular resistance Rex, which is obtained from the EIT measurement at a frequency of 500 kHz. In the experiment, non-invasive screening is applied by a novel integrated EIT–EVA printed circuit board (PCB) sensor to human embryonic kidney (HEK) 293 cells transfected with the human ether-a-go-go-related gene (hERG) ion channel, while the E-4031 antiarrhythmic drug is used for hERG channel inhibition. The extracellular resistance Rex of the HEK 293 cells suspension is measured by EIT as the hERG channels are activated by EVA over time. The Rex is reconstructed into extracellular conductivity distribution change Δσ to reflect the extracellular K+ ion concentration change Δc resulting from the activated hERG channel. Δc is increased rapidly during the hERG channel non-inhibition state while Δc is increased slower with increasing drug concentration cd. In order to evaluate the EIT–EVA system, the inhibitory ratio index (IR) was calculated based on the rate of Δc over time. Half-maximal inhibitory concentration (IC50) of 2.7 nM is obtained from the cd and IR dose–response relationship. The IR from EIT–EVA is compared with the results from the patch-clamp method, which gives R2 of 0.85. In conclusion, EIT–EVA is successfully applied to non-invasive hERG channel screening.
1 Introduction
Drug discovery is a multi-stage process where in the first stage, millions of drug compounds are evaluated for their effects on ion channels.1 The voltage-gated human ether-a-go-go-related gene (hERG) ion channel is one of the most highly screened ion channels in drug compound evaluation because of its abundance in body cells such as neurons and cardiomyocytes.2 In cardiomyocytes, hERG channel disruption prolongs the QT segment in the electrocardiogram (ECG).3–5 QT prolongation potentially causes a dangerous heart rhythm disorder called torsade de pointes (TdP), which can be fatal.6,7 Therefore, an accurate, reliable and high-throughput screening system for hERG channels is vital for a successful and efficient drug discovery.8,9
Traditional methods in hERG channel screening are the patch-clamp method and fluorescence microscopy.10 The patch-clamp method is the gold standard method due to its accuracy and high temporal resolution.11 Fluorescence microscopy has good spatial and temporal resolution.12 Despite their widespread use, the traditional methods for screening hERG channels have several problems, which are: (1) both patch-clamp and fluorescence microscopy methods are invasive to the cell under observation because the required membrane patching in the patch-clamp method can change the physical properties of the cells, while the required addition a dye in the fluorescence microscopy method can change the biochemical properties of the cells.13,14 (2) Both methods are also associated with high cost because the patch-clamp method requires specialized personnel while fluorescence microscopy requires specific fluorescent dyes.15,16 (3) Furthermore, in fluorescence microscopy, hERG channels are activated by pharmacological activation, which is too slow to reflect in the physiological time scale.17,18
To address the limitations of the traditional methods in hERG channel screening, electrical impedance methods have been proposed.19,20 Electrical impedance methods such as electrical impedance spectroscopy (EIS) and electrical impedance tomography (EIT) are non-invasive and low cost because no membrane patching and chemical dyes are required.21–23 EIS has been used in a previous study to distinguish cell types with similar structural features but varying intracellular components, such as the cytoplasm and nucleus, by evaluating ion transport to the extracellular region through ion channels.21 However, EIS is not able to capture the spatial information of the ion distribution in the extracellular region.
To measure the spatial information of ion transport and distribution, EIT has been used. For example, EIT was used to detect cellular heterogeneity within cell spheroids and to evaluate the anisotropic transmembrane transport coefficient vector of these cell spheroids.22,24 A significant advancement has been realized with EIT by successfully evaluating ion transport dynamics and offering insights into the spatiotemporal distribution of extracellular ion concentrations within cells modified with green fluorescent protein (GFP).25 Nevertheless, the main limitation that persists within the current EIT method is the absence of ion channel activation capability, which is an essential requirement for ion transport evaluation in hERG channel screening. Additionally, the existing EIT method encounters challenges in assessing inhibition levels, as it relies on ion transport models that exclusively consider open ion channels in GFP-labelled cells, while ion channel screening necessitates dynamic evaluations covering channel opening and blockage, which evolve over time.26
Based on these considerations, we introduce electrical impedance tomography with extracellular voltage activation (EIT–EVA) as a novel approach addressing the limitations of the existing EIT method. This innovative approach consists of two key innovations: the development of a novel integrated EIT–EVA PCB sensor, enabling non-invasive ion channel activation via extracellular voltage injection, and the introduction of an inhibitory ratio index based on impedance measurement, accommodating ion channel inhibition evaluation within its framework. Our study outlines three primary objectives:
1) Activation of hERG channels through extracellular voltage activation (EVA), coupled with the measurement of extracellular ion concentration using EIT.
2) Evaluation of ion transport through hERG channels using the inhibitory ratio (IR) index based on EIT measurement.
3) Validation of EIT–EVA results through a comprehensive comparative analysis with inhibitory response from previous studies, which used the patch-clamp method.
This work offers a new perspective on a non-invasive hERG channel screening method as it employs a novel miniaturized impedance-based sensor to move forward ion channel research and drug discovery processes.
2 Material and methods
2.1 Integrated EIT–EVA PCB sensor
Fig. 1 shows the overview of the electrical impedance tomography and extracellular voltage activation (EIT–EVA) system which is proposed to realize non-invasive hERG channel screening. Fig. 1(a) shows the schematic of the novel integrated EIT–EVA PCB sensor for imaging the ion concentration distribution outside the hERG channel. The novel integrated EIT–EVA PCB sensor consists of a circuit board, 16 printing electrodes for EIT measurement, one centre electrode for EVA activation, 17 electric lines, and 17 SMB jack connectors. As a preliminary step, only 8 printing electrodes for EIT are used. The circuit board is made of non-conductive epoxy glass fibre material (FR-4 TG130) with the dimensions of 100 mm × 70 mm × 1.6 mm embedded with four copper layers: one top copper layer, 2 inner copper layers for ground plane, and one bottom copper layer. The printing electrodes are made of copper coated by electroless nickel immersion gold (ENIG) with the dimensions of 0.4 mm (in length, le) × 0.09 mm (in width, we) × 4.5 μm (in thickness, the). The centre electrode is made of copper also coated by electroless nickel immersion gold (ENIG) in a circular shape with the dimension of 0.2 mm (in diameter, de). The effect of ENIG coating on the electrode performance is discussed further in the ESI† (S1). The electric lines are made of copper on the top copper layer and the bottom copper layer. Overlapped electric lines are separated on the two layers and connected by through-hole vias. The area outside the electric lines is covered by ground pour which is made of copper. SMB jack connectors are used to connect between the end of each electric line and the core line of the coaxial cables. The coaxial cables are used to connect the novel integrated EIT–EVA PCB sensor to a multiplexer, an impedance analyzer, and a function generator via a sensor switch. The eight outer lines of the coaxial cables are connected to each other and connected to the ground. Impedance control of 50 ohms is applied to the novel integrated EIT–EVA PCB sensor fabrication to match the impedance of the coaxial cables to maximize power transfer and minimize signal reflections. The 50 ohm impedance control was achieved by controlling the electric line width and thickness and the incorporation of ground planes on the two inner copper layers. Fig. 1(b) shows the photo of the novel integrated EIT–EVA PCB sensor and the detail of the sensing region captured by a stereoscopic microscope. The sensing region of the novel integrated EIT–EVA PCB sensor is an inner circle of the 16 printing electrodes with the diameter ds = 2.0 mm.
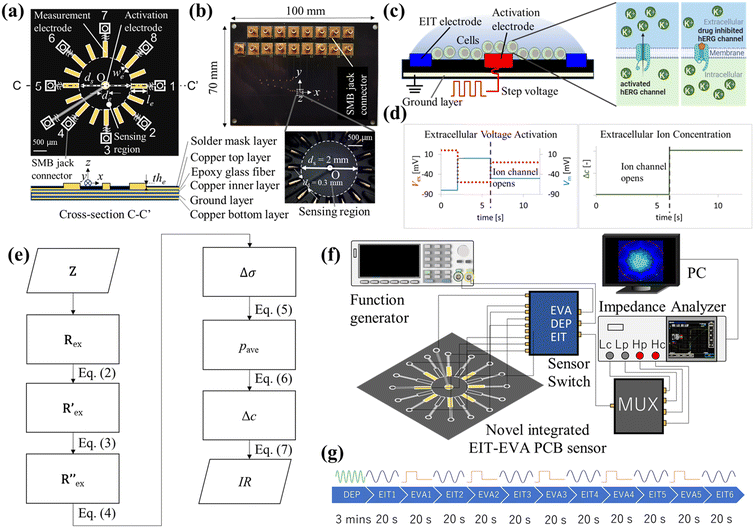 |
| Fig. 1 Overview of the EIT–EVA system: (a) schematic of the novel integrated EIT–EVA PCB sensor. (b) Camera photos of the novel integrated EIT–EVA PCB sensor. (c) Schematic of hERG channel activation by EVA. (d) Step voltage of EVA and the corresponding increase in the relative extracellular K+ ion concentration. (e) Flowchart of data processing for calculating inhibitory concentration index (IC). (f) Experimental setup of hERG channel screening by EIT–EVA. (g) Experiment timeline of DEP, EIT, and EVA. | |
2.2 Extracellular voltage activation
Fig. 1(c) shows the schematic of EVA. A step voltage is applied to the activation electrode on the novel integrated EIT–EVA PCB sensor. This application of extracellular voltage changes the potential distribution of the extracellular medium. The change in the potential distribution causes the change in cell membrane potential. A specific pattern of membrane potential change activates voltage-ion channels, which leads to ions being transported from the intracellular region to the extracellular region. When the ion channels are being blocked by some drug compounds, the ion transport due to activation becomes disrupted.
The conversion of extracellular activation voltage to membrane potential Vm is described by the following equation:
where
Vin is the potential inside the cell and
Vex is the potential outside the cell. Based on a previous study,
Vin has the value of −60 mV.
27
Fig. 1(d) shows the specific pattern of step voltage which is used by means of EVA to activate hERG channels. The rising step of Vm in the pattern forces the hERG channel to the inactive state. The falling step of Vm in the pattern opens and activates the hERG channel rapidly. The steps of the extracellular activation voltage are 20, −60, and −10 mV, respectively, which are corresponding to −80, 0 and −50 mV of membrane potential Vm. The activation of hERG channels by this specific pattern of EVA leads to a significant increase in extracellular potassium ion concentrations, which is detected by EIT measurement. For efficient activation and consistent response, cells need to be placed near the activation electrode. The design of the novel integrated EIT–EVA PCB sensor incorporates this cell gathering function by means of dielectrophoresis (DEP).
2.3 EIT measurement of extracellular ion concentration
Fig. 1(e) shows the flowchart of the data processing for EIT measurements of extracellular ion concentration. The raw data is the complex impedance Z obtained from the impedance analyzer, which consists of M electrode patterns. The resistance Rex is the real part of Z, which is extracted at the frequency f = 500 kHz described as follows:
Rex = [R1ex, R2ex…RMex] ∈ M |
where Rex is considered to be the resistance in the extracellular region, which is affected by the extracellular ion concentration.21 To improve data consistency and result interpretability, Rex is normalized by the following equation: | 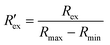 | (2) |
where Rmax and Rmin are the maximum and minimum values of Rex. In order to remove the effect of other ion channels, baseline-subtracted normalized resistance
is calculated using the following equation: |  | (3) |
where
is the normalized resistance under baseline measurement. Baseline measurement is defined as EIT measurement without the activation of hERG channels by EVA.
Then, the conductivity distribution change over time Δσ is calculated from
by solving the following equation using the Gauss–Newton algorithm:28
|  | (4) |

is the change of

over time.
J is the Jacobian matrix obtained by the EIDORS module in MATLAB (MathWorks, Natick, Massachusetts, USA). As explained in a previous study, Δ
σ has been proven to be equivalent to the change in extracellular ion concentration.
25
2.4 Inhibitory ratio index based on EIT–EVA
Fig. 1(e) also shows the flowchart of data processing to evaluate the effect of the drug on ion channel inhibition based on the EIT image. The mean gray value (pave) is obtained by averaging the conductivity distribution change Δσ in the sensing region using the following equation: |  | (5) |
Δσn is the nth pixel intensity of the conductivity distribution change Δσ. N is the number of pixels in the sensing region.
The gradient of pave after the 5th EVA activation in the timeline (EIT6) is calculated to obtain the ion concentration change (Δc) using the following equation:
| Δc = pEIT6ave − pEIT5ave | (6) |
pEIT6ave is
pave after the fifth EVA activation while
pEIT5ave is
pave before the fifth EVA activation. The inhibitory ratio index (IR) is calculated as the ratio between Δ
c and Δ
c in the control case Δ
ccd=0 nM described in the following equation:
|  | (7) |
3 Experiments
3.1 Experimental setup
Fig. 1(f) shows an experimental setup which consists of the novel integrated EIT–EVA PCB sensor which was connected to an impedance analyzer (IM3570, HIOKI, Japan) and a function generator (SDG1032X, Siglent, USA) via a multiplexer and a sensor switch. The eight printing electrodes are connected to the multiplexer to implement 28 pairs of two-wire impedance measurement. The function generator was connected to a centre electrode, which was used to activate the ion channel by extracellular voltage injection (EVA). The function generator was also connected to the surrounding electrodes for gathering the cells in the centre electrode through dielectrophoresis (DEP). The sensor switch was used to change the novel integrated EIT–EVA PCB sensor connection to DEP, EIT or EVA according to the experiment timeline. A PC was used to control the impedance analyzer, multiplexer, and function generator hardware, to store measurement results, to calculate the inhibitory ratio (IR) by Python programming, and to perform image reconstruction by MATLAB EIDORS.
3.2 Experimental conditions and methods
The hERG channel transiently expressed HEK293 cells (Thermo Fisher Scientific, Waltham, MA) were prepared using a transfection method. The plasmids harboring the hERG channel cDNA constructed previously were transfected into Expi293F (HEK293) cells.29 50–55 hours after transfection, the cells were measured using the trypan blue exclusion method with a Countess II (Thermo Fisher Scientific, Waltham, MA, USA) and diluted with HE-200 CD medium (Gmep Inc. Fukuoka, Japan) to a 1 × 106 cells per mL concentration. The experiments were performed within 6 hours to ensure that cell viability was not affected. Previous studies have also shown that EIT measurement and voltage activation of hERG channels do not influence cell viability.17,21
hERG channel inhibition was achieved by mixing E-4031 drug (Thermo Fisher Scientific, Waltham, MA, USA), a known blocker of hERG channels, with various concentrations cd to the cell suspension. The mixed suspension was left for 10 minutes before starting EIT–EVA measurement.30
A cell suspension amount of 10 μL was placed in the sensing region of the EIT–EVA PCB sensor using a micropipette.
Fig. 1(g) shows the timeline of the experiment. DEP was applied to the cell suspension for 3 minutes according to a previous study and a preliminary experiment.31 During the DEP procedure, the 8 printing electrodes were connected to a sine wave with frequency of 10 MHz and amplitude of 20 Vpp from the function generator, while the center electrode was connected to the ground to realize positive DEP (pDEP) configuration.32 Electric field simulation of the pDEP is discussed in the ESI† (S2). Then, EIT measurement was performed for 20 seconds. Next, the EVA activation to activate the hERG channels was applied for 20 seconds between EIT measurements. In total, 5 cycles of EVA and 6 cycles of EIT were implemented for each cell suspension. The measurement for each cell suspension was repeated three times. The effect of evaporation from long measurement time is discussed in the ESI† (S3). Table 1 shows the detailed experimental conditions.
Table 1 Experimental conditions
Parameter |
Value |
HEK293 cell concentration cc [cells per mL] |
1 × 106 |
Cell suspension volume vs [μL] |
10 |
E-4031 drug concentration cd [nM] |
0, 1, 3, 10, 30, 100 |
Temperature T [°C] |
25 |
DEP frequency fDEP [MHz] |
10 |
DEP voltage VDEP [Vpp] |
20 |
EIT measurement pattern Me [—] |
28 |
EIT injection current IEIT [μA] |
10 |
EIT frequency fEIT [kHz] |
500 |
4 Results
4.1 Measurement results
Fig. 2(a) shows results from the hERG channel activation experiment. The normalized extracellular resistance
when no extracellular voltage activation (EVA) was given is compared to
when EVA was given. The data shown is from measurement pattern 4, which is an opposite pair of electrodes 1 and 5. Fig. 2(a) shows a rapid decrease of the normalized extracellular resistance
when EVA was applied, while the normalized extracellular resistance
slightly decreases when EVA was not applied. The normalized extracellular resistance without EVA (baseline)
is decreased with time because of ion transport of endogenous ion channels in HEK-293 cells other than hERG, which are exchanging ions on a regular basis.33
for various drug concentrations are compared and discussed in the ESI† (S4).
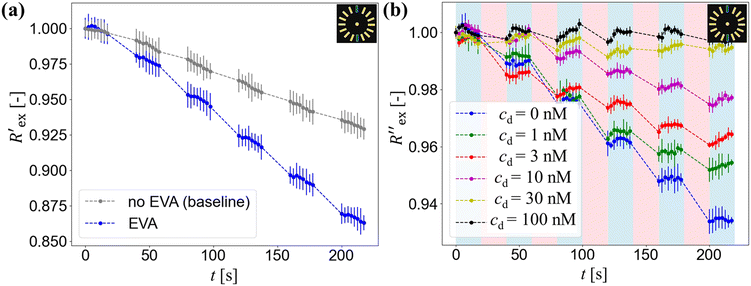 |
| Fig. 2 EIT measurement results: (a) normalized resistance of EIT measurements with (blue curve) and without EVA activation (black curve). (b) Baseline-subtracted resistance of the cell suspension without drug (0 nM) and the cell suspension with different drug concentrations of 1, 3, 10, 30, and 100 nM. | |
Fig. 2(b) shows the normalized extracellular resistance after subtraction with baseline
for different E-4031 drug concentrations cd = 0 nM, 1 nM, 3 nM, 10 nM, 30 nM and 100 nM. The shown data is from pair 4 of the EIT measurement which is an opposite pair. Measurements from an opposite pair better reflect the extracellular ion distribution because the measurements go through the centre electrode where the cells gather. In Fig. 2(b), the higher the drug concentration cd, the slower the decrease of
, which corresponds to less ions being transported from the intracellular region to the extracellular region due to the higher number of hERG channels being blocked by the drug.
4.2 EIT results
Fig. 3 shows the conductivity distribution change Δσ of the case without drug (cd = 0 nM) and the cases with different drug concentrations cd = 1, 3, 10, 30 and 100 nM over time t. Δσ is relative to the initial conductivity distribution at t = 0 s. As t progresses, the case without drug (cd = 0 nM) shows higher Δσ than the cases with drug. As the drug concentration increases, Δσ decreases when compared across the same time points. This is due to the decrease of K+ ions in the extracellular region Δc transported from the intracellular region, which is caused by more hERG channel inhibition. At drug concentration cd = 100 nM, Δσ is very small due to almost complete blocking of hERG channels by E-4031 drug.
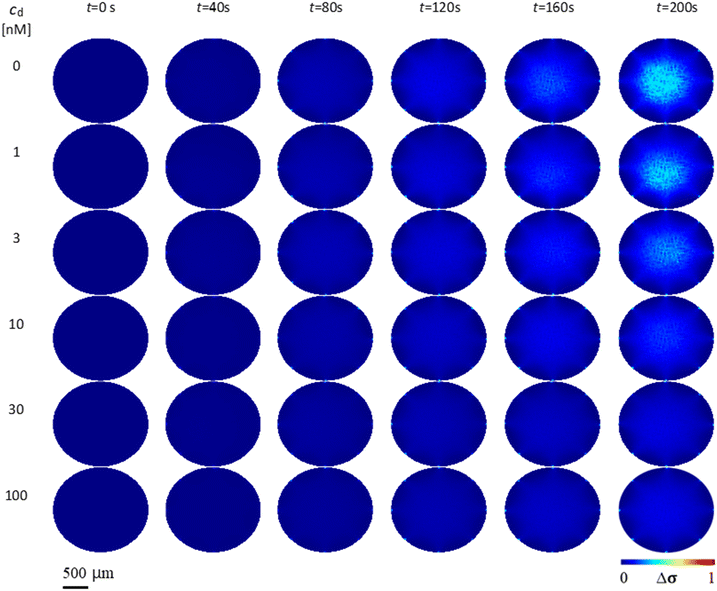 |
| Fig. 3 Mean gray value intensity pave over time of the extracellular conductivity distribution change between the cell suspension without drug (0 nM) and with different drug concentrations cd of 1, 3, 10, 30, and 100 nM. | |
5 Discussion
In Fig. 2(a), the rapid decrease in the normalized extracellular resistance
corresponds to the rapid increase of extracellular ion change Δc due to the hERG channel activation by EVA, while the slight decrease when EVA was not applied corresponds to ion transport phenomena which comes from other ion channels. This is confirmed by a previous study which investigated the endogenous ion channels expressed in HEK293 cells and their effects on ion channel screening results.33
in Fig. 2(b) shows a slight increase during an EIT measurement of 20 seconds although no ion transport was happening involving hERG channels, while the effect of other ion channels was already cancelled by the baseline subtraction described in eqn (3). This slight increase is considered to come from ion diffusion governed by Fick's law as reported in a previous study.25
Fig. 4 shows the mean gray value intensity pave over time from the conductivity distribution change Δσ of different drug concentrations cd = 0, 1, 3, 10, 30 and 100 nM. Since pave is the average of pixel intensity within the sensing region, the higher pave corresponds to the higher extracellular K+ ion concentration increase Δc around the hERG channels in the HEK293 cell suspension, while the lower pave corresponds to the lower extracellular K+ ion concentration increase Δc.
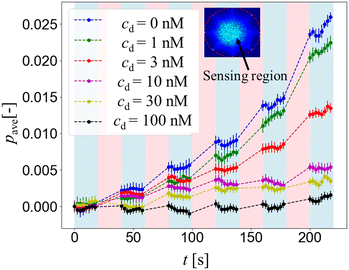 |
| Fig. 4 Comparison of conductivity distribution change Δσ of the extracellular region between the cell suspension without drug (0 nM) and the cell suspension with different drug concentrations cd of 1, 3, 10, 30, and 100 nM. | |
Fig. 5(a) shows the inhibition ratio across EVA activation number 1 through 5 defined in Fig. 1(g). The inhibition ratio was calculated according to eqn (7). The rate of pave increase depends on the activation number and the drug concentration. After one to three times of activation, the hERG channel inhibition has only slowly taken place when low drug concentration is added, while after the fourth activation the inhibition of all drug concentrations reach steady-state where the hERG channels are blocked by the available drug compounds.30 After activation 4 (EVA4), the inhibition ratio started to have a good fit with the sigmoid dose–response function governed by the Hill equation:34
| 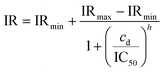 | (8) |
IR
min is the inhibitory ratio when the effect of the drug reaches saturation level. IR
max is the control value when no drug is being used. IC
50 is the drug inhibitory concentration at half of the control value IR
max.
h is the Hill coefficient which indicates the slope of the sigmoid function. Furthermore, inhibition ratio after EVA5 shows the best fit with the dose response function. Therefore, the extracellular K
+ ion concentration change Δ
c data was taken at
t = 200 s which was after five cycles of extracellular voltage activation (EVA5).
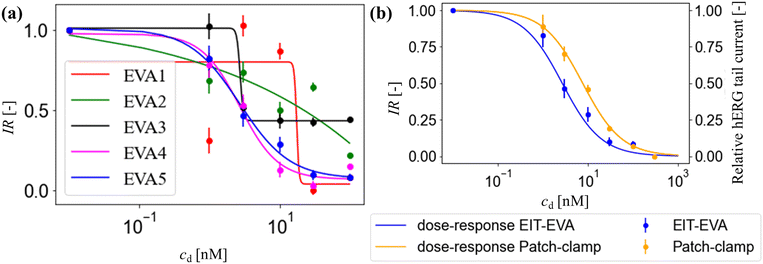 |
| Fig. 5 Inhibition ratio IR over different drug concentrations cd: (a) IR at different activation numbers from 1 (EVA1) to 5 (EVA5). (b) Comparison of IR at EVA5 with relative hERG tail current obtained from the patch-clamp method adapted from a previous study by Z. Zhou, Q. Gong, B. Ye, Z. Fan, J. C. Makielski, G. A. Robertson and C. T. January, Biophys. J., 1998, 74, 238. Copyright © 1998 The Biophysical Society. Published by Elsevier Inc. All rights reserved. | |
Fig. 5(b) shows the IR at EVA5 which is the hERG response to different E-4031 doses: cd = 0 nM, 1 nM, 3 nM, 10 nM, 30 nM, and 100 nM in blue dots. The response is calculated as a ratio of extracellular K+ ion concentration change Δc compared to the control value which is the case when cd = 0 nM. The IC50 obtained from this response is 2.7 nM.
The calculation of patch-clamp results which investigate the inhibition of hERG channel by the E-4031 drug reported that the IC50 value from the dose-response function is 7.7 nM.30,35 The hERG tail current in the study by Zhou et al. was recorded at −50 mV after activating the channel using a 4 s-long depolarizing pulse to 0 mV from a holding potential of −80 mV.30Fig. 5(b) shows the comparison of IR obtained from EIT–EVA with the relative hERG tail current obtained from the patch-clamp method, which gives a very close fit with the value of R2 of 0.85. Different IC50 values between EIT–EVA and the patch-clamp method may come from different measurement methods between single channel measurement achieved by the patch-clamp method and the average of multiple channel measurement achieved by EIT–EVA.36 More importantly, the value difference is still within the range of other IC50 values reported in previous studies.37,38
Based on the results of this study, EIT–EVA has successfully distinguished the uninhibited from inhibited hERG channels with regards to potassium ion transport. A limitation of the EIT–EVA method is that the ion channel state during its activation is unknown. This is due to non-simultaneous activation and measurement steps of the current EIT–EVA system. Another limitation of this method lies in multi-ion channel assay evaluation. This limitation comes from the fact that the current EIT–EVA system does not distinguish between different ion types. Further investigation is needed to realize simultaneous activation and measurement by considering hERG channel ion flux to evaluate the ion channel state under activation and to verify EIT–EVA ability to evaluate multi-ion channel assay and heterogeneous response of cells by considering the system spatial resolution.
Conclusions
This study achieved three main points as follows:
1. Extracellular voltage activation of hERG channels was achieved using an integrated EIT–EVA PCB sensor for ion channel activation and EIT measurement of extracellular ion concentration.
2. Inhibition ratio based on EIT–EVA was established in order to evaluate the hERG channel inhibition response. IC50 of 2.7 nM was obtained from the inhibition ratio dose–response function.
3. Electrical impedance tomography with extracellular voltage activation (EIT–EVA) for non-invasive hERG channel screening was achieved, which shows the comparable IC50 value with a previous patch-clamp study with the correlation value of 0.85.
Compared with the patch-clamp method and fluorescence imaging method, the EIT–EVA method provides an alternative as a non-invasive way to screen hERG channels, which is essential in drug discovery processes.
Author contributions
Muhammad Fathul Ihsan: methodology, investigation, data curation, software, formal analysis, validation, visualization, and writing – original draft. Daisuke Kawashima: conceptualization, funding acquisition, project administration, and writing – review & editing. Songshi Li: writing – review & editing. Satoshi Ogasawara: resources and writing – review & editing. Takeshi Murata: resources. Masahiro Takei: funding acquisition, supervision, and writing – review & editing.
Conflicts of interest
There are no conflicts to declare.
Acknowledgements
This work was supported by grants from the Japan Society for the Promotion of Science Grants-in-Aid for Scientific Research-Young Researchers (No. JP23K17186), the Japan Society for the Promotion of Science Grants-in-Aid for Scientific Research-Challenging Research (No. JP23K18571) and the Japan Science and Technology Agency-Support for Pioneering Research Initiated by the Next Generation (No. JPMJSP2109).
References
- H. Aldewachi, R. N. Al-Zidan, M. T. Conner and M. M. Salman, Bioengineering, 2021, 8, 1–20 CrossRef PubMed.
- J. I. Vandenberg, M. D. Perry, M. J. Perrin, S. A. Mann, Y. Ke and A. P. Hill, Physiol. Rev., 2012, 92, 1393–1478 CrossRef CAS PubMed.
- A. Dennis, L. Wang, X. Wan and E. Ficker, Biochem. Soc. Trans., 2007, 35, 1060–1063 CrossRef CAS.
- M. Recanatini, E. Poluzzi, M. Masetti, A. Cavalli and F. De Ponti, Med. Res. Rev., 2005, 25, 133–166 CrossRef CAS.
- H. J. Witchel, Cardiovasc. Ther., 2011, 29, 251–259 CrossRef CAS.
- K. Finlayson, H. J. Witchel, J. McCulloch and J. Sharkey, Eur. J. Pharmacol., 2004, 500, 129–142 CrossRef CAS PubMed.
- J. C. Hancox, M. J. McPate, A. El Harchi and Y. Hong Zhang, Pharmacol. Ther., 2008, 119, 118–132 CrossRef CAS PubMed.
- G. Gintant, P. T. Sager and N. Stockbridge, Nat. Rev. Drug Discovery, 2016, 15, 457–471 CrossRef CAS PubMed.
- W. Haverkamp, G. Breithardt, A. J. Camm, M. J. Janse, M. R. Rosen, C. Antzelevitch, D. Escande, M. Franz, M. Malik, A. Moss and R. Shah, Eur. Heart J., 2000, 21, 1216–1231 CrossRef CAS PubMed.
- H. B. Yu, M. Li, W. P. Wang and X. L. Wang, Acta Pharmacol. Sin., 2016, 37, 34–43 CrossRef CAS PubMed.
- D. J. Gillie, S. J. Novick, B. T. Donovan, L. A. Payne and C. Townsend, J. Pharmacol. Toxicol. Methods, 2013, 67, 33–44 CrossRef CAS.
- T. Pan, M. Shen, J. Shi, J. Ning, F. Su, J. Liao and Y. Tian, Sens. Actuators, B, 2021, 345, 1–10 Search PubMed.
-
O. B. Mcmanus, M. L. Garcia, D. Weaver, M. Bryant, S. Titus and J. B. Herrington, in The Assay Guidance Manual, ed. S. Markossian, Eli Lilly & Company and the National Center for Advancing Translational Sciences, Bethesda (MD), 2012, ch. Ion Channel Screening, pp. 1–23 Search PubMed.
- B. A. Maki, K. A. Cummings, M. A. Paganelli, S. E. Murthy and G. K. Popescu, J. Visualized Exp., 2014, 88, e51629 Search PubMed.
- N. J. Willumsen, M. Bech, S.-P. Olesen, B. S. Jensen, M. P. G. Korsgaard and P. Christophersen, Recept. Channels, 2003, 9, 3–12 CrossRef CAS.
- W. Zheng, R. H. Spencer and L. Kiss, Assay Drug Dev. Technol., 2004, 2(5), 543–552 CrossRef CAS PubMed.
- P. Burnett, J. K. Robertson, J. M. Palmer, R. R. Ryan, A. E. Dubin and R. A. Zivin, J. Biomol. Screening, 2003, 8, 660–667 CrossRef CAS.
- M. A. Gómez-Herrera, E. Patlán, A. Estrada-Garrido, A. Hernández-Cruz and E. Luis, Front. Pharmacol., 2023, 14, 1–11 Search PubMed.
- N. Gupta, V. Renugopalakrishnan, D. Liepmann, R. Paulmurugan and B. D. Malhotra, Biosens. Bioelectron., 2019, 141, 111435 CrossRef CAS PubMed.
- A. Han and A. B. Frazier, Lab Chip, 2006, 6, 1412–1414 RSC.
- D. Kawashima, S. Li, H. Obara and M. Takei, IEEE Trans. Biomed. Eng., 2021, 68, 1015–1023 Search PubMed.
- S. Li, D. Kawashima, K. O. Okeyo, T. Murata and M. Takei, Meas. Sci. Technol., 2023, 34, 035701 CrossRef CAS.
- O. Pänke, W. Weigel, S. Schmidt, A. Steude and A. A. Robitzki, Biosens. Bioelectron., 2011, 26, 2376–2382 CrossRef.
- S. Li, D. Kawashima, Z. Gao and M. Takei, IEEE Open J. Instrum. Meas., 2022, 1, 1–9 Search PubMed.
- D. Kawashima, T. Yuki, S. Li and M. Takei, Biosens. Bioelectron., 2022, 212, 114432 CrossRef CAS.
- Z. Liu, Y. Zhou, L. Du and M. Li, Analyst, 2015, 140, 8101–8108 RSC.
- X. A. Morven Cameron, A. Al Abed, Y. Buskila, S. Dokos, N. H. Lovell, J. W. Morley and A. A. Abed, J. Neurophysiol., 2017, 117, 2014–2024 CrossRef PubMed.
- A. Lechleiter and A. Rieder, Inverse Probl., 2006, 22, 1967–1987 CrossRef.
- T. Asai, N. Adachi, T. Moriya, H. Oki, T. Maru, M. Kawasaki, K. Suzuki, S. Chen, R. Ishii, K. Yonemori, S. Igaki, S. Yasuda, S. Ogasawara, T. Senda and T. Murata, Structure, 2021, 29, 203–212.e4 CrossRef CAS PubMed.
- Z. Zhou, Q. Gong, B. Ye, Z. Fan, J. C. Makielski, G. A. Robertson and C. T. January, Biophys. J., 1998, 74, 230–241 CrossRef CAS PubMed.
- M. Punjiya, H. R. Nejad, J. Mathews, M. Levin and S. Sonkusale, Sci. Rep., 2019, 9, 11988 CrossRef PubMed.
- M. Costella, Q. Avenas, M. Frénéa-Robin, J. Marchalot, P. Bevilacqua, P. G. Charette and M. Canva, Electrophoresis, 2019, 40, 1417–1425 CrossRef CAS PubMed.
- J. Zhang, H. Yuan, X. Yao and S. Chen, Pflügers Arch., 2022, 474, 665–680 CrossRef CAS PubMed.
- H. Prinz, J. Chem. Biol., 2010, 3, 37–44 CrossRef PubMed.
- D. F. Lehmann, W. D. Eggleston and D. Wang, Pharmacotherapy, 2018, 38, 341–348 CrossRef CAS PubMed.
- M. A. Mansor and M. R. Ahmad, Int. J. Mol. Sci., 2015, 16, 12686–12712 CrossRef CAS.
- M. V. Helliwell, Y. Zhang, A. El Harchi, C. E. Dempsey and J. C. Hancox, Pharmaceuticals, 2023, 16, 1204 CrossRef CAS PubMed.
- K. Ishii, M. Nagai, M. Takahashi and M. Endoh, Cardiovasc. Res., 2003, 57, 651–659 CrossRef CAS PubMed.
|
This journal is © The Royal Society of Chemistry 2024 |
Click here to see how this site uses Cookies. View our privacy policy here.