DOI:
10.1039/D3RA03992G
(Paper)
RSC Adv., 2023,
13, 26630-26639
Metabolomics analysis of MnO2 nanosheets CDT for breast cancer cells and mechanism of cytotoxic action†
Received
14th June 2023
, Accepted 30th August 2023
First published on 6th September 2023
Abstract
Chemodynamic therapy (CDT) has received more and more attention as an emerging therapeutic strategy, especially transition metals with Fenton or Fenton-like activity have good effects in CDT research, manganese dioxide nanosheets (MnO2 NSs) and their complexes have become one of the most favored nanomaterials in CDT of tumors. CDT is mainly based on the role of reactive oxygen species (ROS) in tumor treatment, which have clear chemical properties and produce clear chemical reactions. However, their mechanism of interaction with cells has not been fully elucidated. Here, we performed CDT on mouse breast cancer cells (4T1) based on MnO2 NSs, extracted the metabolites from the 4T1 cells during the treatment, and analyzed the differences in metabolites by using high-resolution liquid chromatography-mass spectrometry (LC-MS). Untargeted metabolomics studies were conducted using the relevant data. This study mainly explored the changes in MnO2 NSs on the metabolite profile of 4T1 cells and their potential impact on tumor therapy, in order to determine the mechanism of action of MnO2 NSs in the treatment of breast cancer. The results of the study showed the presence of 11 different metabolites in MnO2 NSs CDT for 4T1 tumor cells, including phosphoserine, sphingine, phosphocholine, and stearoylcarnitine. These findings provide a deeper understanding of breast cancer treatment, and are beneficial for the further research and clinical application of CDT.
Introduction
Breast cancer accounts for 30% of all cancers diagnosed in women, with a global mortality rate of 15%.1 One in eight women and a small number of men develop breast cancer during their lifetime.2 Besides gender, breast cancer is associated with the degree of socioeconomic development and lifestyle.3 The major factors influencing this disease include mutations in BRCA1 and BRCA2, high breast density, family history, late-term pregnancy, lack of physical activity, smoking, and alcohol consumption.4,5 Five types of breast cancer are known: metastatic cancer, inflammatory carcinoma of the breast, infiltrating lobular carcinoma of the breast, infiltrating ductal cell carcinoma, and inflammatory breast cancer.6,7 Despite considerable research, the treatment of breast cancer remains elusive, emphasizing the need to keep exploring new treatments.
The main methods of cancer treatment in clinics include surgery, chemotherapy, and radiotherapy.8 Recently, chemodynamic therapy (CDT), which exploits in situ Fenton or Fenton-like reactions, has received much attention.9,10 In a Fenton-like reaction, hydrogen peroxide (H2O2) decomposes to produce highly toxic hydroxyl radicals (˙OH), which can induce cancer cell death.11 CDT is a novel approach for treating breast cancer, with a better treatment profile and satisfactory biosafety. However, it also generates high levels of glutathione (GSH) in cancer cells. GSH protects cancer cells by eliminating ˙OH, thereby blunting the effectiveness of CDT.12 MnO2 nanosheets (MnO2 NSs) can undergo a redox reaction with GSH to produce Mn2+ and glutathione disulfide, preventing the production of ˙OH from being scavenged and enhancing the efficacy of CDT.13
The occurrence, treatment, and rehabilitation of diseases are accompanied by varying degrees of metabolic changes.14,15 Studying diseases based on these metabolite changes is a promising approach for establishing a cure. During cancer treatment, different methods and drugs can modify the levels of different metabolites and inhibit or activate different pathways in the cancer cells. In addition, metabolic changes in cancer cells prove underlying metabolic defects. Accordingly, the spread of cancer cells can be decelerated by adopting the appropriate measures. CDT induces apoptosis by generating reactive oxygen species (ROS), while MnO2 NSs can modulate intracellular levels of GSH, H2O2, and ROS, which can influence some metabolites.16–19 Studying the metabolomics associated with CDT can provide great insights into the mechanisms of this cancer treatment method.
In this work, the ability of MnO2 NSs to kill tumor cells by generating ROS was verified by co-culturing the nanosheets with a mouse breast cancer cell line (4T1). As illustrated in Scheme 1, mass spectrometry was used to profile the metabolites in the treated 4T1 cells. Finally, metabolites and metabolic pathways were analyzed using cell-based metabolomics to explore the effects and mechanisms of CDT.
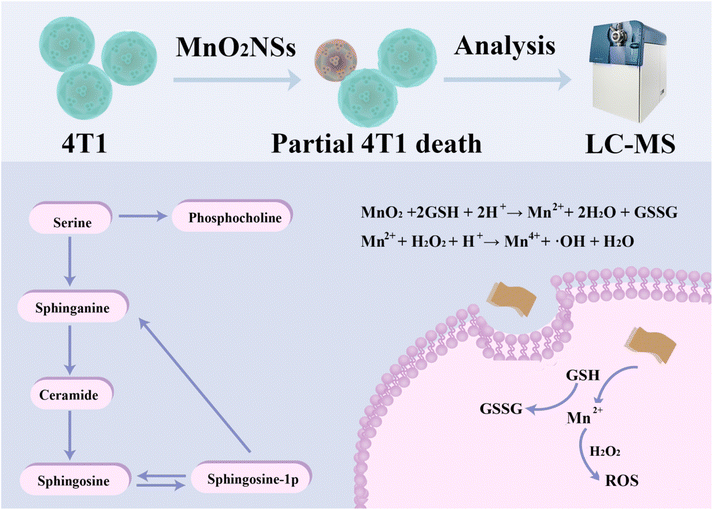 |
| Scheme 1 Schematic illustration of the metabolomics of MnO2 NSs for chemodynamic therapy of breast cancer cells. | |
Experimental section
Materials
Manganese chloride (MnCl2), tetramethylammonium hydroxide (TMAH), methylene blue (MB), H2O2, GSH, dimethyl sulfoxide (DMSO), 2-Chloro-Phe-OH [H-Phe(2-Cl)-OH 98%] were purchased from Shanghai Aladdin Bio-Chem Technology Co., Ltd. Methanol (CH3OH, HPLC) and acetonitrile (CH3CN, HPLC) were purchased from Fisher Chemical. RPMI-1640 medium was purchased from Wolcavi Biotechnology Co., Ltd (Beijing, China). Phosphate buffer and penicillin solution were purchased from Beijing Leagene Biotech Co., Ltd. Trypsase, DCFH-DA, Calcein acetoxymethyl ester (Calcein-AM) and propidium iodide (PI) were purchased from Beyotime Biotechnology (Shanghai, China).
Synthesis of MnO2 NSs
MnO2 NSs were synthesized according to a previously described method.20,21 In short, 20 mL of 0.6 M tetramethylammonium hydroxide pentahydrate containing 3% w/v H2O2 was rapidly added to 10 mL of an aqueous solution of 0.3 M MnCl2 in 15 s with stirring to attain a dark brown solution, which was stirred vigorously for 12 h at room temperature. The final product was collected by centrifugation, washed twice with ultrapure water and methanol, and dried at 60 °C for 12 h to obtain MnO2 NSs. To obtain this solution, 80 mg of MnO2 NSs was dispersed in 20 mL of ultrapure water and sonicated for 10 h. The solution was stored at 4 °C.
Fenton-like reactions and ROS production
Methylene blue (MB) was used to evaluate the generation of ˙OH.22 First, we studied the degradation of MB in Mn2+-mediated Fenton-like reactions after adding different concentrations of GSH to an MnO2 NSs solution. The reaction comprised a 25 mM sodium bicarbonate buffer containing 10 μg per mL MB, 10 mM H2O2, 0.5 mM MnO2 NSs solution, and different concentrations of GSH (0.2, 0.5, 1.0, 5.0, and 10.0 mM). The degradation of MB was monitored at 665 nm using an ultraviolet-visible (UV-Vis) spectrophotometer (UV2600; SHIMADZU, Japan). The absorbance change over time of the MB solution, which contained 1.0 mM GSH, was measured using a UV-Vis spectrophotometer.
Cell culture
4T1 cells were cultured in Dulbecco's Modified Eagle Medium with 10% fetal bovine serum and 10% penicillin–streptomycin at 37 °C along with 5% CO2.23 The medium was changed or the cells were passaged every two days.
Intracellular ROS detection
Intracellular ROS levels were estimated using 2′,7′-dichlorodihydrofluorescein diacetate (DCFH-DA). 4T1 cells were seeded in confocal microscopy dishes at a density of 3 × 105 cells per well for 24 h, and incubated with a final concentration as 200 μg mL−1 of MnO2 NSs solution for 6 h. After washing with phosphate-buffered saline, the cells were incubated with DCFH-DA for 30 min, after which images were captured using a confocal microscope.
Cytotoxicity
Methyl thiazolyl tetrazolium (MTT) was used to evaluate the cytotoxicity of MnO2 NSs toward 4T1 cells. Briefly, 4T1 cells were seeded into 96-well plates at a density of 6 × 103 to 10 × 103 cells per well, cultured for 24 h, treated with different concentrations of MnO2 NSs (0, 2, 5, 10, 20, 50, 100, and 200 μg mL−1), cultured for another 24 h, washed twice, incubated with 20 μL of MTT for 4 h at 37 °C, and finally with 100 μL of dimethyl sulfoxide. UV absorbance was measured at 540 nm and 630 nm using a microplate reader (Cytation 5, BioTek, USA).
Calcein-AM and PI were used to stain the live and dead cells respectively. 4T1 cells were cultured with Calcein-AM (1 μL, 1 mM) and PI (2 μL, 1.5 mM) for 30 min, and handled in the same way as they were while measuring intracellular ROS levels.
Extraction of cellular metabolites
Cellular metabolites were extracted using a previously published method with slight modifications.24 4T1 cells were seeded into 6-well plates at a density of 1 × 106 cells per well, and left to adhere overnight. Subsequently, they were incubated with MnO2 NSs (10, 20, and 40 μg mL−1) for 24 h, washed twice with cold phosphate-buffered saline, digested using trypsin, and collected in centrifuge tubes. The cellular suspensions were centrifuged twice (12
000 rpm for 3 min) to obtain cell pellets, to which 1 mL of precooled methanol, acetonitrile, and ultrapure water (2
:
2
:
1, v/v) was added. The suspension was shaken for 30 s, disrupted for 3 min in an ice bath using a cell disruptor, incubated at −20 °C for 1 h, and centrifuged at 4 °C (12
000 rpm for 10 min). The supernatant was aspirated and filtered through a 0.22 μm microporous membrane before mass detection. The same volume of sample was mixed and used detected as a quality control sample. All samples were stored at −80 °C prior to further analysis.
Untargeted metabolomics analysis
We used a high-resolution mass spectrometer (Triple TOF 6600+; SCIEX) with an untargeted metabolomic analysis method to analyze the metabolites.25 The samples were numbered and randomly sampled. Quality control samples were injected before, during, and after sample collection to reduce the inaccuracies originating from instrument fluctuations. The Aquity UPLC column was used at 40 °C. The temperature of the sampler was 4 °C and the injection volume was 2 μL. Mobile phase A was ultrapure water containing 0.1% formic acid and mobile phase B was acetonitrile containing 0.1% formic acid. The flow rate was 0.3 mL min−1.
Data analysis
The raw data of the metabolites obtained by mass spectrometry were treated with a mass spectrometer to obtain the chromatogram and extract the molecular features. The data were converted into WIFF format files. We performed data alignment and normalization on the raw data, and analyzed preprocessed data to obtain metabolites and pathways using MetDNA (https://metdna.zhulab.cn). When the P-value was less than 0.05, the fold change was more than two. The preprocessed data were imported into MetaboAnalyst 5.0 (https://www.metaboanalyst.ca). Invalid data with large differences were filtered out using relative standard deviation, interquartile range, and normalization.26,27 We observed n values in the experiment, but n was much smaller than the peak number p in the metabolomics plot. Because we could not proceed with the parameter estimation, the traditional method of standard parameter statistics was inaccurate. Many metabolites were not closely related to the problems that we needed to study, but the changes in these metabolites could induce some changes if irrelevant questions.
Raw data were subjected to principal component analysis (PCA), partial least square-discriminant analysis (PLS-DA), and orthogonal PLS-DA (OPLS-DA). PCA is a multidimensional data analysis technique that can identify potential factors and visualize the relationships between biomarkers. However, it cannot handle complex and large amounts of data. PLS-DA and OPLS-DA are expanded models of PCA, helping to discriminate between important biomarkers.28,29 However, it is not capable of handling complex and large amounts of metabolite data. PLS-DA and OPLS-DA are expanded models of PCA and powerful tools for discriminating important biomarkers.30,31 These three analytical methods were used together to make experimental results more accurate and to better verify the differences between the experimental and control groups. The fold change was measured as the relative change in the concentration of metabolites under different conditions. We used t-tests and volcano plots to stabilize the results.32
Results and discussion
Characterizations of MnO2 NSs
The morphology and microstructures of MnO2 NSs (Fig. 1a) were observed using TEM. The nanosheets obtained after oxidation with H2O2 exhibited a homogeneous flake structure. Images obtained through energy-dispersive X-ray spectroscopy (Fig. 1b) and high-angle annular dark-field-scanning TEM, as well as the corresponding elemental mapping images of MnO2 NSs (Fig. 1c–e) showed that elemental Mn and O coexist and are distributed throughout the nanosheets. The X-ray photoelectron spectroscopy (XPS) data confirm Mn is 4+ valence state and Mn 2p1/2 and 2p3/2 peaks are shown around 641.5 and 653.2 eV, respectively (Fig. S1†). FTIR spectrum show characteristic adsorption band of MnO2 around 1450 and 960 cm−1. The nanosheets solution was dark brown, and its maximum UV absorbance wavelength was approximately 380 nm (Fig. 1f), which is consistent with previous literature.33,34 The size distribution of the nanosheets was mainly concentrated at approximately 200 nm (Fig. 1g).
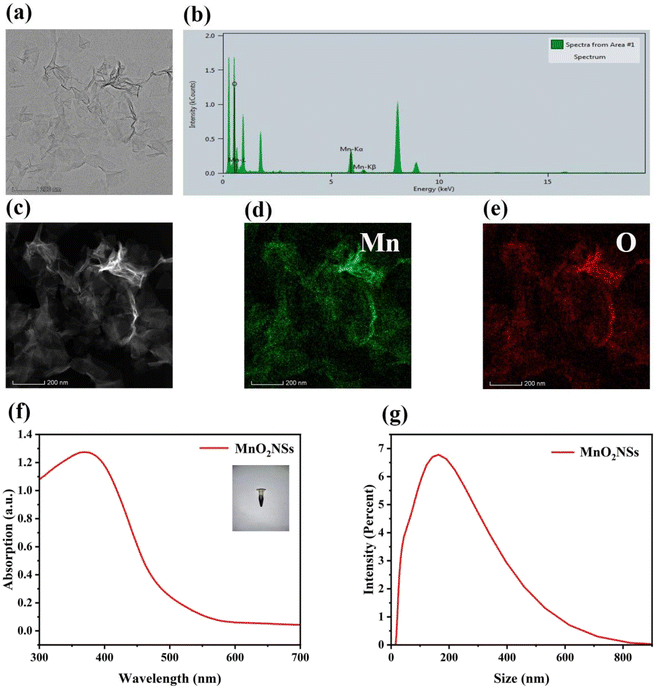 |
| Fig. 1 (a) TEM images of MnO2 NSs. (b) Energy-dispersive X-ray spectroscopy of MnO2 NSs. (c–e) High-angle annular dark-field-scanning TEM images and the corresponding elemental mapping images of MnO2 NSs. (f) UV spectra of MnO2 NSs. (g) Size distribution of MnO2 NSs. | |
Effects of MnO2 NSs on ˙OH production via Fenton-like reactions
The absorbance of MB at 650 nm decreased when the concentration of GSH in the MnO2 NSs solution increased from 0.2 to 1 mM (Fig. 2a). In addition, the blue color of the MB mixture faded. The absorbance of MB gradually increased when the concentration of GSH was more than 1 mM, and the color of the mixture slowly recovered. The absorbance of MB decreased with increasing reaction time when the concentration of GSH was 1 mM, indicating that MB absorbance was related to the reaction time (Fig. 2b and c).
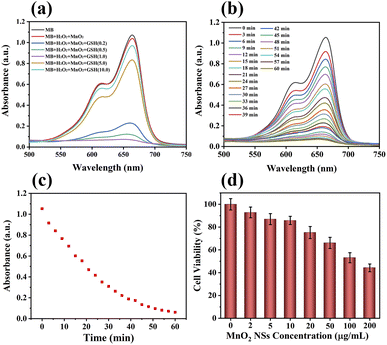 |
| Fig. 2 (a) MB degradation by H2O2 plus various concentrations of GSH-treated MnO2 NSs solutions (GSH: mM). (b) MB degradation after various reaction times. (c) Changes in MB degradation by H2O2 and GSH-treated MnO2 NSs with different reaction times, measured as changes in the UV absorption intensity at 665 nm. (d) In vitro cytotoxicity of MnO2 NSs (0–200 μg mL−1). | |
Cancer cells contain excessive amounts of GSH and 10–100 μM endogenous H2O2.35–38 Being an intracellular antioxidant, GSH has a strong clearing effect on ˙OH, which is produced by a CDT reagent. This experiment simulated the chemical reaction of MnO2 NSs with tumor cells. The highly active ˙OH can destroy the conjugate structure of the organic dye, resulting in the color fading of MB solutions.
In this experiment, the MnO2 NSs underwent a redox reaction with GSH to produce Mn2+ and glutathione disulfide. Mn2+ transforms H2O2 into toxic ˙OH, and MnO2 consumes GSH to inhibit the scavenging of ˙OH. Therefore, the absorbance of MB decreased with increasing GSH concentrations up to 1 mM. At concentrations greater than 1 mM, excessive GSH scavenged ˙OH, leading to a gradual increase in MB absorbance and gradual recovery of the solution color.
Intracellular ROS assay
DCFH-DA can be oxidized by ROS to green fluorescent 2′,7′-dichlorofluorescein (DCF), confirming the generation of ROS.39 Green fluorescence was produced in cells after adding 20 μL of MnO2 NSs solution (100 μg mL−1), indicating the presence of ROS (Fig. 3). Low levels of ROS play an important role in maintaining intracellular balance, however, high levels can irreversibly damage carbohydrates, lipids, DNA, and proteins.40,41 MnO2 NSs kill cancer cells by producing large amounts of ROS.
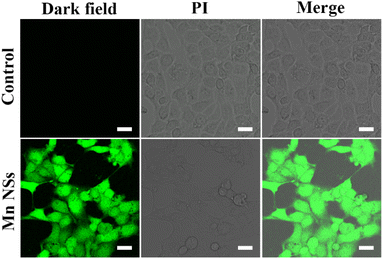 |
| Fig. 3 Fluorescence and bright-field images of ROS generated in 4T1 cells that were treated with MnO2 NSs for 6 h. Green intracellular fluorescence originated from DCF, the oxidation product of DCFH-DA. Scale bar: 25 μm. | |
Cytotoxicity assay
We investigated the cytotoxicity of different concentrations of MnO2 NSs (0, 2, 5, 10, 20, 50, 100, 200 μg mL−1) toward 4T1 cells (Fig. 2d). The survival rate of 4T1 cells decreased with increasing nanosheet concentration. We selected concentrations of 10, 20, and 40 μg mL−1 for metabolomics because nanosheets at these concentrations affected the metabolism of 4T1 cells without causing significant cell death.
Cell death and survival
Calcein-AM can fluorescently stain live cells green, whereas PI can fluorescently stain dead cells red. The experimental groups showed much red fluorescence compared to the control groups (Fig. 4), demonstrating that the addition of MnO2 NSs solution (50 μg mL−1) induced apoptosis in 4T1 cells.
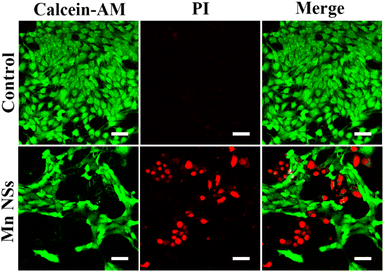 |
| Fig. 4 Calcein-AM/PI double staining of 4T1 cells treated with MnO2 NSs at 0 or 50 μg mL−1. Scale bar: 50 μm. | |
Differential metabolite analysis
Three concentrations of MnO2 NSs (10, 20, and 40 μg mL−1) were used as the low, medium, and high concentrations, respectively. When 4T1 cells were treated with a low nanosheet concentration, a small overlap was observed between the PCA plots of the experimental and control groups, implying that the difference in metabolites between the two groups was not particularly pronounced. This difference became apparent as the nanosheet concentration increased (Fig. 5a–c). The distributions of the experimental and control groups did not aggregate and obvious differences were observed (Fig. 5d). However, the differences between the experimental groups were not obvious. PLS-DA corroborated the conclusions obtained by PCA (Fig. 5e–h).
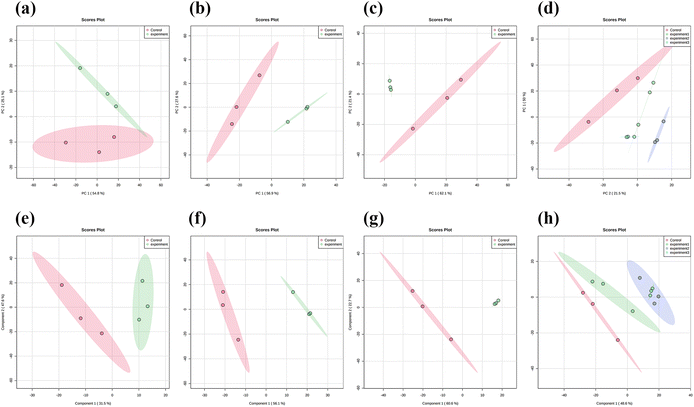 |
| Fig. 5 (a–c) Principal component analysis (PCA) results for 4T1 cells treated with 0, 10, 20, and 40 μg mL−1 of MnO2 NSs. (d) PCA results of all experimental and control groups. (e–g) Partial least square-discriminant analysis (PLS-DA) results of the metabolites in 4T1 cells subjected to the same treatments. (h) PLS-DA results of all experimental and control group. | |
Fig. 6a, e, and i show the OPLS-DA of the three experimental groups, which were different from the control groups when the extraneous variables were removed. In the low concentration group, the R2X values were 0.206, 0.401, 0.234, and 0.0942; the R2Y values were 0.926, 0.0322, 0.0251, and 0.0162; and the Q2 values were 0.534, 0.224, 0.0749, and 0.128, respectively (Fig. 6d, h and l). In the middle concentration group, the R2X values were 0.471 and 0.317, the R2Y values were 0.95 and 0.0445, and the Q2 values were 0.738 and 0.0156. In the high concentration group, the R2X values were 0.502 and 0.283, the R2Y values were 0.903, 0.094, and 0.0162, and the Q2 values were 0.803 and 0.0823, respectively. Variable importance in projection (VIP) plays an important role in measuring the weights of variables in OPLS-DA. Differential metabolites were significant when the VIP was >1. The VIP scores were calculated for all three groups (Fig. 6b, c, f, g, j and k). The top 15 differential metabolites, based on the VIP score, were screened for each group. According to the fold change analysis, the levels of 29, 499, and 1073 metabolites were increased, while those of 367, 518, and 143 metabolites were decreased in the three experimental groups (Fig. 7a–c). According to the t-test analysis, the levels of 434, 487, and 1729 metabolites were found to be upregulated, while those of 367, 518, and 143 metabolites remained unchanged in the three experimental groups, respectively (Fig. 7d–f). Finally, according to the volcano plots, the levels of 10, 803, and 473 metabolites increased, whereas those of 310, 620, and 328 metabolites decreased, respectively (Fig. 7g–i).
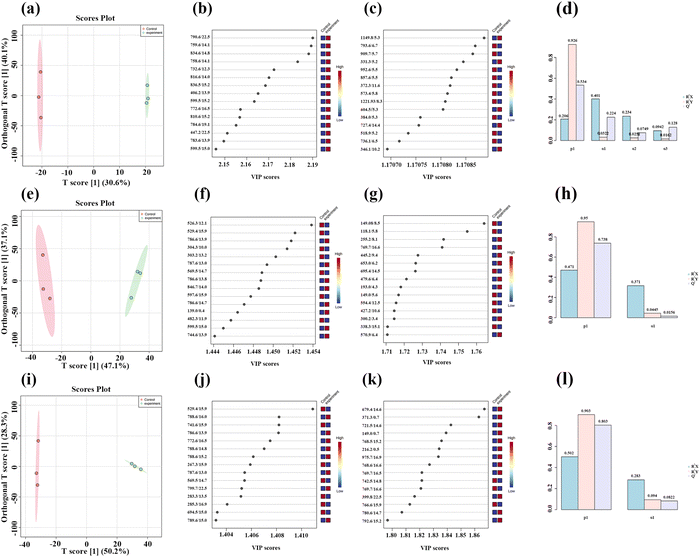 |
| Fig. 6 (a, e and i) PLS-DA results for 4T1 cells treated with 0, 10, 20, and 40 μg mL−1 of MnO2 NSs. (b, c, f, g, j and k) VIP scores of the three concentration groups. (d, h and l) R2X, R2Y, Q2 values. | |
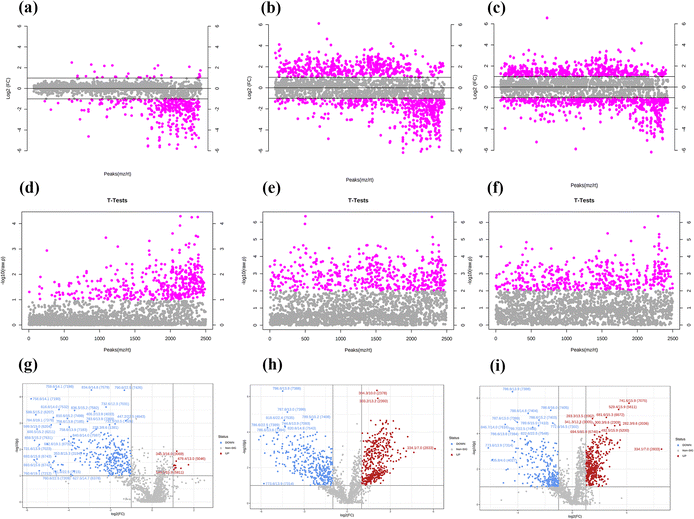 |
| Fig. 7 (a–c) T-test results comparing the metabolites in untreated 4T1 cells with those in cells treated with 10, 20, and 40 μg mL−1 of MnO2 NSs. (d–f) Fold change models of the metabolites in untreated 4T1 cells and of those in cells treated with 10, 20, and 40 μg mL−1 of MnO2 NSs. (g–i) Volcano plots of the metabolites in untreated 4T1 cells and of those in cells treated with 10, 20, and 40 μg mL−1 of MnO2 NSs. | |
According to the fold change analysis, the levels of 29, 499, and 1073 metabolites were increased while those of 367, 518, and 143 metabolites were decreased in the three experimental groups (Fig. 7a–c). According to the t-test analysis, the levels of 434, 487, and 1729 metabolites were found to be upregulated, while those of 367, 518, and 143 metabolites remained unchanged in the three experimental groups, respectively (Fig. 7d–f). Finally, according to the volcano plots, the levels of 10, 803, and 473 metabolites increased, while those of 310, 620 and 328 metabolites decreased, respectively (Fig. 7g–i).
Untargeted metabolomics tests
The raw data were analyzed using MetDNA, and 7817 peaks were detected, among which 74 metabolite peaks were identified using MetDNA. Eleven different metabolites were identified (Table 1), mainly involving phosphoserines, sphingosine, phosphocholine, and stearoylcarnitines. When 4T1 cells were treated with a low nanosheet concentration, the metabolites produced were similar to those produced in untreated cells, as evidenced by a crossover in the heat map (Fig. 8). The medium- and high-concentration treatments produced some obvious differences, and the heat maps of the same concentration treatments were clustered.
Table 1 Metabolic differences
m/z |
Name |
790.5590 |
2-Oleoyl-1-stearoyl-sn-glycero-3-phosphoserine |
300.2894 |
Sphingosine |
524.3711 |
1-Stearoyl-sn-glycerol 3-phosphocholine |
524.3711 |
1-Stearoyl-2-hydroxy-sn-glycero-3-phosphocholine |
524.3707 |
1-Stearoyl-sn-glycerol 3-phosphocholine |
522.3575 |
1-Oleoyl-sn-glycero-3-phosphocholine |
734.5652 |
PC (16 : 0/16 : 0) |
496.3421 |
1-Palmitoyl-sn-glycero-3-phosphocholine |
428.3719 |
Stearoylcarnitine |
468.3115 |
1-Myristoyl-sn-glycero-3-phosphocholine |
298.2756 |
3-Ketosphingosine |
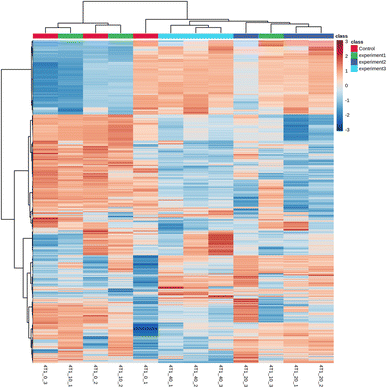 |
| Fig. 8 Heat map of differential metabolites. | |
Metabolomics pathways
Disrupting redox homeostasis by promoting ROS production and suppressing ROS clearance in cancer cells can amplify oxidative stress, leading to more effective cancer treatment.42,43 Mn4+/Cu2+ with high reduction activity are particularly advantageous in terms of kinetics and thermodynamics when catalyzing Fenton-like reactions compared to Fe2+.44–46 Mn4+ can oxidize GSH to glutathione (GSSG), thus enhancing CDT for cancer treatment by consuming GSH. The generated Mn2+ can then further catalyze H2O2 to produce ˙OH.38
The following conclusions were drawn after analyzing the metabolites and related pathways using the Kyoto Encyclopedia of Genes and Genomes. The main metabolic pathways affected included sphingolipid metabolism, ceramide biosynthesis, sphingosine biosynthesis, sphingosine degradation, glycine, serine and threonine metabolism, choline metabolism in cancer, and biosynthesis of fatty acids and unsaturated fatty acids.
The changes detected in some metabolites proved that MnO2 NSs could achieve the function of CDT while exerting other therapeutic functions as well. Serine was more abundant in the experimental groups than in the control groups. More serine produces more GSH, which affects the chemical kinetics. Serine is also involved in folate metabolism; thus, folic acid levels increase. Folic acid affects DNA synthesis, stability, and integrity and inhibits the proliferation of cancer cells.
The amount of sphingosine was decreased in the experimental groups. Sphingomyelins and their derivatives, including ceramides and sphingosine-1-phosphate (S1P), can be converted into each other. Sphingosine is an intermediate in the biosynthesis of the sphingosine base and is also present in small amounts in most complex sphingolipids. Sphingosine is one of sphingomyelin and its derivatives, which also include ceramide (CER), S1P. The three can be converted into each other, CER is the substrate to produce sphingosine, sphingosine is acylated, and CER is produced. CER, sphingosine, and S1P mainly form the core of sphingolipid metabolic pathways and control multiple physiological functions by regulating multiple signal cascades. CER negatively regulates Rb and CDK2 proteins, which act as inhibitors of cell proliferation in normal breast epithelium (Fig. 9). Ceramide metabolism promoted the death of 4T1 cell lines and protected the basement membrane to inhibit the migration and invasion of cancer cells.
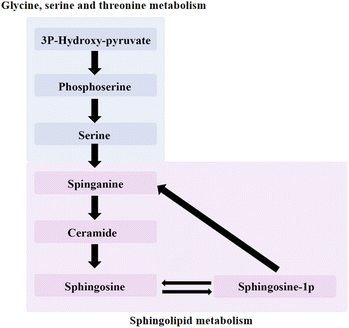 |
| Fig. 9 Relationship between glycine, serine and threonine metabolism and sphingolipid metabolism pathway. | |
The decrease in phosphocholine in the experimental groups compared to that in the control group indicated that MnO2 NSs could kill cancer cells by controlling phosphocholine metabolism. The levels of phosphorylcholine and total choline are elevated in cancer cells, and inhibiting them could decelerate the transport rate of choline to reduce the spread of 4T1 cells.
Conclusions
In summary, MnO2 NSs destroys the antioxidant capacity of tumor cells by depleting GSH, which simultaneously produces Mn2+. The Mn2+ produces ˙OH through a Fenton-like process in the tumor microenvironment to realize CDT. Thus, MnO2 NSs can be used to achieve cancer CDT by simultaneously destroying the cell's antioxidant defense system and delivering ˙OH generators to cells. We investigated the effect of MnO2 NSs on metabolites in 4T1 cells lines. In addition to CDT, three metabolic differences related to cancer cell survival were identified using metabolomics and mass spectrometry. These findings can pave the way for better applications of MnO2 NSs in cancer therapy.
Author contributions
Conceptualization: Jian Liu, Changchun Wen and Xiang-cheng Lin; original draft writing: Jian Liu, Juan Long, Jing Zhang; illustrations: Jian Liu, Miaomiao Hu; proofreading: Minzhe Li, Changchun Wen and Xiang-Cheng Lin; all the authors have read and approved the final manuscript.
Conflicts of interest
There are no conflicts to declare.
Acknowledgements
This work was financially supported by the National Natural Science Foundation of China (21864009, 22064018), Natural Science Foundation of Guangxi Zhuang Autonomous Region (2018GXNSFAA281197), Bagui Scholars Program of Guangxi Zhuang Autonomous Region, and Guangxi Collaborative Innovation Center of Structure and Property for New Energy and Materials.
References
- N. Wu, L. Wei, L. Li, F. Li, J. Yu and J. Liu, Int. J. Clin. Oncol., 2022, 27, 495–511 CrossRef CAS.
- J. Zheng, D. Meng, X. Zheng, Y. Zhang and H. Chen, Int. J. Pharm., 2021, 603, 120644 CrossRef CAS.
- J. Soriano, I. Mora-Espí, M. E. Alea-Reyes, L. Pérez-García, L. Barrios, E. Ibáñez and C. Nogués, Sci. Rep., 2017, 7, 41340 CrossRef CAS PubMed.
- S. Li, F. A. Al-Misned, H. A. El-Serehy and L. Yang, Arabian J. Chem., 2021, 14, 102931 CrossRef CAS.
- K. R. Mattaini, M. R. Sullivan and M. G. Vander Heiden, J. Cell Biol., 2016, 214, 249–257 CrossRef CAS PubMed.
- J. W. Locasale, A. R. Grassian, T. Melman, C. A. Lyssiotis, K. R. Mattaini, A. J. Bass, G. Heffron, C. M. Metallo, T. Muranen, H. Sharfi, A. T. Sasaki, D. Anastasiou, E. Mullarky, N. I. Vokes, M. Sasaki, R. Beroukhim, G. Stephanopoulos, A. H. Ligon, M. Meyerson, A. L. Richardson, L. Chin, G. Wagner, J. M. Asara, J. S. Brugge, L. C. Cantley and M. G. Vander Heiden, Nat. Genet., 2011, 43, 869–874 CrossRef CAS PubMed.
- S. C. Moore, K. M. Mazzilli, J. N. Sampson, C. E. Matthews, B. D. Carter, M. C. Playdon, Y. Wang and V. L. Stevens, Metabolites, 2021, 11, 95 CrossRef CAS PubMed.
- X. Li, Y. Wang, Q. Shi, N. Zhen, J. Xue, J. Liu, D. Zhou and H. Zhang, ACS Omega, 2022, 7, 29256–29265 CrossRef CAS.
- Y. Li, P. Zhao, T. Gong, H. Wang, X. Jiang, H. Cheng, Y. Liu, Y. Wu and W. Bu, Angew. Chem., Int. Ed., 2020, 59, 22537–22543 CrossRef CAS.
- T. He, Y. Yuan, C. Jiang, N. T. Blum, J. He, P. Huang and J. Lin, Angew. Chem., Int. Ed., 2021, 60, 6047–6054 CrossRef CAS.
- X. Liu, Y. Liu, J. Wang, T. Wei and Z. Dai, ACS Appl. Mater. Interfaces, 2019, 11, 23065–23071 CrossRef CAS.
- X. Li, X. Zhang, W. Zhang, L. Li, W. Gao, X. Zhang and D. Gao, ACS Sustainable Chem. Eng., 2020, 8, 13206–13214 CrossRef CAS.
- J. Ou, H. Tian, J. Wu, J. Gao, J. Jiang, K. Liu, S. Wang, F. Wang, F. Tong, Y. Ye, L. Liu, B. Chen, X. Ma, X. Chen, F. Peng and Y. Tu, ACS Appl. Mater. Interfaces, 2021, 13, 38050–38060 CrossRef CAS.
- H. M. I. Abdallah, N. M. Ammar, M. F. Abdelhameed, A. E.-N. G. E. Gendy, T. I. M. Ragab, A. M. Abd-ElGawad, M. A. Farag, M. S. Alwahibi and A. I. Elshamy, Biology, 2020, 9, 195 CrossRef CAS PubMed.
- M. Gaggini, F. Carli, C. Rosso, E. Buzzigoli, M. Marietti, V. Della Latta, D. Ciociaro, M. L. Abate, R. Gambino, M. Cassader, E. Bugianesi and A. Gastaldelli, Hepatology, 2018, 67, 145–158 CrossRef CAS PubMed.
- C. Jia, Y. Guo and F.-G. Wu, Small, 2022, 18, 2103868 CrossRef CAS.
- Y. Liu, Y. Jiang, M. Zhang, Z. Tang, M. He and W. Bu, Acc. Chem. Res., 2018, 51, 2502–2511 CrossRef CAS PubMed.
- H. Li, Y. Liu, B. Huang, C. Zhang, Z. Wang, W. She, Y. Liu and P. Jiang, Anal. Chem., 2022, 94, 10470–10478 CrossRef CAS PubMed.
- Y. Huang, S. Wu, L. Zhang, Q. Deng, J. Ren and X. Qu, ACS Nano, 2022, 16, 4228–4238 CrossRef CAS PubMed.
- F. Chen, M. Bai, Y. Zhao, K. Cao, X. Cao and Y. Zhao, Anal. Chem., 2018, 90, 2271–2276 CrossRef CAS PubMed.
- Q. Tan, R. Zhang, R. Kong, W. Kong, W. Zhao and F. Qu, Microchim. Acta, 2017, 185, 44 CrossRef.
- C. Wen, X. Guo, C. Gao, Z. Zhu, N. Meng, X.-C. Shen and H. Liang, J. Mater. Chem. B, 2022, 10, 4274–4284 RSC.
- Z. Lu, S. Liu, Y. Le, Z. Qin, M. He, F. Xu, Y. Zhu, J. Zhao, C. Mao and L. Zheng, Biomaterials, 2019, 218, 119190 CrossRef CAS.
- J. Zhang, J. Shi, S. Han, P. Zheng, Z. Chen and G. Jia, Toxicol. Appl. Pharmacol., 2022, 444, 116020 CrossRef CAS PubMed.
- X.-C. Lin, F. Chen, K. Zhang, J. Li, J.-H. Jiang and R.-Q. Yu, Anal. Chem., 2022, 94, 6120–6129 CrossRef CAS PubMed.
- R. A. van den Berg, H. C. J. Hoefsloot, J. A. Westerhuis, A. K. Smilde and M. J. van der Werf, BMC Genomics, 2006, 7, 142 CrossRef PubMed.
- G. Nyamundanda, L. Brennan and I. C. Gormley, BMC Bioinf., 2010, 11, 571 CrossRef PubMed.
- A. Giuliani, Drug Discovery Today, 2017, 22, 1069–1076 CrossRef CAS PubMed.
- K. Tanabe, C. Hayashi, T. Katahira, K. Sasaki and K. Igami, Comput. Struct. Biotechnol. J., 2021, 19, 1956–1965 CrossRef CAS PubMed.
- M. Hayashi, K. Matsuo, K. Tanabe, M. Ikeda, M. Miyazawa, M. Yasaka, H. Machida, M. Shida, T. Imanishi, B. H. Grubbs, T. Hirasawa and M. Mikami, Cancers, 2019, 11, 591 CrossRef CAS PubMed.
- K. Matsuo, K. Tanabe, M. Hayashi, M. Ikeda, M. Yasaka, H. Machida, M. Shida, K. Sato, H. Yoshida, T. Hirasawa, T. Imanishi and M. Mikami, Cancers, 2020, 12, 2374 CrossRef CAS PubMed.
- K. Ortmayr, V. Charwat, C. Kasper, S. Hann and G. Koellensperger, Analyst, 2017, 142, 80–90 RSC.
- S. Rong, P. Zhang, J. Wang, F. Liu, Y. Yang, G. Yang and S. Liu, Chem. Eng. J., 2016, 306, 1172–1179 CrossRef CAS.
- Z. Fan, Z. Zhou, W. Zhang, X. Zhang and J.-M. Lin, Talanta, 2021, 225, 121989 CrossRef CAS.
- R. Kumar, W. S. Shin, K. Sunwoo, W. Y. Kim, S. Koo, S. Bhuniya and J. S. Kim, Chem. Soc. Rev., 2015, 44, 6670–6683 RSC.
- G. Yang, L. Xu, Y. Chao, J. Xu, X. Sun, Y. Wu, R. Peng and Z. Liu, Nat. Commun., 2017, 8, 902 CrossRef PubMed.
- L.-S. Lin, J. Song, L. Song, K. Ke, Y. Liu, Z. Zhou, Z. Shen, J. Li, Z. Yang, W. Tang, G. Niu, H.-H. Yang and X. Chen, Angew. Chem., Int. Ed., 2018, 57, 4902–4906 CrossRef CAS.
- H. Wang, D. H. Bremner, K. Wu, X. Gong, Q. Fan, X. Xie, H. Zhang, J. Wu and L.-M. Zhu, Chem. Eng. J., 2020, 382, 122848 CrossRef CAS.
- K. Sun, Z. Gao, Y. Zhang, H. Wu, C. You, S. Wang, P. An, C. Sun and B. Sun, J. Mater. Chem. B, 2018, 6, 5876–5887 RSC.
- Z. Zhou, J. Song, R. Tian, Z. Yang, G. Yu, L. Lin, G. Zhang, W. Fan, F. Zhang, G. Niu, L. Nie and X. Chen, Angew. Chem., Int. Ed., 2017, 56, 6492–6496 CrossRef CAS PubMed.
- D.-K. Ji, Y. Zhang, Y. Zang, J. Li, G.-R. Chen, X.-P. He and H. Tian, Adv. Mater., 2016, 28, 9356–9363 CrossRef CAS PubMed.
- X.-A. Yu, M. Lu, Y. Luo, Y. Hu, Y. Zhang, Z. Xu, S. Gong, Y. Wu, X.-N. Ma, B.-Y. Yu and J. Tian, Theranostics, 2020, 10, 371–383 CrossRef CAS.
- L. Hou, F. Gong, Z. Han, Y. Wang, Y. Yang, S. Cheng, N. Yang, Z. Liu and L. Cheng, Angew. Chem., Int. Ed., 2022, 61, e202208849 CrossRef CAS PubMed.
- X. Hu, L. Zhang, W. Wang, Y. Zhang and J. Wang, Colloids Surf., A, 2023, 656, 130495 CrossRef CAS.
- S. Wang, Y. Pang, S. Hu, J. Lv, Y. Lin and M. Li, Chem. Eng. J., 2023, 451, 138864 CrossRef CAS.
- B. Ma, S. Wang, F. Liu, S. Zhang, J. Duan, Z. Li, Y. Kong, Y. Sang, H. Liu, W. Bu and L. Li, J. Am. Chem. Soc., 2019, 141, 849–857 CrossRef CAS PubMed.
|
This journal is © The Royal Society of Chemistry 2023 |