DOI:
10.1039/D3RA02912C
(Review Article)
RSC Adv., 2023,
13, 22579-22592
The mathematical catalyst deactivation models: a mini review
Received
2nd May 2023
, Accepted 12th July 2023
First published on 26th July 2023
Abstract
Catalyst deactivation is a complex phenomenon and identifying an appropriate deactivation model is a key effort in the catalytic industry and plays a significant role in catalyst design. Accurate determination of the catalyst deactivation model is essential for optimizing the catalytic process. Different mechanisms of catalyst deactivation by coke and metal deposition lead to different deactivation models for catalyst activity decay. In the rigorous mathematical models of the reactors, the reaction kinetics were coupled with the deactivation kinetic equation to evaluate the product distribution with respect to conversion time. Finally, selective and nonselective deactivation kinetic models were designed to identify catalyst deactivation through the propagation of heterogeneous chemical reactions. Therefore, the present review discusses the catalyst deactivation models designed for CO2 hydrogenation, Fischer–Tropsch, biofuels and fossil fuels, which can facilitate the efforts to better represent the catalyst activities in various catalytic systems.
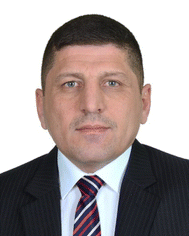 Zaidoon M. Shakor | Prof. Dr Zaidoon M. Shakor is a faculty member in the Chemical Engineering Department, University of Technology. He held a BSc (1997), MSc (1999) and PhD (2004) in chemical engineering from the Chemical Engineering Department of the University of Technology, Iraq. Experience in reaction kinetics modeling from small to large scale industrial catalytic processes. Experience in catalyst synthesis, reactive distillation, azeotropic and extractive distillation, adsorption, modeling, simulation and computational fluid dynamics (CFD). He is a specialist in applying modern simulation software such as MATLAB and HYSYS to improve the performance of chemical engineering processes. |
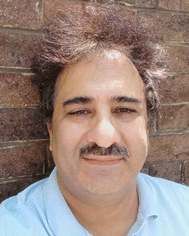 Emad N. Al-Shafei | Dr Emad Al-Shafei, a research consultant at the Research and Development Center, Saudi Aramco, Dhahran KSA. He received a PhD in Chemistry (Catalysts) from the University of Huddersfield (United Kingdom), and an MSc in Analytical Chemistry, and BSc Chemistry with Chemical Engineering from the same university. His research interest focused on catalyst development & characterization, catalytic reaction, industrial catalysts, carbon dioxide utilization for petrochemicals, dry reforming for decarbonization, nano zeolite development, and steam catalytic cracking for heavy hydrocarbons to olefins. |
1. Introduction
All catalytic processes suffer from a decrease in catalytic activity with operation time which greatly affects process stability and consequently decreases the yield and selectivity of the desired products. The time taken by loss of catalyst activity varies from a few seconds during the fluidized catalytic cracking (FCC) process to a few years during hydrodesulfurization (HDS). Accordingly, the rate of catalyst deactivation depends on the process conditions, catalyst type, reactant concentrations and contaminant with feed presence.1–3 Catalyst deactivation is classified by process type as thermal, mechanical, or chemical and by mechanisms such as fouling,4–6 poisoning,7 vapor formation, thermal degradation,8 attrition/crushing,9 vapor–solid and solid–solid reactions.10 The thermal degradation and poisoning are generally slow and irreversible, whereas coke fouling is rapid and reversible.11 Catalyst deactivation models can be categorized into selective and non-selective models according to the mechanism of loss of the active sites of the catalyst. In non-selective deactivation, the rate of active sites consumption depends on the time-on-stream (TOS) only, whereas in selective deactivation, the components of the reaction mixture are affected individually by the rate of consumption/generation of the active sites.
Hundreds of models have been applied to evaluate catalyst activity in the past eighty years, although some of these models are simple as a function of time, while others are more sophisticated as a function of multi deactivation variables.12 Voorhies et al.13 reported a simple power-law correlation for modeling the catalyst deactivation proportional to the amount of coke deposited. Similarly, an exponential form of the catalyst deactivation model developed by Weekman et al.14 was designed to characterize the catalyst activity during gas oil cracking (VGO) in a fixed-bed reactor. Dumez and Froment15 investigated five catalyst deactivation models, in which the catalyst deactivation function was multiplied by the intrinsic reaction rate to estimate the net reaction rate. While a semi-empirical relationship was studied to determine the dependency of the number of active sites on the coke content, Devoldere and Froment16 studied four models to characterize catalyst deactivation from coke formation over K–FeO based catalyst applied at the industrial scale level for ethylbenzene dehydrogenation. Nazarova et al.17 developed a mathematical model for fluidized catalytic cracking (FCC) considering the deactivation of zeolite catalyst by coke and heavy metals, such as V and Ni. The exponential form of the catalyst deactivation model was also studied to correlate the catalyst activity with the coke content. The instantaneous amount of coke was estimated by solving a differential equation based on the hydrocarbon conversion mechanisms. Ebrahimi et al.18 applied a nine-lumped kinetic model to represent the catalytic cracking of vacuum gas oil (VGO) and used the exponential formula of time-on-stream (TOS) and temperature to describe catalyst deactivation. Cordero-Lanzac et al.19 applied a complex reaction networks methodology of fast catalyst deactivation to simulate the methanol-to-olefins (MTO) process with different reactor configurations. The proposed deactivation kinetics were found to depend on the concentrations of the oxygenated and carbon-containing product in the reaction medium. Shakor et al.20 simulated an industrial scale heavy naphtha reforming process using a kinetic model involving 32 lumps and 132 reaction routes and applied first order for the catalyst deactivation rate. Despite this observation, the catalytic activity decayed to 59% of its initial value after 1225 days of operating.
The deactivation model of a catalyst plays a significant role in process simulation, reactor design and control of industrial catalytic reactors. However, several review papers10,21–25 have been published in the past few years, and specific experimental studies on catalyst deactivation for specific chemical processes have been discussed.26,27 Therefore, the present review discusses the mathematical models of catalyst deactivation designed for CO2 hydrogenation, Fischer–Tropsch, biofuels and fossil fuels which can facilitate efforts for a broader representation to comprehend the catalyst activities in current catalytic chemical processes.
2. Catalyst deactivation models
Catalyst deactivation models are either algebraic or differential expressions that correlate changes in the catalyst activity with the reaction. The catalyst activity at any time “t” is equal to a ratio of the reaction rate at any given time “t” and the reaction rate applies to fresh catalyst28 as described in eqn (1). |
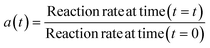 | (1) |
The initial reaction rate was measured at zero time point. Different models have been proposed in the literature to represent catalyst deactivation depending on the time-on-stream (TOS) of hydrocracking catalysts,28 the coke content of fluidized catalytic cracking catalysts,29 the operating conditions of the Fischer–Tropsch catalyst30 and the reaction mixture with two-step deactivation of reversible and irreversible models.31 These models are theoretical, empirical, or semi-empirical, and the most relevant deactivation model is summarized in the following sections.
2.1 Time dependent catalyst deactivation model
Catalyst activity decays with time, resulting in the reduction of active sites on the catalyst surface. The time-on-stream definition has been extensively applied to express approximate deactivation mechanisms. These expressions utilizes either exponential or power law and vary in deactivation order and functionality with respect to the time-on-stream. Voorhies et al.13 studied catalyst deactivation in fluidized catalytic cracking via coke formation (Fig. 1) and applied the earliest empirical catalyst activity model described in eqn (2).
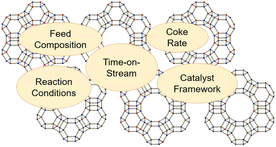 |
| Fig. 1 Catalyst deactivation of fluidized catalytic cracking catalysts. | |
The order of catalyst decay depends on the catalyst and feedstock type.32 Ozawa et al.33 studied PdO/Al2O3 with additive of La and Nd catalyst deactivation at 850 °C, during CH4 oxidation in a fixed-bed reactor at atmospheric pressure. They observed that the catalyst was deactivated by the rapid transformation of oxidation state from PdO to metallic Pd with slow particle agglomeration. Accordingly, two-term deactivation mathematical models were attributed to evaluate the catalyst activity during the reaction as described in eqn (3).
|
a(t) = r1[1/(1 − α1t)]n1 + r2[1/(1 − α2t)]n2
| (3) |
where “
r”, “
α” and “
n” are constants, subscripts 1 and 2 are the rapid and slow deactivation species respectively and “
t” is time on a stream.
Table 1 lists the catalyst deactivation models based on the time-on-stream (TOS). The main drawback of these models is that they do not take into consideration the effects of the reaction temperature, pressure and amount of coke formed over the catalyst.
34 This type of deactivation model is appropriate for systems with fast catalyst deactivation such as fluidized catalytic cracking catalysts
35–38 and catalyst used for biofuel from biomass fermentation.
39 The catalyst activity depends more on the effect of the deactivation rate over time, than on the temperature and reactant concentrations.
2.2 Temperature dependent catalyst deactivation model
Catalyst deactivation increases with time and temperature during catalytic reactions. Subsequently, increasing the severity of the reaction system leads to faster coke deposition and catalyst deactivation, in which temperature has the strongest effect on the reaction rate and consequently catalyst deactivation. The exponential function is shown in eqn (4) has rarely been used to model the deactivation of fluidized catalytic cracking and biomass-derived chemical.18,43 Whereas the catalyst deactivation coefficient (α) attributes as a function of temperature using the Arrhenius-type as described in eqn (5). The Arrhenius-type expression was used to express the effect of temperature on the catalyst deactivation coefficient as shown in eqn (6). |
 | (5) |
|
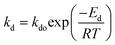 | (6) |
A generalized power-law model (eqn (7)) has been extensively applied to represent catalyst deactivation.44–49 Accordingly, by taken deactivation power as either a “1” or “2” through integration eqn (7), the algebraic expressions for catalyst activity can be achieved as shown in eqn (8) and (9) respectively:
|
 | (7) |
|
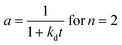 | (9) |
In addition, Chen and Lua
50 added the term residence time to the power-law deactivation model as shown in
eqn (10). Bartholomew
51 confirmed that a generalized power-law expression (GPLE) with residual activity was represented by catalyst deactivation, which was enhanced compared to the power-law expression. The GPLE catalyst deactivation model is shown in
eqn (11), and has been proposed by several authors, mainly for Fe–Co oxide catalysts in Fischer–Tropsch which deactivated from carbidization, change of metal oxidation state and carbon formation during hydrogenation reactions.
51–54 |
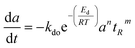 | (10) |
|
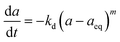 | (11) |
Residual activity “aeq” is achieved when a balance is established between the rate of deactivation and the rate of self-generation for active sites.55 Accordingly, the deactivation model derived by Honken,56 shown in eqn (12), was found to be suitable for representing the catalyst deactivation in many industrial scale applications.57–63 Rahimpour et al.64 applied a seventh-order catalyst deactivation model to study the dynamic catalyst deactivation from carbonic coke formation during naphtha reforming reactions at 502–504 °C and pressure 34–37 bar, in which carried out in a radial flow spherical reactor as shown in eqn (13).
|
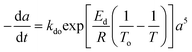 | (12) |
|
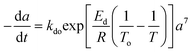 | (13) |
Temperature dependent catalyst deactivation models are presented in Table 2. These models are algebraic and/or differential forms of catalyst deactivation and are applied to deactivation of nickel catalyst from coke during CO2 hydrogenation to methane,65 biofuel,66 iron and cobalt in the Fischer–Tropsch52 catalytic deactivation reactions. For dynamic processes, the differential arrangements of the catalyst deactivation model of reforming20 and hydrocracking catalysts67 are slightly more accurate than those of algebraic route because these models represent instantaneous changes in catalyst activity.64,68
Table 1 Time dependent catalyst deactivation model of fuel catalyst systems
Deactivation model |
Catalyst |
TOSa |
Reactor Type |
Reaction system |
Reference |
TOS: time-on-stream. Not reported. |
a = Atn |
Silica–alumina |
300 min |
Fixed bed reactor |
Catalytic cracking of gas oils |
Voorhies13 |
a = αtos−n |
FCC catalyst |
0–4 s |
Riser reactor |
Gas oil cracking |
Theologos & Markatos35 |
 |
FCC catalyst |
ntb |
Commercial fluidized catalytic cracking reactor |
Vacuum gas oil cracking |
Rainer et al.36 |
FCC catalyst |
0–5 s |
Industrial fluidized catalytic cracking reactor |
Catalytic cracking of vacuum residue |
Olafadehan, et al.37 |
a = e−αtos |
Ni/MgO |
18 h |
Fixed bed reactor |
Hexane reforming |
Trunfio & Arena40 |
HZSM-5, beta, and Y-zeolite |
ntb |
Fluidized bed reactor |
Catalytic pyrolysis of gas oils |
Zhang et al.38 |
 |
FCC catalyst |
0.03–0.09 s |
Plug flow reactor |
Gas oil cracking |
Hagelberg et al.41 |
Pt-based catalyst |
40 h |
Membrane reactor |
Propane dehydrogenation |
Ricca et al.42 |
a = exp(−kdt) |
Pd/MWCNT + HZSM-5 |
5 h |
Plug flow reactor |
Biofuel: biomass fermentation |
Jadon et al.39 |
Table 2 Temperature dependent catalyst deactivation model
Deferential form |
Integral form |
Catalyst |
TOS |
Reactor type |
Reaction |
Reference |
Not reported. |
nta |
a = e−αtos |
FCC catalyst |
90 s |
Fixed bed reactor |
VGO catalytic cracking |
Ebrahimi et al.18 |
 |
FCC catalyst |
nta |
Fixed and fluidized bed |
VGO pyrolysis |
Naik et al.43 |
nta |
a = (1 − kdt) |
Pt/Al2O3 |
nta |
Fixed bed reactor |
Dehydrogenation methylcyclohexane |
Usman et al.69 |
 |
a = e−kdt |
Pt–Sn/Al2O3 |
100 h |
Fixed bed reactor |
Propane dehydrogenation |
Zangeneh et al.44 |
nta |
10–10000 h |
Packed-bed reactor |
Hydrogenation of CO2 |
Sun et al.45 |
FCC catalyst |
2–10 s |
FCC reactor |
Vacuum gas oil (VGO) catalytic cracking |
Sani et al.46 |
TS-1/SiO2 |
1–24 h |
Fixed-bed reactor |
Epoxidation of allyl chloride with hydrogen peroxide |
Lin et al.47 |
nta |
nta |
Fixed-bed reactor |
Ethanol dehydration |
Demuner et al.48 |
Pt/Al2O3 |
1225 day |
Fixed bed reactor |
Heavy naphtha reforming |
Shakor et al.20 |
 |
 |
Pd–Ag/a-Al2O3 |
120 h |
Fixed bed reactor |
Selective hydrogenation of acetylene to ethylene |
Ravanchi et al.49 |
 |
 |
Cu/AC |
12 h |
Fixed bed reactor |
Acetylene hydrochlorination |
Xu et al.70 |
nta |
 |
NiMo/Al2O3 |
0–550 h |
Continuous stirred tank basket reactor (CSTBR) |
Hydrocracking of heavy oil |
Martínez and Ancheyta67 |
 |
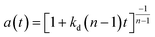 |
H2O2 with TS-1/SiO2 |
0–50 h |
Fixed-bed reactor |
Liquid phase propylene epoxidation |
Feng et al.71 |
Nanocrystalline zeolite beta |
0–6 h |
Fixed-bed micro-reactor |
Catalytic cracking of used palm oil |
Taufiqurrahmi et al.72 |
Pd/carbon |
0–400 |
Fixed bed reactor |
Crude terephthalic acid hydro-purification |
Li et al.73 |
Ni–Al oxide |
0–165 h |
Fixed bed reactors |
CO2 methanation |
Ewald et al.65 |
brewer's spent yeast (BSY) |
120 min |
Batch reactor |
Biodiesel production from FFA |
Arumugamurthy et al.66 |
Ni based catalyst |
0–900 day |
Membrane reformer |
Methane reforming |
Jokar et al.74 |
Ni/Al2O3 |
0–100 h |
Fixed bed reactors |
CO2 methanation |
Morosanu et al.75 |
HY-zeolites |
5 min |
Packed bed reactor |
Dehydration of glycerol to acrolein |
Pala-Rosas et al.76 |
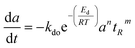 |
nta |
SBA-15 |
500 min |
Fixed bed reactor |
Methane decomposition |
Chen and Lua50 |
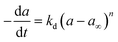 |
nta |
Pt/Al2O3 |
0–350 h |
nta |
nta |
Bartholomew51 |
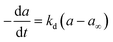 |
a = a∞ + (1 − a∞)e−kdt |
Cobalt based catalyst |
0–1000 h |
Bubble-column reactor |
Fischer–Tropsch |
Argyle et al.52 |
Chiral |
0–242.2 h |
Batch reactor |
Hydrogenation of acetophenone |
Ruelas-Leyva & Fuentes53 |
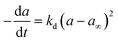 |
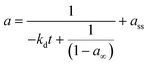 |
Fe/Al2O3 |
0–1300 h |
Slurry bubble column reactor |
Fischer–Tropsch |
Ghofran et al.54 |
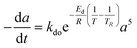 |
nta |
CuO/ZnO/Al2O3 |
nta |
nta |
nta |
Honken56 |
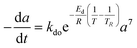 |
nta |
Pt/Re/Al2O3 |
0–800 day |
Spherical packed-bed reactor |
Naphtha reforming |
Rahimpour et al.64 |
nta |
Pt/Re/Al2O3 |
0–800 day |
Spherical packed-bed reactor |
Naphtha reforming |
Iranshahi et al.68 |
2.3 Coke formation dependent catalyst deactivation model
Coke deposition has been proven to be the main reason for catalyst deactivation in catalytic industrial processes, owing to the blocking of the active pore sites of the catalyst. The coke formation rate in the catalyst depends on the feed composition, reaction conditions (516–568 °C) and time-on-stream of propane dehydrogenation at atmospheric pressure using Pt/Al and Pt–Sn/Al oxide catalysts.77 Coking at low temperatures was found to result in higher coke accumulation during catalytic cracking over β-zeolite catalyst at reaction temperature 400–500 °C, used for biofuel than that at higher temperatures, and the lower temperature conversion was attributed to the higher rate of condensation of coke components.72 Catalyst deactivation by coke formation, occurs through a reversible or an irreversible mechanism. The presence of hydrogen in the naphtha reformer unit operated at 507–548 °C and pressure 1.5 bar, contributes to the removal of reversible coke and anticipated to decrease the catalyst deactivation rate.78
A modern catalytic cracking model uses a negative exponential function to represent the fluidized catalytic cracking of zeolite catalyst and coke deactivation.79–82 The coke content has an inverse effect on the active pore sites inside the catalyst particles. Therefore, several researchers have studied the relationship between catalyst activity and coke content over Ni–Ce/Al oxide as dry reforming catalysts, as shown in eqn (14).83–85 Froment et al.26 described dependence of the catalyst activity on the coke formation during 1-butene dehydrogenation at 560–600 °C as shown in eqn (15). Additionally, Dumez and Froment15 proposed five forms of deactivation function depends on carbon content over 20% Cr/Al oxide catalyst for the dehydrogenation of 1-butene to butadiene at 490–600 °C and pressure 0.2–2.7 bar, these deactivation forms are shown in eqn (17)–(21).
|
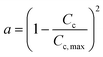 | (14) |
|
 | (15) |
Zavarukhin and Kuvshinov86 proposed a mathematical model to describe the kinetics and catalyst deactivation of the formation of nanofibrous carbon from a mixture of methane and hydrogen over a highly loaded nickel catalyst (90 wt% Ni–Al2O3) at conversion temperature 490–590 °C and atmospheric pressure. They approximated catalytic activity for coke formation using a parabolic formula as described in eqn (22). A simulation on the model proposed by Nayak et al.87 was done to evaluate the performance of a fluidized catalytic cracking riser reactor using four to ten lump models. The catalyst activity was related to the coke deposition on catalyst using eqn (23). Gao et al.88 proposed an eight-lump kinetic model to estimate the process performance of vacuum residue (VR) catalytic cracking at temperature 460–520 °C and WHSV of 15 h−1. A deactivation expression dependent on the catalyst coke content (Cc) was adopted as shown in eqn (24). Xiong et al.89 developed a novel six-lump kinetic model including catalyst deactivation to determine the cracking performance of a fluidized catalytic cracking process at temperature 460–540 °C, catalyst/oil ration 4–10, and WHSV of 5–30 h−1. The catalytic activity was modeled based on the coking content using eqn (24).
|
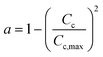 | (22) |
|
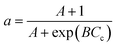 | (23) |
Barghi and Karimzadeh90 proposed a kinetic model for the catalytic cracking at 565–635 °C in presence and absence of steam at atmospheric pressure of liquefied petroleum gas (LPG) conversion to olefin and aromatics using ZnO incorporated into a ZSM-5 catalyst. The deactivation model was extended to include coke formation, metal sintering, delamination of the zeolite framework and steam effect, and the overall catalyst activity as shown in eqn (25). Ivanchina et al.91 developed a mathematical model for the fluidized catalytic cracking process at 495–542 °C, the pressure is 0.08–0.2 MPa, considering the feedstock composition and catalyst deactivation by coke. The catalytic activity was attributed to primary (aY) and secondary (aZSM-5) reactions during catalytic cracking as described by the exponential dependence in eqn (26) and (27).
|
a = 1 − dcoke − dsintering − ddealumination
| (25) |
|
aY = Ao exp(−α1CC)
| (26) |
|
aZSM-5 = Ao exp(−α2CC)
| (27) |
The catalyst deactivation model dependent on coke formation cannot predict catalyst activity decay with a high level of accuracy.92 Table 3 summarizes the most important models attributed to catalyst deactivation including CHZ-4 (ref. 93) and Ni/ZrO2 (ref. 94) used for biofuels which a dependent on coke formation.
Table 3 Catalyst deactivation dependent on coke formation of fuel and dehydrogenation catalyst systems
Deactivation expression |
Catalyst type |
TOS |
Reactor type |
reaction |
Reference |
Not reported. |
a = exp(−αCC) |
Cr2O3–Al2O3 |
nta |
Fixed bed reactor |
i-Butene dehydrogenation |
Dumez and Froment15 |
a = 1 − αCC |
a = (1 − αCC)2 |
a = 1/(1 + αCC) |
a = 1/(1 + αCC)2 |
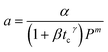 |
FCC catalyst |
1.25–5 min |
Fluidized dense-bed reactor |
Hydrocarbons cracking |
Jacob et al.95 |
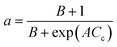 |
nta |
20–40 h |
Plug flow model |
Vacuum gasoil cracking |
Martin et al.96 |
 |
Zeolite |
0–10 s |
Fluidized regenerator and riser cracker |
FCC |
Arandes and Lasa60 |
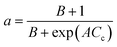 |
FCC catalyst |
nta |
FCC riser reactors |
Vacuum gas oil cracking |
Nayak et al.87 |
a = exp(−αCc) |
Pt–Sn/–Al2O3 |
1–10 day |
Tubular quartz reactor |
Propane dehydrogenation |
Niknaddaf et al.97 |
a = (1 +βCc)−m |
nta |
1–40 s |
Fluidized bed reactor |
Catalytic cracking of vacuum residue |
Gao et al.88 |
a = (1 + αCc)−β |
FCC catalyst |
nta |
Fluidized bed |
Catalytic cracking of heavy oil |
Xiong et al.89 |
a = Ao exp(−A1Cc) |
Y and ZSM-5 |
nta |
Riser reactor |
Vacuum gasoil cracking |
Ivanchina et al.91 |
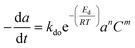 |
CHZ-4 |
50 min |
Fixed bed reactor |
Biomass fast pyrolysis oil |
Wan et al.93 |
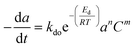 |
Cr2O3/Al2O3 catalyst |
nta |
Moving bed reactor |
Propane dehydrogenation |
Ghodasara, et al.98 |
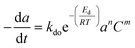 |
Pd–Ag supported α-Al2O3 |
800 day |
Packed bed reactor |
Acetylene hydrogenation |
Dehghani et al.99 |
a = Ao exp(−A1Cc) |
nta |
4.5 s |
Plug flow reactor |
Catalytic cracking of vacuum distillate and residual feedstock |
Nazarova et al.100 |
a = exp(−Kdt) |
Ni/ZrO2 |
60 min |
Tubular packed-bed reactor |
Deoxygenation of palm oil to produce green diesel |
Hafeez et al.94 |
 |
a = Ao exp(−A1Cc) |
nta |
4.5 s |
Riser reactor |
Vacuum gasoil cracking |
Nazarova et al.17 |
2.4 Reactant dependent catalyst deactivation model
The selective catalyst deactivation was model attributed to an individual effects of the reaction mixture composition, temperature and time (Fig. 2). Gayubo et al.101 proposed a catalyst deactivation expression for methanol-to-olefin (MTO) conversion over ZSM-5 zeolite from coke; the process considered ethylene concentration as a precursor in the temperature range 400–500 °C. Aguayo et al.102 applied a deactivation model that considered the effect of lump concentration on the coke formation rate at 360–420 °C and contact time between 0.01–0.15 g h−1, over the ZSM-5 zeolite catalyst used for methanol-to-gasoline (MTG) conversion as shown in eqn (28). They demonstrated that the selective deactivation model provided a better representation of the practical results than the empirical model, which did not account for the composition effect upon deactivation. |
 | (28) |
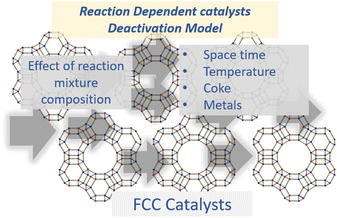 |
| Fig. 2 Selective catalyst deactivation model. | |
Commercial Fischer–Tropsch processes operate at relatively moderate temperatures and pressures. The carbidization, sintering and change of oxidation state cobalt catalysts are the most significant deactivation associated with cobalt-based catalysts.52 Although Pour et al.30 studied the deactivation kinetics, bulk and nano-structured catalysts prepared by the micro-emulsion method of an iron-based catalyst were applied for Fischer–Tropsch synthesis (FTS). The generalized power-law expression (GPLE), rd = kd(a − a∞)m was applied to fit the experimental data of catalyst deactivation rate tested at pressure 17 bar conversion temperature 270–310 °C. Pour et al.103 proposed a different deactivation mechanism to represent the deactivation of a Co-based catalyst supported on carbon nanotubes (CNTs) applied in the Fischer–Tropsch process at pressure 20 bar and temperature 220 °C. They observed that water was the most important deactivating component in Co-based catalysts; therefore, they considered the partial pressure of water in the deactivation kinetic equation. The rate of catalyst deactivation was dependent on the catalyst activity decreasing rate (a − a∞) as shown in eqn (29).
|
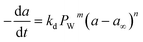 | (29) |
Centeno et al.104 considered both carbon and metal deposition in a deactivation model to fit the experimental result of different gas oil feedstocks for the hydrotreating process over NiMo/Al oxide in a bench-scale reactor. The experiments were conducted at 380, 400 and 420 °C while sulfur, metal and asphaltene content were analyzed every 10 h during 240 h of time-on-stream (TOS). They observed a good agreement between the experimental and practical deactivation results, whereas the asphaltene content had a significant impact on catalyst deactivation. Table 4 summarizes the most important models for integrating complex catalyst deactivation dependent on coke formation. Similarly, the model has also been applied to ZSM-5 zeolite from coke used for steam catalytic reforming at 550–700 °C and steam/carbon ratio of 1.5–6.0,105 coke over Ni/Al oxide for catalytic biomass pyrolysis at 600–700 °C and steam/biomass ratio 1.6–12.5, for biofuel production,106 and CO2/CO hydrogenation using a Cu–Zn–Zr/SAPO-11 catalyst for the Fischer–Tropsch process at temperature 250–325 °C and 10–50 bar.107
Table 4 Catalyst deactivation dependent on coke formation
Differential form |
Catalyst |
TOS |
Reactor type |
Reaction system |
Reference |
 |
HZSM-5 zeolite |
10 h |
Fixed and fluidized bed reactor |
Methanol to gasoline |
Aguayo et al.102 |
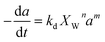 |
HZSM-5 |
2 h |
Fixed-bed reactor |
Methanol to olefin |
Gayubo et al.101 |
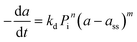 |
Fe/Cu/La |
105 h |
Semi-batch reactor |
Fischer–Tropsch |
Pour et al.30 |
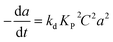 |
AuAl2O3 |
0–440 h |
Plug-flow reactor |
Pentene isomerization |
Solkina et al.108 |
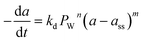 |
Co/CNTs |
430 h |
Fixed-bed reactor |
Fischer–Tropsch |
Pour et al.103 |
,  |
HZSM-5 |
18 h |
Isothermal fixed bed reactor |
Dimethyl ether (DME) to olefins |
Pérez-Uriarte et al.109 |
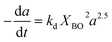 |
HZSM-5 |
5 h |
Fluidized bed reactor |
Steam reforming of bio-oil |
Gayubo et al.105 |
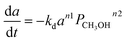 |
Fe–Mo |
353 days |
Packed bed reactor |
Formaldehyde production |
Braz et al.110 |
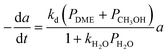 |
CuO/ZnO/Al2O3 |
50 h |
Fixed-bed reactor |
Synthesis of dimethyl ether from syngas |
Peláez et al.111 |
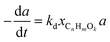 |
Commercial Ni/Al2O3 |
2 h |
Fluidized bed reactor |
Biomass pyrolysis |
Arregi et al.106 |
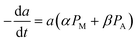 |
HZSM-5 |
9–25 h |
Fixed-bed reactor |
Methanol to aromatic |
Li et al.112 |
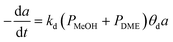 |
CuO–ZnO–ZrO2/SAPO-11 |
48 h |
Fixed-bed reactor |
CO2 + CO hydrogenation to dimethyl ether (DME) |
Ateka et al.107 |
 |
HZSM-5 |
40 h |
Fixed-bed reactor |
Methanol to olefin |
Li et al.113 |
2.5 Deactivation model with residual activity
Depending on the mechanism, catalyst deactivation can be reversible or irreversible under various condition.114 Catalyst deactivation by coke is a reversible process while catalyst poisoning by heavy metals (Ni and V) is irreversible.11 Garetto et al.115 studied the deactivation-regeneration mechanism of a Pt/Al oxide catalyst during hydrodechlorination at temperature 100–130 °C and pressure 2 and 10 bar of carbon tetrachloride. The catalyst deactivation–regeneration model considers the influence of the process operating conditions on catalyst deactivation, as shown in eqn (30), whereas the individual rate of deactivation-regeneration was evaluated using eqn (31) and (32): |
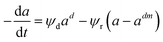 | (30) |
Zambrano et al.85 considered five expressions as shown in eqn (33)–(37) to represent the Ni–Ce/Al oxide catalyst deactivation for methane dry reforming with CO2 at temperature 475–550 °C and space time between 0.5–2 g h mol−1. The best fit was obtained for the residual activity because of the competition between coke formation and coke removal as described in eqn (35).
|
 | (33) |
|
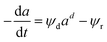 | (34) |
|
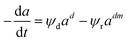 | (35) |
|
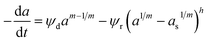 | (36) |
|
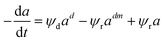 | (37) |
Rimaz et al.116 successfully fitted an experimental data on propane conversion over a Pt–Ge/Al oxide catalyst for propane dehydrogenation vs. 24 h time-on-stream at 500–600 °C using a deactivation model with residual activity (DMRA) (eqn (38)–(41)).
|
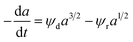 | (38) |
|
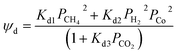 | (39) |
|
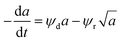 | (41) |
The simplest DMRA model, was used by Cazana et al.117 to express the catalyst activity behavior during the preparation of graphene-related material grown on stainless steel foams at 800–950 °C with methane feed between 3.6–42.9 mol%, via the catalytic decomposition of methane, as shown in eqn (42):
|
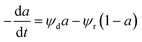 | (42) |
Gromotka et al.12 integrated three-factor kinetic equations for catalyst deactivation in terms of the apparent kinetic parameters. Three factors were applied: (1) the main catalytic cycle; (2) one-step reversible deactivation; and (3) one-directional irreversible deactivation from organic species accumulation. The obtained equation successfully applied to describe the reversible catalyst deactivation between 150–250 °C during the dehydration of acetaldehyde over a TiO2 catalyst. Table 5 illustrates the catalyst-deactivation dependent model for a catalyst with residual activity.
Table 5 Catalyst deactivation dependent with residual activity
Deactivation model |
Catalyst |
TOS |
Reactor type |
Reaction system |
Reference |
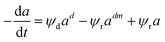 |
Commercial silica–alumina |
0–90 min |
Fixed bed reactor |
Isopropyl-benzene cracking |
Rodríguez et al.118 |
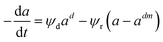 |
Pt/Al2O3 |
0–260 min |
Fixed-bed tubular reactor |
Hydride-chlorination of carbon tetrachloride |
Garetto et al.115 |
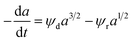 |
Ni–Ce/Al2O3 |
4 h |
Fixed-bed quartz reactor |
Dry reforming of methane |
Zambrano et al.85 |
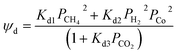 |
ψr = Kr1PCO22 |
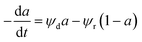 |
Fe nanoparticles |
2 h |
Fixed bed reactor |
Graphene formation by catalytic decomposition of methane |
Cazana et al.117 |
Overall, temperature is the most effective variable for the reaction rate, and can be considered the strongest variable affecting the rate of catalysts deactivation or regeneration. Occasionally, as the temperature of an industrial reactor increases, the reaction rate compensates for the decline in catalytic activity caused by the accumulation of coke and metals.119 Correspondingly, the GPLE is an appropriate deactivation model for cases in which the catalyst activity approaches an asymptotic value over long times such as Fischer–Tropsch catalysts at temperature 200–240 °C and pressure 100 bar.120 The selective deactivation model did not significantly improve the fit of the experimental data.121 A non-selective deactivation model derived from a simple deactivation kinetic equation for optimum estimation of reaction rates was used to integrate the lumped components in propane aromatization in two zone bed reactors.119
3. Mathematical modeling of chemical reactors
Regularly, the mathematical modeling could be classified as deterministic or empirical.122 The deterministic model is designed from the first-principles equation, while the empirical model is a mathematical expression generalized to fit with chemical reaction data of one or more variables.
3.1 Deterministic models
The deterministic model involves solving a system of ordinary differential equations for chemical reactions to estimate process performance. Accurate kinetic and catalyst deactivation models are crucial for the modeling, optimization, and part of chemical reactors control. The rigorous dynamic mathematical model of a reactor consists of solving nonlinear simultaneous equations (algebraic, differential and sometimes partial) in multiple dependent variables (product concentrations, coke content, temperature and pressure). The reactants and product distribution in reaction mixture can be estimated by solving the kinetic model equations. The dynamic coke content can be updated by integrating the corresponding differential equation describing the coke formation in time domain, the catalyst activity is easily related to time, product distribution and coke content using either algebraic or differential form of the catalyst deactivation model. The heat balance equation designed to estimate the temperature of the reaction mixture with respect to time, while Arrhenius equation is usually used to estimate the change of reaction rate and deactivation rate constants as a function of temperature. In the case of the heterogeneous fixed bed reactor model, the differential format of Ergun's equation can be integrated to calculate the pressure drop within the reactor bed. For gaseous reactions the reaction rate can be related to the partial pressures of reactants using at least one of the approved reaction mechanisms (Power law, Eley–Rideal, and Langmuir–Hinshelwood). This set of highly coupled nonlinear differential equations describes the reactants and product distribution, coke content, catalyst deactivation temperature and pressure are integrated through the reactor system. The detailed system of ordinary differential equations (ODEs) intents to capture the dynamic changes over time. Therefore, the ordinary differential equation (ODE) governing the rate of change in catalyst activity is projected to be solved simultaneously the change in the reaction conditions under different pressures and temperatures along with the compositions of the reaction mixture. Accordingly, the non-linear interference is bound by the catalyst activity, operating conditions and composition of the reaction mixture.20,123
These differential equations usually describe the fourth-order Runge–Kutta integration method. The kinetic and deactivation parameters were obtained by benchmarking the experimental and predicted results using a stochastic optimization method. Similarly, the optimization methods applied an artificial neural network (ANN), Genetic Algorithm (GA) and Particle Swarm Optimization (PSO).124 These optimization methods were designed iteratively to estimate the best kinetic and deactivation parameters which were expected to yield the minimum error between the experimental and predicted results. The widely used error evaluation expressions are presented as listed in Table 6.
Table 6 The error evaluation expressions125
Error function |
Expression |
Correlation coefficient of determination (R2) |
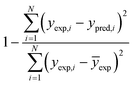 |
Mean absolute error (MAE) |
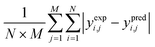 |
Mean relative error (MRE) |
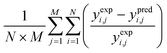 |
Mean square error (MSE) |
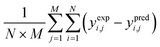 |
Where; yi,j is the mole fraction of ith component in jth experiments, M represents the number of experiments, N is the number of components, exp. and pred. are the superscripts denoting the experimental and predicted results, respectively.
Genetic algorithms (GA) are powerful stochastic optimization method which is designed for the natural selection and natural evaluation concepts.126 A GA applies to a population of individuals presents a selected solution to the optimization complication. Therefore, the individuals are comprised of strings or chromosomes of genes, the genes, are practical allele. GAs applies the principles of survival of the fittest, selection, reproduction, crossover, and mutation to these individuals to obtain a new better individual for each new solution.127,128 Genetic Algorithm creates a new chromosomes population by choosing better-fit results obtained from the existing population and applying genetic operators to produce new offspring from the result. The algorithm for Genetic Algorithm stochastic optimization method is depicted in Fig. 3.
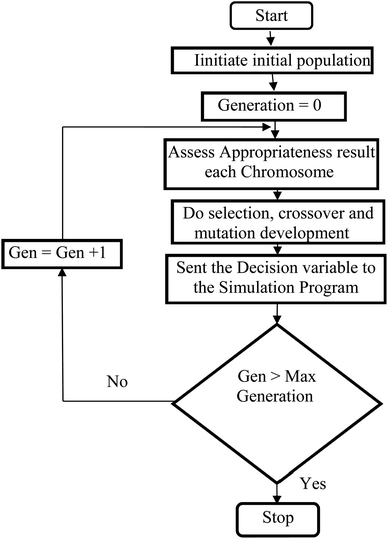 |
| Fig. 3 Genetic Algorithm (GA) computational procedure. | |
3.2 Artificial neural network (ANN) model
The kinetic parameters of catalyst deactivation (kdo and Eda) can be estimated empirically by fitting the response of the reaction mixture with respect to time and other effective variables. The activity at reaction start will be 100% initially and it decays with time. Therefore, the same catalyst has different deactivation kinetic parameters (kdo and Eda) for different reaction systems. For the same catalyst and the same initial activity, solving the deactivation kinetic models having different kinetic parameters will produce different deactivation responses. In case of the rigorous mathematical modeling of chemical reactors, the effect of time, reactant, poison, and products on the catalyst activity can be represented using an appropriate selective deactivation model. Recently, using artificial intelligence (AI) aims to capture the effect of any different input variables on the product distribution, catalyst activity and reaction temperatures. The artificial neural network (ANN) model designed to have the ability to establish relationships between multiple input–output variables. Artificial neural networks (ANNs) mimics the processes of the human brain and are series of mathematical algorithms that used in this study to estimate the relationships between datasets.129,130 ANNs models can intgerate empirical relationships between dependent and independent variables based on experimental data. The ANN contains several layers; an input layer, a hidden layer, and an output layer as shown in Fig. 4.
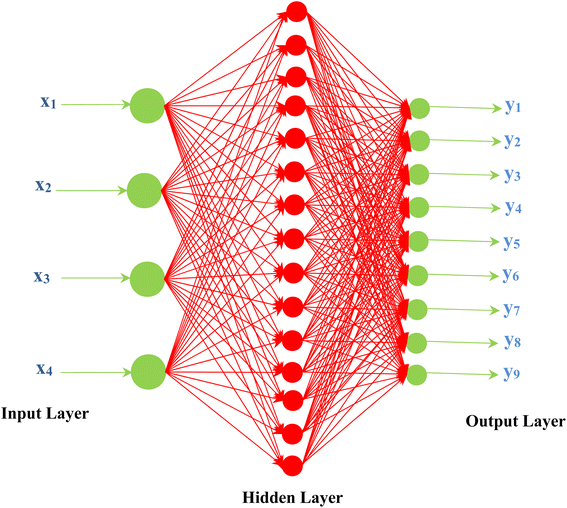 |
| Fig. 4 The neural network (ANN) with three layers. | |
ANNs are utilized to predict variables processed in a rapid manner and provide precise prediction under a complex process. ANNs are broadly applied for modeling and controlling multipart of chemical reactors and processes to accurately simulate atmospheric distillation columns,131,132 various of heat exchangers,133,134 and catalytic reactors.135,136 Approximation of the reaction rate obtained without a kinetic model would eliminate the errors obtained from choosing of the kinetic method related to the catalyst deactivation models for kinetic constant integration.137
4. Conclusion
Catalyst deactivation is a complex phenomenon, and an appropriate deactivation model is essential for process design and control to maximize catalyst utilization and maintain the catalytic production. Several mathematical models have been highlighted in the literature to describe catalyst deactivation in different reaction systems and operating conditions. Deactivation models play a significant role in the simulation, optimization and design of chemical catalytic reactors. This study considered scientific narratives to comprehend different catalyst deactivation models. From this review the following conclusions were drawn:
• The time dependent deactivation model is appropriate for systems with fast catalyst deactivation such as fluidized catalytic cracking, because the catalyst activity depends more on the effects of the deactivation rate over time than on temperature and reactant concentrations.
• For the dynamic process, the differential form of catalyst deactivation is slightly more accurate than the algebraic form; hence it represents the instantaneous catalyst activity in a dynamic system.
• The generalized power-law equation (GPLE) is a powerful equation for a long-term deactivation process.
• Selective deactivation kinetic models are limited because they are often complex and difficult to integrate.
Abbreviations
A, B, r, α, β | Deactivation constants |
Aa | Pre-exponential factor (mol−1 L h−1) |
Ad | Deactivation of pre-exponential factor (mol−1 L h−1) |
a | Activity of catalyst (−) |
a∞ | Catalyst activity infinite reaction time |
Cc | Coke content on the catalyst (wt%.) |
Ci | Concentration of the ith component (mol l−1) |
Ea | Activation energy of the reaction (kJ mol−1) |
Ed | Deactivation energy (kJ mol−1) |
kd | Deactivation rate constant [h−1] |
kr | Regeneration rate constant [h−1] |
kd0 | Pre-exponential factors, [g cm−3]−1 h−1 |
m, n | Activity term power |
P | Reaction pressure (Pa) |
Pi | Partial pressure of species I (Pa) |
R | Gas constant 8.31451 (J mol−1 K−1) |
T | Reaction temperature (K) |
To | Reference temperature (K) |
TOS | Time on stream (h) |
t | Time (h) |
tR | Residence time (h) |
Xi | Weight fraction of lump I (wt%.) |
α1 and α2 | Catalyst deactivation constants |
θ | Term that quantifies the attenuation of the reaction rates by the adsorption |
ψd | Deactivation kinetic function |
ψr | Regeneration kinetic function |
Subscripts
A, C and D | Indicate to oxygenates (methanol and dimethylether), light olefins (ethylene and propylene) and lump of the rest of hydrocarbons respectively |
BO | Bio-oil |
DME | De-methyl ether |
max | Maximum |
MeOH | Methanol |
W | Water |
∞ | Infinity |
Conflicts of interest
The authors declare no competing financial interest.
Acknowledgements
The author would like to acknowledge the scientific support from the Department of Chemical Engineering, University of Technology, Baghdad, Iraq.
References
- A. S. Krishna, Catal. Rev.: Sci. Eng., 1990, 32(4), 279–381 CrossRef CAS.
- G. C. Hadjiloizou, J. B. Butt and J. S. Dranoff, J. Catal., 1992, 135(1), 27–44 CrossRef CAS.
- S. Ordóñez, P. Hurtado, H. Sastre and F. V. Diez, Appl. Catal., A, 2004, 259(1), 41–48 CrossRef.
- L. W. Jossens and E. E. Petersen, J. Catal., 1982, 76(2), 265–273 CrossRef CAS.
- S. Hamoudi, K. Belkacemi and F. Larachi, Chem. Eng. Sci., 1999, 54(15–16), 3569–3576 CrossRef CAS.
- A. Monzón, E. Romeo and A. Borgna, Chem. Eng. J., 2003, 94(1), 19–28 CrossRef.
- Z. Ke, Z. Ya-ping, H. Tian-jiao, L. Bin and S. Kai, J. Fuel Chem. Technol., 2017, 45(11), 1356–1364 CrossRef.
- J. Schirme, J. S. Kim and E. Klemm, J. Anal. Appl. Pyrolysis, 2001, 60(2), 205–217 CrossRef.
- J. Ogonowski and E. Skrzyńska, Catal. Lett., 2008, 121(3–4), 234–240 CrossRef CAS.
- A. Morris and C. Bartholomew, Catalysts, 2015, 5(1), 145–269 CrossRef.
- C. Bartholomew, Appl. Catal., A, 2001, 212, 17–60 CrossRef CAS.
- Z. Gromotka, G. Yablonsky, N. Ostrovskii and D. Constales, Entropy, 2021, 23(7), 818 CrossRef CAS PubMed.
- A. Voorhies Jr, Ind. Eng. Chem., 1945, 37(4), 318–322 CrossRef.
- V. W. Weekman Jr, Ind. Eng. Chem. Process Des. Dev., 1968, 7(1), 90–95 CrossRef.
- F. J. Dumez and G. F. Froment, Ind. Eng. Chem. Process Des. Dev., 1976, 15(2), 291–301 CrossRef CAS.
- K. R. Devoldere and G. F. Froment, Ind. Eng. Chem. Res., 1999, 38(7), 2626–2633 CrossRef CAS.
- G. Nazarova, E. Ivashkina, E. Ivanchina, A. Oreshina, I. Dolganova and M. Pasyukova, Fuel Process. Technol., 2020, 200, 106318 CrossRef CAS.
- A. A. Ebrahimi, H. Mousavi, H. Bayesteh and J. Towfighi, Fuel, 2018, 231, 118–125 CrossRef.
- T. Cordero-Lanzac, A. T. Aguayo, A. G. Gayubo, P. Castaño and J. Bilbao, Chem. Eng. J., 2020, 379, 122260 CrossRef CAS.
- Z. M. Shakor, A. A. AbdulRazak and K. A. Sukkar, Arabian J. Sci. Eng., 2020, 45(9), 7361–7370 CrossRef CAS.
- C. H. Bartholomew, Catalyst Deactivation in Hydrotreating of Residua: A Review, Marcel Dekker, New York, 1994 Search PubMed.
- H. O. Otor, J. B. Steiner, C. Garcia-Sancho and A. C. Alba-Rubio, ACS Catal., 2020, 10(14), 7630–7656 CrossRef CAS.
- A. Ochoa, J. Bilbao, A. G. Gayubo and P. Castaño, Renewable Sustainable Energy Rev., 2020, 119, 109600 CrossRef CAS.
- J. Zhou, J. Zhao, J. Zhang, T. Zhang, M. Ye and Z. Liu, Chin. J. Catal., 2020, 41(7), 1048–1061 CrossRef CAS.
- A. Szymaszek, B. Samojeden and M. Motak, Energies, 2020, 13(15), 3870 CrossRef CAS.
- G. F. Froment, Modeling of catalyst deactivation, Appl. Catal., A, 2001, 212(1–2), 117–128 CrossRef CAS.
- P. U. Okoye, A. Z. Abdullah and B. H. Hameed, Renewable Sustainable Energy Rev., 2017, 74, 387–401 CrossRef CAS.
- I. Elizalde and J. Ancheyta, Fuel Process. Technol., 2014, 123, 114–121 CrossRef CAS.
- T. M. Moustafa and G. F. Froment, Ind. Eng. Chem. Res., 2003, 42(1), 14–25 CrossRef CAS.
- A. N. Pour, M. R. Housaindokht, S. F. Tayyari, J. Zarkesh and M. R. Alaei, J. Mol. Catal. A: Chem., 2010, 330(1–2), 112–120 CrossRef.
- V. N. Parmon and N. M. Ostrovskii, React. Kinet., Mech. Catal., 2020, 131(1), 37–55 CrossRef CAS.
- M. L. Occelli, Fluid Catalytic Cracking 2, American Chemical Society, Washington, DC, United States, 1991 Search PubMed.
- Y. Ozawa, Y. Tochihara, M. Nagai and S. Omi, Chem. Eng. Sci., 2003, 58(3–6), 671–677 CrossRef CAS.
- J. Markos, A. Brunovska and J. Ilavsky, Chem. Pap., 1987, 41, 375 CAS.
- K. N. Theologos and N. C. Markatos, AIChE J., 1993, 39(6), 1007–1017 CrossRef CAS.
- D. R. Rainer, E. Rautiainen and P. Imhof, Appl. Catal., A, 2003, 249(1), 69–80 CrossRef CAS.
- O. A. Olafadehan, O. P. Sunmola, A. Jaiyeola, V. Efeovbokhan and O. G. Abatan, Appl. Petrochem. Res2E, 2018, 8, 219–237 CrossRef CAS.
- R. Zhang, L. Li, Z. Liu and X. Meng, Int. J. Chem. Eng., 2016, 2016, 9148925 Search PubMed.
- T. P. Jadon, A. K. Jana and P. Parikh, Biomass Convers. Biorefin., 2021, 11, 1093–1099 CrossRef CAS.
- G. Trunfio and F. Arena, Catalysts, 2014, 4(2), 196–214 CrossRef.
- P. Hagelberg, I. Eilos, J. Hiltunen, K. Lipiäinen, V. M. Niemi, J. Aittamaa and A. O. Krause, Appl. Catal., A, 2002, 223(1–2), 73–84 CrossRef CAS.
- A. Ricca, F. Montella, G. Iaquaniello, E. Palo, A. Salladini and V. Palma, Catal. Today, 2019, 331, 43–52 CrossRef CAS.
- D. V. Naik, V. Karthik, V. Kumar, B. Prasad and M. O. Garg, Chem. Eng. Sci., 2017, 170, 790–798 CrossRef CAS.
- F. T. Zangeneh, A. Taeb, K. Gholivand and S. Sahebdelfar, J. Energy Chem., 2013, 22(5), 726–732 CrossRef CAS.
- D. Sun, F. M. Khan and D. S. Simakov, Chem. Eng. J., 2017, 329, 165–177 CrossRef CAS.
- A. G. Sani, H. A. Ebrahim and M. J. Azarhoosh, Fuel, 2018, 225, 322–335 CrossRef CAS.
- Y. Lin, Y. Wang, W. Feng, G. Wu, J. Xu, T. Zhang, S. Wang, X. Wu and P. Yao, React. Kinet., Mech. Catal., 2014, 112, 267–282 CrossRef CAS.
- R. B. Demuner, J. G. Soares Santos Maia, A. R. Secchi, P. A. Melo, R. W. do Carmo and G. S. Gusmão, Ind. Eng. Chem. Res., 2019, 58(8), 2717–2726 CrossRef CAS.
- M. T. Ravanchi, S. Sahebdelfar, M. R. Fard, S. Fadaeerayeni and P. Bigdeli, Chem. Eng. Technol., 2016, 39(2), 301–310 CrossRef CAS.
- Q. Chen and A. C. Lua, Chem. Eng. J., 2020, 389, 124366 CrossRef CAS.
- C. H. Bartholomew, Appl. Catal., A, 1993, 107(1), 1–57 CrossRef CAS.
- M. D. Argyle, T. S. Frost and C. H. Bartholomew, Top. Catal., 2014, 57, 415–429 CrossRef CAS.
- J. Ruelas-Leyva and G. Fuentes, Catalysts, 2017, 7(7), 193 CrossRef.
- M. Ghofran Pakdel, H. Atashi, H. Zohdi-Fasaei and A. A. Mirzaei, Pet. Sci. Technol., 2019, 37(5), 500–505 CrossRef CAS.
- N. M. Ostrovskii, Chem. Eng. J., 2006, 120(1–2), 73–82 CrossRef CAS.
- L. Honken, Optimization of methanol reactor, Master Thesis, Department of Chemical Engineering, Norwegian University of Science and Technology, 1995 Search PubMed.
- H. Kordabadi and A. Jahanmiri, Chem. Eng. Process., 2007, 46(12), 1299–1309 CrossRef CAS.
- M. Farsi and A. Jahanmiri, J. Nat. Gas Chem., 2012, 21(4), 407–414 CrossRef CAS.
- M. Farsi and A. Jahanmiri, J. Ind. Eng. Chem., 2014, 20(5), 2927–2933 CrossRef CAS.
- J. M. Arandes and H. I. Lasa, Chem. Eng. Sci., 1992, 47(9–11), 2535–2540 CrossRef CAS.
- E. Rodríguez, G. Elordi, J. Valecillos, S. Izaddoust, J. Bilbao, J. M. Arandes and P. Castaño, Fuel Process. Technol., 2019, 192, 130–139 CrossRef.
- C. Seidel, A. Jörke, B. Vollbrecht and A. Seidel-Morgenstern, Comput.-Aided Chem. Eng., 2018, 43, 85–90 CAS.
- S. Masoudi, M. Farsi and M. R. Rahimpour, Oil Gas Sci. Technol., 2019, 74, 90 CrossRef CAS.
- M. R. Rahimpour, D. Iranshahi and A. M. Bahmanpour, Int. J. Hydrogen Energy, 2010, 35(14), 7498–7511 CrossRef CAS.
- S. Ewald, M. Kolbeck, T. Kratky, M. Wolf and O. Hinrichsen, Appl. Catal., A, 2019, 570, 376–386 CrossRef CAS.
- S. S. Arumugamurthy, P. Sivanandi, S. Pandian, H. Choksi and D. Subramanian, Waste Manage., 2019, 100, 318–326 CrossRef PubMed.
- J. Martínez and J. Ancheyta, Fuel, 2012, 100, 193–199 CrossRef.
- D. Iranshahi, E. Pourazadi, K. Paymooni, A. M. Bahmanpour and M. R. Rahimpour, Int. J. Hydrogen Energy, 2010, 35(23), 12784–12799 CrossRef CAS.
- M. R. Usman, D. L. Cresswell and A. A. Garforth, Int. Scholarly Res. Not., 2013, 2013, 152893 Search PubMed.
- H. Xu, J. Si and G. Luo, Int. J. Chem. React. Eng., 2017, 15(4), 20160165 CAS.
- W. Feng, Y. Wang, G. Wu, Y. Lin, J. Xu, H. Shi, T. Zhang, S. Wang, X. Wu and P. Yao, J. Chem. Technol. Biotechnol., 2015, 90(8), 1489–1496 CrossRef CAS.
- N. Taufiqurrahmi, A. R. Mohamed and S. Bhatia, Chem. Eng. J., 2010, 163(3), 413–421 CrossRef CAS.
- Z. Li, W. Zhong, X. Wang, N. Luo and F. Qian, Comput. Chem. Eng., 2016, 88, 1–2 CrossRef CAS.
- S. M. Jokar, P. Parvasi and A. Basile, Int. J. Hydrogen Energy, 2019, 44(47), 25730–25739 CrossRef CAS.
- E. A. Morosanu, F. Salomone, R. Pirone and S. Bensaid, Catalysts, 2020, 10(3), 283 CrossRef CAS.
- I. Pala-Rosas, J. L. Contreras, J. Salmones, B. Zeifert, R. López-Medina, J. Navarrete-Bolaños, S. Hernández-Ramírez, J. Pérez-Cabrera and A. A. Fragoso-Montes de Oca, Catalysts, 2021, 11(3), 360 CrossRef CAS.
- M. Larsson, M. Hultén, E. A. Blekkan and B. Andersson, J. Catal., 1996, 164(1), 44–53 CrossRef CAS.
- M. Larsson, N. Henriksson and B. Andersson, Stud. Surf. Sci. Catal., 1997, 111, 673–680 CrossRef CAS.
- M. T. Shah, R. P. Utikar, V. K. Pareek, G. M. Evans and J. B. Joshi, Chem. Eng. Res. Des., 2016, 111, 403–448 CrossRef CAS.
- G. F. Froment, Curr. Opin. Chem. Eng., 2014, 5, 1–6 CrossRef.
- F. López-Isunza, Stud. Surf. Sci. Catal., 2001, 134, 187–199 CrossRef.
- P. Varshney, D. Kunzru and S. K. Gupta, Indian Chem. Eng., 2015, 57(2), 115–135 CrossRef CAS.
- J. Corella and J. M. Asua, Ind. Eng. Chem. Process Des. Dev., 1982, 21(1), 55–61 CrossRef CAS.
- J. Corella, J. Adanez and A. Monzon, Ind. Eng. Chem. Res., 1988, 27(3), 375–381 CrossRef CAS.
- D. Zambrano, J. Soler, J. Herguido and M. Menéndez, Top. Catal., 2019, 62, 456–466 CrossRef CAS.
- G. F. Froment and K. B. Bischoff, Chem. Eng. Sci., 1961, 16(3–4), 189–201 CrossRef CAS.
- S. V. Nayak, S. L. Joshi and V. V. Ranade, Chem. Eng. Sci., 2005, 60, 6049–6066 CrossRef CAS.
- H. Gao, G. Wang, C. Xu and J. Gao, Energy Fuels, 2014, 28(10), 6554–6562 CrossRef CAS.
- K. Xiong, C. Lu, Z. Wang and X. Gao, Fuel, 2015, 161, 113–119 CrossRef CAS.
- B. Barghi and R. Karimzadeh, React. Kinet., Mech. Catal., 2017, 120, 753–773 CrossRef CAS.
- E. Ivanchina, E. Ivashkina and G. Nazarova, Chem. Eng. J., 2017, 329, 262–274 CrossRef CAS.
- A. Haghlesan, R. Alizadeh and E. Fatehifar, Pet. Sci. Technol., 2016, 34(6), 499–504 CrossRef CAS.
- L. Wan, S. P. Zhang, S. T. Zhao, Q. L. Xu and Y. J. Yan, Energy Sources, Part A, 2013, 35(9), 800–808 CrossRef CAS.
- S. Hafeez, S. M. Al-Salem, K. N. Papageridis, N. D. Charisiou, M. A. Goula, G. Manos and A. Constantinou, Catalysts, 2021, 11(6), 747 CrossRef CAS.
- S. M. Jacob, B. Gross, S. E. Voltz and V. W. Weekman Jr, AIChE J., 1976, 22(4), 701–713 CrossRef CAS.
- M. P. Martin, C. Derouin, P. Turlier, M. Forissier, G. Wild and J. R. Bernard, Chem. Eng. Sci., 1992, 47(9–11), 2319–2324 CrossRef CAS.
- S. Niknaddaf, M. Soltani, A. Farjoo and F. Khorasheh, Pet. Sci. Technol., 2013, 31(23), 2451–2462 CrossRef CAS.
- K. Ghodasara, S. Hwang and R. Smith, Korean J. Chem. Eng., 2015, 32, 2169–2180 CrossRef CAS.
- O. Dehghani, M. Rahimpour and A. Shariati, Processes, 2019, 7(3), 136 CrossRef CAS.
- G. Y. Nazarova, E. N. Ivashkina, E. D. Ivanchina, A. V. Vosmerikov, L. N. Vosmerikova and A. V. Antonov, Catalysts, 2021, 11(6), 701 CrossRef CAS.
- A. G. Gayubo, A. T. Aguayo, M. Olazar, R. Vivanco and J. Bilbao, Chem. Eng. Sci., 2003, 58(23–24), 5239–5249 CrossRef CAS.
- A. T. Aguayo, A. G. Gayubo, J. Ortega, M. Olazar and J. Bilbao, Catal. Today, 1997, 37(3), 239–248 CrossRef CAS.
- A. A Nakhaei Pour, E. Hosaini and M. Feyzi, J. Iran. Chem. Soc., 2016, 13, 139–147 CrossRef.
- G. Centeno, J. Ancheyta, A. Alvarez, G. Marroquín, F. Alonso and A. Castillo, Fuel, 2012, 100, 73–79 CrossRef CAS.
- A. G. Gayubo, B. Valle, B. Aramburu, C. Montero and J. Bilbao, Chem. Eng. J., 2018, 332, 192–204 CrossRef CAS.
- A. Arregi, G. Lopez, M. Amutio, I. Barbarias, L. Santamaria, J. Bilbao and M. Olazar, Int. J. Hydrogen Energy, 2018, 43(27), 12023–12033 CrossRef CAS.
- A. Ateka, M. Sanchez-Contador, A. Portillo, J. Bilbao and A. T. Aguayo, Fuel Process. Technol., 2020, 206, 106434 CrossRef CAS.
- Y. S. Solkina, S. I. Reshetnikov, M. Estrada, A. Simakov, D. Y. Murzin and I. L. Simakova, Chem. Eng. J., 2011, 176, 42–48 CrossRef.
- P. Pérez-Uriarte, A. Ateka, A. G. Gayubo, T. Cordero-Lanzac, A. T. Aguayo and J. Bilbao, Chem. Eng. J., 2017, 311, 367–377 CrossRef.
- C. G. Braz, A. Mendes, J. Rocha, R. Alvim and H. Matos, Chem. Eng. Sci., 2019, 195, 347–355 CrossRef CAS.
- R. Peláez, E. Bryce, P. Marín and S. Ordóñez, Fuel Process. Technol., 2018, 179, 378–386 CrossRef.
- H. Li, X. G. Li and W. D. Xiao, RSC Adv., 2019, 9(39), 22327–22335 RSC.
- X. G. Li, X. Huang, Y. L. Zhang, H. Li, W. D. Xiao and Z. Wei, Chem. Eng. Sci., 2020, 226, 115859 CrossRef CAS.
- M. Guisnet, and F. R. Ribeiro, Deactivation and regeneration of zeolite catalysts, Catalytic Science Series, Imperial College Press, London, 2011, vol. 9 Search PubMed.
- T. F. Garetto, C. I. Vignatti, A. Borgna and A. Monzón, Appl. Catal., B, 2009, 87(3–4), 211–219 CrossRef CAS.
- S. Rimaz, L. Chen, A. Monz, S. Kawi and A. Borgna, Chem. Eng. J., 2021, 405, 126656 CrossRef CAS.
- F. Caznana, N. Latorre, P. Tarifa, C. J. Royo, V. Sebastián, E. Romeo, M. A. Centeno and A. Monzón, Catal. Today, 2022, 383, 236–246 CrossRef.
- J. C. Rodríguez, J. A. Peña, A. Monzón, R. Hughes and K. Li, Chem. Eng. J. Biochem. Eng. J., 1995, 58(1), 7–13 CrossRef.
- M. P. Gimeno, J. Soler, J. Herguido and M. Menéndez, Ind. Eng. Chem. Res., 2010, 49(3), 996–1000 CrossRef CAS.
- S. A. Eliason and C. H. Bartholomew, Appl. Catal., A, 1999, 186(1–2), 229–243 CrossRef CAS.
- J. Corella, Ind. Eng. Chem. Res., 2004, 43(15), 4080–4086 CrossRef CAS.
- A. Al-Shathr, Z. M. Shakor, H. S. Majdi, A. A. AbdulRazak and T. M. Albayati, Catalysts, 2021, 11(9), 1034 CrossRef CAS.
- A. Al-Shathr, Z. M. Shakor, B. Y. Al-Zaidi, H. S. Majdi, A. A. AbdulRazak, S. Aal-Kaeb, A. A. Shohib and J. McGregor, Int. J. Chem. Eng., 2022, 2022, 8303874 Search PubMed.
- A. Anand and L. Suganthi, Energies, 2018, 11(4), 728 CrossRef.
- S. J. Kim, S. J. Bae and M. W. Jang, Sustainability, 2022, 14(18), 11674 CrossRef.
- Z. M. Shakor and A. H. Sadeiq, Eng. Technol. J., 2018, 36(11A), 1171–1175 CrossRef.
- R. H. Saihod, Z. M. Shakoor and A. A. Jawad, Al-Khawarizmi Eng. J., 2014, 10(1), 47–61 Search PubMed.
- S. Mirjalili, J. S. Dong, A. S. Sadiq and H. Faris, Nature-Inspired Optimizers: Theories, Literature Reviews and Applications, 2020, pp. 69–85 Search PubMed.
- D. Galvan, H. Cremasco, A. C. Mantovani, E. Bona, M. Killner and D. Borsato, Fuel, 2020, 267, 117221 CrossRef CAS.
- X. Y. Tai, R. Ocone, S. D. Christie and J. Xuan, Energy AI, 2022, 7, 100134 CrossRef.
- Á. Ibarra, I. Hita, J. M. Arandes and J. Bilbao, Catalysts, 2020, 10(10), 1157 CrossRef.
- F. Schmutzler, C. Zschiesche, J. Titus, D. Poppitz, J. Freiding, R. Rakoczy, A. Reitzmann and R. Gläser, Chem. Ing. Tech., 2021, 93(6), 981–989 CrossRef CAS.
- M. O. Kazakov, M. Y. Smirnova, M. E. Dubinin, T. S. Bogomolova, P. P. Dik, I. S. Golubev, M. E. Revyakin, O. V. Klimov and A. S. Noskov, Fuel, 2023, 344, 128085 CrossRef CAS.
- J. M. Schweitzer, J. Rey, C. Bignaud, T. Bučko, P. Raybaud, M. Moscovici-Mirande, F. Portejoie, C. James, C. Bouchy and C. Chizallet, ACS Catal., 2022, 12(2), 1068–1081 CrossRef CAS.
- W. Song, V. Mahalec, J. Long, M. Yang and F. Qian, Ind. Eng. Chem. Res., 2020, 59(7), 3077–3090 CrossRef CAS.
- B. D. Vandegehuchte, J. W. Thybaut, A. Martinez, M. A. Arribas and G. B. Marin, Appl. Catal., A, 2012, 441, 10–20 CrossRef.
- D. Baş, F. C. Dudak and I. H. Boyacı, J. Food Eng., 2007, 79(2), 622–628 CrossRef.
|
This journal is © The Royal Society of Chemistry 2023 |