DOI:
10.1039/D3FO00019B
(Paper)
Food Funct., 2023,
14, 4078-4091
(Poly)phenol intake, plant-rich dietary patterns and cardiometabolic health: a cross-sectional study†
Received
2nd January 2023
, Accepted 15th April 2023
First published on 17th April 2023
Abstract
Diet is an important modifiable risk factor for cardiometabolic diseases. Plant foods contain a complex mixture of nutrients and bioactive compounds such as (poly)phenols. Plant-rich dietary patterns have been associated with reduced cardiometabolic risk in epidemiological studies. However, studies have not fully considered (poly)phenols as a mediating factor in the relationship. A cross-sectional analysis was conducted in 525 healthy participants, aged 41.6 ± 18.3 years. Volunteers completed the validated European Prospective Investigation into Diet and Cancer (EPIC) Norfolk Food Frequency Questionnaire (FFQ). We investigated the associations between plant-rich dietary patterns, (poly)phenol intake, and cardiometabolic health. Positive associations were found between (poly)phenols and higher adherence to dietary scores, except for the unhealthy Plant-based Diet Index (uPDI), which was negatively associated with (poly)phenol intake. Correlations were significant for healthy PDI (hPDI), with positive associations with proanthocyanidins (r = 0.39, p < 0.01) and flavonols (r = 0.37, p < 0.01). Among dietary scores, Dietary Approaches to Stop Hypertension (DASH) showed negative associations with diastolic blood pressure (DBP), total cholesterol (TC), low-density lipoprotein cholesterol (LDL-C), and non-high-density lipoprotein cholesterol (Non-HDL-C) (stdBeta −0.12 to −0.10, p < 0.05). The Mediterranean-DASH Intervention for Neurodegenerative Delay (MIND) score was positively associated with flow-mediated dilation (FMD, stdBeta = 0.10, p = 0.02) and negatively associated with the 10-year Atherosclerotic Cardiovascular Disease (ASCVD) risk score (stdBeta = −0.12, p = 0.01). Higher intake of flavonoids, flavan-3-ols, flavan-3-ol monomers, theaflavins, and hydroxybenzoic acids (stdBeta: −0.31 to −0.29, p = 0.02) also showed a negative association with a 10-year ASCVD risk score. Flavanones showed significant associations with cardiometabolic markers such as fasting plasma glucose (FPG) (stdBeta = −0.11, p = 0.04), TC (stdBeta = −0.13, p = 0.03), and the Homeostasis Model Assessment (HOMA) of beta cell function (%B) (stdBeta = 0.18, p = 0.04). Flavanone intake was identified as a potential partial mediator in the negative association between TC and plant-rich dietary scores DASH, Original Mediterranean diet scores (O-MED), PDI, and hPDI (proportion mediated = 0.01% to 0.07%, p < 0.05). Higher (poly)phenol intake, particularly flavanone intake, is associated with higher adherence to plant-rich dietary patterns and favourable biomarkers of cardiometabolic risk indicating (poly)phenols may be mediating factors in the beneficial effects.
Introduction
Cardiovascular disease (CVD) and type 2 diabetes mellitus (T2DM) are leading causes of mortality and increased healthcare cost worldwide.1,2 Healthy lifestyle behaviours could significantly reduce the incidence of such diseases by more than 80%, potentially being more effective than pharmacotherapies.1 Diet in particular is one of the most important lifestyle factors known to affect cardiometabolic disease risk.1 The Global Burden of Disease study showed that diet quality improvement could potentially prevent one-fifth of deaths globally, among which CVD was the leading cause of diet-related deaths. This global study shows that the top factors associated to global death rate were low consumption of wholegrains, nuts and seeds, fruits, vegetables, omega-3 fatty acids and a high consumption of sodium.3 In agreement with this, high consumption of fruit and vegetables has been associated with lower disease risk in a large number of observational studies. A recent meta-analysis of 47 prospective studies unveiled a dose–response decrease in CVD risk associated with higher fruit and vegetable intake, with people consuming 800 g of fruits and vegetables each day having the lowest CVD risk.4 High adherence to healthy eating habits is therefore of great importance in promoting cardiometabolic health.
Free-living individuals consume a wide variety of diets, that are a combination of multiple nutrients and foods, which can interact with each other.5 The role of diet is hard to interpretate in relation to health status due to its complexity. Consequently, nutrition studies have been encouraged to focus on overall dietary patterns instead of the traditional approaches focusing on the health benefits of single nutrients or foods.6A priori dietary scores are pre-defined diet quality scores representing an individual's diet adherence to specific dietary recommendations and based on the current nutritional epidemiological knowledge of relationships between diet and disease risk factors. To date, multiple dietary score systems have been established with various scoring matrix weighing and measuring food and nutrient components separately.7,8
Plant-based diets are one of the most commonly used and proposed healthy dietary patterns, and typically this type of diet implies a high consumption of plant foods including fruits, vegetables, whole grains, nuts, legumes, vegetable oils, etc.9,10 There are many common plant-rich dietary patterns that have been associated with a positive effect on cardiometabolic health, e.g. Plant-based Diet Index (PDI),11 Mediterranean-DASH Intervention for Neurodegenerative Delay (MIND),12 Dietary Approaches to Stop Hypertension (DASH)13 and Mediterranean Diet (MED).14 (Poly)phenols are natural plant compounds widely abundant in fruits, vegetables, tea, coffee, wholegrains, and cocoa products.15 A large body of research has highlighted the protective role of dietary (poly)phenols on cardiometabolic health. Indeed, observational and intervention studies have found evidence that (poly)phenols are associated with a decrease in CVD mortality,16 an increase in flow-mediated dilation (FMD), and a reduction in blood pressure (BP).17 Although the mechanisms of action of (poly)phenols in the cardiovascular system are still not clear, it has been suggested they can act through the modulation of nitric oxide (NO) to maintain the homeostasis of the vascular system.11
Although evidence has shown the benefits of (poly)phenols on cardiometabolic health as one of the health-promoting factors of consuming plant-rich dietary patterns, there is still a lack of research viewing (poly)phenols as the possible potential mediator in the association of plant-rich dietary patterns with cardiometabolic health. For the various plant-rich dietary patterns, only red wine and olive oil in the MED diet have been investigated and reported to be beneficial to cardiometabolic health via the reduction in blood pressure and improvements in serum lipid profile and endothelial function.14,18,19
This study aims to investigate whether (poly)phenol intake mediates part of the benefits of plant-rich dietary patterns using a comprehensive cardiometabolic health marker panel including estimated atherosclerotic CVD (ASCVD), anthropometric, lipid profile, glucose metabolism, and vascular function in a cross-sectional study.
Methods
Study population
The baseline data of 525 healthy participants from nine clinical studies conducted at King's College London from 2017 to 2021 were included in this cross-sectional analysis (Ethics number, RESCM-17/18-5283; HR-15/16-3739; HR-17/18-5338; HR-18/19-9091; HR-18/19-8999; HR-17/18-5703; RESCM-18/19-9036; HR-17/18-5353; HR-19/20-14771; Trial registration number, NCT03434574; NCT03041961; NCT03592966; NCT04084457; NCT04179136; NCT03553225; NCT03995602; NCT03573414; NCT04276974). The studies were conducted according to the Declaration of Helsinki. All participants completed food frequency data and cardiometabolic measurements provided with informed written consent. A detailed flow chart is described in Fig. 1.
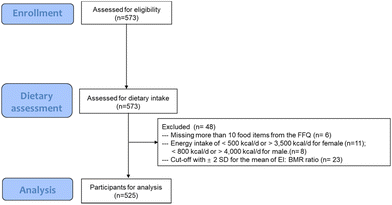 |
| Fig. 1 Flow chart of the cross-sectional study. | |
Dietary intake assessment
Each participant completed the validated European Prospective Investigation into Diet and Cancer (EPIC) Norfolk Food Frequency Questionnaire (FFQ).20 FFQ EPIC and Nutrition Tool for Analysis (FETA) software, which involved composition data of 290 foods from the UK food composition database McCance and Widdowson's ‘The Composition of Foods’ (5th edition) and its associated supplements21 were used in this research to estimate the nutrients and energy intake.
Participants who had more than 10 missing food items from the FFQ were classed as incomplete reporters and excluded from analyses. Females with fewer than 500 kcal d−1 and greater than 3500 kcal d−1 and males with fewer than an average of 800 kcal d−1 and greater than 4000 kcal d−1 were excluded as these values are considered physiological implausible.22 The Goldberg method23 was used as previously described24 to identify the inaccurate reporting of energy intake (EI) with a cut-off of ±2 standard deviations (SD) for the mean of EI
:
BMR ratio (estimated basal metabolic rate, determined by the Harris–Benedict equation). The data cleaning process is shown in Fig. 1.
Plant-rich dietary patterns generation
Five frequently reported cardiovascular health-related dietary patterns were chosen to evaluate the overall food constitution based on the predefined dietary scoring formula: DASH,25 PDI,26 Original Mediterranean Score (O-MED),27 Amended Mediterranean Score (A-MED)28 and MIND.29 The detailed food group descriptions and the calculation method for each dietary score are reported in ESI Tables 1–5.† The correlation between dietary scores was tested by Spearman's correlation.
(Poly)phenol intake assessment
The online open access Phenol–Explorer database,30 USDA database and several published papers31–53 were used to establish a home database in order to estimate the (poly)phenol content of each food item listed in the FFQ. Data coming from normal phase High Performance Liquid Chromatography (HPLC), chromatography, and chromatography after hydrolysis were selected. (Poly)phenol content data of compounds with sugar moieties attached were transformed into the corresponding amount of aglycones in order to be summarized with data from other sources. The procyanidin data analysed by normal phase HPLC were applied first, while data from chromatography was used when no data from the normal phase HPLC method was available. As for cooked foods, if only the raw data food source was available, the processed yield factor from the Phenol-Explorer database multiplied by the unprocessed raw food content was applied to determine the (poly)phenol content of cooked processed foods. If no yield factor was available, a factor of a similar food item or similar processing method of the same item was applied instead. (Poly)phenol content (mg d−1) was calculated using the estimated food intake (g d−1) multiplied by the corresponding (poly)phenol intake from the home database (mg per 100 g) and divided by 100. Total and subclasses of (poly)phenols, following the classification of Phenol-Explorer, were calculated by summing up all compounds within the group. The correlation between (poly)phenols was tested by Spearman's correlation.
Measurements of cardiometabolic health
In the present study, the following parameters were measured: flow-mediated dilation (FMD, %), pulse wave velocity (PWV, m s−1), augmentation index (AIx, %), peripheral and central blood pressure (BP, mmHg), overnight fasting plasma glucose (FPG, mmol L−1), total cholesterol (TC, mmol L−1), total triglycerides (TG, mmol L−1), high-, low-, and non-high-density lipoprotein cholesterol (HDL-C, LDL-C, non-HDL-C, mmol L−1), Homeostasis Model Assessment-insulin beta cell/insulin sensitivity/insulin resistance (HOMA2-B/HOMA2-S/HOMA2-IR) and 10-year Atherosclerotic Cardiovascular Disease (ASCVD) risk score.54
Fasting blood samples were collected in EDTA and heparin tubes (Greiner Bio-One Ltd) and were centrifuged (1700g; 15 min; 4 °C) immediately upon collection.55 Clinical chemistry parameters, including FPG, TC, TG, HDL-C, LDL-C, and non-HDL-C, were analysed according to standard procedures (Biochemistry Department, King's College Hospital, London and Affinity Biomarker Labs, London, United Kingdom).55,56 HOMA2-B/S/IR were calculated by HOMA2 online application (https://www.dtu.ox.ac.uk/homacalculator/).57
All vascular measurements were performed by a trained researcher after 15 minutes rest of the subjects. FMD assessment, as described previously55,58 was performed using a 12 MHz transducer (Vivid I; GE Healthcare, Berlin, Germany) with automatic edge-detection software (Brachial Analyzer; Medical Imaging Applications, Iowa City, IA). The specifically trained operators performed the analysis of all images across all studies. PWV, AIx, and central BP were measured by applanation tonometry using the SphygmoCor system (SMART Medical, Gloucestershire, UK) determined from measurements taken at the carotid and femoral artery.59 Office BP was taken with an automated brachial sphygmomanometer (OMRON HEM 705-CP digital BP monitor). All data analyses were conducted blinded and according to the same protocol across all studies.
CVD risk was estimated using the 10-year ASCVD risk score which is an algorithm used to estimate the cardiovascular risk of the individual using sex, ethnicity, age, smoking status, cholesterol levels, blood pressure, and history of diabetes calculated with the online ASCVD risk estimator (https://tools.acc.org/ASCVD-Risk-Estimator-Plus/). This score indicates the risk of developing hard ASCVD (coronary heart disease (CHD) death, nonfatal myocardial infarction, fatal or nonfatal stroke) in the next ten years.60 The 10-year ASCVD risk score is categorized as low-risk (<5%); borderline risk (5–7.4%); intermediate risk (7.5–19.9%) and high risk (≥20%) and was designed for patients aged 40–79.57
Assessment of covariates
The analysis was adjusted for several possible confounders: age,57 sex,61 ethnicity,62 smoking status,57 alcohol intake,57 energy intake,57 physical activity,57 BMI,61 and trial effect in the models as appropriate. Energy intake (kcal d−1) was estimated from FFQ. Information on age, sex, ethnicity, smoking status (smokers, non-smokers, or ex-smokers), and alcohol intake (unit per w) was collected at the screening visit. Physical activity level was assessed with The International Physical Activity Questionnaire Long Form (IPAQ-LF), which categorized participants into high, moderate, or low levels of activity based on the calculation result of metabolic equivalent (MET) minutes per week across walking, moderate-intensity, and vigorous-intensity activity within four domains (job-related physical activity; transportation physical activity; housework, house maintenance, and caring for family; recreation, sport, and leisure-time physical activity).63 Since volunteers participated in 9 different clinical trials, conducted at different times by different researchers, participants from the same trial were labelled with the same number. This sequence was included as a categoric variable “trial effect” with different trial numbers (1 to 9).
Statistics
Data distribution for dietary scores, (poly)phenols, and cardiometabolic markers was graphically explored and Shapiro–Wilk tested to assess normality. Log-transformation was applied to CSBP, FPG, HDL-C, LDL-C, non-HDL-C, TG, TC and used for statistical analysis when it was required. All association tests included were performed using R version 3.6.2.
The association between dietary patterns, (poly)phenols, and cardiometabolic markers was investigated using linear regression analysis. In the linear regression between (poly)phenols (explanatory variables) and dietary scores (response variables), two models were created: the Crude model and Model I which adjusted for energy intake and trial effect. For the analysis between (poly)phenols (explanatory variables) and cardiometabolic markers (response variables), three models were created: Crude model, Model I: adjusted for age, sex, ethnicity; Model II: Model I + adjustment for smoking status, energy intake, physical activity, and trial effect. Crude model, Model I, and Model II were also used to conduct linear regression analysis between dietary scores (explanatory variables) and cardiometabolic markers (response variables). DASH and PDI also adjusted for alcohol intake in Model II since these two dietary scores do not take alcohol consumption into account57 (ESI Tables 1–5†).
Mediation analysis was further employed with the R package of “mediation”64 to investigate the mediation effect of (poly)phenol intake on the relationship between various plant-rich dietary patterns and cardiometabolic health. The covariates of Model II were age, sex, ethnicity, physical activity, smoking status, energy intake, and trial effect (referred to Assessment of covariates) and Model III consisted of Model II + adjustment for BMI. We constructed a mediation model to quantify both the direct effect of dietary patterns on cardiometabolic health markers independent of (poly)phenol intake and the indirect (mediated) effects of dietary patterns that are mediated by its association with (poly)phenol intake (Fig. 2). The proportion of the association mediated by (poly)phenol intake (the ratio of indirect-to-total effect) was employed to quantify the magnitude of the mediator.65
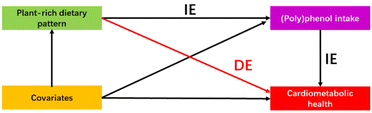 |
| Fig. 2 Mediating pathway of the association of plant-rich dietary patterns with cardiometabolic health. Direct acyclic graph of a structural model of mediation of the association between plant-rich dietary patterns and cardiometabolic health by (poly)phenol intake. DE indicates direct effects; IE indicates indirect effects. | |
The lm.beta R package was used for standardized regression coefficients (stdBeta). Linear associations results with p (adjusted by Benjamini & Hochberg False Discovery Rate, FDR) < 0.05 were considered statistically significant. Three main linear regression analyses we performed: (poly)phenol and dietary scores, (poly)phenol and cardiometabolic health markers, and dietary scores and cardiometabolic health markers. FDR adjustment was performed for each analysis separately. The ‘poolr’ R package were used as well for the Meff-based procedures to control FDR.66
Results
The demographic and cardiometabolic health characteristics of the participants including 227 males and 298 females are shown in Table 1. The average age of subjects was 41.5 (SD 18.3) years. The majority of subjects were from the White ethnic group (70.0%), engaged in high level of physical activity (71.2%), and were non-smokers (74.0%). Their average alcohol and energy intakes were 3.96 unit per w (SD 5.18) and 1610 kcal d−1 (SD 480), respectively.
Table 1 Demographic and cardiometabolic health characteristics of the study population (n = 525)
Characteristics |
Mean (SD)/n (%) |
Missingness (%) |
SBP, systolic blood pressure; DBP, diastolic blood pressure; PWV, pulse wave velocity; CSBP, central systolic blood pressure; CDBP, central diastolic blood pressure; AIx, augmentation index; FMD, flow-mediated dilation; FPG, fasting plasma glucose; TC, total cholesterol; TG, triglycerides; HDL-C, high-density lipoproteins cholesterol; LDL-C, low-density lipoproteins cholesterol; Non-HDL-C, non-high-density lipoproteins cholesterol; ASCVD, atherosclerotic cardiovascular disease; HOMA2-B/S/IR, homeostasis model assessment-insulin beta cell/insulin sensitivity/insulin resistance. |
Age (years) |
41.5 (18.3) |
0 |
Ethnicity |
|
0 |
White |
368 (70.0) |
|
Black |
25 (4.7) |
|
Asian |
112 (21.3) |
|
Mixed |
20 (3.8) |
|
Sex |
|
0 |
Male |
226 (43.0) |
|
Female |
299 (57.0) |
|
Physical activity level |
|
5.3 |
Low |
19 (3.8) |
|
Median |
124 (24.9) |
|
High |
354 (71.2) |
|
Smoking status |
|
0 |
Never |
389 (74.0) |
|
Ex-smoker |
109 (21.0) |
|
Current smoker |
27 (5.1) |
|
Body weight (kg) |
68.4 (11.3) |
0 |
BMI (kg m−2) |
23.8 (3.5) |
0 |
Alcohol intake (unit per w) |
3.96 (5.18) |
0 |
Energy intake (kcal d−1) |
1610 (480) |
0 |
Cardiometabolic markers
|
SBP (mmHg) |
116.29 (12.94) |
0 |
DBP (mmHg) |
74.63 (8.44) |
0 |
PWV (m s−1) |
6.46 (2.37) |
28.4 |
CSBP (mmHg) |
106.97 (14.30) |
24.4 |
CDBP (mmHg) |
75.93 (8.08) |
24.4 |
AIx (%) |
12.23 (16.52) |
24.5 |
FMD (%) |
5.92 (2.06) |
4.2 |
FPG (mmol L−1) |
4.86 (0.69) |
5.9 |
TC (mmol L−1) |
4.94 (1.06) |
5.5 |
TG (mmol L−1) |
0.88 (0.44) |
5.5 |
HDL-C (mmol L−1) |
1.74 (0.51) |
5.5 |
LDL-C (mmol L−1) |
3.15 (1.04) |
5.5 |
Non-HDL-C (mmol L−1) |
3.33 (1.06) |
5.5 |
10-year ASCVD risk score |
6.47 (7.30) |
55.8 |
HOMA2-B |
79.91 (37.95) |
69.0 |
HOMA2-S |
263.12 (371.20) |
69.0 |
HOMA2-IR |
0.70 (0.55) |
69.0 |
Plant-rich dietary patterns assessment
The mean and standard deviation of dietary scores among the overall population is described in Table 2. The study tested five dietary scores, DASH, MIND, O-MED, A-MED, and PDI (along with hPDI and uPDI). The correlation between dietary scores is reported in Fig. 3. Correlation coefficients ranged from −0.58 to 0.84, among which the strongest correlation was observed between A-MED and O-MED (r = 0.84) whereas the weakest correlation was found between PDI and uPDI (r = 0.08). Across the five dietary scores, differences were shown among the number, grouping, and amount of food components included in the scores (ESI Fig. 1†). Whole grain and vegetables were the most common elements across the dietary scores (shared in 4 dietary scores).
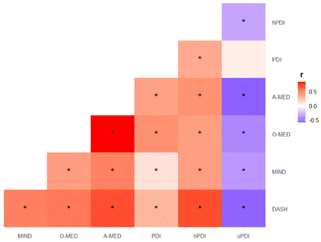 |
| Fig. 3 Correlation among dietary scores. The colour scale indicates the Spearman correlation coefficient between dietary scores. Red and blue illustrated respectively positive and negative correlations and colour intensity represented the degree of the coefficient. The asterisks showed significance (*fdr-adjusted, p < 0.05), DASH, dietary approaches to stop hypertension; O-MED, original mediterranean score; A-MED, amended mediterranean score; MIND, mediterranean-DASH intervention for neurodegenerative delay; PDI, plant-based diet index; hPDI, healthy plant-based diet index; uPDI, unhealthy plant-based diet index. | |
Table 2 Mean and standard deviation of dietary scores
Dietary scores |
Score range |
Mean |
SD |
DASH, dietary approaches to stop hypertension; MIND, mediterranean-DASH intervention for neurodegenerative delay; O-MED, original mediterranean score; A-MED, amended mediterranean score; PDI, plant-based diet index; hPDI, healthy plant-based diet index; uPDI, unhealthy plant-based diet index. |
DASH |
8–40 |
24.7 |
5.0 |
MIND |
0–15 |
8.6 |
1.5 |
O-MED |
0–9 |
4.3 |
1.8 |
A-MED |
0–9 |
4.4 |
2.0 |
PDI |
18–90 |
50.6 |
6.3 |
hPDI |
18–90 |
52.3 |
8.6 |
uPDI |
18–90 |
51.0 |
7.0 |
(Poly)phenol intake assessment
The intake of 22 individual classes of (poly)phenols among the participants is described in Table 3. The median total (poly)phenol intake was 1303.7 mg d−1 (IQR 1,230.0). Correlation among (poly)phenols was tested using the Spearman correlation method, and multiple tests were adjusted by Benjamin & Hochberg FDR. The majority of (poly)phenols (20 out of 22) showed a positive correlation with each other, among which phenolic acids showed the strongest positive correlations with hydroxycinnamic acids (r = 0.99, p < 0.01), as shown in Fig. 4.
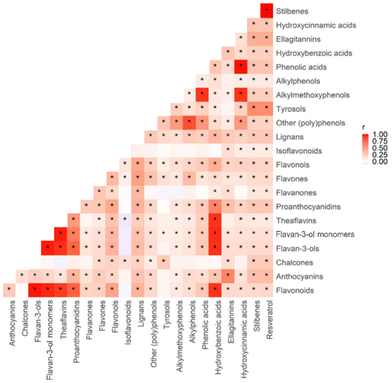 |
| Fig. 4 Correlation among different (poly)phenol classes. The colour scale indicated the Spearman correlation coefficient between (poly)phenols. Red and blue illustrated positive and negative correlations and colour intensity represented the degree of the coefficient. The asterisks showed significance (* fdr-adjusted, P < 0.05.). | |
Table 3 (Poly)phenol intake in the study population measured using food frequency questionnaire and the phenol-explorer database (mg d−1)
(Poly)phenol intake (mg d−1) |
Median |
IQR |
Other (poly)phenols including Curcuminoids, Furanocoumarins, Hydroxybenzaldehydes, Hydroxybenzoketones, Hydroxycinnamaldehydes, Hydroxycoumarins, Hydroxyphenylpropenes, Methoxyphenols, Naphtoquinones, and Phenolic terpenes.
|
Flavonoids |
452.1 |
711.8 |
Anthocyanins |
5.9 |
7.1 |
Chalcones |
0.003 |
0.002 |
Flavan-3-ols |
359.9 |
683.5 |
Flavan-3-ol monomers |
92.1 |
223.3 |
Theaflavins |
18.7 |
55.8 |
Proanthocyanidins |
126.0 |
110.3 |
Flavanones |
20.0 |
35.0 |
Flavones |
3.8 |
2.5 |
Flavonols |
47.9 |
32.4 |
Isoflavonoids |
2.1 |
6.9 |
Lignans |
1.6 |
1.2 |
Other (poly)phenolsa |
15.9 |
14.5 |
Tyrosols |
0.4 |
0.8 |
Alkylmethoxyphenols |
1.8 |
4.2 |
Alkylphenols |
9.3 |
10.0 |
Phenolic acids |
572.9 |
1173.2 |
Hydroxybenzoic acids |
40.7 |
70.0 |
Ellagitannins |
1.5 |
3.8 |
Hydroxycinnamic acids |
540.9 |
1199.7 |
Stilbenes |
0.1 |
0.1 |
Resveratrol |
0.1 |
0.1 |
Total (poly)phenol
|
1303.7
|
1230.0
|
Plant-rich dietary patterns and (poly)phenol intake
Associations between (poly)phenols and dietary scores are shown in Fig. 5. Most negative associations were found between uPDI and (poly)phenols ranging from r = −0.40 to −0.10 (p < 0.01). Correlations were particularly strong for hPDI, with positive associations with proanthocyanidins (r = 0.39, p < 0.01) and flavonols (r = 0.37, p < 0.01).
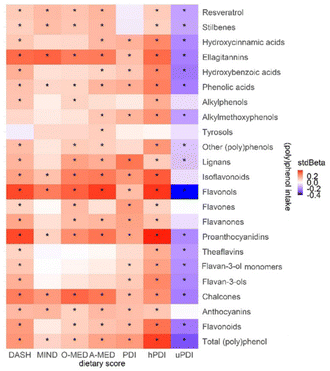 |
| Fig. 5 Associations between (poly)phenol intake and dietary scores (adjusted for energy intake and trial effect). Heatmap was plotted according to the standardized regression coefficients (stdBeta). The colour scale indicates the effect (stdBeta) of each (poly)phenol on dietary scores. Red and blue illustrate respectively positive and negative effects and colour intensity represents the degree of the effects. The asterisks showed significance (*FDR-adjusted, p < 0.05). DASH, dietary approaches to stop hypertension; O-MED/A-MED, original/amended mediterranean score; MIND, mediterranean-DASH intervention for neurodegenerative delay; PDI, plant-based diet index; hPDI/uPDI, healthy/unhealthy plant-based diet index. | |
Plant-rich dietary patterns and cardiometabolic health markers
The association between dietary scores and cardiometabolic markers using Model II is presented in Fig. 6. Negative associations were found between most dietary scores and cardiometabolic markers, except for uPDI, which had positive associations with CDBP (stdBeta = 0.11, p = 0.04) and DBP (stdBeta = 0.14, p < 0.01). FMD was also positively associated with MIND (stdBeta = 0.10, p = 0.02), indicating that an increase per MIND score would elevate FMD by 0.13 (95% CI, 0.02 to 0.24). DBP and CDBP were found to have a negative relationship, especially for A-MED (DBP, stdBeta = −0.13, p < 0.01; CDBP, stdBeta = −0.11, p = 0.03). For the increase per A-MED score, DBP and CDBP corresponded to decreases of 0.56 (95% CI, −0.91 to −0.21) mmHg and 0.50 (95% CI, −0.95 to −0.05) mmHg, respectively.
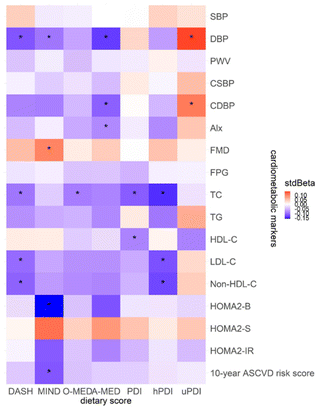 |
| Fig. 6 Associations between dietary scores and cardiometabolic markers of Model II (adjusted for age, sex, ethnicity, smoking status, energy intake, physical activity, and trial effect). Heatmap was plotted according to the standardized regression coefficients (stdBeta). The colour scale indicates the effect (stdBeta) of each dietary score on cardiometabolic markers. Red and blue illustrate respectively positive and negative effects and colour intensity represents the degree of the effects. The asterisks showed significance (*fdr-adjusted, p < 0.05). DASH, dietary approaches to stop hypertension; O-MED/A-MED, original/amended mediterranean score; MIND, mediterranean-DASH intervention for neurodegenerative delay; PDI, plant-based diet index; hPDI/uPDI, healthy/unhealthy plant-based diet index; SBP/DBP, systolic/diastolic blood pressure; PWV, pulse wave velocity; CSBP/CDBP, central SBP/DBP; AIx, augmentation index; FMD, flow-mediated dilation; FPG, fasting plasma glucose; TC, total cholesterol; TG, triglycerides; HDL/LDL/Non-HDL-C, high/low/non-high-density lipoproteins cholesterol; 10-year ASCVD risk score, 10-year atherosclerotic cardiovascular disease risk score; HOMA2-B/S/IR, homeostasis model assessment-insulin beta cell/insulin sensitivity/insulin resistance. | |
In terms of serum biochemical markers, LDL-C, Non-HDL-C, and TC all presented negative relationship with both DASH (LDL-C, stdBeta = −0.10, p = 0.02; Non-HDL-C, stdBeta = −0.11, p = 0.02; and TC, stdBeta = −0.10, p = 0.03) and hPDI (LDL-C, stdBeta = −0.11, p = 0.01; Non-HDL-C, stdBeta = −0.13, p = 0.01; TC, stdBeta = −0.14, p < 0.01). MIND also showed a negative correlation with the 10-year ASCVD risk score (stdBeta = −0.12, p = 0.01).
(Poly)phenol intake and cardiometabolic health markers
The association between (poly)phenol intake and cardiometabolic markers is presented in Fig. 7. In Model II, a negative association was found between CDBP and lignans, ellagitannins (stdBeta = −0.10, −0.13, p = 0.04), PWV, and tyrosols (stdBeta = −0.14, p = 0.03) as well as HOMA2-IR and ellagitannins (stdBeta = −0.20, p = 0.02). In addition, the 10-year ASCVD risk score shows a negative association with flavonoids (stdBeta = −0.29, p = 0.02), flavan-3-ols (stdBeta = −0.29, p = 0.02), flavan-3-ol monomers (stdBeta = −0.30, p = 0.02), theaflavins (stdBeta = −0.31, p = 0.02) and hydroxybenzoic acids (stdBeta = −0.29, p = 0.02). Flavanone intake has a significant association with FPG (stdBeta = −0.11, p = 0.04), TC (stdBeta = −0.13, p = 0.03), and HOMA2-B (stdBeta = 0.18, p = 0.04).
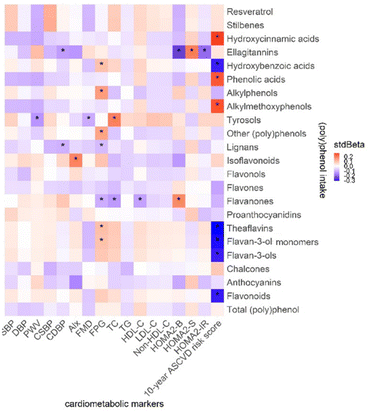 |
| Fig. 7 Associations between (poly)phenols and cardiometabolic markers of Model II (adjusted for age, sex, ethnicity, smoking status, energy intake, physical activity, and trial effect). Heatmap was plotted according to the standardized regression coefficients (stdBeta). The colour scale indicates the effect (stdBeta) of each (poly)phenol on cardiometabolic markers. Red and blue illustrate respectively positive and negative effects and colour intensity represents the degree of the effects. The asterisks showed significance (*FDR-adjusted, p < 0.05). SBP/DBP, systolic/diastolic blood pressure; PWV, pulse wave velocity; CSBP/CDBP, central SBP/DBP; AIx, augmentation index; FMD, flow-mediated dilation; FPG, fasting plasma glucose; TC, total cholesterol; TG, triglycerides; HDL/LDL/Non-HDL-C, high/low/non-high-density lipoproteins cholesterol; 10-year ASCVD risk score, 10-year atherosclerotic cardiovascular disease risk score; HOMA2-B/S/IR, homeostasis model assessment-insulin beta cell/insulin sensitivity/insulin resistance. | |
(Poly)phenol intake and (poly)phenol-rich food items
The intake amount of (poly)phenol-rich food items among the participants is shown in ESI Table 6.† Mean tea and coffee intake was 261.3 (SD 290.9) g d−1 and 182.7 (SD 208.2) g d−1, respectively. The contribution of each (poly)phenol-rich food item is presented in ESI Fig. 2.† Tea and Coffee contributed the most to the total (poly)phenol-rich food consumed, 29.2% and 20.4% respectively, followed by whole grains (9.6%), apple and apple juice (7.4%), citrus fruit and juice (7.1%), and pulses (5.4%). The other food items were lower than 5%, with olive oil contributing the lowest (0.02%).
The association between (poly)phenol intake and (poly)phenol-rich food items among the participants is shown in ESI Fig. 3.† Positive associations were mainly observed among (poly)phenols and (poly)phenol-rich foods. Correlations were particularly strong for flavanones and citrus fruit and juice (stdBeta = 0.99, p < 0.01), theaflavins, flavonoids, flavan-3-ols, flavan-3-ol monomers, hydroxybenzoic acids and tea (stdBeta = 0.97 to 0.99, p < 0.01), ellagitannins, and berries (stdBeta = 0.99, p < 0.01), stilbenes, resveratrol, and red wine (stdBeta = 0.96 to 0.97, p < 0.01).
Nutrients and fibre intake, plant-rich dietary patterns and cardiometabolic health markers
The intake of micro and macronutrients among the participants is described in Table S12.† Mean fibre intake was 16.15 (SD 7.03) g d−1. Associations between nutrients, plant-rich dietary patterns and cardiometabolic health markers scores are shown in ESI Fig. 4 and 5.† Negative associations were mainly observed between uPDI and nutrients ranging from −0.79 to −0.10 (p < 0.01). Correlations were strong for fibre, magnesium, potassium, and iron with positive associations with DASH, hPDI, A-MED and O-MED (r = 0.55 to 0.94, p < 0.01).
As for nutrients and cardiometabolic markers, fibre showed negative association with TC (stdBeta = −0.19, p < 0.01). Negative associations were also found between alpha carotene, beta carotene, total carotene and SBP and DBP (stdBeta = −0.11 to −0.19, p < 0.05). For 1 mg d−1 increase of alpha carotene, beta carotene and total carotene intake, SBP and DBP would decrease by 4 (95%CI, −6 to −0.1) mmHg, 1 (95%CI, −1 to −0.3) mmHg and 0.7 (95%CI, −1 to −0.3) mmHg for SBP; 3 (95%CI, −5 to −2) mmHg, 1 (95%CI, −1 to −0.4) mmHg and 0.6 (95%CI, −1 to −0.3) mmHg for DBP, respectively. Same association was also found between Vitamin C, total carbohydrate, glucose, and TC (Vitamin C, stdBeta = −0.14, p = 0.02; total carbohydrate, stdBeta = −0.26, p = 0.01; glucose, stdBeta = −0.14, p = 0.04). Glucose also showed negative associations with the 10-year ASCVD risk score (stdBeta = −0.06, p = 0.03). For 1 g d−1 increase of glucose, 10-year ASCVD risk score corresponded to 0.004 (95%CI, −0.006 to −0.001) decrease. Zinc showed a positive association with FMD (stdBeta = 0.21, p = 0.03), indicating that an increase of 1 mg d−1 zinc would elevate FMD by 0.14% (95%CI, 0.05 to 0.24) and saturated fatty acids (SFA) also showed a same positive association with TC (stdBeta = 0.18, p = 0.04).
Correlations between plant-rich dietary patterns, (poly)phenol intake, and cardiometabolic health
Based on the analysis between plant-rich dietary patterns, (poly)phenols, and cardiometabolic health markers of Model II and Model III, significant associations were chosen and tested for mediation. Fig. 8A presents the proportion of the association between plant-rich dietary patterns and cardiometabolic health markers mediated by individual (poly)phenols, ellagitannins, lignans and flavanones. Flavanone intake was found to be a mediator on the negative association between TC and the plant-rich dietary patterns DASH, O-MED, PDI, and hPDI (Proportion mediated: 9.0% to 16.9%, p < 0.05).
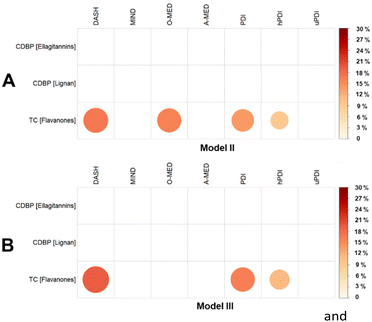 |
| Fig. 8 The proportion of the effects of plant-rich dietary patterns on cardiometabolic health mediated via individual (poly)phenols. The sequential red colour scale and the circle size indicate the proportion percentage (%) with significance calculated by dividing the indirect effect by the total effect of various (poly)phenols (p < 0.05). The Red colour intensity represents the degree of the percentage, and the mediator (poly)phenol is presented in “[]”. DASH, dietary approaches to stop hypertension; O-MED/A-MED, original/amended mediterranean score; MIND, mediterranean-DASH intervention for neurodegenerative delay; PDI, plant-based diet index; hPDI/uPDI, healthy/unhealthy plant-based diet index; CDBP, central diastolic blood pressure; TC, total cholesterol. | |
Mediation analysis based on the significant associations chosen from the analysis between plant-rich dietary patterns, (poly)phenol intake, and cardiometabolic health markers of Model III (Model II + BMI) is shown in Fig. 8B. Compared with the analysis of Model II, no mediation effect of lignan and ellagitannins was found, whereas the results of flavanones are still robust after adjusting for BMI (Proportion mediated for DASH, PDI and hPDI: 11.1% to 19.3%, p < 0.05).
Discussion
To our knowledge, this is the first study to investigate the mediating effect of (poly)phenols in the relationship between plant-rich dietary patterns and markers of cardiometabolic health in a group of healthy individuals living in the UK. Of the five dietary patterns, DASH showed the most beneficial associations with markers of vascular function and lipid metabolism (DBP, TC, LDL-C, and Non-HDL-C). Highly significant positive associations were found between (poly)phenol intake and all dietary patterns tested, except for uPDI, which had negative associations. Correlations were particularly strong for hPDI, with positive associations with flavonols and proanthocyanidins. Flavanones showed a potential mediation effect on the association between TC and the plant-rich dietary patterns DASH, O-MED, PDI, and hPDI.
The DASH diet was initially designed to reduce blood pressure.13 It encourages the intake of vegetables, fruits, whole grain, and low-fat dairy products and set limits for the consumption of red processed meat, sugars, sodium, fat, refined grains, and alcohol.67,68 As a result, this dietary pattern increases the intake of beneficial compounds, such as folate, calcium, vitamin C, K, magnesium, dietary fibre, and phytochemicals. These dietary components would all contribute to the protective effect on cardiometabolic health, for instance vascular function and lipid metabolism by multiple underlying mechanisms, such as anti-inflammatory effect,69 and improvements in endothelial function.70 The current study showed an inverse association between adherence to the DASH diet and DBP, TC, non-HDL-C, and LDL-C. These results are in agreement with past research.67
Not all plant foods show a protective effect on cardiometabolic health, and foods such as potatoes, sugar-sweetened beverages, refined grains, and sweets are often related to a higher risk of chronic diseases.11 This means that the term plant-based diet is not simply equal to a healthy diet.11 To address this, researchers graded plant-based foods into 2 different quality categories (high- and low-quality) based on nutrient profile and established the dietary pattern PDI with two sub-indexes, hPDI and uPDI.9,11 Plant food products are classified as having high nutritional quality, including whole grains, fruits, vegetables, tea, coffee, nuts, legumes, and vegetable oil. Food items such as refined grains, potatoes, and sugar-sweetened beverages in the PDI scoring system are treated as having low nutritional quality.9,11 The hPDI dietary pattern positively assesses the plant products with high quality and negatively weighs the plant products with low quality, whereas the uPDI diet evaluates low-quality plant food items positively and animal foods negatively.9,11 The beneficial effects of overall PDI and its healthy version hPDI on cardiometabolic health are reflected in many biomarkers, such as lipids (LDL-C/HDL-C/non-HDL-C) which were found in many randomised controlled trials and the association with incident CHD was positive for its unhealthy version uPDI.9,11,71 This evidence is in line with the present study, which showed a negative association between PDI and TC, hPDI, and non-HDL-C, LDL-C, TC. Adopting PDI and hPDI may provide meaningful support for the management of cardiometabolic health, and vice versa for uPDI.11 Moreover, amongst all of the plant-rich dietary patterns investigated, only uPDI showed a negative association with (poly)phenol intake, which may be due to the formulation of uPDI that positively scores plant food with low quality and negatively scores the animal food items and plant food of high quality.11 Many high-quality plant foods are rich in (poly)phenols, such as fruit, wholegrain, tea, coffee, legumes, etc. Some of the low-quality food items are overlapping with (poly)phenol-rich foods, such as fruit juices, however, the percentage is lower compared with the high-quality plant foods.
MIND and MED dietary patterns were also found to be associated with markers of cardiometabolic health. Adherence to the MIND dietary pattern was associated with lower DBP, higher FMD, and lower 10-year ASCVD risk score, while adherence to A-MED was associated with lower DBP, CDBP, and AIx. The O-MED dietary pattern was associated to lower levels of TC. Studies have supported the beneficial effect of MED and MIND diets on cardiometabolic health.1,12,14 The main difference between O-MED and A-MED is that A-MED excludes dairy (detrimental component in O-MED) and refined grains (cereals, beneficial component in O-MED) and includes wholegrains as a category.27,28 A-MED also includes pure fruit juice, and excludes starchy vegetables compared with O-MED components.57 Some studies suggest that consumption of refined grains may increase chronic disease risk,11 so this may partially explain the more favourable effect of A-MED compared with the O-MED in this research. The MIND diet is established originally from both MED and DASH diets, with several modifications based on the evidence existing on the effects of diet on dementia.71,72 For instance, an increasing number of animal and cohort studies have found that a higher intake of berries may be protective against age-related cognitive decline.73,74 As a result, the MIND diet has a special emphasis on berries29 and this may explain the positive association between FMD and adherence to the MIND diet in the present study, as many clinical studies have shown that consumption of berries and berry flavonoids like anthocyanins can improve endothelial function.75,76
(Poly)phenol-rich diets have been associated with a reduced risk of CVD, and higher (poly)phenol intake was found to have a reverse association with the prevalence of hypertension in populations at high CVD risk.77,78 When investigating associations between (poly)phenol intake and cardiometabolic health in the present study, several (poly)phenol, i.e., total flavonoids, theaflavins, flavan-3-ols, flavan-3-ol monomers, and hydroxybenzoic acids were also negatively linked with the 10-year ASCVD risk score, which is a continuous score that integrates several risk markers and translates it into a more tangible risk to experience CVD events.79 Flavonoids represents a large group of different subclasses of (poly)phenols, including flavan-3-ols, flavanones, anthocyanins, flavonols, flavones, and isoflavonoids.80 Studies have shown an association between a decrease in CVD mortality risk and higher consumption of dietary flavonoids.16 This favourable association with cardiovascular health markers was consistent in the present study with total flavonoids, its subclass flavan-3-ols, and theaflavins and flavan-3-ol monomers within the family of flavan-3-ols. Flavanones, another subgroup of flavonoids, are biologically active compounds that help decrease cardiovascular disease risk.81 In this study flavanones showed a significant mediation effect on the association between plant-rich dietary patterns DASH, O-MED, PDI, and hPDI, and lipid profile marker TC with the mediation effect proportion for nearly 20%. The flavanones-rich food, namely citrus fruit and juice81 only account for 7.12% of (poly)phenol-rich food intake in the present population. Considering its daily consumption (63.7 g d−1), its mediation proportion of plant-rich dietary patterns on lipid profile might be at a considerable level.
Hydroxybenzoic acids are present in many plant foods but they are also gut microbial metabolites of many types of (poly)phenols.82 Experimental models have shown that they may exert cardiovascular health benefits,82 however human data is lacking. A negative association between lignan intake, CDBP and FPG was also found. However, the mediation analysis did not show any significant mediating effects between plant-rich dietary patterns and CDBP. The main sources of lignans in the diet are seeds such as sesame and flaxseeds, but also fibre-rich wholegrain foods.83,84 The present study found that an increase in lignan intake of 100 mg was associated with reductions of 1 mmHg in CDBP, which is consistent with previous clinical trials showing improvements in blood pressure.85 To date, although accumulating evidence suggests that central pressure is a better predictor of future cardiovascular events than brachial blood pressure, direct evidence supporting that selective targeting long-term central pressure brings added benefit over and above the already established brachial artery pressure is still required.86,87 This study also found that an increase in lignan intake of 10 mg was associated with a reduction of 1.3 mmol L−1 in FPG, in line with previous clinical studies showing beneficial effects of lignans on plasma glucose.88 The associations with reductions in FPG are clinically relevant, as an increase in 1 mmol L−1 FPG was found to be associated with a 17% increase in cardiovascular event risk.89
In the present study, stilbenes, resveratrol, and some flavonoids such as flavonols, flavones, isoflavones and anthocyanins did not show any significant association with markers of cardiometabolic health. This may be due to various reasons, such as the low intake in this study or the potential bias limitation of self-reported diet by FFQ.90
Our work is limited by the observational nature of the cross-sectional study. It does not allow us to determine causal relationships between plant-rich dietary patterns, (poly)phenol intake, and cardiometabolic health. In addition, this data-driven research is based on multiple correlations and linear regressions, which may limit the interpretation of the results. Another notable limitation is the use of self-reported FFQs for (poly)phenol assessment. The EPIC-Norfolk FFQ used in the present study was not designed or validated to assess (poly)phenol intake but only for the assessment of nutrients and food groups.91 Further longitudinal and intervention studies are required to confirm the findings presented here.
Thus far, the project has considered the association between cardiometabolic health, (poly)phenol intake, and various dietary patterns through self-reported FFQ data. (Poly)phenols, especially flavonoids intake, are likely to be implicated in the physiological pathways between plant-rich dietary patterns and markers of cardiometabolic health. However, due to the collinearity between (poly)phenols and micronutrients, further research is needed to understand potential independent and synergistic effects of (poly)phenols and other nutrients on cardiometabolic health.
Conclusions
In conclusion, higher (poly)phenol intake is associated with higher adherence to plant-rich dietary patterns and more favourable cardiometabolic health profiles. Flavanones might be a potential mediator in this favourable effect on lipid profile. DASH showed the strongest association with cardiometabolic health among all dietary scores investigated. Our results indicate that the beneficial association of plant-rich dietary patterns on cardiometabolic health may partially be driven by the (poly)phenols from the plant-rich food items within the habitual diet. Further dietary intervention studies are still required to explore the associations concerning health and cardiovascular diseases to provide sustainable evidence support for plant-rich diet recommendations.
Author contributions
Conceptualisation, Y.L., A.R.M., and R.G.; data curation, Y.L., X.M. Y.X., M.L.S. and H.W.; formal analysis and writing – original draft, Y.L.; methodology, Y.L., Y.X., A.R.M. and R.G.; writing – review & editing; supervision, A.R.M., R.G., C.H., P.D., and C.N.; project administration and funding acquisition, A.R.M.
Conflicts of interest
There are no conflicts to declare.
Acknowledgements
We extend our highest gratitude to the participants of all the clinical studies. Y. L., Y. X. and X. M. are funded by the King's-China Scholarship Council (K-CSC) joint scholarship.
References
- H. Kahleova, J. Salas-Salvado, D. Rahelic, C. W. Kendall, E. Rembert and J. L. Sievenpiper, Dietary Patterns and Cardiometabolic Outcomes in Diabetes: A Summary of Systematic Reviews and Meta-Analyses, Nutrients, 2019, 11(9), 2209 CrossRef CAS PubMed.
- D. Mozaffarian, Dietary and Policy Priorities for Cardiovascular Disease, Diabetes, and Obesity: A Comprehensive Review, Circulation, 2016, 133, 187–225 CrossRef CAS PubMed.
- G. B. D. D. Collaborators, Health effects of dietary risks in 195 countries, 1990–2017: a systematic analysis for the Global Burden of Disease Study 2017, Lancet, 2019, 393, 1958–1972 CrossRef.
- J. Zhan, Y. J. Liu, L. B. Cai, F. R. Xu, T. Xie and Q. Q. He, Fruit and vegetable consumption and risk of cardiovascular disease: A meta-analysis of prospective cohort studies, Crit. Rev. Food Sci. Nutr., 2017, 57, 1650–1663 CrossRef.
- L. H. Skibsted, Mineral nutrient interaction: Improving bioavailability of calcium and iron, Food Sci. Biotechnol., 2016, 25, 1233–1241 CrossRef CAS PubMed.
- F. B. Hu, Dietary pattern analysis: a new direction in nutritional epidemiology, Curr. Opin. Lipidol., 2002, 13, 3–9 CrossRef CAS.
- A. Gil, E. Martinez de Victoria and J. Olza, Indicators for the evaluation of diet quality, Nutr. Hosp., 2015, 31(Suppl. 3), 128–144 Search PubMed.
- D. Panagiotakos, α-priori versus α-posterior methods in dietary pattern analysis: a review in nutrition epidemiology, Nutr. Bull., 2008, 33, 311–315 CrossRef.
- A. Satija and F. B. Hu, Plant-based diets and cardiovascular health, Trends Cardiovasc. Med., 2018, 28, 437–441 CrossRef.
- E. A. Trautwein and S. McKay, The Role of Specific Components of a Plant-Based Diet in Management of Dyslipidemia and the Impact on Cardiovascular Risk, Nutrients, 2020, 12(9), 2671 CrossRef CAS PubMed.
- E. C. Hemler and F. B. Hu, Plant-Based Diets for Cardiovascular Disease Prevention: All Plant Foods Are Not Created Equal, Curr. Atheroscler. Rep., 2019, 21, 18 CrossRef.
- M. Golzarand, P. Mirmiran and F. Azizi, Adherence to the MIND diet and the risk of cardiovascular disease in adults: a cohort study, Food Funct., 2022, 13, 1651–1658 RSC.
- L. J. Appel, T. J. Moore, E. Obarzanek, W. M. Vollmer, L. P. Svetkey, F. M. Sacks, G. A. Bray, T. M. Vogt, J. A. Cutler, M. M. Windhauser, P. H. Lin and N. Karanja, A clinical trial of the effects of dietary patterns on blood pressure. DASH Collaborative Research Group, N. Engl. J. Med., 1997, 336, 1117–1124 CrossRef CAS PubMed.
- P. Ditano-Vazquez, J. D. Torres-Pena, F. Galeano-Valle, A. I. Perez-Caballero, P. Demelo-Rodriguez, J. Lopez-Miranda, N. Katsiki, J. Delgado-Lista and L. A. Alvarez-Sala-Walther, The Fluid Aspect of the Mediterranean Diet in the Prevention and Management of Cardiovascular Disease and Diabetes: The Role of Polyphenol Content in Moderate Consumption of Wine and Olive Oil, Nutrients, 2019, 11(11), 2833 CrossRef CAS PubMed.
- J. A. Rothwell, V. Knaze and R. Zamora-Ros, Polyphenols: dietary assessment and role in the prevention of cancers, Curr. Opin. Clin. Nutr. Metab. Care, 2017, 20, 512–521 CrossRef CAS PubMed.
- G. Grosso, A. Micek, J. Godos, A. Pajak, S. Sciacca, F. Galvano and E. L. Giovannucci, Dietary Flavonoid and Lignan Intake and Mortality in Prospective Cohort Studies: Systematic Review and Dose-Response Meta-Analysis, Am. J. Epidemiol., 2017, 185, 1304–1316 CrossRef.
- L. Hooper, C. Kay, A. Abdelhamid, P. A. Kroon, J. S. Cohn, E. B. Rimm and A. Cassidy, Effects of chocolate, cocoa, and flavan-3-ols on cardiovascular health: a systematic review and meta-analysis of randomized trials, Am. J. Clin. Nutr., 2012, 95, 740–751 CrossRef CAS PubMed.
- S. Martinotti, G. Bonsignore, M. Patrone and E. Ranzato, Mediterranean Diet Polyphenols: Anthocyanins and Their Implications for Health, Mini-Rev. Med. Chem., 2021, 21, 1692–1700 CrossRef CAS.
- E. Scoditti, N. Calabriso, M. Massaro, M. Pellegrino, C. Storelli, G. Martines, R. De Caterina and M. A. Carluccio, Mediterranean diet polyphenols reduce inflammatory angiogenesis through MMP-9 and COX-2 inhibition in human vascular endothelial cells: a potentially protective mechanism in atherosclerotic vascular disease and cancer, Arch. Biochem. Biophys., 2012, 527, 81–89 CrossRef CAS.
- S. A. Bingham, A. A. Welch, A. McTaggart, A. A. Mulligan, S. A. Runswick, R. Luben, S. Oakes, K. T. Khaw, N. Wareham and N. E. Day, Nutritional methods in the European Prospective Investigation of Cancer in Norfolk, Public Health Nutr., 2001, 4, 847–858 CrossRef CAS PubMed.
- A. A. Mulligan, R. N. Luben, A. Bhaniani, D. J. Parry-Smith, L. O'Connor, A. P. Khawaja, N. G. Forouhi, K. T. Khaw and E. P.-N. F. Study, A new tool for converting food frequency questionnaire data into nutrient and food group values: FETA research methods and availability, BMJ Open, 2014, 4, e004503 CrossRef.
- J. C. Banna, M. A. McCrory, M. K. Fialkowski and C. Boushey, Examining Plausibility of Self-Reported Energy Intake Data: Considerations for Method Selection, Front. Nutr., 2017, 4, 45 CrossRef PubMed.
- P. S. Oliveira, J. Levy, E. Carli, I. J. M. Bensenor, P. A. Lotufo, R. A. Pereira, E. M. Yokoo, R. Sichieri, S. P. Crispim and D. M. L. Marchioni, Estimation of underreporting of energy intake using different methods in a subsample of the ELSA-Brasil study, Cad. Saude Publica, 2022, 38, e00249821 CrossRef PubMed.
- X. Ma, Y. Li, Y. Xu, R. Gibson, C. Williams, A. J. Lawrence, C. Nosarti, P. Dazzan and A. Rodriguez-Mateos, Plant-based dietary patterns and their association with mood in healthy individuals, Food Funct., 2023, 14, 2326–2337 RSC.
- T. T. Fung, S. E. Chiuve, M. L. McCullough, K. M. Rexrode, G. Logroscino and F. B. Hu, Adherence to a DASH-style diet and risk of coronary heart disease and stroke in women, Arch. Intern. Med., 2008, 168, 713–720 CrossRef PubMed.
- A. Satija, S. N. Bhupathiraju, D. Spiegelman, S. E. Chiuve, J. E. Manson, W. Willett, K. M. Rexrode, E. B. Rimm and F. B. Hu, Healthful and Unhealthful Plant-Based Diets and the Risk of Coronary Heart Disease in U.S. Adults, J. Am. Coll. Cardiol., 2017, 70, 411–422 CrossRef PubMed.
- A. Trichopoulou, T. Costacou, C. Bamia and D. Trichopoulos, Adherence to a Mediterranean diet and survival in a Greek population, N. Engl. J. Med., 2003, 348, 2599–2608 CrossRef PubMed.
- T. T. Fung, M. L. McCullough, P. K. Newby, J. E. Manson, J. B. Meigs, N. Rifai, W. C. Willett and F. B. Hu, Diet-quality scores and plasma concentrations of markers of inflammation and endothelial dysfunction, Am. J. Clin. Nutr., 2005, 82, 163–173 CrossRef CAS.
- M. C. Morris, C. C. Tangney, Y. Wang, F. M. Sacks, L. L. Barnes, D. A. Bennett and N. T. Aggarwal, MIND diet slows cognitive decline with aging, Alzheimers Dement., 2015, 11, 1015–1022 CrossRef PubMed.
- J. A. Rothwell, J. Pérez-Jiménez, V. Neveu, A. Medina-Ramon, N. M'Hiri, P. Garcia Lobato, C. Manach, K. Knox, R. Eisner, D. Wishart and A. Scalbert, Phenol-Explorer 3.0: a major update of the Phenol-Explorer database to incorporate data on the effects of food processing on polyphenol content, Database, 2013 DOI:10.1093/database/bat070.
- S. Bhagwat and D. B. Haytowitz, USDA Database for the Flavonoid Content of Selected Foods, 2015 DOI:10.15482/USDA.ADC/1324465.
- S. Bhagwat and D. B. Haytowitz, USDA Database for the Isoflavone Content of Selected Foods, 2015 DOI:10.15482/USDA.ADC/1324538.
- S. Bhagwat and D. B. Haytowitz, USDA Database for the Proanthocyanidin Content of Selected Foods, 2015 DOI:10.15482/USDA.ADC/1324621.
- J. I. Alonso-Esteban, J. Pinela, A. Ciric, R. C. Calhelha, M. Sokovic, I. Ferreira, L. Barros, E. Torija-Isasa and M. C. Sanchez-Mata, Chemical composition and biological activities of whole and dehulled hemp (Cannabis sativa L.) seeds, Food Chem., 2022, 374, 131754 CrossRef CAS PubMed.
- L. Alvarez-Jubete, H. Wijngaard, E. K. Arendt and E. Gallagher, Polyphenol composition and in vitro antioxidant activity of amaranth, quinoa buckwheat and wheat as affected by sprouting and baking, Food Chem., 2010, 119, 770–778 CrossRef CAS.
- R. L. Bertin, L. V. Gonzaga, G. D. C. Borges, M. S. Azevedo, H. F. Maltez, M. Heller, G. A. Micke, L. B. B. Tavares and R. Fett, Nutrient composition and, identification/quantification of major phenolic compounds in Sarcocornia ambigua (Amaranthaceae) using HPLC-ESI-MS/MS, Food Res. Int., 2014, 55, 404–411 CrossRef CAS.
- H. Y. Cai, Q. Zhang, L. Z. Shen, J. Luo, R. Y. Zhu, J. W. Mao, M. J. Zhao and C. G. Cai, Phenolic profile and antioxidant activity of Chinese rice wine fermented with different rice materials and starters, LWT – Food Sci. Technol., 2019, 111, 226–234 CrossRef CAS.
- A. V. Carvalho, T. F. F. da Silveira, R. D. Mattietto, M. D. P. de Oliveira and H. T. Godoy, Chemical composition and antioxidant capacity of acai (Euterpe oleracea) genotypes and commercial pulps, J. Sci. Food Agric., 2017, 97, 1467–1474 CrossRef CAS PubMed.
- N. Cicero, A. Albergamo, A. Salvo, G. D. Bua, G. Bartolomeo, V. Mangano, A. Rotondo, V. Di Stefano, G. Di Bella and G. Dugo, Chemical characterization of a variety of cold-pressed gourmet oils available on the Brazilian market, Food Res. Int., 2018, 109, 517–525 CrossRef CAS.
- Q. H. Gao, C. S. Wu, J. G. Yu, M. Wang, Y. J. Ma and C. L. Li, Textural Characteristic, Antioxidant Activity, Sugar, Organic Acid, and Phenolic Profiles of 10 Promising Jujube (Ziziphus jujuba Mill.) Selections, J. Food Sci., 2012, 77, C1218–C1225 CrossRef CAS.
- M. Gundogdu, Determination of antioxidant capacities and biochemical compounds of Berberis vulgaris L. fruits, Adv. Environ. Biol., 2013, 7, 344–348 CAS.
- M. A. Hassan, T. Xu, Y. Tian, Y. Zhong, F. A. Z. Ali, X. Yang and B. Lu, Health benefits and phenolic compounds of Moringa oleifera leaves: A comprehensive review, Phytomedicine, 2021, 93, 153771 CrossRef PubMed.
- A. N. Karunasiri, M. Gunawardane, C. M. Senanayake, N. Jayathilaka and K. N. Seneviratne, Antioxidant and Nutritional Properties of Domestic and Commercial Coconut Milk Preparations, Int. J. Food Sci., 2020, 2020, 3489605 Search PubMed.
- M. Kaspar, T. Bajer, P. Bajerova and P. Cesla, Comparison of Phenolic Profile of Balsamic Vinegars Determined Using Liquid and Gas Chromatography Coupled with Mass Spectrometry, Molecules, 2022, 27(4), 1356 CrossRef CAS PubMed.
- M. Y. Kim, P. Seguin, J. K. Ahn, J. J. Kim, S. C. Chun, E. H. Kim, S. H. Seo, E. Y. Kang, S. L. Kim, Y. J. Park, H. M. Ro and I. M. Chung, Phenolic compound concentration and antioxidant activities of edible and medicinal mushrooms from Korea, J. Agric. Food Chem., 2008, 56, 7265–7270 CrossRef CAS PubMed.
- Q. Lv, F. L. Luo, X. Y. Zhao, Y. Liu, G. B. Hu, C. D. Sun, X. Li and K. S. Chen, Identification of Proanthocyanidins from Litchi (Litchi chinensis Sonn.) Pulp by LC-ESI-Q-TOF-MS and Their Antioxidant
Activity, PLoS One, 2015, 10(3), e0120480 CrossRef PubMed.
- N. Miceli, A. Trovato, A. Marino, V. Bellinghieri, A. Melchini, P. Dugo, F. Cacciola, P. Donato, L. Mondello, A. Guvenc, R. De Pasquale and M. F. Taviano, Phenolic composition and biological activities of Juniperus drupacea Labill. berries from Turkey, Food Chem. Toxicol., 2011, 49, 2600–2608 CrossRef CAS.
- A. Mocan, F. Cairone, M. Locatelli, F. Cacciagrano, S. Carradori, D. C. Vodnar, G. Crisan, G. Simonetti and S. Cesa, Polyphenols from Lycium barbarum (Goji) Fruit European Cultivars at Different Maturation Steps: Extraction, HPLC-DAD Analyses, and Biological Evaluation, Antioxidants, 2019, 8(11), 562 CrossRef CAS PubMed.
- W. C. B. Muala, Z. S. C. Desobgo and N. E. Jong, Optimization of extraction conditions of phenolic compounds from Cymbopogon citratus and evaluation of phenolics and aroma profiles of extract, Heliyon, 2021, 7, e06744 CrossRef CAS PubMed.
- P. S. Prasanthi, N. Naveena, M. V. Rao and K. Bhaskarachary, Compositional variability of nutrients and phytochemicals in corn after processing, J. Food Sci. Technol., 2017, 54, 1080–1090 CrossRef CAS PubMed.
- M. J. Rahman, A. C. de Camargo and F. Shahidi, Phenolic and polyphenolic profiles of chia seeds and their in vitro biological activities, J. Funct. Foods, 2017, 35, 622–634 CrossRef CAS.
- A. Rueda, C. Samaniego-Sanchez, M. Olalla, R. Gimenez, C. Cabrera-Vique, I. Seiquer and L. Lara, Combination of Analytical and Chemometric Methods as a Useful Tool for the Characterization of Extra Virgin Argan Oil and Other Edible Virgin Oils. Role of Polyphenols and Tocopherols, J. AOAC Int., 2016, 99, 489–494 CrossRef CAS PubMed.
- L. Xu, D. Bin and B. J. Xu, A systematic, comparative study on the beneficial health components and antioxidant activities of commercially fermented soy products marketed in China, Food Chem., 2015, 174, 202–213 CrossRef CAS PubMed.
- D. M. Lloyd-Jones, M. D. Huffman, K. N. Karmali, D. M. Sanghavi, J. S. Wright, C. Pelser, M. Gulati, F. A. Masoudi and D. C. Goff Jr., Estimating Longitudinal Risks and Benefits From Cardiovascular Preventive Therapies Among Medicare Patients: The Million Hearts Longitudinal ASCVD Risk Assessment Tool: A Special Report From the American Heart Association and American College of Cardiology, Circulation, 2017, 135, e793–e813 CrossRef PubMed.
- G. Istas, E. Wood, M. Le Sayec, C. Rawlings, J. Yoon, V. Dandavate, D. Cera, S. Rampelli, A. Costabile, E. Fromentin and A. Rodriguez-Mateos, Effects of aronia berry (poly)phenols on vascular function and gut microbiota: a double-blind randomized controlled trial in adult men, Am. J. Clin. Nutr., 2019, 110, 316–329 CrossRef PubMed.
- A. Cheok, Y. Xu, Z. Zhang, P. W. Caton and A. Rodriguez-Mateos, Betalain-rich dragon fruit (pitaya) consumption improves vascular function in men and women: a double-blind, randomized controlled crossover trial, Am. J. Clin. Nutr., 2022, 115, 1418–1431 CrossRef PubMed.
- O. Mompeo, S. E. Berry, T. D. Spector, C. Menni, M. Mangino and R. Gibson, Differential associations between a priori diet quality scores and markers of cardiovascular health in women: cross-sectional analyses from TwinsUK, Br. J. Nutr., 2021, 126, 1017–1027 CrossRef CAS PubMed.
- A. Rodriguez-Mateos, C. Rendeiro, T. Bergillos-Meca, S. Tabatabaee, T. W. George, C. Heiss and J. P. Spencer, Intake and time dependence of blueberry flavonoid-induced improvements in vascular function: a randomized, controlled, double-blind, crossover intervention study with mechanistic insights into biological activity, Am. J. Clin. Nutr., 2013, 98, 1179–1191 CrossRef CAS PubMed.
- L. M. Van Bortel, S. Laurent, P. Boutouyrie, P. Chowienczyk, J. K. Cruickshank, T. De Backer, J. Filipovsky, S. Huybrechts, F. U. Mattace-Raso, A. D. Protogerou, G. Schillaci, P. Segers, S. Vermeersch, T. Weber, Artery Society, European Society of Hypertension Working Group on Vascular Structure and Function and European Network for Noninvasive Investigation of Large Arteries, Expert consensus document on the measurement of aortic stiffness in daily practice using carotid-femoral pulse wave velocity, J. Hypertens., 2012, 30, 445–448 CrossRef CAS PubMed.
- D. M. Lloyd-Jones, L. T. Braun, C. E. Ndumele, S. C. Smith Jr., L. S. Sperling, S. S. Virani and R. S. Blumenthal, Use of Risk Assessment Tools to Guide Decision-Making in the Primary Prevention of Atherosclerotic Cardiovascular Disease: A Special Report From the American Heart Association and American College of Cardiology, J. Am. Coll. Cardiol., 2019, 73, 3153–3167 CrossRef PubMed.
- Z. Xu, L. M. Steffen, E. Selvin and C. M. Rebholz, Diet quality, change in diet quality and risk of incident CVD and diabetes, Public Health Nutr., 2020, 23, 329–338 CrossRef PubMed.
- Z. Shan, Y. Li, M. Y. Baden, S. N. Bhupathiraju, D. D. Wang, Q. Sun, K. M. Rexrode, E. B. Rimm, L. Qi, W. C. Willett, J. E. Manson, Q. Qi and F. B. Hu, Association Between Healthy Eating Patterns and Risk of Cardiovascular Disease, JAMA Intern. Med., 2020, 180, 1090–1100 CrossRef PubMed.
- C. L. Craig, A. L. Marshall, M. Sjostrom, A. E. Bauman, M. L. Booth and B. E. Ainsworth,
et al. International physical activity questionnaire: 12-country reliability and validity, Med. Sci. Sports Exercise, 2003, 35, 1381–1395 CrossRef PubMed.
-
K. Imai, L. Keele, D. Tingley and T. Yamamoto, Causal Mediation Analysis Using R, Lecture Notes in Statistics, Advances in Social Science Research Using R, ed. H. D. Vinod, Springer, New York, 2010, pp. 129–154 Search PubMed.
- A. Dregan, L. Rayner, K. A. S. Davis, I. Bakolis, J. Arias de la Torre, J. Das-Munshi, S. L. Hatch, R. Stewart and M. Hotopf, Associations Between Depression, Arterial Stiffness, and Metabolic Syndrome Among Adults in the UK Biobank Population Study: A Mediation Analysis, JAMA Psychiatry, 2020, 77, 598–606 CrossRef PubMed.
- J. Li and L. Ji, Adjusting multiple testing in multilocus analyses using the eigenvalues of a correlation matrix, Heredity, 2005, 95, 221–227 CrossRef CAS PubMed.
- M. Siervo, J. Lara, S. Chowdhury, A. Ashor, C. Oggioni and J. C. Mathers, Effects of the Dietary Approach to Stop Hypertension (DASH) diet on cardiovascular risk factors: a systematic review and meta-analysis, Br. J. Nutr., 2015, 113, 1–15 CrossRef CAS PubMed.
- A. P. Campbell, DASH Eating Plan: An Eating Pattern for Diabetes Management, Diabetes Spectr., 2017, 30, 76–81 CrossRef PubMed.
- L. Azadbakht, P. J. Surkan, A. Esmaillzadeh and W. C. Willett, The Dietary Approaches to Stop Hypertension eating plan affects C-reactive protein, coagulation abnormalities, and hepatic function tests among type 2 diabetic patients, J. Nutr., 2011, 141, 1083–1088 CrossRef CAS PubMed.
- J. A. Blumenthal, M. A. Babyak, A. Hinderliter, L. L. Watkins, L. Craighead, P. H. Lin, C. Caccia, J. Johnson, R. Waugh and A. Sherwood, Effects of the DASH diet alone and in combination with exercise and weight loss on blood pressure and cardiovascular biomarkers in men and women with high blood pressure: the ENCORE study, Arch. Intern. Med., 2010, 170, 126–135 CrossRef PubMed.
- H. Cena and P. C. Calder, Defining a Healthy Diet: Evidence for The Role of Contemporary Dietary Patterns in Health and Disease, Nutrients, 2020, 12(2), 334 CrossRef PubMed.
- W. Marcason, What Are the Components to the MIND Diet?, J. Acad. Nutr. Diet., 2015, 115, 1744 CrossRef PubMed.
- E. E. Devore, J. H. Kang, M. M. Breteler and F. Grodstein, Dietary intakes of berries and flavonoids in relation to cognitive decline, Ann. Neurol., 2012, 72, 135–143 CrossRef CAS PubMed.
- L. M. Willis, B. Shukitt-Hale and J. A. Joseph, Recent advances in berry supplementation and age-related cognitive decline, Curr. Opin. Clin. Nutr. Metab. Care, 2009, 12, 91–94 CrossRef CAS PubMed.
- A. Rodriguez-Mateos, G. Istas, L. Boschek, R. P. Feliciano, C. E. Mills, C. Boby, S. Gomez-Alonso, D. Milenkovic and C. Heiss, Circulating Anthocyanin Metabolites Mediate Vascular Benefits of Blueberries: Insights From Randomized Controlled Trials, Metabolomics, and Nutrigenomics, J. Gerontol., Ser. A, 2019, 74, 967–976 CrossRef CAS.
- A. Rodriguez-Mateos, R. P. Feliciano, A. Boeres, T. Weber, C. N. Dos Santos, M. R. Ventura and C. Heiss, Cranberry (poly)phenol metabolites correlate with improvements in vascular function: A double-blind, randomized, controlled, dose-response, crossover study, Mol. Nutr. Food Res., 2016, 60, 2130–2140 CrossRef CAS PubMed.
- R. D. Mendonca, N. C. Carvalho, J. M. Martin-Moreno, A. M. Pimenta, A. C. S. Lopes, A. Gea, M. A. Martinez-Gonzalez and M. Bes-Rastrollo, Total polyphenol intake, polyphenol subtypes and incidence of cardiovascular disease: The SUN cohort study, Nutr., Metab. Cardiovasc. Dis., 2019, 29, 69–78 CrossRef CAS PubMed.
- A. Medina-Remon, R. Zamora-Ros, M. Rotches-Ribalta, C. Andres-Lacueva, M. A. Martinez-Gonzalez, M. I. Covas, D. Corella, J. Salas-Salvado, E. Gomez-Gracia, V. Ruiz-Gutierrez, F. J. Garcia de la Corte, M. Fiol, M. A. Pena, G. T. Saez, E. Ros, L. Serra-Majem, X. Pinto, J. Warnberg, R. Estruch, R. M. Lamuela-Raventos and P. S. Investigators, Total polyphenol excretion and blood pressure in subjects at high cardiovascular risk, Nutr., Metab. Cardiovasc. Dis., 2011, 21, 323–331 CrossRef CAS PubMed.
- A. Alborzi, A. Attar, M. Sayadi and F. Nouri, The Effects of Intensive Blood Pressure Control on Cardiovascular Outcomes Based on 10-Year ASCVD Risk Score: An Analysis of a Clinical Trial, Cardiol. Res. Pract., 2021, 2021, 6635345 Search PubMed.
- A. Ullah, S. Munir, S. L. Badshah, N. Khan, L. Ghani, B. G. Poulson, A. H. Emwas and M. Jaremko, Important Flavonoids and Their Role as a Therapeutic Agent, Molecules, 2020, 25(22), 5243 CrossRef CAS PubMed.
- A. Chanet, D. Milenkovic, C. Manach, A. Mazur and C. Morand, Citrus flavanones: what is their role in cardiovascular protection?, J. Agric. Food Chem., 2012, 60, 8809–8822 CrossRef CAS PubMed.
- B. H. Juurlink, H. J. Azouz, A. M. Aldalati, B. M. AlTinawi and P. Ganguly, Hydroxybenzoic acid isomers and the cardiovascular system, Nutr. J., 2014, 13, 63 CrossRef PubMed.
- A. K. Eriksen, C. Brunius, M. Mazidi, P. M. Hellstrom, U. Riserus, K. N. Iversen, R. Fristedt, L. Sun, Y. Huang, N. P. Norskov, K. E. B. Knudsen, C. Kyro, A. Olsen, A. Tjonneland, J. Dicksved and R. Landberg, Effects of whole-grain wheat, rye, and lignan supplementation on cardiometabolic risk factors in men with metabolic syndrome: a randomized crossover trial, Am. J. Clin. Nutr., 2020, 111, 864–876 CrossRef PubMed.
- C. Rodriguez-Garcia, C. Sanchez-Quesada, E. Toledo, M. Delgado-Rodriguez and J. J. Gaforio, Naturally Lignan-Rich Foods: A Dietary Tool for Health Promotion?, Molecules, 2019, 24(5), 917 CrossRef PubMed.
- D. Rodriguez-Leyva, W. Weighell, A. L. Edel, R. LaVallee, E. Dibrov, R. Pinneker, T. G. Maddaford, B. Ramjiawan, M. Aliani, R. Guzman and G. N. Pierce, Potent antihypertensive action of dietary flaxseed in hypertensive patients, Hypertension, 2013, 62, 1081–1089 CrossRef CAS PubMed.
- C. M. McEniery, J. R. Cockcroft, M. J. Roman, S. S. Franklin and I. B. Wilkinson, Central blood pressure: current evidence and clinical importance, Eur. Heart J., 2014, 35, 1719–1725 CrossRef PubMed.
- A. Ochoa, G. Patarroyo-Aponte and M. Rahman, The Role of Central Blood Pressure Monitoring in the Management of Hypertension, Curr. Cardiol. Rep., 2018, 20, 41 CrossRef PubMed.
- W. Zhang, X. Wang, Y. Liu, H. Tian, B. Flickinger, M. W. Empie and S. Z. Sun, Dietary flaxseed lignan extract lowers plasma cholesterol and glucose concentrations in hypercholesterolaemic subjects, Br. J. Nutr., 2008, 99, 1301–1309 CrossRef CAS PubMed.
- S. S. Anand, G. R. Dagenais, V. Mohan, R. Diaz, J. Probstfield, R. Freeman, J. Shaw, F. Lanas, A. Avezum, A. Budaj, H. Jung, D. Desai, J. Bosch, S. Yusuf, H. C. Gerstein and D. I. Epi, Glucose levels are associated with cardiovascular disease and death in an international cohort of normal glycaemic and dysglycaemic men and women: the EpiDREAM cohort study, Eur. J. Prev. Cardiol., 2012, 19, 755–764 CrossRef CAS PubMed.
- L. O. Dragsted, Q. Gao, A. Scalbert, G. Vergeres, M. Kolehmainen, C. Manach, L. Brennan, L. A. Afman, D. S. Wishart, C. Andres Lacueva, M. Garcia-Aloy, H. Verhagen, E. J. M. Feskens and G. Pratico, Validation of biomarkers of food intake-critical assessment of candidate biomarkers, Genes Nutr., 2018, 13, 14 CrossRef CAS PubMed.
- Y. Xu, M. Le Sayec, C. Roberts, S. Hein, A. Rodriguez-Mateos and R. Gibson, Dietary Assessment Methods to Estimate (Poly)phenol Intake in Epidemiological Studies: A Systematic Review, Adv. Nutr., 2021, 12, 1781–1801 CrossRef PubMed.
|
This journal is © The Royal Society of Chemistry 2023 |
Click here to see how this site uses Cookies. View our privacy policy here.