https://2DMat.ChemDX.org: Experimental data platform for 2D materials from synthesis to physical properties†
Received
11th December 2023
, Accepted 19th February 2024
First published on 27th February 2024
Abstract
We present a comprehensive data platform for 2D materials research, https://2DMat.ChemDX.org, and a newly constructed 2D database collected through the platform. This platform integrates efficient data management, specialized visualization, and machine learning toolkits, enhancing research productivity. The platform supports data obtained from reflection high-energy electron diffraction (RHEED), photoluminescence (PL), and Raman measurements, providing a robust foundation for uploading, managing, and sharing research data through a web-based platform. Data templates and parsing systems specialized for handling these data help researchers manage large datasets, reduce manual efforts, and enhance data consistency. The platform features powerful analysis tools for RHEED, PL, and Raman spectra, facilitating easy data comprehension with just a few clicks. Additionally, the platform incorporates machine learning toolkits for investigating 2D film growth mechanisms and super-resolution techniques for analyzing PL/Raman mapping data, promising an increase in efficiency. The modular design and the systematic 2D database of our platform enable not only seamless expansion and adaptation but also valuable data collection, management, and utilization in the evolving field of 2D materials.
1 Introduction
In recent years, integrating data science and artificial intelligence (AI) techniques into scientific research has triggered a transformative shift, particularly in materials exploration and optimization.1–11 This shift has significantly emphasized the values of “data” generated from experimental and computational processes, leading to the emergence of materials data platforms as indispensable tools in data-driven research.12–17 These platforms facilitate convenient data collection, integration, utilization, and sharing. Various materials data platforms have been developed, collecting materials properties from published literature, such as the Perovskite Database,18 Polymer Scholar,19–21 and Starrydata2,22 and electronic structures data obtained from density functional theory (DFT) calculations, such as AFLOWLIB,23 JARVIS-DB,24 Materials Project,25 MatHub-3d,26 NOMAD,27,28 OQMD,29,30 and TEDesignLab.31 These data platforms enable researchers to explore materials properties across vast material spaces, using large-scale data-driven approaches without expensive data generation procedures.32–38 These well-organized and extensive datasets have significantly accelerated materials exploration and discovery, paving the way for integrating AI methodologies in novel research approaches. By harnessing the power of data and AI, researchers have a powerful tool to unlock unprecedented opportunities and insights in the field of materials science.
Materials data platforms diverge in scope with some offering encyclopedic insights across a broad chemical space14,24,25,27–30 while others focus intently on specific areas such as thermoelectrics,22,31,39 perovskite solar cells,18 polymers,16,21 or catalysts.40–42 A prominent example is the burgeoning interest in 2D materials. Their unique properties and broad potential applications in fields such as electronic and optical devices,43–48 energy storage and transformation devices,49–53 catalysts,54–56 or sensors57–60 have led to the development of dedicated platforms and databases including 2DMatPedia,61 band gap database,62 Computational 2D materials database (C2DB),63,64 and Materials Cloud two-dimensional crystal database (MC2D).65,66 These platforms provide access to valuable data on various properties of 2D materials based on DFT calculations, including lattice structures, electronic band structures, the density of states, magnetic properties, and more. Leveraging the wealth of data from dedicated platforms, recent advancements in machine learning (ML) are now paving the way for innovative approaches in predicting fundamental properties and uncovering novel 2D materials.34,37,67–72
While computational data has provided valuable insights into materials properties, validating these predictions and understanding the real-world behavior of materials through experiments are still essential. Experimental data is vital in expanding the scope of data-driven research beyond electronic structures. Therefore, there is a need to develop platforms that facilitate the systematic collection and curation of experimental data, such as Inorganic Crystal Structure Database (ICSD),73 Perovskite Database,18 and TEXplorer.39 In this context, we developed a 2D materials data platform (https://2DMat.ChemDX.org) to construct and utilize an experimental database. This platform is a comprehensive resource for collecting, organizing, and sharing data from synthesis to property measurements of various 2D materials, including graphene, boron-nitride, and transition metal chalcogenides.
In this paper, we present an overview of the key features of the platform and illustrate our specially designed experimental database for 2D materials. The primary objectives of our platform are to construct a systematic database on 2D materials, efficiently manage experimental data, and utilize the data using advanced analysis toolkits, as described in Fig. 1. We collected experimental data on 2D materials within predefined templates, and the parsing systems of the platform automatically converted the raw data files into a standardized readable format. Once researchers upload their data, the platform provides a well-defined and scalable database, enabling web-based data sharing among colleagues. The constructed database through our platform includes experimental data on reflection high-energy electron diffraction (RHEED) images obtained throughout the film growth process, photoluminescence (PL), and Raman spectra for various 2D materials. The collected dataset consists of 278 samples with RHEED images, 289 samples with PL spectra, and 58 samples with Raman spectra and most of the data have been made public. Based on these datasets, the platform offers a range of tools for data visualization, spectrum and image analysis, and ML analyses. Through ML studies, we could uncover hidden patterns within the data and improve the spectra quality in the PL measurements. These analyses significantly contribute to our understanding of 2D materials and their properties. We expect this data platform and the accompanying experimental database will have a vital role in establishing an ecosystem for data collection and utilization, expanding and advancing research on 2D materials, and accelerating data-driven discoveries.
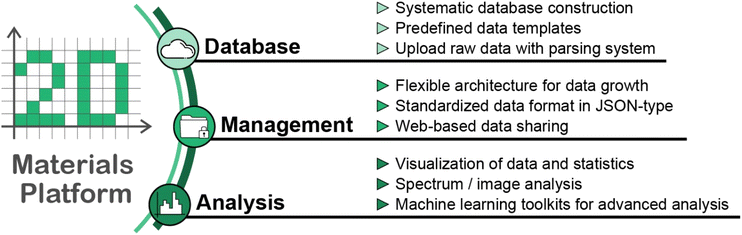 |
| Fig. 1 Key functionalities of the 2D materials platform (https://2dmat.chemdx.org/). The core features of our platform include systematic database construction with predefined templates, flexible data management architecture accommodating growth, and advanced analysis tools, including visualizers and machine learning toolkits for comprehensive insights into 2D materials. | |
2 Results
Fig. 2 demonstrates the workflow of our platform, starting from data generation in the laboratory to its management and applications. Researchers generate their data through various experiments in the laboratory and upload their data into our 2D materials platform. The raw data from the measurements are uploaded to the platform without any corrections, and a pre-designed parsing system automatically extracts valuable data with a Python module. Users can conveniently access and utilize data through Python-based visualization and machine learning toolkits provided by the platform. Data is presented as tables and interactive graphs, enabling researchers to explore and analyze data effectively. Our platform operates on a CentOS 7 server hosted on the Naver Cloud Platform for public institutions, with hosting services provided by Gabia. The backup policy involves a two-tier approach: daily backups are stored for seven days, and regular backups are conducted every seven days and stored in Naver Cloud's Object Storage. Recovery can be performed manually using these backups. The backend is developed with the Laravel library, and our data storage solutions include MariaDB 10.5 and MongoDB 3.6. The web UI is built using the Vue2 and Vuetify2 frameworks, complemented by the interactive graph visualization capabilities of Poltly.js.
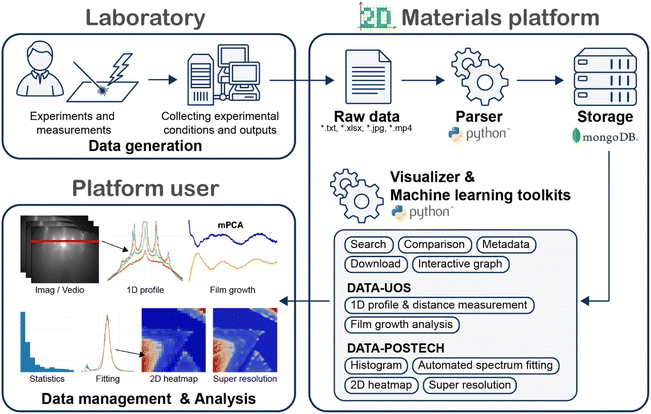 |
| Fig. 2 Illustration of the data flow within the 2D materials platform demonstrating the process from laboratory data generation to end-user presentation. The platform incorporates built-in data parsers developed in Python and offers various features such as data search, downloading, and visualization through interactive graphs. In addition to these general functionalities, the platform provides data-specific tools, such as film growth analysis for time-series data and super-resolution for mapping data. Users can effectively manage and analyze the data using these features in the front end. | |
2.1 Data generation
Following the workflow, we have constructed two databases for 2D materials through the platform. The first database, the DATA-UOS (University of Seoul) group, collects in situ RHEED data specifically from molecular beam epitaxy (MBE) growth of transition metal chalcogenide materials. RHEED is a powerful technique that monitors the surface state during film growth because it provides extensive physical information, including surface morphology, growth rate, lattice spacing, crystalline disorder, and surface reconstruction.74–80 Using the RHEED dataset, we will conduct a more quantitative analysis of the growth modes of 2D materials over time and identify crystal structures during the film growth.81–84 Along with the in situ RHEED data, we collected diverse surface analysis results for targeted samples, including AFM images, XPS, and PL/Raman spectra.
The second database, the DATA-POSTECH (Pohang University of Science and Technology) group, is dedicated to the optical measurement data of 2D materials. Position-mapped PL/Raman data are pivotal in advanced materials research, enabling an in-depth understanding of material properties at a microscopic level.85,86 These mapping techniques offer insights into material quality, defects, and variations in the properties, which are crucial for device optimization.85–90 The position-mapped PL/Raman data were acquired from various exfoliated and chemical vapor deposition-grown transition metal dichalcogenides. The PL/Raman measurements typically require a significant amount of time for high-resolution analysis, and applying ML techniques can potentially reduce the measurement time with our systematic datasets. We expect that our 2D materials dataset will provide a valuable resource for diverse research purposes, and the dataset can be downloaded on the website.
2.2 Data deposition and management
The platform serves as a data repository that collects various types of experimental data, such as numerical values, spectra, images, and videos. The unit data, distinguished by its unique ID number, is a collection of metadata, detailed parameters in experiments, and the measurement result data for a given synthesized sample. The metadata and detailed experimental parameters were written in a spreadsheet file in .xlsx format. The parameter list includes the experiment date, experimenter name, sample composition, substrate type, synthesis conditions, measurement parameters, etc. The complete parameter list is provided in the ESI (See Tables S1 and Table S2 for the two data groups).†
The raw data files of the measurements can be uploaded directly to the platform by only modifying the file name, which includes relevant tags. In the case of RHEED measurements, images are saved as .png files and videos as .mp4 files. To differentiate them, the tag “_rheed” is added to the file name, for example, “sample_rheed.mp4” or “sample_0 min_rheed.png.” Including a timestep in the suffix when uploading images to the DATA-UOS group is essential. For the PL/Raman spectrum measurements, the data is typically stored as .txt files. To indicate the type of measurement, the tags “_pl-pos” and “_raman-pos” are added to the file name, such as “sample_pl-pos.txt” and “sample_ranam-pos.txt.” Incorporating these relevant tags into the file names makes identifying and organizing the specific data types easier when uploading them to the platform. Subsequently, the pre-designed parsing modules verify that the submitted files adhere to the proper schema and contain valid values for visualization through the web UI. These modules are intentionally designed not to alter or assess the data's intrinsic validity, shifting the responsibility for data integrity to the users uploading the data. This decision stems from recognizing that automating the validation and management of data could necessitate complex rules, potentially leading to the misidentification of valid data as outliers and unintended modifications. Therefore, we encourage users to modify only the file names during the upload process as they are obtained from equipment. This strategy not only keeps the contents of the data files unchanged but also minimizes human error in data extraction, ensuring the preservation of the originality and integrity of the raw data.
The platform provides a user-friendly uploading system through a graphical user interface (GUI). Fig. 3(a) illustrates the GUI, which enables users to easily drag and drop their data files for uploading. Once uploading their data, the platform automatically creates a dataset for the experimental data and stores it using MongoDB storage type, thereby augmenting the existing database. Users can conveniently download the data on the web page and easily share it with colleagues. Database management becomes simplified through the platform, making organizing and accessing the data easier. The spreadsheet file, including the metadata and process parameters, is processed through built-in Python modules and displayed on the platform, as shown in Fig. 3(b).
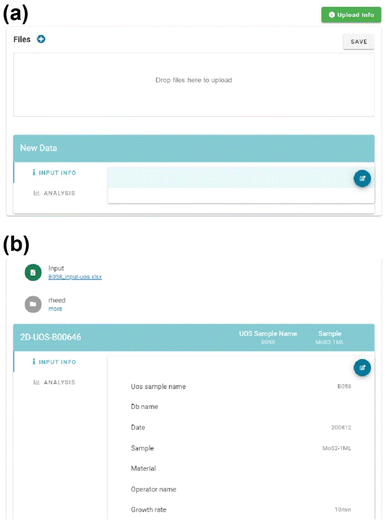 |
| Fig. 3 The platform GUI for data upload. Illustration of (a) a user-friendly drag-and-drop method that streamlines the data upload process and (b) the parsed input information presented as a table, along with the corresponding raw data files. | |
2.3 Visualization and analysis
With the collected dataset, our platform provides users with powerful visualization and automatic analysis tools for RHEED, PL, and Raman spectra on DATA-UOS and DATA-POSTECH tabs. These tools offer valuable insights and facilitate easy data comprehension with a few clicks at each sample data. Furthermore, the platform enables easy comparison among different RHEED images and PL/Raman spectra. Upon selecting the desired measurements from the top of each data group page, corresponding samples that contain the relevant measurement data are listed. By clicking on the desired samples in the list, the associated data is displayed sequentially below. This comparison tool enables users to conveniently perform a simple data comparison and analysis directly on the webpage, enhancing overall user convenience. When specific data is selected from the list, detailed information about the sample first appears. By moving to the analysis tab on the left, measurement data and analysis tools are displayed.
Fig. 4 represents RHEED video of the DATA-UOS database with the ID number 2D-UOS-B00587, measured during the deposition of MoSe2 on graphene/SiC substrates. The visualization GUIs for the RHEED data consist of main and spectrum sections. The main section shows a guideline control panel, a list of thumbnails representing the uploaded RHEED images and videos, and an expanded view of the selected image or video [Fig. 4(a)]. The thumbnails are labeled with parsed time steps, enabling users to verify the timestep of each image directly. In the case of video data, a navigation bar is displayed alongside the expanded view, enabling users to confirm the RHEED patterns along the timesteps. In Fig. 4(b), we designed the spectrum section for users to facilitate the quantitative comparison and analysis of the RHEED images/videos with simple manipulation. A guideline is drawn as a blue line on the image/video in Fig. 4(a). On the left guideline control panel, users can determine the position and thickness of the guideline in the pixels and the direction of the horizontal and vertical lines. Users can also add some guidelines to several images at different timesteps, and the associated information is displayed as a table on top of the spectrum section, including the timestep, location, direction, and thickness, with an option to remove any guideline [upper panel of Fig. 4(b)]. Then, the brightness spectrum, the so-called line profile, is extracted along the guideline for a selected image, as shown in the middle of Fig. 4(b). The line profiles are depicted as colored lines on a graph accompanied by a legend that includes metadata information. The line profiles typically exhibit several peaks with a constant distance originating from the in-plane lattice periodicity of the materials, which means the distance between the peaks enables users to calculate the lattice spacing of the materials. We added a function that enables users to specify two points on the line profile and automatically calculate the distance between these points along both the x and y coordinates. Our RHEED visualization toolkit provides user-friendly functions for observing RHEED patterns over time and extracting line profiles through simple operations.
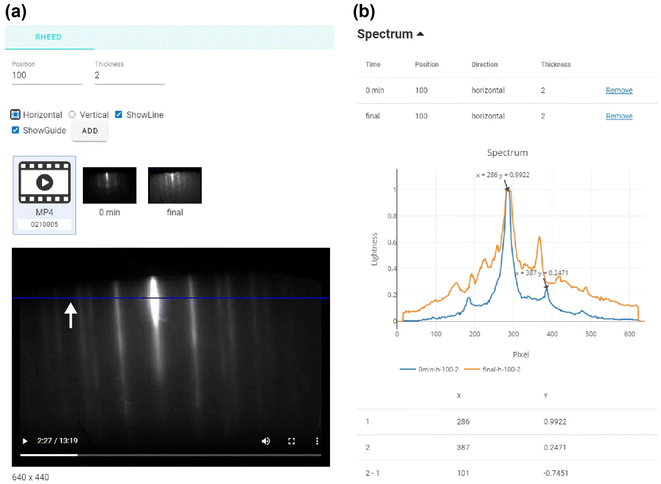 |
| Fig. 4 The visualization GUI for the RHEED data in the DATA-UOS group for the 2D-UOS-B00587 data. (a) The main section consists of a top-positioned guideline control panel and a thumbnail list beneath it, presenting an expanded view of the selected data. (b) The spectrum section exhibits a collection of metadata related to the guidelines added by users, along with their corresponding brightness spectra. | |
For video data, the guideline operates in a distinct manner. Once the guideline is added, the pixel information on the guideline is extracted, frame by frame, throughout the video. This information is then stacked in the direction perpendicular to the line, resulting in a singular image as shown in Fig. S1.† By scanning RHEED lines with the deposition time, changes in RHEED intensity and surface reconstructions can be easily seen.91 This resultant image is denoted with a prefix “stacking”, which is followed by details indicating its orientation (either horizontal or vertical), along with the position and thickness of the guideline, offering a summary of the parameters at a glance. Users can add guidelines to this stacked image, similar to adding them to other images.
Fig. 5 demonstrates the visualization GUI of the PL mapping data, one of the data types uploaded to the DATA-POSTECH group. The example data shown in Fig. 5 has an ID of 2D-POSTECH00128 and consists of PL spectra measured in the 505 to 771 nm range at a resolution of 60 × 60 points in a 20 × 20 μm2 area for a 1L-WS2 sample produced by the chemical vapor deposition method. The PL data consists of position-dependent spectra obtained by scanning a sample region with N × N points, where N is an integer. Each point has the PL spectra measured at a specific position, and the height, width, and the center energy of the PL peak provide significant optical properties of the material. As shown in Fig. 5(c) and (d), the color map for the height of the PL spectra and the histogram are displayed at a given wavelength, which can be modified using the panel located beneath the map. The lower section of the histogram includes a panel for adjusting the bin size and displays the mean and standard deviation of data.
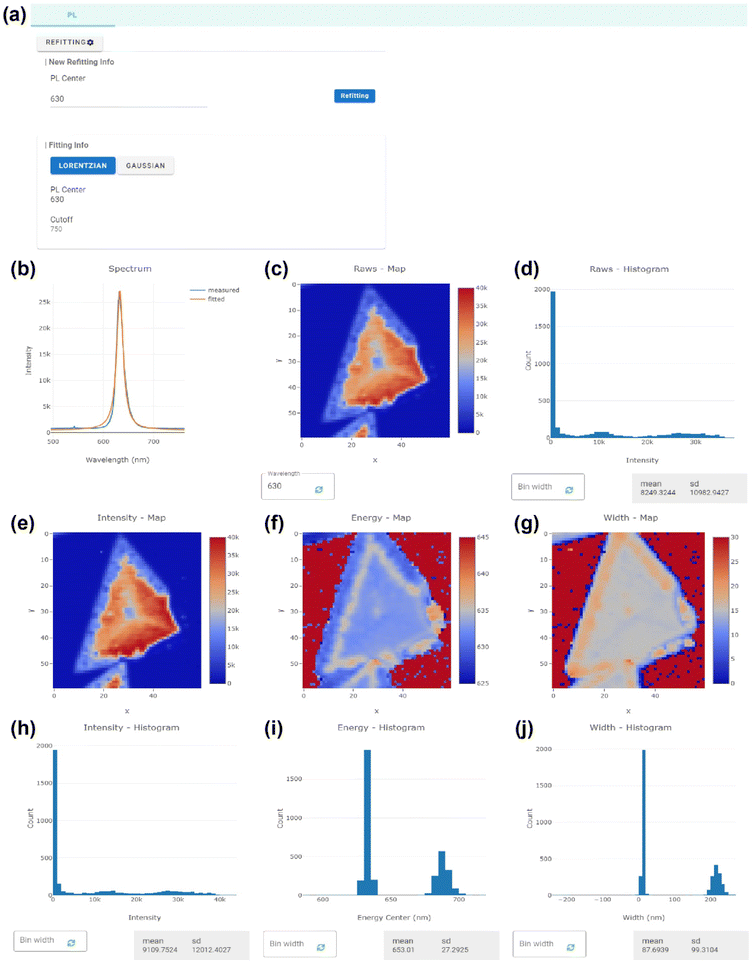 |
| Fig. 5 The visualization GUI for the PL (or Raman) mapping data in the DATA-POSTECH group for the 2D-POSTECH00128 data. The GUI consists of (a) a fitting control panel, (b) raw and fitted spectra, (c) raw intensity map, (d) raw intensity histogram, and (e)–(g) fitted parameter maps and (h)–(j) their corresponding histograms. (a) The panel enables users to adjust the fitting function type, initial fitting center, and baseline through the panel. (b) Raw spectra and fitting functions are visualized by selecting any pixel from the intensity map. (c) The intensity map of raw spectra is visualized at a specified wavelength, adjustable using the panel below. (d) The histogram exhibits intensity statistics at the given wavelength with the mean standard deviation values displayed below. (e)–(g) Maps present maximum intensity, energy of maximum intensity, and full with at half maximum of the fitting function, while (h)–(j) their respective statistics are shown in the histograms below. | |
To easily extract the information of the PL peak, the platform offers an automatic fitting analysis tool for the PL data. Users can determine the function form to be used for fitting, either Lorentzian or Gaussian function, through the control panel of fitting parameters shown in Fig. 5(a). The initial values for fitting the center and baseline cutoff can be specified along with the functional form. When clicking a pixel on the color maps, the raw spectra for a selected position and the corresponding fitted function are plotted together, as shown in Fig. 5(b). Fig. 5(e)–(j) show graphs related to the fitting parameters of the maximum intensity, peak energy (or wavelength), and full width at half maximum, respectively. Color maps of these values are presented in Fig. 5(e)–(g), and their range can be adjusted using the “Input Z range” button on the Plotly hover toolbar. Though the sample and substrate areas can be identified by examining the map at the peak wavelength of 630 nm in this case [Fig. 5(b)], utilizing the fitting parameter maps enables a more refined view of the data. The automated fitting results provide an overall change in the luminescence lines from the excitonic quasiparticles. In Fig. 5(e), the mapping image shows a drastically decreased PL intensity along the edge of the 1L-WS2. The growth procedure leads to a significantly higher defect density (such as sulfur vacancies) along the edge than in the interior part of the monolayer crystal.92,93 Interestingly, Fig. 5(f) shows the interfacial region between these two regions where the peak energy of the PL signal gradually decreases by ∼15 meV. This result can be potentially explained by the strain applied during the growth procedure.85,94 The histograms in Fig. 5(h)–(g) show the distribution of the three parameters. As with the statistics of the raw data, the bin size can be adjusted through the panel located below, and the mean and standard deviation of each distribution are provided. The Raman data is also shown in a similar form.
2.4 Machine learning toolkits
Based on the collected data, we used ML techniques to investigate the 2D film growth mechanism using RHEED videos and super-resolution techniques for analyzing PL/Raman spectra with a reduced time. While each toolkit is featured on the toolkit page with a brief introduction and links, they are not directly available on the web UI due to server resource limitations. The machine learning models are source-intensive, risking server instability if hosted directly. Instead, we provide the toolkits through a GitHub repository, where users can download the source code and examples, allowing for modifications and usage on individual workstations as needed.
2.4.1 RHEED data analysis.
Our previous work introduced an ML-assisted RHEED analysis technique that used principal component analysis (PCA) and K-means clustering to examine MBE grown 2D thin films on graphene substrates.82 The PCA models extract principal patterns from all RHEED image frames with a corresponding time evolution score plot and statistical significance. This technique provides researchers insight to interpret the growth process of the 2D film by deconvoluting the RHEED patterns. The ML-assisted method also provides a function called the modified PCA, filtering out the substrate contribution from the raw RHEED video. In 2D material growth on graphene where the substrate patterns mainly contribute, the modified PCA function enables users to obtain 2D film signals. K-means clustering categorizes the sequence of the RHEED images into multiple clusters based on their similarity without requiring intricate alterations to the RHEED images. This method identifies the transitional moments between different phases during the thin-film growth process. These ML-based RHEED analyses enable a quantitative comprehension of the growth dynamics of 2D materials and pave the way for advancing a sophisticated real-time monitoring system.
2.4.2 Super-resolution of spectrum mapping data.
Acquiring high-resolution mapping data is time-consuming with the measurement time increasing quadratically with the resolution. For example, a scan at a 120 × 120 resolution would require roughly 16 times longer than a scan at a 30 × 30 resolution. This exponential increase in time commitment can significantly hinder research productivity, particularly when multiple high-resolution scans are required. Consequently, developing a super-resolution model can dramatically enhance the efficiency and utility of the mapping techniques.
Our super-resolution toolkit is based on SwinIR, one of the state-of-the-art super-resolution models.95 The PL/Raman mapping data is transformed into a series of images via the integrated data processing pipeline. These images are then subjected to resolution enhancement using the model. The final step involves reassembling these high-resolution images into spectrum mapping data, which is then saved with a suffix indicating the total magnification (e.g., 4x). Further detailed data processing is given in the ESI.†
Fig. 6 shows an exemplary output from the super-resolution toolkit using the 2D-POSTECH00128 dataset. Each column presents data from a distinct wavelength, denoted at the top of the first column. The first row displays the original 60 × 60 resolution data, while the second row displays the input data, down-sampled to a 30 × 30 resolution to mimic the realistic lower resolution data typically encountered by the model. The input row shows a loss of detail, evidenced by the blurred dark spots at the edges of the sample. In contrast, the third row demonstrates the toolkit's enhanced output, elevating the resolution to 120 × 120. Despite some size exaggeration, the output row reveals the retrieval of the previously lost details and presents a more defined line traversing the sample. Remarkably, the transition from a 30 × 30 to a 120 × 120 resolution for 1600 images requires only about 30 seconds on a single RTX3090Ti card. This super resolution process is considerably faster compared to the several hours traditionally required to obtain data of this resolution experimentally, underscoring the efficacy and efficiency of the toolkit (See Fig. S2 for detailed benchmark results†).
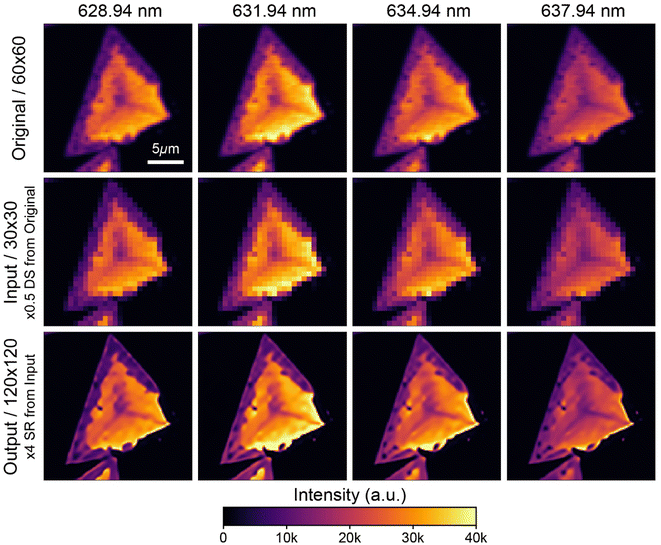 |
| Fig. 6 The result of the super-resolution applied to the photoluminescence (PL) mapping data. The original data is measured at a resolution of 60 × 60 (first row) and down-sampled to a resolution of 30 × 30 to create the input data (second row). The resolution is then enhanced to 120 × 120 (third row). The columns from the first to fourth present the intensity map of the PL spectra at specified wavelengths, as indicated at the top of the first column. | |
3 Discussion and outlook
Establishing a comprehensive data platform within the diverse field of materials science poses significant challenges. Each research area has its unique specificities, requiring platforms to be tailored to meet these particular needs. To this end, we have designed our platform to cater specifically to the field of 2D materials. This specialization provides unique data handling, visualization, and analysis tools that effectively serve the requirements of this research community. The predefined data templates and parsing systems for each data group effectively reduce manual efforts and enhance the robustness of the data. For efficient data management, the platform offers a flexible architecture for accommodating data growth, a standardized data format in JSON-type for further data processing, and a web-based data sharing system. While the platform and data are open for exploration and downloadable to all users, only authorized groups or individuals can upload data. This operation policy is to ensure consistent data quality available on the platform.
The two data groups described in this article, UOS and POSTECH, cover critical stages in the study of 2D materials, from the synthesis process to the physical properties and optical measurements. The RHEED data is expected to provide real-time insights into the physical properties and growth dynamics. The PL/Raman data can be utilized to analyze optical and vibrational characteristics, which can vary depending on the structural/chemical changes. The visualization and analysis functionalities provide various data and statistical visualization tools, spectrum and image analysis, and ML toolkits. These tools, designed through active dialogue with users, enable immediate data inspection and exploration upon uploading, offering a streamlined approach to data processing. Researchers sometimes face time-consuming manual visualization and analysis processes that require repetitive steps for every new dataset. Such operations often involve using standalone tools like MATLAB and Origin Lab, adding to the complexity and time expenditure. By contrast, our platform automates these processes and enables researchers to dive into their data analysis more directly and efficiently. The ML toolkits help to overcome the barriers often associated with implementing ML in research. We note that our entire dataset, consisting of over 250 samples for each of the RHEED and PL datasets, provides an abundant resource for comprehensively examining 2D materials synthesis and optical characteristics. Utilizing RHEED data from numerous compositions, we can construct the ML model to predict compositions or synthetic stages based on the RHEED pattern variations depending on synthetic processes. PL data for various 2D material samples, obtained with different laser powers and resolutions, can also reduce the measurement time and endeavors.
Reflecting on the solid foundation and notable achievements of our platform, we are motivated to extend and refine its capabilities to meet the dynamic and expanding needs of the 2D materials science community. Our parsers and visualizers are developed to facilitate the collection of synthetic conditions essential for ensuring sample reproducibility and identifying samples from analytical results. Initially, our platform's functionalities were designed through collaboration with targeted user groups from UOS and POSTECH, representing a specialized yet limited segment of our potential user base. Recognizing the diverse requirements of an expanding user community, we plan to enhance our UI and expand data collection. By integrating feedback from a broader user base, we aim to develop advanced parsers, visualizers, and ML toolkits to improve the user experience. Our platform is designed with a flexible architecture that enables easy expansion and incorporation of additional groups in the future. This flexibility will ensure that as the field of 2D materials continues to grow and evolve, the platform can adapt and keep updated on different physical properties obtained from different measurements, thus becoming an increasingly valuable resource for researchers. These strategies anticipate the future growth of the 2D materials field and ensure our platform evolves in parallel, ready to meet new challenges and opportunities.
Data availability
All datasets are available on our web-based platform, https://2dmat.chemdx.org/ (DOI: 10.23218/2dmat.chemdx). Users can preview the datasets using integrated visualization tools before individually downloading the raw data and its corresponding metadata. The data is licensed under CC-BY 4.0, and contributed data is owned by the respective contributors. The source code of the machine learning toolkits used in this work is available on GitHub repositories. Including links to these repositories, descriptions of each toolkit can be found on our platform's “toolkit” page (https://2DMat.ChemDX.org/toolkits). The page will be regularly updated to include any new toolkit developments.
Author contributions
J.-H. Y., H. C. and Y.-L. L. designed and directed the study, developed platform, and wrote the manuscript. H. A. and M.-H. J. manufactured the samples and H. K., T. K. and J. K. characterized the samples for the DATA-POSTECH group. H. J. K., T. G. R., Y. G. K., B. K. C. and Y. J. C. manufactured and characterized the samples for the DATA-UOS group. J. K., Y. J. C. and Y.-L. L., revised the manuscript. All authors approved the final version of the manuscript for submission.
Conflicts of interest
There are no conflicts of interest to declare.
Acknowledgements
This study was supported by a major research project from the Korea Research Institute of Chemical Technology (KK2351-10). We acknowledge Virtual Lab Inc. for technical assistance. H. K., T. K, H. A., M.-H. J., and J. K. acknowledge the support of the MSIT (Ministry of Science and ICT), Korea, under the ITRC (Information Technology Research Center) support program (IITP-2023-RS-2022-00164799) supervised by the IITP (Institute for Information & Communications Technology Planning & Evaluation). H. J. K., T. G. R., Y. G. K., B. K. C., and Y. J. C. acknowledge the support from the National Research Foundation of Korea (NRF) grant funded by Korean government (NRF-2020R1A2C200373211, NRF-2021R1A6A3A14040322, RS-2023-00244143, RS-2023-00220471, RS-2023-00284081), and Korea Ministry of Land, Infrastructure and Transport (MOLIT) as Innovative Talent Education Program for Smart City.
References
- R. Gómez-Bombarelli, J. Aguilera-Iparraguirre, T. D. Hirzel, D. Duvenaud, D. Maclaurin, M. A. Blood-Forsythe, H. S. Chae, M. Einzinger, D.-G. Ha, T. Wu, G. Markopoulos, S. Jeon, H. Kang, H. Miyazaki, M. Numata, S. Kim, W. Huang, S. I. Hong, M. Baldo, R. P. Adams and A. Aspuru-Guzik, Design of efficient molecular organic light-emitting diodes by a high-throughput virtual screening and experimental approach, Nat. Mater., 2016, 15, 1120–1127 CrossRef PubMed.
- L. Goswami, M. K. Deka and M. Roy, Artificial Intelligence in Material Engineering: A Review on Applications of Artificial Intelligence in Material Engineering, Adv. Eng. Mater., 2023, 25, 2300104 CrossRef CAS.
- J. E. Gubernatis and T. Lookman, Machine learning in materials design and discovery: Examples from the present and suggestions for the future, Phys. Rev. Mater., 2018, 2, 120301 CrossRef CAS.
- C. Li and K. Zheng, Methods, progresses, and opportunities of materials informatics, InfoMat, 2023, 5(8), e12425 CrossRef.
- G. Pan, F. Wang, C. Shang, H. Wu, G. Wu, J. Gao, S. Wang, Z. Gao, X. Zhou and X. Mao, Advances in machine learning- and artificial intelligence-assisted material design of steels, Int. J. Miner., Metall. Mater., 2023, 30, 1003–1024 CrossRef.
- P. Raccuglia, K. C. Elbert, P. D. F. Adler, C. Falk, M. B. Wenny, A. Mollo, M. Zeller, S. A. Friedler, J. Schrier and A. J. Norquist, Machine-learning-assisted materials discovery using failed experiments, Nature, 2016, 533, 73–76 CrossRef CAS PubMed.
- R. Ramprasad, R. Batra, G. Pilania, A. Mannodi-Kanakkithodi and C. Kim, Machine learning in materials informatics: recent applications and prospects, npj Comput. Mater., 2017, 3, 54 CrossRef.
- R. K. Vasudevan, K. Choudhary, A. Mehta, R. Smith, G. Kusne, F. Tavazza, L. Vlcek, M. Ziatdinov, S. V. Kalinin and J. Hattrick-Simpers, Materials science in the artificial intelligence age: high-throughput library generation, machine learning, and a pathway from correlations to the underpinning physics, MRS Commun., 2019, 9, 821–838 CrossRef CAS.
- H. Wang, T. Fu, Y. Du, W. Gao, K. Huang, Z. Liu, P. Chandak, S. Liu, P. Van Katwyk, A. Deac, A. Anandkumar, K. Bergen, C. P. Gomes, S. Ho, P. Kohli, J. Lasenby, J. Leskovec, T.-Y. Liu, A. Manrai, D. Marks, B. Ramsundar, L. Song, J. Sun, J. Tang, P. Veličković, M. Welling, L. Zhang, C. W. Coley, Y. Bengio and M. Zitnik, Scientific discovery in the age of artificial intelligence, Nature, 2023, 620, 47–60 CrossRef CAS.
- L. Xu, S. Zhang, X. Li, M. Tang, P. Xie and X. Hong, Towards Data-Driven Design of Asymmetric Hydrogenation of Olefins: Database and Hierarchical Learning, Angew. Chem., Int. Ed., 2021, 60, 22804–22811 CrossRef CAS.
- Y. Zhang, X. He, Z. Chen, Q. Bai, A. M. Nolan, C. A. Roberts, D. Banerjee, T. Matsunaga, Y. Mo and C. Ling, Unsupervised discovery of solid-state lithium ion conductors, Nat. Commun., 2019, 10, 5260 CrossRef PubMed.
- P. Gao, A. Andersen, J. Sepulveda, G. U. Panapitiya, A. Hollas, E. G. Saldanha, V. Murugesan and W. Wang, SOMAS: a platform for data-driven material discovery in redox flow battery development, Sci. Data, 2022, 9, 740 CrossRef CAS PubMed.
- H. Gong, J. He, X. Zhang, L. Duan, Z. Tian, W. Zhao, F. Gong, T. Liu, Z. Wang, H. Zhao, W. Jia, L. Zhang, X. Jiang, W. Chen, S. Liu, H. Xiu, W. Yang and J. Wan, A repository for the publication and sharing of heterogeneous materials data, Sci. Data, 2022, 9, 787 CrossRef.
- J. Hu, S. Stefanov, Y. Song, S. S. Omee, S.-Y. Louis, E. M. D. Siriwardane, Y. Zhao and L. Wei, MaterialsAtlas.org: a materials informatics web app platform for materials discovery and survey of state-of-the-art, npj Comput. Mater., 2022, 8, 65 CrossRef.
- Y. A. Ivanenkov, D. Polykovskiy, D. Bezrukov, B. Zagribelnyy, V. Aladinskiy, P. Kamya, A. Aliper, F. Ren and A. Zhavoronkov, Chemistry42: An AI-Driven Platform for Molecular Design and Optimization, J. Chem. Inf. Model., 2023, 63, 695–701 CrossRef CAS.
- C. Kim, A. Chandrasekaran, T. D. Huan, D. Das and R. Ramprasad, Polymer Genome: A Data-Powered Polymer Informatics Platform for Property Predictions, J. Phys. Chem. C, 2018, 122, 17575–17585 CrossRef CAS.
- L. Talirz, S. Kumbhar, E. Passaro, A. V. Yakutovich, V. Granata, F. Gargiulo, M. Borelli, M. Uhrin, S. P. Huber, S. Zoupanos, C. S. Adorf, C. W. Andersen, O. Schütt, C. A. Pignedoli, D. Passerone, J. VandeVondele, T. C. Schulthess, B. Smit, G. Pizzi and N. Marzari, Materials Cloud, a platform for open computational science, Sci. Data, 2020, 7, 299 CrossRef.
- T. J. Jacobsson, A. Hultqvist, A. García-Fernández, A. Anand, A. Al-Ashouri, A. Hagfeldt, A. Crovetto, A. Abate, A. G. Ricciardulli, A. Vijayan, A. Kulkarni, A. Y. Anderson, B. P. Darwich, B. Yang, B. L. Coles, C. A. R. Perini, C. Rehermann, D. Ramirez, D. Fairen-Jimenez, D. Di Girolamo, D. Jia, E. Avila, E. J. Juarez-Perez, F. Baumann, F. Mathies, G. S. A. González, G. Boschloo, G. Nasti, G. Paramasivam, G. Martínez-Denegri, H. Näsström, H. Michaels, H. Köbler, H. Wu, I. Benesperi, M. I. Dar, I. Bayrak Pehlivan, I. E. Gould, J. N. Vagott, J. Dagar, J. Kettle, J. Yang, J. Li, J. A. Smith, J. Pascual, J. J. Jerónimo-Rendón, J. F. Montoya, J.-P. Correa-Baena, J. Qiu, J. Wang, K. Sveinbjörnsson, K. Hirselandt, K. Dey, K. Frohna, L. Mathies, L. A. Castriotta, M. H. Aldamasy, M. Vasquez-Montoya, M. A. Ruiz-Preciado, M. A. Flatken, M. V. Khenkin, M. Grischek, M. Kedia, M. Saliba, M. Anaya, M. Veldhoen, N. Arora, O. Shargaieva, O. Maus, O. S. Game, O. Yudilevich, P. Fassl, Q. Zhou, R. Betancur, R. Munir, R. Patidar, S. D. Stranks, S. Alam, S. Kar, T. Unold, T. Abzieher, T. Edvinsson, T. W. David, U. W. Paetzold, W. Zia, W. Fu, W. Zuo, V. R. F. Schröder, W. Tress, X. Zhang, Y.-H. Chiang, Z. Iqbal, Z. Xie and E. Unger, An open-access database and analysis tool for perovskite solar cells based on the FAIR data principles, Nat. Energy, 2021, 7, 107–115 CrossRef.
- P. Shetty and R. Ramprasad, Automated knowledge extraction from polymer literature using natural language processing, iScience, 2021, 24, 101922 CrossRef CAS PubMed.
- P. Shetty and R. Ramprasad, Machine-Guided Polymer Knowledge Extraction Using Natural Language Processing: The Example of Named Entity Normalization, J. Chem. Inf. Model., 2021, 61, 5377–5385 CrossRef CAS.
- P. Shetty, A. C. Rajan, C. Kuenneth, S. Gupta, L. P. Panchumarti, L. Holm, C. Zhang and R. Ramprasad, A general-purpose material property data extraction pipeline from large polymer corpora using natural language processing, npj Comput. Mater., 2023, 9, 52 CrossRef.
- Y. Katsura, M. Kumagai, T. Kodani, M. Kaneshige, Y. Ando, S. Gunji, Y. Imai, H. Ouchi, K. Tobita, K. Kimura and K. Tsuda, Data-driven analysis of electron relaxation times in PbTe-type thermoelectric materials, Sci. Technol. Adv. Mater., 2019, 20, 511–520 CrossRef CAS.
- S. Curtarolo, W. Setyawan, S. Wang, J. Xue, K. Yang, R. H. Taylor, L. J. Nelson, G. L. W. Hart, S. Sanvito, M. Buongiorno-Nardelli, N. Mingo and O. Levy, AFLOWLIB.ORG: A distributed materials properties repository from high-throughput ab initio calculations, Comput. Mater. Sci., 2012, 58, 227–235 CrossRef CAS.
- K. Choudhary, K. F. Garrity, A. C. E. Reid, B. DeCost, A. J. Biacchi, A. R. Hight Walker, Z. Trautt, J. Hattrick-Simpers, A. G. Kusne, A. Centrone, A. Davydov, J. Jiang, R. Pachter, G. Cheon, E. Reed, A. Agrawal, X. Qian, V. Sharma, H. Zhuang, S. V. Kalinin, B. G. Sumpter, G. Pilania, P. Acar, S. Mandal, K. Haule, D. Vanderbilt, K. Rabe and F. Tavazza, The joint automated repository for various integrated simulations (JARVIS) for data-driven materials design, npj Comput. Mater., 2020, 6, 173 CrossRef.
- A. Jain, S. P. Ong, G. Hautier, W. Chen, W. D. Richards, S. Dacek, S. Cholia, D. Gunter, D. Skinner, G. Ceder and K. A. Persson, Commentary: The Materials Project: A materials genome approach to accelerating materials innovation, APL Mater., 2013, 1, 011002 CrossRef.
- M. Yao, Y. Wang, X. Li, Y. Sheng, H. Huo, L. Xi, J. Yang and W. Zhang, Materials informatics platform with three dimensional structures, workflow and thermoelectric applications, Sci. Data, 2021, 8, 236 CrossRef CAS PubMed.
- C. Draxl and M. Scheffler, NOMAD: The FAIR concept for big data-driven materials science, MRS Bull., 2018, 43, 676–682 CrossRef.
- C. Draxl and M. Scheffler, The NOMAD laboratory: from data sharing to artificial
intelligence, J. Phys.: Mater., 2019, 2, 036001 CAS.
- S. Kirklin, J. E. Saal, B. Meredig, A. Thompson, J. W. Doak, M. Aykol, S. Rühl and C. Wolverton, The Open Quantum Materials Database (OQMD): assessing the accuracy of DFT formation energies, npj Comput. Mater., 2015, 1, 15010 CrossRef CAS.
- J. E. Saal, S. Kirklin, M. Aykol, B. Meredig and C. Wolverton, Materials Design and Discovery with High-Throughput Density Functional Theory: The Open Quantum Materials Database (OQMD), JOM, 2013, 65, 1501–1509 CrossRef CAS.
- P. Gorai, D. Gao, B. Ortiz, S. Miller, S. A. Barnett, T. Mason, Q. Lv, V. Stevanović and E. S. Toberer, TE Design Lab: A virtual laboratory for thermoelectric material design, Comput. Mater. Sci., 2016, 112, 368–376 CrossRef.
- X. Bai, Q. Jiang, S. Lu, P. Song, Z. Jia, P. Shan, Y. Chen, H. Cui, R. Feng, Z. Liang, Q. Kang, Y. Wang, N. Zhou and H. Yuan, Multielement Magnesium-Based Alloys via Machine Learning Screening for Fuel Cell Bipolar Plates, J. Phys. Chem. C, 2023, 127, 16162–16174 CrossRef CAS.
- N. Fu, J. Hu, Y. Feng, G. Morrison, H. zur Loye and J. Hu, Composition Based Oxidation State Prediction of Materials Using Deep Learning Language Models, Advanced Science, 2023, 13, 2301011 CrossRef PubMed.
- H. Jin, X. Tan, T. Wang, Y. Yu and Y. Wei, Discovery of Two-Dimensional Multinary Component Photocatalysts Accelerated by Machine Learning, J. Phys. Chem. Lett., 2022, 13, 7228–7235 CrossRef CAS PubMed.
- G. S. Na, S. Jang, Y.-L. Lee and H. Chang, Tuplewise Material Representation Based Machine Learning for Accurate Band Gap Prediction, J. Phys. Chem. A, 2020, 124, 10616–10623 CrossRef CAS PubMed.
- D. Sauceda, P. Singh, G. Ouyang, O. Palasyuk, M. J. Kramer and R. Arróyave, High throughput exploration of the oxidation landscape in high entropy alloys, Mater. Horiz., 2022, 9, 2644–2663 RSC.
- M. C. Sorkun, S. Astruc, J. M. V. A. Koelman and S. Er, An artificial intelligence-aided virtual screening recipe for two-dimensional materials discovery, npj Comput. Mater., 2020, 6, 106 CrossRef.
- H. Zhang, Z. Wang, J. Cai, S. Wu and J. Li, Machine-Learning-Enabled Tricks of the Trade for Rapid Host Material Discovery in Li–S Battery, ACS Appl. Mater. Interfaces, 2021, 13, 53388–53397 CrossRef CAS PubMed.
- Y.-L. Lee, H. Lee, S. Jang, J. Shin, T. Kim, S. Byun, I. Chung, J. Im and H. Chang, TEXplorer.org: Thermoelectric material properties data platform for experimental and first-principles calculation results, APL Mater., 2023, 11, 41111 CrossRef CAS.
- L. Chanussot, A. Das, S. Goyal, T. Lavril, M. Shuaibi, M. Riviere, K. Tran, J. Heras-Domingo, C. Ho, W. Hu, A. Palizhati, A. Sriram, B. Wood, J. Yoon, D. Parikh, C. L. Zitnick and Z. Ulissi, Open Catalyst 2020 (OC20) Dataset and Community Challenges, ACS Catal., 2021, 11, 6059–6072 CrossRef CAS.
- K. Takahashi, J. Ohyama, S. Nishimura, J. Fujima, L. Takahashi, T. Uno and T. Taniike, Catalysts informatics: paradigm shift towards data-driven catalyst design, Chem. Commun., 2023, 59, 2222–2238 RSC.
- R. Tran, J. Lan, M. Shuaibi, B. M. Wood, S. Goyal, A. Das, J. Heras-Domingo, A. Kolluru, A. Rizvi, N. Shoghi, A. Sriram, F. Therrien, J. Abed, O. Voznyy, E. H. Sargent, Z. Ulissi and C. L. Zitnick, The Open Catalyst 2022 (OC22) Dataset and Challenges for Oxide Electrocatalysts, ACS Catal., 2023, 13, 3066–3084 CrossRef CAS.
- S. Ali, A. Raza, A. M. Afzal, M. Waqas, M. Hussain, M. Imran, M. A. Assiri, S. Ali, A. M. Afzal, M. W. Iqbal, A. Raza, M. Hussain, M. Imran and M. A. Assiri, Recent Advances in 2D-MXene Based Nanocomposites for Optoelectronics, Adv. Mater. Interfaces, 2022, 9, 2200556 CrossRef CAS.
- M. Boota, B. Anasori, C. Voigt, M.-Q. Zhao, M. W. Barsoum, Y. Gogotsi, M. B. Boota, C. Anasori, M.-Q. Voigt, M. W. Zhao, Y. Barsoum and A. J. Gogotsi, Pseudocapacitive Electrodes Produced by Oxidant-Free Polymerization of Pyrrole between the Layers of 2D Titanium Carbide (MXene), Adv. Mater., 2016, 28, 1517–1522 CrossRef CAS PubMed.
- D. W. Park, J. P. Ness, S. K. Brodnick, C. Esquibel, J. Novello, F. Atry, D. H. Baek, H. Kim, J. Bong, K. I. Swanson, A. J. Suminski, K. J. Otto, R. Pashaie, J. C. Williams and Z. Ma, Electrical Neural Stimulation and Simultaneous in Vivo Monitoring with Transparent Graphene Electrode Arrays Implanted in GCaMP6f Mice, ACS Nano, 2018, 12, 148–157 CrossRef CAS PubMed.
- I. Roh, S. H. Goh, Y. Meng, J. S. Kim, S. Han, Z. Xu, H. E. Lee, Y. Kim and S.-H. Bae, Applications of remote epitaxy and van der Waals epitaxy, Nano Convergence, 2023, 10, 20 CrossRef CAS PubMed.
- C. Xie, Y. Wang, Z.-X. Zhang, D. Wang and L.-B. Luo, Graphene/Semiconductor Hybrid Heterostructures for Optoelectronic Device Applications, Nano Today, 2018, 19, 41–83 CrossRef CAS.
- Z. Zhang, Y. Lee, M. F. Haque, J. Leem, E. Y. Hsieh and S. Nam, Plasmonic sensors based on graphene and graphene hybrid materials, Nano Convergence, 2022, 9, 28 CrossRef CAS PubMed.
- M. Chhowalla, H. S. Shin, G. Eda, L.-J. Li, K. P. Loh and H. Zhang, The chemistry of two-dimensional layered transition metal dichalcogenide nanosheets, Nat. Chem., 2013, 5, 263–275 CrossRef PubMed.
- D. Guo, X. Song, L. Tan, H. Ma, W. Sun, H. Pang, L. Zhang and X. Wang, A facile dissolved and reassembled strategy towards sandwich-like rGO@NiCoAl-LDHs with excellent supercapacitor performance, Chem. Eng. J., 2019, 356, 955–963 CrossRef CAS.
- P. Ranjan, S. Gaur, H. Yadav, A. B. Urgunde, V. Singh, A. Patel, K. Vishwakarma, D. Kalirawana, R. Gupta and P. Kumar, 2D materials: increscent quantum flatland with immense potential for applications, Nano Convergence, 2022, 9, 26 CrossRef CAS PubMed.
- J. Yan, C. E. Ren, K. Maleski, C. B. Hatter, B. Anasori, P. Urbankowski, A. Sarycheva and Y. Gogotsi, Flexible MXene/Graphene Films for Ultrafast Supercapacitors with Outstanding Volumetric Capacitance, Adv. Funct. Mater., 2017, 27, 1701264 CrossRef.
- B. Yang, C. Hao, F. Wen, B. Wang, C. Mu, J. Xiang, L. Li, B. Xu, Z. Zhao, Z. Liu and Y. Tian, Flexible Black-Phosphorus Nanoflake/Carbon Nanotube Composite Paper for High-Performance All-Solid-State Supercapacitors, ACS Appl. Mater. Interfaces, 2017, 9, 44478–44484 CrossRef CAS PubMed.
- R. Belgamwar, A. G. M. Rankin, A. Maity, A. K. Mishra, J. S. Gómez, J. Trébosc, C. P. Vinod, O. Lafon and V. Polshettiwar, Boron Nitride and Oxide Supported on Dendritic Fibrous Nanosilica for Catalytic Oxidative Dehydrogenation of Propane, ACS Sustain. Chem. Eng., 2020, 8, 16124–16135 CrossRef CAS.
- D. Le, T. B. Rawal and T. S. Rahman, Single-Layer MoS2 with Sulfur Vacancies: Structure and Catalytic Application, J. Phys. Chem. C, 2014, 118, 5346–5351 CrossRef CAS.
- H. Wang, X. Yang, W. Shao, S. Chen, J. Xie, X. Zhang, J. Wang and Y. Xie, Ultrathin Black Phosphorus Nanosheets for Efficient Singlet Oxygen Generation, J. Am. Chem. Soc., 2015, 137, 11376–11382 CrossRef CAS PubMed.
- Z. Hao, Y. Luo, C. Huang, Z. Wang, G. Song, Y. Pan, X. Zhao and S. Liu, An Intelligent Graphene-Based Biosensing Device for Cytokine Storm Syndrome Biomarkers Detection in Human Biofluids, Small, 2021, 17, 2101508 CrossRef CAS PubMed.
- Y. Lei, W. Zhao, Y. Zhang, Q. Jiang, J. He, A. J. Baeumner, O. S. Wolfbeis, Z. L. Wang, K. N. Salama and H. N. Alshareef, A MXene-Based Wearable Biosensor System for High-Performance In Vitro Perspiration Analysis, Small, 2019, 15, 1901190 CrossRef.
- M. V. Sulleiro, A. Dominguez-Alfaro, N. Alegret, A. Silvestri and I. J. Gómez, 2D Materials towards sensing technology: From fundamentals to applications, Sens. Bio-Sens. Res., 2022, 38, 100540 CrossRef.
- H. Yang, J. Zhou, J. Bao, Y. Ma, J. Zhou, C. Shen, H. Luo, M. Yang, C. Hou and D. Huo, A simple hydrothermal one-step synthesis of 3D-MoS2/rGO for the construction of sensitive enzyme-free hydrogen peroxide sensor, Microchem. J., 2021, 162, 105746 CrossRef CAS.
- J. Zhou, L. Shen, M. D. Costa, K. A. Persson, S. P. Ong, P. Huck, Y. Lu, X. Ma, Y. Chen, H. Tang and Y. P. Feng, 2DMatPedia, an open computational database of two-dimensional materials from top-down and bottom-up approaches, Sci. Data, 2019, 6, 86 CrossRef PubMed.
- F. A. Rasmussen and K. S. Thygesen, Computational 2D Materials Database: Electronic Structure of Transition-Metal Dichalcogenides and Oxides, J. Phys. Chem. C, 2015, 119, 13169–13183 CrossRef CAS.
- M. N. Gjerding, A. Taghizadeh, A. Rasmussen, S. Ali, F. Bertoldo, T. Deilmann, N. R. Knøsgaard, M. Kruse, A. H. Larsen, S. Manti, T. G. Pedersen, U. Petralanda, T. Skovhus, M. K. Svendsen, J. J. Mortensen, T. Olsen and K. S. Thygesen, Recent progress of the Computational 2D Materials Database (C2DB), 2d Mater, 2021, 8, 044002 CrossRef CAS.
- S. Haastrup, M. Strange, M. Pandey, T. Deilmann, P. S. Schmidt, N. F. Hinsche, M. N. Gjerding, D. Torelli, P. M. Larsen, A. C. Riis-Jensen, J. Gath, K. W. Jacobsen, J. Jørgen Mortensen, T. Olsen and K. S. Thygesen, The Computational 2D Materials Database: high-throughput modeling and discovery of atomically thin crystals, 2d Mater, 2018, 5, 042002 CrossRef CAS.
- D. Campi, N. Mounet, M. Gibertini, G. Pizzi and N. Marzari, Expansion of the Materials Cloud 2D Database, ACS Nano, 2023, 17, 11268–11278 CrossRef CAS PubMed.
- N. Mounet, M. Gibertini, P. Schwaller, D. Campi, A. Merkys, A. Marrazzo, T. Sohier, I. E. Castelli, A. Cepellotti, G. Pizzi and N. Marzari, Two-dimensional materials from high-throughput computational exfoliation of experimentally known compounds, Nat. Nanotechnol., 2018, 13, 246–252 CrossRef CAS PubMed.
- A. Bhattacharya, I. Timokhin, R. Chatterjee, Q. Yang and A. Mishchenko, Deep learning approach to genome of two-dimensional materials with flat electronic bands, npj Comput. Mater., 2023, 9, 101 CrossRef.
- X. Guo, S. Zhang, L. Kou, C.-Y. Yam, T. Frauenheim, Z. Chen and S. Huang, Data-driven pursuit of electrochemically stable 2D materials with basal plane activity toward oxygen electrocatalysis, Energy Environ. Sci., 2023, 13, 53303–53313 Search PubMed.
- L. Jin, H. Wang, H. Zhao, Y. Ji and Y. Li, Unfolding the structure-property relationships of Li2S anchoring on two-dimensional materials with high-throughput calculations and machine learning, J. Energy Chem., 2023, 82, 31–39 CrossRef CAS.
- G. M. Nascimento, E. Ogoshi, A. Fazzio, C. M. Acosta and G. M. Dalpian, High-throughput inverse design and Bayesian optimization of functionalities: spin splitting in two-dimensional compounds, Sci. Data, 2022, 9, 195 CrossRef PubMed.
- Y. Song, E. M. D. Siriwardane, Y. Zhao and J. Hu, Computational Discovery of New 2D Materials Using Deep Learning Generative Models, ACS Appl. Mater. Interfaces, 2021, 13, 53303–53313 CrossRef CAS PubMed.
- A. C. Rajan, A. Mishra, S. Satsangi, R. Vaish, H. Mizuseki, K.-R. Lee and A. K. Singh, Machine-Learning-Assisted Accurate Band Gap Predictions of Functionalized MXene, Chem. Mater., 2018, 30, 4031–4038 CrossRef CAS.
- D. Zagorac, H. Muller, S. Ruehl, J. Zagorac and S. Rehme, Recent developments in the Inorganic Crystal Structure Database: Theoretical crystal structure data and related features, J. Appl. Crystallogr., 2019, 52, 918–925 CrossRef CAS PubMed.
- Q. Chen, K. Yang, M. Liang, J. Kang, X. Yi, J. Wang, J. Li and Z. Liu, Lattice modulation strategies for 2D material assisted epitaxial growth, Nano Convergence, 2023, 10, 39 CrossRef CAS PubMed.
-
A. Ichimiya and P. I. Cohen, Reflection High-Energy Electron Diffraction, Cambridge University Press, 2004 Search PubMed.
- N. J. C. Ingle, A. Yuskauskas, R. Wicks, M. Paul and S. Leung, The structural analysis possibilities of reflection high energy electron diffraction, J. Phys. D Appl. Phys., 2010, 43, 133001 CrossRef.
- H. J. Kim, B. K. Choi, I. H. Lee, M. J. Kim, S.-H. Chun, C. Jozwiak, A. Bostwick, E. Rotenberg and Y. J. Chang, Electronic structure and charge-density wave transition in monolayer VS2, Curr. Appl. Phys., 2021, 30, 8–13 CrossRef.
- R. Kim, B. K. Choi, K. J. Lee, H. J. Kim, H. H. Lee, T. G. Rhee, Y. G. Khim, Y. J. Chang and S. H. Chang, Atomic arrangement of van der Waals heterostructures using X-ray scattering and crystal truncation rod analysis, Curr. Appl. Phys., 2023, 46, 70–75 CrossRef.
- G. Liang, L. Cheng, J. Zha, H. Cao, J. Zhang, Q. Liu, M. Bao, J. Liu and X. Zhai, In-situ quantification of the surface roughness for facile fabrications of atomically smooth thin films, Nano Res., 2022, 15, 1654–1659 CrossRef CAS.
- H. Liang, V. Stanev, A. G. Kusne, Y. Tsukahara, K. Ito, R. Takahashi, M. Lippmaa and I. Takeuchi, Application of machine learning to reflection high-energy electron diffraction images for automated structural phase mapping, Phys. Rev. Mater., 2022, 6, 063805 CrossRef CAS.
- J. Jo, Y. Tchoe, G.-C. Yi and M. Kim, Real-Time Characterization Using in situ RHEED Transmission Mode and TEM for Investigation of the Growth Behaviour of Nanomaterials, Sci. Rep., 2018, 8, 1694 CrossRef PubMed.
- H. J. Kim, M. Chong, T. G. Rhee, Y. G. Khim, M.-H. Jung, Y.-M. Kim, H. Y. Jeong, B. K. Choi and Y. J. Chang, Machine-learning-assisted analysis of transition metal dichalcogenide thin-film growth, Nano Convergence, 2023, 10, 10 CrossRef CAS PubMed.
- R. K. Vasudevan, A. Tselev, A. P. Baddorf and S. V. Kalinin, Big-data reflection high energy electron diffraction analysis for understanding epitaxial film growth processes, ACS Nano, 2014, 8, 10899–10908 CrossRef CAS PubMed.
- X. Wang, J. Choi, J. Yoo and Y. J. Hong, Unveiling the mechanism of remote epitaxy of crystalline semiconductors on 2D materials-coated substrates, Nano Convergence, 2023, 10, 1–14 CrossRef PubMed.
- Z. Liu, M. Amani, S. Najmaei, Q. Xu, X. Zou, W. Zhou, T. Yu, C. Qiu, A. G. Birdwell, F. J. Crowne, R. Vajtai, B. I. Yakobson, Z. Xia, M. Dubey, P. M. Ajayan and J. Lou, Strain and structure heterogeneity in MoS2 atomic layers grown by chemical vapour deposition, Nat. Commun., 2014, 5, 5246 CrossRef PubMed.
- K.-D. Park, O. Khatib, V. Kravtsov, G. Clark, X. Xu and M. B. Raschke, Hybrid Tip-Enhanced Nanospectroscopy and Nanoimaging of Monolayer WSe2 with Local Strain Control, Nano Lett., 2016, 16, 2621–2627 CrossRef CAS PubMed.
- Y. Kim and J. Kim, Near-field optical imaging and spectroscopy of 2D-TMDs, Nanophotonics, 2021, 10, 3397–3415 CrossRef CAS.
- K. Tanaka, K. Hachiya, W. Zhang, K. Matsuda and Y. Miyauchi, Machine-Learning Analysis to Predict the Exciton Valley Polarization Landscape of 2D Semiconductors, ACS Nano, 2019, 13, 12687–12693 CrossRef CAS PubMed.
- Y. Zheng, X. Wu, J. Liang, Z. Zhang, J. Jiang, J. Wang, Y. Huang, C. Tian, L. Wang, Z. Chen, C.-C. Chen, Y. Zheng, X. Wu, J. Liang, Z. Zhang, J. Jiang, J. Wang, Y. Huang, C. Tian, L. Wang, Z. Chen and C.-C. Chen, Downward Homogenized Crystallization for Inverted Wide-Bandgap Mixed-Halide Perovskite Solar Cells with 21% Efficiency and Suppressed Photo-Induced Halide Segregation, Adv. Funct. Mater., 2022, 32, 2200431 CrossRef CAS.
- J. Zhou, J. Cui, S. Du, Z. Zhao, J. Guo, S. Li, W. Zhang, N. Liu, X. Li, Q. Bai, Y. Guo, S. Mi, Z. Cheng, L. He, J. C. Nie, Y. Yang and R. Dou, A natural indirect-to-direct band gap transition in artificially fabricated MoS2 and MoSe2 flowers, Nanoscale, 2023, 15, 7792–7802 RSC.
- C. S. Gallinat, G. Koblmüller, F. Wu and J. S. Speck, Evaluation of threading dislocation densities in In- and N-face InN, J. Appl. Phys., 2010, 107, 53517 CrossRef.
- J. Lee, S. J. Yun, C. Seo, K. Cho, T. S. Kim, G. H. An, K. Kang, H. S. Lee and J. Kim, Switchable, Tunable, and Directable Exciton Funneling in Periodically Wrinkled WS2, Nano Lett., 2021, 21, 43–50 CrossRef CAS PubMed.
- M. R. Rosenberger, H.-J. Chuang, K. M. McCreary, C. H. Li and B. T. Jonker, Electrical Characterization of Discrete Defects and Impact of Defect Density on Photoluminescence in Monolayer WS 2, ACS Nano, 2018, 12, 1793–1800 CrossRef CAS PubMed.
- D. Verma, P. Kumar, S. Mukherjee, D. Thakur, C. V. Singh and V. Balakrishnan, Interplay between Thermal Stress and Interface Binding on Fracture of WS2 Monolayer with Triangular Voids, ACS Appl. Mater. Interfaces, 2022, 14, 16876–16884 CrossRef CAS PubMed.
-
J. Liang, J. Cao, G. Sun, K. Zhang, L. Van Gool and R. Timofte, in Proceedings of the 2021 IEEE/CVF International Conference on Computer Vision Workshops (ICCVW), IEEE, 2021, vol. 2021-October, pp. 1833–1844 Search PubMed.
Footnote |
† Electronic supplementary information (ESI) available: Detailed information on sample characterization; data processing method for super-resolution; data templates for 2D materials platform. See DOI: https://doi.org/10.1039/d3dd00243h |
|
This journal is © The Royal Society of Chemistry 2024 |
Click here to see how this site uses Cookies. View our privacy policy here.