DOI:
10.1039/D2MO00325B
(Research Article)
Mol. Omics, 2023,
19, 668-679
Protein network analysis links the NSL complex to Parkinson’s disease via mitochondrial and nuclear biology†
Received
25th November 2022
, Accepted 14th June 2023
First published on 14th June 2023
Abstract
Whilst the majority of Parkinson’s Disease (PD) cases are sporadic, much of our understanding of the pathophysiological basis of the disease can be traced back to the study of rare, monogenic forms of PD. In the past decade, the availability of genome-wide association studies (GWAS) has facilitated a shift in focus, toward identifying common risk variants conferring increased risk of developing PD across the population. A recent mitophagy screening assay of GWAS candidates has functionally implicated the non-specific lethal (NSL) complex in the regulation of PINK1-mitophagy. Here, a bioinformatics approach has been taken to investigate the proteome of the NSL complex, to unpick its relevance to PD pathogenesis. The NSL interactome has been built, using 3 online tools: PINOT, HIPPIE and MIST, to mine curated, literature-derived protein–protein interaction (PPI) data. We built (i) the ‘mitochondrial’ NSL interactome exploring its relevance to PD genetics and (ii) the PD-oriented NSL interactome to uncover biological pathways underpinning the NSL/PD association. In this study, we find the mitochondrial NSL interactome to be significantly enriched for the protein products of PD-associated genes, including the Mendelian PD genes LRRK2 and VPS35. In addition, we find nuclear processes to be amongst those most significantly enriched within the PD-associated NSL interactome. These findings strengthen the role of the NSL complex in sporadic and familial PD, mediated by both its mitochondrial and nuclear functions.
Introduction
Parkinson’s disease (PD) is the most common movement disorder of old age (>65 years).1 Furthermore, global prevalence predictions suggest that the number of affected individuals more than doubled in 25 years, with an estimated 6.1 million people living with PD in 2016.2
The movement aspects of PD are triggered by the progressive degeneration of neurons within the Substantia Nigra pars compacta (SNpc). The consequent depletion of dopamine (DA) within nigro-striatal circuits gives origin to the debilitating triad of PD clinical symptoms: rigidity, asymmetric resting tremor, and bradykinesia. Pathologically, neuronal loss is paired with the deposition of α-synuclein aggregates in intracellular inclusions called Lewy bodies.3 The progression of PD is complex, with involvement of additional brain areas whose degeneration is responsible for the clinical manifestation of additional non-motor symptoms.4 PD has a heterogenous presentation and the interindividual differences in disease onset and progression, typical of complex disorders, hint at the existence of a personal burden of risk, a mix of genetic susceptibility factors and environmental exposures which differ on a case-to-case basis.4 Potentiation of DA signalling is the only available therapeutic intervention, achieved via DA replacement and/or by inhibition of DA catabolism and re-uptake at the synapse. However, this is a symptomatic intervention that does not halt neurodegeneration.5 As such, precise delineation of the molecular mechanisms of PD neurodegeneration is critical, to implicate novel research avenues for the development of disease-modifying treatments.
A minority of PD cases are familial (fPD), caused by highly penetrant mutations that segregate with the disease. The study of monogenic forms of PD has facilitated the elucidation of common cellular phenotypes, and the molecular patterns underpinning them. Functional delineation of PINK1 and PRKN genes, for which loss-of-function mutations are causal for autosomal recessive (AR) PD, has implicated dysfunctional mitophagy as one of the key drivers of the disease.6,7 PINK1 and Parkin act in concert to promote the targeted degradation of depolarised mitochondria.8–11 Additionally, mutations within the AR PD gene DJ-1, as well as parkinsonism genes FBXO7 and VPS35, can also be functionally associated with mitochondrial quality control.12 However, LRRK2 and GBA1 (associated with late onset forms of PD) have been linked to the dysregulation of autophagy and the endolysosmal pathway.13,14 A comprehensive understanding of the relationship between genes and phenotypes in fPD has implicated biological processes that could be relevant for the disease. However, as monogenic forms of PD represent approximately 15% of all cases,15 the question arises as to how appropriately we can model PD in the absence of a comprehensive understanding of the molecular processes underpinning sporadic disease (sPD).
To this end, data from genome-wide association studies (GWAS) provide an unbiased approach to investigate common genetic variations and disease risk across the population. To date, the identification of 90 independent genetic risk signals has supported the existence of a genetic architecture, conferring an increased personal risk of developing sPD.16 However, GWAS pinpoint risk loci, rather than specific genes, so the molecular pathways behind this genetic architecture remain elusive. Translation of genetic data to the precise delineation of relevant molecular pathways represents a rate limiting step in studies of this kind.
Recently, our group has identified, using a high-content screening assay, a functional association between KAT8 (otherwise known as a MOF), a MYST family lysine acetyltransferase, and PINK1-mitophagy.17 The KAT8 gene is located at the 16q 11.2 PD risk locus, near one of the genome wide significant PD risk signals. KAT8 is one of the nine components (HCFC1, KANSL1, KANSL2, KANSL3, KAT8, MCRS1, OGT, PHF20, and WDR5) of the non-specific lethal (NSL) complex. While a nuclear role for the NSL complex has been well defined,18,19 a study by Chatterjee et al. suggests the partial localisation of the complex to the mitochondria.20 Indeed, knock down (KD) of NSL members KANSL1, KANSL2, KANSL3 and MCRS1 has also been shown to impede PINK1-dependent induction of mitophagy.17 Interestingly, the KANSL1 gene is an additional PD-GWAS hit,21 located at the inversion polymorphism on chromosome 17q21, alongside MAPT. Up to 25% of individuals of European descent inherit, within this region, a sequence of ∼1 Mb, in the opposite orientation.22,23 This induces a ∼1.3–1.6 Mb region of linkage disequilibrium (LD), preventing recombination. Thus, haplotype-specific polymorphisms have resulted in the emergence of two major haplotype clades, H1 (the most common) and H2, of which H1 has been strongly linked to neurodegenerative diseases, including PD.24–26 While MAPT, encoding the tau protein, is frequently attributed to the PD risk association at this locus,27 evidence has accrued supporting the risk contribution of KANSL1 at this locus. In summary, there is strong evidence that functional alterations of the members of the NSL complex might underpin the risk signal for sPD at these two loci. These findings provide functional evidence for the importance of mitophagy and mitochondrial quality control in sporadic forms of disease, in addition to fPD. To gain a greater insight into the functional links between the NSL complex and its nuclear functions, mitophagy and PD, we have constructed an in silico protein–protein interaction (PPI) model of the NSL complex, describing its relationship with the mitochondrial proteome in the context of the PD genetic landscape.
Our analysis reveals that while the intersection between the mitochondrial proteome and the NSL interactome is enriched with PD genes, nuclear processes are also highly represented in the functional enrichment of the PD-associated NSL interactors. We therefore propose that alteration in both transcriptional and mitochondrial activities of the NSL complex is associated with the causal events in sPD.
Materials and methods
The methods for the in silico analysis of the NSL complex in PD and mitochondria are detailed in protocols. io: dx.doi.org/10.17504/protocols.io.5qpvorb19v4o/v3.
Nomenclature
A layered approach has been taken to build the NSL protein network (NSL-PN), whereby members of the NSL complex are designated as seeds to derive a list of proteins for which a physical interaction has been experimentally determined. To achieve this, interactors of NSL complex members, termed as protein–protein interactors (PPIs), have been downloaded, filtered, and prioritised.
Herein, the NSL complex members are designated as ‘NSL-seeds’. NSL-seeds along with the first layer interactors will be termed as the ‘first layer’. The ‘second layer’ constitutes direct interactors of the first layer members, proteins that are linked to the NSL-seeds via a “bridge”, which is a protein in the first layer. The “interactome” of an individual seed comprises the seed under investigation, with the direct interactors (in the first layer), plus the indirect interactors (in the second layer).
NSL-seeds, plus the first and second layers, comprise the complete ‘NSL-PN’. Within this analysis, we have taken two approaches to generate the complete NSL-PN: generating a ‘Mito-CORE network’, and a ‘PD-CORE network’ (outlined below). PD-associated first layer members will be termed as ‘PD-seeds’ and mitochondrial first layer members were termed as ‘Mito-seeds’. The pipeline for building each layer of the network has been described hereafter.
Downloading the protein–protein interaction (PPI) data
The pipeline to derive the first layer interactome is displayed in Fig. 1. PPIs for NSL-seeds (ESI,† Table S1; DOI: 10.5281/zenodo.7516685) were collected using 3 different web-based tools: PINOT v1.1 (Protein Interaction Network Online Tool; https://www.reading.ac.uk/bioinf/PINOT/PINOT_form.html [downloaded September 2021 using the lenient filter option]),28 HIPPIE with no threshold on the interaction score (Human Integrated Protein–Protein Interaction Reference; RRID:SCR_014651; https://cbdm-01.zdv.uni-mainz.de/~mschaefer/hippie/network.php; DOI: 10.1371/journal.pone.0031826 [downloaded September 2021])29 and MIST v5.0 (Molecular Interaction Search Tool; https://fgr.hms.harvard.edu/MIST [downloaded September 2021]).30 Each resource permitted the interrogation of a selection of IMEx consortium associated, PPI primary repositories, to obtain the literature-derived, curated PPI data.
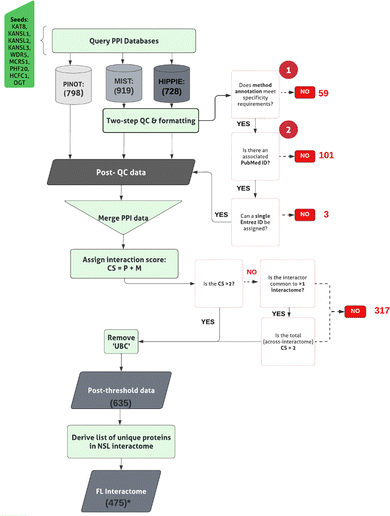 |
| Fig. 1 W-PPI-NA pipeline. Generating the first layer interactome of the NSL complex. The ‘Seeds’ are the nine members of the NSL complex. Circled numbers (1 and 2) indicate the two stages of quality control (QC) applied. Numbers provided in brackets indicate the total number of interactions/interactors retained at each stage. *first layer interactors–NSL-seeds. | |
The PPI data obtained using MIST and HIPPIE have been subjected to quality control (QC), already integrated within the PINOT pipeline, to remove low quality data. Entries lacking ‘interaction detection method’ annotation (QC1) or a PubMed ID (QC2) have been removed. Formatting between the output files has been standardized and interactors’ IDs converted to the approved EntrezID, UniprotID and HGNC gene name. Proteins with nonunivocal conversions to these 3 identifiers were removed.
Where ‘UBC’, a ubiquitin moiety, was identified as an interactor within the first layer, we manually reviewed the supporting publication. Ubiquitin is understood to be conjugated to proteins as a marker for degradation. As such, these were considered as potentially introducing non-specific protein interactions into the analysis. UBC was removed from the first layer interactomes of OGT and WDR5, since both interactions have been identified via high throughput, as opposed to specific, methods.
Merging and thresholding the PPIs
The PPI data from PINOT, HIPPIE and MIST were merged. Prior to merging, for each interaction, we identified the number of times the interaction was observed via a unique methodological technique. To identify unique interaction detection methods, it was first necessary to apply the PINOT method grouping to the interactions downloaded from HIPPIE and MIST. To do so, the ‘method conversion table’ was downloaded (https://www.reading.ac.uk/bioinf/PINOT/PINOT_help.html#select), and interactions were assigned a method annotation according to their corresponding method code (MI code) (ESI,† Table S2; DOI: 10.5281/zenodo.7516685). This is a stringent approach that allows grouping technically equivalent, but semantically different, methods. After combining interactions from PINOT, MIST and HIPPIE, a confidence score (CS) was assigned to each of the interactions according to the total number of single publications (P) and unique interaction detection methods (M, after method grouping) used for its annotation. For each interaction the CS was calculated as follows:
A score threshold (CS > 2) was then applied to filter and remove lower confidence PPI data lacking reproducibility. For an interaction to have a CS of 3, it must be replicated via either two methods or two publications.
Interactions that failed to meet the threshold were interrogated further. In particular, excluded interactors that presented in more than 1 NSL protein interactome were salvaged, considering that they might not have been replicated for a specific NSL-seed but they were replicated across NSL-seeds, thus effectively considering the NSL complex as a single unit.
Generating the Mito-CORE network
The pipeline for steps taken to derive the Mito-CORE network can be found in Fig. 2. First, we prioritised members of the first layer with mitochondrial annotation (-OGT, since it was a seed to derive the first layer interactome), which we termed ‘Mito-seeds’. Proteins with mitochondrial annotation were obtained via 2 independent inventories:
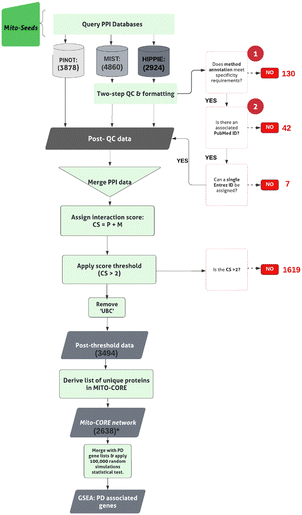 |
| Fig. 2 W-PPI-NA pipeline. Building the ‘Mito-CORE network’, and application of PD gene-set enrichment analysis (GSEA). ‘Mito-seeds’ refers to the mitochondrial first layer members of the NSL interactome. Circled numbers (1 and 2) indicate the two stages of quality control (QC) applied. Numbers provided in brackets indicate the total number of interactions/interactors retained at each stage. *Mito-seeds + second layer interactors (-NSL-seeds). | |
(i) the AmiGO2 encyclopedia31,32 (RRID:SCR_002143) was queried (February 2022), to derive experimentally determined mitochondrial protein lists. Two accession terms were used: GO:0005759, to obtain proteins annotated to the ‘mitochondrial matrix’ and GO:0031966 for proteins annotated to the ‘mitochondrial membrane’; in both cases, ‘Homo sapiens’ was specified as the search organism. (ii) The Human MitoCarta3.0 dataset33 (RRID:SCR_018165) was downloaded (October 2021) to retrieve proteins for which a mitochondrial targeting sequence (MTS) has been identified. Interactors’ IDs were converted to the approved EntrezID, UniprotID and HGNC gene name. Proteins with nonunivocal conversions to these 3 identifiers were removed.
Mito-seeds were input into all three PPI tools to obtain the second layer (downloaded November 2021). The NSL-seeds together with the Mito-seeds and second layer interactors formed the complete Mito-CORE network.
Gene set enrichment analysis (GSEA)
GSEA for PD-associated genes was conducted, by comparing the members of the interactome under investigation to a list of 180 unique PD-associated genes generated by consulting 3 publicly accessible resources: (i) PanelApp v1.68 diagnostic grade genes (green annotations) for PD and parkinsonism34 (Gene Panel: Parkinson’s Disease and Complex Parkinsonism(Version 1.108); https://panelapp.genomicsengland.co.uk/panels/39/ [downloaded October 2021]) and (ii) the latest GWAS meta-analysis16 and (iii) a list of 15 genes associated with Mendelian PD, obtained from the recent W-PPI-NA.35 The genes from i, ii, and iii were combined to generate a PD-associated gene list, herein referred to as the ‘PD genes list’ (ESI,† Table S3; DOI: 10.5281/zenodo.7516685). The genes within this list have been referred to by the name of their protein product.
Statistical evaluation via random network simulation
To test the significance of gene set overlaps, 100
000 random simulations were carried out and used to validate the statistical significance of overlaps of PD genes with the first layer and the complete Mito-CORE network. 100
000 random gene lists, each of them equivalent in length to the first layer/complete Mito-CORE network, were obtained using the R random sampling function, from a list of 19
947 genes. Each list was compared to the PD gene list, keeping track of the matches. The p value has been calculated using the p-norm function in R.
Generating the PD-CORE network
The pipeline is reported in Fig. 3. The genes in the intersection between the first layer and the list of 180 unique PD genes were used as PD-seeds. They were input into PINOT to obtain the second layer interactome (downloaded May 2023). An arbitrary confidence threshold has been applied, retaining data with a PINOT assigned score of >2. This step has eliminated data with just a single publication and method from the downstream analysis. Once again interactors’ IDs were converted to the approved EntrezID, UniprotID and HGNC gene name. Proteins with nonunivocal conversions to these 3 identifiers were removed. To remove background noise, only members of the second layer bridging >1 PD-seed within the PD-CORE network were retained. Protein interactors that were private to 1 PD-seed only were removed, and this resulted in the removal of WDR5B from the PD-CORE network. The NSL-seeds together with the PD-seeds and the non-private second layer interactors have thus formed the complete PD-CORE network.
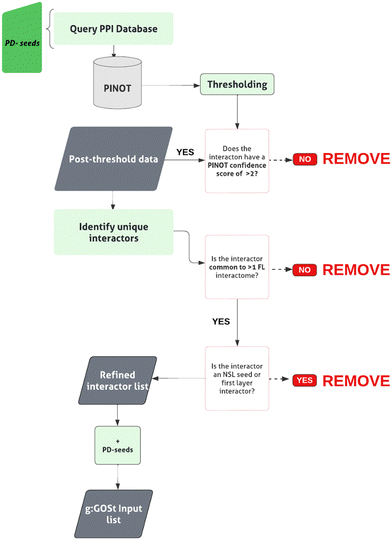 |
| Fig. 3 W-PPI-NA pipeline. The ‘PD-seeds’ refers to the PD-associated first layer members. Numbers provided in brackets indicate the total number of interactions/interactors retained at each stage. | |
Functional enrichment analysis
To assess the enrichment of particular biological processes within the PD-CORE network, members (-NSL-seeds), were input into the g:Profiler search tool31,32 (g:GOSt; RRID:SCR_006809; https://biit.cs.ut.ee/gprofiler/ [downloaded January 2022]). Enrichment for GO terms associated with ‘biological processes (BPs)’ only, was conducted, generating a list of enriched GO:BP terms.
A threshold was applied to the list of enriched GO:BP terms to retain those with a term size of <100 thus effectively removing ‘broad’ GO:BP terms. Remaining terms were assigned to custom-made ‘semantic classes’ (SC), accompanied by a parent ‘functional group’ (FG). Generic terms (classified in the semantic classes of: RNA metabolism, general, metabolism, enzyme, DNA metabolism, transport and response to stimulus) were discarded from further analysis. The pipeline for this analysis is presented in the ESI,† Fig. S1. GO:BP terms contributing to each SC were pooled to identify the list of proteins within the network contributing to the enrichment of this specific SC.
Software and scripts
Where data have been parsed in Rstudio (version 1.3.1093; RRID:SCR_000432; https://www.rstudio.com/), the script can be obtained at 10.5281/zenodo.7875447 within the relevant project files. Additional software programs used are Excel (version 16.6; RRID:SCR_016137; https://www.microsoft.com/en-gb/) and Cytoscape (version 3.8.2; RRID:SCR_003032; https://cytoscape.org/).36
Results
Construction of the NSL-PN: first layer
In order to construct the first layer of the NSL protein network (NSL-PN), the nine members of the NSL complex served as seeds to query three separate tools: PINOT, MIST and HIPPIE, obtaining a set of direct interactors of the NSL complex. Three tools were consulted at this stage to maximize the capture of PPI data available within the literature. Search from PINOT, MIST and HIPPIE yielded a total of 798, 919 and 728 direct interactions, respectively. To pool the data between the three search tools, differences in formatting and protein identification nomenclature needed to be standardized. Similarly, it was necessary to apply quality control steps excluding data without a publication ID and/or an associated interaction detection method. A summary of the excluded and retained data is found in Table 1. Once the data collected from PINOT, MIST and HIPPIE was pooled, 947 interactions were observed across the 9 NSL interactomes. Each single seed-interactome contained the following number of interactors: KAT8: 45, KANSL1: 56, KANSL2: 23, KANSL3: 18, PHF20: 23, WDR5: 256, MCRS1: 184, OGT: 181, and HCFC1: 161.
Table 1 ‘NSL-seeds’ with the corresponding UniProt ID used to interrogate three separate protein–protein interaction (PPI) tools (PINOT, MIST and HIPPIE). Columns contain PPI counts; column headings correspond to the stage of the analysis. QC1 = quality control step 1; step to remove data lacking ‘method annotation’. QC2 = quality control step 2; step to remove data lacking PubMed ID. Here, column ‘-UBC’ corresponds to the total PPI count after the removal of this ubiquitin moiety from the final interactor list
NSL- seed |
UniProt ID |
PPIs downloaded (P, H, M) |
Removed: QC1 |
Removed: QC2 |
Removed: un-resolved multi-entrez ID |
Unique PPIs [post QC and formatting] |
Post-threshold PPIs |
-UBC |
KAT8 |
Q9H7Z6 |
38, 42, 49 |
7 |
0 |
1 |
45 |
41 |
41 |
KANSL1 |
Q7Z3B3 |
49, 48, 54 |
2 |
0 |
0 |
56 |
35 |
35 |
KANSL2 |
Q9H9L4 |
20, 13, 22 |
1 |
0 |
0 |
23 |
14 |
14 |
KANSL3 |
Q9P2N6 |
13, 13, 18 |
1 |
0 |
0 |
18 |
11 |
11 |
PHF20 |
Q9BVI0 |
16, 16, 23 |
3 |
0 |
1 |
23 |
20 |
20 |
WDR5 |
P61964 |
230, 226, 249 |
18 |
9 |
1 |
256 |
201 |
200 |
MCRS1 |
Q96EZ8 |
177, 83, 177 |
0 |
89 |
0 |
184 |
74 |
74 |
OGT |
O15294 |
140, 153, 175 |
14 |
3 |
0 |
181 |
133 |
132 |
HCFC1 |
P51610 |
115, 134, 152 |
13 |
0 |
0 |
161 |
108 |
108 |
Steps were then taken to refine each interactome to remove non-replicated data. To obtain a ‘confidence score’ (CS) for each interaction, we counted the number of ‘observations’ associated with each interaction, defined as the number of publications (P) or unique methods (M) reporting it (please refer to Materials and methods section). To remove lower confidence interactions, an arbitrary score threshold of ‘CS > 2’ was applied. An interaction with a score of 2 indicates that the interaction has been found using a single method via a single publication; thus the interaction was considered as lacking support by literature evidence. A concessionary threshold was applied to those interactors that did not meet this threshold with an individual seed but did so with the NSL complex (between the 9 seeds). This provided a means to prioritise interactors associated with more than one NSL complex member; interactions that could be important for delineating a function of the NSL complex, rather than its constituent members. Following the refinement of the data, ∼67% of interactions were retained across all interactomes (ESI,† Fig. S2).
To the post-threshold total, each single seed interactome contributed to the following number of interactors: KAT8: 41, KANSL1: 35, KANSL2: 14, KANSL3: 11, PHF20: 20, WDR5: 200, MCRS1: 74, OGT: 132, and HCFC1: 108 (ESI,† Table S1; DOI: 10.5281/zenodo.7516685). Of the complete interactor list, ∼87% were captured by all three search tools (ESI,† Fig. S3). Merging the nine interactomes generated the first layer of the NSL-PN, represented by 475 single nodes (i.e., unique interactors) and 635 undirected edges (i.e., unique interactions). None of the nine interactomes within the first layer were isolated, and all were connected in the same unique graph, supporting the functional association of all seeds as part of the NSL complex.
The first layer interactome is enriched for PD-associated genes
We sought to assess whether there was an enrichment of proteins associated with PD in the first layer by overlapping 475 first layer members with the list of 180 PD genes (ESI,† Table S3; DOI: 10.5281/zenodo.7516685). Indeed, an intersection of 14 proteins between the first layer and the list of PD genes was found (p value = 9.4 × 10−7). Out of the 14 genes linked to PD represented within the first layer, the protein products of LRRK2 and VPS35, in which mutations cause autosomal dominant (AD) forms of PD, were found to be direct interactors of the NSL complex. Taken together, these findings strengthen the existence of a functional association between the NSL complex and the disease mechanisms that underpin PD.
Construction of the NSL-PN: second layer
In order to minimise the bias derived from the use of NSL complex members as seeds (i.e., seed centrality bias), a multi-layered NSL-PN was built (i.e., first layer plus second layer interactors). Two different approaches were taken to prioritise members of the first layer and to generate an expansion of the network to the second layer of protein interactions. First, a ‘mitochondrial’ NSL interactome was built, referred to as the Mito-CORE network, to explore the relevance of the NSL mitochondrial interactome to PD. Secondly, a ‘PD-oriented’ NSL interactome was built, referred to as the PD-CORE network, to uncover biological pathways associated with the portion of the NSL complex network that is relevant for PD.
The Mito-CORE network is enriched for PD-associated genes
To establish the Mito-CORE network, members of the first layer interactome were prioritised based on mitochondrial annotation; the pipeline for building this network is shown in Fig. 2. A list of 1346 unique ‘mitochondrial proteins’ were derived from two independent inventories: the AmiGO2 encyclopaedia and the Human MitoCarta3.0 dataset (ESI,† Table S4; DOI: 10.5281/zenodo.7516685). Overlapping this list of 1346 proteins with the components of the first layer NSL interactome revealed an intersection of 17 proteins (Fig. 4(A)). A list of 16 (upon exclusion of OGT as this mitochondrial protein is an NSL- seed) mitochondrial proteins within the first layer were used as ‘Mito-seeds’ and represented the starting point to download the second layer interactors using PINOT, MIST and HIPPIE (a summary of the downloaded data is shown in Table 2). The resultant Mito-CORE network contained 7 out of 9 members of the NSL complex (KANSL2 and KANSL3 were missing) and held 2644 single nodes (unique first and second layer interactors + NSL-seeds) and 3511 undirected edges (unique interactions within the entire network) (second layer interactions of the Mito-CORE network can be found in the ESI,† Table S5; DOI: 10.5281/zenodo.7516685). We next sought to assess whether an enrichment of genes linked to PD was upheld in the complete Mito-CORE network. Notably, there were 40 overlaps between the PD gene list and the complete Mito-CORE network, accounting for 22% of the complete PD gene list being represented (Fig. 4(B)). Thus, there was a significant enrichment of proteins encoded by PD-associated genes within the mitochondrial interactome of the NSL complex (p value = 0.0002; ESI,† Fig. S4) (the complete list of overlaps reported in the ESI,† Table S6; DOI: 10.5281/zenodo.7516685). Moreover, 6/15 genes from the more ‘stringent’ Mendelian PD gene list were represented in the Mito-CORE network: PRKN, SNCA, PRKRA, PARK7, VPS35 and LRRK2 (Fig. 4(B)), an overlap which meets the statistical significance (p value = 0.001; ESI,† Fig. S5). Considering these results, we propose that the mitochondrial interactome of the NSL complex might indeed represent one of the functional links between the NSL complex and PD.
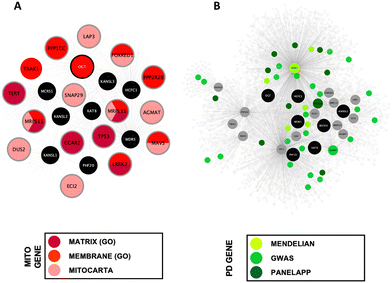 |
| Fig. 4 Building the ‘Mito-CORE network’ (A) 16 members of the first layer interactome were prioritised as ‘Mito-seeds’ for the derivation of the second layer (after the exclusion of OGT as an NSL-seed). Nodes are colour coded according to the repository reporting mitochondrial localisation. (B) The Mito-CORE network was subjected to PD gene set enrichment analysis (GSEA) to reveal the statistically significant enrichment of PD risk genes (40/180; 22%) (p value = 0.0002). We also found 6 out of a stringent list of 15 genes associated with Mendelian PD, represented within the Mito-CORE network: PRKN, SNCA, PRKRA, PARK7, VPS35 and LRRK2, an enrichment which meets the statistical significance (p value = 0.001). | |
Table 2 Mito-seeds with the corresponding UniProt ID used to interrogate three separate PPI tools (PINOT, MIST and HIPPIE) to generate the second layer of the complete Mito-CORE network. Columns contain data counts; column headings indicate the corresponding stage of the analysis. QC1 = quality control step 1; step to remove data lacking ‘method annotation’. QC2 = quality control step 2; step to remove data lacking a PubMed ID. Here, column ‘-UBC’ corresponds to the total PPI count after removal of this ubiquitin moiety from the final interactor list
Mito-seed |
UniProt ID |
PPIs downloaded (P, H, M) |
Removed: QC1 |
Removed: QC2 |
Removed: un-resolved multi-entrez ID |
Unique PPIs [post QC and formatting] |
Post-threshold PPIs |
MRPS15 |
P82914 |
70, 66, 77 |
1 |
0 |
0 |
82 |
71 |
MRPL11 |
Q9Y3B7 |
95, 83, 106 |
30 |
16 |
1 |
110 |
75 |
FOXRED1 |
Q96CU9 |
28, 26, 51 |
0 |
0 |
0 |
52 |
24 |
MAVS |
Q7Z434 |
108, 121, 142 |
23 |
0 |
0 |
150 |
119 |
SNAP29 |
O95721 |
95, 91, 108 |
11 |
8 |
0 |
112 |
72 |
ECI2 |
O75521 |
81, 89, 100 |
8 |
1 |
0 |
100 |
80 |
LAP3 |
P28838 |
46, 40, 80 |
7 |
9 |
0 |
84 |
38 |
DUS2 |
Q9NX74 |
4, 6, 7 |
0 |
0 |
0 |
7 |
4 |
AGMAT |
Q9BSE5 |
22, 24, 27 |
9 |
0 |
0 |
27 |
24 |
LRRK2 |
Q5S007 |
1439, 548, 1850 |
5 |
1 |
1 |
1861 |
1326 |
PPP1CC |
P36873 |
345, 371, 651 |
5 |
3 |
2 |
660 |
324 |
PPP2R2B |
Q00005 |
191, 198, 201 |
2 |
0 |
1 |
206 |
113 |
TRAK1 |
Q9UPV9 |
17, 22, 21 |
3 |
0 |
0 |
23 |
17 |
TERT |
O14746 |
73, 108, 114 |
1 |
1 |
0 |
119 |
49 |
TP53 |
Q96S44 |
1101, 1073, 1190 |
15 |
3 |
2 |
1317 |
1005 |
CCAR2 |
Q8N163 |
163, 166, 189 |
10 |
0 |
0 |
205 |
154 |
The PD-CORE network is enriched for mitochondrial processes
We next expanded the PD-CORE downloading the protein interactions of the 14 PD linked proteins directly interacting with the NSL-seeds thus generating the PD-CORE network. The pipeline for building this network is illustrated in Fig. 3. The input of the PD-associated first layer members into PINOT followed by QC (a summary of the retained data is shown in Table 3) initially returned a total of 4843 protein interactions, of which 815 were retained following the application of the CS threshold (ESI,† Table S7; DOI: 10.5281/zenodo.7516685). The PD-CORE network at this first stage was therefore composed of the NSL-seeds directly connecting to PD proteins and the direct interactors of the PD proteins. We intended to identify the more densely connected unit of this network and therefore we removed the second layer interactors that were unique to a single PD protein and did not generate connectivity within the PD-CORE network. To do so, we removed the second layer nodes connected to <2 first layer interactors; thus, keeping those proteins in the second layer that were able to bind multiple PD-associated proteins of the first layer (ESI,† Table S8; DOI: 10.5281/zenodo.7516685). As expected, this step increased the connectivity of the network, removing second layer members which might represent a ‘background noise’. The final PD-CORE network contained 88 nodes comprising 6 out of 9 of the NSL-seeds, 13 out of 14 of the PD-associated first layer members, and their interactions in the second layer (communal to more than 1 PD proteins in the first layer) (Fig. 5).
Table 3
PD-seeds with the corresponding UniProt ID; used to interrogate PINOT to generate the second layer of the ‘PD-CORE network’. ‘PD association’ column indicates the source of the association. ‘Mendelian’ refers to a list of 15 genes associated with Mendelian PD. GWAS refers to a list of PD risk genes from the latest PD GWAS meta-analysis. ‘PANELAPP’ refers to a list of diagnostic grade genes for PD and Parkinsonism. Columns contain data counts; column headings indicate the corresponding stage of the analysis. ‘Post-threshold PPIs’ correspond to those with PINOT confidence scores >2. The ‘PD-association threshold’ corresponds to the number of PPIs bridging >1 interactome within the PD-CORE network. WDR5B was removed from the PD-CORE network, once private interactors were excluded
PD-seed |
UniProt ID |
PD association (G = GWAS, P = PanelApp, W = W PPI NA) |
Total PPIs downloaded |
Post-threshold PPIs |
Post PD-association threshold |
CAMK2D |
Q13557 |
G |
179 |
20 |
4 |
CSTA |
P01040 |
G |
67 |
6 |
1 |
CYLD |
Q9NQC7 |
G |
622 |
34 |
8 |
ATN1 |
P54259 |
P |
111 |
15 |
4 |
MAPT |
P10636 |
G/P |
622 |
84 |
36 |
PPP2R2B |
Q00005 |
P |
192 |
84 |
16 |
UBTF |
P17480 |
G |
90 |
19 |
2 |
SETD1A |
O15047 |
G |
57 |
16 |
7 |
WDR5B |
Q86VZ2 |
G |
27 |
1 |
0 |
VPS35 |
Q96QK1 |
P/W |
136 |
20 |
1 |
CCAR2 |
Q8N163 |
G |
174 |
26 |
6 |
SGF29 |
Q96ES7 |
G |
114 |
53 |
3 |
LRRK2 |
Q5S007 |
G/P/W |
1448 |
215 |
51 |
HTT |
P42858 |
P |
1004 |
222 |
29 |
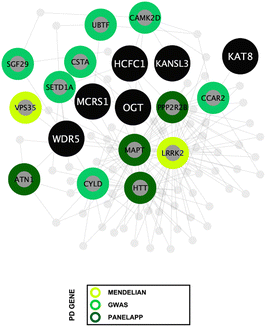 |
| Fig. 5 Generating the ‘PD-CORE network’. The PD-CORE network was derived by prioritising the 14 PD-associated members of the NSL-PN first layer. The second layer was obtained to generate the PD-CORE network. Subsequent refinement of the network, removing ‘private’ interactors, generated the refined network, composed of 13 first layer interactors (PD-seed WDR5B was removed) and 69 second layer interactors of seeds: KAT8, KANSL3, OGT, WDR5, HCFC1, MCRS1 (black nodes). ‘Mendelian’ refers to a list of 15 genes associated with Mendelian PD. GWAS refers to a list of PD risk genes from the latest PD GWAS meta-analysis. ‘PANELAPP’ refers to a list of diagnostic grade genes for PD and AParkinsonism. | |
To determine enriched biological processes within the PD-CORE network, its 82 members (88 excluding the 6 NSL-seeds) were input into the g:Profiler tool (g:GOSt). 456 GO:BP terms were returned, 56 of which were retained after subsequent refinement to remove broader (term size >100) and more general terms (detailed in the materials and methods section). Once each GO:BP had been manually assigned to a ‘functional group’ (FG) and ‘semantic class’ (SC), enrichment of 8 FGs and 20 SCs was observed (ESI,† Table S9; DOI: 10.5281/zenodo.7516685) (Fig. 6(A) and (B)). The top five most significantly enriched SCs (in terms of p value) were “protein modification”, “protein stability”, “nuclear protein localisation”, “nuclear transport” and “protein folding”. However, it is notable that the 3 SCs with the highest number of single GO:BPs allocated to them were all nuclear associated: “nuclear protein localisation”, “nuclear transport” and “chromatin metabolism” (Fig. 6(B)). Taken together, this analysis reveals that alongside processes involved in protein metabolism, nuclear processes are strongly associated with the proteins composing the PD-CORE network. The PD-CORE network was then refined, retaining only those proteins responsible for this enrichment (Fig. 7(A)), allowing the extraction of the PD – nuclear subnetwork. Topological analysis of the extracted subnetwork showed that three of the NSL-seeds contributed to much of the network with 83% (20/24) and 67% (16/24) and 58% (14/24) of the PD-CORE nodes represented by the OGT, HCFC1 and WDR5 interactomes respectively, while only 29% (7/24) and 8% (2/24) of the PD-CORE nodes are represented by the MCRS1 and KAT8 interactomes (Fig. 7(B)). Taken together, these findings point to OGT, HCFC1 and WDR5 as key drivers of the PD association with the NSL nuclear processes, at the protein level.
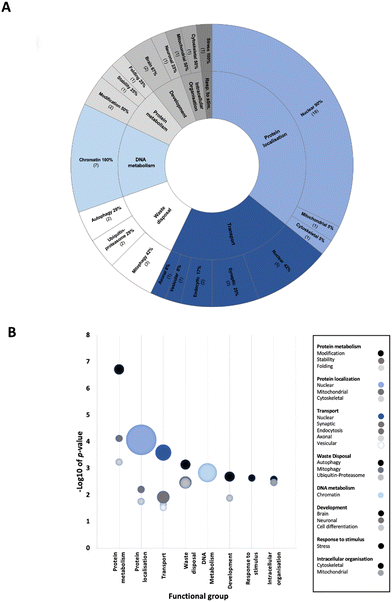 |
| Fig. 6 Nuclear processes are functionally enriched within the ‘PD-CORE network’ (A) The pie chart depicts the proportion of enriched GO terms represented by each functional group (FG) (outside ring), and each semantic class (SC), inner ring. FGs for which there is a nuclear representation amongst SCs are depicted in a shade of blue. Numbers correspond to the number of GO terms represented by each SC. ‘Resp. to stim.’ = ‘response to stimulus.’ (B) The bubble chart illustrates weighted SCs. The lowest p value of associated GO terms has been allocated to the SC. The bubble size represents the number of GO terms within the SC. | |
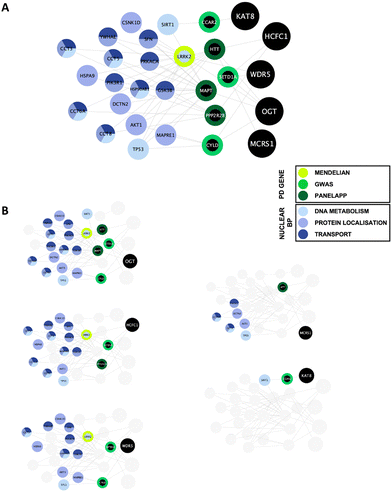 |
| Fig. 7 OGT, HCFC1 and WDR5 are possible key drivers of the PD risk associated with nuclear processes. (A) Visualisation of the refinement of the PD-CORE network, containing proteins that contribute to the enrichment of nuclear processes. The final network contains 24 nodes (FL + SL) + 5 NSL-seeds. ‘NUCLEAR BP’ refers to the ‘nuclear biological process.’ (B) Extraction and isolation of contributing interactomes demonstrates that OGT, HCFC1 and WDR5 contribute most significantly to the enrichment of nuclear processes with 83% (20/24), 67% (16/24) and 58% (14/24) of the “PD-CORE” nodes represented by their interactomes, respectively. | |
Discussion
To date, functional studies of the genetic basis for PD have focused on delineating the precise molecular underpinnings of monogenic PD. However, the genetic architecture of both familial PD and sporadic PD is complex, and an interplay between the genetic and environmental factors has been proposed to be the basis of PD aetiopathogenesis.4 While GWAS have revealed multiple genetic risk factors, these studies identify loci as opposed to specific genes, which are associated with an increase or decrease in the risk of PD development. Recently, our lab has revealed a role for several members of the NSL complex in the regulation of PINK1-mitophagy, in which two members have been proposed as genetic risk factors for sporadic PD development (Soutar et al., 2022).16 Thus, it has been suggested that the NSL complex could play a role in mitochondrial quality control mechanisms within the context of sporadic PD. However, the NSL complex has mainly been characterized in the context of the regulation of nuclear processes. To gain a deeper understanding of how the NSL complex intersects with pathways involved in PD, we re-constructed the protein interactome of the NSL complex. Our results not only demonstrated a link to other PD-associated genes at the mitochondria, but also indicated that a functional link between the NSL complex and PD might be underpinned by the nuclear functionality of the NSL complex. Thus, we here provide a computational prediction that the NSL complex serves as a link between familial PD and sporadic PD via both the mitochondrial and nuclear-associated pathways.
Protein–protein interactions (PPIs) were collected from the peer-reviewed literature to construct the NSL-PN. Initially, we used three separate tools to obtain a comprehensive list of direct interactors for each of our nine ‘NSL-seeds’, to construct the first layer interactome. PPI data have utility in guiding the interpretation of the data generated by genomics studies, to make biological sense of the data, inferring functional associations and pathways associated with disease risk. However, PPI studies are inherently limited by the ascertainment bias, since the available data reflects interactions that have been determined by hypothesis-led experimentation within the wet lab, resulting in an over-representation of interactions reported for proteins with an existing disease-association.37 Indeed, in the present study, we report 200 interactors for WDR5, meeting the confidence threshold, while only 11 interactors for KANSL3 and 14 interactors for KANSL2 were reported. The disparity in the interactome size that we have found could reflect the physiologically relevant difference in the number of cellular interactors of each protein; however, it is not possible to exclude the possibility that it might reflect a bias in the research literature. To minimise the effect of this research bias within our analysis, we have taken a multi-layered approach to build NSL-PN. Rather than looking at individual members of the interactome, we have carried out a set of analyses using the entire NSL-PN, suitable for drawing meaningful conclusions from an inherently partial data set.
In the first approach, we expanded the ‘first layer’ of the NSL-PN to obtain the ‘Mito-CORE network’ of the NSL complex. The NSL complex, with KAT8, is well characterized as a master regulator of transcription, responsible for acetylation of histone 4 at lysine 5, lysine 8 and lysine 16 in the nuclear compartment.18,38 However, a role for the NSL complex at the mitochondria has also been suggested.20 This suggestion is of interest in the context of PD, since mitochondrial quality control/dynamics have been intimately associated with familial PD.39 We therefore filtered the NSL-PN to retain only mitochondrial proteins. The identification of the mitochondrial proteins was conducted using two independent inventories that were pooled to maximise coverage. We retrieved the Human MitoCarta3.0 data set,40 a set of proteins harbouring a mitochondrial targeting sequence (MTS), along with candidates obtained from the AmiGO2 encyclopaedia,31,32 experimentally evidenced to localise to the mitochondrial matrix or the membrane. Using this approach, we found 16/475 members of the NSL-PN first layer to be localised to the mitochondria: ECI2, DUS2, PPP1CCC,PPP2R2B, MAVS, LRRK2, TP53, SNAP29, MRPL11, MRPS15, AGMAT, FOXRED1, LAP3, TRAK1, TERT, and CCAR2. While 16/475 represents a lower proportion than would be expected by chance, we suggest that the incomplete nature of PPI data alongside a research bias toward a nuclear function for the NSL complex could explain this lack of data. Nevertheless, we used these mitochondria localised proteins within the first layer of the NSL-PN to expand the network and download the ‘second layer’ of protein interactions, thus obtaining the Mito-CORE network of the NSL complex. This is the protein interaction network built around the protein interactors of the NSL complex that are suggested to localise to the mitochondria.
The Mito-CORE network of the NSL complex was significantly enriched for the protein products of 180 PD linked genes, implicating a role for the NSL complex in the genetic risk of PD, via its mitochondrial functions. There are challenges in defining PD relevant genes, and here we have opted to include a panel of PD risk candidate genes from the recent GWAS16 along with a set of diagnostic markers obtained from PanelApp v 1.68 (diagnostic grade genes for PD and Parkinsonism).34 Of course, caution must be taken in the interpretation of GWAS candidate genes, for which approaches to annotate causal genes at a given risk locus remain controversial.41 Considering these limitations, the assessment of enrichment of a more stringent list of 15 genes, associated with Mendelian PD, has been carried out. It was observed that gene set enrichment is maintained (6/15 members represented) within the Mito-CORE network. A caveat for this analysis, and for the use of PPIs in general, is the discrepancy in the total number of annotations between disease relevant and non-relevant proteins; however, our results showed an enrichment of proteins encoded by PD relevant genes within this network, reinforcing the argument for a mitochondrial role of the NSL complex bridging sporadic and familial diseases.
As a second approach, we have filtered the first layer NSL-PN to retain the protein products of 14 genes linked to PD present within the first layer of the NSL-PN (CSTA, PPP2R2B, SGF29, ATN1, VPS35, UBTF, SETD1A, CCAR2, CAMK2D, MAPT, CYLD, HTT, WDR5B and LRRK2). We used these 14 proteins to expand the network and download the second layer, thus obtaining the ‘PD-CORE network’ of the NSL complex. This is the protein interaction network built around the protein interactors of the NSL complex that are coded by genes that present a genetic association with PD.
Functional enrichment analysis of the PD-CORE network showed a high representation of nuclear biological processes amongst those significantly enriched within the network, with semantic classes (SCs) “nuclear protein localisation”, “nuclear transport” and “chromatin metabolism” represented by the highest number of GO:BPs. As a final approach, we have refined the PD-CORE network, enabling us to visualise and extract subnetworks that could provide mechanistic insight between NSL and nuclear processes. We have first identified the entities within the PD-CORE network represented by the terms “nuclear protein localisation”, “nuclear transport” and “chromatin metabolism”. We have then highlighted these within the network, using the Cytoscape (v.3.8.2) visualisation tool,36 to reveal the interactions with the NSL complex members that mediate this enrichment. The results of this final analysis point to OGT, HCFC1 and WDR5 as possible key drivers of the PD risk associated with these nuclear processes.
Taken together, this study provides further evidence for the role of the NSL complex in driving PD aetiopathogenesis. Specifically, we have illuminated a significant PD association, strengthening the role of the NSL complex in both familial and sporadic diseases. Additionally, we have shown that this PD association is underpinned, at the protein level, by a set of mitochondrial protein interactions as well as nuclear processes. This bioinformatics-led approach serves as a proof-of-principle, unbiased approach to extract biologically meaningful information from genetic findings and delineate functional predictions to guide experimental investigation to clarify the role of the NSL in PD and eventually facilitate drug discovery.
Data availability
Data are publicly available at the original repositories. The pipeline for data access and processing is available at the links provided in the document. Additional data is available in the ESI.†
Conflicts of interest
There are no conflicts of interest to declare.
Acknowledgements
HPF and PAL are funded by Aligning Science Across Parkinson’s (grant number ASAP-000478) through the Michael J. Fox Foundation for Parkinson’s Research (MJFF). KK is funded by the Masonic Charitable Foundation. PAL and CM received funding from the Biomarkers Across Neurodegenerative Diseases Grant Program 2019, BAND3 (Michael J. Fox Foundation, Alzheimer’s Association, Alzheimer’s Research UK, and the Weston Brain Institute [grant number 18063]). CM acknowledges funding from the MJFF (grant number MJFF-021335). For the purpose of open access, the author has applied a CC BY public copyright license to all Author Accepted Manuscripts arising from this submission.
References
- J. Hardy, P. Lewis, T. Revesz, A. Lees and C. Paisan-Ruiz, Curr. Opin. Genet. Dev., 2009, 19, 254–265 CrossRef CAS PubMed.
- GBD 2016 Parkinson’s Disease Collaborators, Lancet Neurol., 2018, 17, 939–953 CrossRef PubMed.
- C. Klein and A. Westenberger, Cold Spring Harbor Perspect. Med., 2012, 2, a008888 Search PubMed.
- R. Kruger, J. Klucken, D. Weiss, L. Tonges, P. Kolber, S. Unterecker, M. Lorrain, H. Baas, T. Muller and P. Riederer, J. Neural Transm., 2017, 124, 1015–1027 CrossRef PubMed.
- J. Jankovic and W. Poewe, Curr. Opin. Neurol., 2012, 25, 433–447 CrossRef CAS PubMed.
- T. Kitada, S. Asakawa, N. Hattori, H. Matsumine, Y. Yamamura, S. Minoshima, M. Yokochi, Y. Mizuno and N. Shimizu, Nature, 1998, 392, 605–608 CrossRef CAS PubMed.
- E. M. Valente, P. M. Abou-Sleiman, V. Caputo, M. M. Muqit, K. Harvey, S. Gispert, Z. Ali, D. Del Turco, A. R. Bentivoglio, D. G. Healy, A. Albanese, R. Nussbaum, R. Gonzalez-Maldonado, T. Deller, S. Salvi, P. Cortelli, W. P. Gilks, D. S. Latchman, R. J. Harvey, B. Dallapiccola, G. Auburger and N. W. Wood, Science, 2004, 304, 1158–1160 CrossRef CAS PubMed.
- D. G. Hernandez, X. Reed and A. B. Singleton, J. Neurochem., 2016, 139(Suppl 1), 59–74 CrossRef CAS PubMed.
- E. Deas, N. W. Wood and H. Plun-Favreau, Biochim. Biophys. Acta, 2011, 1813, 623–633 CrossRef CAS PubMed.
- D. Narendra, A. Tanaka, D. F. Suen and R. J. Youle, J. Cell Biol., 2008, 183, 795–803 CrossRef CAS PubMed.
- D. P. Narendra, S. M. Jin, A. Tanaka, D. F. Suen, C. A. Gautier, J. Shen, M. R. Cookson and R. J. Youle, PLoS Biol., 2010, 8, e1000298 CrossRef PubMed.
- N. Plotegher and M. R. Duchen, Front. Cell Dev. Biol., 2017, 5, 110 CrossRef PubMed.
- N. Connor-Robson, H. Booth, J. G. Martin, B. Gao, K. Li, N. Doig, J. Vowles, C. Browne, L. Klinger, P. Juhasz, C. Klein, S. A. Cowley, P. Bolam, W. Hirst and R. Wade-Martins, Neurobiol. Dis., 2019, 127, 512–526 CrossRef CAS PubMed.
- P. Gomez-Suaga, B. Luzon-Toro, D. Churamani, L. Zhang, D. Bloor-Young, S. Patel, P. G. Woodman, G. C. Churchill and S. Hilfiker, Hum. Mol. Genet., 2012, 21, 511–525 CrossRef CAS PubMed.
- J. Tran, H. Anastacio and C. Bardy, NPJ Parkinsons Dis., 2020, 6, 8 CrossRef PubMed.
- M. A. Nalls, C. Blauwendraat, C. L. Vallerga, K. Heilbron, S. Bandres-Ciga, D. Chang, M. Tan, D. A. Kia, A. J. Noyce, A. Xue, J. Bras, E. Young, R. von Coelln, J. Simon-Sanchez, C. Schulte, M. Sharma, L. Krohn, L. Pihlstrom, A. Siitonen, H. Iwaki, H. Leonard, F. Faghri, J. R. Gibbs, D. G. Hernandez, S. W. Scholz, J. A. Botia, M. Martinez, J. C. Corvol, S. Lesage, J. Jankovic, L. M. Shulman, M. Sutherland, P. Tienari, K. Majamaa, M. Toft, O. A. Andreassen, T. Bangale, A. Brice, J. Yang, Z. Gan-Or, T. Gasser, P. Heutink, J. M. Shulman, N. W. Wood, D. A. Hinds, J. A. Hardy, H. R. Morris, J. Gratten, P. M. Visscher, R. R. Graham, A. B. Singleton and T. and Me Research, C. System Genomics of Parkinson’s Disease and C. International Parkinson’s Disease Genomics, Lancet Neurol., 2019, 18, 1091–1102 CrossRef CAS PubMed.
- M. P. M. Soutar, D. Melandri, B. O’Callaghan, E. Annuario, A. E. Monaghan, N. J. Welsh, K. D’Sa, S. Guelfi, D. Zhang, A. Pittman, D. Trabzuni, A. H. A. Verboven, K. S. Pan, D. A. Kia, M. Bictash, S. Gandhi, H. Houlden, M. R. Cookson, N. N. Kasri, N. W. Wood, A. B. Singleton, J. Hardy, P. J. Whiting, C. Blauwendraat, A. J. Whitworth, C. Manzoni, M. Ryten, P. A. Lewis and H. Plun-Favreau, Brain, 2022, 145, 4349–4367 CrossRef PubMed.
- B. N. Sheikh, S. Guhathakurta and A. Akhtar, EMBO Rep., 2019, 20, e47630 CrossRef PubMed.
-
R. H. Amy, R. Regina, O. C. Ben, R. Sonia Garcia, M. Ana Luisa Gil, B. Juan, P.-F. Helene and R. Mina, bioRxiv, 2023, preprint DOI:10.1101/2023.01.16.523926.
- A. Chatterjee, J. Seyfferth, J. Lucci, R. Gilsbach, S. Preissl, L. Bottinger, C. U. Martensson, A. Panhale, T. Stehle, O. Kretz, A. H. Sahyoun, S. Avilov, S. Eimer, L. Hein, N. Pfanner, T. Becker and A. Akhtar, Cell, 2016, 167, 722–738 e723 CrossRef CAS PubMed.
- D. Chang, M. A. Nalls, I. B. Hallgrimsdottir, J. Hunkapiller, M. van der Brug, F. Cai, C. International Parkinson’s Disease Genomics, T. andMe Research, G. A. Kerchner, G. Ayalon, B. Bingol, M. Sheng, D. Hinds, T. W. Behrens, A. B. Singleton, T. R. Bhangale and R. R. Graham, Nat. Genet., 2017, 49, 1511–1516 CrossRef CAS PubMed.
- H. Stefansson, A. Helgason, G. Thorleifsson, V. Steinthorsdottir, G. Masson, J. Barnard, A. Baker, A. Jonasdottir, A. Ingason, V. G. Gudnadottir, N. Desnica, A. Hicks, A. Gylfason, D. F. Gudbjartsson, G. M. Jonsdottir, J. Sainz, K. Agnarsson, B. Birgisdottir, S. Ghosh, A. Olafsdottir, J. B. Cazier, K. Kristjansson, M. L. Frigge, T. E. Thorgeirsson, J. R. Gulcher, A. Kong and K. Stefansson, Nat. Genet., 2005, 37, 129–137 CrossRef CAS PubMed.
- M. C. Zody, Z. Jiang, H. C. Fung, F. Antonacci, L. W. Hillier, M. F. Cardone, T. A. Graves, J. M. Kidd, Z. Cheng, A. Abouelleil, L. Chen, J. Wallis, J. Glasscock, R. K. Wilson, A. D. Reily, J. Duckworth, M. Ventura, J. Hardy, W. C. Warren and E. E. Eichler, Nat. Genet., 2008, 40, 1076–1083 CrossRef CAS PubMed.
- A. M. Pittman, A. J. Myers, P. Abou-Sleiman, H. C. Fung, M. Kaleem, L. Marlowe, J. Duckworth, D. Leung, D. Williams, L. Kilford, N. Thomas, C. M. Morris, D. Dickson, N. W. Wood, J. Hardy, A. J. Lees and R. de Silva, J. Med. Genet., 2005, 42, 837–846 CrossRef CAS PubMed.
- M. Hutton, C. L. Lendon, P. Rizzu, M. Baker, S. Froelich, H. Houlden, S. Pickering-Brown, S. Chakraverty, A. Isaacs, A. Grover, J. Hackett, J. Adamson, S. Lincoln, D. Dickson, P. Davies, R. C. Petersen, M. Stevens, E. de Graaff, E. Wauters, J. van Baren, M. Hillebrand, M. Joosse, J. M. Kwon, P. Nowotny, L. K. Che, J. Norton, J. C. Morris, L. A. Reed, J. Trojanowski, H. Basun, L. Lannfelt, M. Neystat, S. Fahn, F. Dark, T. Tannenberg, P. R. Dodd, N. Hayward, J. B. Kwok, P. R. Schofield, A. Andreadis, J. Snowden, D. Craufurd, D. Neary, F. Owen, B. A. Oostra, J. Hardy, A. Goate, J. van Swieten, D. Mann, T. Lynch and P. Heutink, Nature, 1998, 393, 702–705 CrossRef CAS PubMed.
- D. G. Healy, P. M. Abou-Sleiman, A. J. Lees, J. P. Casas, N. Quinn, K. Bhatia, A. D. Hingorani and N. W. Wood, J. Neurol., Neurosurg. Psychiatry, 2004, 75, 962–965 CrossRef CAS PubMed.
- S. Wray and P. A. Lewis, Front. Psychiatry, 2010, 1, 150 CAS.
- J. E. Tomkins, R. Ferrari, N. Vavouraki, J. Hardy, R. C. Lovering, P. A. Lewis, L. J. McGuffin and C. Manzoni, Cell Commun. Signaling, 2020, 18, 92 CrossRef PubMed.
- G. Alanis-Lobato, M. A. Andrade-Navarro and M. H. Schaefer, Nucleic Acids Res., 2017, 45, D408–D414 CrossRef CAS PubMed.
- Y. Hu, A. Vinayagam, A. Nand, A. Comjean, V. Chung, T. Hao, S. E. Mohr and N. Perrimon, Nucleic Acids Res., 2018, 46, D567–D574 CrossRef CAS PubMed.
- M. Ashburner, C. A. Ball, J. A. Blake, D. Botstein, H. Butler, J. M. Cherry, A. P. Davis, K. Dolinski, S. S. Dwight, J. T. Eppig, M. A. Harris, D. P. Hill, L. Issel-Tarver, A. Kasarskis, S. Lewis, J. C. Matese, J. E. Richardson, M. Ringwald, G. M. Rubin and G. Sherlock, Nat. Genet., 2000, 25, 25–29 CrossRef CAS PubMed.
- Gene Ontology Consortium, Nucleic Acids Res., 2021, 49, D325–D334 CrossRef PubMed.
- R. Ferrari, R. C. Lovering, J. Hardy, P. A. Lewis and C. Manzoni, J. Proteome Res., 2017, 16, 999–1013 CrossRef CAS PubMed.
- A. R. Martin, E. Williams, R. E. Foulger, S. Leigh, L. C. Daugherty, O. Niblock, I. U. S. Leong, K. R. Smith, O. Gerasimenko, E. Haraldsdottir, E. Thomas, R. H. Scott, E. Baple, A. Tucci, H. Brittain, A. de Burca, K. Ibanez, D. Kasperaviciute, D. Smedley, M. Caulfield, A. Rendon and E. M. McDonagh, Nat. Genet., 2019, 51, 1560–1565 CrossRef CAS PubMed.
- R. Ferrari, D. A. Kia, J. E. Tomkins, J. Hardy, N. W. Wood, R. C. Lovering, P. A. Lewis and C. Manzoni, BMC Genomics, 2018, 19, 452 CrossRef PubMed.
- P. Shannon, A. Markiel, O. Ozier, N. S. Baliga, J. T. Wang, D. Ramage, N. Amin, B. Schwikowski and T. Ideker, Genome Res., 2003, 13, 2498–2504 CrossRef CAS PubMed.
- G. Kustatscher, T. Collins, A. C. Gingras, T. Guo, H. Hermjakob, T. Ideker, K. S. Lilley, E. Lundberg, E. M. Marcotte, M. Ralser and J. Rappsilber, Nat. Methods, 2022, 19, 774–779 CrossRef CAS PubMed.
- A. Radzisheuskaya, P. V. Shliaha, V. V. Grinev, D. Shlyueva, H. Damhofer, R. Koche, V. Gorshkov, S. Kovalchuk, Y. Zhan, K. L. Rodriguez, A. L. Johnstone, M. C. Keogh, R. C. Hendrickson, O. N. Jensen and K. Helin, Mol. Cell, 2021, 81, 1749–1765 e1748 CrossRef CAS PubMed.
- J. S. Park, R. L. Davis and C. M. Sue, Curr. Neurol. Neurosci. Rep., 2018, 18, 21 CrossRef PubMed.
- S. Rath, R. Sharma, R. Gupta, T. Ast, C. Chan, T. J. Durham, R. P. Goodman, Z. Grabarek, M. E. Haas, W. H. W. Hung, P. R. Joshi, A. A. Jourdain, S. H. Kim, A. V. Kotrys, S. S. Lam, J. G. McCoy, J. D. Meisel, M. Miranda, A. Panda, A. Patgiri, R. Rogers, S. Sadre, H. Shah, O. S. Skinner, T. L. To, M. A. Walker, H. Wang, P. S. Ward, J. Wengrod, C. C. Yuan, S. E. Calvo and V. K. Mootha, Nucleic Acids Res., 2021, 49, D1541–D1547 CrossRef CAS PubMed.
- L. D. Ward and M. Kellis, Nat. Biotechnol., 2012, 30, 1095–1106 CrossRef CAS PubMed.
|
This journal is © The Royal Society of Chemistry 2023 |
Click here to see how this site uses Cookies. View our privacy policy here.