Compositional transferability of deep potential in molten LiF–BeF2 and LaF3 mixtures: prediction of density, viscosity, and local structure†
Received
8th January 2024
, Accepted 25th March 2024
First published on 26th March 2024
Abstract
The accumulation of lanthanide fission products carries the risk of altering the structure and properties of the nuclear fuel carrier salt LiF–BeF2 (Flibe), thereby downgrading the operating efficiency and safety of the molten salt reactor. However, the condition-limited experimental measurements, spatiotemporal-limited first-principles calculations, and accuracy-limited classical dynamic simulations are unable to capture the precise local structure and reliable thermophysical properties of heterogeneous molten salts. Therefore, the deep potential (DP) of LaF3 and Flibe molten mixtures is developed here, and DP molecular dynamics simulations are performed to systemically study the densities, diffusion coefficients, viscosities, radial distribution functions and coordination numbers of multiple molten Flibe + xLaF3, the quantitative relationships between these properties and LaF3 concentration are investigated, and the potential structure–property relationships are analyzed. Eventually, the transferability of DP on molten Flibe + LaF3 with different formulations as well as the predictability of structures and properties are achieved at the nanometer spatial scale and the nanosecond timescale.
1. Introduction
The molten LiF–BeF2 (Flibe) eutectic salt has been considered as one of the most promising fuel carriers and coolants for molten salt reactors (MSRs), due to its lower neutron absorption, high thermal conductivity, large heat capacity, appropriate viscosity, and good tolerance for actinides or fission products.1,2 The thermodynamic properties and structural characteristics of molten Flibe have been extensively investigated by experimental and theoretical methods.3–11 It is suggested that the thermophysical properties of molten fluorides are significantly affected by impurities,12 so the impurity content should be strictly controlled in the application of MSRs. Lanthanide fluorides (LnF3), as main nuclear fission products,13 dissolved in molten Flibe, may affect its thermophysical properties, thereby affecting the thermal energy storage and transfer performance of carrier salts. Therefore, studying the thermodynamic properties of molten Flibe + LnF3 mixtures is critical to the design and development of MSRs, and the transport behavior of lanthanide products in molten Flibe is also essential to the daily operation of MSRs and the pyroprocessing of spent fuels.14 Experimental measurements of the structure and properties of Flibe + LnF3 molten salt are challenged by the high temperatures, toxicity, radioactivity, reactivity, corrosiveness, and data uncertainty. Particularly, the structural characteristics of multivalent cations are hardly accessed due to highly dynamic disorder and heterogeneity in a coordination environment.15
The molecular dynamics simulation technique has been developed rapidly as an alternative or auxiliary technology for experiments, making it possible to predict the macroscopic properties while revealing the microscopic structural information of molten salts.16,17 Classical molecular dynamics simulations based on empirical force field models have been applied to predict the thermodynamic properties of molten fluorides,18–23 whereas the accuracy depends on the expressive power of interionic potentials. In contrast, first-principles molecular dynamics (FPMD) simulations calculate the interionic forces on-the-fly and can achieve responses on the predictions of local structures and partial properties of molten salts with higher precision.24–29 However, the computationally intensive performance makes a typical FPMD simulation prohibitively expensive to run with larger, more complex, and longer times. Besides, the property calculations of viscosity, electrical and thermal conductivity are time-consuming, and the simulation box should be enlarged if the concentration range lanthanide fluoride is widened.30,31 In the age of artificial intelligence, several MD methods based on the machine-learning (ML) interatomic potentials have emerged and have been applied in molten salt systems.32–35 Among them, deep potential molecular dynamics (DPMD) eliminate the dilemma of accuracy versus spatiotemporal scales by using a sufficient function for describing the interionic interactions in a system.36 At present, the DPMD simulations have been successfully performed on molten chlorides37–39 and fluorides.40,41
In this work, LaF3 as a representative lanthanide fluoride is added into molten Flibe eutectic salt to investigate the macroscopic properties and microscopic structure of molten mixtures. Firstly, diverse FPMD simulations of molten Flibe + LaF3 are conducted at 823 K with small simulation cells under the complete consideration of the position of La. Secondly, the deep potential of molten Flibe + LaF3 is trained, verified, and tested at the same temperature by evaluating the root-mean-square errors (RMSEs) of energy and force. Based on the optimized deep potential, the DPMD simulations of multiple molten Flibe + xLaF3 are performed with an enlarged simulation cell and an extended simulation time to verify the transferability of the deep potential over wider concentration ranges. Thirdly, the densities, diffusion coefficients, and viscosities of molten Flibe + LaF3 as well as the radial distribution functions and coordination numbers are predicted. Furthermore, the concentration dependencies of structures and properties for molten Flibe + LaF3 are obtained. In summary, the development of a transferable deep potential model for Flibe + LaF3 fulfills the prediction of structural and thermophysical properties, which also provides insights into the understanding of the physiochemical behavior of lanthanide fission products in molten Flibe.
2. Computational methods
2.1. FPMD simulations
The pure rhombohedral Li2BeF4 crystal (a = b = c = 8.375 Å, a = b = g = 107.8°) composed of 12 LiF and 6 BeF2 (total 42 atoms) is modelled and heated to 823 K.42 Based on the stable configuration, the initial configuration of molten Flibe + LaF3 is built by adding one LaF3. The FPMD simulation is performed based on the density functional theory and Born–Oppenheimer approximation as implemented in the Vienna ab initio simulation package (VASP).43 The generalized gradient approximation (GGA) with the Perdew–Burke–Ernzerhof (PBE) exchange–correlation functional is used for simulations, and core electrons are approximated by pseudopotentials developed using the projector augmented wave method.44 The wave functions for valence electrons, F(2s22p5), Li(1s22s1), Be(1s22s2), and La (5s25p65d16s2) are expanded using the plane wave basis set with a cutoff energy of 650 eV. A 1 × 1 × 1 k-point mesh is chosen in FPMD simulations, which is a suitable compromise between computational accuracy and cost.45 At first, a short-time FPMD simulation within the NVT ensemble via a Nosé thermostat is conducted aiming to bring the Flibe + LaF3 system to the specified temperature. Then, 30
000 steps of simulations are carried out in the NPT ensemble with a time step of 1 fs using the Langevin thermostat to achieve preliminary equilibrium, and the cell volume and lattice parameters of the molten mixture are optimized by using the method of Parrinello and Rahman. Finally, the equilibrium configuration is used as an input of the following simulations, and a 30 ps FPMD simulation of molten Flibe + LaF3 (abbreviated as La1) are implemented within the NVT ensemble with the same time step. According to the last NVT configuration, the positions of Li or Be are manually exchanged with La to overcome the possible dynamic energy barriers while enriching sampling configurations. These new configurations are named by the number of Li (1–12) and Be (1–6), which are simulated by repeating the above NPT–NVT process, respectively.
2.2. Development of the deep potential
For establishing the deep potential (DP) of molten Flibe + LaF3 for DPMD simulations, the datasets are adopted from the above FPMD results. All the FPMD phase–space trajectories simulated under both NVT and NPT ensembles are collected in the datasets (total 62
700), which are shuffled and split by about 2
:
1
:
1 as training, validation, and testing datasets for machine learning as shown in Fig. 1. The DeepMD-kit (version 2.0.1) package, a scheme for neural network training and evaluation of atomic energies and forces, is used to train the DP for molten Flibe + LaF3.46 The deep potential smooth edition (DeepPot-SE) is selected, which incorporates comprehensive atomic configuration information, and the hard cutoff radius (rcut) is set to be 7.5 Å. The selected number of atoms in rcut is {46, 46, 46, 46}, and four hidden layers {25, 50, 100, 200} are included to construct the residual networks architecture between them. The fitting net of {240, 240, 240, 240} is selected, which takes descriptors as inputs and predicts the atomic contribution to target properties.47 And, the decay rate and step are set as 0.95 and 5000, respectively. Meanwhile, the prefactors in the loss function are chosen as prefstart/limite = 0.02/1 and prefstart/limitf = 1000/1, and no virial is incorporated in both training and testing stages based on previous study.39
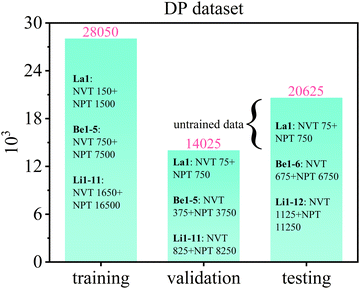 |
| Fig. 1 Training (28 050), validation (14 025), and testing (20 625) datasets of molten Flibe + LaF3 (La1, Be1–6, and Li1–12 represent 19 different initial configurations, for example, ‘La1: NVT 150 + NPT 1500’ represent 150 and 1500 samples from NVT and NPT simulations based on the initial La1 configuration, respectively, and the untrained data includes 6600 NVT and NPT data for Be6 and Li12). | |
2.3. DPMD simulations
The trained DP can be directly used in the large scale atomic/molecular massively parallel simulator (LAMMPS) via the interface of the DeePMD-kit.48 A larger 8050-atom box of Flibe + LaF3 (La175
:
175 La, 1050 Be, 2100 Li, and 4725 F) is simulated, corresponding to the composition of the above FPMD simulations, and the simulation temperature is also set to be 823 K, higher than the inlet temperature of MSR. In DPMD simulations, the system is first relaxed for 1 ns under NVT ensemble using the Nose–Hoover thermostat. Then, another 2 ns simulation under the NPT ensemble is carried out, from which the average volume is obtained to calculate the equilibrium density. As for obtaining the long-term related viscosity properties, the system is simulated within the NVT ensemble at the target temperature by a production run of 5 ns to achieve convergence of the stress tensor, and the ionic self-diffusion coefficients and radial distribution functions of key ionic pairs are also analyzed according to the NVT simulations. Furthermore, the concentration dependences of these properties and structures are accessed by executing repetitive simulations of Flibe + LaF3 systems with different LaF3 concentrations (see Table 1), referring experimental dosages in ref. 49. For all simulations, the periodic boundary conditions are employed, and Newton's equation of motion is solved with a time step of 1 fs using the Verlet algorithm.
Table 1 Composition profile of multiple Flibe + LaF3 systems (the system is abbreviated as Lax, x represents the number of La ions, mol% and wt% represents the mole and mass fraction of LaF3, respectively, BeF2, LiF, and Total represent the corresponding particle numbers)
System |
mol% |
wt% |
LaF3 |
BeF2 |
LiF |
Total |
La6 |
0.19 |
1.12 |
6 |
1050 |
2100 |
7374 |
La18 |
0.57 |
3.28 |
18 |
1050 |
2100 |
7422 |
La36 |
1.13 |
6.36 |
36 |
1050 |
2100 |
7494 |
La76 |
2.36 |
12.54 |
76 |
1050 |
2100 |
7654 |
La175 |
5.26 |
24.84 |
175 |
1050 |
2100 |
8050 |
3. Results and discussion
3.1. Performance of DP
3.1.1. RMSEs of energy and force.
The RMSEs of energy and force evaluated from validation datasets of molten Flibe + 5.26 mol% LaF3 (La175) after the training stage are depicted in Fig. S1 of the ESI,† where the standard deviations of RMSEs of energy and force in the last 5000 training steps are less than 1%. It is suggested the continuous stabilization and equilibrium of the DP training has been achieved, and 1000
000 training steps are enough to reach convergence criteria.37 At the testing stage, the trained DP is further examined by FPMD results as shown in Fig. 2, where all the ratios of energy and force between FPMD and DPMD are close to unity, confirming the excellent accuracy of this DP for reproducing energy and force of molten Flibe + LaF3. A new dataset with the consideration of trained and untrained trajectories is separately used as testing dataset and yields weighted RMSEs of energy (2.0 meV per atoms) and force (3.6 × 10−2 eV Å−1) for molten Flibe + LaF3. The deviations of RMSEs between validation and testing datasets are within the acceptable range, suggesting no occurrence of overfitting in the DP training.50
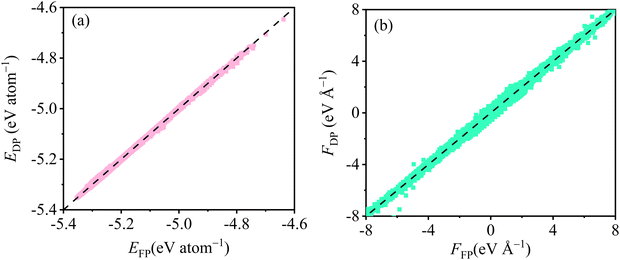 |
| Fig. 2 DPMD (simplified to subscript DP) and FPMD (simplified to subscript FP) predicted (a) energy E and (b) force F for molten Flibe + LaF3 (5.26 mol%). | |
3.1.2. RDFs and MSDs.
The radial distribution functions (RDFs) of molten Flibe + LaF3 (5.26 mol%) from DPMD simulations are compared with those of FPMD results, where the RDF curves of FPMD are evaluated from the average result of 19 different initial configurations as discussed in Section 2.1. In Fig. 3, the RDF curves of DPMD are obviously smoother due to the larger simulation cell, making it easy to identify peak positions and coordination numbers. Besides, all the DPMD RDFs are higher than those of FPMD due to the more particle numbers. The first peak positions (R1) and corresponding coordination numbers (CN1) of Be–F, Li–F and F–F from DPMD agree well with those from FPMD simulations, indicating that the robust DP is able to accurately predict the local structures of molten Flibe + LaF3. From Fig. 4, all the mean square displacements (MSDs) at 823 K follow upward trends as time increases; therefore, the diffusion coefficient (D) from both FPMD (30 ps) and DPMD (5 ns) can be derived from the slope of MSDs with respect to time based on the Einstein equation. Both the orders of D from FPMD and DPMD simulations are Li+ > F− > Be2+ > La3+, and the D values for the same ion are in the same order of magnitudes, consistent with the corresponding experimental and simulated results of pure molten Flibe.11,51 However, the DBe and DF are closer in large-scaled DPMD simulations, agreeing with the Maxwell–Stefan diffusivities,9 which is evident from the strong Be–F interaction. Overall, the reasonable results demonstrate the DP is well-represented and with expected accuracy comparable to those of FPMD results.
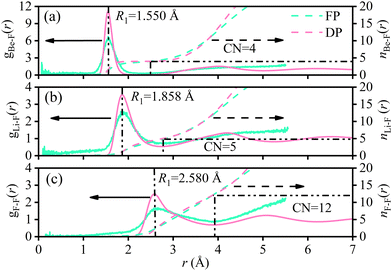 |
| Fig. 3 FPMD (color code: light green) and DPMD (color code: light pink) simulated RDFs for (a) Be–F, (b) Li–F, and (c) F–F of molten Flibe + LaF3 (5.26 mol%). | |
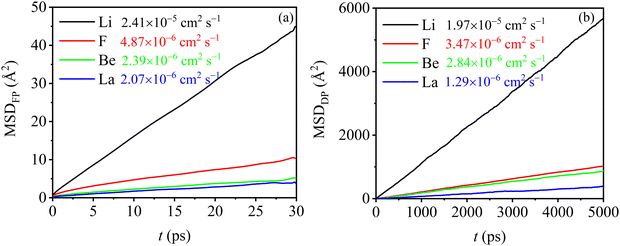 |
| Fig. 4 (a) FPMD and (b) DPMD simulated MSDs of molten Flibe + LaF3 (5.26 mol%) where the data after each ion are the corresponding ionic self-diffusion coefficient. | |
3.1.3. Densities and potential energies.
The density and potential energy per atom of molten Flibe + LaF3 at 823 K evaluated from DPMD simulations are displayed in Fig. 5. It is clear that the density linearly increases from 2.070 to 2.518 g cm−3 as the LaF3 concentration increases from 0.19 to 5.26 mol%, and all densities of molten Flibe + LaF3 are larger than that of pure molten Flibe (2.05 g cm−3).52 The similar phenomena also occur in the molten LiF–CaF2–LaF3 system, where the density increases from 2.071 to 2.423 g cm−3 at 1123 K as the LaF3 concentration increases from 0 to 5 mol%;53 but there is currently no comparable experimental density for molten Flibe + LaF3. It is indicated that the mass increment of molten Flibe caused by lanthanide fission products is larger than volume increment. Besides, the linear relationship between density and LaF3 concentration (cLa) can be expressed as shown in eqn (1), which is in excellent agreement with above experimental value of pure Flibe. | ρDP = 2.05 + 0.089cLa (mol%) | (1) |
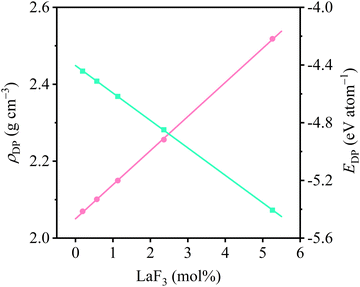 |
| Fig. 5 DPMD predicted densities (color code: light pink) and potential energies per atom (color code: light green) of molten Flibe + LaF3. | |
The potential energy per atom (EDP) of molten Flibe + LaF3 also linearly decreases from −4.4 to −5.4 eV per atom with the rising LaF3 concentration, attributed to the decreased total potential energy and increased atom numbers. The EDP–cLa relationship can be obtained as follows,
| EDP = −4.40 − 0.19cLa (mol%) | (2) |
It is thus concluded that the DP transferability of molten Flibe + LaF3 across different salt compositions satisfies the requirement for the accurate prediction of density and interaction energy. A molecular-level interpretation of the changes in properties with LaF3 concentration will be discussed in Section 3.3.1.
3.2. Transport properties
3.2.1. Self-diffusion coefficients.
As shown in Fig. 6, the ionic self-diffusion coefficients of multiple molten Flibe + LaF3 systems are estimated from the MSD results (see Fig. S2, ESI†). Obviously, the DLi is the largest due to its smallest ionic radius and molecular mass, and DLi is more than 5 times that of DF, hinting that the diffusivity of molten Flibe + LaF3 mainly depends on Li ions. The DF is similar to DBe but slightly larger than DBe, and the strong Be-F interaction leads to their synergistic movement, which will be further discussed in Section 3.3.2. Besides, the DLi, DF, and DBe are basically decreased with the increasing LaF3 concentration. The DLa is the lowest due to its highest mass among four ions, and significantly decreases from 4.0 to 1.3 × 10−6 cm2 s−1, agree with the change in the trend of DLa in molten FLiNaK, where DLa is decreased with increasing concentration when the temperature is below 973 K.54 Regretfully, no experimental DLa in molten FLiBe is available for direct comparison, and the diffusion behavior of lanthanide ions in similar molten salt systems can be used as references. The simulated diffusion coefficient of La3+ in molten Flibe at 823 K is consistent with that of Sm3+ (3.0 × 10−6 cm2 s−1) in molten Flibe,55 lower than that of Eu3+ (1.2 × 10−5 cm2 s−1) in molten Flibe,56 but higher than La3+ in the FLiNaK molten salt (1.8 × 10−7 cm2 s−1).57 Notably, the repulsion force between La ions is not included in the trained DP because only one La in each FPMD model (see Section 2.1) and the La-La interaction is not involved, which may lead to underestimation of DLa and this phenomenon is more prominent in higher concentration LaF3 systems. In addition, the weighted diffusion coefficient (
) of molten Flibe + LaF3 is calculated as, |  | (3) |
and the values are listed in Table 2. The
declines as the LaF3 concentration increases, and the linear relationship is as follows, | (10−6 cm2 s−1) = 10.79 − 0.59cLa | (4) |
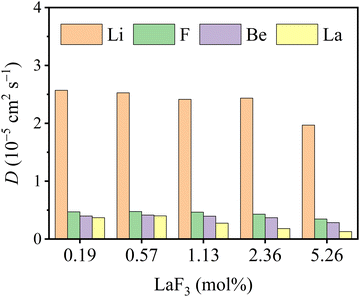 |
| Fig. 6 DPMD predicted ionic self-diffusion coefficients (D) vs. LaF3 concentrations of molten Flibe + LaF3. | |
Table 2 Weighted self-diffusion coefficients (D, 10−6 cm2 s−1), equilibrium volumes (V, Å3), viscosities (η, cP), nearest neighbor distances (Å) of Be–Be (R1Be–Be) and La–F (R1La–F), and coordination numbers of La–F (CN1La–F) for molten Flibe + LaF3 systems
System |
mol% |
|
V
|
η
|
R
1
Be–Be
|
R
1
La–F
|
CN1La–F |
La6 |
0.19 |
10.58 |
84 250 |
9.56 |
2.930 |
2.362 |
8.41 |
La18 |
0.57 |
10.48 |
84 854 |
9.58 |
2.930 |
2.328 |
8.51 |
La36 |
1.13 |
10.01 |
85 657 |
10.80 |
2.930 |
2.327 |
8.63 |
La76 |
2.36 |
9.69 |
87 383 |
11.66 |
2.950 |
2.320 |
8.82 |
La175 |
5.26 |
7.57 |
91 073 |
15.94 |
2.950 |
2.292 |
8.99 |
The fitted index R2 of
and cLa is good, about 0.98, meaning that the eqn (4) can be used to predict the weighted diffusion coefficient of molten Flibe + LaF3 with any LaF3 composition.
3.2.2. Shear viscosities.
The shear viscosity (η) of molten Flibe + LaF3 is calculated via the Green–Kubo (GK) method related to the auto-correlation function of off-diagonal components of the stress tensor (SACF),31 | 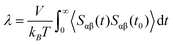 | (5) |
where V is the equilibrium volume of simulation model, kB the Boltzmann constant, Sαβ the stress tensor, the angle bracket denotes the average over all time origins t0, and t is the delay time of the SACF. Fig. 7 shows shear viscosities of multiple molten Flibe + LaF3 over time at 823 K, which increase rapidly before 5 ps, gradually level off after 10 ps, and thus the viscosities are assessed from the mean values between 10 and 15 ps. As summarized in Table 2, the viscosity almost linearly increases from 9.56 to 15.94 cP as rising LaF3 concentration, and therefore, the concentration dependence of shear viscosity can be presented as, | η(cP) = 9.08 + 1.27cLa | (6) |
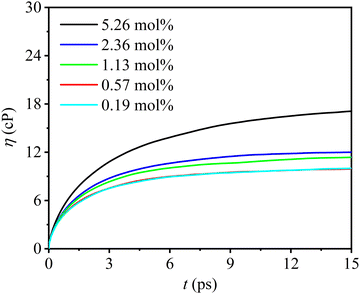 |
| Fig. 7 DPMD predicted shear viscosities (η) of molten Flibe + LaF3 where t is the correlation time. | |
The fitted index R2 is more than 0.98, meaning that the eqn (6) is practical to estimate the shear viscosities for different compositions of Flibe + xLaF3. According to eqn (6), the viscosity of pure Flibe at 823 K is calculated as 9.08 cP, lower than that of pure Flibe (11.5 cP for 66 LiF: 34 BeF2), but consistent with that of 73
:
27 Flibe (9.10 cP).3 The experimental viscosity of molten Flibe with the addition of lanthanide compounds is missing, but it can be confirmed that the introduction of high valence ions will increase the viscosity of the blank salt.58 In addition, structural factors or specific ion interactions that influence the viscosity trend will be further elaborated in Section 3.3.
3.3. Local structural descriptors
3.3.1. Nearest neighbor distances.
Fig. S3 (ESI†) plots the comparisons of RDFs among different LaF3 concentrations at the same temperature, where all the g(r) curves quickly converge to unity with a cutoff distance of 7 Å, indicating that these simulation cells are large enough to accommodate a system that shows intermediate range Coulomb interactions.59 The RDFs and integral curves of Be–F, Li–F, F–F, and Li–Li show minor changes with LaF3 concentration, while the short-range interaction of La–F and Be–Be are evidently different, from which it can be inferred that the density and potential energy (in Fig. 5) are mainly influenced by La–F and Be–Be interactions. Therefore, the RDFs and integral curves of Be–Be and La–F are further magnified in Fig. 8 in the range of 1–5 Å. According to Fig. S3 (ESI†), the R1 of Be–F, Li–F, F–F, and Li–Li are extracted as 1.550, 1.858, 2.580, and 3.118 Å. The average ionic radius of F− is evaluated as 1.290 Å, larger than its crystallographic radius of 1.246 Å in lanthanide fluorides,60 indicating that the repulsive force between F ions is enhanced after salt melting. From Fig. 8a, the peak height of Be–Be greatly increases with the concentration increments, while the peak position slightly right-shifts by about 0.02 Å as shown in Table 2, suggesting that the repulsive force between Be ions is slightly reduced. In Fig. 8b, the peak height prominently increases and peak position left-shifts from 2.362 to 2.292 Å along with the rising LaF3 concentration subjected to eqn (7), | R1La–F(Å) = 2.335 − 0.008cLa | (7) |
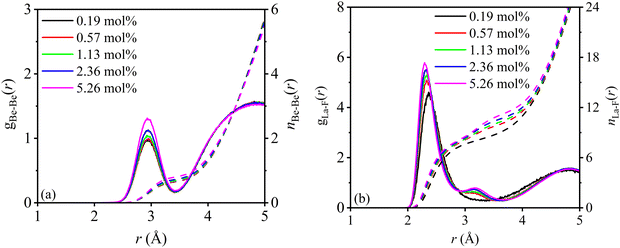 |
| Fig. 8 RDFs and their integral curves of (a) Be–Be and (b) La–F for molten Flibe + LaF3. | |
The fitted R2 index is relatively low (0.96), and the cLa cannot be zero. The shortening of La–F distance means the attraction force between two ions is enhanced; in other words, the dynamics of La ions to drag the surrounding F ions are weakened, which is more remarkable in high LaF3 concentration. Based on the La–F distances, the average ionic radius of La3+ is evaluated at 1.036 Å, similar to the crystallographic radius (1.050 Å) of La3+ when CN = 6, but significantly lower than the radius of 1.186 Å when CN = 9,60 from which it can be deduced that the electron clouds of F and La partially overlap accompanied by the salt melts. Overall, the reduced repulsive force between Be ions and enhanced attraction force between La and F ions are profitable for the structural stability of the molten system.
3.3.2. Coordination numbers.
Similarly, the CN1 of Be–F, Li–F, F–F, and Li–Li at the first shell layers remain almost unchanged with LaF3 concentration, and the average CNs are 4, 5, 12, and 8, respectively. It is demonstrated that the LiF54− clusters are network-type connected referring to the CN1 of Li–F and Li–Li, which is beneficial for the transport of Li ions. However, the CN1 of Be–Be is only 1 as shown in Fig. 8 and the Be–Be distance (2.94 Å) is less than twice the Be–F distance (3.10 Å), indicating that the BeF42− clusters in Flibe + LaF3 are chain-type and not network-type connected. The tight interaction between Be and F, stable tetrahedral configuration, and restricted transport path result in a much lower diffusion coefficient of Be compared to the Li cation. The CN1 of La–F (0.19 mol%) is 8.41 with a cutoff radius of 3.370 Å, larger than that of 6 in KF-rich molten fluorides.54 The CN1 of La–F is increased as the LaF3 concentration increases and the relationship is subjected to eqn (8). | CN1La–F(Å) = 8.367 + 0.255cLa − 0.026cLa2 | (8) |
The polynomial fitting index is up to 0.999, hinting that the CN1 of La–F basically reached the maximum at 5 mol% LaF3, and CN is maintained at 9 for more LaF3 addition. Of interest is that the RDF curves of 0.57–5.26 mol% Flibe + LaF3 are significantly different from that of low LaF3 concentration system, where another shoulder peak appears at 3.15 Å and gradually enhances as the concentration increases. Therefore, the average CN of La–F will be increased if the cutoff radius covers the second small peak, meaning that 1–2 F ions may be stably distributed beyond the first coordination shell of LaF85−, which is detrimental to the stability of the short-range ordered structure of molten Flibe + LaF3. It should be pointed that the DP is trained without considering the repulsion between La–La, which will underestimate the La–La interaction and even shorten the La–La distance, thereby changing the RDF and CN of La–F. Furthermore, the increased coordination number and complex coordination surrounding for La ions lead to a decrease in diffusivity (eqn (4)) and an increase in the viscosity (eqn (6)) of the molten Flibe + LaF3 system with the rising LaF3 concentration.
4. Conclusions
The DP of molten Flibe + LaF3 is developed for the first time based on machine learning methods and applied to investigate the LaF3 concentration dependences of density, diffusion coefficient, viscosity, and structure. The database with 62
700 frames (ergodic 19 initial configurations) is collected from FPMD simulations, and excellent agreements of energies and forces as well as RDFs and MSDs demonstrate the accuracy and predictability of trained DP. The LaF3 concentration dependences of density and potential energy are extremely linear, demonstrating the powerful transferability and flexibility of DP across different salt compositions. Furthermore, the ionic self-diffusion coefficients and shear viscosities of molten Flibe + LaF3 are predicted by DPMD simulations, the relationships of weighted diffusion coefficient and shear viscosity on LaF3 concentration are obtained, and the qualitative structure–property relationships are mined out. Finally, the DP provides more precise structural predictions over a wider concentration range, and the quantitative relationships between structural descriptors and concentration are accessed. Overall, these excellent results indicate the robustness of DPMD simulations, provide transferable DP for further studying other properties of Flibe with any LaF3 concentration, and give insights for property predictions of other molten salts.
Author contributions
X. Li: conceptualization, investigation, methodology, writing-original draft, review & editing, and visualization funding acquisition; T. Xu: methodology, investigation, and visualization; Y. Gong: supervision, project administration, funding acquisition.
Conflicts of interest
There are no conflicts to declare.
Acknowledgements
This work was supported by the Shanghai Pilot Program for Basic Research-Chinese Academy of Sciences, Shanghai Branch (JCYJ-SHFY-2021-003) and the Shanghai Sailing Program (23YF1457200).
References
- C. Griffard, S. G. Penoncello and J. C. Crepeau, Use of the Soft-Sphere Equation of State to predict the thermodynamic properties of the molten salt mixtures LiF–BeF2, NaF–BeF2, and KF–BeF2, Prog. Nucl. Energy, 2013, 68, 188–199 CrossRef CAS.
- B. Li, S. Dai and D. E. Jiang, First-principles molecular dynamics simulations of UCln-MgCl2 (n = 3, 4) molten salts, Phys. Chem. Chem. Phys., 2022, 24(39), 24281–24289 RSC.
- O. Y. Tkacheva, A. V. Rudenko, A. A. Kataev, P. N. Mushnikov, A. S. Kholkina and Y. P. Zaikov, The viscosity of molten salts based on the LiF–BeF2 system, Russ. J. Non-Ferr. Met., 2022, 63(3), 276–283 CrossRef.
- E. Il'ina, P. Mushnikov, S. Pershina, A. Rudenko, A. Redkin, Y. Zaikov, A. Kholkina and V. Voronin, Thermal properties of LiF–BeF2 and LiF–BeF2–UF4 systems as applied to molten salt reactor technologies, J. Mol. Liq., 2021, 344, 117731 CrossRef.
- A. L. Smith, E. Capelli, R. J. M. Konings and A. E. Gheribi, A new approach for coupled modelling of the structural and thermo-physical properties of molten salts. Case of a polymeric liquid LiF–BeF2, J. Mol. Liq., 2020, 299, 112165 CrossRef CAS.
- C. N. A. C. Z. Bahri, W. a M. Al-Areqi, M. I. F. M. Ruf and A. A. Majid, Characteristic of molten fluoride salt system LiF–BeF2 (Flibe) and LiF-NaF-KF (Flinak) as coolant and fuel carrier in molten salt reactor (MSR), AIP Conf. Proc., 2017, 1799(1), 040008 CrossRef.
- S. Liu, T. Su, J. Cheng, X. An, P. Zhang, H. Liu, S. Yao, L. Xie and H. Hou, Investigation on molecular structure of molten Li2BeF4 (FLiBe) salt by infrared absorption spectra and density functional theory (DFT), J. Mol. Liq., 2017, 242, 1052–1057 CrossRef CAS.
- J. Dai, H. Han, Q. Li and P. Huai, First-principle investigation of the structure and vibrational spectra of the local structures in LiF–BeF2 molten salts, J. Mol. Liq., 2016, 213, 17–22 CrossRef CAS.
- B. Chakraborty, Sign crossover in all Maxwell–Stefan diffusivities for molten salt LiF–BeF2: A molecular dynamics study, J. Phys. Chem. B, 2015, 119(33), 10652–10663 CrossRef CAS PubMed.
- B. S. Jabes, M. Agarwal and C. Chakravarty, Structure and transport properties of LiF–BeF2 mixtures: Comparison of rigid and polarizable ion potentials, J. Chem. Sci., 2012, 124(1), 261–269 CrossRef CAS.
- M. Salanne, C. Simon, P. Turq, R. J. Heaton and P. A. Madden, A first-principles description of liquid BeF2 and its mixtures with LiF:
2. Network formation in LiF–BeF2, J. Phys. Chem. B, 2006, 110(23), 11461–11467 CrossRef CAS PubMed.
- G. Zong, Z.-H. Cui, X.-G. Sun and J.-C. Xiao, One-step synthesis of high-purity Li2BeF4 molten salt, J. Fluor. Chem., 2016, 181, 30–35 CrossRef CAS.
- M. T. Robinson, W. A. B. Jr., S. A. Reynolds, H. W. Wright and T. H. Handley, The behavior of fission products in molten fluoride reactor fuels, Nucl. Sci. Eng., 1958, 4(3), 288–296 CrossRef CAS.
-
E. L. Compere; S. S. Kirslis; E. G. Bohlmann; F. F. Blankenship and W. R. Grimes, Fission product behavior in the molten salt reactor experiment; Oak Ridge National Laboratory, 1975.
- S. Roy, Y. Liu, M. Topsakal, E. Dias, R. Gakhar, W. C. Phillips, J. F. Wishart, D. Leshchev, P. Halstenberg, S. Dai, S. K. Gill, A. I. Frenkel and V. S. Bryantsev, A holistic approach for elucidating local structure, dynamics, and speciation in molten salts with high structural disorder, J. Am. Chem. Soc., 2021, 143(37), 15298–15308 CrossRef CAS PubMed.
-
M. P. Allen and D. J. Tildesley, Computer Simulation of
Liquids, Oxford University Press, New York, 2017; pp. 46–92 Search PubMed.
- E. A. Carter, Challenges in modeling materials properties without experimental input, Science, 2008, 321(5890), 800–803 CrossRef CAS PubMed.
- M. Salanne, C. Simon, P. Turq and P. A. Madden, Conductivit-viscosity-structure:
Unpicking the relationship in an Ionic Liquid, J. Phys. Chem. B, 2007, 111(18), 4678–4684 CrossRef CAS PubMed.
- O. Pauvert, M. Salanne, D. Zanghi, C. Simon, S. Reguer, D. Thiaudiere, Y. Okamoto, H. Matsuura and C. Bessada, Ion specific effects on the structure of molten AF-ZrF4 systems (A+ = Li+, Na+, and K+), J. Phys. Chem. B, 2011, 115(29), 9160 CrossRef CAS PubMed.
- L. C. Dewan, C. Simon, P. A. Madden, L. W. Hobbs and M. Salanne, Molecular dynamics simulation of the thermodynamic and transport properties of the molten salt fast reactor fuel LiF–ThF4, J. Nucl. Mater., 2013, 434(1–3), 322–327 CrossRef CAS.
- A. E. Gheribi, M. Salanne and P. Chartrand, Formulation of temperature-dependent thermal conductivity of NaF, β-Na3AlF6, Na5Al3F14, and molten Na3AlF6 supported by equilibrium molecular dynamics and density functional theory, J. Phys. Chem. C, 2016, 120(40), 22873–22886 CrossRef CAS.
- K. Machado, D. Zanghi, V. Sarou-Kanian, S. Cadars, M. Burbano, M. Salanne and C. Bessada, Study of NaF-AlF3 melts by coupling molecular dynamics, density functional theory, and NMR measurements, J. Phys. Chem. C, 2017, 121(19), 10289–10297 CrossRef CAS.
- C. Bessada, D. Zanghi, M. Salanne, A. Gil-Martin, M. Gibilaro, P. Chamelot, L. Massot, A. Nezu and H. Matsuura, Investigation of ionic local structure in molten salt fast reactor LiF-ThF4-UF4 fuel by EXAFS experiments and molecular dynamics simulations, J. Mol. Liq., 2020, 307, 112927 CrossRef CAS.
- R. J. Heaton, R. Brookes, P. A. Madden, M. Salanne, C. Simon and P. Turq, A first-principles description of liquid BeF2 and its mixtures with LiF: 1. Potential development and pure BeF2, J. Phys. Chem. B, 2006, 110, 11454–11460 CrossRef CAS PubMed.
- M. Salanne, C. Simon, P. Turq and P. A. Madden, Heat-transport properties of molten fluorides: Determination from first-principles, J. Fluor. Chem., 2009, 130(1), 38–44 CrossRef CAS.
- H. O. Nam, A. Bengtson, K. Vörtler, S. Saha, R. Sakidja and D. Morgan, First-principles molecular dynamics modeling of the molten fluoride salt with Cr solute, J. Nucl. Mater., 2014, 449(1), 148–157 CrossRef CAS.
- X. Lv, Z. Xu, J. Li, J. Chen and Q. Liu, First-principles molecular dynamics investigation on Na3AlF6 molten salt, J. Fluor. Chem., 2016, 185, 42–47 CrossRef CAS.
- H. Guo, J. Li, H. Zhang, T. Li, J. Luo, X. Yu, S. Wu and C. Zong, First-principles molecular dynamics investigation on KF-NaF-AlF3 molten salt system, Chem. Phys. Lett., 2019, 730, 587–593 CrossRef CAS.
- H. Wang, B. Yue, L. Yan, T. Jiang and S. Peng, First-principles molecular dynamics study of the behavior of tritium in molten LiF–BeF2 eutectic, J. Mol. Liq., 2022, 345, 117027 CrossRef CAS.
- M. Mouas, J. G. Gasser, S. Hellal, B. Grosdidier, A. Makradi and S. Belouettar, Diffusion and viscosity of liquid tin: Green-Kubo relationship-based calculations from molecular dynamics simulations, J. Chem. Phys., 2012, 136(9), 094501 CrossRef PubMed.
- Y. Zhang, A. Otani and E. J. Maginn, Reliable viscosity calculation from equilibrium molecular dynamics simulations: A time decomposition method, J. Chem. Theory Comput., 2015, 11(8), 3537 CrossRef CAS PubMed.
- G. P. P. Pun, R. Batra, R. Ramprasad and Y. Mishin, Physically informed artificial neural networks for atomistic modeling of materials, Nat. Commun., 2019, 10(1), 2339 CrossRef PubMed.
- A. P. Bartok, M. C. Payne, R. Kondor and G. Csanyi, Gaussian approximation potentials: the accuracy of quantum mechanics, without the electrons, Phys. Rev. Lett., 2010, 104(13), 136403 CrossRef PubMed.
- S. T. Lam, Q.-J. Li, R. Ballinger, C. Forsberg and J. Li, Modeling LiF and FLiBe molten salts with robust neural network interatomic potential, ACS Appl. Mater. Interfraces, 2021, 13(21), 24582–24592 CrossRef CAS PubMed.
- Q. J. Li, E. Küükbenli, S. Lam, B. Khaykovich and J. Li, Development of robust neural-network interatomic potential for molten salt, Cell Rep. Phys. Sci., 2021, 100359 CrossRef CAS.
- L. Zhang, J. Han, H. Wang, R. Car and W. E, Deep potential molecular dynamics: A scalable model with the accuracy of quantum mechanics, Phys. Rev. Lett., 2018, 120(14), 143001 CrossRef CAS PubMed.
- T. Feng, J. Zhao, W. Liang and G. Lu, Molecular dynamics simulations of lanthanum chloride by deep learning potential, Compt. Mater. Sci., 2022, 210, 111014 CrossRef.
- W. Liang, G. Lu and J. Yu, Machine-learning-driven simulations on microstructure and thermophysical properties of MgCl2–KCl eutectic, ACS Appl. Mater. Interfaces, 2021, 13(3), 4034–4042 CrossRef CAS PubMed.
- T. Xu, X. Li, Y. Wang and Z. Tang, Development of deep potentials of molten MgCl2–NaCl and MgCl2–KCl salts driven by machine learning, ACS Appl. Mater. Interfaces, 2023, 15, 14184–14195 CAS.
- R. Chahal, S. Roy, M. Brehm, S. Banerjee, V. Bryantsev and S. T. Lam, Transferable deep learning potential reveals intermediate-range ordering effects in LiF–NaF–ZrF4 molten salt, JACS Au, 2022, 2(12), 2693–2702 CrossRef CAS PubMed.
- A. Rodriguez, S. Lam and M. Hu, Thermodynamic and transport properties of LiF and FLiBe molten salts with deep learning potentials, ACS Appl. Mater. Interfaces, 2021, 13(46), 55367–55379 CrossRef CAS PubMed.
- A. Jain, S. P. Ong, G. Hautier, W. Chen, W. D. Richards, S. Dacek, S. Cholia, D. Gunter, D. Skinner and G. Ceder, Commentary: The Materials Project: A materials genome approach to accelerating materials innovation, APL Mater., 2013, 1(1), 011002 CrossRef.
- G. Kresse and D. Joubert, From ultrasoft pseudopotentials to the projector augmented-wave method, Phys. Rev. B: Condens. Matter Mater. Phys., 1999, 59(3), 1758–1775 CrossRef CAS.
- J. P. Perdew, K. Burke and M. Ernzerhof, Generalized gradient approximation made simple, Phys. Rev. Lett., 1996, 77(18), 3865–3868 CrossRef CAS PubMed.
- X. Li, T. Xu, M. Liu and Y. Zuo, Diffusion behaviors of HF in molten LiF–BeF2 and LiF–NaF–KF eutectics studied by FPMD simulations and electrochemical techniques, J. Nucl. Mater., 2022, 572, 154031 CrossRef CAS.
- H. Wang, L. Zhang, J. Han and W. E, DeePMD-kit: A deep learning package for many-body potential energy representation and molecular dynamics, Comput. Phys. Commun., 2018, 228, 178–184 CrossRef CAS.
-
K. He; X. Zhang; S. Ren and J. Sun In Deep residual learning for image recognition, Proceedings of the IEEE conference on computer vision and pattern recognition, 2016; 770–778.
- S. Plimpton, Fast parallel algorithms for short-range molecular dynamics, J. Comput. Phys., 1995, 117(1), 1–19 CrossRef CAS.
- Y. Wang, J. Ge, W. Zhuo, S. Guo and J. Zhang, Electrochemical separation study of LaF3 in molten FLiNaK salt, J. Nucl. Mater., 2019, 518, 162–165 CrossRef CAS.
- G. Pan, J. Ding, Y. Du, D.-J. Lee and Y. Lu, A DFT accurate machine learning description of molten ZnCl2 and its mixtures: 2. potential development and properties prediction of ZnCl2–NaCl–KCl ternary salt for CSP, Compt. Mater. Sci., 2021, 187, 110055 CrossRef CAS.
- H. Ohno, Characteristic diffusion phenomena of fluorine in molten LiF–BeF2 and NaF–BeF2 systems, ESC Proc., 1984, 31–43 CAS.
- A. Redkin, A. Khudorozhkova, E. Il'ina, S. Pershina, P. Mushnikov, A. Isakov, Y. Zaikov, A. Kataev and M. Laptev, Density and heat capacity of some molten mixtures in system LiF–BeF2–UF4, J. Mol. Liq., 2021, 341, 117215 CrossRef CAS.
- B. Kubíková, M. Boča, J. Mlynáriková, V. Gurišová, M. Šimurda, Z. Netriová and M. Korenko, Physicochemical properties of the (LiF + CaF2)eut + LaF3 system: Phase equilibria, volume properties, electrical conductivity, and surface tension, J. Chem. Eng. Data, 2016, 61(4), 1395–1402 CrossRef.
- Y. Wang, Q. Yang and J. Zhang, Transport and kinetics properties of LaF3 in FLiNaK molten salt determined by electrochemical methods, J. Fluor. Chem., 2020, 233, 109502 CrossRef CAS.
- Y. Zuo, X. Li, F. Jiang, C. She, W. Huang and Y. Gong, Electrochemical behavior of Sm(III)/Sm(II) and extraction of Sm on reactive electrode from molten LiF–BeF2, Sep. Purif. Technol., 2023, 315, 123737 CrossRef CAS.
- Y. Zuo, H. Peng, N. Ji, W. Huang and Y. Gong, Electrochemical study of Eu(III)/Eu(II) in LiF–BeF2 molten salt, J. Electrochem. Soc., 2023, 170(1), 013502 CrossRef CAS.
- R. Chesser, S. Guo and J. Zhang, Electrochemical behavior of dysprosium and lanthanum in molten LiF–NaF–KF (Flinak) salt, Ann. Nucl. Energy, 2018, 120, 246–252 CrossRef CAS.
- A. Y. Galashev, O. R. Rakhmanova, K. A. Abramova, K. P. Katin, M. M. Maslov, O. Y. Tkacheva, A. V. Rudenko, A. A. Kataev and Y. P. Zaikov, Molecular dynamics and experimental study of the effect of CeF3 and NdF3 additives on the physical properties of FLiNaK, J. Phys. Chem. B, 2023, 127(5), 1197–1208 CrossRef CAS PubMed.
- X. Li, Influence of ZrF4 additive on the local structures and thermophysical properties of molten NaF–BeF2, J. Mol. Liq., 2024, 393, 123681 CrossRef CAS.
- B. P. Sobolev, Lanthanum and lanthanide trifluorides: Lanthanide contraction and volume of fluorine ion, Crystallogr. Rep., 2020, 65(2), 175–181 CrossRef CAS.
|
This journal is © the Owner Societies 2024 |
Click here to see how this site uses Cookies. View our privacy policy here.