Artificial neural networks to investigate the bioavailability of selenium nanoparticles in soil–crop systems†
Received
21st June 2023
, Accepted 8th December 2023
First published on 15th December 2023
Abstract
While selenium nanoparticles (Se NPs) can effectively enrich crop yield and quality, the limited research on the interactions between Se NPs and soil–crop systems hinders their potential use in agriculture. Hence, the soil application of Se NPs (0 [control] and 0.5 mg kg−1) and Na2SeO3 (1.11 mg kg−1) was used to enhance rice quality and yield. The artificial neural network (ANN) approach was used to model and simulate the response of soil properties (SPs) and plant physiological activities (PPAs) under different treatments at different time stages (30, 60, 90, and 120 days). The results indicate that Se NPs can enhance photosynthesis, leading to increased yield (1.33-fold) and quality of rice (Se-enriched rice, 3.46-fold). The effects of Se NPs on rice growth and development were found to be time-dependent. Soil properties, including soil organic matter (TOC), ammonium nitrogen (NH4+), pH, redox potential (Eh), and conductivity (Ec), emerged as crucial factors influencing the observed effects. With the progression of time, plant physiological activities, including chlorophyll (Chl), net photosynthetic rate (Pn), stomatal conductance (Gs), and optimal/maximal photochemical efficiency of PS II in the dark (Fv/Fm), exhibited an increasing level of importance. Moreover, the processes of Se NPs affecting the yield and quality were distinct, with TOC being more important for rice yield and Ec being more significant for quality. Therefore, this study offers a novel approach to assess the bioavailability of Se NPs in soil–crop systems and provides valuable insights into the potential for using Se NPs to enhance rice productivity and quality. The use of model-based interpretation methods combined with experimental data allows for a more comprehensive understanding of the advantages and disadvantages of NPs in soil–plant systems and facilitates the implementation of safe design options for NPs in agriculture.
Environmental significance
Emerging nano-agricultural technologies have the potential to enhance crop yield and quality while reducing environmental pollution compared to traditional formulations. However, soil–crop systems exhibit complex interactions of factors that hinder the study of nanoparticle (NP) bioavailability. Therefore, this study proposes the use of artificial neural networks (ANNs) for a systematic evaluation of Se NP applications in the plant–soil system. Model-based interpretation methods combined with experimental data allow for a more comprehensive understanding of the advantages and disadvantages of NPs in the soil–plant system. This approach facilitates the implementation of safe design options for NPs in agriculture, ensuring the development of NP-based solutions that are both effective and environmentally sustainable.
|
1 Introduction
In recent years, sustainable agriculture has become crucial in addressing population pressure (9.7 billion by 2050).1 The emerging nano-agricultural technologies (nanopesticides, nanofertilizers, and nanosensors) have the potential to improve crop yield and quality while reducing environmental pollution compared with traditional formulations.2–4 For instance, nanopesticides can specifically target pests, reducing the need for large quantities of pesticides and minimizing the exposure of non-target organisms to harmful chemicals.5,6 Nanofertilizers can increase the efficiency of nutrient uptake by plants and reduce fertilizer requirements for the same yield.7,8 Consequently, this can reduce chemical runoff and environmental damage associated with traditional agricultural practices.9,10 As such, nano-agricultural technologies have the potential to transform traditional agriculture into a sustainable and efficient system capable of meeting the food and nutritional demands of a rapidly growing population while minimizing environmental impacts.11,12
To explain the mechanisms of NPs promoting plant growth, most previous studies have focused on exploring the physiological and molecular responses of NPs to plants.13 Soil–crop systems exhibit complex interactions of factors that hinder the study of the bioavailability of NPs. Therefore, novel approaches, such as machine learning, are necessary to overcome these limitations and enable more systematic research.14 Recent studies have utilized machine learning methods to develop agronomic-based models.15–17 Given the complexity and diversity of soil–crop systems, artificial neural networks (ANNs) are a feasible solution.18 The advantages of ANNs include suitability for managing uncertain data, applicability to large or small datasets, and the ability to reveal nonlinear relationships between various parameters. Previous studies have demonstrated the superior accuracy of ANNs compared with traditional modeling approaches in learning the relationships between variables and targets. For example, Gandhi et al. predicted rice crop yield using multilayer perceptron ANN (accuracy, 97.5%; sensitivity, 96.3%; specificity, 98.1%).19 Some researchers examined the classification of rice leaf diseases using attention-based depthwise separable ANN, achieving an accuracy of 96.45%.20 Rossi et al. (2019) used an ANN to identify key physiological factors (i.e., root fresh weight, the net photosynthesis rate, and Fv/Fm ratio) affecting Brassica napus plant uptake of co-occurring Cd and CeO2 NPs.18 Although ANN has been applied in agriculture systems, its utilization in investigating the bioavailability of NPs in soil–crop systems is limited.
Selenium (Se) NPs have attracted great attention among various types of NPs due to their unique structural, optical, and electronic properties.21 Compared with traditional Se fertilizers, Se NPs have shown more significant advantages in crop Se-enrichment and yield.22 For instance, Li et al. proposed that the Se NPs (20 mg L−1) could enhance the nutrient quality (chlorophyll, +33.7%; soluble sugar, +36.9%; AsA, +48.2%; flavonoids, +79.9%; total phenols, +58.7%) of pepper (Capsicum annuum L.) by activating the capsaicinoid synthetic pathway.23 Cheng et al. demonstrated that foliar sprayed Se NPs (10 mg L−1) could improve the yield (+67.6%) and nutritional quality (Se content, 5.4 and 2.6 times in pericarp and pulp) of cherry radish (Raphanus sativus L. var. radculus pers).24 At some concentrations, Se NPs enhanced the plant root growth, while Se ions inhibited callus growth and root regeneration.25 Hence, Se NPs could be used for Se enrichment in crops and sustainable agriculture. Moreover, consuming selenium-enriched crops provides health benefits to humans. Despite the potential benefits, there is currently a lack of systematic evaluations regarding the effectiveness of Se NP applications in the plant–soil system.
In our study, rice was selected as the test crop because it is one of the main grain crops in the world. More importantly, more than 1/5th of rice is planted in the severely Se deficient areas, thus Se enrichment in rice is imminent. The aim of this study was to investigate the bioavailability of Se NP in a soil–crop system using ANN. The main objectives of this study were (i) to determine the effects of Se NPs on soil properties and physiological activities of rice and (ii) to distinguish whether the physicochemical activity in the soil–crop system has a more profound effect on yield and quality by using ANNs. By conducting a comprehensive investigation into the utilization of Se NPs in the soil–crop system, the findings of this study will significantly contribute to the effective implementation of Se NPs for enhancing both rice yield and quality.
2 Methods
2.1. Synthesis of materials
Se NPs were synthesized according to previous reports.26 SeO2 (0.11 g) and ascorbic acid (0.36 g) were ground in a mortar until they turned red. Then, Se NPs were dispersed in deionized water (20 mL). Subsequently, polyvinylpyrrolidone was added to the mixture in the proportion of 3
:
20. Eventually, Se NPs were collected after ultrasonic treatment for 30 minutes and centrifugation (8000 rpm, 10 min). The characteristics of Se NPs were reported in our previous study.27
2.2. Rice cultivation and determination of physicochemical activity
Rice (Oryza sativa L.) seeds from Anhui Lvyi Seed Industry Co., Ltd. were soaked in deionized water for 8 h and then in 1% sodium hypochlorite for 10 min. Then, the seeds were washed with deionized water until the solution on the surface of the seeds were removed, and the seeds were placed in a dark environment to avoid light for 3 days. Rice seedlings with uniform germination and growth were selected to be transferred to a pot containing 5 kg paddy soil (2 plants per pot), which was filtered through a 5.0 mm sieve in advance to make the soil homogeneous. Finally, 0.5 mg of Se NPs were added to the pot of the Se NPs treatment group (0.5 mg kg−1), while 1.11 mg of sodium selenite (45.0% selenium content) was added to the Se ions (SeO32−) treatment group as a control. Each treatment had 5 repetitions. A Fluorcam chlorophyll fluorescence imaging system and photosynthesis analyzer (PP systems, targas-1, China) and chlorophyll meter (spad-502plus, China) were used to analyze the photosynthetic parameters of rice leaves on the 30th, 60th, 90th, and 120th days of rice growth. After 120 days, the rice was harvested and the fresh weight and dry weight were recorded.
2.3. Determination of soil properties
2.3.1 Soil pH, Ec, and Eh.
Water and soil were evenly mixed in the proportion of 2.5
:
1, and then shaken at 150 r min−1 and 25 °C for 24 h. After standing for a week, pH was obtained by measuring the supernatant with a pH meter (Mettler, Switzerland). The pH pretreatment method remained the same, with the exception that the water–soil ratio was 5
:
1 for the measurement of electrical conductivity (Ec) and redox potential (Eh) indicators. The Ec and Eh of the supernatant were measured with a multiparameter (Mettler, Switzerland).
2.3.2 Soil basic nutrients.
A 15 mg soil sample was weighed, and 3 mL HNO3 (GR, ≥99%), 3 mL primary water and 3 drops of HF (AR, ≥99%) were added into the digestion tube, which was then placed in a microwave digestion instrument (CEM, USA) for digestion at 1900 W and 200 °C. Finally, the digested sample was passed through a 0.22 μ sieve. The contents of iron and selenium in soil samples were determined with inductively coupled plasma mass spectrometry (ICP-MS, Thermo, USA). For quality control (QC) and quality assurance (QA), the standard reference material (GBW 07602, Bush twigs and leaves purchased from Nanjing Alida Biotechnology Co., LTD, China) was digested and measured following the same procedures. The recovery of iron and selenium elements was 91.6 and 90.8%, respectively. Soil organic carbon (TOC) was determined by a TOC analyzer (Elementar, Germany). Soil ammonium nitrogen (NH4–N) was determined by indophenol blue colorimetry.
2.4 Multivariate data analysis
Orthogonal partial least squares-discrimination analysis (OPLS-DA) is considered as a reliable tool to verify the differences between groups. In this study, the OPLS-DA was completed by using the Wekemo Bioincloud (https://www.bioincloud.tech). The variable importance of projection (VIP) is the vector to summarize the total importance of the variable in OPLS-DA. A variable is considered to be an important one if its VIP > 1.0 and its VIP value ranks within 20.
Random forest is an ensemble learning method that consists of multiple decision trees to make accurate and robust predictions. It demonstrates a notable advantage in handling small datasets. In this study, random forests were employed to identify the variables that contribute the highest accuracy to the classification of different groups (Se NPs, Ion, and CK). The contribution of variables to the overall classification accuracy was measured via the mean decrease in accuracy (MDA).28 The detailed calculation method can be found in previous studies.29,30
2.5 Artificial neural networks (ANNs) programming
ANNs are information processing systems that attempt to use computational models to mimic the human biological nervous system.31 The multilayer perceptron ANN was used in this study. The multilayer perceptron ANN consists of an input layer, one or more hidden layers, and an output layer. The number of hidden layer nodes (Zh) was calculated by using the following equation:32 |  | (1) |
where Zi and Zo are the studied conditions and performance measures numbers, respectively. The weights are values that convey the interaction between inputs with each other. At each layer, all nodes are interconnected and the transformation of data is performed by nonlinear techniques. To predict the rice yield (grain number) and quality (Se content in fruit) using ANN, two main different sets of variables (soil properties dataset and plant physiological data) were used as inputs of the network. The abbreviations of inputs and outputs are listed in Table S1.† For further model performance evaluation, the dataset was randomly split into approximately 50% for training, 20% for testing, and 30% for validation. The visualization of raw data distribution was performed using ‘tabplot’ package in R software (Fig. S1–S4†).
2.6 Model performance evaluation
To evaluate the performance and predictive capability of the ANN model, we considered the following statistical parameters, including coefficient of determination (R2), standard deviation (SD), mean squared error (MSE), and mean absolute error (MAE). The MSE measures the amount of error in models. The MAE of a model refers to the average of the absolute values of the errors of all records, which only indicates the average size of the errors independent of the direction.
They were calculated using the following formulas:
| 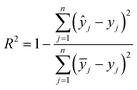 | (2) |
| 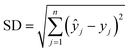 | (3) |
| 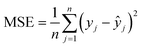 | (4) |
| 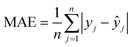 | (5) |
where
n is the number of data,
yj is the observed value of the
jth data,
ŷj is the predicted value of the
jth data, and
j is the average of the observed values.
3 Results and discussion
3.1 Effect of Se NPs on the soil–plant system
The plant physiological activities (PPAs) and soil properties (SPs) after soil applications of Se NPs are shown in Fig. S5 and S6.† The rice yield (i.e., GN_D120) and quality (Se_F) after exposing Se NPs are shown in Fig. S7.† The descriptive statistics of PPA and SP data are presented in Tables S2 and S3.† The distribution of data at different time stages (D30, D60, D90, and D120) is visualized in Fig. S1–S4.† Specifically, compared with CK, the Se NPs significantly increased GN_D120 and Se_F by 1.33- and 3.46-fold, respectively. Besides, compared with ionic Se, the Se NPs also exhibited certain improvements with 1.21- and 1.21-fold in GN_D120 and Se_F. Hence, the soil application of Se NPs significantly improved the yield and quality of the crop, which were consistent with previous findings.33,34 Hence, soil application of Se NPs can alter soil properties and enhance photosynthesis, ultimately leading to increased rice yield and improved quality, including higher Se content.
The OPLS-DA results (Fig. 1) help explore the differences and similarities among various treatments in the soil–plant system. The global OPLS-DA model showed a clear separation of all groups (Fig. 1a). The local OPLS-DA analysis showed a decrease in overlap over time and complete separation on day 120 (Fig. 1b–d). This suggests that the impact of Se NPs on PPAs and SPs is dependent on time. Several studies corroborate this conclusion. For instance, research has shown that Fe NPs enhance Fe availability in soils, leading to enhanced growth of sorghum and its photosynthesis.35 Se NPs have been found advantageous for various soil properties, such as providing a synergistic effect of soil mechanical processing, humic substances, and polymicrobial biofilms on soil fertility.36
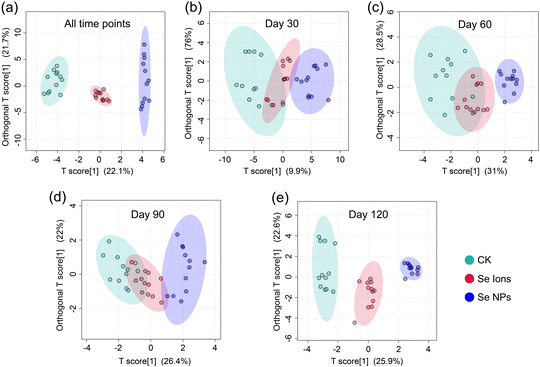 |
| Fig. 1 Score plots from orthogonal partial least-squares discrimination analysis (OPLS-DA) results of the samples. a) All samples were plotted from the global matrix. b) Day 30 samples were plotted. c) Day 60 samples were plotted. d) Day 90 samples were plotted. e) Day 120 were plotted. The X-axis represents the scores of the main components in the orthogonal signal correction (OSC) process, whereas the Y-axis represents the scores of the orthogonal components in the OSC process. | |
A random forest analysis was performed to determine the variables contributing the most to the variance in different treatment groups (Fig. S8†). At D30 and D60, the top four variables included three SPs and one PPA, with soil Se content (S_Se) being the most significant (Fig. S8a and b†). Interestingly, as the plants grew, PPA showed a prominent role in predicting the group (Fig. S8c and d†), indicating a shift from SPs to PPAs as the most significant variables over time. This shift may suggest changes in the impact of Se NPs on plant growth changes over time. These results agree with the findings of other studies, in which some studies have demonstrated that the capacity of NPs to improve vital soil properties, such as nutrient availability.37 As a result, the crop yield and nutrient value can be significantly increased.38
Network analysis was used to distinguish the importance of each variable within the group. To conduct a more comprehensive analysis, variables with moderate relationship (absolute value of Spearman's correlation >0.5, p < 0.05) were included in the network. As displayed in Fig. S9,† the absence of directional arrows suggests a potential correlation or interdependence among the variables, which requires further investigation to better understand their relationship.
3.2 Model-based feature analysis
The multilayer perceptron ANN was used to predict the growth and yield of rice induced by Se NPs. Pearson correlation analysis was used to investigate the interdependence of features (i.e., PPA and SP) and outcomes (i.e., rice yield and quality). The results of Pearson analysis (Table S4†) showed a significant correlation between most of the features. Fig. 2 exhibits the importance of the machine learning-based feature regarding rice yield and quality. The first four features during the initial phase of plant growth (D30) for rice yield and quality were related to both SPs and PPAs. As time went on, PPAs became progressively more important. At day 120, the top four features were only related to PPAs. These findings suggest that while PPAs ultimately (at D120) became more important than SPs, the dynamic processes of SPs still played an important role in determining rice yield and quality.
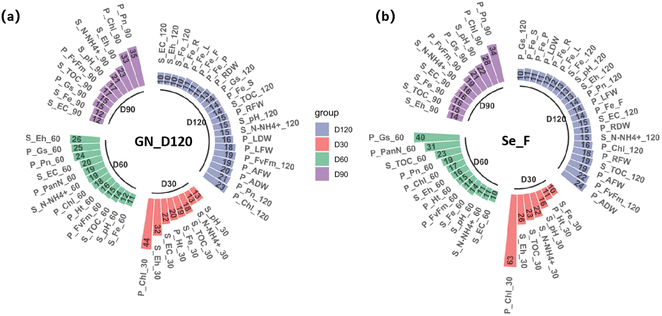 |
| Fig. 2 Machine learning-based feature importance from the ANN model in terms of the (a) GN_D120 prediction and (b) Se_F prediction. | |
3.3 Update ANN model results
The “Topliss and Costello rule” indicates that to minimize the risk of chance correlations, the ratio of training set to input features should be larger than 5.39 Hence, the best performing ANN model was reconceptualized with a reduced set of 4 to 6 input features to obtain better generalization and higher computational efficiency.40,41 The architecture of the ANN, which consists of an input layer, one or more hidden layers, and an output layer, is depicted in Fig. 3 and Text S1.†
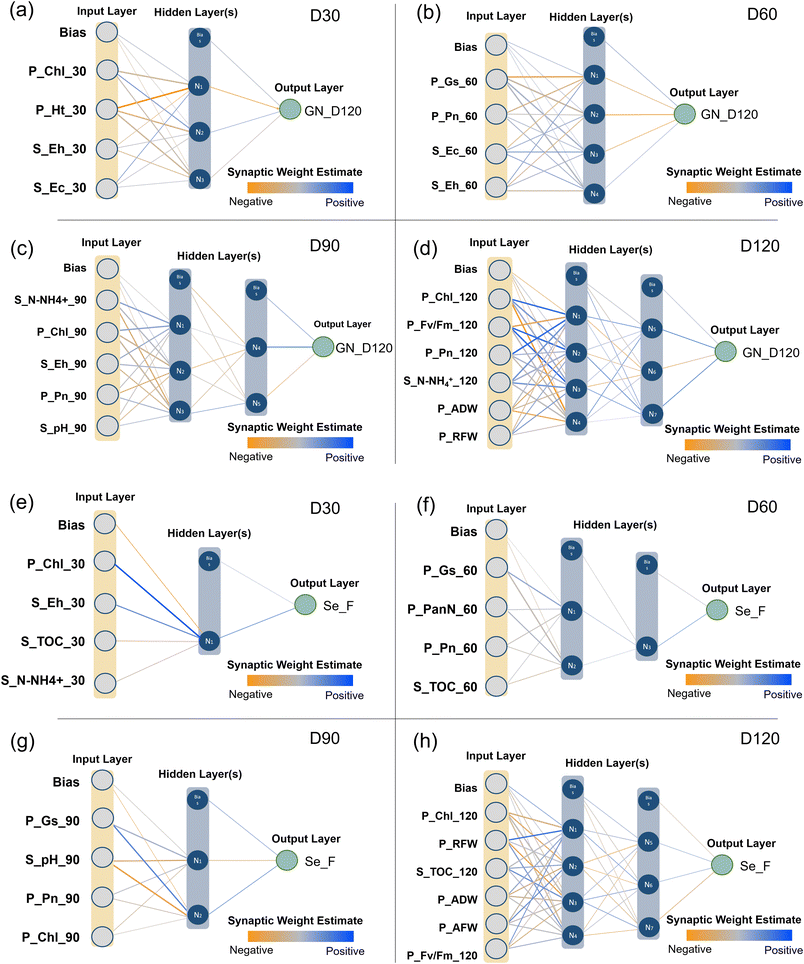 |
| Fig. 3 Architecture of the selected artificial neural network. (a)–(d) are used to predict the GN_D120. (e)–(h) are used to predict Se_F. | |
The actual and predicted values of GN_D120 and Se_F are shown in Fig. 4. The model demonstrated strong capability of the ANN in learning the relationship between input variables and output value (GN_D120 and Se_F). Based on the validation dataset, the R2 values of the ANN model for yield prediction at D30, D60, D90, and D120 were 0.785, 0.714, 0.830, and 0.893, respectively (Fig. 4a–d). For quality prediction (Se_F), the R2 values of ANN model at D30, D60, D90, and D120 were 0.729, 0.867, 0.760, and 0.762, respectively (Fig. 4e and f).
 |
| Fig. 4 ANN simulation correlation in the model training/testing/validation stage of (a)–(d) GN_D120 values and (e)–(h) Se_F values. | |
In addition, the other metrics (RMSE, MAE, SD) used to evaluate model performance are listed at Tables S5 and S6.† In Taylor diagram, a model with good performance should have a high correlation, similar variability (standard deviation marked as blue dashed line), and a low RMSE (solid gray line).42 As can be seen from the Taylor plot (Fig. 5), for both GN_D120 and Se_F prediction, the D120 model had a better performance, while the D60 model had a poorer performance. D30 and D90 models showed an intermediate performance.
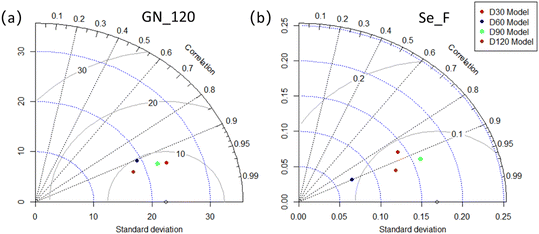 |
| Fig. 5 Taylor diagram presenting a comparison of the (a) GN_D120 and (b) Se_F observations with simulation from the ANN model. The black circular indicates the reference field while the color dot (red, blue, green, and brown) indicates the modeled field. The diagram shows the correlation and ratio of the standard derivation. | |
Taken together, these results suggest that ANN is an accurate modeling approach for predicting rice yield and quality. The results of the validation models show that the ANN algorithm can keep the errors within an acceptable range.43 Both the high R2 (>0.7) and the low RMSE (<11.27 for yield prediction and <0.11 for quality prediction) values of the validation models indicate that ANNs are accurate and reliable tools in practical predictions. The results of this study in terms of the accuracy and reliability of the ANN model are similar to the results of previous studies on related issues in this area, which reported low error values and high correlation coefficient.18,44
3.4 Feature exploration based on updated model results
The Sankey diagram (Fig. 6) illustrates the flow of relative importance in predicting rice yield and quality. Our findings suggest that, initially, SP indicators had a relatively higher important role in rice yield and quality. However, as the plant grew (from D60 to D90), the importance gradually shifted to PPA, indicating that the effects of Se NPs spread from the soil environment to the plant system.
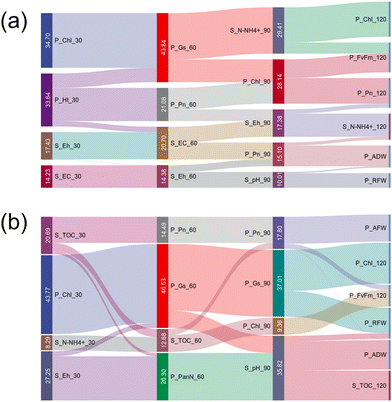 |
| Fig. 6 Sankey flow diagram shows the relative importance flow of (a) GN_D120 and (b) Se_F. The x-axis represents 4 time points (D30, D60, D90, and D120). The total height of the y-axis represents the full sample (100%). | |
Specifically, the ANN model (Fig. 6a) identified soil Ec, Eh, pH, and NH4+ contents as factors affecting rice yield. Similar but not identical, soil TOC, Eh, pH, and NH4+ were identified as responsible for rice quality (Fig. 6b). The Ec levels in soil are crucial for plants receiving the right amount of nutrients and water for optimal growth.45 In this study, the Ec value in the soil initially decreased and then increased throughout plant growth, following a parabolic trend (Fig. S2†). The decrease in the soluble salt content of the soil during rice growth could be attributed to the flooding period and nutrient uptake. The subsequent increase in Ec levels may be due to fertilizer application.46 Furthermore, at day 30 and day 60, the Ec values were identified as input variables of rice yield prediction, indicating a potential link between Ec and rice yield. As shown in Fig. S10,† compared with CK, the Ec level in the Ion group increased, which indicated that the rice might be in a stressed condition. One possible explanation for this phenomenon is that the NP can regulate the soil Ec value in a suitable range for rice growth.47 As noted by García-Gómez et al. (2015), the introduction of Se ions (SeO32−) may facilitate the incorporation of the dissolved salt, ultimately leading to an increase in Ec value.48 In contrast, Se NPs are inherently stable in soil and do not contribute to the increase of soluble Se.49
E
h, pH, and NH4+ were found to be critical for rice yield and quality (Fig. 6). Soil Eh helps provide a unique environment for supporting microbial processes, making it crucial for managing water and soil fertility.50,51 Compared with CK, NP amendments increased the soil Eh value during the flooded period but decreased it during the mature stage (Fig. S11†). These fluctuations in soil Eh resulting from NP treatment can potentially impact the biomass and activities of the rhizosphere microbiome,52 thus influencing nutrient availability and rice growth.53 Soil pH is another fundamental factor affecting microbial and enzyme activity.54 Soil Eh and pH are logically inversely correlated, as oxidation usually leads to acidification.53 Our results of Eh and pH align with previous studies, which showed that a decrease in pH is typically accompanied by an increase in soil Eh.55 The change in soil pH could alter the behavior and fate of NPs in the soil, further impacting their uptake by plants.56 For instance, Eh and pH can impact various elements, including nitrogen assimilation by plants.57 In this study, the pH decreased with plant growth (Fig. S2†). One possible reason is that from the tillering stage (D30) to the maturity stage (D120), rice roots grow vigorously and secrete large amounts of acidic substances, leading to a decreasing trend of soil pH.58 NH4+ is a critical nitrogen source for plant growth, promoting the growth and survival of beneficial soil microorganisms.59 The ANN model results show that ammonium nitrogen influenced rice yield and quality (Fig. 6). During plant growth, the NH4+ content of the soil gradually increased (Fig. S2†). This phenomenon may be attributed to the mineralization process, in which inorganic nitrogen is broken down into NH4+ over time.60 TOC serves as an important indicator of soil health, affecting crop production and the regulation of soil ecosystem services.61 In this study, TOC is particularly important for Se uptake in rice (Fig. 6). According to the control group, the soil TOC was increased from the tillering stage (D30) to the heading stage (D60) (Fig. S2†). This could be attributed to the vigorous growth of rice roots during this time, resulting in the secretion of substantial amounts of root secretions. These secretions enhanced the interaction of organic matter and minerals, leading to an increase in TOC.62,63 However, compared with CK, Se NPs led to a decrease in the TOC content in soil (Fig. S12†). This could be explained by the fact that NPs improved the carbon use efficiency of rice.36,64
Among the PPA index, the photosynthesis-related parameters are more important for predicting the yield and quality of rice, such as Chl, Pn, Gs, and Fv/Fm (Fig. 6). Photosynthesis is a crucial process for plants and strongly affects plant productivity and yield.65 Herein, Se NPs significantly increased Chl, Pn, Gs, Fv/Fm content compared with CK or Ion treatment (Fig. S13–S16†). This is consistent with our previous results that NPs can improve crop yield and quality by enhancing photosynthesis.66,67 Specifically, Chl plays a pivotal role in light absorption,68 and its increase in content suggests that Se NPs could improve light absorption and energy conversion efficiency in photosynthesis. Pn is crucial for plant maintenance as it measures the rate of CO2 conversion into organic matter.69 Gs regulates the exchange of CO2 and water vapor between the leaf and atmosphere, influencing photosynthesis.70 The significant increase in Pn and Gs content indicates that Se NPs may enhance the rate of carbon dioxide conversion and facilitate CO2 diffusion into plant cells. Fv/Fm is an indicator of energy conversion efficiency in photosynthesis, which is closely linked to plant growth and productivity.71 The increase in the Fv/Fm content implies that Se NPs may enhance energy transfer efficiency in photosynthesis, leading to improved plant growth and productivity.
Overall, the Sankey diagram revealed that the initial important reaction under Se NP conditions was SP rather than PPA. However, as time progressed (from D60 to D90), the relative importance of SP gradually shifted to PPA, indicating that the effects of Se NPs spread from the soil environment to the plant system. This shift could be due to Se NPs initially impacting the soil environment and indirectly affecting the plant system through changes in soil nutrient availability, microbial activity, and other soil parameters.72 This process may take time to play out, resulting in a higher relative importance of SP at the D30 stage. As Se NPs entered the plant, the relative importance of PPA gradually increased, influencing parameters related to physiological metabolism and photosynthesis. It is noteworthy that although PPA at D120 showed significant contribution in predicting groups, the valuable role played by SP during the early stages of plant growth (D30) should not be disregarded.
3.5 Potential and the role of developing explainable models in the NPs–plant–soil system
As the interest in nano-enabled agriculture rapidly increases, it is vital to carefully evaluate the risks of using NPs for agriculture.73 Therefore, a thorough understanding of the interactions of NPs in soil–plant systems is becoming increasingly crucial, as it provides the basis for the safe use of NPs. Employing explainable models is a feasible solution since they are powerful tools that provide hypotheses from a complex feature space and feature interactions for experimental scientists. For instance, our study indicates that the effects of Se NPs on rice are time-dependent, with SP playing a primary role and PPA becoming increasingly important over time. As plant growth progresses, the importance of Se NPs shifts from the underground to aboveground parts of the plant, ultimately affecting both yield and quality. The pathways through which NPs stimulate crop yield and quality are differentiated, with TOC being crucial for crop yield and soil Ec being crucial for quality. These findings offer valuable insights into the intricate interactions between nanomaterials, plants, and soil, highlighting the significance of the dynamic changes in soluble salt content within the soil and developing safe design strategies for nanomaterials. However, to further our understanding of the effects of PPA and SP values on plant growth, it is necessary to expand the experimental framework of this study. For instance, it is imperative to investigate the sensitivity of different plant species to ecotoxicological stress in relation to the SP values of the respective soils. Moreover, factors influencing the temporal dynamics of SP values (such as natural weather oscillations and seasonal changes in soil chemistry) ought to be considered in the experimental setup. Additionally, the chemical properties of NPs play a significant role in their behavior within the soil–crop system. Factors such as shape, size, charge, and surface coating have a direct impact on plant uptake and translocation.74,75 The dissolution of NPs is also critical, as it affects material stability, availability, and safety.76 While foliar application has been suggested as an effective and environmentally friendly method,77 it is worth noting that both foliar application and soil fertilization have their own advantages depending on the situation.78 Therefore, the NPs properties, plant properties, and the experiment conditions play a role in determining the interaction between NPs and plants. Hence, machine learning techniques like ANN can be utilized to investigate potential correlations among these variables and identify optimal application methods to save time and effort.79 By enhancing our understanding of plant–soil interactions, we can develop more informed management strategies for sustainable and productivity agriculture and ecosystems. Moreover, with the practice of data-sharing, more data will become available in the future, enabling improved model performance and better detection of data patterns in complex systems.
4 Conclusion
This study demonstrated that Se NPs have a positive impact on the yield and quality of rice by regulating SPs and PPAs. An ANN model was developed to reveal that SPs played a primary role, followed by PPAs. Moreover, it was found that the pathways for yield and quality were differentiated, with D60 and D90 being identified as the equilibrium points for predicting rice yield and quality, respectively. The key SP values were identified as TOC, NH4+, pH, Eh, and Ec; while the key PPA values were identified as Chl, Pn, Gs, and Fv/Fm. This study provides a new approach and perspective for predicting the bioavailability of Se NPs in soil–crop systems, which could improve the ability to assess the contribution of nano-enable agriculture to food security. A comprehensive understanding of NPs application in soil–plant systems will facilitate safe design solutions for nanoagriculture. Future research should expand beyond laboratory conditions to field studies. As more data becomes available, the performance of the training algorithm will improve, and ANNs will be better equipped to identify data patterns from more complex systems.
Model availability
The PMML type file used to perform our calculations are available at https://github.com/Jingz11/ANN_Se-NPs_Rice.
Author contributions
Jing Li: investigation, writing – original draft, data analysis. Le Yue: investigation, data analysis. Feiran Chen: investigation, data analysis. Xuesong Cao: investigation, data analysis. Bingxu Cheng: investigation, data analysis. Chuanxi Wang: writing – review & editing, data analysis, formal analysis. Zhenyu Wang: experimental design, data analysis, writing – review & editing. Baoshan Xing: writing – review & editing, formal analysis.
Conflicts of interest
The authors declare that they have no known competing financial interests or personal relationships that could have appeared to influence the work reported in this paper.
Acknowledgements
This research was supported by the National Natural Science Foundation of China (42077296, 42322705, and 42192572), the Fundamental Research Funds for the Central Universities (JUSRP622019 and JUSRP221023), the Natural Science Foundation of Jiangsu Province (BK20230044), Postgraduate Research & Practice Innovation Program of Jiangsu Province (KYCX22_2381), and USDA NIFA Hatch program (MAS 00549).
References
- G. V. Lowry, A. Avellan and L. M. Gilbertson, Opportunities and challenges for nanotechnology in the agri-tech revolution, Nat. Nanotechnol., 2019, 14, 517–522 CrossRef CAS PubMed.
- M. A. Farooq, F. Hannan, F. Islam, A. Ayyaz, N. Zhang, W. Chen, K. Zhang, Q. Huang, L. Xu and W. Zhou, The potential of nanomaterials for sustainable modern agriculture: present findings and future perspectives, Environ. Sci.: Nano, 2022, 9, 1926–1951 RSC.
- D. Singh and B. R. Gurjar, Nanotechnology for agricultural applications: Facts, issues, knowledge gaps, and challenges in environmental risk assessment, J. Environ. Manage., 2022, 322, 116033 CrossRef CAS.
- X. Jing, Y. Liu, X. Liu, X.-F. Wang, C. You, D. Chang and S. Zhang, Nitrogen-doped carbon dots enhanced seedling growth and salt tolerance with distinct requirements of excitation light, RSC Adv., 2023, 13, 12114–12122 RSC.
- S. Kumar, M. Nehra, N. Dilbaghi, G. Marrazza, S. K. Tuteja and K. H. Kim, Nanovehicles for Plant Modifications towards Pest- and Disease-Resistance Traits, Trends Plant Sci., 2020, 25, 198–212 CrossRef CAS.
- M. Nehra, N. Dilbaghi, G. Marrazza, A. Kaushik, C. Sonne, K. H. Kim and S. Kumar, Emerging nanobiotechnology in agriculture for the management of pesticide residues, J. Hazard. Mater., 2020, 401, 123369 CrossRef PubMed.
- E. Yusefi-Tanha, S. Fallah, A. Rostamnejadi and L. R. Pokhrel, Zinc oxide nanoparticles (ZnONPs) as a novel nanofertilizer: Influence on seed yield and antioxidant defense system in soil grown soybean (Glycine max cv. Kowsar), Sci. Total Environ., 2020, 738, 140240 CrossRef CAS.
- S. Ullah, M. Adeel, M. Zain, M. Rizwan, M. K. Irshad, G. Jilani, A. Hameed, A. Khan, M. Arshad, A. Raza, M. A. Baluch and Y. Rui, Physiological and biochemical response of wheat (Triticum aestivum) to TiO2 nanoparticles in phosphorous amended soil: A full life cycle study, J. Environ. Manage., 2020, 263, 110365 CrossRef CAS.
- D. M. Mahapatra, K. C. Satapathy and B. Panda, Biofertilizers and nanofertilizers for sustainable agriculture: Phycoprospects and challenges, Sci. Total Environ., 2022, 803, 149990 CrossRef CAS PubMed.
- M. Kalwani, H. Chakdar, A. Srivastava, S. Pabbi and P. Shukla, Effects of nanofertilizers on soil and plant-associated microbial communities: Emerging trends and perspectives, Chemosphere, 2022, 287, 132107 CrossRef CAS.
- M. Kah and R. Kookana, Emerging investigator series: nanotechnology to develop novel agrochemicals: critical issues to consider in the global agricultural context, Environ. Sci.: Nano, 2020, 7, 1867–1873 RSC.
- G. Srivastava, C. K. Das, A. Das, S. K. Singh, M. Roy, H. Kim, N. Sethy, A. Kumar, R. K. Sharma, S. K. Singh, D. Philip and M. Das, Seed treatment with iron pyrite (FeS2) nanoparticles increases the production of spinach, RSC Adv., 2014, 4, 58495–58504 RSC.
- N. Fan, C. Zhao, Z. Chang, L. Yue, F. He, Z. Xiao and Z. Wang, Silicon quantum dots promote radish resistance to root herbivores without impairing rhizosphere microenvironment health, Environ. Sci.: Nano, 2023, 10, 2232–2244 RSC.
- R. Prăvălie, M. Niculiţă, B. Roşca, G. Marin, M. Dumitraşcu, C. Patriche, M.-V. Birsan, I.-A. Nita, A. Tişcovschi, I. Sîrodoev and G. Bandoc, Machine learning-based prediction and assessment of recent dynamics of forest net primary productivity in Romania, J. Environ. Manage., 2023, 334, 117513 CrossRef PubMed.
- K. N. Palansooriya, J. Li, P. D. Dissanayake, M. Suvarna, L. Li, X. Yuan, B. Sarkar, D. C. W. Tsang, J. Rinklebe, X. Wang and Y. S. Ok, Prediction of Soil Heavy Metal Immobilization by Biochar Using Machine Learning, Environ. Sci. Technol., 2022, 56, 4187–4198 CrossRef.
- X. Wang, D. Yu, L. Ma, X. Lu, J. Song and M. Lei, Using big data searching and machine learning to predict human health risk probability from pesticide site soils in China, J. Environ. Manage., 2022, 320, 115798 CrossRef.
- G. P. Gakis, I. G. Aviziotis and C. A. Charitidis, A structure–activity approach towards the toxicity assessment of multicomponent metal oxide nanomaterials, Nanoscale, 2023, 15, 16432–16446 RSC.
- L. Rossi, M. Bagheri, W. Zhang, Z. Chen, J. G. Burken and X. Ma, Using artificial neural network to investigate physiological changes and cerium oxide nanoparticles and cadmium uptake by Brassica napus plants, Environ. Pollut., 2019, 246, 381–389 CrossRef CAS.
-
N. Gandhi, O. Petkar and L. J. Armstrong, Rice crop yield prediction using artificial neural networks, 2016 IEEE Technological Innovations in ICT for Agriculture and Rural Development (TIAR), 2016, pp. 105–110 Search PubMed.
- P. A. Gunawan, E. N. Kencana and K. Sari, Classification of rice leaf diseases using artificial neural network, J. Phys.: Conf. Ser., 2021, 1722, 012013 CrossRef.
- L. Kang, Y. Wu, J. Zhang, Q. An, C. Zhou, D. Li and C. Pan, Nano-selenium enhances the antioxidant capacity, organic acids and cucurbitacin B in melon (Cucumis melo L.) plants, Ecotoxicol. Environ. Saf., 2022, 241, 113777 CrossRef CAS.
- S. Huang, K. Yu, Q. Xiao, B. Song, W. Yuan, X. Long, D. Cai, X. Xiong and W. Zheng, Effect of bio-nano-selenium on yield, nutritional quality and selenium content of radish, J. Food Compos. Anal., 2023, 115, 104927 CrossRef CAS.
- D. Li, C. Zhou, J. Zhang, Q. An, Y. Wu, J.-Q. Li and C. Pan, Nanoselenium Foliar Applications Enhance the Nutrient Quality of Pepper by Activating the Capsaicinoid Synthetic Pathway, J. Agric. Food Chem., 2020, 68, 9888–9895 CrossRef CAS PubMed.
- B. Cheng, C. Wang, F. Chen, L. Yue, X. Cao, X. Liu, Y. Yao, Z. Wang and B. Xing, Multiomics understanding of improved quality in cherry radish (Raphanus sativus L. var. radculus pers) after foliar application of selenium nanomaterials, Sci. Total Environ., 2022, 824, 153712 CrossRef CAS.
- E. Domokos-Szabolcsy, L. Marton, A. Sztrik, B. Babka, J. Prokisch and M. Fari, Accumulation of red elemental selenium nanoparticles and their biological effects in Nicotiniatabacum, Plant Growth Regul., 2012, 68, 525–531 CrossRef CAS.
- R. X. Wang, S. Y. Zhang and M. Z. Liu, Synthesis of nanometer Se by solid state reaction at ambient temperature, Anhui Shifan Daxue Xuebao, Ziran Kexueban, 2004, 3, 302–305 Search PubMed.
- B. Cheng, J. Zhang, C. Wang, J. Li, F. Chen, X. Cao, L. Yue and Z. Wang, Selenium nanomaterials alleviate Brassica chinensis L cadmium stress: Reducing accumulation, regulating microorganisms and activating glutathione metabolism, Chemosphere, 2023, 344, 140320 CrossRef CAS.
- L. B. Williams, S. E. Haydel and R. E. Ferrell, Jr., Bentonite, Bandaids, and Borborygmi, Elements, 2009, 5, 99–104 CrossRef CAS.
-
H. Hong, G. Xiaoling and Y. Hua, presented in part at the 2016 7th IEEE International Conference on Software Engineering and Service Science (ICSESS), 2016 Search PubMed.
- C. Strobl, A.-L. Boulesteix, T. Kneib, T. Augustin and A. Zeileis, Conditional variable importance
for random forests, BMC Bioinf., 2008, 9, 307 CrossRef.
-
S. Shanmuganathan, in Artificial Neural Network Modelling, ed. S. Shanmuganathan and S. Samarasinghe, Springer International Publishing, Cham, 2016, pp. 1–14, DOI:10.1007/978-3-319-28495-8_1.
- D. E. Fletcher, Nest Association of Dusky Shiners (Notropis cummingsae) and Redbreast Sunfish (Lepomis auritus), a Potentially Parasitic Relationship, Copeia, 1993, 1993, 159–167 CrossRef.
- C. Wang, X. Liu, F. Chen, L. Yue, X. Cao, J. Li, B. Cheng, Z. Wang and B. Xing, Selenium content and nutritional quality of Brassica chinensis L enhanced by selenium engineered nanomaterials: The role of surface charge, Environ. Pollut., 2022, 308, 119582 CrossRef CAS PubMed.
- C. Wang, L. Yue, B. Cheng, F. Chen, X. Zhao, Z. Wang and B. Xing, Mechanisms of growth-promotion and Se-enrichment in Brassica chinensis L. by selenium nanomaterials: beneficial rhizosphere microorganisms, nutrient availability, and photosynthesis, Environ. Sci.: Nano, 2022, 9, 302–312 RSC.
- A. Soliemanzadeh and M. Fekri, Effects of green iron nanoparticles on iron changes and phytoavailability in a calcareous soil, Pedosphere, 2021, 31, 761–770 CrossRef CAS.
- S. V. Gudkov, G. A. Shafeev, A. P. Glinushkin, A. V. Shkirin, E. V. Barmina, I. I. Rakov, A. V. Simakin, A. V. Kislov, M. E. Astashev, V. A. Vodeneev and V. P. Kalinitchenko, Production and Use of Selenium Nanoparticles as Fertilizers, ACS Omega, 2020, 5, 17767–17774 CrossRef CAS PubMed.
- M. Kah, R. S. Kookana, A. Gogos and T. D. Bucheli, A critical evaluation of nanopesticides and nanofertilizers against their conventional analogues, Nat. Nanotechnol., 2018, 13, 677–684 CrossRef CAS PubMed.
- Y. S. Perea Vélez, R. Carrillo-González and M. d. C. A. González-Chávez, Interaction of metal nanoparticles–plants–microorganisms in agriculture and soil remediation, J. Nanopart. Res., 2021, 23, 206 CrossRef.
- J. G. Topliss, Utilization of operational schemes for analog synthesis in drug design, J. Med. Chem., 1972, 15, 1006–1011 CrossRef CAS.
- J. G. Topliss, Utilization of operational schemes for analog synthesis in drug design, J. Med. Chem., 1972, 15, 1006–1011 CrossRef CAS.
- A. Cherkasov, E. N. Muratov, D. Fourches, A. Varnek, M. Baskin, II, J. Cronin, P. Dearden, Y. C. Gramatica, R. Martin, V. Todeschini, V. E. Consonni, R. Kuz'min, R. Cramer, C. Benigni, J. Yang, L. Rathman, J. Terfloth, A. Richard Gasteiger and A. Tropsha, QSAR modeling: where have you been? Where are you going to?, J. Med. Chem., 2014, 57, 4977–5010 CrossRef CAS PubMed.
- K. E. Taylor, Summarizing multiple aspects of model performance in a single diagram, J. Geophys. Res.: Atmos., 2001, 106, 7183–7192 CrossRef.
- A. Chlingaryan, S. Sukkarieh and B. Whelan, Machine learning approaches for crop yield prediction and nitrogen status estimation in precision agriculture: A review, Comput. Electron. Agric., 2018, 151, 61–69 CrossRef.
- M. Liu, X. Liu, M. Li, M. Fang and W. Chi, Neural-network model for estimating leaf chlorophyll concentration in rice under stress from heavy metals using four spectral indices, Biosyst. Eng., 2010, 106, 223–233 CrossRef.
- A. Bhatt and A. Santo, Germination and recovery of heteromorphic seeds of Atriplex canescens (Amaranthaceae) under increasing salinity, Plant Ecol., 2016, 217, 1069–1079 CrossRef.
- L. Qing, D. Qigen, H. Jian, W. Hongjun and C. Jingdu, Profiles of tetracycline resistance genes in paddy soils with three different organic fertilizer applications, Environ. Pollut., 2022, 306, 119368 CrossRef CAS PubMed.
- M. Gil-Díaz, L. T. Ortiz, G. Costa, J. Alonso, M. L. Rodríguez-Membibre, S. Sánchez-Fortún, A. Pérez-Sanz, M. Martín and M. C. Lobo, Immobilization and Leaching of Pb and Zn in an Acidic Soil Treated with Zerovalent Iron Nanoparticles (nZVI): Physicochemical and Toxicological Analysis of Leachates, Water, Air, Soil Pollut., 2014, 225, 1990 CrossRef.
- C. García-Gómez, M. Babin, A. Obrador, J. M. Álvarez and M. D. Fernández, Integrating ecotoxicity and chemical approaches to compare the effects of ZnO nanoparticles, ZnO bulk, and ZnCl2 on plants and microorganisms in a natural soil, Environ. Sci. Pollut. Res., 2015, 22, 16803–16813 CrossRef.
- J. Liu, W.-Y. Qi, H. Chen, C. Song, Q. Li and S.-G. Wang, Selenium Nanoparticles as an Innovative Selenium Fertilizer Exert Less Disturbance to Soil Microorganisms, Front. Microbiol., 2021, 12, 746046 CrossRef PubMed.
-
I. L. Pepper and T. J. Gentry, in Environmental Microbiology (Third Edition), ed. I. L. Pepper, C. P. Gerba and T. J. Gentry, Academic Press, San Diego, 2015, pp. 59–88, DOI:10.1016/B978-0-12-394626-3.00004-1.
- J. Ren, H. Huang, Z. Zhang, X. Xu, L. Zhao, H. Qiu and X. Cao, Enhanced microbial reduction of Cr(VI) in soil with biochar acting as an electron shuttle: Crucial role of redox-active moieties, Chemosphere, 2023, 328, 138601 CrossRef CAS.
- S. Frenk, T. Ben-Moshe, I. Dror, B. Berkowitz and D. Minz, Effect of metal oxide nanoparticles on microbial community structure and function in two different soil types, PLoS One, 2013, 8, e84441 CrossRef PubMed.
- O. Husson, A. Brunet, D. Babre, H. Charpentier, M. Durand and J.-P. Sarthou, Conservation Agriculture systems alter the electrical characteristics (Eh, pH and EC) of four soil types in France, Soil Tillage Res., 2018, 176, 57–68 CrossRef.
- S. Ding, B. Wang, Y. Feng, H. Fu, Y. Feng, H. Xie and L. Xue, Livestock manure-derived hydrochar improved rice paddy soil nutrients as a cleaner soil conditioner in contrast to raw material, J. Cleaner Prod., 2022, 372, 133798 CrossRef CAS.
- Y.-S. Han, J.-H. Park, S.-J. Kim, H. Y. Jeong and J. S. Ahn, Redox transformation of soil minerals and arsenic in arsenic-contaminated soil under cycling redox conditions, J. Hazard. Mater., 2019, 378, 120745 CrossRef CAS.
- M. Bakshi and A. Kumar, Copper-based nanoparticles in the soil-plant environment: Assessing their applications, interactions, fate and toxicity, Chemosphere, 2021, 281, 130940 CrossRef CAS PubMed.
- O. Husson, Redox potential (Eh) and pH as drivers of soil/plant/microorganism systems: a transdisciplinary overview pointing to integrative opportunities for agronomy, Plant Soil, 2013, 362, 389–417 CrossRef CAS.
- Q. Zhang, P. Yuan, W. Liang, Z. Qiao, X. Shao, W. Zhang and C. Peng, Exogenous iron alters uptake and translocation of CuO nanoparticles in soil-rice system: A life cycle study, Environ. Int., 2022, 168, 107479 CrossRef CAS.
- Q. Hou, Y. Ni, S. Huang, T. Zuo, J. Wang and W. Ni, Effects of manure substitution for chemical fertilizers on rice yield and soil labile nitrogen in paddy fields of China: A meta-analysis, Pedosphere, 2022, 33(1), 172–184 CrossRef.
- T. T. N. Nguyen, C.-Y. Xu, I. Tahmasbian, R. Che, Z. Xu, X. Zhou, H. M. Wallace and S. H. Bai, Effects of biochar on soil available inorganic nitrogen: A review and meta-analysis, Geoderma, 2017, 288, 79–96 CrossRef CAS.
- R. Begum, M. M. R. Jahangir, M. Jahiruddin, M. R. Islam, S. M. Bokhtiar and K. R. Islam, Reduced tillage with residue retention improves soil labile carbon pools and carbon lability and management indices in a seven-year trial with wheat-mung bean-rice rotation, Pedosphere, 2022, 32, 916–927 CrossRef.
- T. Wen, G.-H. Yu, W.-D. Hong, J. Yuan, G.-Q. Niu, P.-H. Xie, F.-S. Sun, L.-D. Guo, Y. Kuzyakov and Q.-R. Shen, Root exudate chemistry affects soil carbon mobilization via microbial community reassembly, Fundam. Res, 2022, 2, 697–707 CrossRef CAS.
- M. Keiluweit, J. J. Bougoure, P. S. Nico, J. Pett-Ridge, P. K. Weber and M. Kleber, Mineral protection of soil carbon counteracted by root exudates, Nat. Clim. Change, 2015, 5, 588–595 CrossRef CAS.
- G. M. Shah, H. Ali, I. Ahmad, M. Kamran, M. Hammad, G. A. Shah, H. F. Bakhat, A. Waqar, J. Guo, R. Dong and M. I. Rashid, Nano agrochemical zinc oxide influences microbial activity, carbon, and nitrogen cycling of applied manures in the soil-plant system, Environ. Pollut., 2022, 293, 118559 CrossRef CAS PubMed.
- A. Makino, Y. Suzuki and K. Ishiyama, Enhancing photosynthesis and yield in rice with improved N use efficiency, Plant Sci., 2022, 325, 111475 CrossRef CAS.
- Y. Ji, L. Yue, X. Cao, F. Chen, J. Li, J. Zhang, C. Wang, Z. Wang and B. Xing, Carbon dots promoted soybean photosynthesis and amino acid biosynthesis under drought stress: Reactive oxygen species scavenging and nitrogen metabolism, Sci. Total Environ., 2022, 856, 159125 CrossRef PubMed.
- C. Wang, X. Liu, J. Li, L. Yue, H. Yang, H. Zou, Z. Wang and B. Xing, Copper nanoclusters promote tomato (Solanum lycopersicum L.) yield and quality through improving photosynthesis and roots growth, Environ. Pollut., 2021, 289, 117912 CrossRef CAS.
- A. J. Simkin, L. Kapoor, C. G. P. Doss, T. A. Hofmann, T. Lawson and S. Ramamoorthy, The role of photosynthesis related pigments in light harvesting, photoprotection and enhancement of photosynthetic yield in planta, Photosynth. Res., 2022, 152, 23–42 CrossRef CAS PubMed.
- A. Stirbet, D. Lazár, Y. Guo and G. Govindjee, Photosynthesis: basics, history and modelling, Ann. Bot., 2020, 126, 511–537 CrossRef CAS PubMed.
- W. Yamori, K. Kusumi, K. Iba and I. Terashima, Increased stomatal conductance induces rapid changes to photosynthetic rate in response to naturally fluctuating light conditions in rice, Plant, Cell Environ., 2020, 43, 1230–1240 CrossRef CAS.
- J. Flexas and M. Carriquí, Photosynthesis and photosynthetic efficiencies along the terrestrial plant's phylogeny: lessons for improving crop photosynthesis, Plant J., 2020, 101, 964–978 CrossRef CAS PubMed.
-
B. Hussain, L. Riaz, K. Javeed, M. J. Umer, Y. Abbas, S. ur-Rahman, S. W. Khan, H. Ali, Q. Abbas, M. Zafar and M. N. Ashraf, in Sustainable Plant Nutrition, ed. T. Aftab and K. R. Hakeem, Academic Press, 2023, pp. 153–178, DOI:10.1016/B978-0-443-18675-2.00008-0.
- D. Wang, N. B. Saleh, A. Byro, R. Zepp, E. Sahle-Demessie, T. P. Luxton, K. T. Ho, R. M. Burgess, M. Flury, J. C. White and C. Su, Nano-enabled pesticides for sustainable agriculture and global food security, Nat. Nanotechnol., 2022, 17, 347–360 CrossRef CAS PubMed.
- R. P. Mendoza and J. M. Brown, Engineered nanomaterials and oxidative stress: Current understanding and future challenges, Curr. Opin. Toxicol., 2019, 13, 74–80 CrossRef.
- M. Kusiak, P. Oleszczuk and I. Jośko, Cross-examination of engineered nanomaterials in crop production: Application and related implications, J. Hazard. Mater., 2022, 424, 127374 CrossRef CAS PubMed.
- E. J. Petersen, M. Mortimer, R. M. Burgess, R. Handy, S. Hanna, K. T. Ho, M. Johnson, S. Loureiro, H. Selck, J. J. Scott-Fordsmand, D. Spurgeon, J. Unrine, N. W. van den Brink, Y. Wang, J. White and P. Holden, Strategies for robust and accurate experimental approaches to quantify nanomaterial bioaccumulation across a broad range of organisms, Environ. Sci.: Nano, 2019, 6, 1619–1656 RSC.
- J. Hong, C. Wang, D. C. Wagner, J. L. Gardea-Torresdey, F. He and C. M. Rico, Foliar application of nanoparticles: mechanisms of absorption, transfer, and multiple impacts, Environ. Sci.: Nano, 2021, 8, 1196–1210 RSC.
- J. Li, L. Yue, Q. Zhao, X. Cao, W. Tang, F. Chen, C. Wang and Z. Wang, Prediction models on biomass and yield of rice affected by metal (oxide) nanoparticles using nano-specific descriptors, NanoImpact, 2022, 28, 100429 CrossRef CAS.
- P. Zhang, Z. Guo, S. Ullah, G. Melagraki, A. Afantitis and I. Lynch, Nanotechnology and artificial intelligence to enable sustainable and precision agriculture, Nat. Plants, 2021, 7, 864–876 CrossRef.
|
This journal is © The Royal Society of Chemistry 2024 |