DOI:
10.1039/D3RA01584J
(Paper)
RSC Adv., 2023,
13, 15086-15098
Theoretical predictions and experimental verifications of SERS detection in colorants
Received
10th March 2023
, Accepted 25th April 2023
First published on 16th May 2023
Abstract
Synthetic colorants added during food processing not only fail to provide nutrients, but also can be harmful to human health when used in excess. To establish a simple, convenient, rapid and low-cost surface-enhanced Raman spectroscopy (SERS) detection method for colorants, an active surface-enhanced substrate of colloidal gold nanoparticles (AuNPs) was prepared in this study. The density functional theory (DFT) method of B3LYP with 6-31G(d) was applied to determine the theoretical Raman spectra of erythrosine, basic orange 2, 21 and 22, and to attribute their characteristic spectral peaks. The SERS spectra of the four colorants were pre-processed using local least squares (LLS) and morphological weighted penalized least squares (MWPLS), and multiple linear regression (MLR) models were established to quantify the four colorants in beverages. The results showed that the prepared AuNPs with a particle size of about 50 nm were reproducible and stable, with a good enhancement of the SERS spectrum of rhodamine 6G at 10−8 mol L−1. The theoretical Raman frequencies were in good agreement with the experimental Raman frequencies, and the peak position differences of the main characteristic peaks of the four colorants were within 20 cm−1. The MLR calibration models for the concentrations of the four colorants showed relative errors of prediction (REP) of 2.97–8.96%, root mean square errors of prediction (RMSEP) of 0.03–0.94, R2 of 0.973–0.999, and limits of detection of 0.06 μg mL−1. The present method could be used to quantify erythrosine, basic orange 2, 21, and 22, revealing its wide range of applications in food safety.
1 Introduction
Food additives are natural or synthetic substances added to food by manufacturers to prevent spoilage or to enhance appearance, taste, texture, or nutritional value, and excessive consumption is harmful to human health.1 Erythrosine, basic orange 2, 21 and 22 are four common food additives. Erythrosine, a synthetic xanthophyll colorant widely used in the food industry, poses a potential health hazard to humans when consumed in excess.2 The Joint FAO/WHO Expert Committee on Food Additives 86th Meeting Summary and Conclusions 2018 established an acceptable daily intake of 0–0.1 mg kg−1 bodyweight for erythrosine,3 where excessive consumption can cause potential reproductive and neurobehavioral toxicity.4 Basic orange 2, 21 and 22 belong to industrial dyes, all of which are moderately toxic carcinogenic compounds and have been explicitly banned from use in food.5 However, due to their strong coloring power, stable color and cheap availability, many manufacturers use them illegally in food products. A survey of children aged 3 years and 8–9 years showed that children who ingested basic dyes had higher global hyperactivity aggregate scores compared to children who consumed placebo.6 In addition to these basic dyes, other food additives, either directly (chemicals intentionally added to foods) or indirectly (materials that may contaminate foods as part of packaging or manufacturing), also have potentially adverse health effects on children. Preservatives such as nitrates and nitrites are prone to cause methemoglobinemia and brain tumors in infants.7,8 Bisphenol A, used to make baby bottles, can cause childhood obesity and albuminuria.9,10 Phthalates, used in the manufacture of personal care products and flexible plastic tubing, can also cause childhood obesity and insulin resistance.11
The commonly and widely used methods for the detection of food colorants are chromatography, mass spectrometry, gas chromatography-mass spectrometry and spectrophotometry since their good accuracy and high precision.12–16 Moreover, a number of improved and emerging techniques have made the detection of food additives more portable, convenient, and visualized. For instance, colorimetry, fluorometry, combination of colorimetry and fluorometry, surface-enhanced Raman spectroscopy (SERS), and their derivatives combining with filter paper and/or smartphone for visual readout have made great progress in enhancing portability and practicality.17–24 These techniques can be very useful and promising for food colorant detection due to their good sensitivity, repeatability and stability.
In the current work, we aimed to establish a convenient, rapid and low-cost SERS detection method for food colorants to facilitate the food safety monitoring. SERS is a highly sensitive, fast and simple analysis technique that adsorbs the molecules to be measured on the surface of rough nano-metal materials to enhance the Raman signal of the subject by 106–1015. It not only enables non-destructive in situ detection of trace substances, but also provides information about the molecular structure of the substance to be tested, which has great potential for development in the food industry.25,26 The SERS effect depends on the stability and sensitivity of the active substrate.27 Currently, the main substrates used in SERS for the detection of colorants in food are metal sol nano-active substrates, gold (Au) or silver (Ag) nano-rod active substrates and metal electrode active substrates.28 Most of the novel nanomaterial substrates are improved based on metal nano-active substrates, thus improving the performance of the new nano-substrates. Such as using colloidal Au active substrates as gold seeds, adding cetyl trimethyl ammonium bromide, synthesizing Au nano-rod active substrates and detecting temptation red as well as sunset yellow in beverages.29 The preparation of uniformly sized, effect-enhancing active substrates is important for the development of SERS substrates.
To the best of our knowledge, there have been few studies of direct detection of the erythrosine, basic orange 2, 21 and 22 by SERS. In this study, we prepared stable, sensitive and uniform-sized colloidal gold nanoparticles (AuNPs) as a SERS-enhanced substrate to construct a simple, rapid, sensitive and environmentally friendly SERS technique that can be used to detect food colorants.
2 Methods and materials
2.1 Reagents
Gold(III) chloride (CAS#27988-77-8) and sodium citrate tribasic (CAS#6858-44-2) were purchased from Sigma-Aldrich (Shanghai, China). Nitric acid (CAS#7697-37-2) was bought from Sinopharm Chemical Reagent Co., Ltd (Shanghai, China). Rhodamine 6G (R6G) was obtained from (CAS#989-38-8) Sangon Biotech (Shanghai, China). The standard substances erythrosine (GBW(E)100163, CAS#16423-68-0), basic orange 2 (CAS#532-82-1), 21 (CAS#3056-93-7) and 22 (CAS#4657-00-5) were supplied by Bjhongmeng Co., Ltd (Beijing, China). Four types of beverages FDDR, FDDY, VCD and DPD are commercially available.
2.2 Apparatus
The SERS experiments were detected by a Polaris-R80 portable Raman spectrometer (Polaris Scientific, Suzhou, China). Transmission electron microscopy (TEM) images were obtained on a JEM-2100 instrument (JEOL Ltd, Mitaka-shi, Japan). The ultraviolet (UV) absorption spectrum was measured by a UV-1700SPC UV spectrophotometer (Macylab instrument, Shanghai, China).
2.3 AuNPs preparation and characterization methods
AuNPs were prepared using the chemical reduction method.30 50 mL gold(III) chloride solution (1.00 mmol L−1) was transferred to a 250 mL three-mouth flask, stirred and heated to boil in a refluxing manner. Then 1.85 mL sodium citrate tribasic solution (38.80 mmol L−1) was slowly added to the flask after reflux and continuously heated for 15 min. The solution was allowed to cool naturally to room temperature and the colloidal AuNPs were obtained. The UV spectrophotometer was preheated for 30 min. Using distilled water as a reference, the prepared AuNPs were diluted three times and placed in a cuvette for absorption spectroscopy. Subsequently, 10 μL of colloidal AuNPs were placed on a clean copper net and dried naturally. The morphological feature and particle sizes of AuNPs were observed at 30KX, 50KX and 100KX magnifications with TEM operating at a voltage of 20 kV.
2.4 Beverage samples preparation
Each beverage sample was accurately weighed at 10.00 g and placed in a beaker for ultrasonic exhaust treatment to reduce the effect of CO2 in the beverage on the target signal. After degassing, the samples were allowed to stand for 5 min and diluted to 2, 5 and 10 times as needed. Appropriate volume of supernatant was filtrated through a 0.22 μm hydrophilic filter membrane, and 100 μL of the filter liquor was taken into a sample bottle for testing.
2.5 SERS measurements
100 μL of either R6G or beverage samples was taken in the sample bottle, and then 500 μL of colloidal AuNPs and 100 μL of nitric acid solution (agglomeration agent, 0.5 mol L−1) were added in turn. After mixing, the detection was carried out on the Raman spectrometer instrument. The laser power was 250 mW and the integration time was 15 s.
2.6 Density functional theory (DFT) calculation
Density Functional Theory (DFT) B3LYP method and 6-31G(d) basis set were used to calculate the theoretical SERS spectra of erythrosine, basic orange 2, 21 and 22 by GaussView5.0 and Gaussian 09.31,32 We compared the experimental SERS spectra with the theoretical SERS spectra, determined the attribution of the characteristic spectral bands and correlated the vibration wavenumber and vibration mode of the molecular group.
2.7 Data analysis
Multivariate data analysis was completed using PLS Toolbox 4.0 (Eigenvector Research, Inc., US) and in-house written routines in the MATLAB environment (The MathWorks, Inc., US) on a standard PC.
3 Results and discussion
3.1 Characterization of AuNPs
Gold(III) chloride can react with sodium citrate tribasic to form gold particles with a diameter of tens of nanometers.33 The particle sizes and morphological features of the AuNPs were observed by TEM at 20KX, 50KX and 100KX magnifications, which showed that the AuNPs were in uniform-sized, with a particle size of about 55 nm and a spherical shape (Fig. 1A–C). The characterization detection results showed that the AuNPs had only one absorption spectral peak at 450–650 nm. The largest UV absorption peak was at 535 nm, the half-peak width of the absorption peak was 46 nm, and the peak shape was narrow (Fig. 1D), indicating that the size distribution of AuNPs was uniform and no other sizes of AuNPs were produced.
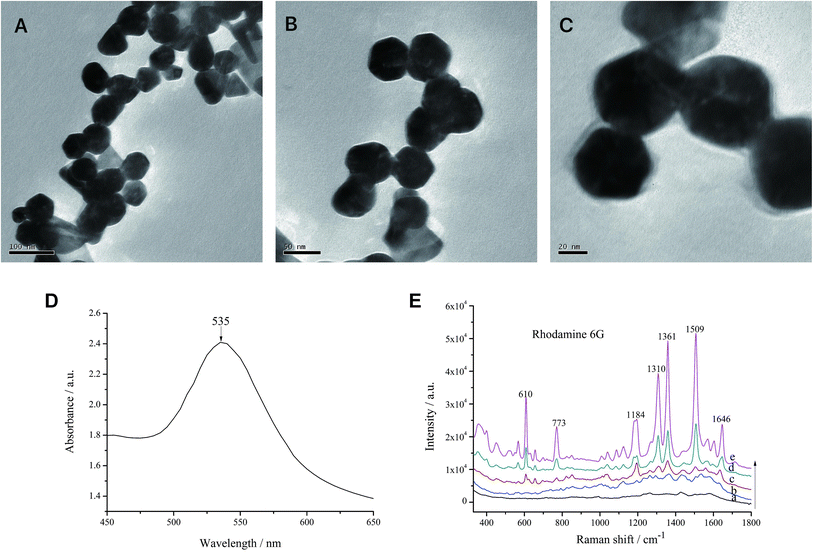 |
| Fig. 1 Characterization of AuNPs. (A–C) TEM images of AuNPs observed at 30KX, 50KX and 100KX. Scale bar, 100, 50 and 20 μm; (D) UV absorption spectrum of AuNPs; (E) overlay SERS spectra of R6G solutions at different concentrations of 0 (a), 10−8 (b), 10−7 (c), 10−6 (d) and 10−5 (e) mol L−1. | |
The SERS characterization of AuNPs using R6G as a probe showed that when the concentration of R6G was as low as 10−8 mol L−1, it still produced obvious characteristic peaks at 610, 773, 1184, 1136 and 1509 cm−1. These results indicated that AuNPs prepared in this experiment increased the sensitivity of SERS (Fig. 1E).
3.2 Stability and reproducibility of colloidal AuNPs
The stability and reproducibility of AuNPs were examined every seven days using R6G as the probe. The results showed that the AuNPs had the best stability and R6G had the best spectral enhancement effect at day 7 and day 14, which then decreased with time (Fig. 2A). During the preservation, the AuNPs interacted with each other and formed agglomerates, which led to a decrease in stability and affected the enhancement effect of colloidal AuNPs.34 The relative variance of the eight SERS spectra collected within the range of 330–1800 cm−1 was 14.61% in 0–49 days and 8.30% in 0–28 days. Therefore, colloidal AuNPs can be stored for about 28 days at room temperature away from light.
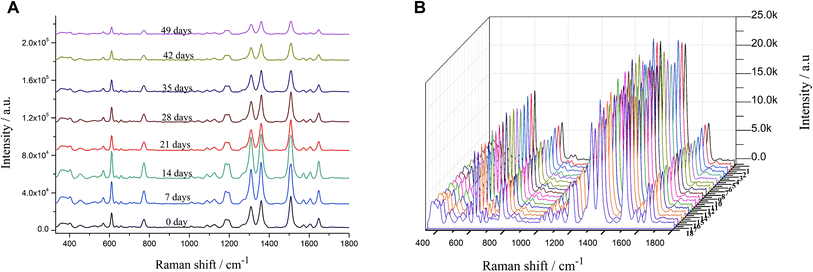 |
| Fig. 2 SERS spectra acquired from R6G probe in 10–6 mol L−1 for testing. (A) The stability of AuNPs colloid over a storage period of 49 days; (B) the reproducibility of AuNPs preparation for different batches. | |
To determine the reproducibility of AuNPs prepared from different batches, SERS were examined by using R6G at a concentration of 10−6 mol L−1 as a probe, and three SERS spectra were randomly collected from each batch. The relative variance of the 18 calculated SERS spectra in 330–1800 cm−1 wave number was 0.50%, indicating that the SERS spectra varied little between different batches and the colloidal AuNPs were reproducible (Fig. 2B).
3.3 Optimization of SERS measurements
We investigated the effect of coagulants on the SERS signal of erythrosine in this section. The role of the reunion agent is to promote the agglomeration of AuNPs, forming “hot spots” between the particles, thus making the signal of the test substance enhanced.35 After adding 0.05 mol L−1 nitric acid, the SERS signal showed no difference, indicating that there was no agglomeration between AuNPs or no agglomeration to the “hot spots” generation. When the nitric acid concentration was 0.10 mol L−1, the SERS signal was significantly enhanced (Fig. 3A). However, as the concentration of nitric acid increased, the SERS signal of erythrosine did not increase but decreased significantly (Fig. 3B). The above results suggested that the reunion agent increased the agglomeration effect of the particles, with the disappearance of some of the “hot spots”. We speculate that when the reunion agent is added to the system, it increased the agglomeration effect of the particles, causing them to clump together and form larger aggregates. This can result in the redistribution of particles across the system, leading to a decrease in local concentrations and ultimately causing the hot spots to disappear.36,37 Nitric acid is a strong acid and may be hazardous to the environment and the operators. We therefore chose 0.10 mol L−1 nitric acid as the optimal concentration without affecting the signal enhancement.
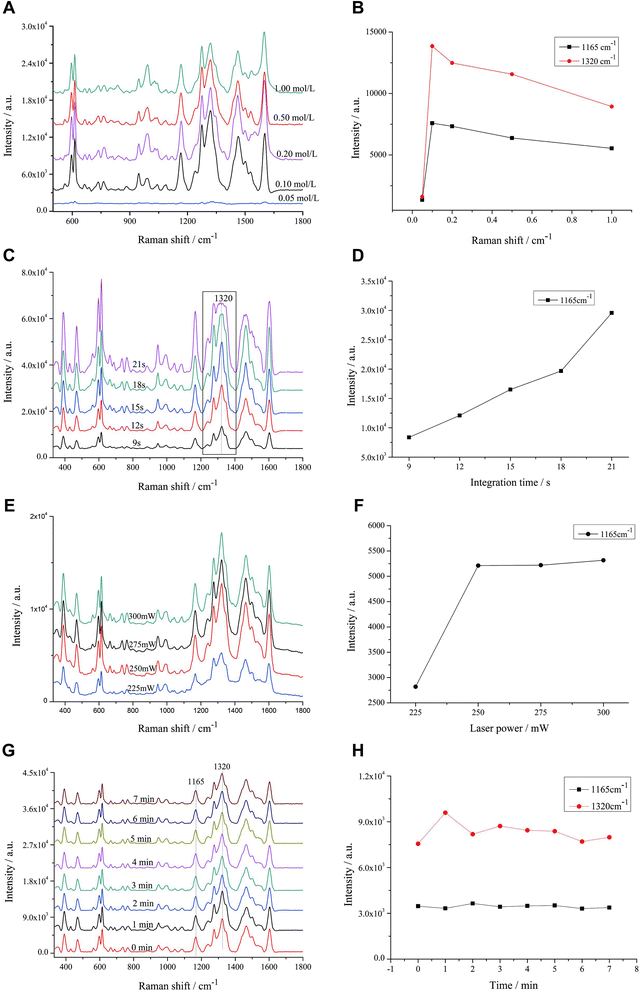 |
| Fig. 3 Optimization of SERS measurements by testing erythrosine solution in 10 μg mL−1. (A and B) Effect of different concentrations of reunion agent nitric acid (0.05, 0.01, 0.2, 0.5 and 1.0 mol L−1) on SERS of erythrosine; (C and D) effect of integration time on SERS of erythrosine; (E and F) effect of laser power on SERS of erythrosine; (G and H) effect of mixing time of AuNPs and sample to be tested on SERS. | |
This section explored the effect of integration time on the SERS signal of erythrosine. The longer the integration time, the stronger the SERS signal of the target substance. The integration time started from 9 s, and when the integration time increased to 18 s, the peak at 1320 cm−1 showed a “plateau” phenomenon. When the integration time was further increased, the “plateau” phenomenon became more obvious (Fig. 3C), indicating that the AuNPs were highly sensitive and had a good enhancement effect on the erythrosine, and the peak reached the maximum range of the instrument. Therefore, the SERS data obtained from the integration time of 18 s and later would be inaccurate for qualitative and quantitative analysis. Even though the concentration of erythrosine gradually increased with the integration time (Fig. 3D), 15 s was still selected as the optimal integration time.
This section investigated the effect of laser power on the SERS signal of erythrosine. The power was set at 225, 250, 275 and 300 mW. The SERS signal intensity at the laser power of 250 mW increased significantly compared to that at 225 mW, but the subsequent increase in laser power had almost no effect on the SERS signal (Fig. 3E). Therefore, in combination with the results in Fig. 3F, 250 mW was finally selected as the optimal laser power to ensure the signal intensity and the instrument lifetime.
This section was to investigate the effect of mixing time with AuNPs colloids on the SERS signal of erythrosine. When AuNPs colloids were added to the erythrosine sample, they interacted with each other. Some of the sample molecules were adsorbed onto the surface of the AuNPs, while others were shed from the AuNPs, and the whole process is a dynamic one. Thus, signal instability is a common problem encountered in SERS detection when AuNPs are added to the sample.
After mixing the sample with colloidal AuNPs well, SERS spectra were collected every 1 min. The relative variance of the eight spectra collected from 0 to 7 min was 0.63% in 330–1800 cm−1 wave number (Fig. 3G). In addition, the dependence of the intensity changes on the mixing time at 1165 and 1320 cm−1 from 0 to 7 min was investigated. The signal intensity of erythrosine was stable within 7 min (Fig. 3H). It is suggested that the use of AuNPs as the active substrate stabilizes the SERS signal.
Currently, the adsorption mechanism of erythrosine, basic orange 2, 21 and 22 by colloidal gold nanoparticles is not yet fully understood and requires further investigation. However, it is believed that the adsorption may involve several factors such as electrostatic interactions, hydrogen bonding, hydrophobic interactions, and van der Waals forces between the dyes and the surface of the colloidal gold nanoparticles. The specific contributions of these factors may vary depending on the properties of the dye molecules and the surface of the nanoparticles. Additionally, the adsorption mechanism may also be influenced by the size, shape, and surface charge of the colloidal gold nanoparticles, as well as the concentration and pH of the solution. In this study, the surface of AuNPs becomes negatively charged due to the adsorption of citrate ions, while basic orange 2, 21 and 22 are classified as basic dyes carrying positive charges. Therefore, it is speculated that the adsorption of these dyes by AuNPs may be related to electrostatic interactions. Further research is needed to better understand the underlying mechanisms involved in the adsorption of these dyes by AuNPs.
3.4 Theoretical and experimental Raman spectra of erythrosine, basic orange 2, 21 and 22
In this section, we determined the theoretical Raman spectra of erythrosine, basic orange 2, 21 and 22 by using the DFT B3LYP method with a 6-31G(d) basis set. The ratio of the overlapping areas of theoretical and experimental Raman spectra to the experimental Raman spectral areas was calculated, and the main characteristic peak positions of the two spectra can be well matched. Then, according to the optimized molecular spatial structures, the characteristic peaks of the four colorants were identified by combining the theoretical and experimental spectra, which provided theoretical support for the qualitative analysis of erythrosine, basic orange 2, 21 and 22.
Firstly, the spatial structure of erythrosine was optimized and computed. Since erythrosine has iodine atoms, the SDD pseudopotential basis set was also used (Fig. 4A). Next, we compared the theoretical and experimental Raman spectra. Both spectra were normalized to compare the similarities and differences more clearly between them. The overlapping areas of the two spectra are calculated by using the spline fitting function and trapezoidal rule. In the 330-1800 cm−1 wave number, the ratio of the overlapping areas to the experimental Raman spectra areas was 28.67% (Fig. 4B). The similarity between the theoretical and experimental Raman spectra was compared in terms of peak positions, and the vibrational attribution of the main Raman characteristic peaks of erythrosine was obtained. The peak position differences between the main characteristic peaks of theoretical and experimental Raman spectra were within 20 cm−1 (Table 1). There are some differences between the theoretical and experimental characteristic peaks, which may be due to the following two reasons: (1) the theoretical calculation considers the optimal configuration of a single molecule in an ideal state, while the actual state of existence of molecules is likely to be multiple molecules clustered together. Theoretical Raman spectra do not consider the interactions between multiple molecules, and thus lead to some discrepancies between theory and experiment;38 (2) experimental Raman spectra were obtained by dissolving erythrosine in water for detection, ignoring solvent effects. Moreover, many functional groups can be better bound to the surface-active substrate after hydrolysis, and the peaks with insignificant intensity in the theoretical Raman spectra are enhanced in the experimental Raman spectra, which may be one of the reasons for the smaller ratio of the overlapping areas to the experimental Raman spectra areas.
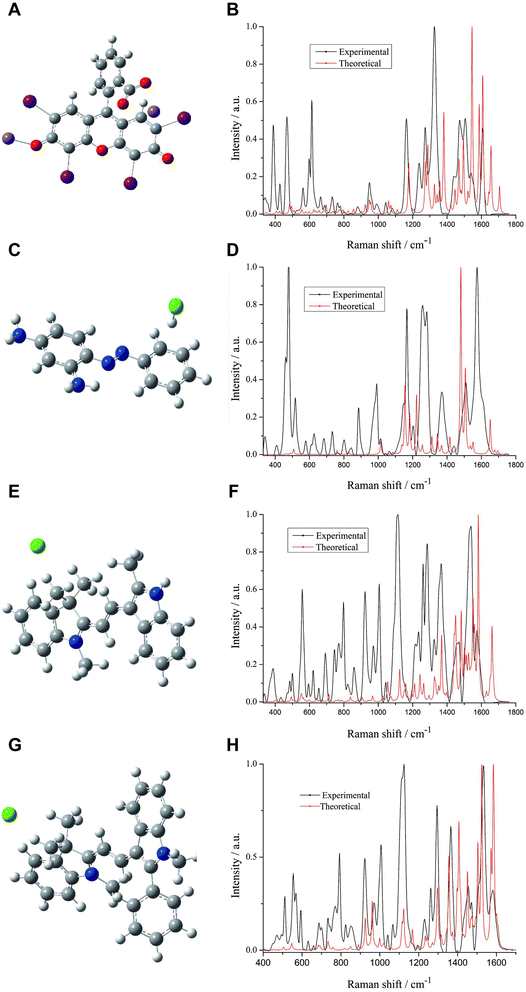 |
| Fig. 4 Theoretical Raman and experimental SERS of four colorants and their molecular structures. (A) erythrosine, (B) basic orange 2, (C) basic orange 21, and (D) basic orange 22. Red curve represented the theoretical Raman spectra, and black denoted the experimental SERS, respectively. | |
Table 1 Comparison of the theoretical vibration modes and experimental Raman spectra of erythrosinea
No. |
Experimental (cm−1) |
Theoretical (cm−1) |
Assignment |
Vibration modes: ν, stretching; δ, in-lpane deformation. |
1 |
466 |
482 |
δ(phenyl) |
2 |
613 |
624 |
δ(phenyl) |
3 |
944 |
947 |
ν(C–H) |
4 |
1165 |
1176 |
ν(C–C), δ(C–H) |
5 |
1274 |
1273 |
δ(C–H), ν(C–O) |
6 |
1461 |
1468 |
ν(C C), δ(C–H) |
7 |
1598 |
1607 |
δ(phenyl) |
The spatial structures of basic orange 2, 21 and 22 were obtained by the same method (Fig. 4C, E, and G). The ratios of the theoretical and experimental spectra overlapping areas of basic orange 2, 21 and 22 to the experimental Raman spectral areas were 12.18%, 19.70% and 27.12%, respectively (Fig. 4D, F, and H). The vibrational attribution of the main characteristic peaks of basic orange 2, 21 and 22 was identified and the results are shown in Tables 2–4.
Table 2 Comparison of the theoretical vibration modes and experimental Raman spectra of basic orange 2a
No. |
Experimental (cm−1) |
Theoretical (cm−1) |
Assignment |
Vibration modes: ν, stretching; δ, in-lpane deformation; subscript s: symmetric. |
1 |
517 |
507 |
δ(phenyl) |
2 |
993 |
995 |
δ(C–H) |
3 |
1147 |
1155 |
ν(C–N), δ(C–H) |
4 |
1165 |
1182 |
δ(C–H), ν(C–N) |
5 |
1260 |
1256 |
ν(C–N), ν(C N), δ(C–H) |
6 |
1370 |
1368 |
ν(C–H), δ(C–H), δ(phenyl) |
7 |
1507 |
1506 |
δ(C–H), ν(N N) |
8 |
1615 |
1612 |
νs(phenyl), δ(N–H) |
Table 3 Comparison of the theoretical vibration modes and experimental Raman spectra of basic orange 21a
No. |
Experimental (cm−1) |
Theoretical (cm−1) |
Assignment |
Vibration modes: ν, stretching; δ, in-lpane deformation. |
1 |
557 |
557 |
δ(phenyl) |
2 |
800 |
820 |
δ(phenyl) |
3 |
1115 |
1109 |
ν(C–N), δ(C–H) |
4 |
1189 |
1196 |
δ(C–H) |
5 |
1261 |
1269 |
ν(C–N), ν(C–N), δ(C–H) |
6 |
1285 |
1295 |
ν(C–N), δ(C–H), ν(C–C) |
7 |
1365 |
1368 |
δ(C–H), ν(C N) |
8 |
1457 |
1451 |
δ(C–H), ν(C N) |
9 |
1470 |
1469 |
δ(C–H) |
10 |
1539 |
1536 |
δ(C–H), δ(phenyl) |
11 |
1570 |
1584 |
δ(C–H), ν(C N), ν(C C) |
Table 4 Comparison of the theoretical vibration modes and experimental Raman spectra of basic orange 22a
No. |
Experimental (cm−1) |
Theoretical (cm−1) |
Assignment |
Vibration modes: ν, stretching; δ, in-lpane deformation. |
1 |
555 |
566 |
δ(phenyl) |
2 |
923 |
925 |
δ(C–H) |
3 |
1115 |
1115 |
δ(C–H) |
4 |
1124 |
1123 |
δ(C–H) |
5 |
1294 |
1296 |
δ(C–H) |
6 |
1365 |
1355 |
δ(C–H), ν(C–C) |
7 |
1532 |
1525 |
δ(C–H), δ(C–H) |
8 |
1581 |
1584 |
δ(C–H), ν(C C), ν(C N) |
3.5 SERS measurements of erythrosine, basic orange 2, 21 and 22 from beverages
3.5.1 Data pre-processing. The four selected beverages (FDDR, VCD, FDDY, DPDY) were added as substrates to the standard substances of the four colorants respectively, formulated as positive samples with different concentrations, and the multiple linear regression (MLR) calibration models were established separately. The Raman spectrum is mainly influenced by the Mie scattering before 300 cm−1 wave number, which is constantly changing. After 1800 m−1 wave number, it is mainly influenced by water. Therefore, it has an impact on the predictive power of the MLR model.39 In this work, 330–1800 cm−1 wave numbers were selected for pre-processing, MLR model establishment and SERS measurements.A total of eight MLR models were established. The concentrations of FDDR-erythrosine were 0.10, 0.20, 0.50, 0.80, 1.00, 2.00, 5.00, 8.00 and 10.00 μg mL−1. The concentrations of VCD-erythrosine were 1.00, 2.00, 5.00, 8.00, 10.00, 12.00, 15.00 and 20.00 μg mL−1. The concentrations of FDDY-basic orange 2 were 1.00, 2.00, 5.00, 8.00, 10.00, 12.00 and 20.00 μg mL−1. The concentrations of FDDY-basic orange 21 were 0.05, 0.08, 0.10, 0.20, 0.50, 0.80, 1.00, 2.00 and 5.00 μg mL−1. The concentrations of FDDY-basic 22 were 0.05, 0.08, 0.10, 0.20, 0.50, 0.80 and 1.00 μg mL−1. The concentrations of DPDY-basic orange 2 were 0.10, 0.20, 0.50, 0.80, 1.00, 2.00, 5.00 and 8.00 μg mL−1. The concentrations of DPDY-basic orange 21 were 0.10, 0.20, 0.50, 0.80, 1.00, 2.00, 5.00 and 8.00 μg mL−1. The concentrations of DPDY-basic orange 22 were 0.10, 0.20, 0.50, 0.80, 1.00, 2.00, 5.00 and 8.00 μg mL−1.
3.5.2 Spectra calibration. The same chemical substance has the same spectrum, but the spectrum of the same batch of samples measured under the same conditions may have small drifts in their peaks due to the influence of ambient temperature, instrument, laser source and sample concentration. The MLR model is constructed based on the entire spectrum, and its accuracy and sensitivity can be affected by the peak drift of the Raman spectrum. In this study, the Local Least Square (LLS) was used to pre-process the obtained spectra.40 Taking the spectrum obtained from the measurement of DPD-basic orange 21 as an example, the characteristic peak of the Raman spectrum drifted to the right with increasing concentration of basic orange 21 at 1536 cm−1 wave number (Fig. 5A). After the calibration, the drift was eliminated (Fig. 5B).
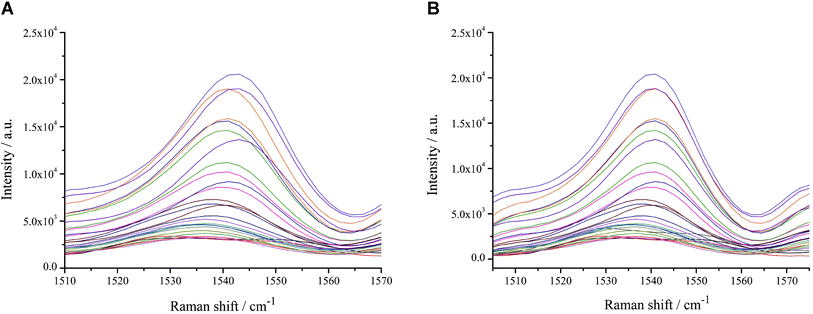 |
| Fig. 5 Illustration of band alignment of the spectra of the DPD-basic orange 21 mixtures before (A) and after (B) spectra calibration, concerning the 1536 cm–1 band. | |
3.5.3 Background correction. During the detection process, the signal generated by the interference of the inductively coupled detector, the laser source (producing diffuse reflection, Mie scattering and Rayleigh scattering) and solvent fluorescence is called the background. The background signal itself contains no sample information. The presence of background in the SERS spectrum affects the validity of the target signal and increases the difficulty of qualitative analysis and quantitative detection of the target substance. Background interference also affects the selectivity and sensitivity of the analysis method and reduces the accuracy and precision of the spectral modeling. For further accurate analysis, it is necessary to eliminate or correct the background interference.In the present study, we used Morphological Weighted Penalized Least Squares (MWPLS) to correct the background interference from the SERS spectral data.41
The spectra of basic orange 22 before and after background correction are shown in Fig. 6A and B. The SERS spectra of FDDY-basic orange 21 were pre-processed with spectra calibration and background correction separately to construct the MLR model. The effect of the pre-processing method on the MLR model was evaluated by comparing the main parameters of the two models. The relative errors of prediction (REP) and root mean square error of prediction (RMSEP) were used to compare the effect of the two pre-processing methods on the predictive ability of the MLR model, and smaller REP and RMSEP indicate better predictive ability. As shown in Table 5, both pre-processing methods improved the MLR model. Therefore, it is necessary to perform spectra calibration and background correction before constructing the MLR model. The background correction pre-processing method had a greater impact on REP and RMSEP, suggesting the background interference may be the main factor affecting the predictive ability. Although spectra calibration showed less effectiveness in improving SERS, it may be more effective in severe peak drift conditions.
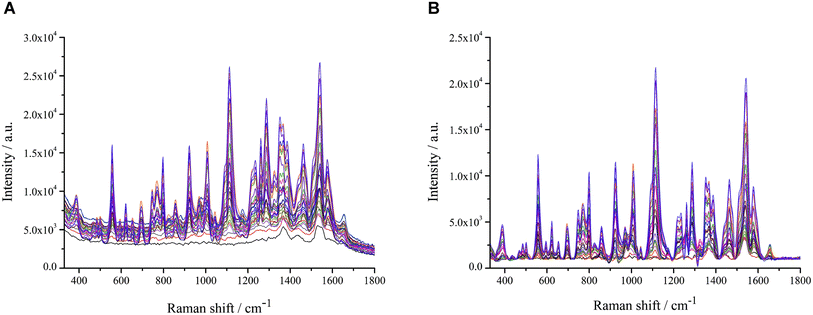 |
| Fig. 6 SERS of the FDDY-basic orange 22 mixtures before (A) and after (B) background correction. | |
Table 5 The MLR model of DPD-basic orange 21 SERS spectra with different pre-processing
MLR modelling data |
REP (%) |
RMSEP (μg mL−1) |
RV (%) |
Raw data |
11.36 |
0.16 |
5.63 |
LLS |
11.06 |
0.12 |
7.87 |
MWPLS |
3.12 |
0.03 |
27.45 |
LLS + MWPLS |
2.97 |
0.03 |
28.67 |
y |
— |
— |
66.01 |
To further discuss the effect of the two pre-processing methods on the data, the relative variances of the raw data, the spectra calibration data, the background correction data, the spectra calibration and background correction data and the concentration values corresponding to the spectra were calculated separately. The results showed that the relative variance of the spectra improved from 5.63% to 5.71% (1.42% improvement) after spectra calibration. The relative variance of the spectra improved from 5.63% to 27.45% (79.48% improvement). The relative variance of SERS after background correction was closer to the relative variance of the concentration values because the common interference between SERS was eliminated after background correction. And the differences between SERS at each concentration were also highlighted, leading to a more conducive quantitative analysis.
3.5.4 Model construction of erythrosine, basic orange 2, 21 and 22 in beverages. FDDY-basic orange 21 was used as an example for SERS measurement. Compared to the SERS with the addition of basic orange 21, no characteristic peak was shown in the blank control, indicating that the FDDY contains no basic orange 21. The SERS spectrum of the blank had a signal within 1300–1800 cm−1 wave numbers, which was mainly caused by the absorption of water (Fig. 7A). The established MLR model is shown in Fig. 7B. The R2, REP and RMSEP of the standard curve are 0.997, 5.85% and 0.02, respectively. For linear calibration curves, the instrument response (y) is assumed to be linearly related to the standard concentration (x) over a finite concentration range, expressed in the model as y = a + bx. The limit of detection (LOD) and limit of quantification (LOQ) are expressed as LOD = 3Sa/b and LOQ = 10Sa/b, respectively, where Sa is the standard deviation of 20 blank responses and b is the slope of the calibration curve.42
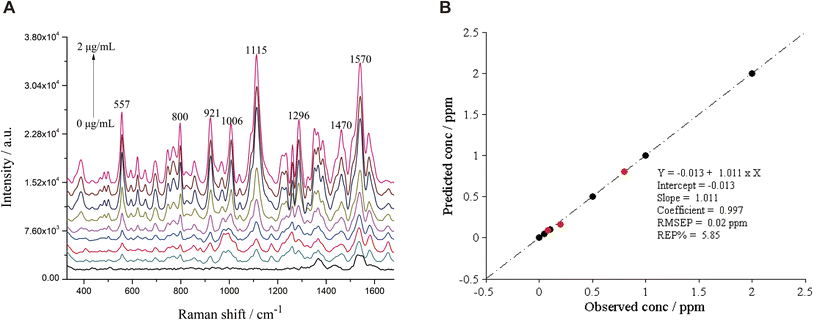 |
| Fig. 7 The SERS (A) and MLR model (B) of different concentrations of basic orange 21 in FDDY. Black and red solid circles respectively labelled the FDDY-basic orange 21 mixture samples in the calibration and prediction sets. | |
The eight MLR models developed in this study had good predictive ability, with an R2 range of 0.973–0.999, REP range of 2.97–8.96%, RMSEP range of 0.03–0.94, and low detection limit of 0.06 μg mL−1 (Table 6). The relative variance was 6.28% for 20 repeated measurements of basic orange 21 (0.6 μg mL−1) in FDDY beverages over the course of a day. Different concentrations of standard substances of erythrosine, basic orange 2, 21 and 22 were added to FDDR, FDDY, VCD, and DPD samples within a linear range. The concentrations of FDDR-erythrosine were 0.90, 4.00 and 6.00 μg mL−1, VCD-erythrosine were 7.00, 9.00 and 11.00 μg mL−1, VCD-erythrosine were 4.00, 6.00 and 7.00 μg mL−1, FDDY-basic orange 2 were 4.00, 6.00 and 7.00 μg mL−1, FDDY-basic orange 21 were 0.40, 0.60 and 0.70 μg mL−1, FDDY-basic orange 22 were 0.07, 0.09, 0.40 and 0.60 μg mL−1, DPDY-basic orange 2 were 0.40, 0.60 and 0.70 μg mL−1, DPDY-basic orange 21 were 0.60, 0.70 and 0.90 μg mL−1, and DPDY-basic orange 22 were 0.40, 0.60 and 0.70 μg mL−1. Five batches of predicted samples were simulated to evaluate the predictive ability of the model with two indicators, REP and RMSEP, ranging from 6.67 to 9.79% for REP and 0.04 to 0.54 μg mL−1 for RMSEP (Table 7). The above results indicated that the SERS technique combined with the chemometric method is suitable for the rapid quantitative analysis of erythrosine, basic orange 2, 21 and 22 in beverages.
Table 6 Calibration models of four colorants in spiking beverage mixtures, established on the SERS spectra in the region of 330–1800 cm–1 after background correction and band alignment
Mixtures |
Linear range (μg mL−1) |
Regression equation |
R2 |
REP (%) |
RMSEP (μg mL−1) |
LOD (μg mL−1) |
LOQ (μg mL−1) |
FDDR-erythrosine |
0.10–10.00 |
y = 0.102 + 1.009x |
0.999 |
7.13 |
0.18 |
0.27 |
0.90 |
VCD-erythrosine |
1.00–20.00 |
y = −1.305 + 1.122x |
0.998 |
8.96 |
0.75 |
0.69 |
2.31 |
FDDY-basic orange 2 |
1.00–20.00 |
y = 1.195 + 0.845x |
0.973 |
8.17 |
0.94 |
0.39 |
1.23 |
FDDY-basic orange 21 |
0.05–2.00 |
y = −0.013 + 1.011x |
0.997 |
5.58 |
0.02 |
0.06 |
0.20 |
FDDY-basic orange 22 |
0.05–1.00 |
y = 0.025 + 1.050x |
0.999 |
6.77 |
0.04 |
0.06 |
0.20 |
DPD-basic orange 2 |
0.10–8.00 |
y = −0.040 + 1.033x |
0.980 |
8.93 |
0.10 |
0.15 |
0.51 |
DPD-basic orange 21 |
0.10–8.00 |
y = −0.020 + 0.983x |
0.999 |
2.97 |
0.03 |
0.06 |
0.20 |
DPD-basic orange 22 |
0.10–8.00 |
y = −0.380 + 1.057x |
0.991 |
3.29 |
0.33 |
0.06 |
0.20 |
Table 7 Predictions of four colorants in spiking beverage mixtures, by eight calibration models using the SERS spectra in the region of 330–1800 cm–1 after background correction and band alignment
Mixture scenarios |
RMSEP (μg mL−1) |
REP (%) |
FDDR-erythrosine |
0.41 |
7.22 |
VCD-erythrosine |
0.26 |
6.35 |
FDDY-basic orange 2 |
0.55 |
9.79 |
FDDY-basic orange 21 |
0.38 |
7.65 |
FDDY-basic orange 22 |
0.15 |
8.74 |
DPD-basic orange 2 |
0.04 |
6.67 |
DPD-basic orange 21 |
0.24 |
8.62 |
DPD-basic orange 22 |
0.54 |
9.44 |
4 Limitations of the study
The present study on the development of a SERS detection method for colorants has several limitations that need to be addressed. Firstly, we only focused on four synthetic colorants. Future studies should evaluate a wide range of colorants to determine the applicability of this method in food safety. Secondly, we did not evaluate the cross-reactivity of the method to other substances present in beverages, which could lead to false-positive results or interference. Thirdly, we did not examine the effect of environmental factors such as temperature and humidity on the stability and reproducibility of the AuNPs. More studies are needed to investigate the impact of these factors on the performance of the method and ensure that the method remains reliable under different environmental conditions.
5 Conclusion
In this work, the SERS-enhanced active colloidal AuNPs substrate was successfully synthesized, with great reproducibility, high stability and satisfactory enhancement of the SERS spectra of erythrosine, basic orange 2, 21 and 22. Based on DFT B3LYP method with a 6-31G(d) basis set, the theoretical Raman spectra of the four colorants were calculated. The theoretical and experimental Raman spectra can be well matched and the main characteristic peak position differences were within 20 cm−1. Moreover, the characteristic peaks of the four colorants were assigned according to the optimized molecular spatial structures, which provides theoretical support for their qualitative analysis. Finally, the SERS technique combined with chemometrics, LLS and MWPLS was used to pre-process the SERS spectra of the four synthetic colorants in beverage samples, and the MLR models of the SERS spectra and the concentration of colorants in beverages were established. The predictive ability of the models was good, as assessed by R2, REP, RMSEP, LOD and LOQ. The SERS combined with chemometric analysis offered a rapid and viable method suitable for the detection of colorants or industrial pigments, which could be artificially used as synthetic additives to beverage in an excessive or illegal way, so as to ensure food safety.
Author contributions
Mingyan Cao: data curation, formal analysis, writing - original draft. Jiamin Chen: data curation, validation. Boyan Li: Conceptualization, methodology, formal analysis, writing – review & editing, funding acquisition. Feng Xie: resources, project administration, supervision, writing – review & editing. Xiaohong Sun: supervision, writing – review & editing.
Conflicts of interest
The authors have declared no conflict of interest.
Acknowledgements
This work was funded by the National Natural Science Foundation of China (Grant No. 21864008), Guizhou Provincial Science and Technology Projects ([2018]1130), and Plan Project of Science and Technology of Guizhou Province (Qiankehefuqi [2019]4001 and Qiankehefuqi [2021]6).
References
- P. Pressman, R. Clemens, W. Hayes and C. Reddy, Food additive safety: A review of toxicologic and regulatory issues, Toxicol. Res. Appl., 2017, 1 DOI:10.1177/2397847317723572.
- P. Amchova, H. Kotolova and J. Ruda-Kucerova, Health safety issues of synthetic food colorants, Regul. Toxicol. Pharmacol., 2015, 73(3), 914–922 CrossRef CAS PubMed.
- Joint FAO/WHO Expert Committee on Food Additives 86th Meeting Summary and Conclusions, 2018. Available online at: https://www.fao.org/food/food-safety-quality/scientific-advice/jecfa/summary-reports/en/.
- T. Tanaka, Reproductive and neurobehavioural toxicity study of erythrosine administered to mice in the diet, Food Chem. Toxicol., 2001, 39(5), 447–454 CrossRef CAS PubMed.
- H. Zhang, D. Chen and X. Lv, et al., Energy-efficient photodegradation of azo dyes with TiO(2) nanoparticles based on photoisomerization and alternate UV-visible light, Environ. Sci. Technol., 2010, 44(3), 1107–1111 CrossRef CAS PubMed.
- D. McCann, A. Barrett and A. Cooper, et al., Food additives and hyperactive behaviour in 3-year-old and 8/9-year-old children in the community: a randomised, double-blinded, placebo-controlled trial, Lancet, 2007, 370(9598), 1560–1567 CrossRef CAS PubMed.
- J. M. Pogoda, S. Preston-Martin and G. Howe, et al., An international case-control study of maternal diet during pregnancy and childhood brain tumor risk: a histology-specific analysis by food group, Ann. Epidemiol., 2009, 19(3), 148–160 CrossRef PubMed.
- Y. Grosse, R. Baan and K. Straif, et al., Carcinogenicity of nitrate, nitrite, and cyanobacterial peptide toxins, Lancet Oncol., 2006, 7(8), 628–629 CrossRef PubMed.
- R. Bhandari, J. Xiao and A. Shankar, Urinary bisphenol A and obesity in U.S. children, Am. J. Epidemiol., 2013, 177(11), 1263–1270 CrossRef PubMed.
- L. Trasande, T. M. Attina and H. Trachtman, Bisphenol A exposure is associated with low-grade urinary albumin excretion in children of the United States, Kidney Int., 2013, 83(4), 741–748 CrossRef CAS PubMed.
- L. Trasande, T. M. Attina and S. Sathyanarayana, et al., Race/ethnicity-specific associations of urinary phthalates with childhood body mass in a nationally representative sample, Environ. Health Perspect., 2013, 121(4), 501–506 CrossRef PubMed.
- J. Zhou, L. J. Liang and B. Zeng, Separation and determination of basic orange II, acid orange II and auramine O in soybean products based on ionic liquid reverse micelle microextraction and ultra-high-performance liquid chromatography, J. Chromatogr., 2022, 1673, 463042 CrossRef CAS PubMed.
- T. Vail, P. R. Jones and O. D. Sparkman, Rapid and unambiguous identification of melamine in contaminated pet food based on mass spectrometry with four degrees of confirmation, J. Anal. Toxicol., 2007, 31(6), 304–312 CrossRef CAS PubMed.
- X. M. Xu, Y. P. Ren and Y. Zhu, et al., Direct determination of melamine in dairy products by gas chromatography/mass spectrometry with coupled column separation, Anal. Chim. Acta, 2009, 650(1), 39–43 CrossRef CAS PubMed.
- G. Fang, Y. Wu and X. Dong, et al., Simultaneous determination of banned acid orange dyes and basic orange dyes in foodstuffs by liquid chromatography-tandem electrospray ionization mass spectrometry via negative/positive ion switching mode, J. Agric. Food Chem., 2013, 61(16), 3834–3841 CrossRef CAS PubMed.
- M. Snehalatha, C. Ravikumar and I. Hubert Joe, et al., Spectroscopic analysis and DFT calculations of a food additive carmoisine, Spectrochim. Acta, Part A, 2009, 72(3), 654–662 CrossRef CAS PubMed.
- F. Zeng, W. Duan and B. Zhu, et al., Paper-Based Versatile Surface-Enhanced Raman Spectroscopy Chip with Smartphone-Based Raman Analyzer for Point-of-Care Application, Anal. Chem., 2019, 91(1), 1064–1070 CrossRef CAS PubMed.
- K. Ponlakhet, K. Phooplub and N. Phongsanam, et al., Smartphone-based portable fluorescence sensor with gold nanoparticle mediation for selective detection of nitrite ions, Food Chem., 2022, 384, 132478 CrossRef CAS PubMed.
- H. A. H. Refat, A. I. Hassan, Y. F. Hassan and M. M. El-Wekil, Colorimetric and fluorometric nanoprobe for selective and sensitive recognition of hazardous colorant indigo carmine in beverages based on ion pairing with nitrogen doped carbon dots, Food Chem., 2021, 349, 129160 CrossRef PubMed.
- A. A. Dudkina, T. N. Volgina and N. V. Saranchina, et al., Colorimetric determination of food colourants using solid phase extraction into polymethacrylate matrix, Talanta, 2019, 202, 186–189 CrossRef CAS PubMed.
- S. Wang, Y. Ding and L. Zhang, et al., Combination of colorimetry, inner filter effect-induced fluorometry and smartphone-based digital image analysis: A versatile and reliable strategy for multi-mode visualization of food dyes, J. Hazard. Mater., 2023, 445, 130563 CrossRef CAS PubMed.
- S. Wang, L. Zhang and Q. Jin, et al., Filter paper-based colorimetric analysis: An instrument-free strategy for semiquantitative naked-eye detection of food colorants, Food Chem., 2022, 390, 133087 CrossRef CAS PubMed.
- S. Wang, H. Wang and Z. Yuan, et al., Colorimetry Combined with Inner Filter Effect-Based Fluorometry: A Versatile and Robust Strategy for Multimode Visualization of Food Dyes, ACS Appl. Mater. Interfaces, 2022, 14(51), 57251–57264 CrossRef CAS PubMed.
- S. Wang, H. Wang and Y. Ding, et al., Filter Paper- and Smartphone-Based Point-of-Care Tests for Rapid and Reliable Detection of Artificial Food Colorants, Microchem. J., 2022, 183, 108088 CrossRef CAS.
- J. Langer, D. Jimenez de Aberasturi and J. Aizpurua, et al., Present and future of surface-enhanced Raman scattering, ACS Nano, 2020, 14(1), 28–117 CrossRef CAS PubMed.
- C. Zong, M. Xu and L. J. Xu, et al., Surface-enhanced Raman spectroscopy for bioanalysis: Reliability and challenges, Chem. Rev., 2018, 118(10), 4946–4980 CrossRef CAS PubMed.
- X. Yu, E. Y. Hayden and P. Wang, et al., Ultrasensitive amyloid β-protein quantification with high dynamic range using a hybrid graphene-gold surface-enhanced Raman spectroscopy platform, J. Raman Spectrosc., 2020, 51(3), 432–441 CrossRef CAS PubMed.
- A. Nilghaz, S. Mahdi Mousavi and A. Amiri, et al., Surface-enhanced Raman spectroscopy substrates for food safety and quality analysis, J. Agric. Food Chem., 2022, 70(18), 5463–5476 CrossRef CAS PubMed.
- Y. Ou, L. Pei and K. Lai, et al., Rapid analysis of multiple sudan dyes in chili flakes using surface-enhanced Raman spectroscopy coupled with Au–Ag core-shell nanospheres, Food Anal. Methods, 2017, 10(3), 565–574 CrossRef.
- P. C. Lee and D. Meisel, Adsorption and surface-enhanced Raman of dyes on silver and gold sols, J. Phys. Chem., 1982, 86(17), 3391–3395 CrossRef CAS.
- W. Zhan, Y. Shu and Y. Sheng, et al., Surfactant-assisted stabilization of Au colloids on solids for heterogeneous catalysis, Angew. Chem., Int. Ed., 2017, 56(16), 4494–4498 CrossRef CAS PubMed.
- R. G. Parr, Density functional theory, Annu. Rev. Phys. Chem., 1983, 34(1), 631–656 CrossRef CAS.
- M. Prochazka, Basics of surface-enhanced Raman scattering (SERS), in Surface-Enhanced Raman Spectroscopy: Bioanalytical, Biomolecular and Medical Applications, ed. M. Prochazka, Springer International Publishing, Cham, 2016, pp. 21–59 Search PubMed.
- J. Krajczewski, M. Kędziora, K. Kołątaj and A. Kudelski, Improved synthesis of concave cubic gold nanoparticles and their applications for Raman analysis of surfaces, RSC Adv., 2019, 9(32), 18609–18618 RSC.
- Y. Fang, N.-H. Seong and D. D. Dlott, Measurement of the distribution of site enhancements in surface-enhanced Raman scattering, Science, 2008, 321(5887), 388–392 CrossRef CAS PubMed.
- L. Zhang, Y. J. Weng and X. Liu, et al., Fe(III) Mixed IP6@Au NPs with Enhanced SERS Activity for Detection of 4-ATP, Sci. Rep., 2020, 10(1), 5752 CrossRef CAS PubMed.
- H. V. Bandarenka, K. V. Girel and V. P. Bondarenko, et al., Formation Regularities of Plasmonic Silver Nanostructures on Porous Silicon for Effective Surface-Enhanced Raman Scattering, Nanoscale Res. Lett., 2016, 11(1), 262 CrossRef PubMed.
- C. Zhang, K. Liu, M. Xu and J. Yao, Theoretical simulation on SERS effect of au nanoparticles dimer in different medias (in Chinese), Spectrosc. Spectral Anal., 2018, 38(10), 187–188 Search PubMed.
- S. S. Andoh, T. Nuutinen, C. Mingle and M. Roussey, Qualitative analysis of Sudan IV in edible palm oil, J. Eur. Opt. Soc.-Rapid Publ., 2019, 15(1), 21 CrossRef.
- B. Li, Y. Liang and Y. Hu, et al., Correction of retention time shift of components for chromatographic fingerprints of herbal medicine (in Chinese), Chin. J. Anal. Chem., 2004, 32(3), 313–316 CAS.
- Y. Liang and O. M. Kvalheim, Resolution of two-way data: theoretical background and practical problem-solving. Part 1: theoretical background and methodology, Fresenius. J. Anal. Chem., 2001, 370(6), 694–704 CrossRef CAS PubMed.
- A. Shrivastava, Methods for the determination of limit of detection and limit of quantitation of the analytical methods, Chron. Young Sci., 2011, 2, 21–25 CrossRef.
|
This journal is © The Royal Society of Chemistry 2023 |