Climate change and population aging may impact the benefits of improved air quality on cardiovascular mortality in Guangzhou: epidemiological evidence and policy implications
Received
3rd December 2022
, Accepted 11th January 2023
First published on 13th January 2023
Abstract
Air pollution is the primary environmental risk factor contributing to global cardiovascular mortality. In China, a series of air pollution control policies launched in 2013 have led to substantial improvements in air quality over the past 10 years. However, the health benefits of improved air quality on cardiovascular mortality remain unclear under the combined effects of climate change and population aging. In this study, we investigated dynamic changes in the contribution of air pollution, meteorological conditions and aging to cardiovascular mortality over 9 years (2013–2021) in Guangzhou, China using generalized additive models and machine learning analysis. Although the air quality in Guangzhou has continuously improved since 2013, cardiovascular mortality has increased since 2019 and approached 2013 levels in 2021. Use of the SHapley Additive exPlanation (SHAP) approach to interpret the model outputs revealed that meteorological factors have gradually replaced air pollutants as the main environmental factors affecting cardiovascular mortality since 2016. Concurrently, the impact of population aging on cardiovascular mortality has increased year-on-year. Our results provide important insights into improved air quality related health benefits that could aid development of an early warning service system and national environmental and public health policy related to climate change and population aging.
Environmental significance
Aside from personal lifestyle (unhealthy diet, physical inactivity, tobacco use and harmful use of alcohol), current environmental and social risk factors, such as air pollution, meteorological conditions and population aging, contribute substantially to deaths from CVDs. In our study, we investigated dynamic changes in the contribution of air pollution, meteorological conditions and aging to cardiovascular mortality over 9 years (2013–2021) in Guangzhou, China using generalized additive models and machine learning analysis. Our results provide important insights into improved air quality related health benefits that could aid development of an early warning service system and national environmental and public health policy related to climate change and population aging.
|
1. Introduction
Like most countries and regions in the world, the incidence and mortality of cardiovascular diseases (CVDs) remain high in China. The Annual Report on Cardiovascular Health and Diseases in China (2021) stated that 330 million individuals suffer from CVDs and two out of every five deaths were due to CVDs in 2019.1 Growing evidence has demonstrated that air pollution is significantly associated with cardiovascular mortality, especially in some polluted regions in Asia.2–6 In response, governments are increasingly adopting policies to improve air quality, and such prevention and control actions to some extent have curbed further increases of air pollution. However, the health benefits of improved air quality on cardiovascular mortality remain unclear and quantifying the impact of current policies is important to meet people's requirements on environment health.
Aside from personal lifestyle (unhealthy diet, physical inactivity, tobacco use and harmful use of alcohol), current environmental and social risk factors, such as air pollution, meteorological conditions and population aging, contribute substantially to deaths from CVDs. In the last decade, climate change has been identified as one of the greatest health threats of the 21st century. Cardiovascular mortality attributable to extreme meteorological conditions is estimated to be increasing in the world and is greatest in Asia.7,8 Meanwhile, population aging is accelerating globally at an unprecedented rate. Therefore, age structure cannot be neglected when assessing the responses of improved air quality on health and developing further local or regional air quality policies to reduce the health impact of air pollution.9–11 However, although several studies have investigated the health outcomes of continuously improved air quality under the combined effects of climate change and rapid population aging, there is still a lack of systematic and comprehensive analysis.12–14
Most air pollutants and meteorological conditions, as well as age, affect cardiovascular health in a nonlinear way.15–17 The main strategy of classical epidemiological approaches like general linear or additive models is to isolate the risk factors of cardiovascular death by assuming other factors are fixed. Such models can explain the direction and corresponding uncertainty of an association between one or several (environmental) risk factors and health, thereby adjusting for potential confounding factors.18,19 However, they are of limited use in cases of high correlation and/or high-dimensional interactions between the covariates. In contrast, machine learning approaches can (partly) handle these issues and enable the importance and predictive performance of a multitude of environmental predictors to be compared.20
Guangzhou, with a permanent population of over 18.8 million in 2021, is the region with the highest degree of openness, strongest economic vitality and most improved air quality in China.21 However, it also suffers the health burden of both climate change and population aging. In this study, we applied multiple statistical methods to evaluate the health benefits of improved air quality on cardiovascular health under the combined effects of climate change and population aging, in an effort to provide strong epidemiologic evidence and policy implications to better frame environmental and public health policy.
2. Methods
2.1. Study domain and data collection
Guangzhou, a central city of the Pearl River Delta, is the economic center of south China. Its climate is mostly affected by subtropical monsoons. It was estimated to have a permanent population of 18.8 million at the end of 2021.21
Data concerning local air pollutants (PM2.5, SO2, NO2, CO and O3) and meteorological conditions (temperature, relative humidity, rainfall, air pressure, wind speed and illumination time) were obtained from monitoring stations in Guangzhou established by the Guangzhou Municipal Ecological Environment Agency and Guangzhou Meteorological Service. Diurnal statistics on cardiovascular deaths (ICD – 10 codes I00–I99) from 2013 to 2021 were obtained from the Guangzhou Center for Disease Control and Prevention, including information on education level, gender, address, age, date of death and cause of death.
2.2. Overview of research framework
This study attempts to use data mining and machine learning methods to analyze and predict the main environmental exposure factors affecting cardiovascular mortality in Guangzhou. The data sets used include meteorological, pollutant concentrations and cardiovascular mortality. Firstly, the data mining method of generalized additive model is used to analyze and determine the lag days of each factor to generate a new data set. The 85% part is used as the training set of machine learning, and 15% is used as the verification set to complete the ten-fold cross validation (CV). Then SVM, ANN, XGBoost and LGBM are used to construct the prediction model. Then the model is evaluated, the performance of each model is compared, and the most suitable machine learning method is selected. Finally, we use the best prediction model to predict cardiovascular mortality in Guangzhou and Qijiang District of Chongqing, in order to modify the model and verify its robustness. An overview of the research framework is shown in Fig. 1.
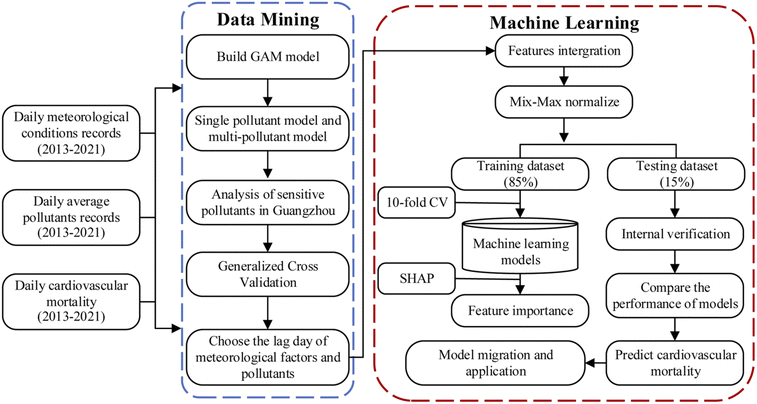 |
| Fig. 1 The block diagram of the research process. | |
2.3. Feature extraction based on the association between environmental factors and cardiovascular mortality
Daily cardiovascular deaths are a small probability event for the general population, with a statistical distribution similar to the Poisson distribution.22 Therefore, this study used a Poisson regression model for time series data. On the basis of excluding the long time series effect, weekend effect and meteorological factors, the pollutant concentration was introduced into the model as a linear variable to determine the correlation between different types of pollutants and cardiovascular mortality. Then, based on the annual air quality in Guangzhou, we divided the study dates into three stages: 2013–2015, 2016–2018, and 2019–2021 to explore the changes in the impact of pollutants on cardiovascular mortality at different stages. In the model, the single pollutant model was fitted by a smooth spline function to calculate the excess risk (ER) and 95% confidence interval (95% CI) of daily cardiovascular death risk increased or decreased after the fixed value of pollutants (PM2.5, SO2, NO2, O3 increased by 10 μg m−3, CO increased by 1 mg m−3) increased. At the same time, the stepwise regression method was used to fit the multi-pollutant model and control the collinearity, so as to establish the exposure-response relationship between the concentration of pollutants and the percentage increase of cardiovascular events. Similarly, after controlling the confounding factors of pollutants, we can also use the generalized additive model to explore the influence of meteorological factors on cardiovascular mortality. The specific formula of the model is as follows. | Log[E(Yi)] = βXi + s(time, df1) + s(meteorological factors/pollutants, df2) + DOW + α | (1) |
where i is the day of the observation; E(Yi) represents the data expectation of cardiovascular mortality on the observation day; β is the regression coefficient of the model; Xi refers to the level of atmospheric pollutants/meteorological factors on the day of observation; s means the smoothing spline to control for the nonlinear relationships and α is the model residual; DOW denotes the Sunday variable used to control short-term fluctuations in the daily death sequence, which means that mortality varies between different days of the week; time was used to represent the sequence of daily cardiovascular disease deaths; pollutants include PM2.5, SO2, O3, CO and NO2. Meteorological factors include temperature, air pressure, relative humidity (RH), illumination time (IT), rainfall and wind speed. df1 is the degree of freedom in the nonparametric smoothing function to control the long-term and seasonal trends of daily death sequences; df2 is the degree of freedom of controlling meteorological factors/pollutants in the nonparametric smoothing functions. According to previous studies, df1 is set at 7 per year and df2 at 3.23,24
An increasing number of epidemiological studies have shown that the effects of meteorological conditions and air pollutants on cardiovascular mortality lag to an extent that depends on the regional environment.25 Therefore, we separated the hysteresis effects of air pollutants and meteorological conditions on cardiovascular mortality in Guangzhou using an over-dispersed GAM and chose the lag day based on minimum generalized cross validation (GCV) values, which measure the model's fit.26 Effects of single day lags (from lag0 to lag6) and cumulative day lags (from lag01 to lag06) were taken into consideration.27
2.4. Establishment and verification of machine learning models
Four different machine learning methods, i.e., SVM (support vector machines), ANN (artificial neural network), XGBoost (extreme gradient boosting) and LGBM (light gradient boosting machine) were used to establish and compare predictive models and conduct external validation to determine the best model performance. SVM28 can be divided into linear SVM (linear SVR) and nonlinear SVR in the regression task. Considering that the relationship between environmental factors and cardiovascular diseases is not necessarily linear correlation, this study uses the Sklearn library to construct a nonlinear SVM model to fit the data. The inspiration of ANN comes from the network structure of human nervous system, and the activity of neurons is simulated by mathematical model.29,30 In this study, the Pytorch package is used to construct a three-layer ANN model. XGBoost is improved based on the decision tree machine learning algorithm.31,32 The regularization term is added to the objective function, and the loss function uses the first-order derivative and the second-order Taylor series as the cost function to retain more information of the objective function, avoiding the over-fitting of the model and ensuring the robustness of the model. LGBM is an improvement of XGBoost algorithm, which has made great progress in the processing of feature discretization data. In particular, the efficiency of processing large data sets is higher than that of the ordinary decision tree model.33,34
All the above models are trained and tested on the 85/15 percentage segmentation of the dataset by stratified random sampling. Due to the different scales of each variable, we normalize the independent variable by min–max in this study. In terms of model hyperparameters, this study uses grid search to optimize the parameters of each machine learning model. In order to avoid the possibility of overfitting, this study used k-fold based on sklearn to cross-validate the model (10 flod), and each method was trained 30 times and compared.
To evaluate the performance of the model, we use R2 (coefficient of determination, regression score function), RMSE (root of mean squared error regression loss), MSE (mean squared error regression loss), MAE (mean absolute error regression loss), MAPE (mean absolute percentage error regression loss), max_error (the max_error metric calculates the maximum residual error), MPD (mean poisson deviance regression loss) and MGD (mean gamma deviance regression loss) were used as evaluation indexes to analyze the relationship between the actual value and the predicted value of cardiovascular mortality. At the same time, according to the data set of environmental exposure and cardiovascular mortality in Chongqing City from 2020 to 2021, we used the optimal model to predict the local cardiovascular mortality, so as to verify the robustness and portability of the model.
In addition, as the above machine learning “black box” models lack interpretability, Lundberg and Lee proposed a SHAP (SHapley Additive exPlanation) method to explain the output of the models.35 SHAP constructs an additive interpretation model based on game theory36 and local interpretation.37 All features are regarded as “contributors”. Its core idea is to calculate the marginal contribution of the output of the model to explain the “black box model”. In this study, we applied the SHAP method to LGBM modeling results to identify and quantify the key environmental exposure factors for cardiovascular mortality.
2.5. Effect decomposition of population aging on cardiovascular mortality
To explore the contribution of various factors to cardiovascular mortality, we used an improved method by Chen et al. to decompose the annual mortality change from 2013 to 2021 into two components: mortality caused by population aging and mortality caused by factor changes.38,39 The factors could be any factor other than population aging, including protective or risk factors associated with changes in mortality. We represented this process using the following formulae: | 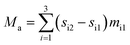 | (2) |
| 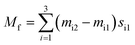 | (3) |
| 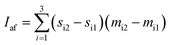 | (4) |
where Ma and Mf represent the main effects of population aging and factor variation, respectively, Iaf represents the bidirectional interaction of two components, mij and sij represent the mortality rate by age and the proportion of the ith age group (three age groups: 0–44, 45–64, 65+), respectively, and j represents the year. Mortality changes due to population aging and changing factors were calculated as follows:where A is the mortality rate attributable to population aging and F is the mortality rate attributable to factor variation.
3. Results and discussion
3.1. Trends in environmental factors associated with cardiovascular mortality
Fig. 2 shows trends in the cardiovascular mortality and main environmental factors in China. From 2013–2021, a total of 181
171 cardiovascular deaths occurred within the whole area of Guangzhou, with an average daily death rate of 55. Cardiovascular mortality showed a fluctuating downward trend from 2013 to 2018, then increased to 226.19 per 100
000 people in 2021, close to the level of 2013 (Fig. 2a and Table 1). From 2013 to 2021, concentrations of SO2, PM2.5, CO and NO2 decreased over time by 62.12%, 53.94%, 32.30% and 30.58%, respectively (Fig. 2b and Table 1), possibly due to the State Council of China implementing the Air Pollution Prevention and Control Action Plan (APPCAP) in 2013.40,41 However, O3 was the only air pollutant that demonstrated increased levels during the study period. This result is consistent with those reported in other Chinese cities and might be due to unbalanced reductions in its precursors of NOx or volatile organic compounds (VOC) levels from anthropogenic sources, as well as warmer and drier meteorological conditions.42–46 Additionally, two excessive pollutants, PM2.5 and SO2, reached the National Ambient Air Quality Standards of China since 2016 and 2019, respectively.47 Among the six main meteorological parameters, the change in temperature was the most stable and significant. From 2013, the temperature continued to rise, reaching 24 °C in 2021, an increase of 1.5 °C compared with 2013 (Fig. 2c).
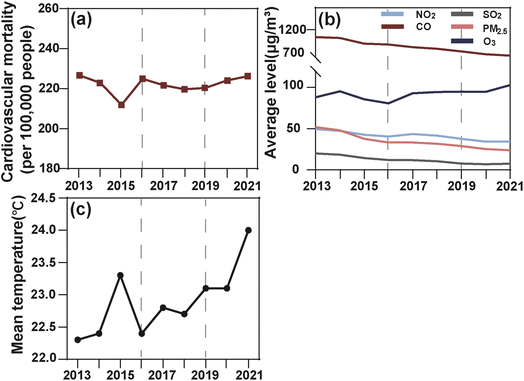 |
| Fig. 2 Variations in cardiovascular mortality and key environmental factors during 2013–2021 in Guangzhou: (a) annual cardiovascular mortality, (b) annual average concentrations of PM2.5, SO2, NO2, CO and O3, (c) annual average temperature. | |
Table 1 Annual air pollutant concentration data and meteorological data for Guangzhou, China, 2013–2021
Year |
2013 |
2014 |
2015 |
2016 |
2017 |
2018 |
2019 |
2020 |
2021 |
Wind speed (m s−1) |
2.45 |
2.22 |
2.30 |
2.23 |
2.20 |
2.23 |
2.18 |
2.39 |
2.19 |
Temperature (°C) |
22.30 |
22.40 |
23.30 |
22.40 |
22.80 |
22.70 |
23.10 |
23.10 |
24.00 |
Air pressure (hpa) |
1004.7 |
1004.9 |
1005.2 |
1004.7 |
1005.3 |
1004.7 |
1004.5 |
1005.4 |
1004.8 |
RH (%) |
80.77 |
78.45 |
78.12 |
81.81 |
80.33 |
81.71 |
81.84 |
79.29 |
76.19 |
IT (h) |
1637 |
1743 |
1630 |
1520 |
1753 |
1598 |
1650 |
1683 |
1879 |
Rainfall (mm) |
2015 |
2009 |
2045 |
2638 |
2034 |
1926 |
2336 |
1821 |
1436 |
SO2 (μg m−3) |
20.02 |
18.57 |
14.37 |
11.97 |
11.95 |
10.58 |
7.86 |
6.87 |
7.58 |
PM2.5 (μg m−3) |
51.93 |
47.97 |
37.76 |
33.34 |
33.27 |
31.70 |
28.87 |
25.34 |
23.92 |
NO2 (μg m−3) |
49.45 |
47.45 |
42.67 |
40.61 |
43.58 |
41.68 |
37.67 |
34.36 |
34.33 |
O3 (μg m−3) |
87.88 |
95.26 |
85.64 |
80.67 |
92.82 |
94.44 |
94.80 |
94.67 |
102.67 |
CO (μg m−3) |
1059.27 |
1044.23 |
938.87 |
922.05 |
873.17 |
844.96 |
797.61 |
744.54 |
717.17 |
Mortality (per 100 000 people) |
226.67 |
222.87 |
211.81 |
224.92 |
221.70 |
219.73 |
220.40 |
224.06 |
226.19 |
Fig. 3 displays the excess risk and 95% confidence intervals of concentration changes of atmospheric pollutants in Guangzhou from three stages on cardiovascular mortality in single pollutant model. We observed that all five pollutants were significantly associated with cardiovascular mortality in 2013–2015 and CO had the most significant effect. SO2 had the most significant impact in 2016–2018, with an increase of 10 μg m−3, cardiovascular mortality increased by 5.54% (95%CI: 2.02–9.19%) at lag1. It is worth noting that during 2019–2021 when the environmental quality was improved, the impacts of PM2.5 and SO2 at lag1 were particularly significant, reaching 1.53% (95%CI: 0.84–2.23%) and 16.61% (95%CI: 9.19–24.53%), respectively. Similarly, some domestic studies in China have also found this phenomenon. For example, a previous study in Hefei city reported that when SO2 concentrations were low, the impact of SO2 on cardiovascular mortality was greater than high concentrations.48 Wu et al. found that although the environmental PM level in Guangzhou decreased significantly in recent years, the risk of cardiopulmonary death related to PM2.5 and PM10 was still significant, and the risk of respiratory death even increased.49 These results indicated that, even in the low concentration of PM2.5 and SO2 environment, the government cannot ignore the impact of the above two pollutants on the health of residents.
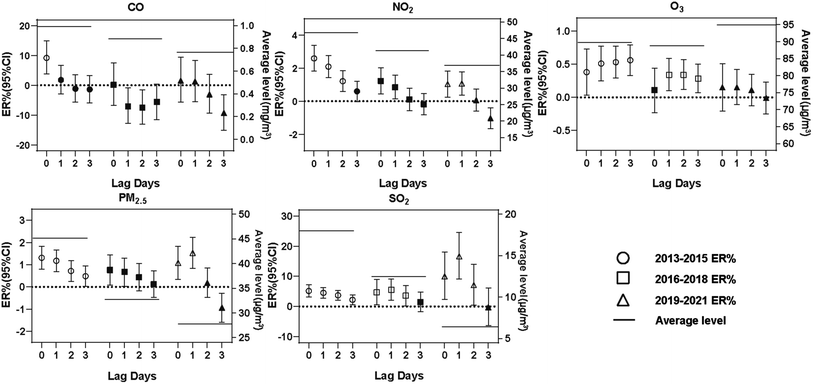 |
| Fig. 3 Excess risk (ER) and 95% confidence interval (95%CI) of cardiovascular mortality in a single pollutant model with increased concentrations of pollutants (PM2.5, SO2, NO2, O3 increased by 10 μg m−3, CO increased by 1 mg m−3) with different lag days. Statistically significant associations are represented by lines with hollow circles. Horizontal lines represent the average concentration of pollutants in each period. Vertical bars denote 95% confidence intervals. | |
Fig. 4 shows the percentage change of cardiovascular mortality for every 10 units increases in the level of meteorological factors under different lag days in the model. After controlling for air pollution and other factors, temperature and air pressure were significantly associated with increased/decreased cardiovascular mortality. In the stage of 2019–2021 with the highest annual average temperature, the influence of extreme high temperature is also the strongest, for every 10 units increase in temperature, cardiovascular mortality increased by 11.09%. Exposure to high temperature may stimulate the body's self-regulation to maintain heat balance, which leads to increased cardiac load, dehydration and salt exhaustion.50 In addition, for every 10 units increase in air pressure, cardiovascular mortality decreased by 4.69%, 3.77% and 8.97%, respectively. A previous study in Guangzhou also found an association between barometric pressure and cardiovascular mortality, indicating that there was a 2.27% increase in cardiovascular mortality compared with the 5th and 25th percentile of atmospheric pressure.51 This finding is consistent with our results.
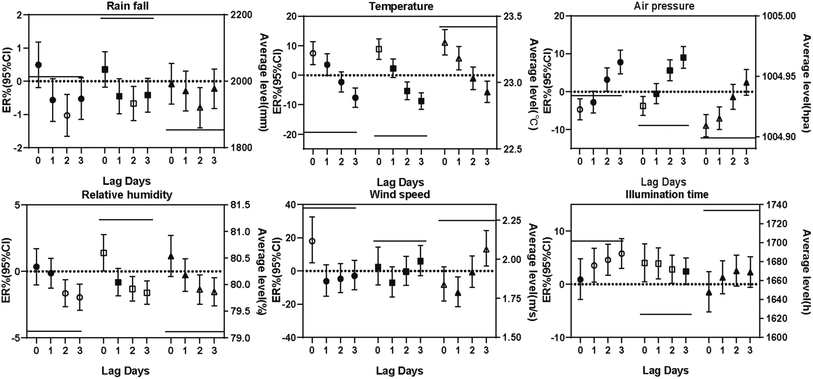 |
| Fig. 4 Excess risk (ER) and 95% confidence interval (95%CI) for cardiovascular mortality after 10 unit increases in meteorological factors for different lag days. Statistically significant associations are represented by lines with hollow circles. Horizontal lines represent the average concentration of pollutants in each period. Vertical bars denote 95% confidence intervals. | |
3.2. Assessing the importance of environmental factors toward cardiovascular mortality via machine learning models
When using machine learning to predict cardiovascular mortality, we considered features of time series characteristics, air pollutants and meteorological conditions. Based on the above association analysis between environmental factors and cardiovascular mortality, GCV values of temperature, relative humidity, rainfall, wind speed, air pressure, illumination time, PM2.5, SO2, NO2, CO and O3 were the smallest at lag06, lag05, lag05, lag06, lag06, lag04, lag1, lag1, lag0, lag0 and lag2, respectively.
The results are similar to a previous study in Chengdu, but somewhat inconsistent.26 Due to differences in pollution characteristics and climatic factors between cities, the lag time of environmental factors on cardiovascular mortality is also different.
After identifying 16 time features and environmental factors through GAM, we used machine learning methods for model construction to avoid the problem of traditional epidemiological methods of not handling high correlations between covariates. Compared to the constructed SVM, ANN, and XGBoost models, LGBM performed best across eight different model evaluation metrics (Table 2). SVM as a machine learning using hyperplane segmentation sample has poor performance in dealing with high latitude and nonlinear correlation data sets (train R2: 0.5988–0.6191, test R2: 0.4231–0.5388). ANN algorithm tends to follow the sample mean prediction, and it is difficult to predict specific peak data in the dataset. Gabriela Polezer et al. have also found this problem in using ANN to evaluate the impact of PM2.5 on respiratory diseases in Curitiba, Brazil.52 At the same time, ANN algorithm is prone to over-fitting when setting too many neurons and the network structure is too complex. The ensemble learning method embodies high accuracy in this data. Compared with common machine learning methods, the ensemble learning method combines multiple weak learners to form a strong learner, so that the model has stronger generalization ability. In the data set of this study, such as year, month, day, holiday and other category data, LGBM method can directly model the category data without one-hot key encoding. Compared with machine learning methods such as SVM and ANN, LGBM can learn the implicit information in the data set as much as possible, and has higher prediction ability than other machine learning algorithms. The R2 of LGBM model migration to Qijiang District of Chongqing for prediction is 0.793. After replacing the study area, the LGBM model still maintained high accuracy, indicating that the model has strong robustness and portability.
Table 2 Performance evaluation of four alternative prediction models
Method |
SVM |
ANN |
XGBoost |
LGBM |
Score |
Train |
Test |
Train |
Test |
Train |
Test |
Train |
Test |
R
2
|
0.6081 |
0.4906 |
0.6460 |
0.6240 |
0.7735 |
0.7462 |
0.8824 |
0.8676 |
RMSE |
0.0808 |
0.0913 |
0.0766 |
0.0788 |
0.0613 |
0.0646 |
0.0441 |
0.0466 |
MSE |
0.0065 |
0.0083 |
0.0059 |
0.0062 |
0.0038 |
0.0042 |
0.0019 |
0.0022 |
MAE |
0.0649 |
0.0650 |
0.0549 |
0.0590 |
0.0426 |
0.0425 |
0.0302 |
0.0353 |
MAPE |
0.1213 |
0.1332 |
0.1147 |
0.1185 |
0.0921 |
0.0946 |
0.0654 |
0.0698 |
Max_Error |
0.4660 |
0.2928 |
0.4510 |
0.3002 |
0.2740 |
0.2195 |
0.2083 |
0.1478 |
MPD |
0.0123 |
0.0123 |
0.0115 |
0.0115 |
0.0074 |
0.0074 |
0.0041 |
0.0041 |
MGD |
0.0209 |
0.0253 |
0.0197 |
0.0208 |
0.0129 |
0.0140 |
0.0072 |
0.0077 |
After studying the SHAP map (Fig. 5) and the results of independent variable importance ranking in the process of machine learning training (Fig. 6), we found that the contribution of climatic factors to cardiovascular mortality in Guangzhou gradually increased. In 2013–2015, air pollutants were the main environmental factors affecting cardiovascular mortality. However, over the next two periods, i.e., 2016–2018 and 2019–2021, the contribution of air pressure, rainfall and illumination time gradually increased and exceeded the contribution of pollutants.
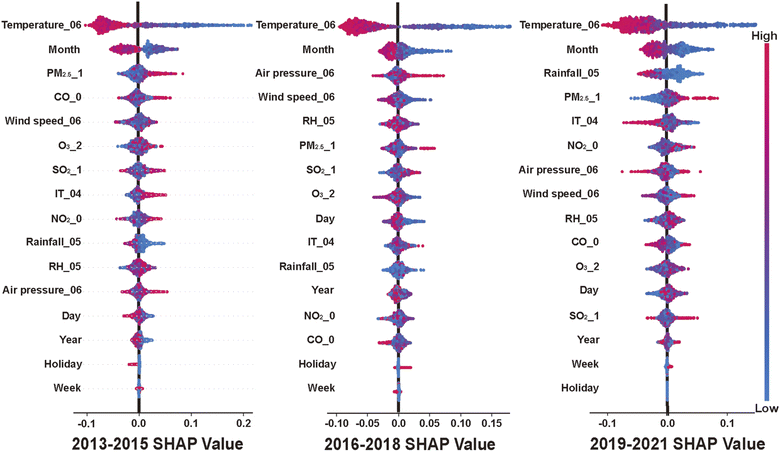 |
| Fig. 5 SHAP summary plot. Each factor is arranged from high to low according to the average value of its SHAP absolute value. Each line provides more details about how each risk factor affects cardiovascular mortality. Red (blue) points represent high (low) values of risk factors. | |
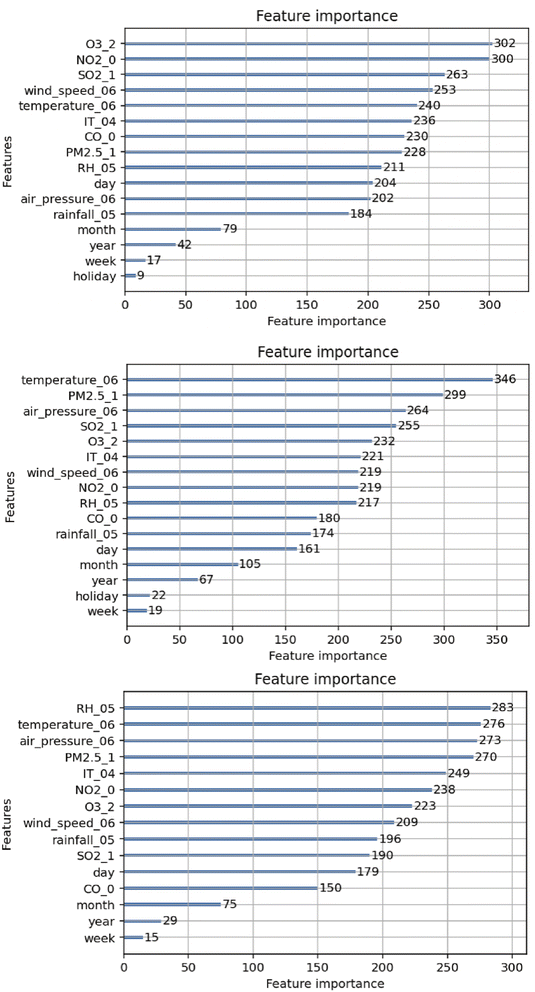 |
| Fig. 6 Independent variable importance ranking diagram. | |
Low temperature was the main environmental factor affecting cardiovascular mortality in Guangzhou in 2013–2015. As mentioned above, low temperatures can aggravate the impact of some pollutants on cardiovascular mortality. In addition, low temperatures can have a direct effect on cardiovascular mortality. Studies have shown that cold temperatures can adversely alter plasma lipid concentrations, making abnormal thrombosis more likely and having a potential chronic atherogenic effect, increasing the risk of cardiovascular death.53 In China and the Czech Republic, national long-term series studies on the relationship between ambient temperature and cardiovascular mortality have consistently found that low temperatures cause significant cardiovascular mortality risks and burdens to the region.54,55
In 2016–2018, the contribution of air pressure to cardiovascular mortality in Guangzhou exceeded that of pollutants and became the main environmental factor after temperature. Atmospheric pressure controls the circulation pattern of air masses. Therefore, changes in atmospheric pressure can change the temperature, rainfall and wind, resulting in a huge impact of climate change on health.51 A study in the US state of Texas linked a rapid decline in air pressure to the onset of acute myocardial infarction, confirming the effects of air pressure on CVDs.56
It is worth noting that during 2019–2021, rainfall and illumination time were key factors affecting cardiovascular mortality in Guangzhou. From 2019–2021, rainfall decreased by 28.77% compared to 2013 (Table 1). We found that rainfall negatively correlated with cardiovascular mortality, consistent with other studies in some regions. For example, a study from the United States found that increased rainfall was associated with reduced cardiovascular hospitalization mortality. The odds ratio (OR) for cardiovascular mortality was calculated as 0.95 for every 10 mm increase in rainfall (CI 0.90–1.00, P = 0.035).57 Further, Khamutian et al. found that in Kermanshah, Iran, reduced rainfall increased hospitalizations for CVDs.58 Similarly, in the past 3 years in Guangzhou, illumination time has been shown to be negatively correlated with cardiovascular mortality. Studies have shown that ultraviolet radiation mobilizes nitric oxide from nitrates stored in the skin, thereby reducing blood pressure, which may be the reason for the observed decrease in CVDs and all-cause mortality as sun exposure increases.59 This may also explain the phenomenon of high cardiovascular mortality under conditions of low illumination time. Although studies have shown the effects of rainfall and illumination time on cardiovascular mortality, further studies are needed to identify the potential mechanisms and interaction between these factors.
3.3. Cardiovascular mortality burden due to population aging
Like other regions in the world, Guangzhou is experiencing problems associated with population aging. In 2021, the proportion of the elderly population in Guangzhou reached 13.17% (Fig. 7a), much higher than the international aging standard line of 7%.60 The proportion of the population aged 65 and over in Guangzhou rose from 10.86% in 2013 to 13.17% in 2021, and life expectancy rose from 80.62 years in 2013 to 83.18 years in 2021. Like other regions in the world, Guangzhou is experiencing problems associated with population aging, e.g., an increased medical burden of CVDs.
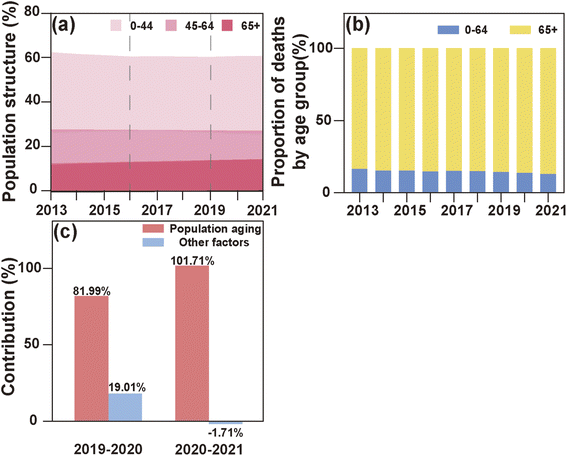 |
| Fig. 7 Effect of population aging on cardiovascular mortality in Guangzhou: (a) changes in population structure, (b) changes in the proportion of deaths by age group, (c) contribution of different factors to the increase in cardiovascular mortality. | |
Older persons are particularly vulnerable to adverse health effects of environmental exposure because of their high prevalence of chronic diseases, declining physical protection mechanisms and social isolation.61 Although air pollution levels in Guangzhou have decreased in recent years, cardiovascular mortality has continued to rise, with the elderly group contributing the most deaths (Fig. 7b). After decomposing the influencing factors of cardiovascular mortality, it was found that 101.71% of the increase in cardiovascular mortality in Guangzhou from 2020 to 2021 was attributable to population aging, which was higher than the contribution from 2019 to 2020, and the contribution far exceeded the influence of non-demographic factors. The impact of population aging on cardiovascular mortality is still deepening (Fig. 7c). In recent years, the material living conditions and medical standards of Guangzhou residents have gradually improved,21 resulting in an increasing elderly population. The aging of the population structure faces an accelerated period, which will make the burden of CVDs increasingly serious.
4. Conclusion
Overall, the case study of Guangzhou demonstrated that climate change and population aging may offset the benefits of improved air quality on cardiovascular health, which has implications for other megacities facing the dual challenge of climate change and air pollution as well as an increasingly aging population. Although it would be difficult to prevent the current trend of climate change and aging over the short-term, the government should step up efforts to control air pollution and establish an effective warning and tracking system for extreme pollution and weather conditions. Strategies to increase human adaptability and strengthen the health status and response measures to mitigate against potentially catastrophic effects posed by climate change and aging should also receive sufficient attention.
Conflicts of interest
The authors declare no conflict of interest with the study or preparation of the manuscript.
Acknowledgements
This work was supported by the General Research Fund from the Hong Kong Research Grants Council (12303320), Special Funds for the Cultivation of Guangdong College Students' Scientific and Technological Innovation, “Climbing Program” Special Funds (pdjh2023a0162), the National Key Research and Development Project (2019YFC1804604), Guangzhou Science and Technology Planning Project (202201011617), Key R&D Plans of Guangzhou Science and Technology (202206010190).
References
-
National Center for Cardiovascular Diseases, Annual Report on Cardiovascular Health and Diseases in China 2021, Beijing, 2022 Search PubMed.
- C. Liu, R. Chen, F. Sera, A. M. Vicedo-Cabrera, Y. Guo, S. Tong, M. Coelho, P. H. N. Saldiva, E. Lavigne, P. Matus, N. Valdes Ortega, S. Osorio Garcia, M. Pascal, M. Stafoggia, M. Scortichini, M. Hashizume, Y. Honda, M. Hurtado-Diaz, J. Cruz, B. Nunes, J. P. Teixeira, H. Kim, A. Tobias, C. Iniguez, B. Forsberg, C. Astrom, M. S. Ragettli, Y. L. Guo, B. Y. Chen, M. L. Bell, C. Y. Wright, N. Scovronick, R. M. Garland, A. Milojevic, J. Kysely, A. Urban, H. Orru, E. Indermitte, J. J. K. Jaakkola, N. R. I. Ryti, K. Katsouyanni, A. Analitis, A. Zanobetti, J. Schwartz, J. Chen, T. Wu, A. Cohen, A. Gasparrini and H. Kan, Ambient Particulate Air Pollution and Daily Mortality in 652 Cities, N. Engl. J. Med., 2019, 381(8), 705–715 CrossRef CAS PubMed.
- R. T. Burnett, C. A. Pope 3rd, M. Ezzati, C. Olives, S. S. Lim, S. Mehta, H. H. Shin, G. Singh, B. Hubbell, M. Brauer, H. R. Anderson, K. R. Smith, J. R. Balmes, N. G. Bruce, H. Kan, F. Laden, A. Pruss-Ustun, M. C. Turner, S. M. Gapstur, W. R. Diver and A. Cohen, An integrated risk function for estimating the global burden of disease attributable to ambient fine particulate matter exposure, Environ. Health Perspect., 2014, 122(4), 397–403 CrossRef PubMed.
- C. Huang, A. E. Moran, P. G. Coxson, X. Yang, F. Liu, J. Cao, K. Chen, M. Wang, J. He, L. Goldman, D. Zhao, P. L. Kinney and D. Gu, Potential Cardiovascular and Total Mortality Benefits of Air Pollution Control in Urban China, Circulation, 2017, 136(17), 1575–1584 CrossRef PubMed.
- Y. Sun, Y. Zhang, C. Chen, Q. Sun, Y. Wang, H. Du, J. Wang, Y. Zhong, W. Shi, T. Li and X. Shi, Impact of Heavy PM2.5 Pollution Events on Mortality in 250 Chinese Counties, Environ. Sci. Technol., 2022, 56(12), 8299–8307 CrossRef CAS PubMed.
- C. Liu, K. H. Chan, J. Lv, H. Lam, K. Newell, X. Meng, Y. Liu, R. Chen, C. Kartsonaki and N. Wright,
et al., Long-term exposure to ambient fine particulate matter and incidence of major cardiovascular diseases: a prospective study of 0.5 million adults in China, Environ. Sci. Technol., 2022, 56(18), 13200–13211 CrossRef CAS PubMed.
- D. Campbell-Lendrum and A. Pruss-Ustun, Climate change, air pollution and noncommunicable diseases, Bull. W. H. O., 2019, 97(2), 160–161 CrossRef PubMed.
- R. A. Silva, J. J. West, J. F. Lamarque, D. T. Shindell, W. J. Collins, G. Faluvegi, G. A. Folberth, L. W. Horowitz, T. Nagashima, V. Naik, S. T. Rumbold, K. Sudo, T. Takemura, D. Bergmann, P. Cameron-Smith, R. M. Doherty, B. Josse, I. A. MacKenzie, D. S. Stevenson and G. Zeng, Future Global Mortality from Changes in Air Pollution Attributable to Climate Change, Nat. Clim. Change, 2017, 7(9), 647–651 CrossRef PubMed.
- T. Menz and H. Welsch, Population aging and environmental preferences in OECD countries: The case of air pollution, Ecol Econ, 2010, 69(12), 2582–2589 CrossRef.
-
WHO, Ageing and Health, Available online: https://www.who.int/news-room/fact-sheets/detail/ageing-and-health, (accessed on 1 February 2022), 2022.
- Q. Xing, Z. Sun, Y. Tao, J. Shang, S. Miao, C. Xiao and C. Zheng, Projections of future temperature-related cardiovascular mortality under climate change, urbanization and population aging in Beijing, China, Environ. Int., 2022, 163, 107231 CrossRef PubMed.
- S. Zhao, S. Liu, X. Hou, Y. Sun and R. Beazley, Air pollution and cause-specific mortality: A comparative study of urban and rural areas in China, Chemosphere, 2021, 262, 127884 CrossRef CAS PubMed.
- Z. Zhang, J. Wang, J. C. Kwong, R. T. Burnett, A. van Donkelaar, P. Hystad, R. V. Martin, L. Bai, J. McLaughlin and H. Chen, Long-term exposure to air pollution and mortality in a prospective cohort: The Ontario Health Study, Environ. Int., 2021, 154, 106570 CrossRef CAS PubMed.
- A. Juginovic, M. Vukovic, I. Aranza and V. Bilos, Health impacts of air pollution exposure from 1990 to 2019 in 43 European countries, Sci. Rep., 2021, 11(1), 22516 CrossRef CAS PubMed.
- P. L. Kinney, Interactions of Climate Change, Air Pollution, and Human Health, Curr. Environ. Health Rep., 2018, 5(1), 179–186 CrossRef CAS PubMed.
- N. L. Fann, C. G. Nolte, M. C. Sarofim, J. Martinich and N. J. Nassikas, Associations Between Simulated Future Changes in Climate, Air Quality, and Human Health, Jama Netw Open, 2021, 4(1), e2032064 CrossRef PubMed.
- W. Zhang, G. Du, L. Xiong, T. Liu, Z. Zheng, Q. Yuan, J. Yang, Y. Wu, R. Zhu and G. Hu, Extreme temperatures and cardiovascular mortality: assessing effect modification by subgroups in Ganzhou, China, Glob. Health Action, 2021, 14(1), 1965305 CrossRef PubMed.
- Q. Zhang, Y. Zheng, D. Tong, M. Shao, S. Wang, Y. Zhang, X. Xu, J. Wang, H. He, W. Liu, Y. Ding, Y. Lei, J. Li, Z. Wang, X. Zhang, Y. Wang, J. Cheng, Y. Liu, Q. Shi, L. Yan, G. Geng, C. Hong, M. Li, F. Liu, B. Zheng, J. Cao, A. Ding, J. Gao, Q. Fu, J. Huo, B. Liu, Z. Liu, F. Yang, K. He and J. Hao, Drivers of improved PM2.5 air quality in China from 2013 to 2017, Proc. Natl. Acad. Sci. U. S. A., 2019, 116(49), 24463–24469 CrossRef CAS PubMed.
- T. Xue, J. Liu, Q. Zhang, G. N. Geng, Y. X. Zheng, D. Tong, Z. Liu, D. B. Guan, Y. Bo, T. Zhu, K. B. He and J. M. Hao, Rapid improvement of PM2.5 pollution and associated health benefits in China during 2013-2017, Sci. China: Earth Sci., 2019, 62(12), 1847–1856 CrossRef.
- L. Marien, M. Valizadeh, W. zu Castell, C. Nam, D. Rechid, A. Schneider, C. Meisinger, J. Linseisen, K. Wolf and L. Bouwer, Machine learning models to predict myocardial infarctions from past climatic and environmental conditions, Nat. Hazards Earth Syst. Sci., 2022, 1–36 Search PubMed.
-
Statistics Bureau of Guangzhou Municipality, 2021 Guangzhou National Economic and Social Development Statistical Bulletin, http://tjj.gz.gov.cn/zzfwzq/tjgb/content/post_8543009.html, accessed on 1 February 2022.
-
J. M. Samet, F. Dominici, S. L. Zeger, J. Schwartz and D. W. Dockery, The National Morbidity, Mortality, and Air Pollution Study, Part I: methods and methodologic issues, 2000, 94 Pt 1, pp. 5–14, discussion 75 Search PubMed.
- J. Wang, Q. Yin, S. Tong, Z. Ren, M. Hu and H. Zhang, Prolonged continuous exposure to high fine particulate matter associated with cardiovascular and respiratory disease mortality in Beijing, China, Atmos. Environ., 2017, 168, 1–7 CrossRef CAS.
- E. Ge, K. Lai, X. Xiao, M. Luo, Z. Fang, Y. Zeng, H. Ju and N. Zhong, Differential effects of size-specific particulate matter on emergency department visits for respiratory and cardiovascular diseases in Guangzhou, China, Environ. Pollut., 2018, 243(Pt A), 336–345 CrossRef CAS PubMed.
- Z. Soleimani, A. Darvishi Boloorani, R. Khalifeh, D. W. Griffin and A. Mesdaghinia, Short-term effects of ambient air pollution and cardiovascular events in Shiraz, Iran, 2009 to 2015, J. Environ. Sci. Pollut. Res, 2019, 26(7), 6359–6367 CrossRef CAS PubMed.
- H. Qiu, L. Luo, Z. Su, L. Zhou, L. Wang and Y. Chen, Machine learning approaches to predict peak demand days of cardiovascular admissions considering environmental exposure, BMC Med. Inf. Decis. Making, 2020, 20(1), 83 CrossRef PubMed.
- H. Qiu, X. Zhu, L. Wang, J. Pan, X. Pu, X. Zeng, L. Zhang, Z. Peng and L. Zhou, Attributable risk of hospital admissions for overall and specific mental disorders due to particulate matter pollution: A time-series study in Chengdu, China, Environ. Res., 2019, 170, 230–237 CrossRef CAS.
-
C. Cortes and V. Vapnik, Support-vector networks, 1995, vol. 20, 3, pp. 273–297 Search PubMed.
- M. van Gerven and S. Bohte, Editorial: Artificial Neural Networks as Models of Neural Information Processing, Front. Comput. Neurosci., 2017, 11, 114 CrossRef PubMed.
- N. Q. Hung, M. S. Babel, S. Weesakul, N. J. H. Tripathi and E. S. Sciences, An artificial neural network model for rainfall forecasting in Bangkok, Thailand, 2009, 13(8), 1413–1425 Search PubMed.
-
J. H. Friedman, Greedy Function Approximation: a Gradient Boosting Machine, 2001, pp. 1189–1232 Search PubMed.
-
T. Chen, T. He, M. Benesty, V. Khotilovich, Y. Tang, H. Cho and K. Chen, Xgboost: extreme gradient boosting, 2015, vol. 1, 4, pp. 1–4 Search PubMed.
-
G. Ke, Q. Meng, T. Finley, T. Wang, W. Chen, W. Ma, Q. Ye and T.-Y. Liu, Lightgbm: A Highly Efficient Gradient Boosting Decision Tree, 2017, 30 Search PubMed.
- X. Sun, M. Liu and Z. Sima, A novel cryptocurrency price trend forecasting model based on LightGBM, Finance Res. Lett., 2020, 32, 101084 CrossRef.
-
S. M. Lundberg and S. Lee, A Unified Approach to Interpreting Model Predictions, 2017, 30 Search PubMed.
- E. Štrumbelj and I. Kononenko, Explaining prediction models and individual predictions with feature contributions, Knowl. Inf. Syst., 2013, 41(3), 647–665 CrossRef.
-
M. T. Ribeiro, S. Singh and C. Guestrin, “Why Should I Trust You?”. in Proceedings of the 22nd ACM SIGKDD International Conference on Knowledge Discovery and Data Mining, 2016, pp. 1135–1144 Search PubMed.
- Y. Chen, C. Yang, N. Li, Z. Wang, P. Wu, J. Du and J. Jiang, Effects of population aging on the mortality burden of related cancers in urban and rural areas of China, 2004-2017: a population-based
study, Cancer Biol. Med., 2022, 19, 696–706 CrossRef PubMed.
- X. Cheng, L. Tan, Y. Gao, Y. Yang, D. C. Schwebel and G. Hu, A new method to attribute differences in total deaths between groups to population size, age structure and age-specific mortality rate, PLoS One., 2019, 14(5), e0216613 CrossRef PubMed.
- H. Yue, C. He, Q. Huang, D. Yin and B. A. Bryan, Stronger policy required to substantially reduce deaths from PM2.5 pollution in China, Nat. Commun., 2020, 11(1), 1462 CrossRef CAS PubMed.
-
The State Council of China, Air Pollution Prevention and Control Action Plan, http://www.gov.cn/zhengce/content/2013-09/13/content_4561.htm, accessed on 1 February 2022.
- N. Cheng, Z. Chen, F. Sun, R. Sun, X. Dong, X. Xie and C. Xu, Ground ozone concentrations over Beijing from 2004 to 2015: variation patterns, indicative precursors and effects of emission-reduction, Environ. Pollut., 2018, 237, 262–274 CrossRef CAS PubMed.
- X. Lu, J. Hong, L. Zhang, O. R. Cooper, M. G. Schultz, X. Xu, T. Wang, M. Gao, Y. Zhao and Y. Zhang, Severe surface ozone pollution in China: a global perspective, Environ. Sci. Technol. Lett., 2018, 5(8), 487–494 CrossRef CAS.
- Z. Zhong, J. Zheng, M. Zhu, Z. Huang, Z. Zhang, G. Jia, X. Wang, Y. Bian, Y. Wang and N. Li, Recent developments of anthropogenic air pollutant emission inventories in Guangdong province, China, Sci. Total Environ., 2018, 627, 1080–1092 CrossRef CAS.
- R. Wu, X. Song, D. Chen, L. Zhong, X. Huang, Y. Bai, W. Hu, S. Ye, H. Xu and B. J. Feng, Health benefit of air quality improvement in Guangzhou, China: results from a long time-series analysis (2006–2016), Environ. Int., 2019, 126, 552–559 CrossRef CAS PubMed.
- L. Wen, C. Yang, X. Liao, Y. Zhang, X. Chai, W. Gao, S. Guo, Y. Bi, S.-Y. Tsang and Z.-F. Chen,
et al., Investigation of PM2. 5 pollution during COVID-19 pandemic in Guangzhou, China, J. Environ. Sci., 2022, 115, 443–452 CrossRef CAS PubMed.
-
Ministry of Ecology and Environment of the People's Republic of China, Ambient Air Quality Standards, https://www.mee.gov.cn/ywgz/fgbz/bz/bzwb/dqhjbh/dqhjzlbz/201203/t20120302_224165.shtml, accessed on 1 February 2022.
- C. Zhang, R. Ding, C. Xiao, Y. Xu, H. Cheng, F. Zhu, R. Lei, D. Di, Q. Zhao and J. Cao, Association between air pollution and cardiovascular mortality in Hefei, China: A time-series analysis, Environ. Pollut., 2017, 229, 790–797 CrossRef CAS PubMed.
- R. Wu, L. Zhong, X. Huang, H. Xu, S. Liu, B. Feng, T. Wang, X. Song, Y. Bai, F. Wu, X. Wang and W. Huang, Temporal variations in ambient particulate matter reduction associated short-term mortality risks in Guangzhou, China: A time-series analysis (2006-2016), Sci. Total Environ., 2018, 645, 491–498 CrossRef CAS PubMed.
- R. Basu, High ambient temperature and mortality: a review of epidemiologic studies from 2001 to 2008, Environ. Health, 2009, 8, 40 CrossRef.
- C. Q. Ou, J. Yang, Q. Q. Ou, H. Z. Liu, G. Z. Lin, P. Y. Chen, J. Qian and Y. M. Guo, The impact of relative humidity and atmospheric pressure on mortality in Guangzhou, China, Biomed. Environ. Sci., 2014, 27(12), 917–925 Search PubMed.
- G. Polezer, Y. S. Tadano and H. V. Siqueira,
et al., Assessing the impact of PM2. 5 on respiratory disease using
artificial neural networks, Environ. Pollut., 2018, 235, 394–403 CrossRef CAS PubMed.
-
P. Wilmshurst, Temperature and cardiovascular mortality, 1994, 309, 6961, pp. 1029–1030 Search PubMed.
- R. Chen, P. Yin, L. Wang, C. Liu, Y. Niu, W. Wang, Y. Jiang, Y. Liu, J. Liu, J. Qi, J. You, H. Kan and M. Zhou, Association between ambient temperature and mortality risk and burden: time series study in 272 main Chinese cities, BMJ, 2018, 363, k4306 CrossRef PubMed.
- J. Kysely, L. Pokorna, J. Kyncl and B. Kriz, Excess cardiovascular mortality associated with cold spells in the Czech Republic, BMC Public Health, 2009, 9, 19 CrossRef PubMed.
- P. D. Houck, J. E. Lethen, M. W. Riggs, D. S. Gantt and G. J. Dehmer, Relation of atmospheric pressure changes and the occurrences of acute myocardial infarction and stroke, Am. J. Cardiol., 2005, 96(1), 45–51 CrossRef.
- S. Y. Chu, M. Cox, G. C. Fonarow, E. E. Smith, L. Schwamm, D. L. Bhatt, R. A. Matsouaka, Y. Xian and K. N. Sheth, Temperature and Precipitation Associate With Ischemic Stroke Outcomes in the United States, J. Am. Heart Assoc., 2018, 7(22), e010020 CrossRef.
- R. Khamutian, K. Sharafi, F. Najafi and M. Shahhoseini, Association of air pollution and hospital admission for cardiovascular disease: a case study in Kermanshah, Iran, Zahedan J. Res. Med. Sci., 2014, 16(11), 43–46 Search PubMed.
- F. Wright and R. B. Weller, Risks and benefits of UV radiation in older people: More of a friend than a foe?, Maturitas, 2015, 81(4), 425–431 CrossRef CAS PubMed.
-
B. Pichat, Population Aging and its Social Economic Implication, 1956, pp. 15–27 Search PubMed.
- K. L. Shumake, J. D. Sacks, J. S. Lee and D. O. Johns, Susceptibility of older adults to health effects induced by ambient air pollutants regulated by the European Union and the United States, Aging: Clin. Exp. Res., 2013, 25(1), 3–8 Search PubMed.
Footnote |
† Chenguang Wu and Hang Dong contributed equally to this work and are co-first authors. |
|
This journal is © The Royal Society of Chemistry 2023 |
Click here to see how this site uses Cookies. View our privacy policy here.