Source to tap investigation of natural organic matter in non-disinfected drinking water distribution systems†
Received
21st April 2023
, Accepted 29th September 2023
First published on 2nd October 2023
Abstract
Despite being reduced by treatment, natural organic matter (NOM) is ubiquitous in drinking water distribution systems (DWDSs) from sources to consumers' taps where it can potentially have negative impacts on drinking water quality. While a few studies have investigated its behaviour in disinfected and NOM-rich DWDSs, its dynamics in non-disinfected systems, characterized by low NOM content, have not yet been explored. In this study, we monitored the NOM variations occurring between groundwater sources and consumers' taps of a non-disinfected DWDS, including three drinking water treatment plants, using both fluorescence and absorbance, selected due to their increasing adoption by water utilities. PARAFAC analysis of fluorescence data, combined with absorbance indices, highlighted how NOM characteristics in groundwater vary due to the combination of multiple factors (e.g., well depth, pumping rate), especially in the case of shallower aquifers. The treatment processes display different effects on NOM when monitored by fluorescence and absorbance, due to the differences among fluorophores and between fluorescent and chromophoric molecules. Variations of the NOM characteristics between the treatment plant outlets and sampling locations within the network were detected only in few locations, suggesting the importance of the processes occurring in specific sections of the network and the last meter before consumption. These findings highlight the overall stability of water quality within non-disinfected NOM-poor DWDSs, but they stress the importance of (i) properly selecting the analytical method to be used for monitoring and (ii) localized water quality variations mainly related to pipe materials, suggesting several implications for DWDS monitoring and management.
Water impact
Understanding natural organic matter (NOM) behaviour in drinking water is crucial given its impact on treatment performance, water quality, and consumer safety. This study investigated NOM within a non-disinfected groundwater-fed drinking water system, sampling at water treatment works and consumers taps. Fluorescence and absorbance measurements provided new knowledge of NOM characteristics within these systems, guiding future monitoring and management strategies.
|
1 Introduction
Natural organic matter (NOM) is a complex mixture of compounds which is present in all water matrices ranging from environmental to drinking water.1 Monitoring and controlling NOM concentration and composition in drinking water distribution systems (DWDSs), including treatment and distribution, is fundamental as NOM can potentially be a nuisance to consumers (e.g., contributing to the water organoleptic properties)2 or can even be detrimental to the quality of the delivered water. In fact, NOM can interact with residual disinfectant, favouring the production of toxic disinfection by-products.3 NOM can also affect the reaction rates of contaminants within the network4 and interact with the metallic materials of the pipes, eventually aiding their release.5 In addition, NOM can cause biological instability supporting microbial growth in drinking water distribution networks (DWDNs),6 favouring the development of biofilms, possibly resulting in pipe biocorrosion and offering a suitable habitat for pathogens.7
Due to NOM's heterogeneity and complexity, several techniques are required to fully characterize its properties. Commonly, in the field of drinking water monitoring, NOM is measured through the estimation of total or dissolved organic carbon (TOC, DOC). However, such measurement provides only a quantitative estimate of the NOM concentration, without providing any qualitative information regarding its composition.8,9 Several advanced analytical methods, such as liquid chromatography coupled with organic carbon and nitrogen detection (LC-OCD-OND), nuclear magnetic resonance spectroscopy (NMR) and Fourier-transform ion cyclotron resonance (FT-ICR-MS), overcome this limitation assessing different NOM characteristics.8,10,11 However, recently, fluorescence and absorbance spectroscopy techniques have started to be adopted by several water utilities, due to both their simplicity with respect to the above-mentioned methods and the possibility of achieving real-time monitoring thanks to online instruments.9,12 In fact, these techniques, coupled with advanced post-processing methods, such as PARAllel FACtor analysis (PARAFAC) and differential absorption spectroscopy,13,14 allow the fluorescent and chromophoric NOM fractions to be probed without the need for complex analytical workflows.15–18
Several studies exist regarding the variation of concentration and composition of NOM within drinking water treatment plants (DWTPs) (e.g., ref. 19–21). However, only a few studies have focused on NOM variations in DWDNs, despite the importance of distribution networks in guaranteeing both microbiological (e.g., presence of opportunistic pathogens) and chemical (e.g., organoleptic properties) water quality at the point of use.22 Such studies report contrasting results on the spatial and temporal NOM variations within DWDNs. In some studies (e.g., ref. 23), a significant correlation between chlorine residuals and fluorescence intensity within a DWDN has been highlighted. Also, Kurajica and collaborators24 observed an increase of the intensity of fluorescent components likely caused by microbial activity in two groundwater-fed DWDNs with TOC concentrations between 1 and 2.5 mg L−1. Instead, Wang, Wang and collaborators25 highlighted how the NOM concentration and composition did not vary with the residence time within the DWDN, in the presence of relatively high NOM content (i.e., 2.5–8.9 mgDOC L−1). This finding could be likely due to the presence of a pre-oxidation treatment in the monitored DWTP, which could have resulted in a more complete oxidation of NOM before its entrance in the distribution network, leading to its reduced reactivity, e.g., compared to other studies (e.g., ref. 26). Even Heibati and collaborators27 observed the absence of notable NOM variations in the DWDN, likely caused by the low disinfectant residuals used in the monitored network (i.e., 0.03 mg L−1). Such heterogeneity suggests that NOM variability is highly affected by the specific DWDS characteristics, including, but not limited to, NOM concentrations and composition, source water, type of treatment and characteristics of the network.
Even though the published studies investigate DWDSs fed on different source waters (i.e., surface and groundwater), all the investigated systems are disinfected, reflecting the widespread application of this practice. However, specific water utilities or even countries (e.g., The Netherlands, Switzerland) distribute disinfectant-free water, achieving limited microbial regrowth by a reduced presence of a substrate.28 Extending the results of disinfected DWDSs to these systems might not be accurate, as residual disinfectant is known to interact with NOM.3 In addition to the lack of disinfectant, all available studies monitored DWDSs with relatively high DOC concentrations (i.e., >1 mg L−1). However, half of worldwide groundwaters present DOC concentrations below 1.2 mg L−1 (ref. 29) and, especially, non-disinfected systems are typically characterized by low NOM content,28 making available studies not fully representative of groundwater-fed and/or non-disinfected systems. Hence, previous results can only provide limited support to the water utilities managing such systems.
In this context, the aim of this study is to (i) evaluate the applicability of TOC, fluorescence and absorbance for NOM monitoring within non-disinfected DWDSs fed by NOM-poor groundwater and (ii) deepen our understanding of NOM temporal dynamics occurring within such DWDSs and the changes of NOM characteristics from water sources to consumers' taps. For this reason, a monitoring campaign was organized over one year collecting samples in 16 locations within a DWDS in northern Italy, for a total of 212 samples. Data were interpreted and compared through a PARAFAC model, as for fluorescence, and absorbance indices, as for absorbance. Generalizing our results, we provide guidance for future monitoring campaigns of non-disinfected systems fed by NOM-poor groundwater.
2 Materials and methods
2.1 Sampling campaign
The non-disinfected groundwater-fed DWDS monitored in this study comprises three DWTPs and serves a 5800-inhabitant city in northern Italy. It is fed by three wells spread across the city which, depending on the wells' depth, adopt different treatment strategies. In DWTP A (well filter depth = 84–133 m), ferrous sulphate is dosed prior to rapid sand filtration to remove hexavalent chromium; in DWTP B (well filter depth = 48–70 m), the dosage of the reducing agent is followed by a granular activated carbon (GAC) filter. DWTP C (well filters depth = 126–186 m) directly pumps the withdrawn water in the distribution network. A total of 212 samples from 16 locations were collected in three periods between November 2020 and February 2021, July and October 2021 and January and February 2022 in order to discern between seasonal cyclic fluctuations and other temporal variations. Water was sampled from all DWTPs, collecting both raw and treated water, and from 11 public water fountains or commercial buildings, spread throughout the DWDN. In addition, a specific sampling campaign was dedicated exclusively to each DWTP, sampling both raw and treated waters multiple times to assess the daily variability of water quality characteristics. Details of all sampling points are presented in Table S1 and Fig. S1.† At each sampling location, water was allowed to flow for at least 5 minutes before collection using 0.5 L glass bottles with Teflon-coated caps and 250 mL polyethylene (PE) bottles, according to indications provided by standard methods30 and other literature studies31 about materials to be used for sample collection for the analytes of interest. The bottles were previously thoroughly washed using deionized water and rinsed three times with the water at the collection point in order to minimize the possibility of sample contamination. After collection, the samples were stored in the dark in a refrigerated container during transport to the laboratory. The samples collected in glass bottles were then kept at 4 °C and analysed within 5 days, while the samples contained in PE bottles were frozen and analysed successively, within 1 month. Whenever possible, the water temperature was measured directly upon sample collection. In addition, the monthly abstraction flowrate data for each DWTP during the monitoring period were provided by the water utility.
2.2 Water quality characterization and analytical methods
The samples in glass bottles were analysed without preliminary filtration, given the negligible turbidity highlighted in previous sampling campaigns carried out by the water utility (data not shown). To support this protocol in the case of fluorescence measurements, preliminary tests were performed to assess the effect of sample filtration, using samples taken from the DWDS (see ESI† S1). Negligible differences were observed in fluorescence in most of the emission–excitation matrices (EEMs), with the exception of fluorescence at low emission and excitation wavelengths (Ex < 290 nm, Em < 375), for which a slight increase was observed after filtration, likely due to the extra handling step. The samples were first warmed to room temperature using a water bath. Such water samples were then used to measure the pH and conductivity using a multi-meter (HQ40D, Hach, USA) after daily pH calibration, and UV-vis absorbance (range: 190–800 nm) using both 1 cm and 4 cm cuvettes on a UV-vis DR6000 spectrophotometer (Hach Lange, Germany). Fluorescence EEMs were collected every 5 nm between 230 and 450 nm excitation and 270 and 600 nm emission using a Varian Cary Eclipse spectrofluorometer (Agilent, USA) in two analytical replicates, using a PMT voltage of 720 V, a slit width of 10 nm, an averaging time of 0.125 s and default correction factors. Both the spectrophotometer blanks and daily Raman scattering profiles32 were obtained using MilliQ water.
Analyses of TOC and metals (Cr, Fe, Mg, Zn) were performed in the water utility laboratory on samples collected in PE bottles, using a QbD1200 TOC analyser (Hach, USA) and an ICP-MS Agilent 7700 (Agilent, USA), in accordance with the Italian and ISO standard procedure.33,34 Such metals were selected being the most variable across the DWDN based on the water utility data and presenting evidence of interactions with NOM.35,36 Duplicated aliquots of 10 samples were frozen and measured at different dates to test the accuracy of the TOC analyses.
2.3 Source water estimation of DWDN samples
The origin of the water at the various sampling points within the DWDN was estimated using conductivity, considered as a passive tracer.37,38 It was measured in samples collected at both the DWTP outlet and the locations within the DWDN. A confidence interval of conductivity variability was estimated thanks to the repeated conductivity measurements conducted at the DWTP outlet and it was applied around the conductivity measured at the DWTP outlet in each sampling day. In the case where the conductivity of a DWDN sample was within the confidence bounds of a DWTP, the sample was entirely attributed to it. In the case where the conductivity lay between the confidence bounds of different DWTPs (i.e., not belonging to the confidence bounds of any DWTP), the sample was considered as mixed between the two sources, and the relative contribution of each one was estimated.
Concurrently, the relative contribution of each source was predicted using a calibrated dynamic hydraulic model of the DWDN provided by the water utility, considering only the hours of the day during which sampling was performed (i.e., approximately 7:00–14:00).
2.4 Natural organic matter characterization
The EEMs were analysed using the drEEM v6.4 toolbox39 in Matlab 2020 (MathWorks, USA). The inner filter effect was corrected using the absorbance spectra collected using the 1 cm cuvette,40 after which the EEMs were normalized using the Raman scattering intensity collected in the same day.32 The first and second order Rayleigh and Raman scattering were excised from the affected EEM regions.39 Finally, the samples’ fluorescence was normalized using the 3/2nd root of the standard deviation of each EEM41 due to the high variability of fluorescence intensities. After sample pre-processing, PARAFAC modelling was carried out imposing a non-negative constraint on all modes. The fitting of preliminary PARAFAC models allowed us to identify, manually inspect and eventually remove 12 outliers out of 212 samples and excise EEM scans with high leverage and fitting errors.39 The final PARAFAC model was validated using a split-half analysis, maintaining replicates of the same samples in the same split to preserve their independence.39 Validated components were then compared to previously published fluorescence spectra present within the OpenFluor database.42 Finally, the intensity of the fluorescent components obtained by PARAFAC in the duplicated measurements of each sample was averaged sample-wise in order to limit the effect of measurement noise.
The absorbance spectra collected with the 4 cm cuvette were first corrected by subtracting the average absorbance between 750 nm and 800 nm and then used to estimate the absorption coefficient at 254 nm (UV254), as indicated by Helms and collaborators.43 To characterize the variability in the average molecular weight of chromophoric NOM, the same data were used to estimate the absorbance index S275–295 fitting a linear regression in the log-transformed spectra between 275 nm and 295 nm.43
2.5 Data elaborations and statistical analyses
Removal percentages in the DWTPs were estimated for each sampling date as 1 – Vin/Vout, with V representing the value of interest (i.e., PARAFAC component intensity, absorption coefficient value). The effect of the distribution network on organic matter was isolated by subtracting the mean value of the variable of interest at the DWTP outlet to the values measured in the samples attributed to such a DWTP. Samples considered as composed of mixed sources were not included in this analysis.
Statistical analyses were performed using R v4.2.2.44 The homogeneity of variance was tested using the Levene test, as implemented in the package car v3.0-13.45 Depending on the normality of the data, homoscedasticity and the numerosity of the groups, either parametric (i.e., t-test, ANOVA, Tukey HSD) or non-parametric (i.e., Wilcox test, Kruskal–Wallis test, Dunn test with Holm correction) tests were used to assess the differences among groups relying on either built-in functions or the package dunn.test v1.3.5.46 To perform a multivariate analysis of NOM behaviour within the investigated DWDN, including both the intensity of the fluorescence components identified through PARAFAC and the absorption at 254 nm, such variables were used to estimate the Euclidean distance among samples. Differences among groups of samples were tested using PERMANOVA, as implemented in vegan v2.6-2.47 A principal component analysis (PCA) was used to extract and plot the two most variable components, being equivalent to an ordination in linear space.48
3 Results and discussion
Presently, the excessive time required to obtain results and low sensitivity of traditional analytical techniques used to monitor DWDSs limit prompt management of drinking water distribution systems. Such limitations have recently led to increased interest towards spectroscopic techniques (i.e., absorbance, fluorescence) for the assessment of water quality due to the simplicity of their analytical procedures and the possibility of implementing real-time monitoring systems.16,49 However, it is of paramount importance to better understand the sensitivity of these techniques with respect to the multiple factors affecting water quality, especially in non-disinfected distribution systems fed by NOM-poor groundwater, in which the absolute values of variations could be so low that they are difficult to detect. For this reason, we explored the suitability of the above-mentioned techniques to characterize NOM variability, as early-warning for routinary and extraordinary maintenance interventions. Firstly, we characterize the NOM composition in terms of fluorescent components to evaluate the effective ability of fluorescence coupled to PARAFAC to describe organic matter in a non-disinfected DWDS fed by NOM-poor groundwater. Then, we explored the ability of fluorescence to be used for the monitoring of specific sections of the DWDS (source water, DWTP and DWDN) paying attention to the specificities of these sections (e.g., type of treatment, piping materials).
3.1 Fluorescent organic matter characteristics
The validated PARAFAC model described the fluorescence of the samples collected throughout the DWDS thanks to the use of three components, namely C1, C2 and C3 (Fig. S3†). When compared to previously published fluorescent components, C1 was associated with humic-like components indicated as relatively aliphatic and with low molecular weight; C2, despite still being classified as humic-like, was indicated as representing high molecular weight compounds with signatures related to highly degraded aromatic organic matter.50 Both components correspond to the ones found in other groundwater samples51 and drinking water.52 On the other hand, C3 was classified as protein-like and potentially derived from microbial processes53 and detected in the distribution systems of recycled wastewater.54 Previous studies linked protein-like fluorescence to either bacterial concentrations or microbial activity caused by growth, biofilm detachment or possible release of proteinaceous compounds from biofilm extracellular polymeric substances.15,55,56
As expected for a non-disinfected groundwater-fed DWDS, the observed fluorescence intensities, despite their inherent variations, were lower than those for several disinfected and/or surface water-fed systems typically characterized by higher NOM content.20,25,27,57 The fluorescence intensities were comparable to the ones measured in a disinfected groundwater-fed system24 and the ones present at the outlet of a DWTP of a non-disinfected DWDN.19 However, while lower NOM concentrations tend to result in lower fluorescence intensity, this parameter is affected by several other factors such as, for example, the presence of chemical disinfectants, which confounds the relationship between the NOM concentration (i.e., TOC/DOC) and fluorescence.23,58
3.2 Seasonal variability of water sources
The NOM concentration and composition in raw and treated water are shown in Fig. 1 in terms of fluorescent components, absorbance and TOC concentration. Both fluorescence intensity and UV254 differ between the three DWTP inlets (Dunn test, p-values < 0.001), with the highest values in DWTP B and the lowest in DWTP C. Comparing the different fluorescent components, the majority of the fluorescence belongs to humic-like NOM (i.e., components C1 and C2), rather than the protein-like one (C3), in concordance with the lower biodegradability of humic-like NOM and the link between protein-like fluorescence and contaminated water sources.9 TOC measurements, on the other hand, present only significant differences between the inlets of DWTPs B and C (Tukey HSD, p-value = 0.021). The decrease in NOM content with increasing groundwater depth is concordant with recent results.29 However, while fluorescence and absorbance show overall similar patterns in the DWTP inlets (Spearman correlations; ρ > 0.88, p-values < 0.001), TOC deviates from the other two measurements, showing only limited, if not non-significant (i.e., C3), correlations (Spearman correlations; ρ < 0.47, p-values < 0.044). This divergence might partly be due to the presence of organic molecules not detectable using optical methods such as fluorescence and absorbance.59 However, part of this divergence is also due to the precision of the analytical methods. Indeed, the absolute TOC deviations from the mean value ranged from 1 to 53% (median ± interquartile range = 13 ± 23%), higher than the repeated fluorescence (C1: 2 ± 3%; C2: 2 ± 3%; C3: 4 ± 9%) and absorbance (1 ± 4%) measures, indicating the lower precision of the TOC measurement at the concentrations present in the investigated DWDS. Focusing on the absolute relative deviations observed for the fluorescent components, the greater value observed for C3 highlights the greater difficulty of measuring fluorescence for protein-like components, caused both by the lower fluorescence intensity and technical limitations of the measurement.60,61 Due to the low precision, TOC measurements are not taken into account in further analyses.
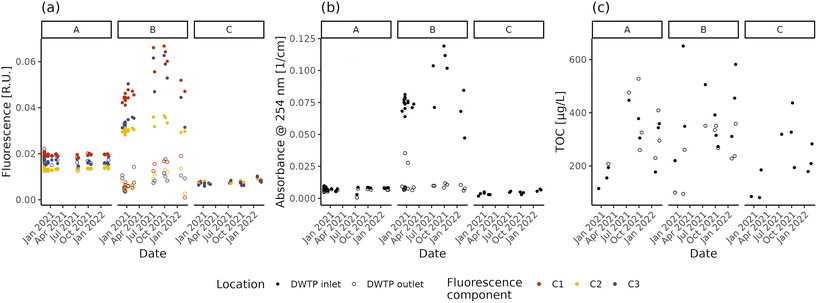 |
| Fig. 1 Intensity of fluorescent components C1, C2 and C3 (a), absorbance (b) and TOC (c) measured at the inlet and outlet of the monitored DWTPs (A–C) as a function of the sampling date. Closed and open markers indicate the DWTP inlet and outlet, respectively. | |
Focusing on the temporal variations, fluorescence and absorbance lead to the same observations. The fluorescence and UV254 at the inlets of DWTP A and C remain mostly stable, presenting only limited (i.e., C3 in DWTP C) or no evidence of monotonic correlations with time (fluorescence: Spearman correlation; p-values > 0.062; UV254: Spearman correlation; ρ = 0.51, p-values = 0.022), possibly suggesting the arrival of a water plume with higher organic content. Conversely, the raw water in DWTP B varies throughout the monitoring period both in fluorescence and UV254, due to the combination of several factors. In fact, DWTP B is characterized by the greatest variation in water extraction throughout the year (ratio between the maximum and minimum monthly values: A = 1.90, B = 2.22, C = 1.59), resulting in the greatest variation of the area of influence. Increased pumping rates might have affected the quality of the abstracted water, as reported by Graham62 and Kwon63 and respective collaborators. Furthermore, other 7 wells (average depth = 18–35 m) are present within 1 km from DWTP B for both irrigation and other uses. Finally, not presenting any clay lens at depths above its well head, DWTP B could also be more influenced by seasonal rainfall recharges.64 The combination of all these factors could have affected groundwater flowlines, possibly altering the water reaching DWTP B.65 Besides changes in the concentration of fluorophores, other factors are known to affect fluorescence measurements, including for instance pH and metal concentrations.66 However, during the monitoring period, the pH values displayed variations below 1 unit and the inlet water temperature did not significantly change across seasons (ANOVA; p-value = 0.097), suggesting their limited effect on the measured values.67 As for metals, except for the correlation between relative fluorescence (i.e., the fraction of the total fluorescence attributed to a specific component) of C1 in DWTP C and Mg (Spearman correlation; ρ = 0.7, p-values = 0.023), neither the intensity of fluorescent components (both absolute or relative) nor the absorption coefficient values present significant correlations with the measured metal concentrations at the DWTP inlets (Spearman correlations; p-values > 0.061). This suggests that the observed values of fluorescence and absorbance are not affected by NOM–metal complexation,35 supporting the attribution of the observed changes to variations of fluorophore concentrations.
In summary, both fluorescence and absorbance can provide indications about the NOM content and composition in source water, which affect the management of the DWTPs. In fact, in non-disinfected DWDSs it is important to feed the network with water having a stable NOM content during the year, to avoid the establishment of conditions favourable to biofilm growth.28 Monitoring this variability is especially critical in the case of relatively shallow and unprotected wells which can show important seasonal fluctuations.
3.3 Treatment removal efficiency
While in the case of DWTP C abstracted water is directly pumped within the DWDN, DWTPs A and B employ ferrous sulphate dosing and, respectively, a sand and a GAC filter. NOM removal efficiency is reported in Fig. 2 for DWTPs A and B, both in terms of fluorescence and absorbance. The rapid sand filter installed in DWTP A (Fig. 2a) shows no significant removal for any fluorescent component (t-test; p-values > 0.3), in agreement with the low removal reported by Yang and collaborators.12 Conversely, similar to the results provided by Vera and collaborators,20 such treatment reduces absorption coefficient values in median by 13% (interquartile range = 9%), indicating the removal of certain chromophoric, but not fluorescent molecules. The increase of absorbance index S275–295 across DWTP A (Fig. 2b) suggests that filtration removes prevalently chromophoric molecules characterized by high molecular weight, SUVA and aromaticity.43,68
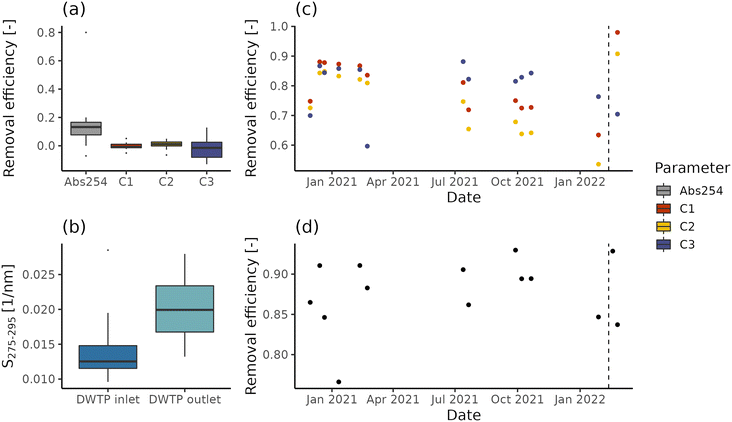 |
| Fig. 2 Removal efficiency of organic matter (a) and the change of absorbance index S275–295 (b) in DWTP A (a and b), and temporal variations in organic matter removal in DWTP B (c and d) in terms of fluorescence (c) and absorbance (d). | |
On the other hand, the GAC filter in DWTP B results in fluorescence removal between 60% and 98% (Fig. 2c), with humic-like components C1 and C2 presenting negative trends until mid-February 2022, due to their breakthrough before the activated carbon substitution (Spearman correlation; C1: ρ = −0.75, p-values = 0.007; C1: ρ = −0.83, p-values < 0.001). Interestingly, the protein-like component C3 does not show any significant trend before the activated carbon substitution (Spearman correlation; p-value = 0.44) and its removal efficiency is not increased by this operation, indicating that the adsorption capacity for protein-like fluorescent compounds was not yet saturated, in contrast with humic-like compounds. In fact, different sorption mechanisms contribute to the removal of humic- and protein-like fluorescent components.60 The removal estimated on UV254 values ranges between 76% and 93% (Fig. 2d) and similar to component C3, it shows neither a negative trend during the monitoring period, nor a marked increase after the activated carbon substitution. These differences are likely caused by the differences in the pools of molecules probed by the two techniques. In fact, while fluorescent molecules are chromophoric, the opposite is not true.59 In addition, while different fluorescent components allow distinct groups of molecules to be tracked, UV254 is a bulk parameter, tracking both allochthonous and autochthonous NOM.69 Besides having a potential detrimental effect in DWDNs, the NOM presence affects the sorption of anthropogenic contaminants by occupying sorption sites or forming complexes.70,71 The breakthrough of such contaminants occurs at different, often higher, bed-volumes than NOM molecules measured by fluorescence and absorbance.72,73
Based on our observations, neither fluorescence nor absorbance provides exhaustive information about NOM removal. Given that likely both chromophoric and fluorescent molecules shape drinking water quality and treatment efficiency (e.g., reaction with disinfectants, if used; adsorption competition, biodegradation and biofilm formation), the analysis of both parameters can provide more information for managing the drinking water infrastructure. For example, the combination of fluorescent components and absorption values for modelling NOM sorption could provide useful management information for DWTP managers regarding the breakthrough of contaminants of interest. Furthermore, even not in this specific case study, a similar technique could be used to assess the DBP precursor removal, given the high correlations between organic matter measurements and DBP formation potential.3 Given these tasks, the ability to disentangle the dynamics of different NOM fractions provided by EEM measurements coupled with PARAFAC analysis would likely provide a greater benefit than absorption coefficients.
3.4 Variability within the distribution network
Due to the high level of interconnection of the DWDN, especially in the proximity of DWTPs A and B (Fig. S1†), it was necessary to assess the origin of the water at each DWDN sampling location before analysing the effect of distribution on the organic matter. Fig. 3 shows the comparison between the estimated contributions of DWTP B using either the calibrated hydraulic model provided by the water utility or the conductivity measurements. In most cases, both methods agree on the source of the water, but in several cases the hydraulic model predicts a different source than what was assessed experimentally. In fact, the model predicts water mixing from DWTPs A and B only in three sampling locations (P05, P12, P13), while this was observed in six locations (P01, P02, P03, P04, P05, P13). Notably, in P09, while not detecting water mixing, the water origin alternated between two sources, while only one source was predicted by the model. In contrast, while water in P12 was predicted to be a mix between the ones from DWTPs A and B, no water mixing was detected. Noteworthily, these differences were found especially during the first sampling period likely due to the enforcement of a “soft” lockdown74,75 which affected water consumption patterns.76 These discrepancies highlight how the DWDS behaviour is not stable in time, but it can vary depending on water consumption. Due to the stochastic behaviour of drinking water demands, their uncertainty should be included in DWDS models. Without its inclusion, calibrated models represent snapshots at a given point in time which might not be representative of other conditions, either due to specific events (i.e., holidays) or fluctuations in consumer behaviors.67 Considering these divergences, it is expected that also the water residence times within the network estimated by the model will not provide an accurate representation of what is occurring within the network and for this reason they were not used.
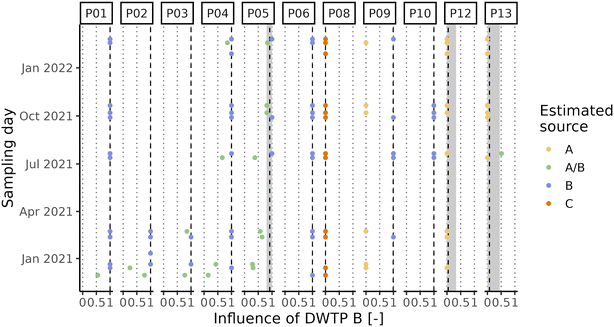 |
| Fig. 3 Estimated source and influence of DWTP B on each DWDN sampling location. Markers show the experimental results based on conductivity measurements, while vertical dashed lines and shaded areas indicate the mean and 90% confidence interval of the influence of DWTP B estimated by the dynamic hydraulic model. The presence of confidence intervals on the DWTP B influence highlights sampling locations where source mixing is expected, while markers not aligned on the dashed lines indicate samples with discording source attribution. | |
A PCA was performed to take into account both the fluorescence and absorbance characteristics of the samples collected at the DWTP outlets and within the DWDN. Fig. 4 shows the projection of the first two PCA components (variance explained = 91.82%) in order to represent the differences of the NOM characteristics in the samples collected. Once more the effect of source mixing is evident: samples influenced by both DWTPs A and B are generically placed between the ones served by the two DWTPs, indicating how the NOM characteristics observed in such samples lie between the ones of the two water sources. Focusing on the samples with water derived from DWTP B, it is possible to observe a shift in their position with time due to the effect on the DWDN of the combination of multiple factors, as mentioned above: the variability of source water characteristics, the GAC filter breakthrough and the activated carbon substitution. Comparing the organic carbon characteristics at the DWTP outlets and within the DWDN, no significant differences are found for the public fountains served by either DWTP A or B (PERMANOVA; A: R2 = 0.13, p-value = 0.08; B: R2 = 0.05, p-value = 0.07). Given the change of NOM characteristics at the outlet of DWTP B across the different seasons, statistical tests were also repeated for each season to remove the possible confounding effect given by this dynamics, although resulting again in no significant differences (PERMANOVA; R2 < 0.1, p-value > 0.067). In fact, even though few samples originating from DWTP B show large differences from DWTP B outlet samples, such variations were not systematically observed in the majority of samples, indicating the predominant stability of NOM quality at such locations. Conversely, significant differences were found between the sampling locations served by DWTP C (P08) and the DWTP outlet (PERMANOVA; R2 = 0.33, p-value < 0.001) and between the samples collected from premise plumbing and the outlet of DWTP A (PERMANOVA; R2 = 0.33, p-value < 0.001).
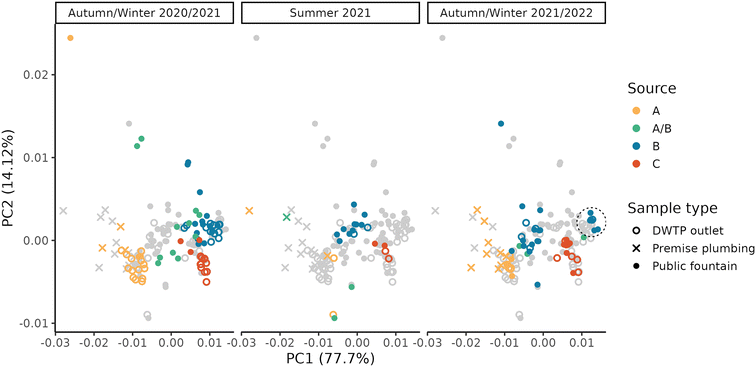 |
| Fig. 4 Principal component plot of the spectroscopic characteristics of the samples collected at the DWTP outlets and within the DWDN. The labels on the top of each panel indicate the period to which the coloured markers belong, while grey markers represent the samples collected in other seasons, in order to aid the comparison among facets. Markers inside the dashed circle indicate the samples from DWTP B collected after the activated carbon substitution. | |
Fig. 5 displays the difference of fluorescence and absorbance characteristics between the samples collected within the DWDN and the daily mean value at the DWTP outlets. By removing the effect of source water and DWTP removal variability, this analysis highlights the effect of water distribution on NOM characteristics, pointing out differences which reach values comparable to the intensities present at the DWTP outlets (Fig. 1). Such analysis highlighted also the extent of the daily dynamics of NOM characteristics at the DWTP outlets which, on average, reached up to about 20% of the mean daily value, although no changes in the DWTP operations besides flowrate were present. Given this intrinsic variability at the inlet of the DWDN, such variations are also expected at consumers’ taps. For this reason, fluctuations of this entity cannot be considered a sign of water instability, but only a sign of the dynamicity of the system. In light of this, variations in water quality can be considered as instability in the case when they are greater than this inherent dynamicity or present a systematic difference compared to the DWTP outlets. Comparing the different fluorescent components, the protein-like component C3 tends to have a larger variability than the humic-like ones (C1 and C2) (Levene test; A: p-value = 0.067; B: p-value < 0.001; C: p-value = 0.058), possibly linked both to the lower measurement precision60 or to variations in the microbiological quality within the DWDN.15,78 The extent of fluorescence and absorbance variability shows differences between the DWTP outlets and the different DWDN sampling locations. This is observable for the samples coming from DWTP A for components C1 and C3, and UV254 (Levene test; p-values < 0.048), while for the samples derived from DWTP B, this occurs only for component C3 (Levene test; p-value = 0.051). Both fluorescent components and UV254 show greater variability at P08 than at the DWTP C outlet (Levene test; p-values < 0.003). Besides the different extent of the variability across DWDN locations, the comparison between the differences at the DWTP outlets and the ones recorded at the sampling points within the DWDN was used to pinpoint specific locations which presented systematically different NOM characteristics from the DWTP outlets. For the samples originating from DWTP A, this occurs, in agreement with the PERMANOVA analysis, at the premise plumbing locations (P12 and P13) for all fluorescent components (Dunn test, Holm correction; p-values < 0.034) and at P09 and P12 for absorbance (Dunn test, Holm correction; p-values < 0.055). Among the sampling locations served by DWTP B, only component C1 at sampling point P10 presents values different from the DWTP outlet (Dunn test, Holm correction; p-values = 0.035). As already highlighted by the PERMANOVA analysis, both all fluorescent components (Wilcox test; p-values < 0.033) and absorbance (Wilcox test; p-value < 0.001) differ between the DWTP C outlet and P08. Looking at the effect of distribution on NOM apparent molecular weight (Fig. 5c), it is possible to observe how the S275–295 index of several samples deviates in many sampling locations from the corresponding DWTP outlet values, resulting in statistically lower values in P04, P08 and P12 (Dunn test, Holm correction; p-values < 0.044). This difference suggests the presence of higher molecular weight compounds at such locations compared to the DWTP outlets.43
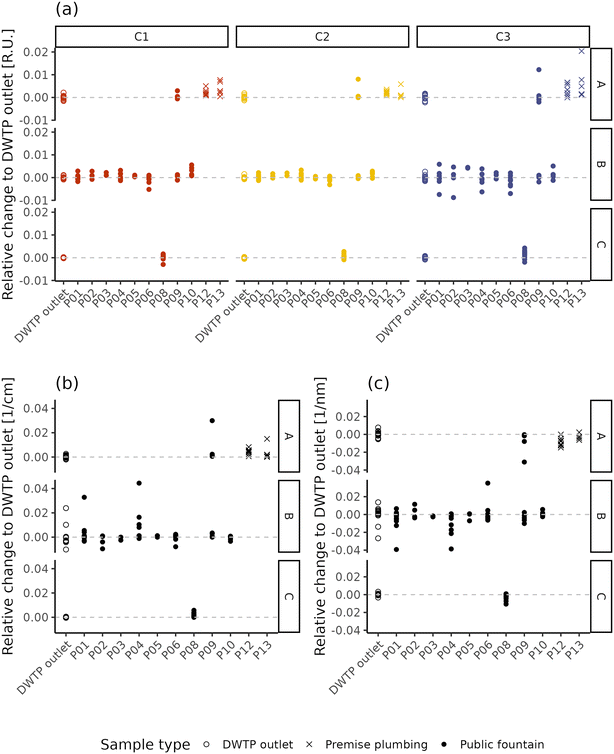 |
| Fig. 5 Relative change of NOM characteristics between the DWTP outlets and DWDN in terms of fluorescence (a) and UV254 (b) and S275–295 (c). | |
Previous research on NOM variations in disinfected DWDNs has highlighted both localized25,27,79 and systematic24,80 variations within DWDSs, stressing the importance of site-specific conditions in determining NOM behaviour. Both the multivariate and the univariate analyses highlight the overall stability of the NOM characteristics within the investigated non-disinfected DWDN, as most sampling points showed no differences with the DWTP outlet NOM characteristics (Fig. 4) and presented differences to the DWTP outlets comparable to the daily fluctuations observed at the DWTP outlets (Fig. 5). Given that variations of around 60% of the TOC content have been linked to changes in the contaminant reaction kinetics,4 it is possible that the variations might also have direct impacts on consumers’ health. However, more studies are needed to assess what are the maximum thresholds (either relative or absolute) which ensure consumers’ safety.
Given the detection of only localized variations of NOM characteristics, the results obtained remark the importance of the localized characteristics of the distribution network and, especially, the “last meter” before consumption, as pointed out by the differences found at certain water fountains (P04, P08, P10) and premise plumbing locations (P12, P13), similar to what was found by Heibati and collaborators in a disinfected system with low disinfectant residuals.27 In fact, even though the variation of component C1 in P10 might be explained by its greater distance from DWTP B compared to most sampling locations (Table S1†), such a difference is not observed in the nearby location P09; this suggests that the variation originates between the water main and the public fountain tap. In fact, in the case when the cause of water quality variation is localized in a specific location along the network (i.e., the “last meter”) the entity of the variation cannot be expected to correlate with water residence time (e.g., ref. 27), as this assumes a continuous rate of change along the distribution (i.e., as the case of the reaction of disinfectant residuals with NOM). Besides localized changes in NOM characteristics caused by premise plumbing, the change observed in P08 might also be caused by the pipe materials on the water mains. In fact, while on the entire network the percentage of plastic materials amounts to only about 17% and mostly in locations not directly affecting sampling locations, the percentage increases up to 60% in the area located in the vicinity of DWTP C. However, given that P08 is the only location supplied by DWTP C, it is not possible to determine whether this difference is due to the composition of the water mains or due to the factors present in the “last meter” before this sampling point. Premise plumbing locations showed remarkably different NOM characteristics compared to public fountains (Fig. 4). However, when observing the variations between such locations and the DWTP outlets (Fig. 5), the sign of the relative change of the monitored parameters observed at these sampling points is mostly consistent with the one observed in the other locations for which a change was detected. A difference lies in the prevalence of such a difference. In fact, different NOM characteristics were observed in 100% of the premise plumbing locations sampled, but only in 44% of the public fountains, indicating how the detection of changes in NOM characteristics is more common at taps located within buildings. Previous research has highlighted the variety of plastic materials used in premise plumbing and pipe fittings81 and their potential leaching of organic molecules.82 Organic leaching from pipes favours biofilm development,7 which can, as well, store, cycle and affect the presence of organic matter in bulkwater.83 Similar to what was indicated by the S275–295 values at some locations, the first layers of biologically activated filters have been shown to increase NOM molecular weight84 suggesting the occurrence of biological NOM transformations within the DWDN. However, due to the difficulties related to sampling water pipes present within DWDSs and, especially, buildings, it was not possible to further explain the changes in NOM characteristics observed. Further studies, possibly conducted with the aid of pilot plants, should focus on elucidating the possible causes responsible for these results. Even though already present in certain water fountains, possibly due to the presence of plastic pipes within the distribution system, these effects are exacerbated in buildings where a greater premise plumbing system is in place, which offers greater surface-to-volume ratios and greater opportunities for biofilm development.81 While the similarity of the sign of relative changes suggests the likeness of processes occurring in these different types of sampling points, such homogeneity in the responses indicates that more sophisticated techniques are needed to better characterize the possible diversity among locations with potential different characteristics. The detection of these variations highlights the role of the materials in contact with drinking water and stresses the importance of their characterization, as recently emphasized by the recent European Directive regarding water for human consumption.85
3.5 Implications for DWDS monitoring and management
Monitoring the quality of drinking water up to the point of use is a key task in water safety plans86 recently introduced in the European drinking water legislation.85 In order to be effective, a monitoring program should be tailored for each individual DWDS, taking into account its specific characteristics.
In the case where multiple sources are present within the same DWDS, distinguishing the water origin within the DWDN is a necessary step to avoid misinterpretation of the results. While this task can be achieved through DWDS simulations, experimental confirmation using a water quality parameter not significantly affected by distribution (e.g., conductivity) is highly recommended. Such verification is needed because, as highlighted in Fig. 3, the water source at single DWDN locations might fluctuate between different DWTPs due to the instantaneous water demands which might deviate from the ones collected for model calibration,77 limiting the usefulness of traditional DWDS models in supporting day to day management. Digital Twins87 and data assimilation techniques88 could solve this limitation, employing immediately the DWDS sensor data for model refinement. In addition, the right attribution of a sampling location to a DWTP is fundamental in the case of an early-warning system focused on water quality, to put in place the most appropriate actions where they are actually needed.
As environmental causes and human interventions can affect groundwater quality,89,90 sampling frequencies should be adapted to track water quality changes either based on previous knowledge or automated algorithms (e.g., ref. 91) possibly intensifying monitoring frequency when variations which could potentially affect water treatment or distribution are expected. Observed values should be compared with the degree of short-term (daily) variability, possibly with confirmatory sampling performed rapidly (24 h) after unusual results, as possibly required in state-level US regulations.92 Finally, sampling locations should be evaluated for their representativeness. The results of this study indicate that premise plumbing systems affect NOM characteristics, leading to distinct results compared to water fountains. On the one hand, when testing the quality of the water at consumers' taps, the ability of fluorescence to detect the changes of organic molecules at consumers' taps due to organic leaching and microbial transformations in specific sections of the network or within premise plumbing could be used to localize and quantify this phenomenon, and to focus further analyses. On the other hand, the presence of this difference highlights the non-representativeness of the water samples collected within buildings to assess NOM quality within DWDN mains. This fact should be taken into account in the design of sampling campaigns, avoiding sampling from premise plumbing locations when assessing the quality of the water distributed in favour of the ones which can be representative of the conditions within the network and not of the specific building sampled.
Besides a correct choice of sampling locations, attribution of water sources and monitoring frequency, the analytical method chosen for monitoring affects what variations can be observed. TOC measurement provides only bulk information of the NOM content, without providing any information on its quality.8,9 In addition, TOC data reliability is questionable in the case of NOM-poor groundwater. On the other hand, fluorescence and absorbance allow us to obtain qualitative information regarding NOM characteristics and better understand the fate of fluorescent and chromophoric NOM.10 While fluorescence measurements present higher sensitivity compared to absorbance,9,93 only a limited fraction of organic molecules can be detected by fluorescence, limiting the extension of the results to the whole NOM pool.10,94 On the other hand, chromophoric molecules make up a larger fraction of the entire NOM pool,59 but environmental samples present mostly featureless spectra, providing often limited qualitative information compared to fluorescence.14 In fact, while the application of PARAFAC to fluorescence data allows us to distinguish between fluorophores with different characteristics and compare them to other spectra to identify matches,42 most absorbance analysis methods involve the estimation of ratios and/or slope coefficients which have been previously correlated to changes in NOM properties.14 While more advanced analyses of absorption data have been developed, such as the Gaussian fitting of differential absorbance spectra, little guidance exists regarding their use with most applications limited to laboratory settings.95–97 Given the mentioned pros and cons, fluorescence measurements in DWDSs seem to be more suitable to track the specific NOM fraction identified by PARAFAC components and characterize its fate during water treatment and its different abundances within DWDSs. On the other hand, absorbance seems to be better suited to describe NOM bulk characteristics and abundance given the ability to probe a larger NOM fraction, while, in contrast, providing less details on its composition.14 For example, while PARAFAC analysis of the fluorescence spectra in DWTP B allowed us to distinguish between the different behaviours of humic-like and protein-like components, only absorbance was able to detect the change in NOM characteristics caused by the treatment in DWTP A as this affected mostly chromophoric compounds. In any case, both approaches could be used to screen for sampling locations with peculiar NOM characteristics which could then be inspected by more advanced targeted or un-targeted methods,11,98 we believe that their combination could be the most valuable option, allowing the limitations of the single methods to be overcome, as shown, for example, in the analysis of the treatment performances shown in section 3.3. Regardless of the aim of the sampling campaign, the analytical method chosen should guarantee sufficient accuracy to allow for the detection of the expected changes. For example, due to the low precision of TOC measurements at the concentrations present in the investigated case study, such a technique would not be able to identify small NOM changes occurring along the network. Regarding the use of fluorescence analysis, Heibati and collaborators27 suggested humic-like fluorescence over protein-like fluorescence as an indicator of external contamination due to its higher stability within the investigated DWDN and higher measurement precision. The validity of such a suggestion is also supported by the results of this monitoring campaign which detected larger fluctuations of protein-like fluorescence compared to the humic-like one. Given the diversity of the water quality in the DWDS monitored, this observation will likely be valid in other distribution systems, representing useful information for water utility managers.
Finally, besides analytical methods, also data analysis methods to detect variations in water quality should be rigorous and statistically-based in order to avoid false positives and the expenses linked to additional verifications. Comparisons should be carried out accounting for the inherent variability of the DWDS behaviour considering both seasonal and daily dynamics. In particular, daily variations at the DWTP outlets can be used as a “baseline variability” against which comparing the one observed in the distribution network. Both univariate and multivariate analyses provide comparable results, as shown in the analysis of the differences occurring within the distribution network. Anyway, the two methods can provide different extents of insights regarding the phenomena affecting water quality, such as the stability of NOM characteristics. In fact, multivariate analyses are more suited to the description of the overall water or NOM quality, while, given their focus on single parameters, univariate analyses are likely more useful when addressing specific hypothesis or to deepen the results of multivariate analyses.
4 Conclusions
In this study, fluorescence and absorbance measurements were used to track NOM characteristics within a non-disinfected DWDS from different NOM-poor groundwater sources to consumers' taps throughout a year. While two of the three monitored groundwater sources were characterized by mostly stable NOM characteristics, a significant variability was found in one of the sources likely due to the combination of changes in DWTP operations and other groundwater abstractions and recharges. Water treatment affected the NOM presence differently between chromophoric and humic-like and protein-like fractions. One DWTP, equipped with a rapid sand filter, provided only a limited reduction of high molecular weight compounds, without affecting fluorescent compounds. In contrast, a second DWTP, characterized by the presence of a GAC filter, showed substantial NOM removal, even though highlighting different behaviours between humic-like, protein-like and chromophoric compounds. Within the DWDN, both multivariate and univariate analyses highlighted general stability of the NOM, while, coincidentally, highlighting variations at specific water fountains and at premise plumbing locations, which showed distinct NOM characteristics from the rest of the DWDN. While this effect was often attributed to localized effects occurring in the “last meter” connections before the point of use, this effect was also detected in an area characterized by a large fraction of plastic pipes in the main distribution network. Besides the NOM variations, the performed sampling campaign identified discrepancies between the actual DWDS behaviour and the one expected by a calibrated hydraulic model, stressing the need to assess experimentally the water origin when sampling within the DWDN.
In conclusion, our results suggest that, in the case of well-managed DWDSs, NOM characteristics are generally stable even in the case of very low NOM concentrations and the absence of residual disinfectants to control the water microbiological quality. While both fluorescence and absorbance can provide valuable information for the management of NOM-poor systems, relying on management decisions on TOC measurements is not recommended due to the limits of this analytical method. In fact, these parameters permit the leaching of organic molecules from plastic materials or organic carbon cycling within biofilms to be studied, especially in the case of fluorescence thanks to the ability to track specific NOM fractions compared to the bulk characteristics provided by absorbance. In any case, monitoring campaigns should be designed to take into account not only the possible variabilities in water source characteristics, but also both the dynamicity of DWDS conditions and the effect of premise plumbing on NOM characteristics which might lead to non-representative results.
Author contributions
MG: conceptualization; methodology; formal analysis; investigation; writing – original draft; visualization. FP: investigation. GB: formal analysis; investigation. MA: conceptualization; writing – review & editing; supervision; funding acquisition.
Conflicts of interest
There are no conflicts of interest to declare.
Acknowledgements
The authors would like to thank CAP Holding S.p.A. (CAP) for funding the Ph.D. scholarship of M. G., Maria Giovanna Guido and Fabio Ferrari for laboratory support and Gloria Salinetti, Cristina Floris and Ambra Lombardo for their helpful cooperation.
References
- S. A. Baghoth, S. K. Maeng, S. G. S. Rodríguez, M. Ronteltap, S. Sharma, M. Kennedy and G. L. Amy, An urban water cycle perspective of natural organic matter (NOM): NOM in drinking water, wastewater effluent, storm water, and seawater, Water Supply, 2008, 8, 701–707 CrossRef CAS.
- Y. Zhang, X. Zhao, X. Zhang and S. Peng, A review of different drinking water treatments for natural organic matter removal, Water Supply, 2015, 15, 442–455 CrossRef CAS.
- Y. Wang, L. Li, Z. Sun, H. Dong, J. Yu and Z. Qiang, Removal of disinfection by-product precursors in drinking water treatment processes: Is fluorescence parallel factor analysis a promising indicator?, J. Hazard. Mater., 2021, 418, 126298 CrossRef CAS.
- P.-L. Rantanen, M. M. Keinänen-Toivola, M. Ahonen, A. González-Martínez, I. Mellin and R. Vahala, Decreased natural organic matter in water distribution decreases nitrite formation in non-disinfected conditions, via enhanced nitrite oxidation, Water Res.: X, 2020, 9, 100069 CAS.
- C.-Y. Peng, J. F. Ferguson and G. V. Korshin, Effects of chloride, sulfate and natural organic matter (NOM) on the accumulation and release of trace-level inorganic contaminants from corroding iron, Water Res., 2013, 47, 5257–5269 CrossRef CAS PubMed.
- I. Kasuga, M. Suzuki, F. Kurisu and H. Furumai, Molecular-level characterization of biodegradable organic matter causing microbial regrowth in drinking water by non-target screening using Orbitrap mass spectrometry, Water Res., 2020, 184, 116130 CrossRef CAS PubMed.
- S. Liu, C. Gunawan, N. Barraud, S. A. Rice, E. J. Harry and R. Amal, Understanding, Monitoring, and Controlling Biofilm Growth in Drinking Water Distribution Systems, Environ. Sci. Technol., 2016, 50, 8954–8976 CrossRef CAS PubMed.
- Y. Pan, H. Li, X. Zhang and A. Li, Characterization of natural organic matter in drinking water: Sample preparation and analytical approaches, Trends Environ. Anal. Chem., 2016, 12, 23–30 CrossRef CAS.
- W. Shi, W.-E. Zhuang, J. Hur and L. Yang, Monitoring dissolved organic matter in wastewater and drinking water treatments using spectroscopic analysis and ultra-high resolution mass spectrometry, Water Res., 2021, 188, 116406 CrossRef CAS PubMed.
- W. Chen and H.-Q. Yu, Advances in the characterization and monitoring of natural organic matter using spectroscopic approaches, Water Res., 2021, 190, 116759 CrossRef CAS PubMed.
- M. Derrien, S. R. Brogi and R. Gonçalves-Araujo, Characterization of aquatic organic matter: Assessment, perspectives and research priorities, Water Res., 2019, 163, 114908 CrossRef CAS PubMed.
- L. Yang, J. Hur and W. Zhuang, Occurrence and behaviors of fluorescence EEM-PARAFAC components in drinking water and wastewater treatment systems and their applications: a review, Environ. Sci. Pollut. Res., 2015, 22, 6500–6510 CrossRef CAS PubMed.
- L. Li, Y. Wang, W. Zhang, S. Yu, X. Wang and N. Gao, New advances in fluorescence excitation-emission matrix spectroscopy for the characterization of dissolved organic matter in drinking water treatment: A review, Chem. Eng. J., 2020, 381, 122676 CrossRef CAS.
- P. Li and J. Hur, Utilization of UV-Vis spectroscopy and related data analyses for dissolved organic matter (DOM) studies: A review, Crit. Rev. Environ. Sci. Technol., 2017, 47, 131–154 CrossRef CAS.
- J. Bridgeman, A. Baker, D. Brown and J. B. Boxall, Portable LED fluorescence instrumentation for the rapid assessment of potable water quality, Sci. Total Environ., 2015, 524–525, 338–346 CrossRef CAS.
- E. M. Carstea, C. L. Popa, A. Baker and J. Bridgeman,
In situ fluorescence measurements of dissolved organic matter: A review, Sci. Total Environ., 2020, 699, 134361 CrossRef CAS PubMed.
- S. S. Ruhala and J. P. Zarnetske, Using in situ optical sensors to study dissolved organic carbon dynamics of streams and watersheds: A review, Sci. Total Environ., 2017, 575, 713–723 CrossRef CAS PubMed.
- J. P. R. Sorensen, D. J. Lapworth, B. P. Marchant, D.
C. W. Nkhuwa, S. Pedley, M. E. Stuart, R. A. Bell, M. Chirwa, J. Kabika, M. Liemisa and M. Chibesa,
In situ tryptophan-like fluorescence: A real-time indicator of faecal contamination in drinking water supplies, Water Res., 2015, 81, 38–46 CrossRef CAS PubMed.
- S. A. Baghoth, S. K. Sharma and G. L. Amy, Tracking natural organic matter (NOM) in a drinking water treatment plant using fluorescence excitation–emission matrices and PARAFAC, Water Res., 2011, 45, 797–809 CrossRef CAS PubMed.
- M. Vera, S. Cruz, M. R. Boleda, J. Mesa, J. Martín-Alonso, S. Casas, O. Gibert and J. L. Cortina, Fluorescence spectroscopy and parallel factor analysis as a dissolved organic monitoring tool to assess treatment performance in drinking water trains, Sci. Total Environ., 2017, 584–585, 1212–1220 CrossRef CAS PubMed.
- M. Yan, D. Dryer and G. V. Korshin, Spectroscopic characterization of changes of DOM deprotonation–protonation properties in water treatment processes, Chemosphere, 2016, 148, 426–435 CrossRef CAS PubMed.
-
Water Quality in Drinking Water Distribution Systems, ed. M. Blokker, J. Van Summeren and V. L. Speight, MDPI, 2020 Search PubMed.
- T. Maqbool, J. Zhang, Y. Qin, M. Bilal Asif, Q. Viet Ly and Z. Zhang, Fluorescence moieties as a surrogate for residual chlorine in three drinking water networks, Chem. Eng. J., 2021, 411, 128519 CrossRef CAS.
- L. Kurajica, M. Ujević Bošnjak, A. S. Kinsela, J. Štiglić, T. D. Waite, K. Capak and Z. Pavlić, Effects of changing supply water quality on drinking water distribution networks: Changes in NOM optical properties, disinfection byproduct formation, and Mn deposition and release, Sci. Total Environ., 2021, 762, 144159 CrossRef CAS PubMed.
- H.-J. Wang, Y. Wang, X. Han, J. Zhang, J.-L. Liu, Y.-F. Xiang, M.-X. Zhou, P.-L. Guo, F. Tang and A.-L. Liu, Monitoring DOM in drinking water supply systems using DOC, COD-Mn, UV and fluorescence measurements, Environ. Sci.: Water Res. Technol., 2021, 7, 2307–2320 RSC.
- X. Han, X. Ji, X. Ma, J.-L. Liu, Z.-Y. He, W. Chang, F. Tang and A.-L. Liu, An investigation of changes in water quality throughout the drinking water production/distribution chain using toxicological and fluorescence analyses, J. Environ. Sci., 2020, 87, 310–318 CrossRef CAS PubMed.
- M. Heibati, C. A. Stedmon, K. Stenroth, S. Rauch, J. Toljander, M. Säve-Söderbergh and K. R. Murphy, Assessment of drinking water quality at the tap using fluorescence spectroscopy, Water Res., 2017, 125, 1–10 CrossRef CAS PubMed.
- E. I. Prest, F. Hammes, M. C. M. van Loosdrecht and J. S. Vrouwenvelder, Biological Stability of Drinking Water: Controlling Factors, Methods, and Challenges, Front. Microbiol., 2016, 7, 45 Search PubMed.
- L. K. McDonough, I. R. Santos, M. S. Andersen, D. M. O'Carroll, H. Rutlidge, K. Meredith, P. Oudone, J. Bridgeman, D. C. Gooddy, J. P. R. Sorensen, D. J. Lapworth, A. M. MacDonald, J. Ward and A. Baker, Changes in global groundwater organic carbon driven by climate change and urbanization, Nat. Commun., 2020, 11, 1279 CrossRef CAS PubMed.
-
American Public Health Association, American Water Works Association and Water Environment Federation and American Public Health Association, Standard methods for the examination of water and wastewaters, ed. R. B. Baird, A. D. Eaton and E. W. Rice, Washington, DC, 23rd edn, 2017 Search PubMed.
-
Aquatic Organic Matter Fluorescence, ed. P. G. Coble, J. Lead, A. Baker, D. M. Reynolds and R. G. M. Spencer, Cambridge University Press, 1st edn, 2014 Search PubMed.
- K. R. Murphy, K. D. Butler, R. G. M. Spencer, C. A. Stedmon, J. R. Boehme and G. R. Aiken, Measurement of Dissolved Organic Matter Fluorescence in Aquatic Environments:
An Interlaboratory Comparison, Environ. Sci. Technol., 2010, 44, 9405–9412 CrossRef CAS.
-
APAT/IRSA-CNR, Metodi analitici per le acque, Roma, 2003 Search PubMed.
-
ISO, Water quality. Application of inductively coupled plasma mass spectrometry (ICP-MS) Determination of selected elements including uranium isotopes (ISO 17294-2:2016), 2016 Search PubMed.
- J. Adusei-Gyamfi, B. Ouddane, L. Rietveld, J.-P. Cornard and J. Criquet, Natural organic matter-cations complexation and its impact on water treatment: A critical review, Water Res., 2019, 160, 130–147 CrossRef CAS PubMed.
- J. P. Gustafsson, I. Persson, A. G. Oromieh, J. W. J. van Schaik, C. Sjöstedt and D. B. Kleja, Chromium(III) Complexation to Natural Organic Matter: Mechanisms and Modeling, Environ. Sci. Technol., 2014, 48, 1753–1761 CrossRef CAS PubMed.
- F.-J. Delisle, S. Rochette, G. Pelletier and M. J. Rodriguez, Tracer study to verify hydraulic limits and determine water residence times in a distribution system: Part I, J. Water Supply: Res. Technol.--AQUA, 2015, 64, 365–377 CrossRef.
- P. J. Skipworth, J. Machell and A. J. Saul, Empirical travel time estimation in a distribution network, Proceedings of the Institution of Civil Engineers - Water and Maritime Engineering, 2002, 154(1), 41–49 CrossRef.
- K. R. Murphy, C. A. Stedmon, D. Graeber and R. Bro, Fluorescence spectroscopy and multi-way techniques. PARAFAC, Anal. Methods, 2013, 5, 6557 RSC.
- D. N. Kothawala, K. R. Murphy, C. A. Stedmon, G. A. Weyhenmeyer and L. J. Tranvik, Inner filter correction of dissolved organic matter fluorescence: Correction of inner filter effects, Limnol. Oceanogr.: Methods, 2013, 11, 616–630 CrossRef.
- U. J. Wünsch and K. Murphy, A simple method to isolate fluorescence spectra from small dissolved organic matter datasets, Water Res., 2021, 190, 116730 CrossRef PubMed.
- K. R. Murphy, C. A. Stedmon, P. Wenig and R. Bro, OpenFluor- an online spectral library of auto-fluorescence by organic compounds in the environment, Anal. Methods, 2014, 6, 658–661 RSC.
- J. R. Helms, A. Stubbins, J. D. Ritchie, E. C. Minor, D. J. Kieber and K. Mopper, Absorption spectral slopes and slope ratios as indicators of molecular weight, source, and photobleaching of chromophoric dissolved organic matter, Limnol. Oceanogr., 2008, 53, 955–969 CrossRef.
-
R Core Team, R: A Language and Environment for Statistical Computing, R Foundation for Statistical Computing, Vienna, Austria, 2023 Search PubMed.
-
J. Fox and S. Weisberg, An R Companion to Applied Regression, Sage, Thousand Oaks CA, 3rd edn, 2019 Search PubMed.
-
A. Dinno, dunn.test: Dunn's Test of Multiple Comparisons Using Rank Sums, 2017 Search PubMed.
-
J. Oksanen, G. L. Simpson, F. G. Blanchet, R. Kindt, P. Legendre, P. R. Minchin, R. B. O'Hara, P. Solymos, M. H. H. Stevens, E. Szoecs, H. Wagner, M. Barbour, M. Bedward, B. Bolker, D. Borcard, G. Carvalho, M. Chirico, M. D. Caceres, S. Durand, H. B. A. Evangelista, R. FitzJohn, M. Friendly, B. Furneaux, G. Hannigan, M. O. Hill, L. Lahti, D. McGlinn, M.-H. Ouellette, E. R. Cunha, T. Smith, A. Stier, C. J. F. T. Braak and J. Weedon, vegan: Community Ecology, Package, R package version 2.6-4, 2022 Search PubMed.
-
P. Legendre and L. Legendre, Numerical ecology, Elsevier, Amsterdam, 3rd edn, 2012 Search PubMed.
- Z. Shi, C. W. K. Chow, R. Fabris, J. Liu and B. Jin, Applications of Online UV-Vis Spectrophotometer for Drinking Water Quality Monitoring and Process Control: A Review, Sensors, 2022, 22, 2987 CrossRef CAS.
- P. L. Tomco, R. C. Zulueta, L. C. Miller, P. A. Zito, R. W. Campbell and J. M. Welker, DOC export is exceeded by C fixation in May Creek: A late-successional watershed of the Copper River Basin, Alaska, PLoS One, 2019, 14, e0225271 CrossRef CAS PubMed.
- H. V. Kulkarni, N. Mladenov, K. H. Johannesson and S. Datta, Contrasting dissolved organic matter quality in groundwater in Holocene and Pleistocene aquifers and implications for influencing arsenic mobility, Appl. Geochem., 2017, 77, 194–205 CrossRef CAS.
- Y. Shutova, A. Baker, J. Bridgeman and R. K. Henderson, Spectroscopic characterisation of dissolved organic matter changes in drinking water treatment: From PARAFAC analysis to online monitoring wavelengths, Water Res., 2014, 54, 159–169 CrossRef CAS PubMed.
- S.-A. Lee, T.-H. Kim and G. Kim, Tracing terrestrial versus marine sources of dissolved organic carbon in a coastal bay using stable carbon isotopes, Biogeosciences, 2020, 17, 135–144 CrossRef CAS.
- K. R. Murphy, A. Hambly, S. Singh, R. K. Henderson, A. Baker, R. Stuetz and S. J. Khan, Organic Matter Fluorescence in Municipal Water Recycling Schemes: Toward a Unified PARAFAC Model, Environ. Sci. Technol., 2011, 45, 2909–2916 CrossRef CAS PubMed.
- K. Fish, A. M. Osborn and J. B. Boxall, Biofilm structures (EPS and bacterial communities) in drinking water distribution systems are conditioned by hydraulics and influence discolouration, Sci. Total Environ., 2017, 593–594, 571–580 CrossRef CAS PubMed.
- B. G. Fox, R. M. S. Thorn, A. M. Anesio and D. M. Reynolds, The in situ bacterial production of fluorescent organic matter; an investigation at a species level, Water Res., 2017, 125, 350–359 CrossRef CAS PubMed.
- T. Maqbool, C. Li, Y. Qin, J. Zhang, M. B. Asif and Z. Zhang, A year-long cyclic pattern of dissolved organic matter in the tap water of a metropolitan city revealed by fluorescence spectroscopy, Sci. Total Environ., 2021, 771, 144850 CrossRef CAS PubMed.
- D. L. Gone, J.-L. Seidel, C. Batiot, K. Bamory, R. Ligban and J. Biemi, Using fluorescence spectroscopy EEM to evaluate the efficiency of organic matter removal during coagulation–flocculation of a tropical surface water (Agbo reservoir), J. Hazard. Mater., 2009, 172, 693–699 CrossRef CAS PubMed.
- J. D'Andrilli, V. Silverman, S. Buckley and F. L. Rosario-Ortiz, Inferring Ecosystem Function from Dissolved Organic Matter Optical Properties: A Critical Review, Environ. Sci. Technol., 2022, 56, 11146–11161 CrossRef PubMed.
- N. Moona, U. J. Wünsch, M. Bondelind, O. Bergstedt, T. Sapmaz, T. J. R. Pettersson and K. R. Murphy, Temperature-dependent mechanisms of DOM removal by biological activated carbon filters, Environ. Sci.: Water Res. Technol., 2019, 5, 2232–2241 RSC.
- K. R. Murphy, S. A. Timko, M. Gonsior, L. C. Powers, U. J. Wünsch and C. A. Stedmon, Photochemistry Illuminates Ubiquitous Organic Matter Fluorescence Spectra, Environ. Sci. Technol., 2018, 52, 11243–11250 CrossRef CAS PubMed.
- P. W. Graham, A. Baker and M. S. Andersen, Dissolved Organic Carbon Mobilisation in a Groundwater System Stressed by Pumping, Sci. Rep., 2015, 5, 18487 CrossRef CAS PubMed.
- M. J. Kwon, R. A. Sanford, J. Park, M. F. Kirk and C. M. Bethke, Microbiological Response to Well Pumping, Ground Water, 2008, 46, 286–294 CrossRef CAS PubMed.
- D. Sartirana, M. Rotiroti, T. Bonomi, M. De Amicis, V. Nava, L. Fumagalli and C. Zanotti, Data-driven decision management of urban underground infrastructure through groundwater-level time-series cluster analysis: the case of Milan (Italy), Hydrogeol. J., 2022, 30, 1157–1177 CrossRef.
- M. De Caro, R. Perico, G. B. Crosta, P. Frattini and G. Volpi, A regional-scale conceptual and numerical groundwater flow model in fluvio-glacial sediments for the Milan Metropolitan area (Northern Italy), J. Hydrol. Reg. Stud., 2020, 29, 100683 CrossRef.
-
C. Osburn, R. Del Vecchio and T. Boyd, in Aquatic Organic Matter Fluorescence, ed. P. Coble, J. Lead, A. Baker, D. Reynolds and R. G. M. Spencer, Cambridge University Press, 2014, pp. 233–277 Search PubMed.
- M. Groeneveld, N. Catalán, K. Einarsdottir, A. G. Bravo and D. N. Kothawala, The influence of pH on dissolved organic matter fluorescence in inland waters, Anal. Methods, 2022, 14, 1351–1360 RSC.
- P. Roccaro, M. Yan and G. V. Korshin, Use of log-transformed absorbance spectra for online monitoring of the reactivity of natural organic matter, Water Res., 2015, 84, 136–143 CrossRef CAS PubMed.
- N. Moona, A. Holmes, U. J. Wünsch, T. J. R. Pettersson and K. R. Murphy, Full-Scale Manipulation of the Empty Bed Contact Time to Optimize Dissolved Organic Matter Removal by Drinking Water Biofilters, ACS ES&T Water, 2021, 1, 1117–1126 Search PubMed.
- D. N. Kothawala, S. J. Köhler, A. Östlund, K. Wiberg and L. Ahrens, Influence of dissolved organic matter concentration and composition on the removal efficiency of perfluoroalkyl substances (PFASs) during drinking water treatment, Water Res., 2017, 121, 320–328 CrossRef CAS PubMed.
- Y. Matsui, Y. Fukuda, T. Inoue and T. Matsushita, Effect of natural organic matter on powdered activated carbon adsorption of trace contaminants: characteristics and mechanism of competitive adsorption, Water Res., 2003, 37, 4413–4424 CrossRef CAS PubMed.
- T. Anumol, M. Sgroi, M. Park, P. Roccaro and S. A. Snyder, Predicting trace organic compound breakthrough in granular activated carbon using fluorescence and UV absorbance as surrogates, Water Res., 2015, 76, 76–87 CrossRef CAS PubMed.
- B. Cantoni, A. Turolla, J. Wellmitz, A. S. Ruhl and M. Antonelli, Perfluoroalkyl substances (PFAS) adsorption in drinking water by granular activated carbon: Influence of activated carbon and PFAS characteristics, Sci. Total Environ., 2021, 795, 148821 CrossRef CAS PubMed.
-
Gazzetta ufficiale, Decreto del Presidente del Consiglio dei Ministri 3 novembre 2020, 2020 Search PubMed.
-
Ministero della Salute, Decreto Ministeriale 30 aprile 2020, 2020 Search PubMed.
- S. Alvisi, M. Franchini, C. Luciani, I. Marzola and F. Mazzoni, Effects of the COVID-19 Lockdown on Water Consumptions: Northern Italy Case Study, J. Water Resour. Plan. Manag., 2021, 147, 05021021 CrossRef.
- B. M. Brentan, E. Luvizotto Jr., M. Herrera, J. Izquierdo and R. Pérez-García, Hybrid regression model for near real-time urban water demand forecasting, J. Comput. Appl. Math., 2017, 309, 532–541 CrossRef.
- L. Kurajica, M. Ujević Bošnjak, A. S. Kinsela, M. Bieroza, J. Štiglić, T. D. Waite, K. Capak and Ž. Romić, Mixing of arsenic-rich groundwater and surface water in drinking water distribution systems: Implications for contaminants, disinfection byproducts and organic components, Chemosphere, 2022, 292, 133406 CrossRef CAS PubMed.
- S. Moradi, E. Sawade, R. Aryal, C. W. K. Chow, J. van Leeuwen, M. Drikas, D. Cook and R. Amal, Tracking changes in organic matter during nitrification using fluorescence excitation–emission matrix spectroscopy coupled with parallel factor analysis (FEEM/PARAFAC), J. Environ. Chem. Eng., 2018, 6, 1522–1528 CrossRef CAS.
- X. Han, X. Ji, X. Ma, J.-L. Liu, Z.-Y. He, W. Chang, F. Tang and A.-L. Liu, An investigation of changes in water quality throughout the drinking water production/distribution chain using toxicological and fluorescence analyses, J. Environ. Sci., 2020, 87, 310–318 CrossRef CAS PubMed.
- L. Neu and F. Hammes, Feeding the Building Plumbing Microbiome: The Importance of Synthetic Polymeric Materials for Biofilm Formation and Management, Water, 2020, 12, 1774 CrossRef.
- A. J. Whelton and T. Nguyen, Contaminant Migration From Polymeric Pipes Used in Buried Potable Water Distribution Systems: A Review, Crit. Rev. Environ. Sci. Technol., 2013, 43, 679–751 CrossRef.
- F. C. Pick, K. E. Fish and J. B. Boxall, Assimilable organic carbon cycling within drinking water distribution systems, Water Res., 2021, 198, 117147 CrossRef CAS PubMed.
- J. Kang, S. Liu, T. Ma and X. Gao, Production mechanism and characteristics of dissolved organic nitrogen derived from soluble microbial products (SMPs-DON) in a drinking water biological aerated filter, Water Supply, 2019, 19, 1994–2000 CrossRef.
-
European Commission, Directive (EU) 2020/2184 of the European Parliament and of the Council of 16 December 2020 on the quality of water intended for human consumption, 2020 Search PubMed.
-
World Health Organization, Guidelines for drinking-water quality: fourth edition incorporating first addendum, World Health Organization, Geneva, 4th edn + 1st add., 2017 Search PubMed.
- P. Conejos Fuertes, F. Martínez Alzamora, M. Hervás Carot and J. C. Alonso Campos, Building and exploiting a Digital Twin for the management of drinking water distribution networks, Urban Water J., 2020, 17, 704–713 CrossRef.
- X. Zhou, S. Guo, K. Xin, W. Xu, T. Tao and H. Yan, Maintaining the long-term accuracy of water distribution models with data assimilation methods: A comparative study, Water Res., 2022, 226, 119268 CrossRef CAS PubMed.
- J. D. Ayotte, Z. Szabo, M. J. Focazio and S. M. Eberts, Effects of human-induced alteration of groundwater flow on concentrations of naturally-occurring trace elements at water-supply wells, Appl. Geochem., 2011, 26, 747–762 CrossRef CAS.
- N. M. Burri, R. Weatherl, C. Moeck and M. Schirmer, A review of threats to groundwater quality in the anthropocene, Sci. Total Environ., 2019, 684, 136–154 CrossRef CAS PubMed.
- M. Gabrielli, F. Trovò and M. Antonelli, Automatic optimization of temporal monitoring schemes dealing with daily water contaminant concentration patterns, Environ. Sci.: Water Res. Technol., 2022, 8, 2099–2113 RSC.
-
USEPA, National Primary Drinking Water Regulation, 2022 Search PubMed.
- R. K. Henderson, A. Baker, K. R. Murphy, A. Hambly, R. M. Stuetz and S. J. Khan, Fluorescence as a potential monitoring tool for recycled water systems: A review, Water Res., 2009, 43, 863–881 CrossRef CAS PubMed.
-
G. Aiken, in Aquatic Organic Matter Fluorescence, ed. P. Coble, J. Lead, A. Baker, D. Reynolds and R. G. M. Spencer, 2014, pp. 35–74 Search PubMed.
- B. Chen, C. Zhang, Y. Zhao, D. Wang, G. V. Korshin, J. Ni and M. Yan, Interpreting main features of the differential absorbance spectra of chlorinated natural organic matter: Comparison of the experimental and theoretical spectra of model compounds, Water Res., 2020, 185, 116206 CrossRef CAS PubMed.
- S. Huang, W. Gan, M. Yan, X. Zhang, Y. Zhong and X. Yang, Differential UV–vis absorbance can characterize the reaction of organic matter with ClO2, Water Res., 2018, 139, 442–449 CrossRef CAS PubMed.
- M. Yan, D. Dryer, G. V. Korshin and M. F. Benedetti,
In situ study of binding of copper by fulvic acid: Comparison of differential absorbance data and model predictions, Water Res., 2013, 47, 588–596 CrossRef CAS PubMed.
- K. Kadokami and D. Ueno, Comprehensive Target Analysis for 484 Organic Micropollutants in Environmental Waters by the Combination of Tandem Solid-Phase Extraction and Quadrupole Time-of-Flight Mass Spectrometry with Sequential Window Acquisition of All Theoretical Fragment-Ion Spectra Acquisition, Anal. Chem., 2019, 91, 7749–7755 CrossRef CAS PubMed.
|
This journal is © The Royal Society of Chemistry 2024 |