DOI:
10.1039/D4LC00181H
(Paper)
Lab Chip, 2024,
24, 3840-3849
A novel microfluidic tool for the evaluation of local drug delivery systems in simulated in vivo conditions
Received
26th February 2024
, Accepted 16th July 2024
First published on 24th July 2024
Abstract
A 3D-printed microfluidic tool for assessing local drug delivery systems (LDD) in simulated in vivo conditions was developed and evaluated. The device was designed considering the oral environment and dental applications, and it was fabricated with a high-precision resin 3D printer. Chitosan scaffolds loaded with different concentrations of doxycycline were used for evaluating our device. The concentration of the released drug was measured through in-line UV-VIS spectroscopy, and to verify the repeatability and accuracy of our measurements, comparisons with standard HPLC results were made (5% deviation). Cumulative drug release profiles in steady-state conditions were obtained and compared to the Weibull model. The behaviour of the LDD system in a dynamic environment was also evaluated during experiments where step changes in pH were introduced. It was demonstrated that under infection-like conditions, there is an immediate response from the polymer and a clear increase in the concentration of the released drug. Continuous flow and recirculation experiments were also conducted, revealing significant differences in the drug release profiles. Specifically, in the case of continuous flow, the quantity of the released drug is much higher due to the higher driving force for diffusion (concentration gradient). Overall, the proposed microfluidic tool proved to be ideal for evaluating LDD systems, as the in vivo microenvironment can be replicated in a better way than with currently used standard systems.
1. Introduction
Bacterial infections are a common problem in regenerative medicine and particularly in orthopaedics and clinical dentistry. The formation of bacterial biofilm can compromise the healing process of the tissue and in severe cases can lead to implant/scaffold failure and eventually to revision surgery.1–3 For many years the standard approach to prevent or deal with post-operation infections was the systemic intake of antibiotics. However, this strategy is not always effective, mainly because of the antibacterial resistance that is developed by microorganisms.4–7 A promising solution to the problem is the development of local drug delivery (LDD) systems. In this case a biomaterial-based drug delivery8,9 system releases active compounds directly to the site of action preventing the appearance of infection. The great advantages of this approach are that a) the required quantity of drug to deal with infection is much lower comparing to systemic administration; b) the drug acts locally reducing potential side-effects to other tissues and organs; c) local drug release, in response to environmental stimuli enables prevention strategies and minimises the chances of severe complications due to infection. The development of an effective LDD system became a topic of increased interest in the field of tissue engineering in the last decade. However, this is a challenging task since there are many questions that one needs to answer. How much drug should be loaded to the initial material? How fast the drug is released after implantation? What is the concentration of drug at the microenvironment around the LDD after implantation? How does the LDD system respond to changes of the in vivo microenvironment? To answer all these questions in-depth, understanding of both the properties of the biomaterial that acts as drug carrier but also of the expected in vivo conditions is needed. The latter are defined by the type of cells and microorganisms present, pH, temperature, the type of biological fluids in contact with the LDD system (e.g. saliva, blood) but also by the expected flow conditions (velocity, wall shear stress, amount of fluid).
All these variables affect the behaviour of the biomaterial and can determine the drug release rate and local drug concentrations. The same LDD system could give different drug release profiles if it is exposed for example to oral conditions (dynamic pH, high quantities of saliva, changes of temperature) and if is used for treating large bone defects (low flow rate of blood, relatively constant pH and temperature). Advanced engineering tools, with which we can replicate in vitro the expected in vivo microenvironment, could improve the development process of LDD systems and help scientists and clinicians to better predict the expected in vivo behaviour and based on this to optimise the drug release profile according to the healing needs of each clinical case.
Motivated by this, the objective of this work is the design and evaluation of a novel microfluidic device in which we can replicate in vivo conditions (pH, temperature and flow) and can be used for the study of pharmacokinetics during the development of LDD systems. Microfluidics is an ideal option to achieve the in vivo-like environment mainly due to the perfusion of small volumes of liquid, similar to what we expect around implanted medical devices in human body, allowing replication of fluid shear, local drug concentrations and chemical gradients. These are clear advantages comparing to the standard dissolution-HPLC systems that are used at the moment and are mainly designed for studying drug delivery systems for systemic administration.9–11
The proposed system consists of four sections (Fig. 1a and b). First is the inlet section where two inlets have been designed, one for the main liquid and the second for introducing different buffers (e.g., for adjusting pH). Two mixing sections for homogenising the fluids in the inlet and outlet; the first for achieving uniform pH across the fluid profile ensuring uniform dissolution across the LDD's surface, and the second for uniform drug concentration across the fluid at the outlet for more accurate UV-VIS absorbance measurements. A drug release section where the loaded sample is exposed to uniform flow conditions. Finally, a drug concentration measurement section. This uses in-line UV-VIS spectrometers to measure local concentration across the outlet micro-stream in real-time. Spectroscopy for detection on micro-streams has been presented before in literature.12–14
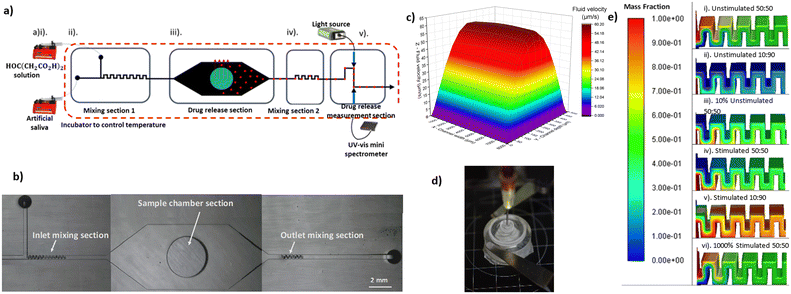 |
| Fig. 1 Experimental setup and microfluidic design; a) different elements of experimental setup: i) syringe pumps, ii) mixing section 1, iii) drug release section, iv) mixing section 2, v) drug release measurement section; b) optical image of 3D printed microfluidic device; c) velocity profile obtained from CFD simulation at the sample chamber section; d) sample filling apparatus; e) CFD results of the inlet mixing section at different flow conditions: i) unstimulated 50 : 50, ii) unstimulated 90 : 10, iii) 10% unstimulated 50 : 50, iv) stimulated 50 : 50, v) stimulated 10 : 90, vi) 1000% stimulated 50 : 50. | |
The proposed setup will allow us not only to test the samples at steady state but to simulate different scenarios where one or more variables are changing with time. Such cases are not uncommon in vivo. For example, the local pH could change in case of infection due to the presence of lactic acid as product of bacteria metabolism (from 7.4 to 5.5). Another case is the condition known as inflammatory hyperthermia where there is increased efflux of blood, due to inflammation, that eventually causes rise in the local temperature of the tissue. Understanding the response of the different biomaterials to these changes of the in vivo environment is important in the study and development of advanced LDD systems. Capturing dynamic data using the standard HPLC methods, requires a bespoke expensive setup and large number of samples and thus the microfluidic tools could be a cheaper alternative.15
After the design and fabrication of the proposed device, different experiments were conducted to evaluate it in terms of measuring capabilities, accuracy of results, and repeatability. Chitosan has been selected as the testing LDD system. Chitosan is a natural polymer that is widely used in biomedical engineering both as a biomaterial for tissue regeneration but also as a matrix for drug loading applications.16–20 Its dissolution rate is greatly affected by the pH, and thus different drug release profiles are expected when simulating different scenarios in our device. Chitosan samples were loaded with doxycycline (DOX), a common antibiotic that is used to control infection in dentistry. DOX has two reported absorption peaks at 230–280 nm and 350–370 nm, making it easy to detect using UV-VIS spectrometry.21,22
2. Materials and methods
Design and fabrication of microfluidic device
A commercial computational dynamics software (Ansys Fluent v19.2) was utilised to simulate the flow for the initial design of the microfluidic device. Velocity for both inlets was set as boundary condition, while appropriate values were used to mimic the oral environment. A mesh dependency study was performed and it was found that between 1.4 M and 1.5 M cells (22 micron cell size) the velocity profile in the sample chamber deviated by less than 0.1%.
After investigating the impact of mixing step geometry, and number of steps, on achieving fluid homogeneity at different volumetric flowrates, it was determined that 24 mixing steps (100 μm × 150 μm) are required for the initial mixing section (Fig. 1e). In the same way it was found that in order to get a homogenous drug-liquid mixture at the exit of the microfluidic device a mixing section of 12 mixing steps is required. For the design of the sample chamber the velocity profile at different flow rates was considered (Fig. 1c). A height to width to height aspect ratio was chosen (8
:
0.5 mm) that results into a broad face profile. This is to assure that the shear stress over the whole sample area is the same (diameter of sample 5 mm).
Device geometries were drawn in Autodesk Fusion 360 and then exported as an STL file to Miicraft software (Miicraft 125, version 4.01, Miicraft Inc) to set the printing parameters. The design presented consists of 2 parts; the main device with sections I, II and III, and an external consumable sample holder that is necessary for fixing the sample at the bottom of the microfluidic device. The latter is open from one side, via a threaded hole where the sample holder is placed. The sample holder is a screw-cup design, allowing 10 μl of sample to be loaded. The holder has two threads across its body and a pocket for a rubber 9 mm outer diameter O-ring (RS PRO FKM O-Ring), for sealing purposes. When the sample holder is in position the testing sample is at the same level with the bottom wall of our device leaving a gap of 500 μm for fluid flow. This is in accordance with literature data that suggest the gap expected between a dental implant and surrounding tissue to be between 200–500 μm.23,24
The 3DP files were printed using a 4k pixel high-resolution digital light processing (DLP) 3D printer (Creative CADworks 3D, Pr-101). The printing material was a clear propriety resin (BV007A, Creative CADworks) absorbing at 390 nm. For cleaning the 3D printed parts, exterior surfaces were washed quickly and thoroughly with IPA, followed by water. Residual fluid was removed with compressed air. Any internal channels were flushed with IPA, water and finally with compressed air. The device was then cured under ultraviolet (UV) light for 2 min.
An in house built μ-PIV system was utilised for verifying the velocity profile in the microfluidic chip. The system consists of an inverted microscope (Nikon Eclipse TE-2000), a CMOs camera (pco.panda) and a dual pulse laser emitting at 520 nm (Optolution). For the measurements, the flow was traced by adding Nile red fluorescent carboxylate microspheres (Invitrogen) with mean diameter of 1 μm. For each flow rate studied, 40 image pairs were captured and the velocity vectors were obtained after processed with PIVlab on MATLAB.25–28
Fabrication of drug loaded chitosan samples
For the preparation of the drug loaded chitosan samples, doxycycline in powder form (Sigma-Aldrich, CAS: 24390-14-5) was dissolved in a 2% v/v aqueous acetic acid solution (Fisher Scientific CAS: 64-19-7). Depending on the desired concentration, appropriate quantity of medium molecular weight chitosan powder (Sigma-Aldrich, CAS: 9012-76-4) was added to the solution and left to dissolve under continuous mixing for 2 h, at 40 °C. The chitosan solutions were left for 24 h to de-aerate. The scaffolds were fabricated by micro dosing the chitosan solution using a high precision microfluidic pump, into the 3D printed cup sample holder. The sample holder is a 500 μm circular pore and 500 μm depth. The indicative size for pores for in vivo use if 300–600 μm.29
The samples were frozen at −25 °C overnight and then placed for 24 h into a freeze dryer (Virtis, BenchTop Pro), that operates at −85 °C and pressure of 200 mTorr. In total five scaffolds were fabricated and tested with various concentrations of doxycycline and chitosan (Table 1).
Table 1 Chitosan samples used for testing the microfluidic device. CS-4* is a crosslinked sample of CS-4
Sample code |
% w/v chitosan |
Doxycycline mg ml−1 |
CS-1 |
3 |
5 |
CS-2 |
3 |
10 |
CS-3 |
3 |
20 |
CS-4 |
3 |
40 |
CS-5 |
1.5 |
10 |
CS-4* |
3 |
40 |
Experimental setup and flow conditions
The fluid phases, during continuous flow experiments, were contained in plastic 60 ml syringed (BD-14955461) and injected into the fluid network using single channel syringe pumps (AL-1000). Flangeless fittings (Darwin microfluidics – CIL-XP-245X) were used for fluid network connections. Tygon tubing, ID: 0.51 mm, and OD: 1.52 mm was used. A peristaltic pump was used for re-circulation experiments (Darwin microfluidics – IM-78018-40), and L/SL 13 pump tubing was used for the pump head (Darwin microfluidics – MF-96419-13). 3D printed bubble traps were used within the fluidic network to avoid interference of air bubbles with spectroscopic measurements.30
The two liquids pass through a micro-mixing section (I) before entering the sample chamber (II). Here the sample is placed in a cavity at the bottom of the channel and is exposed to controlled conditions i.e. pH, temperature, liquid flow and shear stress. The chamber provides a more in vivo like drug release interface, as the LDD system is only exposed to the liquid medium on a singular surface, opposed to the current methods that expose all the LDD surfaces to the fluid. The liquid that exits the sample chamber is passing through a second micro-mixing section (III) to get a homogenous medium-drug mixture. Then is going to a flow cell (IV) where relying on spectroscopic measurements we can have real time monitoring of the drug released, by the sample.
The oral micro-environment during onset of infection is simulated as a case study to demonstrate the metrology capabilities. The flow of saliva in the oral cavity typically has two modes of flow; stimulated and unstimulated. Unstimulated is the dominant but lesser value occurring during homeostasis. Stimulated flow is the non-dominant but higher value, responsive, mode of flow, arising in response to consumption of acidic substances. The expected flow rate for a single tooth can be estimated based on literature values;31,32 stimulated – 13.125 mL h−1, and unstimulated – 0.5625 mL h−1.
Citric acid (Fisher Scientific CAS: 5949-24-1) dissolved in de-ionised water, was used as buffer to adjust pH under normal and infection like conditions. Onset of infection is characterised by a reduction in local pH, driven by bacterial lactic acid production. Literature values estimate the pH drop to be between 6.7 and 5.5, and thus the value of 5.5 is used as a case study.33,34 For controlling temperature, the microfluidic device is submerged in a water bath (Polyscience WBE02A11B) at 37 °C.
Spectroscopic measurements and calibration
A UV-vis spectrometer (Ocean ST 185–650 nm, Ocean Insight) and a flow cell (FIA-Z-SMA-ML, Ocean Insight) were used for real-time spectroscopic measurements and monitoring of DOX concentration. A deuterium–halogen light source (DH-2000-BAL, 210–2500 nm) was used with the mini spectrometers. The mini-spectrometers were controlled, and settings programmed using software OceanView 2.0.12, from Ocean Insight Inc.
For calibrating our system standard aqueous solutions of doxycycline, and chitosan. Based on measured calibration curves for DOX and chitosan, an absorbance/concentration relationship was derived at the two DOX peaks (eqn (1) and (2)). The final concentration of DOX and chitosan was measured, and relationships of was based on an average of the two (eqn (3)). During these experiments, it was validated that the flow rate of the solution in the flow cell has no effect on the absorption measurements.
|
Absorbance276nm = 0.0277CDOX + 3.1 × 10−5CChitosan | (1) |
|
Absorbance347nm = 0.0234CDOX + 1.2 × 10−5CChitosan | (2) |
|
 | (3) |
Scanning Electron Microscopy (SEM) and image analysis
The morphology of the samples was examined at high vacuum SEM. Chitosan scaffolds were observed using a FEI Quanta 650 microscope at the accelerating voltage of 10 kV, while the microchannels were observed using a FEI Quanta 200 microscope under 20 kV. All the samples were fixed on aluminium stubs, coated with a 4 nm Au/Pd (80
:
20) layer using a Quorum coater and a small amount of silver paint was applied.
Image analysis is conducted on the greyscale SEM images. Pores will be the darkest areas of the image. Otsu multi-level threshold is used to detect the darkest portion of the image, with the lowest standard deviation.35 These darkest portions can be further segmented to individual pores, estimate an average pore size and distribution.36–38 Algorithm programming was done using MATLAB (MathWorks) for automated image processing.39,40
High Performance Liquid Chromatography (HPLC)
The doxycycline standard solutions and solutions collected from an experimental assay were quantified using an Agilent HPLC system (Agilent 1260 Infinity 11 RP, DAD, SemiPrep) a gradient pump, a variable wavelength detector (set at 276 nm) and a solvent degasser system. A Hyperclone LC Phenomenex column was used (250 × 4.6 mm, 5 μm, BDS C18 130A). A mobile phase of 60% methanol, 40% water and a flow rate of 1 ml min−1. The solution injection volume was 5 ml and 100 ml. A total run time of 30 min was set. The column was set at an isothermal temperature of 25 °C. The peak at time 4.6 min and 3.3 min was used to quantify the doxycycline. The concentration is calculated from the peak area.
3. Results and discussion
Velocity profile
In Fig. 2 the velocity vectors and streamlines for three different sections of the microfluidic device are presented. At the inlet and outlet of the sample chamber, because of an expansion and contraction respectively, there is risk of recirculation to occur. However, even at the highest flow rate used in our experiments no vortexes or recirculation were detected. At the middle section of the chamber and above the position of the sample, vectors of the same length were identified. This is reasonable considering the high width to height ratio that results into a broad face velocity profile, that was also confirmed with the initial CFD simulations (Fig. 1c).
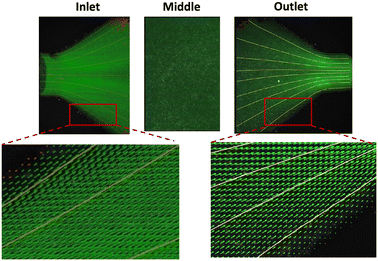 |
| Fig. 2 Velocity vectors and streamlines at the inlet, middle section and outlet of the microfluidic chip for stimulated flow (13.125 mL h−1). | |
Measurement accuracy and repeatability
During experiments, the drug concentration in the liquid medium is captured every minute and based on these data the DOX concentration over time graph can be constructed. A typical example of local concentration release profile over a 24 h period is shown in Fig. 3. Calculating the area below that graph the average drug concentration for the whole duration of the experiment can be obtained. In order to determine the measuring accuracy of our system, the liquid medium was collected at the end of the experiment and the drug concentration was measured with HPLC which is considered the gold standard method for chemical quantification. For the case presented in Fig. 3, the average concentration measured with our system was 8 ppm while with HPLC it was measured 7.73 ppm (deviation of 3.25%). Similar experiments were conducted for different conditions, and it was found that the deviation between our system and HPLC results is less 5% for all the low velocity cases tested. However, when we have stimulated flow conditions, the deviation is increased to 23% (for same sample as in Fig. 3). This is related to the measurement accuracy of the spectrometer. Higher flow rate in the microfluidic device means that the real time drug concentration is lower and closer to the detection limits of the spectrometer (1 ppm). None of the other experimental parameters (e.g. pH) found to have any effect on the measurement accuracy.
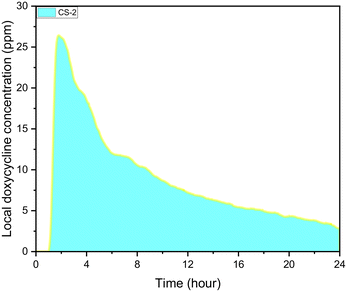 |
| Fig. 3 CS-2 24 h local doxycycline concentration profile, pH 5.5, 37 °C cross sectional area of 7 mm2, depth of 0.5 mm, and 10 μL volume, unstimulated flow. | |
To determine the repeatability of our experiments the cumulative drug release profile for three identical samples was obtained. The values were normalised based on total sample volume. As it is depicted in Fig. 4, all samples show similar exponential release profile with the deviation between the three to be around 10% at the beginning of the experiment when the burst drug release occurs. The difference decreases with time; less than 10% after 10 hours and below 5% after 16 hours. The initial large sample differences are attributed to inconsistences on the surface of the chitosan samples that occur during fabrication. These can affect the swelling process and can result in differences to the initial burst release. Since there is a good control of the total chitosan volume in each sample, the total amount of drug that is released would be the same and this is reflected to the low deviation at the end of the experiment after 24 h.
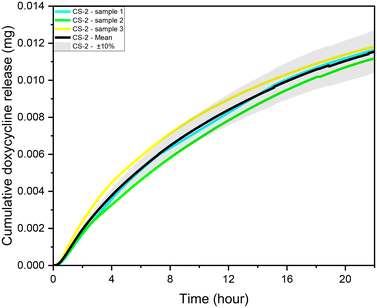 |
| Fig. 4 Repeatability test in the microfluidic device. Three identical samples were tested, and the deviation of final drug concentration was less than 5%, pH 5.5, 37 °C cross sectional area of 7 mm2, depth of 0.5 mm, and 10 μL volume, unstimulated flow. | |
Effect of drug loading concentration
One of the key questions during the development of LDD systems concerns the amount of drug that needs to be loaded onto the scaffold so that when this is found in vivo, the local drug concentration would be appropriate for preventing bacterial colonisation but also not too high to cause toxicity. The impact of initial drug loading quantity on the cumulative release profile was explored, for concentrations of 5, 10, 20, and 40 mg ml−1 (Fig. 5a). Then the steady state concentration (concentration values at 22 h) for each sample was compared to the minimum inhibition concentration (MIC) of common oral bacteria (Fig. 5b). For all the cases tested, a high release rate that corresponds to the burst release is observed during the first 8 h but after that the rate of release remains constant. Although all samples follow a similar general trend, the actual release rates and the final local drug concentration depends on the initial amount of DOX. The local doxycycline concentration after 22 h for CS-2, CS-3 and CS-4 loaded scaffold is above the MIC of E. coli, Staphylococcus aureus, P. multocida, and Streptococcus pneumoniae.41–44
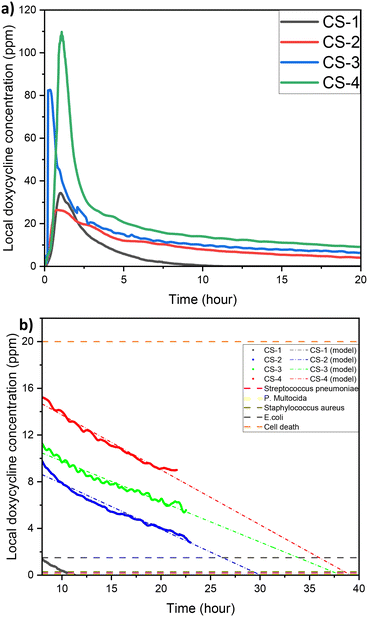 |
| Fig. 5 a) Release profiles for CS-4, CS-3, CS-2, CS-1 over a 24 hour period, b) linear best fit line for release profiles to completion, pH 5.5, 37 °C cross sectional area of 7 mm2, depth of 0.5 mm, and 10 μL volume, unstimulated flow. | |
Long-term exposure to concentrations above 20 ppm can cause damage to tissue.45 Based on this graph the appropriate initial amount of drug on the scaffold can be identified taking also into account the expected bacterial systems and the desired period of drug release.
Another interesting finding is that the final local concentration is not linearly proportional to the initial amount of drug. As it is presented in Fig. 5b although there is a high increase of the steady state local concentration between 5 and 20 mg ml−1 this will not increase significantly above 30 mg ml−1. Similar results have been reported in literature and according to Li et al., higher drug loading leads to a longer release profile, due to a reduction of porosity.46 Porosity is a finite volume and once saturated the release profile will no longer be impacted. This can be verified after examination of our samples with SEM (Fig. 6). As expected for chitosan scaffolds,47–49 the porosity was found to reduce with increasing initial drug loading, (from 60% for the no drug sample to 42% for 10 mg mL−1 of DOX and eventually to 28% for 40 mg mL−1). It is clear the reduction is non-linear further verifying the theory of pore saturation.
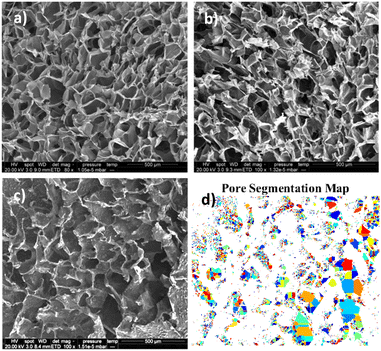 |
| Fig. 6 SEM captured images of 3 (wt)% chitosan scaffold; a). 0 mg ml−1 doxycycline loaded, b). 10 mg ml−1 doxycycline loaded, c). 40 mg ml−1 doxycycline loaded; d) indicative segmentation map for determining porosity for 40 mg ml−1 DOX sample. | |
In Fig. 7, a comparison of the release profile of scaffolds with different polymer concentration but same initial drug amount is presented (CS-2 and CS-5). For the sample with the lower polymer concentration (CS-5), the release rate is higher, and the maximum concentration is achieved after 9 hours while for CS-2 the maximum concentration is reached after 38 h. This is justified if we consider that the lower concentration of polymers, the faster the dissolution rate and thus the drug release.
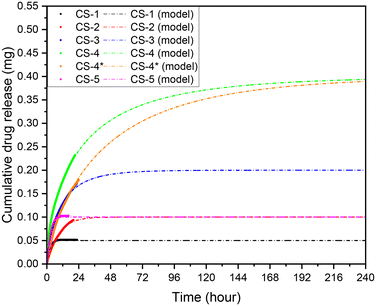 |
| Fig. 7 Weibull models for; CS-1, CS-2, CS-3, CS-4, CS-5, CS-4* pH 5.5, 37 °C cross sectional area of 7 mm2, depth of 0.5 mm, and 10 μL volume, unstimulated flow. | |
The release profiles very strongly fit asymptotic models, as expected of chitosan-based scaffolds.50–53 Various asymptotic models are deployed across literature, commonly the Korsmeyer–Peppas, Weibull, and Peppas–Sahlin.3,52,54 The samples fitting coefficients for these models were evaluated in Table 2.
Table 2 Samples asymptotic models fitting coefficients; Korsmeyer–Peppas, Peppas Sahlin, and Weibull
|
Korsmeyer–Peppas |
Peppas–Sahlin |
Weibull |
CS-1 |
0.77095 |
0.86681 |
0.98917 |
CS-2 |
0.99404 |
0.99941 |
0.95972 |
CS-3 |
0.99845 |
0.99969 |
0.9943 |
CS-4 |
0.993 |
0.99603 |
0.99586 |
CS-5 |
0.87348 |
0.97664 |
0.94314 |
CS-4* |
0.99408 |
0.99906 |
0.99791 |
The Weibull cumulative distribution model is cited as a good data fit across sources, reflected in high fitting coefficient for all samples (Table 2).
The pharmacokinetics of each scaffold can be quantitatively assessed by comparing the model parameters, allowing for the best scaffold based on desired characteristics to be selected. Ideal for early screening of biomaterials on the clinical pathway. The parameters impact relating to the pharmacokinetics is described in
Table 3.
Table 3 List of Weibull model parameters and description in respect to the chitosan scaffolds
Parameter |
Description |
(A0 + Y0) |
Amplitude and offset – determines the final value of the function, large values reflect higher final cumulative drug release value. The total drug load released into the in vivo environment |
a
|
Scale – determines the gradient of the function, larger values stretch out the function. The drug release would be slower at higher parameter values, indicating a scaffold more suitable for long-term release applications |
b
|
Shape – determines the peakedness of the function, higher values indicate sharper functions. Drug release during swelling would be higher for larger parameter values, indicating a scaffold more suitable for short-term release applications |
The amplitude and offset parameters were bounded based on initial drug loading values. The scale parameter value and shape function increase with drug loading, indicating a decrease in dissolution rate and swelling, due to a reduction in porosity. The scale parameter significantly decreases with a reduction in mass weight, reflecting an increase in dissolution rate. When cross linking CS-4, the scale parameter significantly increases indicating a decrease in dissolution rate. The impact of drug loading on Weibull function parameters is show in Fig. 8. The scale parameter linear relationship, and shape parameter exponential relationship, with drug loading quantifies the morphological impact highlighted in the SEM. Increased drug loading is both reducing the dissolution rate and swelling rate in the LDD systems, driven by tabletting affect and decrease in porosity.
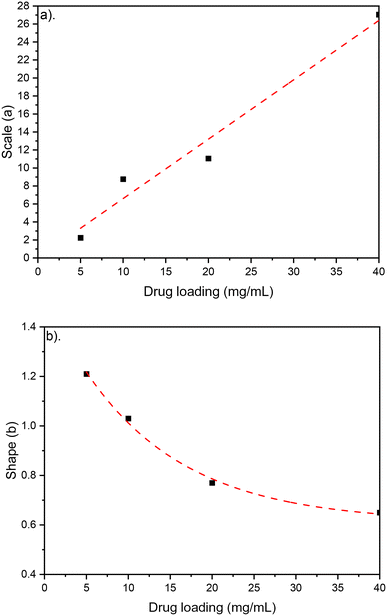 |
| Fig. 8 a: Weibull scale parameter plotted against drug loading with fitted linear relationship (a = 0.66 mg ml−1, R2 = 0.98), b: Weibull shape parameter plotted against drug loading with fitted asymptotic linear relationship (b = 0.61 + 0.92 × 0.92 mg mL−1, R2 = 0.99. | |
Testing in dynamic conditions
A unique capability of this experimental setup is the potential to dynamically change the pH to which the biomaterial is exposed to, better reflecting the in vivo micro-environment. The release response to example pH pulses, is shown in Fig. 9. The scaffold experiences a homeostatic pH of 7.5 with 4 hour pH 5.5 pulses at 10, 18, and 26 hour marks. Scaffold responsiveness to pH changes is assessed, considering the time interval to a measurable increase in local doxycycline concentration (minus 30 min device residence time), peak response magnitude, and average response magnitude during pulses.
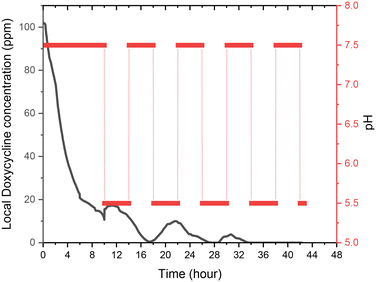 |
| Fig. 9 Release profile for 3 (wt)% and 40 mg mL−1 doxycycline loaded scaffold exposed to pH pulses, cross sectional area of 7 mm2, pH 5.5 and 7.5, 37 °C cross sectional area of 7 mm2, depth of 0.5 mm, and 10 μL volume, unstimulated flow. | |
Following the first pulse, a rapid change in doxycycline concentration occurs. The response from the polymer to the change of pH is immediate (0 min), and the drug concentration is increased from an average of 15.4 ppm to a peak magnitude of 17.3 ppm. Second pulse responsiveness is also 0 min, but this time the peak concentration is at 10.0 ppm, from an average of 6.3 ppm before the change of pH. The response during the third pulse is dramatically slower (150 min) and the peak magnitude is 2.9 ppm, from an average of 1.0 ppm. Prolonged response time by the third pulse suggests slower solution penetration, possibly due to structural changes accelerated by prior pulses. Peak and average magnitudes decrease with each pulse, reflecting diminishing doxycycline content. Local concentration tends toward zero at pH 7.5 post-pulse, signifying minimal release.
Zero release occurs when no drug remains, the scaffold is fully worn, or if the dissolution at non-acidic pH is negligible. Low dissolution rates at pH 7.5 favour scaffold longevity, maintaining the drug loading for longer amount of time to combat infections.
Continuous flow versus re-circulation measurement
The case of recirculation is closer to the standard experiments that take place in dissolution setups where the liquid around the drug loaded material remains the same. As it is presented in Fig. 10, the rate of drug release from identical samples is much higher during the continuous flow experiments. Under stimulated conditions the initial drug release difference is at its lowest at 5 min, exponentially increasing afterwards. The differential exponential increase is in-line with scaffold drug release experienced during swelling, reducing the re-circulation release rate due to reduction in diffusion. The difference begins to plateau out, expected as the doxycycline release decreases with time, so the impact on diffusion diminishes. After the first 2 h it was found that the cumulative concentration is almost 100% higher comparing to the recirculation case. This is easy to explain if we consider the convection–diffusion eqn (4);Comparing the two experiments we can ignore the convective term (vc) as the fluid velocity across the LDD surface was the same in both cases. The concentration gradient between the scaffold and the fluid medium above it (−∇c) is the driving force for drug transfer to occur. In the case of continuous flow, fresh liquid with zero drug concentration is always in touch with the sample. In the recirculation case the concentration of the drug to the liquid medium is building up with time, resulting in lower gradient and consequently lower diffusion flux (J).
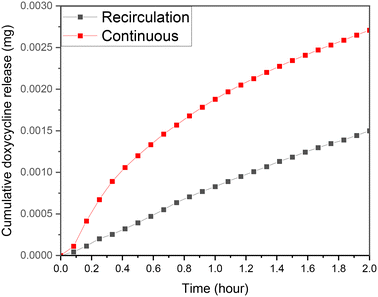 |
| Fig. 10 Release profile for CS-2 under stimulated flow continuous flow and re-circulatory flow, pH 5.5, 37 °C cross sectional area of 7 mm2, depth of 0.5 mm, and 10 μL volume. | |
4. Conclusion
In this proof-of-concept study, we have successfully designed, and fabricated a novel microfluidic device, in which we are able to replicate specific in vivo micro-environments, through precise control of fluids, velocity, wall shear stress, temperature, pH and chemical gradients. Coupling it with in-line UV-vis spectroscopy resulted in the capability to measure drug concentrations in real time and obtain detailed drug release profiles. The accuracy of our measurements was verified after a comparison to HPLC results where a 5% deviation was found for low flow rates. Drug release profiles from the chitosan scaffolds were evaluated, at a pH representative of bacterial infection, and by fitting the data to the Weibull asymptotic model, the specific impact of material changes on the pharmacokinetic profile parameters could be compared. A dynamic pH assay was developed replicating the dynamic change in pH occurring from repeated onset of bacterial infection. The example LDD system was evaluated using the dynamic assay, quantifying response time, magnitude and response change to repeated onset of infection.
One of our key findings is the difference in the drug concentrations between continuous and recirculation flow conditions. The first case represents better the in vivo microenvironment where there is a continuous flow of biological fluid around the drug loaded medical device. Use of the standard setups that have been designed for the investigation of drugs for systemic administration will result in great underestimation of drug release, since the driving force for diffusive mass transfer is lower. Moreover, in the proposed microfluidic device the volume of sample required is extremely low (∼5 μl), and there is better representation of the surface-fluid medium interface found in vivo. The use of additive manufacturing facilitated a cheap and quick iterative design process. Different geometries can be designed for better replicating in vivo conditions depending on the scenario that is to be investigated. Use of bio-resins during fabrication can allow experiments in the presence of cells in the chip to take place.
In this work the focus was on the evaluation of the microfluidic system in acellular environment, however the next steps include experiments with both bacteria and cells. In this case different spectrometers will be tested not only to capture drug release but to identify substances resulted from the metabolic activity of the micro-organisms. Overall, the microfluidic device and measuring methodology presented here can result in faster and more efficient evaluation of LDD systems. The potential to obtain accurate drug release profiles in vitro can translate to better design of biomaterials and shorter development time. More advanced systems that can accurately replicate in vivo microenvironment can eventually contribute in long term to simplify and reduce the need for extensive animal trials.
Data availability
Data for this article, are available at Zenodo at https://doi.org/10.5281/zenodo.12670433.
Conflicts of interest
There are no conflicts to declare.
Acknowledgements
The authors would like to acknowledge EU funded I-SMarD 953128 for the financial support.
Notes and references
- H. Ephros, S. Kim and R. DeFalco, Dent. Clin. North Am., 2020, 64, 305–313 CrossRef PubMed.
- V. John, D. Shin, A. Marlow and Y. Hamada, Case Rep. Dent., 2016, 2016, 1–8 Search PubMed.
- G. M. S. Soares, L. C. Figueiredo, M. Faveri, S. C. Cortelli, P. M. Duarte and M. Feres, J. Appl. Oral Sci., 2012, 20, 295–309 CrossRef CAS.
-
P. Stoica, M. C. Chifiriuc, M. Rapa and V. Lazăr, in Biofilms and Implantable Medical Devices, Elsevier, 2017, pp. 3–23 Search PubMed.
- R. Donlan, Emerging Infect. Dis., 2001, 7, 277–281 CrossRef CAS.
- S. S. Talsma, Home Healthc. Nurse, 2007, 25, 589–594 CrossRef.
- D. Sharma, L. Misba and A. U. Khan, Antimicrob. Resist. Infect. Control, 2019, 8, 76 CrossRef PubMed.
- J. V. Costa, J. Portugal, C. B. Neves and A. F. Bettencourt, Drug Delivery Transl. Res., 2022, 12, 1395–1407 CrossRef CAS.
- A. M. Zalfen, D. Nizet, C. Jérôme, R. Jérôme, F. Frankenne, J. M. Foidart, V. Maquet, F. Lecomte, P. Hubert and B. Evrard, Acta Biomater., 2008, 4, 1788–1796 CrossRef CAS PubMed.
- G. Fontana, M. Licciardi, S. Mansueto, D. Schillaci and G. Giammona, Biomaterials, 2001, 22, 2857–2865 CrossRef CAS PubMed.
- M. Witzler, A. Alzagameem, M. Bergs, B. E. Khaldi-Hansen, S. E. Klein, D. Hielscher, B. Kamm, J. Kreyenschmidt, E. Tobiasch and M. Schulze, Molecules, 2018, 23, 1885 CrossRef PubMed.
- J. Yue, F. H. Falke, J. C. Schouten and T. A. Nijhuis, Lab Chip, 2013, 13, 4855 RSC.
- W. Ferstl, T. Klahn, W. Schweikert, G. Billeb, M. Schwarzer and S. Loebbecke, Chem. Eng. Technol., 2007, 30, 370–378 CrossRef CAS.
- B. Kuswandi, Nuriman, J. Huskens and W. Verboom, Anal. Chim. Acta, 2007, 601, 141–155 CrossRef CAS PubMed.
- B. Zhang, J. Nasereddin, T. McDonagh, D. von Zeppelin, A. Gleadall, F. Alqahtani, R. Bibb, P. Belton and S. Qi, Int. J. Pharm., 2021, 604, 120626 CrossRef CAS PubMed.
- L. Elviri, M. Asadzadeh, R. Cucinelli, A. Bianchera and R. Bettini, Carbohydr. Polym., 2015, 132, 50–58 CrossRef CAS.
- M. Jumaa, F. H. Furkert and B. W. Müller, Eur. J. Pharm. Biopharm., 2002, 53, 115–123 CrossRef CAS.
- T. M. Ways, W. Lau and V. Khutoryanskiy, Polymer, 2018, 10, 267 Search PubMed.
- M. Rinaudo, Prog. Polym. Sci., 2006, 31, 603–632 CrossRef CAS.
- M. Thanou, J. C. Verhoef and H. E. Junginger, Adv. Drug Delivery Rev., 2001, 52, 117–126 CrossRef CAS.
- A. C. Kogawa and H. R. N. Salgado, J. Chromatogr. Sci., 2013, 51, 919–925 CAS.
- P. J. Ramesh, K. Basavaiah, M. R. Divya, N. Rajendraprasad, K. B. Vinay and H. D. Revanasiddappa, J. Anal. Chem., 2011, 66, 482–489 CrossRef CAS.
- K. Siormpas, M. Mitsias, E. Kontsiotou-Siormpa, D. Garber and G. Kotsakis, Int. J. Oral Maxillofac. Implants, 2014, 29, 1397–1405 CrossRef PubMed.
- Root Membrane Group, M. Mitsias, M. Bratos, K. Siormpas, M. Pikos and G. Kotsakis, Int. J. Oral Maxillofac. Implants, 2020, 35, 379–385 CrossRef PubMed.
- W. Thielicke and R. Sonntag, J. Open Res. Softw., 2021, 9, 12 CrossRef.
- W. Thielicke and E. J. Stamhuis, J. Open Res. Softw., 2014, 2, 30 Search PubMed.
- T. Neuner, M. Meister, M. Pillei, M. Koch and W. Rauch, Chem. Eng. Sci., 2022, 264, 118129 CrossRef CAS.
- L. Sarno, A. Carravetta, Y.-C. Tai, R. Martino, M. N. Papa and C.-Y. Kuo, Adv. Powder Technol., 2018, 29, 3107–3123 CrossRef.
- A. Bandyopadhyay, S. Ghosh, A. R. Boccaccini and S. Bose, J. Mater. Res., 2021, 36, 3713–3724 CrossRef CAS.
- Y. Gao, M. Wu, Y. Lin and J. Xu, Lab Chip, 2020, 20, 4512–4527 RSC.
- S. P. Humphrey and R. T. Williamson, J. Prosthet. Dent., 2001, 85, 162–169 CrossRef CAS PubMed.
- J. J. Pytko-Polonczyk, A. Jakubik, A. Przeklasa-Bierowiec and B. Muszynska, J. Physiol. Pharmacol., 2017, 68, 807–813 CAS.
- G. Eidt, C. G. de Andrade, T. d. C. Negrini and R. A. Arthur, J. Appl. Oral Sci., 2019, 27, e20180593 CrossRef CAS PubMed.
- S. Mura, J. Nicolas and P. Couvreur, Nat. Mater., 2013, 12, 991–1003 CrossRef CAS PubMed.
- N. Otsu, IEEE Trans. Syst., Man, Cybern., 1979, 9, 62–66 Search PubMed.
- A. Rabbani, S. Ayatollahi, R. Kharrat and N. Dashti, Adv. Water Resour., 2016, 94, 264–277 CrossRef.
- A. Rabbani and S. Ayatollahi, Spec. Top. Rev. Porous Media, 2015, 6, 71–89 CrossRef.
- A. Rabbani, S. Jamshidi and S. Salehi, J. Pet. Sci. Eng., 2014, 123, 164–171 CrossRef CAS.
-
C. P. Ezeakacha, A. Rabbani, S. Salehi and A. Ghalambor, Integrated Image Processing and Computational Techniques to Characterize Formation Damage, Paper presented at the SPE International Conference and Exhibition on Formation Damage Control, Lafayette, Louisiana, USA, February 2018, DOI:10.2118/189509-MS.
- A. Rabbani and S. Salehi, J. Nat. Gas Sci. Eng., 2017, 42, 157–168 CrossRef.
- S. Bhambri and G. Kim, J. Clin. Aesthet. Dermatol., 2009, 2, 45–50 Search PubMed.
- S. D. Dallas, L. McGee, B. Limbago, J. B. Patel, M. L. McElmeel, L. C. Fulcher, D. R. Lonsway and J. H. Jorgensen, J. Clin. Microbiol., 2013, 51, 1798–1802 CrossRef CAS PubMed.
- C.-C. Lai, C.-C. Chen, H.-L. Huang, Y.-C. Chuang and H.-J. Tang, Sci. Rep., 2016, 6, 31964 CrossRef CAS PubMed.
- A. Mead, P. Lees, J. Mitchell, A. Rycroft, J. F. Standing, P.-L. Toutain and L. Pelligand, J. Vet. Pharmacol. Ther., 2019, 42, 52–59 CrossRef CAS.
- M. Sourdeval, Front. Biosci., 2006, 11, 3036 CrossRef CAS PubMed.
- L. Li, X. Zhu, H. Yang, B. Liang, L. Yuan, Y. Hu, F. Chen and X. Han, Mol. Pharmaceutics, 2022, 19, 2854–2867 CrossRef CAS PubMed.
- N. Iqbal, T. M. Braxton, A. Anastasiou, E. M. Raif, C. K. Y. Chung, S. Kumar, P. V. Giannoudis and A. Jha, Materials, 2022, 15, 6245 CrossRef CAS PubMed.
- M. Liu, C. Wu, Y. Jiao, S. Xiong and C. Zhou, J. Mater. Chem. B, 2013, 1, 2078 RSC.
- J. Xu, W. Guan, Y. Kong, F. Liu, Y. Zhao, G. Li and Y. Yang, Coatings, 2022, 12, 1742 CrossRef CAS.
- R. Bharathi, S. S. Ganesh, G. Harini, K. Vatsala, R. Anushikaa, S. Aravind, S. Abinaya and N. Selvamurugan, Int. J. Biol. Macromol., 2022, 222, 132–153 CrossRef CAS PubMed.
- L. Dornjak, M. Kovačić, K. Ostojić, A. Angaits, J. Szpunar, I. Urlić and A. Rogina, Polymer, 2022, 14, 4753 CAS.
- Y. Herdiana, N. Wathoni, S. Shamsuddin and M. Muchtaridi, Heliyon, 2022, 8, e08674 CrossRef CAS PubMed.
- G. Deepa, K. C. Sivakumar and T. P. Sajeevan, 3 Biotech, 2018, 8, 493 CrossRef CAS PubMed.
- L. Serra, J. Doménech and N. A. Peppas, Biomaterials, 2006, 27, 5440–5451 CrossRef CAS PubMed.
|
This journal is © The Royal Society of Chemistry 2024 |
Click here to see how this site uses Cookies. View our privacy policy here.