Application of established computational techniques to identify potential SARS-CoV-2 Nsp14-MTase inhibitors in low data regimes†
Received
12th January 2024
, Accepted 13th May 2024
First published on 20th May 2024
Abstract
The COVID-19 pandemic, caused by the SARS-CoV-2 virus, has led to significant global morbidity and mortality. A crucial viral protein, the non-structural protein 14 (nsp14), catalyzes the methylation of viral RNA and plays a critical role in viral genome replication and transcription. Due to the low mutation rate in the nsp region among various SARS-CoV-2 variants, nsp14 has emerged as a promising therapeutic target. However, discovering potential inhibitors remains a challenge. In this work, we introduce a computational pipeline for the rapid and efficient identification of potential nsp14 inhibitors by leveraging virtual screening and the NCI open compound collection, which contains 250
000 freely available molecules for researchers worldwide. The introduced pipeline provides a cost-effective and efficient approach for early-stage drug discovery by allowing researchers to evaluate promising molecules without incurring synthesis expenses. Our pipeline successfully identified seven candidates that inhibit the MTase activity of nsp14. Among these, one compound, NSC62033, demonstrated strong binding affinity to nsp14, exhibiting a dissociation constant of 427 ± 84 nM. In addition, we gained new insights into the structure and function of this protein through molecular dynamics simulations. Furthermore, our molecular dynamics simulations suggest potential new conformational states of the protein, with residues Phe367, Tyr368, and Gln354 in the binding pocket potentially playing a role in stabilizing interactions with novel ligands, though further validation is required. Our findings also indicate that metal coordination complexes may be important for the function of the binding pocket. Lastly, we present the solved crystal structure of the nsp14-MTase complexed with SS148 (PDB:8BWU), a potent inhibitor of methyltransferase activity at the nanomolar level (IC50 value of 70 ± 6 nM).
1 Introduction
The COVID-19 pandemic, caused by the Severe Acute Respiratory Syndrome Coronavirus 2 (SARS-CoV-2), has had a profound impact on global health, economies, and daily life. SARS-CoV-2 is a single-stranded, positive-sense RNA virus belonging to lineage B of the genus Beta-coronavirus in the Coronaviridae family.1 Since its emergence in December 2019, it has led to a staggering number of infections and deaths worldwide, overwhelming healthcare systems and prompting unprecedented public health measures.2 While the development of vaccines has been a significant step in combating the virus, the continued emergence of new variants highlights the ongoing need for effective antiviral treatments. A significant area of research focus has been the SARS-CoV-2 main protease (Mpro),3,4 which is pivotal in the virus's replication cycle and thus an appealing target for antiviral drug development. A wide array of efforts, including innovative crowdfunding initiatives,5,6 have aimed at discovering potent Mpro inhibitors. These efforts aim to obstruct the virus's ability to replicate, which could mitigate the severity of infections and play a vital role in informing future pandemic management strategies. Nonetheless, the pursuit of alternative viral protein targets is crucial for broadening the spectrum of antiviral strategies and improving the overall effectiveness of treatments.7
The SARS-CoV-2 genome is notably large, containing nearly 30
000 base pairs and encoding 16 nonstructural proteins (nsps) that play vital roles in viral replication and transcription.8 Because of this, nsps represent potential targets for therapeutics, and the availability of their structural data has facilitated the rapid development of antivirals.9 One such target is non-structural protein 14 (nsp14), which has dual functions as an exonuclease (ExoN) and a methyltransferase (MTase). The ExoN function ensures replication fidelity through 3′–5′ proofreading, while the MTase function catalyzes N7-methylation of the guanosine triphosphate cap of the viral RNA, which is crucial for its stability and function.10,11 Due to its low mutation rate among different SARS-CoV-2 variants when compared to other viral proteins such as the spike protein,12 nsp14 is hypothesized to be essential for the function of the virus, and therefore it has emerged as a promising therapeutic target. This persistence increases the likelihood that inhibitors targeting nsp14 will remain effective against a broader range of evolving viral strains. Additionally, the amino acid sequence of SARS-CoV-2 nsp14 shows high homology with nsp14 from other coronaviruses, including SARS-CoV, MERS-CoV, and common human coronaviruses such as OC43, HKU1, 229E, and NL63.12 This sequence conservation suggests that inhibitors targeting nsp14 in SARS-CoV-2 could potentially also inhibit nsp14 MTase activity in other coronaviruses, which may have implications for the development of broad-spectrum antiviral drugs.13
In this study, we developed a computational pipeline for rapid and efficient identification of potential nsp14 inhibitors. It is of interest to note that prior approaches targeting this protein have typically combined synthesis with docking strategies to refine and enhance the potency of existing inhibitors.14,15 In contrast, our approach exploits the power of virtual screening across various levels of accuracy, alongside the use of the NCI open compound collection16,17—a database encompassing over 250
000 molecules freely available to researchers worldwide. For an overview of the property distributions within this collection, refer to Fig. S10.† This strategy allowed us to identify promising molecules without incurring the significant expense and time usually required for synthesis. This cost-effective and efficient strategy proves particularly valuable for early-stage hit identification, in cases where resources are constrained and time is of the essence. We utilized molecular docking18,19 and Molecular Mechanics Generalized Born Surface Area (MM/GBSA) calculations20,21 to gauge the strength of interaction between potential inhibitors and nsp14. Through these computational methodologies, we successfully identified a novel inhibitor for nsp14, with activity in the micromolar range, labelled NSC620333 (IC50 value of 5.3 ± 1.0 μM), which was confirmed by orthogonal in vitro and in vivo assays. This inhibitor was unearthed through computational screening of the NCI open compound collection, with only the top 40 compounds undergoing experimental testing, highlighting the efficiency of our pipeline. Furthermore, to deepen our understanding of the protein structure and function, we conducted molecular dynamics (MD) simulations22–24 exceeding 5 microseconds. These simulations unveiled previously unknown conformational states of nsp14, offering valuable insights into its overall function. Within the MTase lateral cavity, we identified flexible residues—specifically Phe367, Tyr368, and Gln354—that form stabilizing interactions with potential ligands. Our simulations also revealed that the two alpha-helices of the MTase domain undergo a significant shift/bend, corresponding to the opening and closing of the lateral cavity. Importantly, these conformational shifts were not observed in the absence of metal coordination complexes, emphasizing their crucial role in stabilizing interactions. Our MD simulations also shed light on the interaction of nsp14 with nsp10, a key process in viral replication and transcription. Nsp10 has been demonstrated to stimulate the ExoN and MTase activities of nsp14, making it a critical cofactor for the functionality of the protein.25 In summary, our research showcases an advanced computational pipeline, built upon the robust capabilities of VirtualFlow 2.0,26 specifically the VirtualFlow Unity (VFU) module. This component not only accommodates a wide spectrum of docking programs, callable through a python pipeline, but also allows scoring at diverse levels of precision, thereby facilitating the rapid and proficient detection of prospective nsp14 inhibitors. This is achieved by integrating virtual screening strategies and harnessing the expansive NCI open compound collection. Through this methodology, we discovered NSC620333, a compound exhibiting strong binding affinity to nsp14, and gained fresh insights into the structure and function of the protein. Furthermore, we report the crystal structure of SS148, a potent inhibitor (IC50 value of 70 ± 6 nM) of methyltransferase activity, in complex with the nsp14-MTase domain.27,28 By targeting the nsp14 protein, we aspire to contribute to the development of effective antiviral therapies against SARS-CoV-2 and its variants, and other coronaviruses as well. Our computational pipeline offers a cost-effective and efficient strategy for early-stage drug discovery, potentially providing significant assistance to researchers battling the rapidly mutating virus responsible for COVID-19 and aiding in the response to future disease outbreaks.
2 Results
2.1 Employing molecular docking for identifying nsp14 inhibitors
The widespread use of structure-based drug discovery techniques has proven instrumental in the search for small molecules that can specifically target macromolecules.29 The efficacy of molecular docking and free energy simulation methods is particularly noteworthy in assessing the binding strength between proteins and ligands.30 These methods enabled us to identify and prioritize the most promising candidates, thereby facilitating subsequent experimental testing. Our aim was to identify inhibitors of the SARS-CoV-2 nsp14 MTase activity. To achieve this, we performed a computational screening of the Developmental Therapeutics Program (DTP) open compound collection.16,17 Comprising approximately 250
000 molecules synthesized and tested for potential efficacy against cancer and acquired immunodeficiency syndrome, this collection offers a considerable resource for researchers. Importantly, these molecules are readily accessible for academic researchers, with the possibility to request up to 40 samples on a monthly basis. Despite the broader compatibility of VirtualFlow26 with various docking tools, our study opted for Glide over QuickVina to prioritize accuracy over computational speed, given the manageable size of our compound library.
We employed the Glide31 SP docking program to target the MTase binding site, as depicted in Fig. 1. This approach allowed us to evaluate all the molecules in the ligand library. To enhance the reliability of our findings, we rigorously examined the top 1000 molecules—those displaying the lowest docking scores—using the Glide XP setting to increase precision, ensuring that the highest-potential candidates were selected for further analysis. Subsequently, we selected the top 100 performing molecules for further consideration, factoring in aspects such as diversity (see Methods: Docking V A, compound availability, and docking scores. In an effort to further refine our selection and improve the accuracy of binding prediction, we conducted a Molecular Mechanics Generalized Born Surface Area (MM/GBSA) analysis20,21 (see Methods: MM/GBSA Calculation V B). This step assisted us in narrowing down our list to the top 40 compounds, which we subsequently obtained from the NCI. ESI Tables S2, S8, and Fig. S5† present the selected compounds alongside their corresponding SMILES and structures, as well as their docking scores and Molecular Mechanics Generalized Born Surface Area (MM/GBSA) binding affinities. Notably, NSC620333 stood out, showcasing the most promising results, as demonstrated by its lowest docking and MM/GBSA scores. In Fig. 2(D), we provide the docked poses and 2D interaction diagrams of the experimentally validated inhibitor NSC620333. These 2D interaction diagrams highlight the fact that the majority of residues in the binding pocket are hydrophobic or non-polar. Detailed information regarding the docking procedure and protein preparation can be found in the Methods section (see Methods: Docking V A).
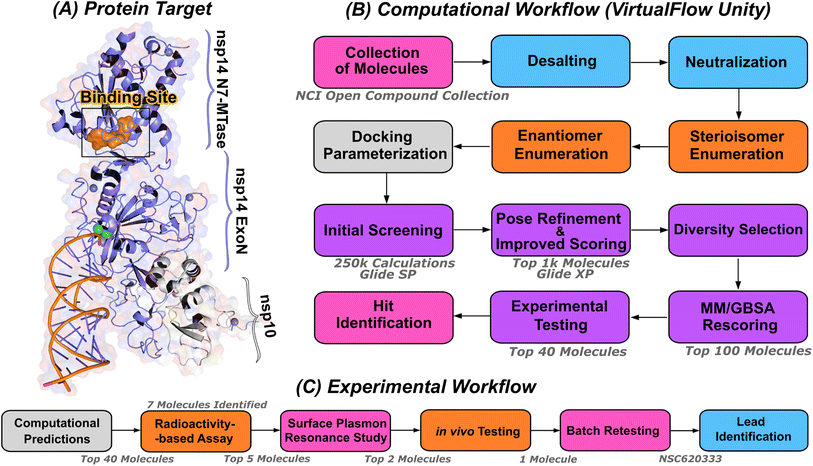 |
| Fig. 1 Computational pipeline for identification of lead compounds. (A) Depiction of the SARS-CoV-2 nsp14-MTase protein target and computational workflow. The protein target (PDB:7N0B), in complex with nsp10, is employed for the modeling process. The highlighted binding site (indicated in orange) within the MTase domain acts as the docking site for molecular interactions. (B) The computational workflow powered by VirtualFlow Unity (VFU), which processes SMILES strings from the NCI open compound collections as input. The procedure includes desalting, neutralizing, and generating stereoisomers of the input molecule. Subsequently, all processed molecules from the database undergo docking onto the binding site using Glide-SP. The output docked compounds are ranked based on their docking scores, with the top 1000 subjected to a more precise scoring algorithm, Glide-XP. A diverse subset of 100 molecules is chosen for an advanced binding energy estimation using Molecular Mechanics Generalized Born Surface Area (MM/GBSA). The top 40 of these compounds, ranked by their binding potential, are then selected for experimental evaluation. (C) The selected top-40 compounds are initially assessed using a radioactivity-based assay, yielding seven compounds with promising results. Subsequently, a detailed binding study is performed with the top-5 compounds utilizing surface plasmon resonance. The in vivo evaluation follows, focusing on the top-2 compounds with SARS-CoV-2 infected Calu-3 cells. Ultimately, the compound identified as the most promising is re-tested after a custom synthesis process, leading not only to improved purity, but also confirming the identification of a lead compound. | |
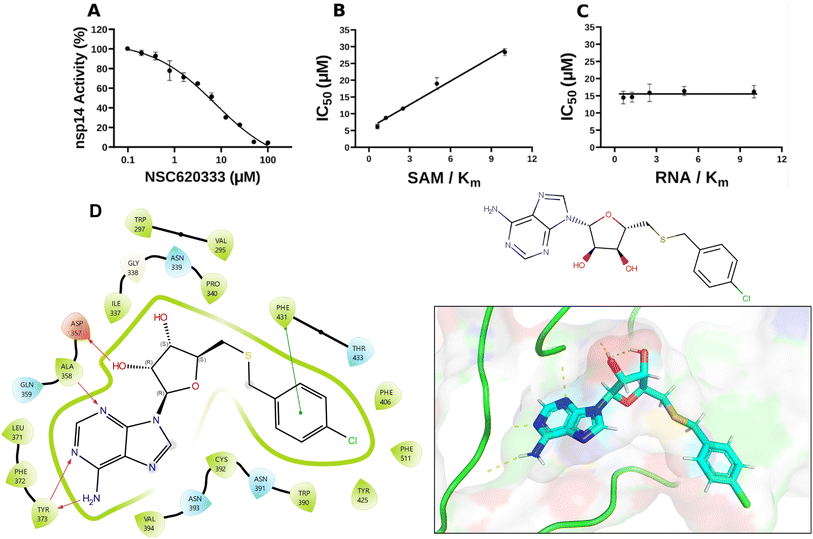 |
| Fig. 2 Inhibition and interaction analysis of NSC620333 with nsp14 MTase. (A) The IC50 value was determined for NSC620333 at Km of both SAM and RNA substrates (5.3 ± 1.0 μM, hill slope: −0.9). The mechanism of action (MOA) of NSC620333 was also determined by IC50 determination for NSC620333 at (B) varying concentrations of SAM (from 0.15 to 2.5 μM) at fixed concentration of RNA substrate (0.25 μM, 5x Km) and (C) varying concentrations of RNA substrate (from 31 to 500 nM) at fixed concentration of SAM (1.25 μM, 5x Km). All values are presented as mean ± standard deviation of three independent experiments (n = 3). NSC620333 is a SAM competitive nsp14 inhibitor (B), and noncompetitive with respect to RNA substrate (C). (D) Structural diagrams and 2D interaction plots show NSC620333 interacting within the lateral cavity of nsp14, as evidenced by docking studies within the PDB structure 7N0B. | |
2.2 Experimental evaluation of lead compounds
In this study, we harnessed both the precision and accuracy of a state-of-the-art high-throughput radioactivity-based assay,27 to experimentally test the MTase activity of nsp14 under the influence of our selected compounds. Out of the 40 assessed compounds, seven manifested inhibitory properties (Table 1). Among these, NSC76988 and NSC620333 emerged as particularly promising candidates, due to their relatively low IC50 values 6 and 5 μM, respectively, suggestive of a potent inhibitory effect. To further substantiate these findings and confirm the binding of these inhibitors to nsp14, we employed surface plasmon resonance (SPR).32 This technique was leveraged to investigate the binding interactions of the top five compounds from the radioactivity-based assay. Notably, none of the compounds other than NSC620333 exhibited reliable SPR measurements. For reference, we have included the corresponding sensorgrams of the four compounds with inconclusive results in Fig. S8.†Fig. 2 provides detailed insight into these molecular interactions. It presents the 2D structural diagrams of the top experimentally validated compounds that inhibit MTase activity. Alongside these are the 2D interaction diagrams derived from docking studies, and 3D poses showing how these compounds fit within the lateral cavity of nsp14. Interestingly, of these five inhibitors, NSC620333 had the lowest KD value of 544 ± 22 nM (cf.Fig. 2 (top) and S1†). This observation points to the potential of NSC620333 as an effective nsp14 inhibitor. Furthermore, the batch of NSC620333 used in re-testing was obtained through custom synthesis, guaranteeing enhanced purity for the confirmation of our results, as illustrated in ESI Fig. S3 and S6.† We note that although NSC620333 exhibited the strongest docking scores across all three methods (Glide SP, XP, and MM/GBSA), we observed that the docking scores do not strictly correlate with the experimentally observed inhibition of the protein (see Fig. S11†).
Table 1 High-throughput screening results of the top 40 compounds obtained for the Inhibition of nsp14 Methyltransferase (MTase) Activity. This table summarizes the inhibitory effects of the top 40 compounds on nsp14 MTase activity as assessed by high-throughput screening. For each compound, data from two replicate experiments are included, revealing the inhibition percentages at a compound concentration of 100 μM. The IC50 values, indicating the concentration at which half-maximal inhibition is achieved, are reported for the most promising compounds. The table also provides the observed quenching effects of each compound. Additionally, it includes SPR Kd for five of the top seven compounds that exhibited inhibition of MTase activity in the radioactivity-based assay. The designation “ND” is used to indicate that a value has not been determined, while “inconclusive” specifies instances where experiments were conducted but yielded measurements that were not reliable
Compound |
nsp14 inhibition % (100 μM) |
IC50 (μM) |
Quenching effect |
SPR Kd (nM) |
Exp 1 |
Exp 2 |
NSC620333 |
100 |
99 |
5 |
23 |
427 ± 84 |
NSC76988 |
100 |
99 |
6 |
8 |
Inconclusive |
NSC4348 |
73 |
61 |
34 |
9 |
Inconclusive |
NSC77131 |
99 |
99 |
9 |
52 |
Inconclusive |
NSC2269 |
64 |
60 |
27 |
1 |
Inconclusive |
NSC400718 |
89 |
88 |
38 |
23 |
ND |
NSC34443 |
86 |
81 |
57 |
−7 |
ND |
NSC630814 |
84 |
82 |
ND |
75 |
ND |
NSC102798 |
77 |
74 |
ND |
61 |
ND |
NSC400937 |
73 |
66 |
ND |
70 |
ND |
NSC4624 |
65 |
47 |
ND |
49 |
ND |
NSC255523 |
59 |
33 |
ND |
−7 |
ND |
NSC670682 |
50 |
54 |
ND |
43 |
ND |
NSC99790 |
46 |
36 |
ND |
33 |
ND |
NSC646375 |
26 |
20 |
ND |
1 |
ND |
NSC27605 |
26 |
10 |
ND |
2 |
ND |
NSC107661 |
18 |
7 |
ND |
−2 |
ND |
NSC293892 |
15 |
3 |
ND |
−5 |
ND |
NSC44037 |
15 |
9 |
ND |
8 |
ND |
NSC77680 |
13 |
7 |
ND |
21 |
ND |
NSC80136 |
13 |
2 |
ND |
−3 |
ND |
NSC114010 |
11 |
−3 |
ND |
−2 |
ND |
NSC268226 |
9 |
0 |
ND |
3 |
ND |
NSC163444 |
9 |
5 |
ND |
2 |
ND |
NSC377438 |
8 |
−3 |
ND |
17 |
ND |
NSC131119 |
8 |
0 |
ND |
14 |
ND |
NSC39302 |
7 |
−2 |
ND |
12 |
ND |
NSC317609 |
7 |
1 |
ND |
8 |
ND |
NSC232469 |
7 |
−7 |
ND |
−4 |
ND |
NSC655184 |
7 |
−11 |
ND |
5 |
ND |
NSC92432 |
6 |
−1 |
ND |
8 |
ND |
NSC137050 |
6 |
−1 |
ND |
3 |
ND |
NSC158437 |
5 |
1 |
ND |
15 |
ND |
NSC613624 |
4 |
−1 |
ND |
6 |
ND |
NSC60360 |
4 |
−4 |
ND |
10 |
ND |
NSC330685 |
4 |
2 |
ND |
1 |
ND |
NSC54251 |
4 |
−4 |
ND |
−1 |
ND |
NSC313453 |
3 |
−5 |
ND |
5 |
ND |
NSC132916 |
−1 |
−3 |
ND |
3 |
ND |
In the quest to further understand the specificity of NSC620333, we evaluated its inhibitory activity against a diverse panel of 32 human RNA-, DNA-, and protein-MTases (ESI Table S3†). A critical part of our investigation focused on potential off-target effects within the host to ensure the safety and efficacy of the compounds. Our analyses revealed that NSC620333 inhibited only human RNMT33 (N7 guanosine RNA methyltransferase) and PRMT7 (ref. 34) (protein arginine methyltransferase 7), with IC50 values of 8.6 ± 1.3 and 7 ± 1.5 μM, respectively (ESI Fig. S2†). Notably, RNMT is involved in the process of mRNA capping, which is essential for maintaining mRNA stability, facilitating its export, and ensuring efficient translation.35 On the other hand, PRMT7 participates in the methylation of arginine residues on specific proteins, thereby impacting cellular processes such as gene transcription, DNA repair, and signal transduction.36 Furthermore, to extrapolate our in vitro findings to a physiologically-relevant context, we tested NSC620333 and NSC76988 in an in vivo assay. This experiment was based on a lung epithelial SARS-CoV-2 infection model using Calu-3 cells. Encouragingly, both compounds demonstrated anti-SARS-CoV-2 activity (ESI Fig. S5†), providing supportive evidence for their potential as therapeutic agents. Taken together, the data from all three complementary assays converges towards a common conclusion: NSC620333 stands out as a potential therapeutic candidate against SARS-CoV-2. This compound exhibited a potent inhibitory effect, significant binding to nsp14, favorable selectivity, and in vivo efficacy. These promising attributes warrant further investigations into its potential for clinical application in SARS-CoV-2 treatment. Interestingly, while NSC76988 was pinpointed as a potential inhibitor in our radioactivity-based assay, further SPR assays did not corroborate direct binding S8, presenting an intriguing case that highlights the potential for compounds to exert inhibitory effects through indirect mechanisms.
2.3 MD simulations of nsp14
Structural insights into nsp14 were obtained through molecular dynamics (MD) simulations performed under three different conditions: (i) the protein only, (ii) the protein in complex with NSC620333, and (iii) nsp14 in/out of complex with metal coordination complexes. Our findings are presented as follows.
2.3.1 Protein dynamics.
Investigations into ligand binding within the MTase lateral cavity were conducted via numerous extended molecular dynamics simulations of the nsp14-nsp10 complex, all performed under a consistent thermal condition of 303.15 K (details in Methods: Molecular dynamics simulations V G). Throughout these simulations, we monitored the average motion of residues located within a 5 angstrom radius of the SS148 binding region, as depicted in Fig. 3(A). A thorough evaluation of the complete motion of the associated residues is presented in ESI Fig. S7.† Interestingly, we found that residues Phe367, Tyr368, and Gln354 on average exhibited the most significant movement across all simulations. These residues appear on disordered loops surrounding the pocket, which displayed considerable stabilization when NSC620333 was present in the binding pocket. Specifically, the root-mean-square deviation (RMSD) was calculated for the backbone, using a binding pocket alignment consisting of residues listed in Fig. 2(D), which showed a reduction in movement of Phe367, Tyr368, and Gln354 to 0.25, 0.29, and 0.26 angstroms respectively in the presence of NSC620333. This result is expected and supported by the bound structure of SS148 with nsp14, which shows direct interaction with Phe367, Tyr368, and Ala353. Significantly, we observed a limited number of conformational changes in the nsp10 protein when it was complexed with nsp14 (see Fig. 3(B) (Left)), indicating a high level of interaction stability between the two proteins based on our sampling. Fig. 3(B) (Middle/Right) showcases the key residues involved in this interaction. Specifically, through the MM/GBSA calculations for estimated free energy (see Methods: MM/GBSA calculation), Phe24, Glu11, His85, Thr10, and Val26 emerged as the key residues driving this interaction, each contributing significantly to the delta free energy (see Fig. 3(B) (Right)).
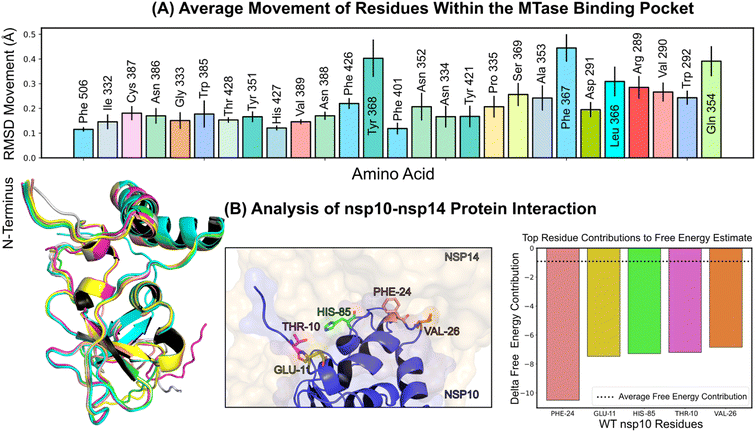 |
| Fig. 3 Protein conformation and interaction dynamics from molecular simulations. (A) Bar charts presenting the mean displacement (expressed in angstroms) of amino acid residues within a 5 angstrom vicinity of the binding pocket, derived from 1 microsecond molecular dynamics simulations conducted at a stable temperature of 303.15 K. The error bars indicate the standard deviation of the mean, based on five distinct simulation runs. (B) (Left) A graphical representation of the most notable conformational shifts in nsp10, as determined through a series of MD-simulations involving nsp10–14, with only minor changes due to the consistent stability of the nsp10–nsp14 interaction. (Middle) Select residues at the nsp10 binding interface making the most significant contribution to the interaction with nsp16. (Right) Breakdown of individual residues of nsp10 with the highest free energy contributions (kcal mol−1) to the overall binding free energy estimation of nsp10–14 interactions using MM/GBSA; the average contribution from a single residue is indicated by a dotted line. | |
2.3.2 Conformational shifts.
To gain a more comprehensive understanding of the conformational dynamics of the nsp14 complex, we conducted an in-depth analysis of the gathered molecular dynamics simulations.22 This process involved amalgamating data from receptor-ligand simulations to scrutinize the specific dynamics of the MTase binding cavity and, in a separate analysis, the overall dynamics of the complex backbone across independent trajectories.37 We employed time-lagged independent component analysis (tICA)38 to project pertinent trajectory features onto a lower-dimensionality landscape, which facilitated clustering and visualization of the sampled metastable states39 (see Methods: tICA 2D projections for details). The features of the binding pocket were defined by pairwise distances between the heavy atoms of the NSC620333 ligand and the backbone α-carbons of 27 crucial residues within the MTase lateral cavity. The analysis of global dynamics similarly employed pairwise distances between α-carbons. The generated projections were clustered using the K-means algorithm,40 resulting in eight states that spanned the sampled landscape and were aligned for comparative visualization. In both instances, the cluster with the highest population (comprising approximately 60% of the frames) closely mirrored the anticipated native state, with one or two additional states constituting the majority of the remaining configurations. The visualization of the states underpinning global dynamics revealed that nsp10 and the exoribonuclease domain of nsp14 remained stable. In contrast, the MTase domain exhibited a bent configuration in a minor proportion of frames. Our comparative analysis predominantly focused on trajectories that included metal ions, which best represent the physiological state of the nsp14/nsp10 complex. To establish a foundational understanding of the intrinsic structural dynamics, we also considered metal-devoid conditions. This dual approach demonstrated consistent overall dynamics across both conditions. However, the inclusion of metal ions distinctly led to a pronounced bending of the main alpha-helix MTase (as depicted in Fig. 4) in a considerably larger fraction of the ion-inclusive trajectories, observed in Clusters 5 and 7 (4.6% vs. approximately 1%). Notably, this bending coincided with the opening of the lateral binding pocket, illustrating the significant structural rearrangements induced by metal ions compared to simulations excluding coordination complexes (see Fig. S13†). These observations highlight the crucial role of metal ions in enhancing the conformational flexibility of the MTase domain and offer vital insights into how coordination complexes modulate protein structure and function for therapeutic targeting.
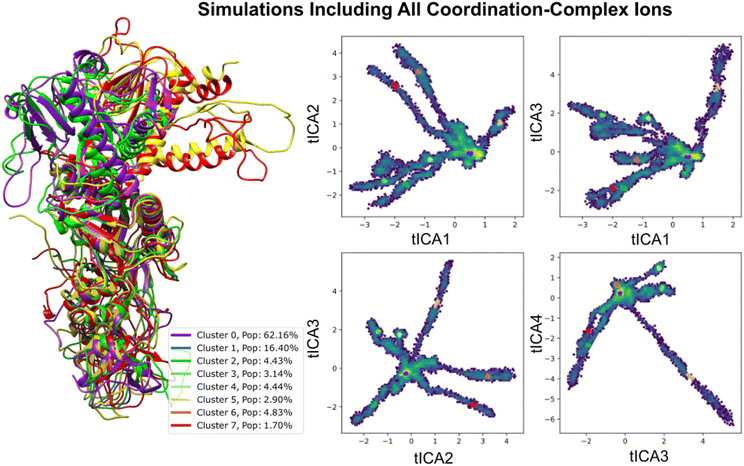 |
| Fig. 4 Analyzing Nsp14-nsp10 conformational variability through tICA projections influenced by coordination complexes. This figure delineates the conformational differences in the nsp10–nsp14 complex under metal-enriched conditions, emphasizing structures from four representative cluster centers with high variance. Trajectories were derived from independent runs between 200 ns–1 μs, focusing on pairwise distances between alternating backbone α-carbons to determine simulation features and sampling more diverse conformations. | |
2.3.3 Structural insights into inhibition.
The application of tICA decomposition on protein-ligand interactions in the simulations identified a variety of binding poses for NSC620333 within the nsp14 MTase binding cavity. From this analysis, eight distinct clusters emerged, which were further refined into three primary states, each visualized in Fig. 5. Detailed examination of these states facilitated the identification of specific interactions that contribute to the increased binding affinity of NSC620333, as supported by Wagner et al.41 Of particular interest were the hydrogen bonds observed to form from ASN538 to the purine group of the ligand, and the favorable lipophilic interactions of the chlorobenzene component of the ligand with the lipophilic subpocket, predominantly in cluster 2 and cluster 6.42 These interactions seem to play a crucial role in establishing a more stable binding configuration. Moreover, the varied poses of NSC620333 within the binding pocket further underscore the flexibility of the nsp14 MTase binding cavity, a factor that could be key in the design of effective therapeutic agents.43 The presence of different binding modes, each with unique interaction profiles, may influence the ligand's functional impact on the protein, potentially offering multiple pathways for pharmacological intervention.44
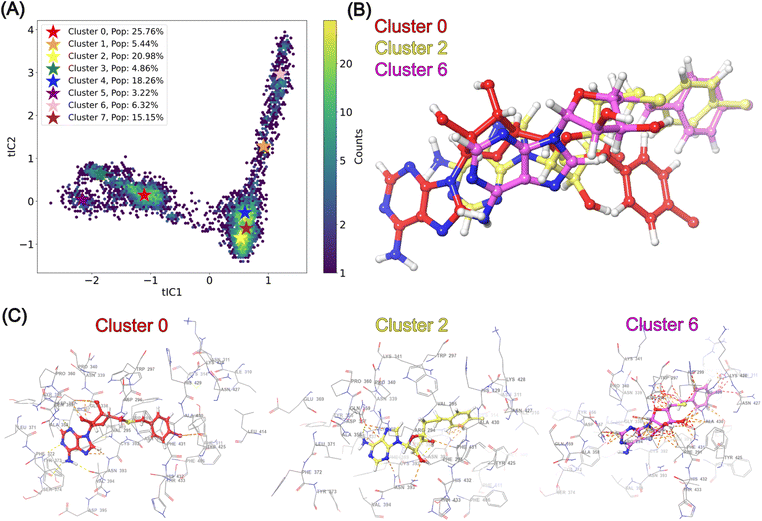 |
| Fig. 5 tICA decomposition and representative structures of NSC620333 alternative binding poses. (A) tICA scatter plot representation of molecular dynamics simulations, color-coded to differentiate eight clusters of NSC620333 binding poses with corresponding population percentages. Cluster centroids are highlighted with stars. (B) Overlay of NSC620333 poses from clusters 0, 2, and 6, shown after alignment based on their corresponding binding pockets to emphasize the variation in ligand orientation. (C) Representative binding site interactions for NSC620333 from clusters 0, 2, and 6, detailing the ligand in various conformations alongside neighboring protein residues. | |
3 Crystal structure of nsp14 MTase in complex with SS148
To uncover the atomic details of the nsp14-SS148 interaction, we performed the crystallographic analysis of the nsp14/SS148 complex. We employed the fusion protein-assisted crystallization approach using the MTase domain of nsp14 (residues 300–527) N-terminally fused to a small crystallization tag TELSAM developed by Kottur et al.45 The nsp14 MTase-TELSAM/SS148 crystals belonged to the hexagonal P65 space group and diffracted to the 2.6 Å resolution. The structure of the nsp14 MTase-TELSAM/SS148 complex was subsequently solved by molecular replacement and further refined to good R factors and geometry, as summarized in ESI Table S4.†
The SS148 ligand was bound to the SAM/SAH-binding site of nsp14 as expected (Fig. 6(a)). The structure of SS148 is derived from SAH and thus, the mechanism of its interaction with nsp14 is also similar to SAH. The nsp14-SS148 interaction is mediated by multiple interactions including both direct (via Asp352, Ala353, Tyr368, and Trp385) and indirect water-mediated hydrogen bonds (via Gln313, Asp331, and Ile332) (Fig. 6(b)). The amino acid moiety of SS148 binds to nsp14 amino acid residues Gln313, Trp385, and Asp331, while the central ribose-derived moiety of nsp14 interacts mainly with Asp352, and the basic adenine-derived moiety binds to Ala353 and Tyr368.
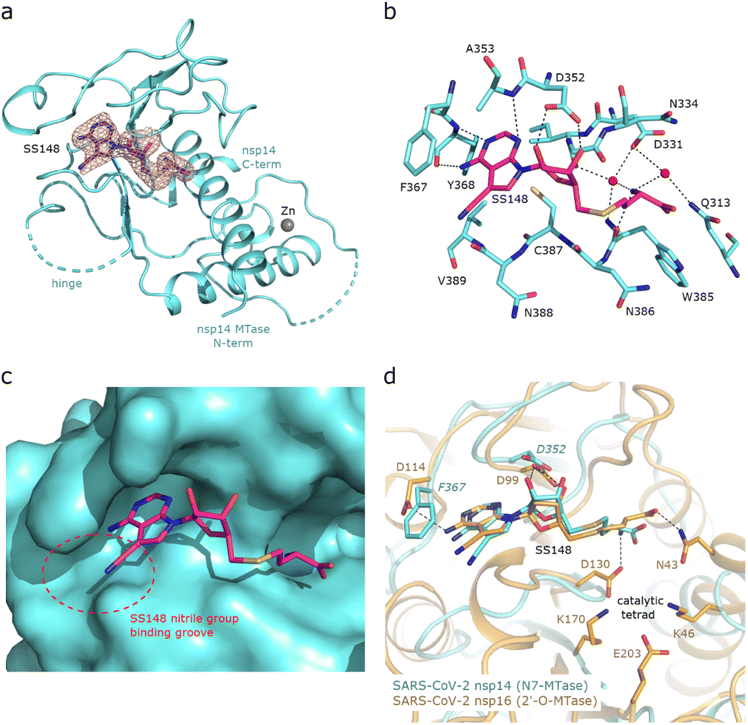 |
| Fig. 6 Crystal structure of nsp14 MTase in complex with SS148. (a), Overall view of the nsp14/SS148 complex. The protein backbone of the nsp14 MTase domain is shown in cartoon representation and colored in light black. The SS148 ligand is shown in stick representation and colored according to elements: carbon, magenta; nitrogen, black; oxygen, red; sulfur, yellow. The unbiased Fo-Fc omit map contoured at 2 s is shown around the SS148 ligand. The TELSAM crystallization tag N-terminally fused to the nsp14 MTase domain is not shown. (b), Detailed view of the SS148 ligand binding site. The SS148 ligand and side chains of selected nsp14 amino acid residues are shown in stick representation, with carbon atoms colored according to the protein assignment and other elements colored as in (a). Water molecules are presented as red spheres; hydrogen atoms are not shown. Selected hydrogen bonds involved in the nsp14–SS148 interaction are depicted as dashed black lines. (c), SS148 ligand binding site with nsp14 shown in surface representation. The nitrile group binding groove on the surface of nsp14 potentially accepting larger substituents is highlighted with a red dashed circle. (d), Structural alignment of the SS148 binding sites of SARS-CoV-2 N7-MTase nsp14 and 2′-O-MTase nsp16. Protein backbones are shown in cartoon representation, while SS148 and side chains of selected residues are shown in stick representation. The carbon atoms of the nsp14/SS148 and nsp16/SS148 complexes are depicted in light black and yellow, respectively; other elements are colored as in (a). | |
Compared to SAH, SS148 contains a nitrile group bound to the C7-position of the 7-deaza-adenine heterocyclic moiety. This nitrile group is bound to nsp14 in the groove formed by the nsp14 residues Phe367, Asn388, and Val389 (Fig. 6(c)). Our study documents that introduction of substituents at the C7-position of this 7-deaza-adenine moiety, physically larger than the presented nitrile group, can be a promising strategy potentially leading to development of more potent and more specific nsp14 inhibitors.
SS148 is an inhibitor with dual activity against both SARS-CoV-2 N7-MTase nsp14 and 2′-O-MTase nsp16. Therefore, we compared the SS148 binding site of nsp14 with the previously crystallized SS148 binding site of nsp16 (ref. 28) (Fig. 6(d)). Despite significant differences in the organization of the SS148 binding pockets in nsp14 and nsp16, we observed similar conformation of SS148. The conserved catalytic tetrad characteristic for 2′-O-MTases formed by Lys46, Asp130, Lys170, and Glu203 in nsp16 is not present in nsp14.
To judge the reliability of our computational pipeline, we performed re-docking of SS148 onto the nsp14 binding site. Our methodological approach was successful in accurately replicating the binding pose of the SS148 ligand within the nsp14 binding site, showcasing a striking alignment (RMSD 1.426 Å) with the experimentally determined crystal structure (depicted in ESI Fig. S4†). The high degree of concordance between the computationally determined re-docking pose and the experimental crystal structure offers a sanity check for the chosen docking method, especially in light of SS148's structural similarities to the endogenous substrate SAM/SAH.
4 Conclusion and outlook
Our comprehensive study has provided valuable insights into the complex dynamics of the SARS-CoV-2 nsp14 protein and the therapeutic potential of compounds aimed at inhibiting it. In particular, we found that the compound NSC620333 demonstrates promising attributes as a therapeutic agent against SARS-CoV-2, primarily due to its potent inhibitory effects on the nsp14 Methyltransferase (MTase) activity and its substantial binding affinity to nsp14. The observed in vivo efficacy and selectivity of this compound for development of therapeutics in treating COVID-19. In our study, we identified several critical residues within the MTase binding pocket of nsp14, and elucidated their dynamic behavior in the presence of potential inhibitors, such as NSC620333. Our findings lay a solid foundation for future drug design efforts, paving the way for the development of novel strategies to disrupt the nsp14 function. This could potentially lead to new therapeutic options for COVID-19. While NSC620333 shares structural similarities with the known inhibitor SS148—and by extension, the endogenous substrate and product (SAM/SAH)—the value of our research extends beyond compound novelty. It lies in the detailed elucidation of compound interactions with nsp14, crucial for advancing antiviral development. SS148, informed by SAM/SAH, serves as a foundational precursor in our work, positioning NSC620333 as an alternative starting point for optimization.
Interestingly, we observed conformational changes in nsp14, particularly in the presence and absence of metal coordination complexes, which emphasizes the role of this metal ion in the structural dynamics of nsp14. The diverse binding poses of the NSC620333 ligand identified in our study highlight the necessity for a dynamic view of protein–ligand interactions. It suggests that a single static view of a protein–ligand complex is insufficient to capture the full complexity of these interactions, further emphasizing the value of molecular dynamics simulations in comprehending these systems. Our structural studies also revealed the binding mechanism of another potential inhibitor, SS148. By visualizing its binding mode and interactions with nsp14, we proposed that introducing substituents at the C7-position of the 7-deaza-adenine moiety could enhance the potency and specificity of nsp14 inhibitors. The crystal structure also revealed the differences and similarities in the SS148 binding site between nsp14 and nsp16, providing valuable structural insights for drug design.
While our results are promising, they represent only the initial steps in a much longer journey. Further studies are needed to fully understand the therapeutic potential of these compounds. Future work should focus on optimizing these lead compounds, assessing their pharmacokinetic and pharmacodynamic properties, and evaluating their safety and efficacy in pre-clinical and clinical trials. Furthermore, the mechanistic exploration of NSC76988 and its potential allosteric mode of inhibition should also be pursued. Overall, this study underscores the potential of computational methods in understanding the complexities of biological systems and guiding the development of new therapeutics. We hope that our findings will inspire and inform future research efforts towards the development of effective treatments for COVID-19 and potentially other related viral threats.
5 Methods
5.1 Docking
The Cryo-EM structure of SARS-CoV-2 nsp10-nsp14 (PDB:7N0B46) was utilized as a reference for molecular docking, as it was the highest resolution structure available to us at the time that included the complete nsp14 complex with nsp10 and wild-type RNA. The receptor was prepared through Schrödinger's protein preparation tool,47 which involved a series of critical steps. These steps included modeling missing residues, capping missing termini, removing water molecules, and assigning protonation states. The ligand binding site was identified by superimposing SAM/SAH (PDB: 5C8S/5C8T48) onto the Cryo-EM structure. Upon completion of the overlay, a minimization was performed using the OPLS4 force field.49
The process aimed to optimize the overall structure of the receptor–ligand complex, minimize potential clashes, and maximize binding interactions. For the molecular docking process, we employed Glide-SP and Glide-XP software,31 integrated within the VirtualFlow Unity26 (VFU) pipeline (https://github.com/VirtualFlow/VFU). In the interest of scientific inclusivity, we provide a VFU setup that utilizes QuickVina50 and Smina,51 two open-source docking programs.
The NCI Open Compound collection was procured from NCBI PubChem (https://pubchem.ncbi.nlm.nih.gov/) by selecting “DTP/NCI” as the Data Source. The provided isomeric smiles were employed as starting points for processing the initial 250
000 molecules. At the stage of identifying the top-100 molecules, the molecules were manually inspected to ensure correct stereochemistry.
Diversity for a set of molecules was calculated as:
| 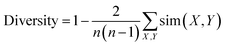 | (1) |
The expression sim(X, Y) computes the pairwise molecular similarity for all n structures calculated as the Tanimoto distance of the Morgan fingerprint (calculated with a radius of size 3 and a 2048 bit size).52
5.2 MM/GBSA calculation
The AMBER ff14SB force field53 and the General AMBER force field (GAFF2)54 were employed for parameterizing proteins and ligands, respectively, in the complex systems. The antechamber module55 was utilized to calculate the partial atomic charges using the AM1-BCC charge model for ligand molecules. To eliminate bad steric contacts, the systems were subjected to energy minimization with the steepest descent algorithm and conjugate gradient methods, without restraints. This process involved 2500 iterations using the Sander MD engine.56 The MM/GBSA energies were evaluated using the MMPBSA.py20 script, which is part of the AmberTools21 package.57 To calculate MM/GBSA energies, we utilized the following equation: | ΔGbind = Gcomplex − (Gprotein + Gligand) | (2) |
where Gcomplex, Gprotein and Gligand represent the free energies of the protein-ligand complex, the protein alone, and the ligand alone, respectively. The binding free energy (ΔGbind) reflects the net energy change upon binding. Further, the binding free energy calculation was refined to include contributions from molecular mechanics energy changes in the gas phase (ΔEMM), differences in solvation-free energy (ΔGsol), and the entropy term (−TΔS), as shown in the equation: | ΔGbind = ΔEMM + ΔGsol − TΔS | (3) |
Here, ΔEMM comprises the van der Waals (ΔEvdW) and electrostatic (ΔEele) energy contributions. The solvation-free energy (ΔGsol) is further divided into polar (ΔGpol) and nonpolar (ΔGnonpol) components based on the solvent-accessible surface area and employing the Generalized Born (GB) model for polar contributions. The total calculated binding free energy (ΔGbind) is the sum of the gas-phase interaction energy and solvation-free energy, excluding the entropy term for simplicity. Additionally, we conducted per-residue decomposition to delineate the contributions of individual residues to the effective binding energies.
5.3 Protein expression and purification
The SARS-CoV-2 nsp14 MTase domain-encoding sequence (GenBank: YP_009725309.1, residues 300–527) was codon-optimized for expression in E. coli and synthesized by GeneArt (Thermo Scientific). The gene was cloned into a modified pRSFDuet vector containing an N-terminal hexahistidine (His6) purification tag, followed by a SUMO solubility and folding tag, and a TELSAM crystallization tag.45 The plasmid was transformed into E. coli BL21 DE3 NiCo bacterial strain (New England Biolabs), and the protein was overexpressed using autoinduction ZY medium. Cells were collected by centrifugation and resuspended in lysis buffer (50 mM Tris, pH 8.0, 400 mM NaCl, 20 mM imidazole, 10 mM MgCl2, 10 μM ZnCl2, 3 mM β-mercaptoethanol, and 250 U of DNA endonuclease DENERASE (c-LEcta)). The cells were then sonicated using the Q700 Sonicator instrument (QSonica). The lysate was subsequently cleared by centrifugation, and the supernatant was incubated with Ni-NTA agarose (Thermo Scientific), followed by extensive washing with lysis buffer. The protein was finally eluted using lysis buffer supplemented with 300 mM imidazole. Post-elution, the protein was treated with Ulp1 protease to cleave off the His6-SUMO tag. The nsp14 MTase-TELSAM protein was further purified by size exclusion chromatography using Superdex 200 16/600 (GE Healthcare) pre-equilibrated with size-exclusion buffer (25 mM Tris pH 8.3, 200 mM KCl, and 2 mM TCEP). Fractions containing the nsp14 MTase-TELSAM protein were concentrated to 4 mg ml−1, flash-frozen, and stored at −80 °C.
5.4 Crystallization and crystallographic analysis
Crystallization trials were conducted with a concentration of 4 mg ml−1 of the nsp14 MTase-TELSAM protein, supplemented with a twofold molar excess of the SS148 ligand. Crystals were observed to grow within two days at 18 °C. This was achieved in a sitting drop composed of a 200 nl mixture of the protein and 200 nl of the mother liquor, which contained 100 mM bicine/Trizma pH 8.5; 10% w/v PEG 20,000; 20% v/v PEG MME 550; 20 mM D-glucose; 20 mM D-mannose; 20 mM D-galactose; 20 mM L-fucose; 20 mM D-xylose; 20 mM N-acetyl-D-glucosamine.
The crystallographic dataset was collected from a single crystal at the BL14.1 beamline, located at the BESSY II electron storage ring operated by Helmholtz-Zentrum Berlin.58 Data integration and scaling were performed using XDS.59 Molecular replacement was used to solve the structure of the nsp14 MTase-TELSAM/SS148 complex, with the nsp14 MTase-TELSAM/sinefungin complex structure serving as the search model (PDB entry 7TW945). Phaser from the Phenix package was used for the initial model.60,61 Model improvement was accomplished via automatic model refinement with Phenix. refine62 and manual model building with Coot.63 Statistics regarding data collection, structure solution, and refinement are provided in ESI Table S4.† Structural figures were generated using PyMOL Molecular Graphics System v2.5 (Schrödinger, LLC).64 The atomic coordinates and structural factors have been deposited in the Protein Data Bank (https://www.rcsb.org) under the accession code 8BWU.
5.5 Radioactivity-based assay conditions
The assay conditions for nsp14 were as follows: The final concentrations in the reaction mixture were 1.5 nM for nsp14, 50 nM for Biotin-RNA (5′GpppACCCCCCCCC-Biotin 3′), and 250 nM for 3H-SAM. The incubation was carried out for 20 minutes at 23 °C. The buffer used contained 20 mM Tris HCl at pH 7.5, 250 μM MgCl2, 5 mM DTT, and 0.01% Triton X-100, with an additional 5% DMSO.
5.6 SPR conditions
For Surface Plasmon Resonance (SPR), the full-length biotinylated nsp14 was immobilized on the flow cell of a SA sensor chip in 1x HBS-EP buffer, achieving a response of 8000 RU. The assay was performed using the same buffer but with 2% DMSO added. Single-cycle kinetics were employed with a contact time of 60 seconds and a dissociation time of 120 seconds, at a flow rate of 75 μL min−1. NSC620333 was tested at a concentration of 10 μM as the highest concentration, and a dilution factor of 0.25 was used to yield five different concentrations.
5.7 Molecular dynamics simulations
The crystal structure of nsp14 in complex with nsp10 was obtained from the Protein Data Bank (PDB) under the entry code 7N0B. All missing residues and variants relative to the wild-type were modeled using Schrodinger's protein preparation tool. The RNA complex was removed for the purposes of our simulations. The system was solvated in a periodic cubic box containing TIP3P water with 150 mM KCl using CHARMM-GUI.65 The CHARMM 36 m force field66 with hydrogen mass repartitioning was employed, and input files were sourced directly from CHARMM-GUI. Molecular dynamics simulations were performed using the Gromacs 2020.3 software package.67 The simulations employed a Langevin thermostat and a Nosé–Hoover Langevin piston barostat to maintain a pressure of 1 atm. Long-range interactions were treated using the particle mesh Ewald method, and non-bonded interactions were smoothed between 10 to 12 Å. The system underwent energy minimization for 1500 steps, followed by equilibration to 303.15 K with a timestep of 2 fs for 10 ns using the SHAKE and SETTLE algorithms.68 Production simulations were conducted with a 4 fs timestep for a total of 1.0 μs, with five independent replicates. Simulations that included NSC620333 were parameterized using the Charmm General Force Field (CGenFF) framework.69 Throughout the simulations, the root-mean-square deviation (RMSD) of the protein residues was calculated using the MDTraj package.70 All our simulations can be downloaded from: https://drive.google.com/drive/folders/1tKiIsDxBbjZLCxceSoDXlJAczd4UgHIJ?usp=sharing.
5.8 tICA 2D projections
5.8.1 Time-lagged independent component analysis (tICA).
To dissect the conformational dynamics of NSC620333, we employed time-lagged independent component analysis (tICA). This dimensionality reduction technique is designed to capture the slowest motions in the system by identifying linear combinations of input features that exhibit the highest autocorrelation at a specific lag time.
5.8.2 Data preparation and featurization.
The molecular dynamics simulation data was preprocessed to extract meaningful features for the tICA. The backbone α-carbons were selected for featurization because they effectively describe the overall movements of the protein-ligand complex. We calculated the pairwise distances between these α-carbons and the heavy atoms of NSC620333 to capture the ligand's positioning in relation to the protein's structure.
5.8.3 tICA computation and clustering.
A time-lagged covariance matrix was constructed from the pairwise distance matrix using a lag time of 1 ns, a value determined through trial and optimization for this system. The tICA transformation then reduced the data to a two-dimensional representation that separates the distinct conformational clusters. Density-based clustering was applied to the tICA-transformed data to identify discrete metastable states, with the assumption that regions of higher density in the tICA plot indicate energetically favorable and stable conformations.
5.8.4 Selection of representative frames.
To accurately represent each identified cluster, representative frames were selected based on their proximity to the centroid of the cluster. This was achieved by calculating the Euclidean distance from each frame within a cluster to its centroid in the reduced tICA space. The frame with the shortest distance was chosen as the representative for that cluster because it most closely approximates the central conformation assumed by the protein-ligand complex in that metastable state.
5.9 Anti-SARS-CoV-2 and cytotoxicity assays in Calu-3 cells
Anti-SARS-CoV-2 activity of NSC620333 and NSC76988 compounds were determined in the human lung adenocarcinoma cell line Calu-3 (ATCC, cat. no. HTB-55) using SARS-CoV-2 (isolate hCoV-19/Czech Republic/NRL_6632_2/2020). Briefly, two-fold eight serial dilutions of the compounds starting from 100 μM were added to 40
000 Calu-3 cells seeded for 1 day in DMEM supplemented with 2% fetal bovine serum, 100 U of penicillin per mL and 100 μg of streptomycin per mL. After 1 h incubation at 37 °C, SARS-CoV-2 was added at a multiplicity of infection (MOI) of 0.03 IU ml−1, incubated 3 days at 37 °C, and the virus-induced cytopathic effect (CPE) was quantified by formazan-based cell viability assay (XTT assay). XTT solution was added and after 4 h incubation, the absorbance at 450 nm was measured using the EnVision plate reader. After plotting the percentage cell viability versus log10 concentrations, the compound concentrations required to reduce viral cytopathic effect by 50% (EC50) were calculated by nonlinear regression. To determine compound cytotoxicity, Calu-3 cells were exposed to the same compound dilutions as above but without virus, and cell viability was determined using XTT assay. The compound concentrations causing 50% reduction in cell viability (CC50) were calculated similarly. Three-fold serial dilutions of Remdesivir from 10 μM served as a control in both experiments.
5.10 Assay technique
The MTase activity of nsp14 was measured using a radiometric assay as described previously.27 Chlorobenzothiophene 3 was tested at various concentrations ranging from 200 nM to 200 μM to determine the half-maximal inhibitory concentration (IC50) value. The final reaction mixture consisted of 1.5 nM nsp14, 250 nM3H-SAM, and 50 nM RNA in buffer (20 mM Tris HCl, pH 7.5, 250 μM MgCl2, 5 mM DTT, and 0.01% Triton X-100). The reaction time was 20 min at 23 °C. Data were fitted to the four-parameter logistic equation using GraphPad Prism 8.
5.11 Synthesis of SS148
The synthesis of SS148 (also referred to as CN-SAH in the literature) was carried out according to previously published procedures.71 Comprehensive synthetic procedures and associated 1H and 13C-NMR data for SS148 can be found in the referenced literature.
Author contribution
Conceptualization: A. K. N., M. F. D. H., R. L. K., V. A. V., M. V., A. A.-G. data curation: A. K. N., M. F. D. H., F. L., S. S. C., S. P. formal analysis: M. F. D. H., F. L., E. K., M. K. funding acquisition: A. K. N., R. P., H. N., M. F. D. H., V. A. V., A. A.-G. investigation: M. F. D. H., F. L., E. K., R. P., J. T., H. Ü. K., Y. H. methodology: M. F. D. H., F.L., E. K., R. P., J. T., R. L. K., J. H., M. S., H.-L. N., H. Ü. K., Y. H. project administration: A. K., J. J., V. A. V., J. W., R. N., E. B. Resources: M. K., R. P., J. T., H. Ü. K., Y. H. Software: M. S., H.-L. N. Supervision: A. K., J. J., V. A. V., J. W., R. N., E. B. validation: M. F. D. H., F. L., E. K., M. K., J. T. visualization: M. K. writing – original draft preparation: AK. N., S. S., M. F. D. H., R. L. K., C. G., M. V. writing – review & editing: AK. N., R. L. K., C. G., M. V., all authors reviewed and approved the final draft.
Conflicts of interest
There are no conflicts to declare.
Acknowledgements
AK. N. acknowledges funding from the Bio-X Stanford Interdisciplinary Graduate Fellowship (SGIF). Special thanks are extended to A. K. and Michael Bassik for their invaluable assistance in securing the SGIF funding. R. P. acknowledges funding through a Postdoc. Mobility fellowship by the Swiss National Science Foundation (SNSF, Project No. 191127). A. A.-G. thanks Dr Anders G. Frøseth for his generous support. A. A.-G. also acknowledges the generous support of Natural Resources Canada and the Canada 150 Research Chairs program. M. F. D. H. and V. A. V. were supported in part by the National Institutes of Health grant 1R01GM123296. Runs of the molecular dynamics simulations were performed on high-performance computing (HPC) resources supported in part by the National Science Foundation through major research instrumentation grant number 1625061 and by the US Army Research Laboratory under contract number W911NF-16-2–0189. Additional simulations were conducted on the CB2RR cluster, which was made possible through an NIH Research Resource computer instrumentation grant S10-OD020095. S. S. is a Damon Runyon Quantitative Biology Fellow supported by the Damon Runyon Cancer Research Foundation (DRQ-14-22). This research was enabled in part by support provided by Calcul Québec (https://www.calculquebec.ca) and Compute Canada (https://www.computecanada.ca). HN acknowledges NVIDIA and Intel for funding. MD simulations for all compounds were performed on the Béluga supercomputer situated at the École de technologie supérieure in Montreal. We thank the Helmholtz-Zentrum Berlin für Materialien und Energie for the allocation of synchrotron radiation beamtime. This work was supported by the project National Institute Virology and Bacteriology (Programme EXCELES, Project No. LX22NPO5103) – Funded by the European Union – Next Generation EU. The research was also, in part, made possible thanks to funding provided to the University of Toronto's Acceleration Consortium by the Canada First Research Excellence Fund (CFREF-2022-00042).
Notes and references
- C. I. Paules, H. D. Marston and A. S. Fauci, Coronavirus infections—more than just the common cold, Jama, 2020, 323(8), 707–708 CrossRef CAS PubMed
.
- W. H. Organization,
et al., The corona virus disease 2019 (COVID-19), Braz. J. Implantol. Health Sci., 2022, 4(6), 45–46 Search PubMed
.
-
H. Achdout, A. Aimon, E. Bar-David and G. Morris, COVID moonshot: open science discovery of SARS-CoV-2 main protease inhibitors by combining crowdsourcing, high-throughput experiments, computational simulations, and machine learning, BioRxiv, 2020 Search PubMed.
- J. Chodera, A. A. Lee, N. London and F. von Delft, Crowdsourcing drug discovery for pandemics, Nat. Chem., 2020, 12(7), 581 CrossRef CAS PubMed
.
- F. von Delft, M. Calmiano, J. Chodera, E. Griffen, A. Lee and N. London,
et al., A white-knuckle ride of open COVID drug discovery, Nature, 2021, 594(7863), 330–332 CrossRef CAS PubMed
.
- J. Schimunek, P. Seidl, K. Elez, T. Hempel, T. Le and F. Noé,
et al., A community effort in SARS-CoV-2 drug discovery, Mol. Inf., 2024, 43(1), e202300262 CrossRef CAS PubMed
.
-
R. Sharma, M. Agarwal, M. Gupta, S. Somendra, S. K. Saxena, Clinical characteristics and differential clinical diagnosis of novel coronavirus disease 2019 (COVID-19), Coronavirus Disease 2019 (COVID-19) Epidemiology, Pathogenesis, Diagnosis and Therapeutics, 2020, pp. 55–70 Search PubMed
.
- A. A. T. Naqvi, K. Fatima, T. Mohammad, U. Fatima, I. K. Singh and A. Singh,
et al., Insights into SARS-CoV-2 genome, structure, evolution, pathogenesis and therapies: Structural genomics approach, Biochim. Biophys. Acta - Mol. Basis Dis., 2020, 1866(10), 165878 CrossRef CAS PubMed
.
- T. I. Ng, I. Correia, J. Seagal, D. A. DeGoey, M. R. Schrimpf and D. J. Hardee,
et al., Antiviral drug discovery for the treatment of COVID-19 infections, Viruses, 2022, 14(5), 961 CrossRef CAS PubMed
.
- M. Bouvet, C. Debarnot, I. Imbert, B. Selisko, E. J. Snijder and B. Canard,
et al., In vitro reconstitution of SARS-coronavirus mRNA cap methylation, PLoS Pathog., 2010, 6(4), e1000863 CrossRef PubMed
.
- Y. Chen, H. Cai, J. Pan, N. Xiang, P. Tien and T. Ahola,
et al., Functional screen reveals SARS coronavirus nonstructural protein nsp14 as a novel cap N7 methyltransferase, Proc. Natl. Acad. Sci. U. S. A., 2009, 106(9), 3484–3489 CrossRef CAS PubMed
.
- V. M. Corman, D. Muth, D. Niemeyer and C. Drosten, Hosts and sources of endemic human coronaviruses, Adv. Virus Res., 2018, 100, 163–188 CAS
.
- A. von Delft, M. D. Hall, A. D. Kwong, L. A. Purcell, K. S. Saikatendu and U. Schmitz,
et al., Accelerating antiviral drug discovery: lessons from COVID-19, Nat. Rev. Drug Discovery, 2023, 1–19 Search PubMed
.
- M. Stefek, D. Chalupská, K. Chalupsky, M. Zgarbová, A. Dvorakova and P. Krafcikova,
et al., Rational design of highly potent SARS-CoV-2 nsp14 methyltransferase inhibitors, ACS omega, 2023, 8(30), 27410–27418 CrossRef CAS PubMed
.
- T. Otava, M. Sala, F. Li, J. Fanfrlik, K. Devkota and S. Perveen,
et al., The structure-based design of SARS-CoV-2 nsp14 methyltransferase ligands yields nanomolar inhibitors, ACS Infect. Dis., 2021, 7(8), 2214–2220 CrossRef CAS PubMed
.
- W. D. Ihlenfeldt, J. H. Voigt, B. Bienfait, F. Oellien and M. C. Nicklaus, Enhanced CACTVS browser of the Open NCI Database, J. Chem. Inf. Comput. Sci., 2002, 42(1), 46–57 CrossRef CAS PubMed
.
- J. H. Voigt, B. Bienfait, S. Wang and M. C. Nicklaus, Comparison of the NCI open database with seven large chemical structural databases, J. Chem. Inf. Comput. Sci., 2001, 41(3), 702–712 CrossRef CAS PubMed
.
- N. S. Pagadala, K. Syed and J. Tuszynski, Software for molecular docking: a review, Biophys. Rev., 2017, 9, 91–102 CrossRef CAS PubMed
.
- A. Nigam, R. Pollice, G. Tom, K. Jorner, J. Willes, L. Thiede, A. Kundaje and A. Aspuru-Guzik, A benchmarking platform for realistic and practical inverse molecular design,
Advances in Neural Information Processing Systems
, 2023, 36, 3263–306 Search PubMed
.
- I. I. I. B. R. Miller, Jr T. D. McGee, J. M. Swails, N. Homeyer, H. Gohlke and A. E. Roitberg, MMPBSA. py: an efficient program for end-state free energy calculations, J. Chem. Theory Comput., 2012, 8(9), 3314–3321 CrossRef PubMed
.
- M. Ylilauri and O. T. Pentikainen, MMGBSA as a tool to understand the binding affinities of filamin–peptide interactions, J. Chem. Inf. Model., 2013, 53(10), 2626–2633 CrossRef CAS PubMed
.
- S. A. Hollingsworth and R. O. Dror, Molecular dynamics simulation for all, Neuron, 2018, 99(6), 1129–1143 CrossRef CAS PubMed
.
- A. Hospital, J. R. Goñi, M. Orozco and J. L. Gelpí, Molecular dynamics simulations: Advances and applications, Adv. Appl. Bioinf. Chem., 2015, 8, 37–47 Search PubMed
.
-
R. Levin-Konigsberg, K. Mitra, A. Nigam, K. Spees, P. Hivare, K. Liu, et al., SLC12A9 is a lysosome-detoxifying ammonium-chloride co-transporter, bioRxiv, 2023, pp. 2023–2105 Search PubMed.
- N. S. Ogando, J. C. Zevenhoven-Dobbe, Y. van der Meer, P. J. Bredenbeek, C. C. Posthuma and E. J. Snijder, The enzymatic activity of the nsp14 exoribonuclease is critical for replication of MERS-CoV and SARS-CoV-2, J. Virol., 2020, 94(23), 10–1128 CrossRef PubMed
.
-
C. Gorgulla, A. Nigam, M. Koop, S. S. Cinaroglu, C. Secker, M. Haddadnia, et al., VirtualFlow 2.0-The Next Generation Drug Discovery Platform Enabling Adaptive Screens of 69 Billion Molecules, bioRxiv, 2023, pp. 2023–2104 Search PubMed.
- K. Devkota, M. Schapira, S. Perveen, A. Khalili Yazdi, F. Li and I. Chau,
et al., Probing the SAM binding site of SARS-CoV-2 Nsp14 in vitro using SAM competitive inhibitors guides developing selective bisubstrate inhibitors, SLAS DISCOVERY: Advancing the Science of Drug Discovery, 2021, 26(9), 1200–1211 CrossRef CAS PubMed
.
- M. Klima, A. Khalili Yazdi, F. Li, I. Chau, T. Hajian and A. Bolotokova,
et al., Crystal structure of SARS-CoV-2 nsp10–nsp16 in complex with small molecule inhibitors, SS148 and WZ16, Protein Sci., 2022, 31(9), e4395 CrossRef CAS PubMed
.
- M. Schenone, V. Dančík, B. K. Wagner and P. A. Clemons, Target identification and mechanism of action in chemical biology and drug discovery, Nat. Chem. Biol., 2013, 9(4), 232–240 CrossRef CAS PubMed
.
- S. Wan, A. P. Bhati, S. J. Zasada and P. V. Coveney, Rapid, accurate, precise and reproducible ligand–protein binding free energy prediction, Interface Focus, 2020, 10(6), 20200007 CrossRef PubMed
.
- R. A. Friesner, J. L. Banks, R. B. Murphy, T. A. Halgren, J. J. Klicic and D. T. Mainz,
et al., Glide: a new approach for rapid, accurate docking and scoring. 1. Method and assessment of docking accuracy, J. Med. Chem., 2004, 47(7), 1739–1749 CrossRef CAS PubMed
.
-
J. Homola and M. Piliarik, Surface plasmon resonance (SPR) sensors, Springer, 2006 Search PubMed
.
- D. Varshney, A. P. Petit, J. A. Bueren-Calabuig, C. Jansen, D. A. Fletcher and M. Peggie,
et al., Molecular basis of RNA guanine-7 methyltransferase (RNMT) activation by RAM, Nucleic Acids Res., 2016, 44(21), 10423–10436 CrossRef CAS PubMed
.
- K. Jain and S. G. Clarke, PRMT7 as a unique member of the protein arginine methyltransferase family: A review, Arch. Biochem. Biophys., 2019, 665, 36–45 CrossRef CAS PubMed
.
- A. Ramanathan, G. B. Robb and S. H. Chan, mRNA capping: biological functions and applications, Nucleic Acids Res., 2016, 44(16), 7511–7526 CrossRef PubMed
.
- S. Pal and S. Sif, Interplay between chromatin remodelers and protein arginine methyltransferases, J. Cell. Physiol., 2007, 213(2), 306–315 CrossRef CAS PubMed
.
- V. S. Pande, K. Beauchamp and G. R. Bowman, Everything you wanted to know about Markov State Models but were afraid to ask, Methods, 2010, 52(1), 99–105 CrossRef CAS PubMed
.
- L. Molgedey and H. G. Schuster, Separation of a mixture of independent signals using time delayed correlations, Phys. Rev. Lett., 1994, 72(23), 3634 CrossRef PubMed
.
- Y. Naritomi and S. Fuchigami, Slow dynamics in protein fluctuations revealed by time-structure based independent component analysis: the case of domain motions, J. Chem. Phys., 2011, 134(6), 065101 CrossRef PubMed
.
- I. Hartigan and A. Algoritm, 136: A K-Means Clustering Algorithm/JA Hartigan, MA Wong, J. R. Stat. Soc., C: Appl. Stat., 1979, 28(1), 100–108 Search PubMed
.
- J. R. Wagner, C. T. Lee, J. D. Durrant, R. D. Malmstrom, V. A. Feher and R. E. Amaro, Emerging computational methods for the rational discovery of allosteric drugs, Chem. Rev., 2016, 116(11), 6370–6390 CrossRef CAS PubMed
.
- P. Andrews, D. Craik and J. Martin, Functional group contributions to drug-receptor interactions, J. Med. Chem., 1984, 27(12), 1648–1657 CrossRef CAS PubMed
.
- S. J. Teague, Implications of protein flexibility for drug discovery, Nat. Rev. Drug Discovery, 2003, 2(7), 527–541 CrossRef CAS PubMed
.
- H. Li and C. Li, Multiple ligand simultaneous docking: orchestrated dancing of ligands in binding sites of protein, J. Comput. Chem., 2010, 31(10), 2014–2022 CrossRef CAS PubMed
.
- J. Kottur, O. Rechkoblit, R. Quintana-Feliciano, D. Sciaky and A. K. Aggarwal, High-resolution structures of the SARS-CoV-2 N7-methyltransferase inform therapeutic development, Nat. Struct. Mol. Biol., 2022, 29(9), 850–853 CrossRef CAS PubMed
.
- C. Liu, W. Shi, S. T. Becker, D. G. Schatz, B. Liu and Y. Yang, Structural basis of mismatch recognition by a SARS-CoV-2 proofreading enzyme, Science, 2021, 373(6559), 1142–1146 CrossRef CAS PubMed
.
-
ödinger Release S. 2: Protein Preparation Wizard, Epik, Schrödinger, LLC, New York, NY, 2021 Search PubMed.
- Y. Ma, L. Wu, N. Shaw, Y. Gao, J. Wang and Y. Sun,
et al., Structural basis and functional analysis of the SARS coronavirus nsp14–nsp10 complex, Proc. Natl. Acad. Sci. U. S. A., 2015, 112(30), 9436–9441 CrossRef CAS PubMed
.
- C. Lu, C. Wu, D. Ghoreishi, W. Chen, L. Wang and W. Damm,
et al., OPLS4: Improving force field accuracy on challenging regimes of chemical space, J. Chem. Theory Comput., 2021, 17(7), 4291–4300 CrossRef CAS PubMed
.
- A. Alhossary, S. D. Handoko, Y. Mu and C. K. Kwoh, Fast, accurate, and reliable molecular docking with QuickVina 2, Bioinformatics, 2015, 31(13), 2214–2216 CrossRef CAS PubMed
.
- D. R. Koes, M. P. Baumgartner and C. J. Camacho, Lessons learned in empirical scoring with smina from the CSAR 2011 benchmarking exercise, J. Chem. Inf. Model., 2013, 53(8), 1893–1904 CrossRef CAS PubMed
.
- D. Rogers and M. Hahn, Extended-connectivity fingerprints, J. Chem. Inf. Model., 2010, 50(5), 742–754 CrossRef CAS PubMed
.
- J. A. Maier, C. Martinez, K. Kasavajhala, L. Wickstrom, K. E. Hauser and C. Simmerling, ff14SB: improving the accuracy of protein side chain and backbone parameters from ff99SB, J. Chem. Theory Comput., 2015, 11(8), 3696–3713 CrossRef CAS PubMed
.
- J. Wang, R. M. Wolf, J. W. Caldwell, P. A. Kollman and D. A. Case, Development and testing of a general amber force field, J. Comput. Chem., 2004, 25(9), 1157–1174 CrossRef CAS PubMed
.
- J. Wang, W. Wang, P. A. Kollman and D. A. Case, Antechamber: an accessory software package for molecular mechanical calculations, J. Am. Chem. Soc., 2001, 222(1), 2001 Search PubMed
.
- J. W. Kaus, L. T. Pierce, R. C. Walker and J. A. McCammon, Improving the efficiency of free energy calculations in the amber molecular dynamics package, J. Chem. Theory Comput., 2013, 9(9), 4131–4139 CrossRef CAS PubMed
.
-
D. A. Case, H. M. Aktulga, K. Belfon, I. Ben-Shalom, S. R. Brozell, D. S. Cerutti, et al., Amber 2021, University of California, San Francisco, 2021 Search PubMed.
- U. Mueller, R. Förster, M. Hellmig, F. U. Huschmann, A. Kastner and P. Malecki,
et al., The macromolecular crystallography beamlines at BESSY II of the Helmholtz-Zentrum Berlin: Current status and perspectives, Eur. Phys. J. Plus, 2015, 130, 1–10 CrossRef
.
- W. Kabsch, xds, Acta Crystallogr., Sect. D: Biol. Crystallogr., 2010, 66(2), 125–132 CrossRef CAS PubMed
.
- A. J. McCoy, R. W. Grosse-Kunstleve, P. D. Adams, M. D. Winn, L. C. Storoni and R. J. Read, Phaser crystallographic software, J. Appl. Crystallogr., 2007, 40(4), 658–674 CrossRef CAS PubMed
.
- D. Liebschner, P. V. Afonine, M. L. Baker, G. Bunkóczi, V. B. Chen and T. I. Croll,
et al., Macromolecular structure determination using X-rays, neutrons and electrons: recent developments in Phenix, Acta Crystallogr., Sect. D: Struct. Biol., 2019, 75(10), 861–877 CrossRef CAS PubMed
.
- P. V. Afonine, R. W. Grosse-Kunstleve, N. Echols, J. J. Headd, N. W. Moriarty and M. Mustyakimov,
et al., Towards automated crystallographic structure refinement with phenix. refine, Acta Crystallogr., Sect. D: Biol. Crystallogr., 2012, 68(4), 352–367 CrossRef CAS PubMed
.
- P. Emsley and K. Cowtan, Coot: model-building tools for molecular graphics, Acta Crystallogr., Sect. D: Biol. Crystallogr., 2004, 60(12), 2126–2132 CrossRef PubMed
.
-
W. L. DeLano, The PyMOL molecular graphics system, 2002, https://www.pymol.org/ Search PubMed
.
- S. Jo, T. Kim, V. G. Iyer and W. Im, CHARMM-GUI: a web-based graphical user interface for CHARMM, J. Comput. Chem., 2008, 29(11), 1859–1865 CrossRef CAS PubMed
.
- J. Huang, S. Rauscher, G. Nawrocki, T. Ran, M. Feig and B. L. De Groot,
et al., CHARMM36m: an improved force field for folded and intrinsically disordered proteins, Nat. Methods, 2017, 14(1), 71–73 CrossRef CAS PubMed
.
- D. Van Der Spoel, E. Lindahl, B. Hess, G. Groenhof, A. E. Mark and H. J. Berendsen, GROMACS: fast, flexible, and free, J. Comput. Chem., 2005, 26(16), 1701–1718 CrossRef CAS PubMed
.
- S. Miyamoto and P. A. Kollman, Settle: An analytical version of the SHAKE and RATTLE algorithm for rigid water models, J. Comput. Chem., 1992, 13(8), 952–962 CrossRef CAS
.
- K. Vanommeslaeghe and Jr A. D. MacKerell, Automation of the CHARMM General Force Field (CGenFF) I: bond perception and atom typing, J. Chem. Inf. Model., 2012, 52(12), 3144–3154 CrossRef CAS PubMed
.
- R. T. McGibbon, K. A. Beauchamp, M. P. Harrigan, C. Klein, J. M. Swails and C. X. Hernández,
et al., MDTraj: a modern open library for the analysis of molecular dynamics trajectories, Biophys. J., 2015, 109(8), 1528–1532 CrossRef CAS PubMed
.
- S. S. Spurr, E. D. Bayle, W. Yu, F. Li, W. Tempel and M. Vedadi,
et al., New small molecule inhibitors of histone methyl transferase DOT1L with a nitrile as a non-traditional replacement for heavy halogen atoms, Bioorg. Med. Chem. Lett., 2016, 26(18), 4518–4522 CrossRef CAS PubMed
.
Footnotes |
† Electronic supplementary information (ESI) available. See DOI: https://doi.org/10.1039/d4dd00006d |
‡ Current affiliation: Stratingh Institute for Chemistry, University of Groningen, The Netherlands. |
|
This journal is © The Royal Society of Chemistry 2024 |