DOI:
10.1039/D2MO00224H
(Research Article)
Mol. Omics, 2023,
19, 48-59
Lipopolysaccharide and statin-mediated immune-responsive protein networks revealed in macrophages through affinity purification spacer-arm controlled cross-linking (AP-SPACC) proteomics†
Received
15th August 2022
, Accepted 17th October 2022
First published on 18th October 2022
Abstract
Toll-like receptor 4 (TLR4), a pattern recognition receptor, is activated by lipopolysaccharides (LPS) and induces the MyD88 pathway, which subsequently produces pro-inflammatory cytokines through activation of transcriptional nuclear factor (NF)-κB. Statins have been widely prescribed to reduce cholesterol synthesis for patients with cardiovascular disease. Statins may have pleiotropic effects, which include anti- and pro-inflammatory effects on cells. The molecular mechanism of the sequential influence of LPS and statin on the innate immune system remains unknown. We employed affinity purification-spacer-arm controlled cross-linking (AP-SPACC) MS-based proteomics analysis to identify the LPS- and statin-LPS-responsive proteins and their networks. LPS-stimulated RAW 264.7 macrophage cells singly and combined with the drug statin used in this study. Two chemical cross-linkers with different spacer chain lengths were utilized to stabilize the weak and transient interactors. Proteomic analysis identified 1631 differentially expressed proteins. We identified 151 immune-response proteins through functional enrichment analysis and visualized their interaction networks. Selected candidate protein-coding genes were validated, specifically squamous cell carcinoma antigens recognized by T cells 3, sphingosine-1-phosphate lyase 1, Ras-related protein Rab-35, and tumor protein D52 protein-coding genes through transcript-level expression analysis. The expressions of those genes were significantly increased upon statin treatment and decreased in LPS-stimulated macrophage cells. Therefore, we presumed that the expression changes of genes occurred due to immune response during activation of inflammation. These results highlight the immune-responsive proteins network, providing a new platform for novel investigations and discovering future therapeutic targets for inflammatory diseases.
Introduction
Immune cell macrophages play critical roles in our body's inflammatory responses and immunity. An effective immune response is essential in eliminating pathogen invasion and enhancing wound healing.1 Sometimes, inflammation can lead to harmful consequences and is connected to several diseases and conditions, including sepsis,2 cancer,3 asthma,4 tuberculosis,5 arthritis,6 hepatitis,7 allergy,8 and hay fever.9 The Toll-like receptors (TLRs) activate an acute inflammatory response caused by microbial infections, especially bacterial infections.10 The early recognition of infection is facilitated by various macrophage cells, leading to inflammatory mediators’ production, including chemokines and cytokines.1 Macrophages, large white blood cells, are strategically localized in the body tissue. At the same time, they use the process of phagocytosis to ingest and degrade dead cells, cellular debris, and foreign materials and activate the innate immune systems.11
Ten functional Toll-like receptors have been identified in humans so far. Apart from endosomal compartment localized TLRs 3, 7, 8, and 9, the rest of the six TLRs are expressed on various immune cell surfaces as membrane-spanning proteins. Among all these TLRs, TLR4 has gained the most attention due to its ability to identify multiple pathogenic ligands. TLR4 can recognize lipopolysaccharides (LPSs) from the outer membrane of Gram-negative bacteria in association with host accessory proteins MD-2 and CD-14. Upon activation, TLR4 can exert functions through two distinctive pathways, e.g., the MyD88 dependent and the TRIF-dependent pathway.
Lipopolysaccharides (LPS), Gram-negative bacterial ligands, participate in various intracellular events,12 including activation of transcriptional nuclear factor (NF)-κB by TLR4, leading to cellular response and production of pro-inflammatory cytokines, such as tumor necrosis factor (TNF)-α and a variety of interleukins.13 Simvastatin is a widely prescribed medicine to limit cholesterol in hyperlipidemic patients. Statins, an inhibitor of 3-hydroxy-3-methyl coenzyme A (HMG-CoA) reductase within the mevalonate pathway, have anti-inflammatory and immunomodulatory effects in atherosclerosis, rheumatoid arthritis, cerebrovascular, pneumonia, and kidney disease.14–20 Additionally, statins reduce pro-inflammatory cytokines from mononuclear and broncho-epithelial cells by inhibiting HMG-CoA, followed by RhoDa activation.17,21
Identifying protein interacting partners using mass spectrometry (MS)-based proteomics has emerged as a decisive technique.22 However, the typical immunoprecipitation approach has some limitations, such as the requirement for the immunoprecipitation-based specific antibody. Therefore, the biotin–avidin affinity purification approach has gained popularity due to its specificity and user-friendly experimental nature. Previously, we identified 1153 proteins through immunoprecipitation-based proteomics due to the treatment of Pam3CSK4 and statin in TLR2-transfected HEK293 cells,22 while 1103 proteins were identified upon the treatment of LPS and statin,12 and 1206 proteins were identified upon the treatment of LPS and low-dose alcohol12 through label-free quantitative proteomics.
The affinity-purification (AP) technique combined with mass spectrometry (MS)-based proteomics was employed to identify the LPS and statin-LPS responsive proteins upon the treatment of biotinylated-LPS and drug statin in RAW 264.7 macrophage cells. After cell harvesting, we treated the cells with two different chemical cross-linkers sequentially with LPS and statin treatment. The chemical cross-linking agents, namely dual cleavable cross-linking technology (DUCCT) and bissulfosuccinimidyl suberate (BS3), were used to stabilize the transient and weak interactions in the cells.22,23 This mass spectrometry-based affinity proteomics combined with chemical cross-linking, which we call spacer arm controlled cross-linking (SPACC), allowed us to identify 1631 differentially expressed proteins in RAW 264.7 macrophages. Moreover, 151 differentially expressed proteins were examined based on immune response activities and visualized their interactions through Cytoscape software with the application of the STRING database. To this end, we validated six selected candidate protein-coding gene expressions using quantitative real-time PCR. Using the AP-SPACC approach, we concluded that LPS- and statin-LPS-responsive proteins, sideroflexin 1 (SFXN1), acid phosphatase 6, lysophosphatidic (ACP6), squamous cell carcinoma antigen recognized by T cells 3 (SART3), sphingosine-1-phosphate lyase 1 (SGPL1), Ras-related protein Rab-35 (RAB35), and tumor protein D52 (TPD52) were differentially expressed, interacted in RAW 264.7 macrophage cells, and functionally responded to pro-and anti-inflammatory agents. Moreover, many proteins with unknown immune-responsive functions were identified and differentially expressed in the proteome of LPS and statin-LPS-treated macrophage cells.
Experimental procedures
Cell culture and avidin-based protein purifications
RAW 264.7 macrophages were cultured and maintained in dulbecco's modified essential medium (DMEM) as described previously.12,24 The 70% of confluent cells were treated with simvastatin (Sigma) at 10 μM12,22,25,26 of final concentration for 24 h. The cells were further stimulated with biotinylated-LPS (Bio-LPS) at 1 μg mL−1 (tlrl-3blps, InvivoGen) in fresh medium for 1 h.12 Then, the treated cells were incubated with dual cleavable cross-linking technology (DUCCT) cross linkers (XL) and commercial bissulfosuccinimidyl suberate (BS3, Thermo Fisher Scientific, MA) cross-linkers (XLs).23 The XLs were then added to cells to a final concentration of 1 μmol mL−1 for 30 min at room temperature, then the reaction is quenched with 50 mM Tris-HCl (Sigma-Aldrich, MO) at pH 8.0. RAW 264.7 macrophage cells were lysed with RIPA buffer (Thermo Fisher Scientific, MA) for immunoblotting.12 Protein concentrations were measured in the supernatant using a BCA protein assay kit using bovine serum albumin as a standard. Pierce Monomeric avidin UltraLink Resin (Thermo Scientific, MA) washed three times with 1X phosphate buffer saline (PBS, pH 7.4, Thermo Fisher Scientific, MA). Statin and Bio-LPS as well as cross-linker-treated cell lysate were incubated into the pre-washed beads and gently rotated at 4 °C overnight. After extensive washes with 1X PBS, 10 mM NaCl, and ultra-pure water, the bounded proteins were eluted in SDS sample buffer and heated at 95 °C for 5 min. The reduced samples were loaded onto SDS-PAGE gels (12%) for separating the proteins and stained with Sypro Ruby (Thermo Fisher Scientific, MA) after centrifugation (Fig. S1, ESI†).
In-gel digestion, mass spectrometry (MS) analysis (nano-LC–MS/MS), and data analysis
The SDS-PAGE gels were sliced using scalpel, then the sliced gels were squeezed with acetonitrile and air dried. Proteins in gels were reduced and alkylated, then digested with MS Grade Trypsin at 37 °C for 12–16 h.12 Formic acid (10 μL, 0.1%, pH < 3) was added for stopping the trypsin activity by acidifying the sample.12 The gels were dried, and peptides were recovered in 0.1% formic acid. In the end, the samples were spin down at 20
000 × g for 30 min at 4 °C to collect the peptides.
A Velos Pro Dual-Pressure Linear Ion Trap Mass Spectrometer (Thermo Fisher Scientific, MA) coupled to an UltiMate 3000 UHPLC (Thermo Fisher Scientific, MA) was used to analyze digested peptides by nano LC–MS/MS. The method was described previously in detail along with MS parameters.12,22,24 The acquired mass spectra were obtained through Proteome Discoverer software (ver. 2.0, Thermo Fisher Scientific) using the UniProt mouse (Mus musculus) protein sequence database (75
568 sequences, and 3
22
32
886 residues) and the proteins were identified as described earlier in detail along with the used search criteria.12,22,24 Normalization of peptide spectra matches (PSMs) data was achieved using the ratio to individual protein data and the total number of PSMs. Fold changes were assessed by measuring the mean of % fold changes (e.g. a ratio of control to treatment) for different treatments.
Bioinformatics analysis
For gene ontology classifications, we used PANTHER gene classification systems such as biological processes, cellular components and molecular activity.27 The expression of proteins was visualized through heat maps and generated by MeV software (ver. 4.9; https://www.tm4.org/).28 All identified protein-coding genes were used using the public database in Cytoscape (Ver. 3.6.1), namely STRING for the protein query with a confidence cutoff score at 40%, and maximum additional interactions were set at 40.29 Then, functional enrichment categories based on gene ontology were retrieved by STRING enrichment application with high confidence (p ≤ 0.05).30 Protein–protein interaction networks of classified immune response proteins were mapped again using the STRING database.
RNA extraction from cultured cells and real-time PCR analysis
Manufacturer protocols were used to extract RNA from the RAW 264.7 macrophage cells using TRIzol® (Invitrogen). RNA was extracted as per manufacturer protocols. First-strand cDNA synthesis was carried out using a one-step cDNA synthesis kit (Origene, MD, USA) according to manufacturer protocols. The CFX96 real-time system (Bio-Rad) along with the SsoAdvanced™ Universal SYBR® Green Supermix (Bio-Rad) was used to analyze the mRNA expression. The ESI† provides the primers’ sequences (Table S1).
Statistical analysis
GraphPad Prism (ver. 6, GraphPad Software, Inc) was used for all statistical analysis. The mean ± standard deviation (SD) is shown in the graph. Multiple and student t-tests determined the statistical significance.
Results
LPS and statin-LPS responsive proteins
RAW 264.7 macrophage cells were treated with biotin-LPS and statin along with chemical cross-linkers DUCCT (∼18 Å) and BS3 (∼11 Å) for identifying the LPS and statin-LPS responsive-proteins and their interacting partners using affinity-purification spacer-arm controlled cross-linking mass spectrometry (AP-SPACC-MS) based proteomics. Earlier, we reported the activation of macrophages upon treating LPS in Raw 264.7 macrophages, where we showed that TNF-α was significantly increased upon the treatment of LPS.24 After evaluating several procedures for protein elution from avidin beads (data are not shown), we implemented the SDS sample buffer for protein elution. After that, proteins were preferentially enriched and separated by SDS-PAGE (Fig. 1). The separated proteins were digested enzymatically, and the peptides were analyzed by mass spectrometry. In the four conditions (control, Bio-LPS, statin-Bio-LPS, and statin), 1631 proteins were identified, while 1, 74, 146, and 98 proteins were exclusively identified in each category, respectively (Fig. 2A and Fig. S2, ESI†). However, BS3 cross-linker recruited more proteins than DUCCT-XL and without cross-linkers (Fig. 2). When DUCCT-XL treatment was compared with the control, a total of 1291 proteins were identified, whereas 32, 169, 59, and 76 proteins were identified exclusively under each treatment condition. (Fig. 2B and Fig. S2, ESI†). However, when BS3-XL treatment was compared with regular treatment, 1460 proteins were identified. Further analysis showed that 86, 24, 78, and 237 proteins were identified exclusively under four treatment conditions (Fig. 2C and Fig. S2, ESI†). After missing value imputation using KNN methods, identified protein intensities were plotted as scatter plots (control versus treatments). The scatter plot showed a significant data correlation (R2 ≥ 0.80 out of 1) between the control and all treatment conditions (Fig. 3). The total identified protein (1631 proteins) coding genes in this study were categorized using PANTHER gene classification systems specific to their biological processes. These results based on gene ontology indicated that many proteins were associated with cellular (31.76%) and metabolic processes (28.41%). Immune-system process-related proteins were predominantly only 1.29% (151 proteins) (Fig. 4). From further analysis, we identified 151 (9.2%) immune system proteins using String database in AP-SPACC-MS (Table S3, ESI†). In contrast, Chen et al., 202031 reported 4.84% of immune system proteins upon treatment of LPS in macrophage cells using a global proteomic approach along with the data independent acquisition (DIA) LC–MS method without affinity purification.
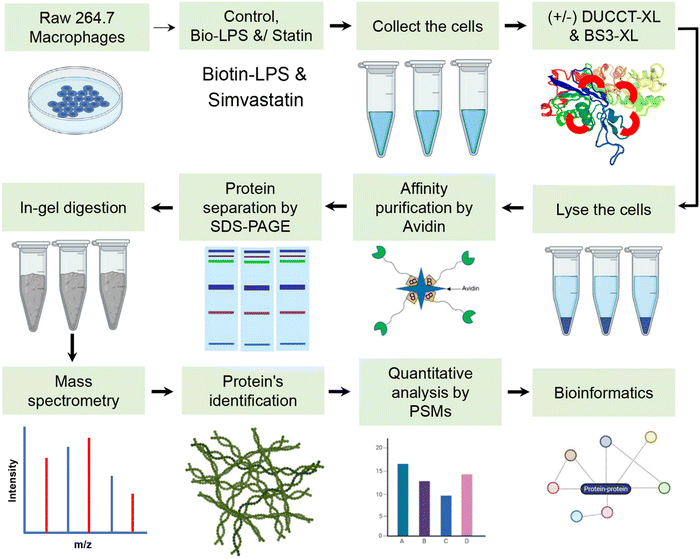 |
| Fig. 1 Schematic overview of spacer-chain controlled affinity purification-based cross-linking proteomics. Cells were cultured and treated with statin and Bio-LPS along with cross-linkers DUCCT and BS3. Avidin-based pull-down of proteins was employed, separated by SDS-PAGE, identified by mass spectrometry, and then quantified using PSMs. | |
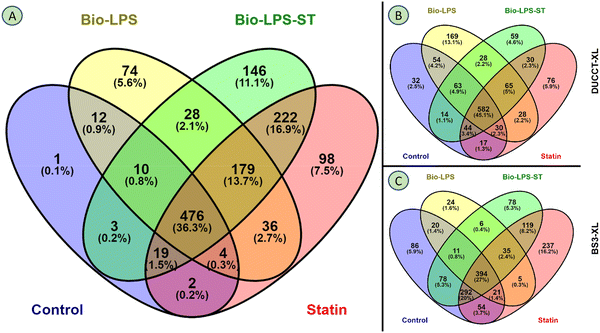 |
| Fig. 2 Venn diagram of differentially expressed proteins. This experiment revealed that a total of 1310 proteins were identified, 476 of which were shared among the regular treatments (A). A total of 1291 proteins were identified, 582 of which were shared among the treatments with DUCCT-XL (B). A total of 1460 proteins were identified, 394 of which were shared among the treatments with BS3-XL (C). | |
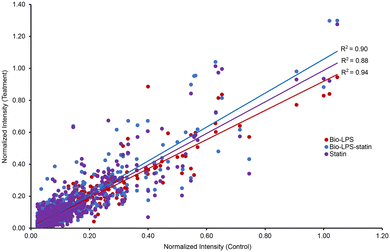 |
| Fig. 3 Scatter plots of control versus treated samples, visualized with the normalized intensity of identified proteins. Protein intensities have systematically verified the correlation between control and treated samples and are represented by Pearson's correlation coefficients R2 of 1. | |
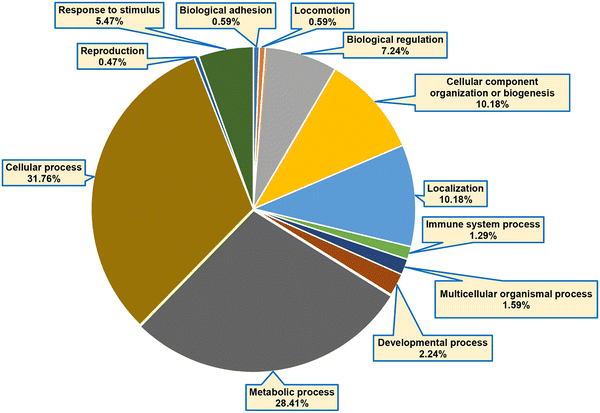 |
| Fig. 4 Functional classification based on gene ontology. The identified proteins-coding genes were grouped based on biological processes using the PANTHER classification system. | |
Exclusively identified proteins by cross-linking treatments
Identified proteins were rigorously filtered using the control, statin (treatment control), and XL-control. A total of 463 proteins were revealed in the regular study, whereas 28 proteins were commonly shared, and 289 and 146 proteins were exclusively identified upon the treatment of Bio-LPS, and Bio-LPS-ST, respectively (Fig. 5A and Fig. S2, ESI†). Immune-responsive proteins identified were gag protein (GAG), isocitrate dehydrogenase (IDH1), and thymopoietin (TMPO). A heatmap showed these proteins’ relative expressions, where TMPO sharply declined. In contrast, GAG and IDH1 were only expressed in Bio-LPS-ST compared to Bio-LPS (Fig. 5A). A total of 156 proteins were identified due to the additional treatment of DUCCTs sequentially after Bio-LPS and Bio-LPS-ST. In the DUCCT-treated samples, 15 proteins were commonly shared, and 126 and 15 proteins were exclusively identified in the Bio-LPS and Bio-LPS-ST treatment (Fig. 5B and Fig. S2, ESI†). In the heatmap, 6 immune-responsive protein coding genes such as integrin-associated protein (CD47), inosine-5′-monophosphate dehydrogenase (IMPDH1), immunity-related GTPase family M protein 1 (IRGM1), proline-rich AKT1 substrate 1 (PRAS), ubiquitin-conjugating enzyme E2 N (UBE2N), and Vav guanine nucleotide exchange factor 1 (VAV1) were found through bioinformatics analysis. In particular, all the immune-associated protein coding gene expressions were decreased upon the treatment of Bio-LPS-ST compared to Bio-LPS (Fig. 5B). A total of 212 proteins were revealed upon the treatment of Bio-LPS, and Bio-LPS-ST along with BS3-XLs, whereas five proteins commonly shared and 102 and 105 proteins were exclusively identified in the treatment of Bio-LPS, and Bio-LPS-ST, respectively (Fig. 5C and Fig. S2, ESI†). In the heatmap, 18 immune-responsive protein coding genes e.g. toll-like receptors 2 (TLR2), Ras-related protein Rab-32 (RAB32), and MutS homolog (MSH) were found through bioinformatics analysis. Additionally, all the immune-associated protein-coding genes were decreased in the treatment of Bio-LPS-ST compared to Bio-LPS (Fig. 5C).
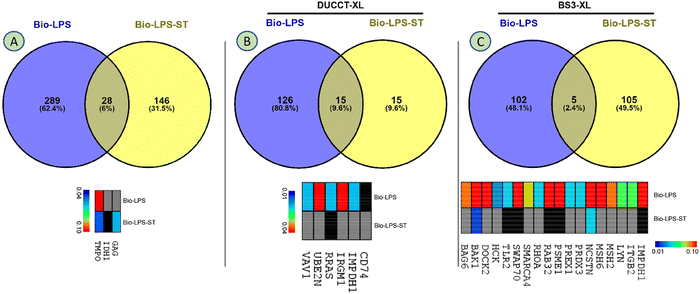 |
| Fig. 5 Venn diagram of exclusively identified proteins. Exclusively identified proteins were scrutinized by stringent filtering in a regular sample (A) as well as DUCCT (B) and BS3 (C) cross-link treated samples. In this study, we used three controls: sample control without ligands, cross-linked control that is only treated with cross-linkers, and treatment control is only statin without biotin. The heatmap shows the differential expression of immune response proteins. | |
Immune-responsive proteins and their interactions networks
Through STRING enrichment analysis by Cytoscape, a total of 151 proteins were mapped out of 1631 proteins due to the treatment of Bio-LPS and Bio-LPS-ST along with XLs (Table S3, ESI† and Fig. 6 and 7). The 151 immune-response proteins were visualized through a heatmap that showed differential protein expressions upon the treatment of Bio-LPS and Bio-LPS-ST along with XLs. The heatmap provides a clear portrait of immune-responsive proteins that allowed us to find quantitative patterns across treated samples simultaneously (Fig. 6). Identified immune-response proteins were generated in the interaction network using the STRING protein database using the Cytoscape platform, where the yellow color nodes indicated the experimentally identified proteins and the green color nodes showed the linker genes. Eight protein-coding genes such as Sideroflexin 1 (SFXN1), acid phosphatase 6, lysophosphatidic (ACP6), squamous cell carcinoma antigen recognized by T cells 3 (SART3), TROVE domain family member 2 (TROVE2), sphingosine-1-phosphate lyase 1 (SGPL1), Ras-related protein Rab-35 (RAB35), tumor protein D52 (TPD52), and regulator of differentiation 1 (ROD1) did not fall into the interaction networks, even though they were identified in this study due to the differential treatments. Consequently, these eight immune-responsive proteins were considered new interaction partners upon treating Bio-LPS and Bio-LPS-ST in RAW 264.7 macrophage cells (Fig. 7).
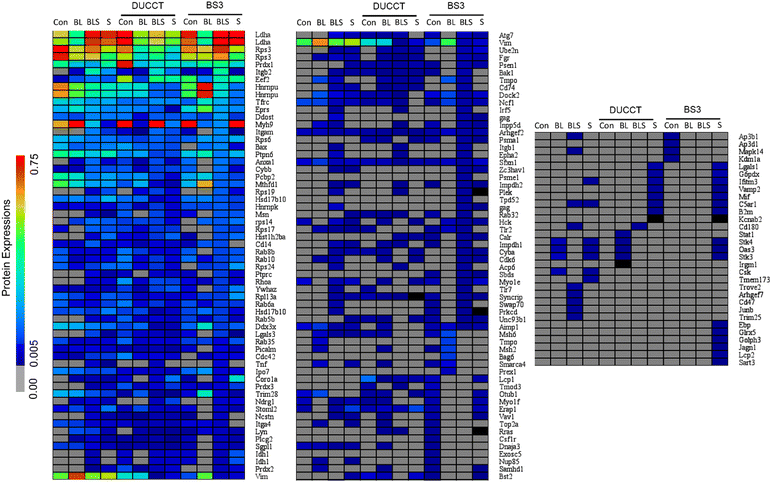 |
| Fig. 6 Heatmap displays differential protein expressions. The identified immune-responsive proteins using the STRING database were visualized upon treating bio-LPS, statin-bio-LPS, and statin along with cross-linkers (Table S3, ESI†). | |
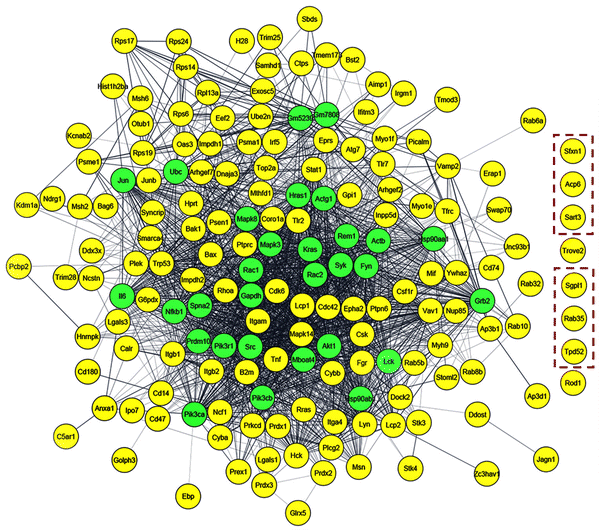 |
| Fig. 7 Protein interaction network of the identified immune-responsive proteins. Those proteins were classified using STRING enrichment application in Cytoscape. The yellow color represents the identified proteins in this study, while the green color represents the linker genes. | |
Validation of selected immune-responsive protein-coding genes
Six selected immune-responsive protein-coding genes (Rab35, SGPL1, SFXN1, ACP6, SART3, and TPD52) were further validated by quantitative real-time PCR (qRT-PCR) analysis. These six proteins were selected based on the STRING enrichment and protein network analysis and their functions and differential expressions because of the treatment of Bio-LPS and Bio-LPS-ST in RAW 264.7 macrophage cells (Fig. 6–8). In the heatmap, these proteins displayed their quantitative patterns based on mass spectrometry results upon the treatment of Bio-LPS and Bio-LPS-ST together with or without XLs in RAW 264.7 macrophage cells (Fig. 8). Based on the qRT-PCR analysis, Rab35, SGPL1, and SART3 expressions were decreased upon the treatment of Bio-LPS and Bio-LPS-ST, while these expressions markedly increased compared to the control sample in the treatment of statin alone in RAW 264.7 macrophage cells. Furthermore, ACP6 and SFXN1 expressions were decreased by the treatment of Bio-LPS, Bio-LPS-ST, and statin compared to the control samples. Interestingly, SFXN1 expression was profoundly reduced by the treatment of statin alone in RAW 264.7 macrophage cells. In contrast, TPD52 was highly expressed in the statin-treated RAW 264.7 macrophage cells (Fig. 8).
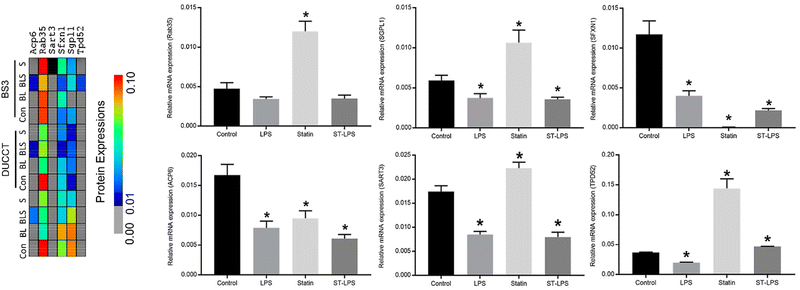 |
| Fig. 8 Validation of selected immune response proteins. The heatmap shows the differential protein expression of selected proteins that were validated by mRNA expression analysis. mRNA expression analysis was done using qRT-PCR. All data presented as mean ± SD (n = 3 in each group) with **P < 0.05, as per multiple t-test. | |
Discussions
Over the past years, the AP- and AP-MS/MS approach has shown to be convincing at revealing protein interaction partners in a complex sample.32–39 In this study, we discovered the LPS and LPS-statin responsive proteins and their interactions using a newly developed method AP-SPACC tandem mass spectrometry approach. Two different cross-linkers with different reactive distances were used. Besides, RAW 264.7 macrophage cells were stimulated by bio-LPS and statin drug alone or simultaneously. Additionally, the harvested cells were treated with DUCCT and BS3 cross-linkers, allowing transient and weak interactions to be preserved and captured stably in a complex. Non-covalent binding methods such as immunoprecipitation and affinity-based purification have gained popularity for robust interactions. Still, it fails to keep transient and weak protein–protein interactions attached during purification processes. Recently, a chemistry-based fixation method called cross-linking was recognized for stabilizing weak and transient interactions under harsh purification conditions.23,40–45 In this study, we found that cross-linking application in intact cells increased protein identifications (Fig. 2 and Table S2, ESI†). We utilized avidin to capture the biotinylated proteins from the complex protein samples. The sample buffer eluted the attached proteins; then, the proteins were separated using SDS-PAGE gels. The digested gels were analyzed by high throughput mass spectrometry, and we quantified the proteins through peptide-spectrum matches (Fig. 1). After stringent filtering between cross-linking and non-cross-linking samples, LPS and LPS-statin responsive proteins were percolated and identified. To the best of our knowledge, this is the first report to identify LPS and LPS-statin-responsive proteins along with immune-responsive protein interactions in RAW 264.7 macrophage cells combined with cross-linking applications using AP-MS/MS. All identified immune-responsive protein interaction networks were generated using STRING application via the Cytoscape platform (Fig. 7 and Table S3, ESI†). While using existing interaction databases, eight immune-responsive proteins have remained uninteracted in the protein networks. Perhaps, these proteins were newly identified interaction partners and immune-responsive proteins due to the treatment of LPS and statin in RAW 264.7 macrophage cells (Fig. 7 and Table S3, ESI†).
The newly identified interacting protein-coding genes were differentially expressed in RAW 264.7 macrophage cells at the transcriptomics level after treatment with LPS and statin. In this study, Rab35, SGPL1, SART3, and TPD52 protein-coding genes were significantly upregulated upon the treatment of the statin drug, whereas these genes were downregulated by the treatment of LPS and stain-LPS compared to the regular control and statin (Fig. 8). Ras-related proteins are small guanosine triphosphatases (GTPase) in the Ras superfamily, involved in the movement, vesicular formation, fusion processes, and also controlling the membrane trafficking of cell surface receptors for cytokines, hormones, and chemokines.46–49 Previously, Rab10 protein enhanced the LPS-induced cytokines and impacted the LPS-induced NF-κB, MAPK, and IFN regulatory factor 3 signaling pathways.50 Rab10 proteins were downregulated in our study upon LPS and statin drug treatment in RAW 264.7 macrophage cells (Table S2, ESI†). Rab35 is a plasma membrane protein that actively participates in the endocytic recycling pathway.51 It has been indicated in the involvement of phagosomes, phagolysosome fusion, and actin cytoskeletal remodeling and assembling in macrophages.52–54 Previously, it was reported that it has been associated with Cdc42 and Rac1 transport during phagocytosis.52 Here, Cdc42 and Rac1 proteins were found in the LPS and statin-treated samples (Table S2, ESI†). Rab35 proteins, together with Cdc42 and Rac1 proteins, might enhance the phagocytosis process and be involved in actin cytoskeletal rearrangements in RAW 264.7 macrophage cells during statin drug treatment.
Interestingly, SGPL1, SART3, and TPD52 protein-coding genes are decreased in the treatment of LPS and increased in the treatment of statin in RAW 264.7 macrophage cells (Fig. 8). In contrast, treatment of statin-LPS decreased SART3 and SGPL1 genes significantly except TPD52 (Fig. 8). SGPL1 protein is a single-pass type III membrane protein in the endoplasmic reticulum that is the part of pyridoxal 5′-phosphate-dependent carboxy-lyases55 and a primary regulator of sphingosine 1-phosphate (S1P).56 The protein is expressed in the stomach, kidney, liver, intestine, thymus, colon, and spleen.57 SGPL1 protein, a bioactive lipid metabolite, has been influencing the different physiological functions, including inflammation, cell survival and apoptosis, migration, cell proliferation and differentiation, angiogenesis, platelet aggregation, cardio-protection, vascular permeability, and lymphocyte trafficking and development.58–60 Several researchers noted that SGPL1 protein actively contributes to inflammation and immunity in cancer progression and autoimmune diseases.58,61 It has been shown that SGPL1 protein is a potential regulator of T-cell trafficking, and this protein's signaling may involve the host's response to infections.62,63 However, activating S1P kinase by LPS also promotes cell invasion, progression, and metastasis in pancreatic tumors.59,64 SGPL1 protein is critical in cancer metastasis and inflammations through T-cell trafficking. We believe, due to its involvement with high-density lipoprotein, SGPL1 protein had shown significant upregulation upon the treatment of statin. Possibly, SGPL1 protein might have a regulatory function in cholesterol biosynthesis.56
SART3 is a tumor rejection antigen in cytosol and nucleus and recognized by HLA- class I-restricted and tumor-specific cytotoxic T lymphocytes in cancer cells and tissue with various histologic types, including colorectal, breast, head, neck, esophageal, and musculoskeletal cancer. SART3 peptide coding antigen is a powerful agent in tumor-specific immunotherapy.65–68 SART3 protein has been involved with cellular and molecular activities, including RNA-binding, cell proliferation, and protein phosphorylation.69 SART3 protein-coding gene expression was analyzed at transcript levels in our study. In contrast, it is moderately high compared to control but decreased upon treating LPS and statin-LPS in macrophage cells (Fig. 8). SART3 showed an antagonistic relation to the treatment of statin-LPS. Furthermore, SART3 was established as a tumor-specific immunotherapeutic molecule while actively participating in the immune response. SART3 may play an essential role in anti-inflammation during immune response upon treating LPS and statin in macrophage cells.
TPD52 protein is a small hydrophobic polypeptide that is a widely studied candidate oncogene at chromosome 8q21, including breast, prostate, ovarian, and other cancers.70,71 TPD52 protein-coding gene facilitates cell proliferation, migration, and metastasis, while inhibition of TPD52 induces breast and prostate cancer cell death.72 TPD52 in the transcript level has been increased in mouse and human adipose tissue,73 whereas its inhibition significantly decreased the lipid storage in C. elegans.74 Previously, several researchers reported that overexpression of TPD52 induces lipogenic cancers, including breast and prostate cancer,72,75–77 by actively promoting lipid storage and lipogenesis in the cells.78 In this study, TPD52 protein and mRNA levels were increased by statin treatment in RAW 264.7 macrophage cells, and a possible reason might be an accumulation of lipid droplets or lipogenesis. Previously, several studies reported that statin reportedly increased fat accumulation and triggered fatty acids and cholesterol biosynthesis.79,80
Furthermore, ACP6 and SFXN1 protein-coding genes were downregulated by the treatment of LPS, statin, and stain-LPS compared to the treatment of the control (Fig. 8). ACP6 is a mitochondrial lysophosphatidic acid (LPA)-specific phosphatase that hydrolyzes LPA to monoacylglycerol and phosphate.81 It is involved in phospholipid metabolism and balancing the lipid raft composition in the cell.82 LPA has been associated with inducing biological activities, including cell growth, proliferation, survival, and migration.81,83 LPA is also involved in oncogenesis, progression, and metastasis and has found significant clinical involvement in esophageal squamous cell carcinoma.84,85 Based on previous findings and our results, ACP6 protein limits lipid biosynthesis and metabolism upon statin treatment in RAW 264.7 macrophage cells. SFXN1 protein, tricarboxylate carrier protein, is localized in inner-mitochondria. Some isoforms of SFXN1 proteins have been involved in cell growth and morphology, melanocyte development, and differentiation of pancreatic beta- and endocrine-cells,86–88 whereas pancreatic beta- and endocrine-cells released insulin and glucagon in the bloodstream.89,90 In our study, the SFXN1 protein-coding gene was significantly decreased at statin drug treatment (Fig. 8). This result indicated that SFXN1 protein or protein-coding genes might play an important role in interfering with the insulin signaling pathway during the pro-inflammatory response in immune cells.
Conclusions
In this study, we performed AP-MS/MS proteomics using spacer arm-controlled cross-linking (SPACC) in an immune cell macrophage. Our studies identified several potential interactors in LPS signaling pathways and denote the superiority of the AP-SPACC-MS technique for protein interaction studies. Although we mainly focused on identifying the interactors by looking at non-modified peptides using our method, our future attempts will concentrate on identifying cross-linked peptides. At this moment, we analyzed the unmodified peptides due to the unavailability of large-scale data analysis software capability with DUCCT. We have a prototype software Cleave-XL, but a large-scale data analysis software is under construction. We expect more data with a high-resolution mass spectrometry platform. Nevertheless, we identified several novel interactors using our method. We believe that our data from the discovery-based interactomics studies using AP-SPACC-MS-based proteomics will significantly impact LPS and TLR4-based protein interaction studies.
Author contributions
Conceptualization, S. M. C., A. H. M. K.; methodology, S. M. C., A. H. M. K.; validation, A. H. M. K.; formal analysis, A. H. M. K., J. K. C.; data curation, A. H. M. K.; writing – original draft preparation, A. H. M. K., S. M. C.; writing – review and editing, S. M. C., A. H. M. K., J. K. C.; visualization, A. H. M. K.; supervision, S. M. C.; project administration, S. M. C.; funding acquisition, S. M. C. All authors have read and agreed to the published version of the manuscript.
Data availability
“The mass spectrometry proteomics data have been deposited to the ProteomeXchange Consortium via the PRIDE91 partner repository with the dataset identifier PXD034209”.
Abbreviations
Bio-LPS | Biotinylated-lipopolysaccharide |
AP-MS | Affinity purification-mass spectrometry |
XL | Cross-linking |
TLR4 | Toll-like receptor 4 |
Conflicts of interest
There are no conflicts to declare.
Acknowledgements
We acknowledge the support from NIGMS, NIH grant number 1UA5GM113216-01, for funding of this research. Authors also recognize start-up fund supports to PI Dr. Chowdhury from UT Arlington. UT Systems Shared Proteomics Networks are also acknowledged for a Thermo LTQ-velos pro mass spectrometer.
References
- R. Medzhitov, Nature, 2008, 454, 428–435 CrossRef CAS
.
- H. Minasyan, J. Crit. Care, 2017, 40, 229–242 CrossRef CAS
.
- F. Pandolfi, S. Altamura, S. Frosali and P. Conti, Clin. Ther., 2016, 38, 1017–1028 CrossRef CAS
.
- K. Hirahara, M. Yamashita, C. Iwamura, K. Shinoda, A. Hasegawa, H. Yoshizawa, H. Koseki, F. Gejyo and T. Nakayama, J. Allergy Clin. Immunol., 2008, 122, 512–520.e11 CrossRef CAS PubMed
.
- A. Zumla, M. Rao, S. K. Parida, S. Keshavjee, G. Cassell, R. Wallis, R. Axelsson-Robertsson, M. Doherty, J. Andersson and M. Maeurer, J. Intern. Med., 2015, 277, 373–387 CrossRef CAS PubMed
.
- S. K. Jain, S. Singh, A. Khajuria, S. K. Guru, P. Joshi, S. Meena, J. R. Nadkarni, A. Singh, S. S. Bharate, S. Bhushan, S. B. Bharate and R. A. Vishwakarma, J. Med. Chem., 2014, 57, 7085–7097 CrossRef CAS PubMed
.
- L. G. Guidotti, M. Isogawa and F. V. Chisari, Curr. Opin. Immunol., 2015, 36, 61–66 CrossRef CAS PubMed
.
- S. R. Naik and S. M. Wala, Recent Pat. Inflammation Allergy Drug Discovery, 2013, 7, 62–95 CrossRef CAS
.
- P. C. Schroder, V. I. Casaca, S. Illi, M. Schieck, S. Michel, A. Bock, C. Roduit, R. Frei, A. Lluis, J. Genuneit, P. Pfefferle, M. Roponen, J. Weber, C. Braun-Fahrlander, J. Riedler, R. Lauener, D. A. Vuitton, J.-C. Dalphin, J. Pekkanen, E. von Mutius, M. Kabesch and B. Schaub, Pediatr. Allergy Immunol., 2016, 27, 687–695 CrossRef
.
- G. M. Barton, J. Clin. Invest., 2008, 118, 413–420 CrossRef CAS PubMed
.
- C. Varol, A. Mildner and S. Jung, Annu. Rev. Immunol., 2015, 33, 643–675 CrossRef CAS PubMed
.
- A. H. M. Kamal, J. K. Chakrabarty, S. M. N. Udden, M. H. Zaki and S. M. Chowdhury, Sci. Rep., 2018, 8, 164 CrossRef
.
- W. Wang, J. Weng, L. Yu, Q. Huang, Y. Jiang and X. Guo, BMC Pulm. Med., 2018, 18, 178 CrossRef
.
- G. J. Blake and P. M. Ridker, Curr. Controlled Trials Cardiovasc. Med., 2000, 1, 161–165 CrossRef CAS PubMed
.
- V. M. Campese and J. Park, Kidney Int., 2007, 71, 1215–1222 CrossRef CAS PubMed
.
- R. Gilbert, A. Al-Janabi, O. Tomkins-Netzer and S. Lightman, Porto Biomed. J., 2017, 2, 33–39 CrossRef
.
- A. Iwata, R. Shirai, H. Ishii, H. Kushima, S. Otani, K. Hashinaga, K. Umeki, K. Kishi, I. Tokimatsu, K. Hiramatsu and J. Kadota, Clin. Exp. Immunol., 2012, 168, 234–240 CrossRef CAS PubMed
.
- A. Nassief and J. D. Marsh, Stroke, 2008, 39, 1042–1048 CrossRef CAS
.
- V. R. Taqueti and P. M. Ridker, Circ. Cardiovasc. Imaging, 2017, 10, e006676 CrossRef PubMed
.
- C. J. Vaughan, A. M. Gotto and C. T. Basson, J. Am. Coll. Cardiol., 2000, 35, 1–10 CrossRef CAS
.
- H. Bessler, H. Salman, M. Bergman, R. Straussberg and M. Djaldetti, Clin. Immunol., 2005, 117, 73–77 CrossRef CAS PubMed
.
- A. H. M. Kamal, J. J. Aloor, M. B. Fessler and S. Chowdhury, Mol. Cell. Proteomics, 2019, 1732–1744 CrossRef CAS
.
- J. K. Chakrabarty, A. G. Naik, M. B. Fessler, G. R. Munske and S. M. Chowdhury, Anal. Chem., 2016, 88, 10215–10222 CrossRef CAS PubMed
.
- A. H. M. Kamal, M. B. Fessler and S. M. Chowdhury, PLoS One, 2018, 13, e0193104 CrossRef PubMed
.
- S. Wang, Y. Ran, X. Chen, C. Li, S. Cheng and J. Liu, Front. Pharmacol., 2020, 11, 101 CrossRef CAS PubMed
.
- S. M. de, V. Lobo, B. M. R. Quinto, L. Oyama, R. Nakamichi, A. B. Ribeiro, M. T. Zanella, M. A. Dalboni and M. C. Batista, Cytokine, 2012, 60, 150–156 CrossRef
.
- H. Mi, A. Muruganujan, J. T. Casagrande and P. D. Thomas, Nat. Protoc., 2013, 8, 1551–1566 CrossRef PubMed
.
- A. I. Saeed, V. Sharov, J. White, J. Li, W. Liang, N. Bhagabati, J. Braisted, M. Klapa, T. Currier, M. Thiagarajan, A. Sturn, M. Snuffin, A. Rezantsev, D. Popov, A. Ryltsov, E. Kostukovich, I. Borisovsky, Z. Liu, A. Vinsavich, V. Trush and J. Quackenbush, Biotechniques, 2003, 34, 374–378 CrossRef CAS PubMed
.
- D. Szklarczyk, J. H. Morris, H. Cook, M. Kuhn, S. Wyder, M. Simonovic, A. Santos, N. T. Doncheva, A. Roth, P. Bork, L. J. Jensen and C. von Mering, Nucleic Acids Res., 2017, 45, D362–D368 CrossRef CAS PubMed
.
- D. Szklarczyk, A. Franceschini, S. Wyder, K. Forslund, D. Heller, J. Huerta-Cepas, M. Simonovic, A. Roth, A. Santos, K. P. Tsafou, M. Kuhn, P. Bork, L. J. Jensen and C. von Mering, Nucleic Acids Res., 2015, 43, D447–52 CrossRef CAS PubMed
.
- L. Li, L. Chen, X. Lu, C. Huang, H. Luo, J. Jin, Z. Mei, J. Liu, C. Liu, J. Shi, P. Chen and Y. Jiang, Proteomics, 2020, 20, e1900203 CrossRef PubMed
.
- A. Breitkreutz, H. Choi, J. R. Sharom, L. Boucher, V. Neduva, B. Larsen, Z. Y. Lin, B. J. Breitkreutz, C. Stark, G. Liu, J. Ahn, D. Dewar-Darch, T. Reguly, X. Tang, R. Almeida, Z. S. Qin, T. Pawson, A. C. Gingras, A. I. Nesvizhskii and M. Tyers, Science, 2010, 328, 1043–1046 CrossRef CAS PubMed
.
- E. M. Conn, M. A. Madsen, B. F. Cravatt, W. Ruf, E. I. Deryugina and J. P. Quigley, J. Biol. Chem., 2008, 283, 26518–26527 CrossRef CAS PubMed
.
- A.-C. Gavin, P. Aloy, P. Grandi, R. Krause, M. Boesche, M. Marzioch, C. Rau, L. J. Jensen, S. Bastuck, B. Dumpelfeld, A. Edelmann, M.-A. Heurtier, V. Hoffman, C. Hoefert, K. Klein, M. Hudak, A.-M. Michon, M. Schelder, M. Schirle, M. Remor, T. Rudi, S. Hooper, A. Bauer, T. Bouwmeester, G. Casari, G. Drewes, G. Neubauer, J. M. Rick, B. Kuster, P. Bork, R. B. Russell and G. Superti-Furga, Nature, 2006, 440, 631–636 CrossRef CAS PubMed
.
- C. Jeronimo, D. Forget, A. Bouchard, Q. Li, G. Chua, C. Poitras, C. Therien, D. Bergeron, S. Bourassa, J. Greenblatt, B. Chabot, G. G. Poirier, T. R. Hughes, M. Blanchette, D. H. Price and B. Coulombe, Mol. Cell, 2007, 27, 262–274 CrossRef CAS PubMed
.
- N. J. Krogan, G. Cagney, H. Yu, G. Zhong, X. Guo, A. Ignatchenko, J. Li, S. Pu, N. Datta, A. P. Tikuisis, T. Punna, J. M. Peregrin-Alvarez, M. Shales, X. Zhang, M. Davey, M. D. Robinson, A. Paccanaro, J. E. Bray, A. Sheung, B. Beattie, D. P. Richards, V. Canadien, A. Lalev, F. Mena, P. Wong, A. Starostine, M. M. Canete, J. Vlasblom, S. Wu, C. Orsi, S. R. Collins, S. Chandran, R. Haw, J. J. Rilstone, K. Gandi, N. J. Thompson, G. Musso, P. Onge, S. Ghanny, M. H. Y. Lam, G. Butland, A. M. Altaf-Ul, S. Kanaya, A. Shilatifard, E. O’Shea, J. S. Weissman, C. J. Ingles, T. R. Hughes, J. Parkinson, M. Gerstein, S. J. Wodak, A. Emili and J. F. Greenblatt, Nature, 2006, 440, 637–643 CrossRef CAS PubMed
.
- D. Forget, A.-A. Lacombe, P. Cloutier, R. Al-Khoury, A. Bouchard, M. Lavallee-Adam, D. Faubert, C. Jeronimo, M. Blanchette and B. Coulombe, Mol. Cell. Proteomics, 2010, 9, 2827–2839 CrossRef CAS PubMed
.
- M. Lavallée-Adam, J. Rousseau, C. Domecq, A. Bouchard, D. Forget, D. Faubert, M. Blanchette and B. Coulombe, J. Proteome Res., 2013, 12, 272–281 CrossRef
.
- M. P. Washburn, D. Wolters and J. R. Yates 3rd, Nat. Biotechnol., 2001, 19, 242–247 CrossRef CAS PubMed
.
- T. Berggard, S. Linse and P. James, Proteomics, 2007, 7, 2833–2842 CrossRef PubMed
.
- V. P. R. Chichili, V. Kumar and J. Sivaraman, Intrinsically Disord. Proteins, 2013, 1, e25464 CrossRef PubMed
.
- G. W. Preston and A. J. Wilson, Chem. Soc. Rev., 2013, 42, 3289–3301 RSC
.
- Y. Tanaka, M. R. Bond and J. J. Kohler, Mol. BioSyst., 2008, 4, 473–480 RSC
.
- B. Yang, S. Tang, C. Ma, S. T. Li, G. C. Shao, B. Dang, W. F. DeGrado, M. Q. Dong, P. G. Wang, S. Ding and L. Wang, Nat. Commun., 2017, 8, 1–10 CrossRef PubMed
.
- S. H. Hu, A. E. Whitten, G. J. King, A. Jones, A. F. Rowland, D. E. James and J. L. Martin, PLoS One, 2012, 7(8), e41731 CrossRef CAS PubMed
.
- A. F. Bruns, L. Bao, J. H. Walker and S. Ponnambalam, Biochem. Soc. Trans., 2009, 37, 1193–1197 CrossRef CAS PubMed
.
- S. Ishikura, A. Koshkina and A. Klip, Acta Physiol., 2008, 192, 61–74 CrossRef CAS PubMed
.
- N. F. Néel, E. Schutyser, J. Sai, G.-H. Fan and A. Richmond, Cytokine Growth Factor Rev., 2005, 16, 637–658 CrossRef
.
- H. Stenmark, Nat. Rev. Mol. Cell Biol., 2009, 10, 513–525 CrossRef CAS PubMed
.
- D. Wang, J. Lou, C. Ouyang, W. Chen, Y. Liu, X. Liu, X. Cao, J. Wang and L. Lu, Proc. Natl. Acad. Sci. U. S. A., 2010, 107, 13806–13811 CrossRef CAS
.
- I. Kouranti, M. Sachse, N. Arouche, B. Goud and A. Echard, Curr. Biol., 2006, 16, 1719–1725 CrossRef CAS PubMed
.
- Y. Egami, M. Fukuda and N. Araki, J. Cell Sci., 2011, 124, 3557–3567 CrossRef CAS PubMed
.
- T. M. Robinson-Smith, I. Isaacsohn, C. A. Mercer, M. Zhou, N. Van Rooijen, N. Husseinzadeh, M. M. McFarland-Mancini and A. F. Drew, Cancer Res., 2007, 67, 5708–5716 CrossRef CAS PubMed
.
- J. Shim, S.-M. Lee, M. S. Lee, J. Yoon, H.-S. Kweon and Y.-J. Kim, Mol. Cell. Biol., 2010, 30, 1421–1433 CrossRef CAS PubMed
.
- P. P. Van Veldhoven, Methods Enzymol., 2000, 311, 244–254 CAS
.
- M. Serra and J. D. Saba, Adv. Enzyme Regul., 2010, 50, 349–362 CrossRef PubMed
.
- N. Engel, A. Adamus, M. Frank, K. Kraft, J. Kuhn, P. Muller, B. Nebe, A. Kasten and G. Seitz, PLoS One, 2018, 13, e0196854 CrossRef PubMed
.
- P. Bandhuvula and J. D. Saba, Trends Mol. Med., 2007, 13, 210–217 CrossRef CAS PubMed
.
- M. Maceyka, K. B. Harikumar, S. Milstien and S. Spiegel, Trends Cell Biol., 2012, 22, 50–60 CrossRef CAS PubMed
.
- B. Oskouian and J. D. Saba, Semin. Cell Dev. Biol., 2004, 15, 529–540 CrossRef CAS PubMed
.
- S. Milstien and S. Spiegel, Cancer Cell, 2006, 9, 148–150 CrossRef CAS PubMed
.
- J. M. Carr, S. Mahalingam, C. S. Bonder and S. M. Pitson, Rev. Med. Virol., 2013, 23, 73–84 CrossRef CAS PubMed
.
- J. G. Cyster and S. R. Schwab, Annu. Rev. Immunol., 2012, 30, 69–94 CrossRef CAS PubMed
.
- C.-F. Lee, A. Dang, E. Hernandez, R.-C. Pong, B. Chen, R. Sonavane, G. Raj, P. Kapur, H.-Y. Lin, S.-R. Wu, C.-J. Ko, U.-G. Lo, H.-Y. Lee, J.-T. Hsieh and M.-S. Lee, Oncogene, 2019, 38, 5580–5598 CrossRef CAS PubMed
.
- T. Sasatomi, Y. Suefuji, K. Matsunaga, H. Yamana, Y. Miyagi, Y. Araki, Y. Ogata, K. Itoh and K. Shirouzu, Cancer, 2002, 94, 1636–1641 CrossRef PubMed
.
- Y. Suefuji, T. Sasatomi, S. Shichijo, S. Nakagawa, H. Deguchi, T. Koga, T. Kameyama and K. Itoh, Br. J. Cancer, 2001, 84, 915–919 CrossRef PubMed
.
- N. Tsuda, K. Murayama, H. Ishida, K. Matsunaga, S. Komiya, K. Itoh and A. Yamada, J. Orthop. Res., 2001, 19, 346–351 CrossRef CAS PubMed
.
- Y. Miyagi, N. Imai, T. Sasatomi, A. Yamada, T. Mine, K. Katagiri, M. Nakagawa, A. Muto, S. Okouchi, H. Isomoto, K. Shirouzu, H. Yamana and K. Itoh, Clin. Cancer Res., 2001, 7, 3950–3962 CAS
.
- J. Gu, S. Shimba, N. Nomura and R. Reddy, Biochim. Biophys. Acta, Gene Struct. Expression., 1998, 1399, 1–9 CrossRef CAS PubMed
.
- J. A. Byrne, R. L. Balleine, M. Schoenberg Fejzo, J. Mercieca, Y.-E. Chiew, Y. Livnat, L. St Heaps, G. B. Peters, K. Byth, B. Y. Karlan, D. J. Slamon, P. Harnett and A. Defazio, Int. J. Cancer, 2005, 117, 1049–1054 CrossRef CAS PubMed
.
- E. Tiacci, P.-L. Orvietani, B. Bigerna, A. Pucciarini, G. L. Corthals, V. Pettirossi, M. P. Martelli, A. Liso, R. Benedetti, R. Pacini, N. Bolli, S. Pileri, K. Pulford, M. Gambacorta, A. Carbone, C. Pasquarello, A. Scherl, H. Robertson, M. T. Sciurpi, G. Alunni-Bistocchi, L. Binaglia, J. A. Byrne and B. Falini, Blood, 2005, 105, 2812–2820 CrossRef CAS PubMed
.
- M. Shehata, I. Bieche, R. Boutros, J. Weidenhofer, S. Fanayan, L. Spalding, N. Zeps, K. Byth, R. K. Bright, R. Lidereau and J. A. Byrne, Clin. Cancer Res., 2008, 14, 5050–5060 CrossRef CAS PubMed
.
- K. Clement, N. Viguerie, C. Poitou, C. Carette, V. Pelloux, C. A. Curat, A. Sicard, S. Rome, A. Benis, J.-D. Zucker, H. Vidal, M. Laville, G. S. Barsh, A. Basdevant, V. Stich, R. Cancello and D. Langin, FASEB J., 2004, 18, 1657–1669 CrossRef CAS PubMed
.
- K. Ashrafi, F. Y. Chang, J. L. Watts, A. G. Fraser, R. S. Kamath, J. Ahringer and G. Ruvkun, Nature, 2003, 421, 268–272 CrossRef CAS
.
- R. Wang, J. Xu, O. Saramäki, T. Visakorpi, W. M. Sutherland, J. Zhou, B. Sen, S. D. Lim, N. Mabjeesh, M. Amin, J.-T. Dong, J. A. Petros, P. S. Nelson, F. F. Marshall, H. E. Zhau and L. W. K. Chung, Cancer Res., 2004, 64, 1589–1594 CrossRef CAS
.
- N. Roslan, I. Bieche, R. K. Bright, R. Lidereau, Y. Chen and J. A. Byrne, Mol. Carcinog., 2014, 53, 807–819 CrossRef CAS PubMed
.
- M. A. Rubin, S. Varambally, R. Beroukhim, S. A. Tomlins, D. R. Rhodes, P. L. Paris, M. D. Hofer, M. Storz-Schweizer, R. Kuefer, J. A. Fletcher, B.-L. Hsi, J. A. Byrne, K. J. Pienta, C. Collins, W. R. Sellers and A. M. Chinnaiyan, Cancer Res., 2004, 64, 3814–3822 CrossRef CAS PubMed
.
- A. Kamili, N. Roslan, S. Frost, L. C. Cantrill, D. Wang, A. Della-Franca, R. K. Bright, G. E. Groblewski, B. K. Straub, A. J. Hoy, Y. Chen and J. A. Byrne, J. Cell Sci., 2015, 128, 3223–3238 CAS
.
- M. Abe, M. Matsuda, H. Kobayashi, Y. Miyata, Y. Nakayama, R. Komuro, A. Fukuhara and I. Shimomura, Arterioscler., Thromb., Vasc. Biol., 2008, 28, 871–877 CrossRef CAS PubMed
.
- L. Aguirre, E. Hijona, M. T. Macarulla, A. Gracia, I. Larrechi, L. Bujanda, L. Hijona and M. P. Portillo, J. Physiol. Pharmacol., 2013, 64, 281–288 CAS
.
- J. Li, Y. Dong, X. Lü, L. Wang, W. Peng, X. C. Zhang and Z. Rao, Protein Cell, 2013, 4, 548–561 CrossRef CAS PubMed
.
- M. Hiroyama and T. Takenawa, J. Biol. Chem., 1999, 274, 29172–29180 CrossRef CAS PubMed
.
- W. H. Moolenaar, K. Jalink and E. J. van Corven, Rev. Physiol., Biochem. Pharmacol., 1992, 119, 47–65 CAS
.
- T. Ando, H. Ishiguro, Y. Kuwabara, M. Kimura, A. Mitsui, H. Kurehara, N. Sugito, K. Tomoda, R. Mori, N. Takashima, R. Ogawa and Y. Fujii, Oncol. Rep., 2006, 15, 1551–1555 CAS
.
- G. B. Mills and W. H. Moolenaar, Nat. Rev. Cancer, 2003, 3, 582–591 CrossRef CAS PubMed
.
- D. C. Bennett and M. L. Lamoreux, Pigment Cell Res., 2003, 16, 333–344 CrossRef CAS PubMed
.
- N. Kory, G. A. Wyant, G. Prakash, J. uit De Bos, F. Bottanelli, M. E. Pacold, S. H. Chan, C. A. Lewis, T. Wang, H. R. Keys, Y. E. Guo and D. M. Sabatini, Science, 2018 DOI:10.1126/science.aat9528
.
- D. Xi, Y. He, Y. Sun, X. Gou, S. Yang, H. Mao and W. Deng, Mol. Biol. Rep., 2011, 38, 1883–1887 CrossRef CAS PubMed
.
- P. V. Röder, B. Wu, Y. Liu and W. Han, Exp. Mol. Med., 2016, 48, e219 CrossRef PubMed
.
- Z. Fu, E. R. Gilbert and D. Liu, Curr. Diabetes Rev., 2013, 9, 25–53 CrossRef PubMed
.
- Y. Perez-Riverol, J. Bai, C. Bandla, D. García-Seisdedos, S. Hewapathirana, S. Kamatchinathan, D. J. Kundu, A. Prakash, A. Frericks-Zipper, M. Eisenacher, M. Walzer, S. Wang, A. Brazma and J. A. Vizcaíno, Nucleic Acids Res., 2022, 50, D543–D552 CrossRef CAS PubMed
.
|
This journal is © The Royal Society of Chemistry 2023 |