An improved framework for efficiently modeling organic aerosol (OA) considering primary OA evaporation and secondary OA formation from VOCs, IVOCs, and SVOCs†
Received
15th May 2024
, Accepted 11th August 2024
First published on 13th August 2024
Abstract
Organic aerosols (OA) constitute an important fraction of fine particulate matter (PM2.5) air pollution, yet accurate and efficient OA modeling within chemical transport models (CTM) remains a challenge. Volatility basis set (VBS) schemes for OA have demonstrated improved performance in simulating OA, particularly for primary organic aerosol (POA), but their computational complexity impedes application to advanced modeling tasks, such as detailed source apportionment. Conversely, simpler “two-product” schemes are efficient and compatible with source apportionment techniques but many of them tend to overestimate POA by treating it as non-volatile. Either VBS or 2-product schemes can perform well for secondary organic aerosol (SOA) depending upon the data and assumptions used to model SOA formation from precursors. In this study, we update the Comprehensive Air Quality Model with extensions (CAMx) “SOAP” 2-product modeling framework by (1) treating POA as semivolatile using an efficient scheme, (2) adding SOA formation from semivolatile organic compounds (SVOCs), and (3) adopting SOA yields derived from the widely-used Community Multiscale Air Quality (CMAQ) AERO7 scheme. The first update allows temperature-dependent partial evaporation of POA to SVOC, which is subsequently oxidized in the gas phase. For the latter two updates, SOA yields are updated to emulate the AERO7 scheme based on an offline conceptual model. We implemented these changes within the existing SOAP2 scheme of CAMx to create a new scheme called “SOAP3”. A series of CTM simulations were conducted with the SOAP3 scheme to simulate OA and its components in China during July and November 2018. Results were validated against surface observations and compared to the SOAP2 and AERO7 schemes. Compared to SOAP2, SOAP3 substantially reduced POA proportions (by 10–24%) and increased SOA concentrations (by 45–193%) for selected regions. SOAP3 performs more like the AERO7 scheme than SOAP2 in terms of the simulated OA components and improved accuracy compared to observations. Uncertainties and limitations of the current SOAP3 scheme are also discussed. Our study demonstrates a feasible and readily implemented methodology for improving two-product OA modeling, which is currently employed in many CTMs.
Environmental significance
Organic aerosol (OA) is a major contributor to ambient PM2.5 and has different chemical components originating from diverse sources. Models are an essential tool for understanding OA sources, but model accuracy is important, and detailed model source attribution is highly desirable. Our new OA modeling approach can better predict the impacts of human activities and natural emissions on air quality and climate. This improved understanding can inform policy decisions aimed at reducing PM2.5 levels, protecting human health, and mitigating climate change.
|
1. Introduction
Human exposure to fine particulate matter (PM2.5) is associated with adverse health outcomes and is reported to cause 4.14 million deaths worldwide annually (95% confidence interval: 3.45 to 4.80).1 Consequently, PM2.5 is a focus of air quality management efforts over the past decades worldwide. Organic aerosol (OA) is an important contributor to PM2.5, with relative mass contribution ranging from 20–90% across different regions.2 OA sources can be complex to characterize because the primary and secondary contributions are not necessarily distinct.3,4 The relative contributions of primary OA (POA) and secondary (SOA) vary spatially and temporally. SOA is formed in the atmosphere when gas-phase reactive organic compounds (ROC) are oxidized and form products that can migrate to the particle-phase either by condensation5 or via heterogeneous reaction.6 When atmospheric conditions favor secondary pollution events, SOA can account for the majority (60–90%) of OA.7–10 Traditionally, POA emissions were considered non-volatile, i.e., existing permanently in the particle phase.11,12 However, for many sources, this characterization over-simplifies the reality that the emitted ROC has many constituents with differing vapor pressures that consequently partition between the gas phase and an organic-aerosol phase according to ambient conditions.5,13 A state-of-the-science viewpoint considers ROC emissions as a continuum in volatility3 that spans from the most (i.e., volatile organic compounds, VOCs) to least volatile compounds (i.e., POA).
Chemical transport models (CTMs) are crucial tools for understanding the sources of OA and evaluating the effectiveness of air quality management policies. The widely adopted volatility basis set (VBS) approach to OA modeling13 follows the “volatility-continuum” conceptual model by grouping OA precursor emissions into volatility bins defined by saturation vapor pressure (C*) ranging from 10−3 μg m−3 (least volatile) to >106 μg m−3 (most volatile). However, the computational complexity of the VBS approach restricts its application in advanced modeling techniques like online source apportionment14 and sensitivity analysis.15 Quantifying OA source contributions is particularly important for air quality planners to develop effective emission control strategies. Some modeling studies have addressed this need by combining a VBS approach with “brute-force” sensitivity analysis,16–18 which defines the change in simulated SOA concentration resulting from an emission change as representing the source contribution. The “brute-force” method, however, is practically cumbersome (i.e. number of simulations scales linearly with the number of source contributions) and may not be suitable to retrieve source contributions when the relationship between concentration and emissions is non-linear.15,19,20 On the other hand, tagged species methodology, such as the Particulate Source Apportionment Technology (PSAT) implemented in the Comprehensive Air Quality Model with Extensions21 (CAMx) is an efficient tool for source apportionment and is considered more appropriate under non-linear situations.20,22 Unfortunately, the application species-tagging to VBS is challenging due to its complexity and would be computationally expensive.
The two-product SOA scheme, proposed by Odum et al.,23 is simpler and more efficient than VBS and has been coupled with tagged species source tracking.21,24 The two-product model describes SOA formation from ROC oxidation using two semivolatile products, usually with high and low volatility that are derived by fitting chamber experiments.23 Two-product schemes were developed to model SOA and have been implemented in CTMs in combination with a simple non-volatile POA scheme24,25 which tends to overestimate OA near emission sources.26
This study aims to develop an efficient “volatility-continuum” modeling scheme that can support detailed source apportionment to assist air quality management. To do this, we start with an existing two-product scheme and make targeted modifications that improve coherence with the “ROC-continuum” concept. Meanwhile, we retain the simplicity and computational efficiency of the two-product scheme to preserve compatibility with efficient source-tracking methodologies (work in progress). Our results demonstrate a feasible and readily implemented methodology for improving the existing two-product OA modeling employed in many CTMs.
2. Methods
2.1 Development of the CAMx SOAP3 scheme
CAMx is a widely used air quality model and provides a two-product scheme for SOA modeling, which is referred to as SOAP2.2 (hereafter SOAP2). The SOAP2 scheme, illustrated by the grey lines in Fig. 1, represents the oxidation of anthropogenic VOCs (AVOCs, including benzene, toluene, and xylene), intermediate volatility organic compounds (IVOCs), and biogenic VOCs (BVOCs, including isoprene, monoterpenes, and sesquiterpenes) into condensable gases (CGs), followed by equilibrium partitioning between CG and SOA: | SOA precursor + oxidant → CGs ↔ SOA | (1) |
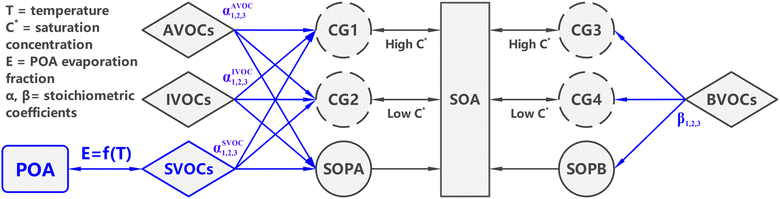 |
| Fig. 1 Schematic diagram of the SOAP3 scheme (updates to the existing SOAP2 scheme are presented in blue). | |
Two condensable products, one more-volatile (CG1 for anthropogenic and CG3 for biogenic) and one less-volatile product (CG2 for anthropogenic and CG4 for biogenic), are utilized. In addition, non-volatile products (SOPA for anthropogenic and SOPB for biogenic, respectively) from the oxidation step instantly condense to form SOA. POA is treated separately from SOA and considered non-volatile in SOAP2.
The Community Multiscale Air Quality (CMAQ) model (version 5.30) includes the “AERO7” which tracks SOA formation from AVOCs, BVOCs and IVOCs using a combination of VBS, two-product and one-product approaches27 (Table S3†). For instance, AERO7 uses a VBS approach with four bins to represent SOA formation from AVOCs and a VBS with seven bins for monoterpenes. Isoprene and sesquiterpene oxidation products are parameterized with two and one semivolatile products, respectively. IVOC in AERO7 is represented by pcVOC, which oxidizes with OH to form a low-volatility condensable vapor (pcSOG, C* = 10−5 μg m−3) with a molar yield of 1. The SOA aging process in the CMAQ AERO7 scheme involves particle-phase oligomerization (applied to SOA from isoprene, sesquiterpenes, and aromatics) or hydrolysis (applied to SOA from monoterpene-derived organic nitrates). POA is represented using a VBS in AERO7 with gas-phase OH oxidation to form SOA (see details in Section S1†).
CMAQ with AERO7 is widely-used, has been applied for OA over East Asia and achieved acceptable model performance,28,29 and has gone through frequent model updates with respect to OA formation.27,30–33 Therefore, we built the new SOAP3 scheme upon the existing SOAP2 scheme utilizing parameters derived from the AERO7 scheme. The updates made in the SOAP3 scheme are highlighted in blue in Fig. 1, and the key differences between SOAP2 and SOAP3 are summarized in Table 1. It is important to note that although SOAP3 was developed to emulate the AERO7 scheme, the SOAP3 and AERO7 schemes have fundamentally different structures. Another difference is that SOAP3 includes condensed-phase SOA photolysis in contrast to AERO7. The impact of SOA photolysis on simulated SOA concentrations is discussed in later section.
Table 1 Comparison of OA treatment in SOAP2 vs. SOAP3
Species |
Existing SOAP2 scheme |
Newly developed SOAP3 scheme |
POA |
Non-volatile |
Semivolatile, with temperature-dependent evaporation fraction derived from AERO7 |
AVOCs/IVOCs |
Oxidized into CG1/CG2 and non-volatile product (SOPA) |
Same as SOAP2 but with updated SOA yields derived from AERO7 |
SVOCs |
Not considered |
Added in SOAP3, with SOA yields derived from AERO7 |
BVOCs |
Oxidized into CG3/CG4 and non-volatile product (SOPB) |
Same as SOAP2 but with updated SOA yields derived from AERO7; adding SOA formation pathways from heterogeneous uptake of GLY/MGLY and monoterpenes + NO3 |
2.1.1 Partial evaporation of POA to SVOCs.
The first SOAP3 update treats POA as being semivolatile instead of non-volatile in SOAP2. A new species, semi-volatile organic compounds (SVOCs), is added to the SOAP3 scheme to represent the fraction of POA that promptly evaporates to the gas phase after emission (Fig. 1). SVOCs reside in the gas phase and can undergo oxidation to form condensable products and therefore SOA. The evaporation of POA to SVOCs is parameterized as a temperature-dependent function (E = f(T), where E is the fraction of POA that evaporates to SVOCs) that mimics the POA treatment in the AERO7 scheme, as described below.
In the AERO7 scheme, POA emissions are allocated to five bins with volatility (i.e., effective saturation concentration, C*) ranging from 0.1 μg m−3 to 1000 μg m−3 at 298 K (Table S1†). For each bin (i), C* can be adjusted to the local ambient temperature using the Clausius Clapeyron equation (eqn (2)).34
| 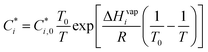 | (2) |
where

and

are the saturation concentrations for volatility bin
i at
T and
T0 (=298 K), respectively.
R is the universal gas constant, and Δ
Hvapi denotes the enthalpy of vaporization for volatility bin
i. The detailed values for each parameter are given in Table S1.
† For each bin, the particle phase fraction of POA is calculated using Pankow's partitioning theory:
5,13,23 |  | (3) |
where
Fi is the fraction of POA in the particle phase for bin
i, and
COA is the ambient OA concentration. A
COA of 50 μg m
−3 was used to represent the high concentrations near the emission source. The influence of different OA concentrations on POA evaporation is discussed in Section 3.4. By summing over the five bins, the total fraction of POA that exists in the particle phase is calculated as:
|  | (4) |
where
fi is the volatility distribution factor used to allocate POA emissions to each volatility bin (Table S1
†). Take an ambient temperature of 290 K as an example. At 290 K, it is calculated that 45% of POA emissions exist in the particle phase. We performed the above calculation at various ambient temperatures to obtain the overall POA gas-particle partitioning as a function of temperature and fitted these results to a polynomial function between the particle fraction of POA (
i.e., 1 −
E) and the ambient temperature (
Fig. 2a). The high
R2 values (0.994) indicate that a polynomial function captures the relationship quite well, which SOAP3 applies to allocate the POA emissions between the gas phase (
i.e., SVOCs) and the remaining particle phase (
i.e., POA) according to the local temperature.
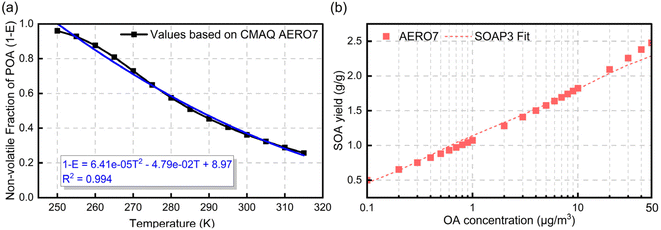 |
| Fig. 2 (a) Polynomial fitting between particle fraction of POA (1 − E) and temperature with COA = 50 μg m−3; (b) fitting of SOAP3 SOA formation from SVOCs to AERO7 over a range of total OA concentration from 0.1 μg m−3 to 50 μg m−3. | |
2.1.2 Adding SOA formation from SVOCs.
POA that evaporates to SVOCs in the SOAP3 scheme undergoes oxidation and partitioning similar to the scheme for AVOCs and IVOCs: | SVOCs + OH = α1,SVOCs × CG1 + α2,SVOCs × CG2 + α3,SVOCs × SOPA | (5) |
where α1,SVOCs, α2,SVOCs, and α3,SVOCs are stoichiometric coefficients. The rate constant for SVOC + OH is set to be 4 × 10−11 cm3 per (molecules per s). We derived SVOCs α values to reproduce the SOA mass yields simulated by the AERO7 scheme. To do so, we first calculate SOA yields from the evaporated fraction of POA after a representative amount of OH exposure (e.g., 1010 molecules per cm3) in the AERO7 scheme, using an offline conceptual model28 (also see example calculation in Section S1†). This calculation is performed for a wide range of total OA concentrations from 0.1 μg m−3 to 50 μg m−3 (Fig. 2b). Meanwhile, the corresponding SOA yields from SVOCs in the SOAP3 scheme at different OA concentrations are calculated as: |  | (6) |
where N now equals 3 for eqn (5) (compared to 10 in AERO7). A scatter plot of SOAP3-generated and AERO7-generated SOA mass at different OA concentrations can be established based on the αi values. The three coefficients (α1,SVOCs, α2,SVOCs, and α3,SVOCs) were fitted to obtain the highest R2 value and slope closest to one (Fig. 2b). The final stoichiometric coefficients were converted from g g−1 to ppm ppm−1 (Table 2) for model implementation.
Table 2 SOA molar-yield coefficients (under high/low NOx conditions) in the SOAP3 scheme
C* (μg m−3)@300 K |
CG1 14 |
CG2 0.31 |
SOPA 0 |
SOA mass yields (g g−1) at COA = 10 μg m−3 |
See Section S2 for organic nitrates yields from monoterpenes + NO3.
|
Benzene |
0.1874/0 |
0/0 |
0.0036/0.1314 |
0.160/0.370 |
Toluene |
0.0921/0 |
0.0123/0 |
0/0.126 |
0.082/0.300 |
Xylene |
0.0637/0 |
0/0 |
0.005/0.174 |
0.047/0.360 |
IVOCs |
0 |
0 |
0.964 |
1.000 |
SVOCs |
1.79 |
1.73 |
0.16 |
1.813 |
C* (μg m−3)@300 K |
CG3 26 |
CG4 0.45 |
SOPB 0 |
SOA mass yields at COA = 10 μg m−3 |
Isoprene |
0.038 |
0.0076 |
0 |
0.047 |
Monoterpenesa |
0.1665 |
0.038 |
0.031 |
0.159 |
Sesquiterpenes |
1.405 |
0.1133 |
0 |
0.440 |
2.1.3 Adjusting SOA yields from AVOCs and IVOCs.
The AVOCs oxidation and partitioning process in the existing SOAP2 scheme is as follows: | AVOCs + OH = α1,AVOCs × CG1 + α2,AVOCs × CG2 + α3,AVOCs × SOPA | (7) |
In SOAP3, we only updated the stoichiometric coefficients (α1,AVOCs, α2,AVOCs, α3,AVOCs) based on the AERO7 parameterization. To do this, we first calculated the AERO7 SOA yields from different AVOCs (e.g., benzene, toluene, and xylene) at different OA concentrations (range from 0.1 to 50 μg m−3) using the same method illustrated by eqn (6), with relevant information listed in Table S3.† Similarly to SVOCs, the three coefficients were fitted to obtain results closest to AERO7 under high and low NOx conditions (Fig. 3a–c). In AERO7, SOA aging from AVOCs is represented by oligomerization processes in the particle phase to form non-volatile products with a rate of 9.49 × 10−6 s−1 (equivalent to a lifetime of 20.5 h), which is adopted in SOAP3.
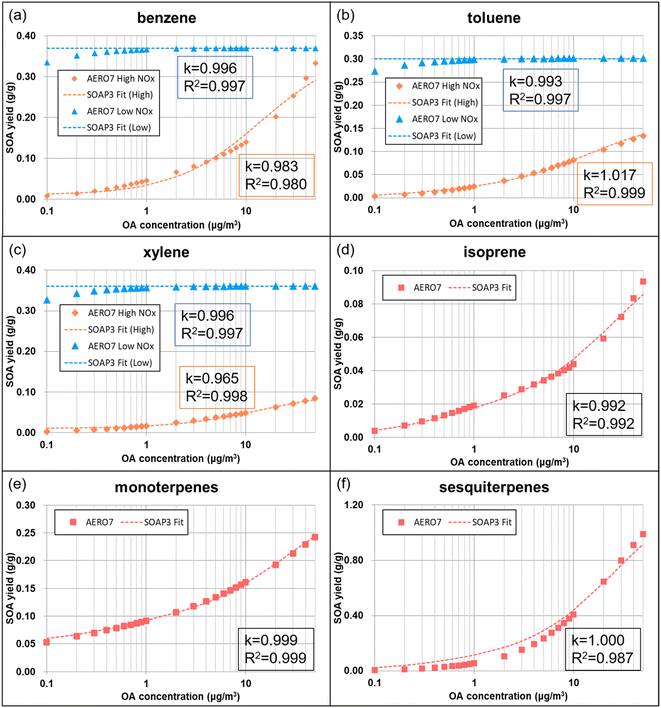 |
| Fig. 3 Fitting of SOAP3 SOA formation from (a) benzene, (b) toluene, (c) xylene, (d) isoprene, (e) monoterpenes, and (f) sesquiterpenes to AERO7 over a range of total OA concentration from 0.1 μg m−3 to 50 μg m−3. | |
IVOCs emissions have been reported to be an important precursor of SOA.35–38 The IVOCs oxidation and partitioning process in the existing SOAP2 scheme is similar to SVOCs (eqn (5)) and AVOCs (eqn (7)). The current values of α1, α2, and α3 used in the SOAP2 scheme underestimate SOA yields from IVOC as compared to AERO7 (Section 3.2). Therefore, we updated the coefficients of IVOCs by fitting the SOA yields based on the AERO7 scheme. In AERO7, IVOCs are oxidized by OH to form an extremely low-volatility product (C* = 10−5 μg m−3) with a molar yield of 1.32 Accordingly, we set the molar yield from IVOCs as 1 in SOAP3 by setting α3,IVOCs to 1 and α1,IVOCs and α2,IVOCs to 0.
2.1.4 Adjusting SOA yields from BVOCs.
The SOA formation from BVOCs in the existing SOAP2 scheme is similar to AVOCs: | BVOCs + oxidant = β1 × CG3 + β2 × CG4 + β3 × SOPB | (8) |
where β1, β2, and β3 are stoichiometric coefficients. As shown in Section 3.2.4, SOA formed from BVOCs (i.e., BSOA) in the existing SOAP2 scheme is significantly underestimated compared to AERO7. To resolve these discrepancies, we made several updates to the SOA formation from BVOCs in the new SOAP3 scheme. First, we updated the stoichiometric coefficients for isoprene, monoterpenes, and sesquiterpenes in SOAP3 by fitting them to AERO7 results using the same method as AVOCs (Fig. 3e and f). Second, while testing, we noticed that the organic nitrates from monoterpenes oxidation by NO3 in CMAQ AERO7 represent an important contribution to BSOA aging by hydrolysis. In contrast, in the existing SOAP2 scheme, the formation of organic nitrates is significantly underestimated. Therefore, we adjusted the yield coefficients of organic nitrates in SOAP3 and accounted for the aging effects due to hydrolysis in AERO7 (see details in Section S2†). Lastly, SOA formation via heterogeneous uptakes of glyoxal (GLY) and methylglyoxal (MGLY) on the aerosol surface, which is also found to be a non-negligible pathway in AERO7, was now added in CAMx SOAP3 (see details in Section S2†).
2.2 Model configurations
We tested the new SOAP3 scheme and compared the results with the existing SOAP2 scheme in CAMx and the AERO7 scheme implemented in CMAQ using the same model configuration as our previous study.28 The CAMx model configuration utilized the CB6 photochemical gas-phase mechanism,39 with a static two-mode coarse/fine (CF) PM chemistry option incorporating the ISORROPIA inorganic gas-aerosol partitioning scheme,40 along with the Regional Acid Deposition Model (RADM) for aqueous phase chemistry, the Zhang dry deposition option,41 and wet deposition. For CMAQ simulations, the model configuration included the CB6 gas-phase mechanism, RADM aqueous phase chemistry, ISORROPIA inorganic particulate thermodynamics, and the AERO7 aerosol scheme.27 SOA concentrations in China during July (summer) and November (autumn) 2018 were simulated, covering the entire country with a spatial resolution of 36 km (Fig. 4). Meteorological fields were simulated using the Weather Research and Forecasting (WRF) model (version 4.0)42 with configurations detailed in our previous studies.36 The anthropogenic emissions comprised the Multi-resolution Emission Inventory of China (MEIC, https://www.meicmodel.org, accessed on December 1, 2022) for conventional air pollutants and the S/IVOCs emission inventory developed by Wu et al.43 BVOCs emissions were calculated using a recent offline version of the Model of Emissions of Gases and Aerosols from Nature (MEGAN version 3.2, https://aqrp.ceer.utexas.edu/projects.cfm, accessed on September 25, 2022). For this study, the partial evaporation of POA emissions to SVOCs was treated outside CAMx as a pre-processing step (offline scheme) using gridded monthly averaged 2 m temperature simulated by the WRF model. POA evaporation is being moved inside CAMx (in-line scheme) for the next public release version of CAMx.
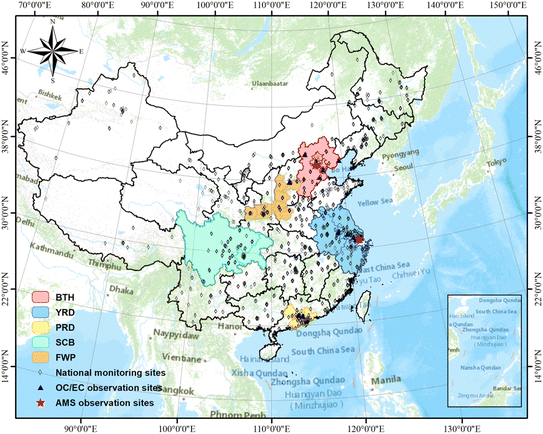 |
| Fig. 4 Modeling domain with definitions of key regions and locations of 1477 national monitoring sites (in black diamonds), 13 sites with OC/EC observations (in blue triangles) and 2 AMS sites (in red stars). | |
Simulations were conducted over all of China for July and November 2018, with a horizontal resolution of 36 km (Fig. 4). For each model/scheme, we conducted three parallel simulations with different configurations of the input emissions (Table S4†). For the “Base” scenarios, all SOA precursors, including VOCs, IVOCs, and SVOCs, were included. For the “No_SVOCs” scenarios, SVOCs emissions were excluded, and similarly, in the “No_S/IVOCs” scenarios, only VOCs emissions were considered for SOA formation. Different OA components, including POA, BSOA, and SOA formed from AVOCs (ASOA), IVOCs (IVOC-SOA), and SVOCs (SVOC-SOA), were either direct outputs (e.g. POA, BSOA) or calculated as differences between two sets of scenarios. For example, IVOC-SOA is calculated as the difference of SOA between the “Base” and “No_SVOCs” scenario while SVOC-SOA is the difference between the “No_SVOCs” and “No_S/IVOCs” scenario. We compared results for five key regions in China (Fig. 4), including the Beijing–Tianjin–Hebei (BTH), Yangtze River Delta (YRD), Pearl River Delta (PRD), Sichuan Basin (SCB), and Fenwei Plain (FWP).
2.3 Model performance evaluation
We evaluated the simulated concentration of PM2.5, primary organic carbon (POC), and secondary organic carbon (SOC) following the same approach in our previous study.28 The simulated concentrations of PM2.5 were compared against surface observations of hourly PM2.5 at 1477 national monitoring sites. Hourly observed OC/EC at 13 monitoring sites (see detailed locations in Table S5†) were used to evaluate simulated POC and SOC by applying the minimum OC/EC ratio method:44 | SOC = OC − (OC/EC)pri × EC | (9) |
where (OC/EC)pri represents the minimum value of OC/EC ratio during the observation period (see Table S5†). We calculated the values of SOC/OC from the observed data and compared it with the simulated values of the SOAP2, SOAP3, and AERO7 schemes.
In addition, SOA factors resolved using the positive matrix factorization (PMF) analysis of measurements obtained with an aerosol mass spectrometer (AMS) at a supersite in Shanghai45 and an Aerodyne time-of-flight aerosol chemical speciation monitor (ToF-ACSM) in Beijing46 were used to further validate the model performance of simulated SOA. The assessment of model performance was conducted using well-established statistical metrics, including the correlation coefficient (R), mean bias (MB), normalized mean bias (NMB), normalized mean error (NME), root mean square error (RMSE), and fractional bias (FB).
3. Results and discussion
3.1 Model performance of the SOAP3 scheme
Fig. 5 shows the spatial distribution of monthly averaged PM2.5 simulated by the SOAP3 scheme with observed values from 1477 national monitoring sites superimposed. The simulation results generally align with the spatial distributions and seasonal variations of observed PM2.5. Both months show slight overestimation, with MB (NMB) of 1.8 μg m−3 (7%) in July and 0.6 μg m−3 (1%) in November. Regionally, an overestimation of PM2.5 is observed over SCB (Table S6†), which is also found in our previous study.28 PM2.5 is underestimated over PRD and FWP in July and over BTH and FWP in November. We further evaluated simulated POC, SOC, and the ratio of SOC to OC (SOC/OC) at limited observation sites (Fig. S1–S5†). The model performance for simulated SOC varies across different sites (NMB ranging from −39.8% to 55.8% in July and −51.4% to 66.7% in November), with a general pattern of underestimation in November. In terms of SOC/OC (Fig. S3†), the original SOAP2 scheme notably underestimated observed SOC/OC, especially in November, while the results from the AERO7 scheme were closer to the observations. The newly developed SOAP3 scheme demonstrates substantial improvements, yielding slightly lower values compared to AERO7. However, all schemes failed to capture observed SOC/OC for specific sites in November, indicating the presence of other uncertainties, such as those related to emissions. Fig. S6† further compares the observed and modeled POA fraction at various monitoring sites, including observations collected from other studies (e.g. Chen et al.47). The results indicate a comparable or slightly lower POA fraction between the observed values and those simulated by SOAP3, with the exception of four sites in the BTH region. The time series plots (Fig. S4†) reveal that the simulated POC concentrations demonstrate good performance for sites in the YRD region (specifically JS_CZ, SH, and SZ). However, for sites in the BTH region (namely BJ, TJ, and HB_CZ), the simulated POC concentrations and the fraction of POA/OA are notably higher than the observed values, regardless of the models used. This may suggest that the over-predictions were due to bias in the POA emissions in the BTH region.
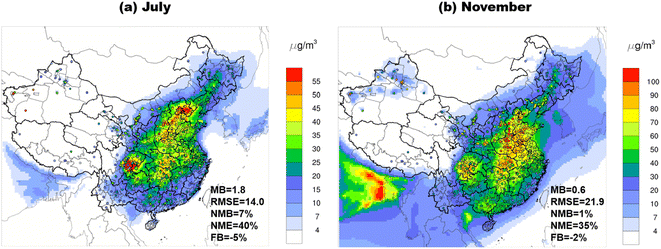 |
| Fig. 5 Comparison of the monthly average simulations and observations of PM2.5 by 1477 national monitoring sites, (a) July 2018, (b) November 2018. | |
Fig. 6 shows the simulated and AMS-based SOA concentration at the ToF-ACSM site in Beijing (July) and the AMS site in Shanghai (November). The original SOAP2 scheme underestimated the observed SOA concentrations, with an NMB of −60% in Beijing and −69% in Shanghai. In contrast, the AERO7 scheme showed a slight underestimation at one site (NMB of −7% for Beijing) and an overestimation at the other site (NMB of 6% for Shanghai). With the new SOAP3 scheme, the large underestimation of SOA in the SOAP2 scheme was greatly mitigated, resulting in NMB values of −11% and −24% at the Beijing and Shanghai sites, respectively. While not entirely comparable, the performance of the SOA simulation with SOAP3 met the “goal” benchmarks for OC and OM proposed by Huang et al.48 for the application of CTMs in China. The “goal” benchmarks, defined as NMB < ± 35% for OC/OM, were derived from the top 33rd percentile values collected from existing studies. However, it is worth noting that during the initial half of the observation period in Shanghai, all schemes failed to capture the observed SOA concentrations. The exact cause for this underestimation remains elusive, as the biases in the simulated meteorological conditions were insufficient to explain the phenomenon. One potential explanation could be unaccounted emissions, highlighting the need for future investigation in future studies.
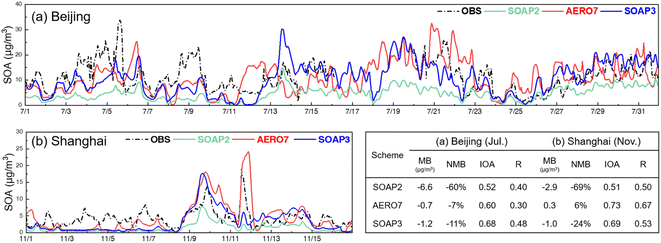 |
| Fig. 6 Simulated and AMS-based concentrations (a) by ToF-ACSM in Beijing (July 2018) and (b) by AMS in Shanghai (November 1 to 16, 2018). | |
3.2 OA concentrations simulated by SOAP3
Fig. 7 shows the domain-averaged absolute concentrations of OA components simulated by the SOAP3 scheme and the other two schemes (i.e., the original SOAP2 scheme and AERO7 scheme) for the selected five key regions. The corresponding spatial distributions for each component are presented in Fig. S7 and S8.† With the newly developed SOAP3 scheme, the simulated OA concentrations ranged from 7.5 μg m−3 (PRD) to 11.7 μg m−3 (FWP) in July and from 8.8 μg m−3 (SCB) to 14.8 μg m−3 (YRD) in November (Table S7†). Spatially, high OA concentrations were observed over BTH and YRD in July and November. Compared to the original SOAP2 scheme, SOAP3 simulated OA concentrations generally show a substantial increase of 29–77%. As discussed below, the changes in total OA concentrations from SOAP2 to SOAP3 reflect the contribution of two opposing effects of reduced POA proportion (by 10–24%) and increased SOA concentration (by 45–193%) from different precursors in the SOAP3 scheme. On the other hand, compared to the AERO7 scheme, the SOAP3 simulated OA concentrations are lower by 4–21% (except for FWP in July and SCB in both months).
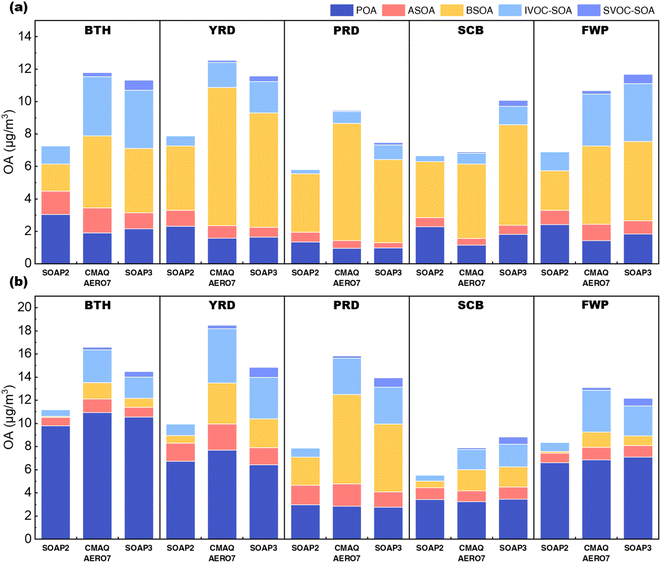 |
| Fig. 7 Domain-averaged concentrations of OA components simulated with different schemes in (a) July and (b) November 2018. | |
3.2.1 POA evaporation.
POA simulated by SOAP2 and AERO7 show similar spatial and seasonal variations, although differing in magnitudes. In July, SOAP2 shows significantly higher values than AERO7, while in November, SOAP2 indicates slightly lower values. Both models simulate high POA concentrations over Northeast China, BTH, northern YRD, and Sichuan Basin, which closely follows the spatial distribution of POA emissions. With the SOAP2 scheme, domain-averaged POA concentrations ranged from 1.3 μg m−3 (PRD) to 3.0 μg m−3 (BTH) in July and from 3.0 μg m−3 (PRD) to 9.8 μg m−3 (BTH) in November. With AERO7, the simulated POA concentrations ranged from 1.0 μg m−3 (PRD) to 1.9 μg m−3 (BTH) in July and from 2.9 μg m−3 (PRD) to 10.9 μg m−3 (BTH) in November. Despite the higher POA concentrations in SOAP2 due to non-volatile treatment, AERO7 compensates by introducing SVOCs emissions, which are not accounted for in SOAP2. The inclusion of SVOCs in AERO7, allocated partially to non-volatile bins, contributes to higher POA concentrations. Consequently, in November, where less POA evaporation occurs due to lower temperature, AERO7 shows slightly higher simulated POA concentrations compared to SOAP2.
The newly developed SOAP3 scheme incorporates a temperature-dependent evaporation function, allowing POA to partially evaporate into the gas phase. With this feature, POA concentrations simulated by SOAP3 are lower than SOAP2 by 21–29% in July, bringing results closer to those of AERO7. The SOAP3 simulated POA concentrations ranged from 1.0 μg m−3 (PRD) to 2.2 μg m−3 (BTH) in July and from 2.8 μg m−3 (PRD) to 10.6 μg m−3 (BTH) in November. Similarly to the AERO7 scheme, SVOCs are now incorporated into SOAP3, with the non-volatile fraction of SVOCs emissions offsetting the reductions in POA concentrations due to evaporation, particularly in November when evaporation effects are weaker than in July.
3.2.2 SOA formation from the newly added SVOCs.
SOA formation from the gas-phase portion of SVOCs is included in the SOAP3 scheme, with SOA yields based on the AERO7 scheme. As shown by Fig. 7 and Table S7,† the SVOC-SOA concentrations simulated by the SOAP3 scheme ranged from only 0.2 μg m−3 (PRD) to 0.6 μg m−3 (BTH) in July and from 0.5 μg m−3 (BTH) to 0.9 μg m−3 (YRD) in November, making it an overall small fraction (<10%) of the total OA concentrations. Meanwhile, the AERO7 scheme also indicates a low contribution of SOA formation from the SVOCs emissions, with even lower magnitudes. The small discrepancies between AERO7 and SOAP3 are explained in Section S1 of the ESI.†
The relatively small contribution from SVOC-SOA was also noticed in our previous study28 but is different from Miao et al.,49 who found a dominant role of SVOC-SOA (30–39% in July) to total OA concentrations over eastern China. The uncertainties associated with the estimated SVOCs emissions could be causing these differences in addition to the differences in model parameterizations.
3.2.3 Increased SOA formation from IVOCs.
IVOCs emissions are considered an important SOA precursor. In the original SOAP2 scheme, however, the simulated IVOC-SOA concentrations were significantly lower (by 2–5 times) than those simulated by AERO7. For instance, in BTH, the domain-averaged IVOC-SOA concentration simulated in the SOAP2 scheme was 1.1 μg m−3 in July and 0.6 μg m−3 in November. When the AERO7 scheme is applied, the corresponding values are 3.6 μg m−3 and 2.8 μg m−3, respectively. In the newly developed SOAP3 scheme, the SOA yields from IVOCs emissions were adjusted to mimic the AERO7 scheme. With this change, the SOAP3 simulated IVOC-SOA concentrations increase substantially, as compared to SOAP2, by 207–232% in July and 222–307% in November. Domain-averaged IVOC-SOA simulated with the SOAP3 scheme ranged from 0.9 μg m−3 (PRD) to 3.6 μg m−3 (BTH) in July and from 1.8 μg m−3 (BTH) to 3.6 μg m−3 (YRD). These values are much closer to those predicted by the AERO7 scheme. Although magnitudes differ, all three schemes exhibit similar seasonal variations with higher values in November (except for BTH and FWP). Regionally, elevated IVOC-SOA concentrations were observed in BTH, FWP, and YRD in July and shifted southward in November.
3.2.4 SOA formation from traditional VOCs.
Simulated ASOA concentrations are relatively consistent across the three schemes compared to the other SOA components. With the SOAP3 scheme, domain-averaged ASOA concentrations ranged from 0.3 μg m−3 (PRD) to 1.0 μg m−3 (BTH) in July and from 0.8 μg m−3 (BTH) to 1.5 μg m−3 (YRD) in November. Spatially, higher levels of ASOA were predominantly observed in the BTH and FWP regions in July, whereas in November, elevated ASOA concentrations were mainly observed in the YRD and PRD regions (Fig. S7 and S8†). Similar seasonal and spatial variations were observed for the other two models.
BSOA simulated by the SOAP2 and AERO7 schemes showed similar spatial (higher concentrations in southern regions, e.g., PRD and YRD) and seasonal (higher values in summer) variations, consistent with existing studies.50–52 However, the magnitudes differ significantly, with AERO7 exhibiting much higher values. In July, for instance, domain-averaged BSOA concentration simulated by the AERO7 scheme ranged from 4.4 μg m−3 (BTH) to 8.5 μg m−3 (YRD), approximately two times the value simulated by SOAP2. The much higher BSOA in the CMAQ AERO7 scheme, compared to the SOAP2 scheme, is likely associated with higher SOA yields from monoterpene53 and more SOA pathways included.30,33 With updated SOA yield coefficients and additional formation pathways (Section S2†) in the SOAP3 scheme, simulated BSOA concentrations significantly increased and exhibited greater alignment with the AERO7 results. In July, domain-averaged BSOA concentrations ranged from 4.0 μg m−3 (BTH) to 7.1 μg m−3 (YRD), while in November, they ranged from 0.8 μg m−3 (BTH) to 5.9 μg m−3 (PRD).
3.3 Relative contribution of different OA components
Fig. 8 compares the relative contribution of OA components predicted by SOAP3, SOAP2, and AERO7. In July, BSOA emerges as the most abundant component for all five regions, with relative contributions ranging from 35.1% in BTH to 68.5% in PRD with SOAP3. This trend holds for the other two schemes (except BTH in SOAP2), and AERO7 anticipates slightly higher BSOA contributions (37.7% in BTH to 76.3% in PRD). Meanwhile, IVOC-SOA contribution is comparable to BSOA in BTH and FWP under the SOAP3 scheme, reaching 31.7% and 30.4%, respectively. These values significantly exceed SOAP2 predictions and align with AERO7. The relative contribution from POA ranges from 13.1% (PRD) to 19.2% (BTH) with SOAP3, falling in between SOAP2 and AERO7 and showing closer agreement with AERO7. ASOA stands as a moderate contributor with SOAP3, ranging from 4.4% (PRD) to 8.6% (BTH), which is slightly lower than AERO7.
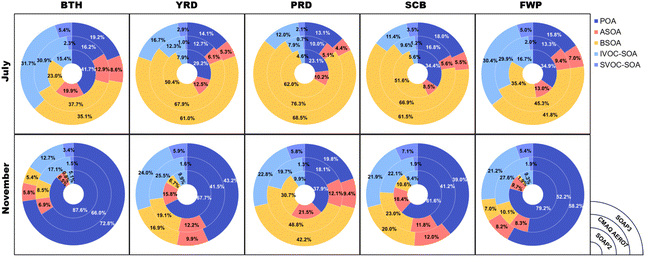 |
| Fig. 8 Domain-averaged OA proportion in July and November 2018 (including POA, ASOA, BSOA, IVOC-SOA, and SVOC-SOA). | |
November sees POA ascend to the dominant OA component for all regions except PRD, reaching 39.0% (SCB) to 72.8% (BTH) with SOAP3. These values are considerably lower than SOAP2. BSOA remains dominant in PRD with 42.2%. IVOC-SOA retains its importance, ranging from 12.7% (BTH) to 24.0% (YRD). Both SOAP3 and AERO7 identify SVOC-SOA as the least abundant component, with SOAP3 predicting a range of 2.1% (PRD) to 5.4% (BTH) in July and 3.4% (BTH) to 7.1% (SCB) in November. Overall, AERO7 predicts the highest BSOA contribution, while SOAP2 predicts the highest POA contribution. The modifications implemented in SOAP3 position it between the other two schemes and much closer to the AERO7 scheme.
3.4 Uncertainties and limitations
Compared to the original SOAP2 scheme, the updated SOAP3 scheme exhibited substantial improvement in capturing the observations. However, there are still some uncertainties and limitations associated with the current implementation. Firstly, the evaporation of POA emissions in the current version of SOAP3 was implemented offline (i.e., outside CAMx) using monthly averaged temperatures instead of in-line using hourly temperatures. POA emissions exhibit diurnal variations, with higher emissions during the daytime and lower emissions during the nighttime. The higher daytime temperatures are expected to result in more POA evaporation, thus leading to lower POA concentrations. The full implementation of the dynamic POA evaporation in response to variations in hourly temperatures is under development and will be available in the public release of the latest CAMx version. The current POA partitioning scheme simplifies the dynamics of POA evaporation and further aging and, therefore, does not fully account for POA aging. This may cause the scheme to overestimate the POA/SOA ratio in aged air masses.
Secondly, when deriving the temperature-dependent POA evaporation, a COA of 50 μg m−3 was used to calculate the particle phase fraction of POA. Fig. S9† demonstrates the impact of different OA concentrations on the partition of POA between gas and particle phases. Higher OA concentrations are expected to lead to higher fractions in the particle phase. At T = 290 K, the particle-phase fraction increases from 32% to 53% when COA increases from 10 μg m−3 to 100 μg m−3. We conducted a CAMx sensitivity simulation (case 1) using a POA evaporation function derived with a COA of 20 μg m−3 (Fig. S10†) and found more POA evaporation into the gas-phase, as expected, resulting in POA reductions of 14.7–16.8% in July and 14.6–15.7% in November for different regions. The impact on SOA was minimal (−0.1% to 1.0% in July and 2.2–5.8% in November) because of compensating impacts of having less POA for SOA to condense on being offset by having more gas-phase precursors to produce SOA. However, it should be pointed out that the impact of COA on POA evaporation is less pronounced compared to the variations with temperature. Moreover, explicitly incorporating the dependence on COA, as in VBS scheme, could introduce additional uncertainty due to the introduction of a feedback loop where underpredicted COA would lead to more evaporation, thus resulting in further underestimated COA.
Thirdly, SOA yields have many uncertainties including the effect of NOx on yields and SOA production from the IVOC category which has chemically diverse constituents. We investigated sensitivity to these two SOA yield uncertainties by conducting another CAMx sensitivity simulation (case 2) with two changes: (a) reducing the SOA yields from IVOC by half and (b) reducing the SOA yields of BVOCs (i.e., isoprene, monoterpene, sesquiterpenes) under high-NOx conditions by 30% based on chamber studies by Sarrafzadeh et al.54 and Wildt et al.55 that carefully investigated this issue. As shown by Fig. S11,† the SOA yields from BVOCs in case 2 are reduced by 18.1–22.7% in July and less than 10% in November (except for PRD, 15.1%). The SOA yields from IVOC in case 2 are reduced by 49.6–50.8% and these reductions are similar among different regions and seasons. Consequently, the relative importance of different OA components changed slightly in case 2. For instance, for BTH, YRD and FWP in July, POA exceeds the IVOC-SOA, becoming the second most abundant OA component following BSOA. In November, the SOA yields from IVOC are less than BSOA in SCB and YRD. Thus there is a continuing need to refine SOA yield parameterizations for IVOC, BVOC and the effect of NOx on yields.
Lastly, condensed-phase SOA photolysis has been shown to exhibit a substantial effect on SOA formation.56–58 For example, by applying an empirical SOA photolysis rate (JSOA) of 0.04% × JNO2 (NO2 photolysis rate) in GEOS-Chem, Hodzic et al.56 showed a decrease of 40–60% in SOA yields at mid-latitudes in the summer. This condensed-phase SOA photolysis is not considered in the CAMQ AERO7 scheme and but was implemented in the SOAP3 scheme. A sensitivity simulation was conducted on top of the current SOAP3 scheme by turning off the SOA photolysis. As illustrated by Fig. S12,† particle-phase SOA photolysis reduced the simulated SOA concentrations by 14% (PRD) to 33% (FWP) in July and by 17% (BTH) to 22% (SCB) in November. We consider representing SOA photolysis to be necessary in SOAP3 although the current parameterization is simple and can be improved as more information becomes available.
4. Conclusions and recommendations
Existing schemes for modeling OA in CTMs have strengths and limitations inherent to their formulation. VBS schemes unify the representation of POA and SOA, can describe complex relationships between emissions composition, atmospheric conditions and OA concentration, but incur relatively greater computational cost due to their complexity. The combination of a two-product SOA scheme with non-volatile POA is relatively simple and efficient, compatible with powerful modeling tools for detailed source contribution and sensitivity analysis, but fails to describe the partial evaporation of POA following emission and dilution to ambient conditions. In this study, we improved the CAMx SOAP2 scheme (non-volatile POA with two-product SOA) to a new SOAP3 scheme, resulting in better performance in simulating OA components while retaining computational simplicity. Improvements for SOAP3 are derived by parameterizing behaviors simulated by the CMAQ AERO7 scheme. First, by virtue of the fact that POA is semivolatile, we developed temperature-dependent partial evaporation of POA to SVOCs for SOAP3. Second, we added atmospheric oxidation of SVOCs to SOA by fitting parameters to the POA aging scheme in AERO7. We also updated SOA formation from IVOCs and VOCs by fitting parameters to the AERO7 scheme. Finally, we added formation pathways from monoterpene nitrates and heterogeneous uptake of GLY/MGLY to improve the simulation of BSOA. As a result, POA concentrations decreased (21–29%) with SOAP3 compared to SOAP2 in July. The SOA production from IVOCs increased by 2–3 times with SOAP3 compared to SOAP2. The newly-added SOA production from SVOCs contributed a small part of OA (2–7%) with SOAP3. In summary, SOAP3 performs more like the CMAQ AERO7 scheme than SOAP2 in terms of the simulated OA components and improved accuracy in comparison with observations. While we acknowledge that our results are subject to uncertainties, especially uncertainties associated with the precursor emissions (e.g. Ling et al., 59 Huang et al., 28 and Chen et al.47), our primary focus is developing an efficient modeling framework and we recommend that this framework should be maintained to account for improvements in science and community knowledge.
In terms of computational performance, a direct comparison of computational time between CAMx SOAP3 and CMAQ AERO7 simulations would be uninformative because many model processes, not only the SOA scheme, influence computational performance. The efficiency of the SOAP3 scheme relative to VBS schemes is evident from the smaller number of model species which influences computational requirements throughout the model. SOAP3 requires 12 model species (POA, SVOC, CG1, CG2, CG3, CG4, SOA1, SOA2, SOA3, SOA4, SOPA, and SOPB) to represent OA, whereas the CAMx 1.5D VBS OA scheme employs 50 model species and the CMAQ AERO7 OA scheme employs more than 60 model species. Furthermore, the efficiency of SOAP3 extends to advanced tagged-species source apportionment techniques like CAMx Particulate Source Apportionment Technology (PSAT) which is widely-used to track contributions from many source sectors and/or regions in a single simulation (e.g. Li et al.,60 Liu et al.,61 Lu et al.,62 and Wang et al.63). SOAP3 requires fewer PSAT tagging species than a VBS-style framework such as CAMx 1.5D VBS and the PSAT implementation is much simpler for SOAP3.
The current implementation of SOAP3 has several limitations that can be addressed by follow-up work. In this study, we modeled partial evaporation of POA offline using monthly average gridded temperatures, which will be improved by implementing an in-line calculation using hourly gridded temperatures. Sensitivity simulations indicate a non-negligible impact of photolysis on simulated SOA concentrations, highlighting the need for further investigation. We developed parameters for SOAP3 by analyzing behaviors of the CMAQ AERO7 scheme, but these parameters could also be derived by analyzing different schemes utilized in other CTMs, such as WRF-Chem64 and CHIMERE.65 Nevertheless, our study demonstrates a feasible and readily implemented methodology for improving the existing two-product OA modeling framework employed in many CTMs.
Data availability
The code for SOAP3 and data will be available upon request to the corresponding authors.
Conflicts of interest
The contact author has declared that none of the authors has any competing interests.
Acknowledgements
This study has been supported by the National Natural Science Foundation of China (Grant No. 42375103, 42005112, 42375102). This work is supported by the Shanghai Technical Service Center of Science and Engineering Computing, Shanghai University.
References
- GBD, Global burden of 87 risk factors in 204 countries and territories, 1990–2019: a systematic analysis for the Global Burden of Disease Study 2019, Lancet, 2020, 396, 1223–1249 CrossRef PubMed
.
- J. L. Jimenez, M. R. Canagaratna, N. M. Donahue, A. S. H. Prevot, Q. Zhang, J. H. Kroll, P. F. DeCarlo, J. D. Allan, H. Coe, N. L. Ng, A. C. Aiken, K. S. Docherty, I. M. Ulbrich, A. P. Grieshop, A. L. Robinson, J. Duplissy, J. D. Smith, K. R. Wilson, V. A. Lanz, C. Hueglin, Y. L. Sun, J. Tian, A. Laaksonen, T. Raatikainen, J. Rautiainen, P. Vaattovaara, M. Ehn, M. Kulmala, J. M. Tomlinson, D. R. Collins, M. J. Cubison, E. J. Dunlea, J. A. Huffman, T. B. Onasch, M. R. Alfarra, P. I. Williams, K. Bower, Y. Kondo, J. Schneider, F. Drewnick, S. Borrmann, S. Weimer, K. Demerjian, D. Salcedo, L. Cottrell, R. Griffin, A. Takami, T. Miyoshi, S. Hatakeyama, A. Shimono, J. Y. Sun, Y. M. Zhang, K. Dzepina, J. R. Kimmel, D. Sueper, J. T. Jayne, S. C. Herndon, A. M. Trimborn, L. R. Williams, E. C. Wood, A. M. Middlebrook, C. E. Kolb, U. Baltensperger and D. R. Worsnop, Evolution of organic aerosols in the atmosphere, Science, 2009, 326, 1525–1529 CrossRef CAS PubMed
.
- B. N. Murphy, D. Sonntag, K. M. Seltzer, H. O. T. Pye, C. Allen, E. Murray, C. Toro, D. R. Gentner, C. Huang, S. Jathar, L. Li, A. A. May and A. L. Robinson, Reactive organic carbon air emissions from mobile sources in the United States, Atmos. Chem. Phys., 2023, 23, 13469–13483 CrossRef CAS PubMed
.
- A. L. Robinson, N. M. Donahue and W. F. Rogge, Photochemical oxidation and changes in molecular composition of organic aerosol in the regional context, J. Geophys. Res.: Atmos., 2006, 111, 3302 Search PubMed
.
- J. F. Pankow, An absorption model of the gas/aerosol partitioning involved in the formation of secondary organic aerosol, Atmos. Environ., 1994, 28, 189–193 CrossRef CAS
.
- J. D. Blando and B. J. Turpin, Secondary organic aerosol formation in cloud and fog droplets: a literature evaluation of plausibility, Atmos. Environ., 2000, 34, 1623–1632 CrossRef CAS
.
- Q. Zhang, J. L. Jimenez, M. R. Canagaratna, J. D. Allan, H. Coe, I. Ulbrich, M. R. Alfarra, A. Takami, A. M. Middlebrook, Y. L. Sun, K. Dzepina, E. Dunlea, K. Docherty, P. F. DeCarlo, D. Salcedo, T. Onasch, J. T. Jayne, T. Miyoshi, A. Shimono, S. Hatakeyama, N. Takegawa, Y. Kondo, J. Schneider, F. Drewnick, S. Borrmann, S. Weimer, K. Demerjian, P. Williams, K. Bower, R. Bahreini, L. Cottrell, R. J. Griffin, J. Rautiainen, J. Y. Sun, Y. M. Zhang and D. R. Worsnop, Ubiquity and dominance of oxygenated species in organic aerosols in anthropogenically-influenced Northern Hemisphere midlatitudes, Geophys. Res. Lett., 2007, 34(13) DOI:10.1029/2007GL029979
.
- J. Li, Z. Han, J. Wu, J. Tao, J. Li, Y. Sun, L. Liang, M. Liang and Q. Wang, Secondary organic aerosol formation and source contributions over east China in summertime, Environ. Pollut., 2022, 306, 119383 CrossRef CAS PubMed
.
- J. Gao, L. P. Qiao, S. R. Lou, R. S. Yan, M. Zhou, Y. C. Liu, J. L. Feng and D. D. Huang, Secondary Aerosol Formation in Urban Shanghai: Insights into the Roles of Photochemical Oxidation and Aqueous-Phase Reaction, Huanjing Kexue, 2019, 40, 2510–2518 Search PubMed
.
- B. A. Nault, D. S. Jo, B. C. McDonald, P. Campuzano-Jost, D. A. Day, W. Hu, J. C. Schroder, J. Allan, D. R. Blake, M. R. Canagaratna, H. Coe, M. M. Coggon, P. F. Decarlo, G. S. Diskin, R. Dunmore, F. Flocke, A. Fried, J. B. Gilman, G. Gkatzelis, J. F. Hamilton, T. F. Hanisco, P. L. Hayes, D. K. Henze, A. Hodzic, J. Hopkins, M. Hu, L. G. Huey, B. T. Jobson, W. C. Kuster, A. Lewis, M. Li, J. Liao, M. O. Nawaz, I. B. Pollack, J. Peischl, B. Rappenglück, C. E. Reeves, D. Richter, J. M. Roberts, T. B. Ryerson, M. Shao, J. M. Sommers, J. Walega, C. Warneke, P. Weibring, G. M. Wolfe, D. E. Young, B. Yuan, Q. Zhang, J. A. De Gouw and J. L. Jimenez, Secondary organic aerosols from anthropogenic volatile organic compounds contribute substantially to air pollution mortality, Atmos. Chem. Phys., 2021, 21, 11201–11224 CrossRef CAS
.
- N. M. Donahue, A. L. Robinson and S. N. Pandis, Atmospheric organic particulate matter: From smoke to secondary organic aerosol, Atmos. Environ., 2009, 43, 94–106 CrossRef CAS
.
-
EPA, Compilation of Air Pollutant Emission Factors, AP-42, US Environmental Protection Agency, Research Triangle Park, NC, 5th edn, 1995 Search PubMed
.
- N. M. Donahue, A. L. Robinson, C. O. Stanier and S. N. Pandis, Coupled partitioning, dilution, and chemical aging of semivolatile organics, Environ. Sci. Technol., 2006, 40, 2635–2643 CrossRef CAS PubMed
.
- K. M. Wagstrom, S. N. Pandis, G. Yarwood, G. M. Wilson and R. E. Morris, Development and application of a computationally efficient particulate matter apportionment algorithm in a three-dimensional chemical transport model, Atmos. Environ., 2008, 42, 5650–5659 CrossRef CAS
.
- B. Koo, G. M. Wilson, R. E. Morris, A. M. Dunker and G. Yarwood, Comparison of source apportionment and sensitivity analysis in a particulate matter air quality model, Environ. Sci. Technol., 2009, 43, 6669–6675 CrossRef CAS PubMed
.
- X. Chang, B. Zhao, H. Zheng, S. Wang, S. Cai, F. Guo, P. Gui, G. Huang, D. Wu, L. Han, J. Xing, H. Man, R. Hu, C. Liang, Q. Xu, X. Qiu, D. Ding, K. Liu, R. Han, A. L. Robinson and N. M. Donahue, Full-volatility emission framework corrects missing and underestimated secondary organic aerosol sources, One Earth, 2022, 5, 403–412 CrossRef
.
- J. An, C. Huang, D. Huang, M. Qin, H. Liu, R. Yan, L. Qiao, M. Zhou, Y. Li, S. Zhu, Q. Wang and H. Wang, Sources of organic aerosols in eastern China: A modeling study with high-resolution intermediate-volatility and semivolatile organic compound emissions, Atmos. Chem. Phys., 2023, 23, 323–344 CrossRef CAS
.
- L. Wu, X. Wang, S. Lu, M. Shao and Z. Ling, Emission inventory of semi-volatile and intermediate-volatility organic compounds and their effects on secondary organic aerosol over the Pearl River Delta region, Atmos. Chem. Phys., 2019, 19, 8141–8161 CrossRef CAS
.
- A. M. Dunker, B. Koo and G. Yarwood, Source apportionment of organic aerosol and ozone and the effects of emission reductions, Atmos. Environ., 2019, 198, 89–101 CrossRef CAS
.
- A. Clappier, C. A. Belis, D. Pernigotti and P. Thunis, Source apportionment and sensitivity analysis: Two methodologies with two different purposes, Geosci. Model Dev., 2017, 10, 4245–4256 CrossRef CAS
.
-
G. Yarwood, R. E. Morris and G. M. Wilson, Particulate Matter Source Apportionment Technology (PSAT) in the CAMx Photochemical Grid Model, Air Pollution Modeling and its Application XVII, 2007, pp. 478–492 Search PubMed
.
- P. Thunis, A. Clappier, L. Tarrason, C. Cuvelier, A. Monteiro, E. Pisoni, J. Wesseling, C. A. Belis, G. Pirovano, S. Janssen, C. Guerreiro and E. Peduzzi, Source apportionment to support air quality planning: Strengths and weaknesses of existing approaches, Environ. Int., 2019, 130, 104825 CrossRef CAS PubMed
.
- J. Odum, T. Hoffmann, F. Bowman, D. Collins, R. Flagan and J. Seinfeld, Gas/Particle Partitioning and Secondary Organic Aerosol Yields, Environ. Sci. Technol., 1996, 30, 2580–2585 CrossRef CAS
.
-
Ramboll, User Guide Comprehensive Air Quality Model with Extensions, Version 7.2 Search PubMed
.
- F. S. Binkowski and S. J. Roselle, Models-3 Community Multiscale Air Quality (CMAQ) model aerosol component 1. Model description, J. Geophys. Res.: Atmos., 2003, 108, 4183 CrossRef
.
- A. L. Robinson, N. M. Donahue, M. K. Shrivastava, E. A. Weitkamp, A. M. Sage, A. P. Grieshop, T. E. Lane, J. R. Pierce and S. N. Pandis, Rethinking organic aerosols: Semivolatile emissions and photochemical aging, Science, 2007, 315, 1259–1262 CrossRef CAS PubMed
.
- K. Wyat Appel, J. O. Bash, K. M. Fahey, K. M. Foley, R. C. Gilliam, C. Hogrefe, W. T. Hutzell, D. Kang, R. Mathur, B. N. Murphy, S. L. Napelenok, C. G. Nolte, J. E. Pleim, G. A. Pouliot, H. O. T. Pye, L. Ran, S. J. Roselle, G. Sarwar, D. B. Schwede, F. I. Sidi, T. L. Spero and D. C. Wong, The Community Multiscale Air Quality (CMAQ) model versions 5.3 and 5.3.1: System updates and evaluation, Geosci. Model Dev., 2021, 14, 2867–2897 CrossRef PubMed
.
- L. Huang, H. Liu, G. Yarwood, G. Wilson, J. Tao, Z. Han, D. Ji, Y. Wang and L. Li, Modeling of secondary organic aerosols (SOA) based on two commonly used air quality models in China: Consistent S/IVOCs contribution but large differences in SOA aging, Sci. Total Environ., 2023, 903, 166162 CrossRef CAS PubMed
.
- H. Y. Park, S. C. Hong, J. B. Lee and S. Y. Cho, Modeling of Organic Aerosol in Seoul Using CMAQ with AERO7, Atmosphere, 2023, 14, 874 CrossRef CAS
.
- H. O. T. Pye, R. W. Pinder, I. R. Piletic, Y. Xie, S. L. Capps, Y.-H. Lin, J. D. Surratt, Z. Zhang, A. Gold, D. J. Luecken, W. T. Hutzell, M. Jaoui, J. H. Offenberg, T. E. Kleindienst, M. Lewandowski and E. O. Edney, Epoxide Pathways Improve Model Predictions of Isoprene Markers and Reveal Key Role of Acidity in Aerosol Formation, Environ. Sci. Technol., 2013, 47, 11056–11064 CrossRef CAS PubMed
.
- H. O. T. Pye, D. J. Luecken, L. Xu, C. M. Boyd, N. L. Ng, K. R. Baker, B. R. Ayres, J. O. Bash, K. Baumann, W. P. L. Carter, E. Edgerton, J. L. Fry, W. T. Hutzell, D. B. Schwede and P. B. Shepson, Modeling the Current and Future Roles of Particulate Organic Nitrates in the Southeastern United States, Environ. Sci. Technol., 2015, 49, 14195–14203 CrossRef CAS PubMed
.
- B. N. Murphy, M. C. Woody, J. L. Jimenez, A. M. G. Carlton, P. L. Hayes, S. Liu, N. L. Ng, L. M. Russell, A. Setyan, L. Xu, J. Young, R. A. Zaveri, Q. Zhang and H. O. T. Pye, Semivolatile POA and parameterized total combustion SOA in CMAQv5.2: Impacts on source strength and partitioning, Atmos. Chem. Phys., 2017, 17, 11107–11133 CrossRef CAS PubMed
.
- H. O. T. Pye, B. N. Murphy, L. Xu, N. L. Ng, A. G. Carlton, H. Guo, R. Weber, P. Vasilakos, K. W. Appel, S. H. Budisulistiorini, J. D. Surratt, A. Nenes, W. Hu, J. L. Jimenez, G. Isaacman-VanWertz, P. K. Misztal and A. H. Goldstein, On the implications of aerosol liquid water and phase separation for organic aerosol mass, Atmos. Chem. Phys., 2017, 17, 343–369 CrossRef CAS PubMed
.
- S. A. Epstein, I. Riipinen and N. M. Donahue, A semiempirical correlation between enthalpy of vaporization and saturation concentration for organic aerosol, Environ. Sci. Technol., 2010, 44, 743–748 CrossRef CAS PubMed
.
- H. Zheng, X. Chang, S. Wang, S. Li, D. Yin, B. Zhao, G. Huang, L. Huang, Y. Jiang, Z. Dong, Y. He, C. Huang and J. Xing, Trends of Full-Volatility Organic Emissions in China from 2005 to 2019 and Their Organic Aerosol Formation Potentials, Environ. Sci. Technol. Lett., 2023, 10, 137–144 CrossRef CAS
.
- L. Huang, Q. Wang, Y. Wang, C. Emery, A. Zhu, Y. Zhu, S. Yin, G. Yarwood, K. Zhang and L. Li, Simulation of secondary organic aerosol over the Yangtze River Delta region: The impacts from the emissions of intermediate volatility organic compounds and the SOA modeling framework, Atmos. Environ., 2021, 246, 118079 CrossRef CAS
.
- Y. Zhao, N. T. Nguyen, A. A. Presto, C. J. Hennigan, A. A. May and A. L. Robinson, Intermediate Volatility Organic Compound Emissions from On-Road Diesel Vehicles: Chemical Composition, Emission Factors, and Estimated Secondary Organic Aerosol Production, Environ. Sci. Technol., 2015, 49, 11516–11526 CrossRef CAS PubMed
.
- Y. Zhao, C. J. Hennigan, A. A. May, D. S. Tkacik, J. A. De Gouw, J. B. Gilman, W. C. Kuster, A. Borbon and A. L. Robinson, Intermediate-volatility organic compounds: A large source of secondary organic aerosol, Environ. Sci. Technol., 2014, 48, 13743–13750 CrossRef CAS PubMed
.
-
G. Yarwood, J. Jung, G. Whitten, G. Heo, J. Mellberg and M. Estes, Updates to the Carbon Bond Mechanism for Version 6 (CB6) Search PubMed
.
- A. Nenes, S. N. Pandis and C. Pilinis, ISORROPIA: A new thermodynamic equilibrium model for multiphase multicomponent inorganic aerosols, Aquat. Geochem., 1998, 4, 123–152 CrossRef CAS
.
- L. Zhang, J. R. Brook and R. Vet, A revised parameterization for gaseous dry deposition in air-quality models, Atmos. Chem. Phys., 2003, 3, 2067–2082 CrossRef CAS
.
- W. C. Skamarock and J. B. Klemp, A time-split nonhydrostatic atmospheric model for weather research and forecasting applications, J. Comput. Phys., 2008, 227, 3465–3485 CrossRef
.
- L. Wu, Z. Ling, H. Liu, M. Shao, S. Lu, L. Wu and X. Wang, A gridded emission inventory of semi-volatile and intermediate volatility organic compounds in China, Sci. Total Environ., 2021, 761, 143295 CrossRef CAS PubMed
.
- J. J. Cao, S. C. Lee, K. F. Ho, S. C. Zou, K. Fung, Y. Li, J. G. Watson and J. C. Chow, Spatial and seasonal variations of atmospheric organic carbon and elemental carbon in Pearl River Delta Region, China, Atmos. Environ., 2004, 38, 4447–4456 CrossRef CAS
.
- D. D. Huang, S. Zhu, J. An, Q. Wang, L. Qiao, M. Zhou, X. He, Y. Ma, Y. Sun, C. Huang, J. Z. Yu and Q. Zhang, Comparative Assessment of Cooking Emission Contributions to Urban Organic Aerosol Using Online Molecular Tracers and Aerosol Mass Spectrometry Measurements, Environ. Sci. Technol., 2021, 55, 14526–14535 CrossRef CAS PubMed
.
- Z. Li, Y. Sun, Q. Wang, J. Xin, J. Sun, L. Lei, J. Li, P. Fu and Z. Wang, Nitrate and secondary organic aerosol dominated particle light extinction in Beijing due to clean air action, Atmos. Environ., 2022, 269, 118833 CrossRef CAS
.
- Q. Chen, R. Miao, G. Geng, M. Shrivastava, X. Dao, B. Xu, J. Sun, X. Zhang, M. Liu, G. Tang, Q. Tang, H. Hu, R. J. Huang, H. Wang, Y. Zheng, Y. Qin, S. Guo, M. Hu and T. Zhu, Widespread 2013-2020 decreases and reduction challenges of organic aerosol in China, Nat. Commun., 2024, 15, 1–8 Search PubMed
.
- L. Huang, Y. Zhu, H. Zhai, S. Xue, T. Zhu, Y. Shao, Z. Liu, C. Emery, G. Yarwood, Y. Wang, J. Fu, K. Zhang and L. Li, Recommendations on benchmarks for numerical air quality model applications in China - Part 1: PM2.5 and chemical species, Atmos. Chem. Phys., 2021, 21, 2725–2743 CrossRef CAS
.
- R. Miao, Q. Chen, M. Shrivastava, Y. Chen, L. Zhang, J. Hu, Y. Zheng and K. Liao, Process-based and observation-constrained SOA simulations in China: The role of semivolatile and intermediate-volatility organic compounds and OH levels, Atmos. Chem. Phys., 2021, 21, 16183–16201 CrossRef CAS
.
- Y. Ren, G. Wang, J. Li, C. Wu, C. Cao, J. Wang, L. Zhang, F. Meng and H. Li, Seasonal variation and size distribution of biogenic secondary organic aerosols at urban and continental background sites of China, J. Environ. Sci., 2018, 71, 32–44 CrossRef CAS PubMed
.
- F. Jiang, Q. Liu, X. Huang, T. Wang, B. Zhuang and M. Xie, Regional modeling of secondary organic aerosol over China using WRF/Chem, J. Aerosol Sci., 2012, 43, 57–73 CrossRef CAS
.
- M. Qin, X. Wang, Y. Hu, X. Ding, Y. Song, M. Li, P. Vasilakos, A. Nenes and A. G. Russell, Simulating Biogenic Secondary Organic Aerosol During Summertime in China, J. Geophys. Res.: Atmos., 2018, 123, 11100–11119 Search PubMed
.
- L. Xu, H. O. T. Pye, J. He, Y. Chen, B. N. Murphy and N. L. Ng, Experimental and model estimates of the contributions from biogenic monoterpenes and sesquiterpenes to secondary organic aerosol in the southeastern United States, Atmos. Chem. Phys., 2018, 18, 12613–12637 CrossRef CAS PubMed
.
- M. Sarrafzadeh, J. Wildt, I. Pullinen, M. Springer, E. Kleist, R. Tillmann, S. H. Schmitt, C. Wu, T. F. Mentel, D. Zhao, D. R. Hastie and A. Kiendler-Scharr, Impact of NOx and OH on secondary organic aerosol formation from β-pinene photooxidation, Atmos. Chem. Phys., 2016, 16, 11237–11248 CrossRef CAS
.
- J. Wildt, T. F. Mentel, A. Kiendler-Scharr, T. Hoffmann, S. Andres, M. Ehn, E. Kleist, P. Müsgen, F. Rohrer, Y. Rudich, M. Springer, R. Tillmann and A. Wahner, Suppression of new particle formation from monoterpene oxidation by NO x, Atmos. Chem. Phys., 2014, 14, 2789–2804 CrossRef
.
- A. Hodzic, S. Madronich, P. S. Kasibhatla, G. Tyndall, B. Aumont, J. L. Jimenez, J. Lee-Taylor and J. Orlando, Organic photolysis reactions in tropospheric aerosols: Effect on secondary organic aerosol formation and lifetime, Atmos. Chem. Phys., 2015, 15, 9253–9269 CrossRef CAS
.
- A. Hodzic, P. S. Kasibhatla, D. S. Jo, C. D. Cappa, J. L. Jimenez, S. Madronich and R. J. Park, Rethinking the global secondary organic aerosol (SOA) budget: Stronger production, faster removal, shorter lifetime, Atmos. Chem. Phys., 2016, 16, 7917–7941 CrossRef CAS
.
- M. A. Zawadowicz, B. H. Lee, M. Shrivastava, A. Zelenyuk, R. A. Zaveri, C. Flynn, J. A. Thornton and J. E. Shilling, Photolysis Controls Atmospheric Budgets of Biogenic Secondary Organic Aerosol, Environ. Sci. Technol., 2020, 54, 3861–3870 CrossRef CAS PubMed
.
- Z. Ling, L. Wu, Y. Wang, M. Shao, X. Wang and W. Huang, Roles of semivolatile and intermediate-volatility organic compounds in secondary organic aerosol formation and its implication: A review, J. Environ. Sci., 2022, 114, 259–285 CrossRef CAS PubMed
.
- L. Li, J. An, M. Zhou, L. Qiao, S. Zhu, R. Yan, C. G. Ooi, H. Wang, C. Huang, L. Huang, S. Tao, J. Yu, A. Chan, Y. Wang, J. Feng and C. Chen, An Integrated Source Apportionment Methodology and Its Application over the Yangtze River Delta Region, China, Environ. Sci. Technol., 2018, 52, 14216–14227 CrossRef PubMed
.
- X. Liu, X. Bai, H. Tian, K. Wang, S. Hua, H. Liu, S. Liu, B. Wu, Y. Wu, W. Liu, L. Luo, Y. Wang, J. Hao, S. Lin, S. Zhao and K. Zhang, Fine particulate matter pollution in North China: Seasonal-spatial variations, source apportionment, sector and regional transport contributions, Environ. Res., 2020, 184, 109368 CrossRef CAS PubMed
.
- X. Lu, Y. Chen, Y. Huang, C. Lin, Z. Li, J. C. H. Fung and A. K. H. Lau, Differences in concentration and source apportionment of PM2.5 between 2006 and 2015 over the PRD region in southern China, Sci. Total Environ., 2019, 673, 708–718 CrossRef CAS PubMed
.
- Y. Wang, L. Li, C. Chen, C. Huang, H. Huang, J. Feng, S. Wang, H. Wang, G. Zhang, M. Zhou, P. Cheng, M. Wu, G. Sheng, J. Fu, Y. Hu, A. G. Russell and A. Wumaer, Source apportionment of fine particulate matter during autumn haze episodes in Shanghai, China, J. Geophys. Res.: Atmos., 2014, 119, 1903–1914 CrossRef CAS
.
- G. A. Grell, S. E. Peckham, R. Schmitz, S. A. McKeen, G. Frost, W. C. Skamarock and B. Eder, Fully coupled “online” chemistry within the WRF model, Atmos. Environ., 2005, 39, 6957–6975 CrossRef CAS
.
- H. Schmidt, C. Derognat, R. Vautard and M. Beekmann, A comparison of simulated and observed ozone mixing ratios for the summer of 1998 in Western Europe, Atmos. Environ., 2001, 35, 6277–6297 CrossRef CAS
.
|
This journal is © The Royal Society of Chemistry 2024 |
Click here to see how this site uses Cookies. View our privacy policy here.