Designing an energy efficient, economically feasible, and environmentally robust integrated farming system model for sustainable food production in the Indian Himalayas†
Received
13th September 2022
, Accepted 10th November 2022
First published on 19th December 2022
Abstract
Globally, the agricultural production system is threatened by the adversities of climate change, land degradation, low energy productivity, and poor environmental outcomes. Thus, there is a dire need to design and develop sustainable agricultural food production technologies that can potentially ensure household-level food security with a minimum environmental footprint. Achieving a balance in the food-energy trade-off while conserving the ecosystem base and minimizing greenhouse gas (GHG) emissions for achieving sustainable environmental livelihoods is a pressing challenge in the Indian Himalayas. Therefore, it was hypothesized that the integrated farming model involving organic cultivation practices shall reduce farmland waste, and arrest land degradation while sustaining higher productivity, energy use efficiency, and profitability. Thus, the productivity, profitability, energy security, and environmental sustainability of four integrated organic farming system (IOFS) models (viz., PS-I, mono-cropping + livestock; PS-II, diversified cropping + livestock; PS-III, diversified cropping + livestock + poultry, and PS-IV, diversified cropping + livestock + poultry + piggery) were assessed during 2016–19 in the Meghalaya region of the Indian Himalayas. All the designed integrated farming/production systems had higher energy profitability and eco-efficiency and substantially reduced greenhouse gas intensity (GHGI) compared to PS-I. PS-IV recorded a significantly higher net economic return (US$ 2828.5 per y), energy productivity (0.22 kg MJ−1), and eco-efficiency (0.31 US$ per kg CO2eq), and the lowest GHGI (0.33 kg CO2eq per kg food production) compared to other production systems. GHG (per kg production) emissions of PS-IV were also 1.7 times lower than those of PS-I. Furthermore, PS-IV had recycled 81.1, 68.2, and 68.8% higher N, P, and K than the usual business (PS-1), respectively. Thus, the study suggested that PS-IV could be a profitable, energy-efficient, environmentally friendly, and economically viable sustainable production system. Hence, PS-IV can be recommended as an economically feasible and environmentally robust production model for ensuring household-level livelihood security in the Indian Himalayas.
1. Introduction
Environmental and livelihood security is the main focus of interest amidst climate change challenges1 and increasing population pressure for achieving the target of sustainable development goals (SDGs). Bridging the food-energy demand and supply gap while maintaining environmental sustainability and food security is one of the pressing challenges for agricultural scientists, environmental planners, and policymakers worldwide.2–4 The farming sector is highly sensitive to climatic uncertainties and is also highly impacted by population growth, resource scarcity, and degradation. It is supposed to follow a similar trend in the future also.5
In recent years, anthropogenic activities and intensive agricultural practices have disturbed the natural agroecosystems substantially.6 The intensive use of synthetic fertilizers/pesticides, fossil energy, and water has resulted in agricultural system degradation and inefficiency. However, with time, agricultural practices and technologies have been evolving rapidly. Modern agriculture has focused on advanced technological interventions that can be proved as time-saving production-enhancing processes and practices. Though these approaches have increased profits immensely, at the same time, they have resulted in increased water and energy demand, increased resource inputs, and pollution loads such as GHGs, farm waste, etc.7,5 Additionally, linear economy-led agricultural practices generated diverse and a large amount of heterogeneous solid and liquid waste.8 Direct dumping of heterogeneous waste in the environment harms human livelihood, and soil health, and disrupts the various gainful environmental processes by imposing pollution loads.9,10 Along with environmental deterioration, they cause biodiversity losses and functional soil fatigue that lead to environmental degradation and affect ecosystem services.10–13 The United Nations SDGs also aim to check environmental adversities to safeguard global social, economic, and ecological security by the end of 2030.14 Cleaner agricultural production technologies like integrated production of various commodities in a complementary fashion promote a circular economy that may help in improving livelihood also. The circular agricultural economic model emphasizes minimizing the amount of external inputs for farming by closing the nutrient loops which reduces the negative environmental outcomes by eliminating/minimizing liquid and solid waste disposal.9,10,15,16 Biomass is a potential green energy source that needs to be recycled judiciously and intelligently for developing a sustainable economy.16–18 Thus, making economic sense of all the agricultural products (edibles or biomass waste) and processes is very important in the context of the present global warming scenario. Thus, a circular economy (CE)-based farm production system can be a more promising, efficient, and appealing strategy concerning environmental sustainability and resource management by reducing environmental adversities as well as increasing economic efficiency.18–21 The CE-based production system offers multi-environmental, social, and financial welfare by conversion of waste into wealth.18,22 However, the linear agricultural economic model-based technological interventions are designed to obtain the maximum economic returns without ecological consideration,16,17 reduced by 27% to limit global warming below 2 °C.23,24 Despite several efforts, the progress does not seem to be aligned with the targeted goals of climate change mitigation and SDGs. Global GHG emission ranges between 51 and 56 Gt CO2eq per y. Out of this, the agriculture sector accounts for ∼11.1 Gt CO2eq per y GHG emission.23–26 With this background of the significant impact of agricultural activities on climate change, countries participated at the Conference of Parties-26 (COP26) on 5th November 2021, as part of the discussion on agriculture and agreed on a transition toward climate-resilient sustainable food systems. It was recognized by the parties that climate-resilient agricultural practices would be crucial for ensuring food security and ending global hunger besides fulfilling COP26's commitment to minimizing 45% of emissions by 2030.27
There is an immediate need to curtail the current level of GHG emissions by adopting cleaner agricultural production technologies. Taking these unfavorable trends into consideration, designing and developing a CE-based sustainable food production system, therefore, is imperative for safeguarding environmental well-being which simultaneously improves the food, nutritional, and livelihood securities. Agricultural waste can be used for many economic purposes but the cost involved in its collection, processing, and transportation is much higher than the economic returns.16,28–30 Annualy, global agricultural production systems generates about 4.6 billion tonnes of utilizable agri-residue.17,31 About 500 Tg crop residues are generated by Indian agriculture every year, out of which more than 25% are burnt on the farm.28 Dumping/burning of agricultural waste not only deteriorates soil fertility but also negatively affects the environmental quality. Furthermore, intensive energy use may increase the energy lead CO2 emissions by 6% upto 2050.25,32 Hence, in situ recycling is the best-suited economical recycling option for agri-waste management. The location-specific integrated production system facilitates efficient waste recycling with minimal negative impacts on ecosystems, animals, and human health.
Carbon footprint (CF), defined as the cumulative GHG emissions caused by a product, is an important parameter of environmental monitoring. It is often expressed in terms of carbon dioxide equivalent to all GHGs emitted, total ecological footprint (EFP), and energy auditing, and its relation to economic gain that provides a more comprehensive and quantitative measure of environmental degradation and livelihood security.33 Conservation effective farming systems allow appropriate and effective combinations of different enterprises that efficiently utilize scarce resources while minimizing the risks and uncertainties.34,35 Closed and complementary interactions between the different enterprises in mixed production systems keep the resources within the framework of the economy. Complementary mixed production systems aim to ensure livelihood and nutritional security while reducing environmental pressure and ecosystem service degradation36,37 and advocate the adoption of integrated farming/production systems to fight environmental issues, food demand, and supply targets.
Integration of diverse farm enterprises like crops, livestock, and other farm enterprises plays a synergistic role in food production, growers' livelihood, and the provisioning of various tangible and non-tangible benefits.38–40 Thus, building up the inextricable soil–water–food and energy nexus for a strong agricultural production system should play a vital role in bringing in a balance between higher productivity and ecosystem sustainability. An integrated farming system offers effective management of various enterprises which has the potential to mitigate the release of GHGs and improve input use efficiencies and other ecosystem services.11 It provides opportunities to capture ecological interactions among the various enterprises,40 and provides opportunities to utilize labor and other resources more efficiently.41 Thus, futurist food, nutritional and livelihood security, and environmental sustainability are supposed to be achieved by integrated farming systems.42
The Himalayan region of India has a scarce industrial infrastructure, but high potential for organic farming. The region is a unique ecosystem that receives >200 cm of rainfall per annum.15 The wettest place in the world Mawsynram which receives ≃1200 cm annual rainfall, is also located in the eastern Himalayan region of India. High rainfall and steep hilly topography are always associated with the problem of severe soil erosion to the extent of 45–460 Mg per ha per annum soil loss.3 Despite very high annual rainfall, an extremely dry period from November to April is very common in the Himalayan region of India which forces the farmers to keep their land fallow. Hilly land is ∼3–4 times poor in production as compared to plain fertile land.43 This poses a great challenge to the livelihood of hill farmers. To overcome these challenges and feed the increasing population there is an urgent need to design sustainable farming system models, which can minimize the farming risk and ensure livelihood security.
Therefore, in the present study, it was hypothesized that the integration of crops, livestock, poultry, and piggery, has the potential to mitigate the problems related to food, nutritional, economic, and environmental securities, and sustainability (SDGs, CoP26's agenda) and help to improve the other ecosystem services, like biodiversity protection, soil sustainability, and resource conservation thereby reversing the deteriorating ecosystem and factor productivity, and decreasing profitability due to conventional specialized agricultural production systems. Crop-livestock systems are complex farming systems, hence, a comprehensive assessment of the energy input–output relationship, economic sustainability, and environmental footprint needs to be made in a precise and comprehensive manner. Hence, the effects of scientifically and strategically designed farmers' managed integrated production/farming systems were investigated for three years (2016–2019) in Meghalaya, India. Considering this background, a field experiment was conducted with the objectives (i) to quantify the effect of integration of different enterprises in the IOFS on the system productivity and economic efficiency, (ii) to measure the effect of different enterprises of the IOFS on the greenhouse gases emission and energy dynamics, and (iii) to evaluate the eco-efficiency of different IOFS models vis-á-vis farmers' practice.
2. Materials and methods
2.1 Study area
The study was conducted and executed at Ri-Bhoi, Meghalaya, India, which lies between 90°55′15 to 91°16′ latitude and 25°40′ to 25°21′ longitude. The area is characterized by hilly terrain having inadequate infrastructure and is prone to degradation of various kinds. The area is having a subtropical climate with three distinct seasons: summer, rainy, and winter. The average maximum and minimum temperatures of the site were 28 °C and 4 °C, respectively.
2.2 System design and management
The study objective was to assess and compare the energy input–output relationship, economic profitability, greenhouse gas emissions, and eco-efficiency of an integrated production system with those of the conventional production system. Hence, three integrated organic production systems were designed at the farm level in a participatory mode and compared with the conventional production system. The enterprise in each production system was allocated in a complementary fashion. All the production systems were replicated five times. In totality, all the production systems were tested at 20 farms. All the crops were managed organically as per the recommended package of the practices. The required feed for animal components was met out from the system itself except in PS-I where the external feed was purchased to meet the minimum requirement. Details of the input used in each production system are given in ESI Table 1.† As the majority of the households (>85%) in India belong to the small and marginal farming group, all the production systems in this study were designed and tested in holding sizes of ∼0.5 ha area. Furthermore, we did not extrapolate the results to one hectare to ensure higher precision and actuating applicability of the results under field conditions.
2.3 System productivity
The system productivity of different systems was evaluated to assess their production capacity. For a meaningful comparison of different production systems, all products were converted into the rice equivalent yield (REY), i.e., system productivity. The market value was considered for estimating the system's productivity. The REY was calculated as per eqn (1). | 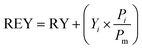 | (1) |
where REY is the rice equivalent yield (Mg ha−1); RY is the economic productivity of rice (Mg ha−1); Yi is the economic production of the ith item (Mg ha−1); Pi is the economic value of the ith item (US$) and Pm is the economic value of the rice grain (US$).
Per-day food production from the particular systems was also assessed concerning system production efficiency. The SPE was estimated with the following equation.
|  | (2) |
2.5 Environmental performance indicators assessment
The carbon footprint both at a spatial scale and an yield scale and eco-efficiency considering energy input and GHG emission per unit of economic gain were assessed to compare the environmental competency of different integrated farming/production systems.
2.5.1 Carbon footprint (CF) measurement of crops.
The total extent of GHG emissions (CH4, CO2, and N2O) emitted throughout the entire cropping period was assessed as a CO2 equivalent (CO2-eq.).44–47 CO2e is also called a CF. In the current investigation, all the crops except rice were grown on well-drained upland terraces. Therefore, carbon dioxide (CO2) and nitrous oxide (N2O) gases were taken into account for the estimation of the CF under upland crop production. As CH4 emission occurred under submerged conditions, it was accounted for in estimating the total CF from the rice crop. Total CH4, CO2, and N2O released from a particular production system were expressed through CO2e by multiplying the GWP equivalent of 28, 1, and 265 for CH4, CO2, and N2O, respectively, for a 100-year timeframe.48,49
The CH4 emission from the rice system and N2O emissions from organic N sources were calculated using the formula outlined in46,47
| CH4 emission (kg year−1) = EF × SF0 × (Aj + [Aj × SFj])/10 | (11) |
EF = 10 g m
−2 year
−1 for India,
Aj = area under rice paddy, ha year
−1, SF
0 = 1.4 correction factor for organic amendments, and SFj = 0.7 scaling factor for
A.
To quantify the N2O emission, the 0.01 emission factor was multiplied by the total N employed through various organic sources and expressed in N2O kg per N input.50
| N2O emission (kg year−1) = N contributed by N sources × 0.01 × 44/28 | (12) |
The global warming potential (GWP) from all crops except rice was estimated as per eqn (13)
| GWP = total N2O emission × 265 + total CO2 emission | (13) |
The global warming potential (GWP) from rice was estimated as per eqn (14) and (15)
| GWP = total CH4 emission × 25 + total N2O emission × 265 + total CO2 emission | (14) |
| Total GWP from crops = ∑CF1, …, ith crop | (15) |
The carbon footprint in system productivity (CFsp) point of view was calculated as per the following equation.
|  | (16) |
2.5.2 CF measurement of dairy/livestock.
To estimate the CF, CH4 emissions due to enteric fermentation, and CH4 and N2O emissions throughout the manure production were calculated. Because annual net CO2 emissions are zero, CO2 emissions were not considered in this study. N2O emissions are from manure handling and management systems. Thus, gaseous loss of nitrogen from the manure system is an imperative step for assessing the quantity of nitrogen available in manure. The CH4 emission by enteric fermentation and the manure management system was estimated as per eqn (17).50where CH4 enteric = methane emissions from enteric fermentation, kg CH4 year−1, n = number of dairy cattle, and EF = CH4 emission factor for the Indian subcontinent (58 kg CH4 per head per year is for milking cows)
In the present study, the manure was stored as a heap on the open ground. The emission of CH4 during manure management was calculated using the tier I approach.51,52 Emission from a given period is determined from the following equation:
where CH
4 manure = methane emissions from the manure system, kg CH
4 year
−1,
n = number of dairy cattle, and EF = CH
4 emission factor for the Indian subcontinent (5 kg CH
4 per head per year)
| Total CH4 emission (kg per year) = ∑eqn (17) + eqn (18) | (19) |
Direct and indirect N2O emissions were estimated from the manure management system.53 In the present study, we have adopted the solid storage method for manure handling. Direct N2O emission was measured using the following equation.
| 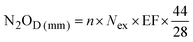 | (20) |
where N
2O
D (mm) indicates direct N
2O emissions from manure systems (kg N
2O year
−1),
n is the number of cattle,
Nex is the N excretion kg per animal per year and EF is 0.005 kg N
2O-N (kg nitrogen excreted) for solid storage systems in India.
53
The annual N excretion rate was calculated using the following equation:
| 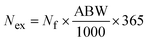 | (21) |
where
Nex is the annual N excretion (kg N per animal per year),
Nf is the nitrogen excretion factor for the dairy cattle in Asia (0.47 kg N per animal per day)
53 and ABW represents the average body weight of the animal (kg).
Indirect N2O emissions: indirect emissions from manure management systems also included volatilization and leaching losses of N.
The volatilization loss as indirect N2O emissions is expressed as eqn (22).
| Nvol = n × Nex × 0.30 | (22) |
where
Nvol = amount of nitrogen lost due to volatilization of NH
3 and NO
x, kg N year
−1,
n = number of dairy cattle,
Nex = annual average N excretion (kg N per animal per year), and 0.30 = fraction of gaseous N losses from the solid storage management system.
53
N is converted into N2O using the following equation:
| N2Ovol = Nvol × EF × 1.57 | (23) |
where N
2O
vol = volatilization loss of N as indirect N
2O emissions from the manure system (kg N
2O year
−1),
Nvol = volatilization loss of N as NH
3 and NO
x, kg N year
−1, and EF = 0.01 for the manure management system.
53
N losses due to leaching from manure management systems
| 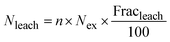 | (24) |
where
Nleach = amount of nitrogen leached from manure management systems, kg N year
−1,
n = number of dairy cattle,
Nex = annual average N excretion (kg N per animal per year), and Frac
leach = percent of loss of nitrogen due to leaching and runoff during manure storage (Av. 10%).
N is converted into N2O using the following equation:
| N2Oleach = Nleach × EF × 1.57 | (25) |
N2Oleach indicates leaching and runoff (nitrogen) contribution to indirect N2O emissions from manure management (kg N2O year−1) whereas EF is the emission factor for N2O from leaching and runoff loss of nitrogen, kg N2O-N per kg N leached and runoff (0.0075 kg N2O–N/(kg N leaching/runoff))
| GWP (kg CO2e) = total CH4 emission × 28 + total N2O emission × 265 | (26) |
In this assessment, 1 kg of fat-and protein-corrected milk (FPCM) produced at the farm gate was taken as a functional unit. For estimation of the CF of dairy production, total raw cow milk produced in a particular production system was corrected to 3.5% fat and 3.0% protein and expressed as kg CO2-eq. per kg FPCM (Alexandratos 1995)54
| FPCM (kg) = milk production (kg) × [0.337 + 0.116 × fat (%) + 0.06 × protein (%)]. | (27) |
|  | (28) |
where GHGI, GWP, and EPCM are greenhouse gas intensity, global warming potential, and fat-and protein-corrected milk, respectively.
2.5.3 CF measurement of poultry.
In the present study, CH4 and N2O emissions from manure management were accounted for in the estimation of the CF. Emission from a given period is determined using the following equation.51,52where CH4 manure = methane emissions from manure management, kg CH4 per year, n = number of poultry birds at a given time, and EF = emission factor from poultry for India (0.03 kg CH4 per bird per year).
N2O emissions were categorized into direct and indirect emissions from the manure management system. The direct N2O emission was estimated using53 with slight modifications.
| 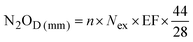 | (30) |
where N
2O
D (mm) = direct N
2O emissions from manure management (kg N
2O per year),
n = number of poultry birds,
Nex = annual average N excretion (kg N per bird per year), and EF = 0.001 kg N
2O-N excreted for poultry with litter systems in India (Eggleston
et al., 2006; Buendia
et al., 2006).
51,52
The annual N excretion rate was calculated using the following equation.
|  | (31) |
where
Nex = annual N excretion from poultry (kg N per bird per year) and
Nf = nitrogen excretion factor for poultry in Asia (0.82 kg N layer per bird per day).
53
Indirect N2O emissions from the manure management system included volatilization and leaching N losses.
The indirect N2O emissions from volatilization of N are in the forms of NH3 and NOx (N2Ovol)
| Nvol = n × Nex × 0.40 | (32) |
where
Nvol = volatilization N losses of NH
3 and NO
x, kg N year
−1,
n = number of poultry birds,
Nex = annual average N excretion (kg N per bird per year), and 0.40 = fraction of gaseous N losses from poultry with litter systems.
51,52
N is converted into N2O using the following equation
| N2Ovol = Nvol × EF × 1.57 | (33) |
where N
2O
vol = indirect N
2O emissions from the manure system (kg N
2O year
−1),
Nvol = nitrogen losses due to NH
3 and NO
x, kg N year
−1, and EF = 0.01 for the manure management system.
N losses are due to leaching from manure management systems
| 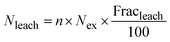 | (34) |
where
Nleach = amount of manure nitrogen leached from manure management systems, kg N year
−1,
n = number of poultry birds,
Nex = annual average N excretion (kg N per bird per year), and Frac
leach = nitrogen losses due to leaching (Av. 10%).
N is converted into N2O using the following equation
| N2Oleach = Nleach × EF × 1.57 | (35) |
where N
2O
leach = indirect N
2O emissions due to leaching and runoff from manure management (kg N
2O year
−1), and EF = emission factor for N
2O emissions from nitrogen leaching and runoff, kg N
2O–N per kg N leached and runoff (0.0075 kg N
2O–N per kg N leaching/runoff).
The GWP was calculated as per the following expression.
| GWP (kg CO2e) = total CH4 emission × 25 + total N2O emission × 265 | (36) |
In this assessment, kg egg was taken as the functional unit for the GHG emission measurement and expressed as kg carbon dioxide equivalents (CO2-eq.) per kg of egg per year at the farm gate.55
|  | (37) |
where GHGI = greenhouse gas intensity and GWP = global warming potential.
2.5.4 CF measurement from piggery.
The CH4 emissions from enteric fermentation and manure management were considered to compute the CF from piggery.
The CH4 emission from enteric fermentation was calculated by using the following equation
where CH
4 enteric = methane emissions from enteric fermentation (kg CH
4 year
−1),
n = total number of pigs, and EF = CH
4 emission factor for developing countries in the Indian subcontinent (1.0 kg CH
4 per animal per year).
In the present study, the manure was stored in a deep bedding system. Emission from a given period is determined using the following equation.
where CH
4 manure = methane emissions from manure management (kg CH
4 year
−1),
n = number of pigs, and EF = emission factor for the Indian subcontinent (5 kg CH
4 per head per year).
Therefore, total CH4 emissions can be calculated as:
|  | (40) |
N2O emissions occur both directly as well as indirectly from manure. In the present study, the manure was managed as deep bedding. The direct N2O emissions from manure management were computed through the following equation.
| 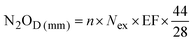 | (41) |
where N
2O
D (mm) = direct N
2O emissions from manure management (kg N
2O year
−1),
n = number of pigs,
Nex = annual average N excretion (kg N per pig per year), and EF = 0.07 kg N
2O–N (kg nitrogen excreted) for deep bedding with active mixing in India (IPCC, 2006).
The annual N excretion rate was calculated using the following equation
| 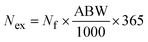 | (42) |
where
Nex = annual N excretion (kg N per animal per year),
Nf = nitrogen excretion factor for pigs in Asia (0.42 kg N per animal per day),
53 and ABW = average body weight of the animal (kg).
Indirect N2O emission from the manure management system includes volatilization and leaching N losses.
The indirect N2O emissions from volatilization of N are in the forms of NH3 and NOx (N2Ovol)
| Nvol = n × Nex × 0.40 | (43) |
where
Nvol = amount of manure nitrogen lost due to volatilization of NH
3 and NO
x, kg N year
−1,
n = total number of pigs,
Nex = annual average N excretion (kg N per animal per year), and 0.40 = fraction of gaseous N losses from the deep bedding system.
53
N was converted into N2O using the following equation:
| N2Ovol = Nvol × EF × 1.57 | (44) |
where N
2O
vol = indirect N
2O emissions due to volatilization of N from manure (kg N
2O year
−1),
Nvol = nitrogen lost due to volatilization of NH
3 and NO
x, kg N year, and EF = emission factor for the deep bedding system (0.01).
53
N losses are due to leaching from manure management systems
| 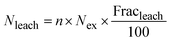 | (45) |
where
Nleach = amount of manure nitrogen leached from manure management systems (kg N year
−1),
n = number of pigs,
Nex = annual average N excretion (kg N per animal per year), and Frac
leach = nitrogen losses due to leaching (Av. 10%).
N is converted into N2O using the following equation
| N2Oleach = Nleach × EF × 1.57 | (46) |
where N
2O
leach = indirect N
2O emissions due to leaching and runoff (kg N
2O year
−1) and EF = emission factor for N
2O emissions from nitrogen leaching and runoff, kg N
2O-N kg N leached and runoff (0.0075).
The GWP was calculated as per the following expression.
| GWP (kg CO2e) = total CH4 emission × 25 + total N2O emission × 265 | (47) |
In this assessment, kg live weight was taken as the functional unit for GHG emission measurement and expressed as kg CO2-eq. per kg of live weight per year.55
|  | (48) |
where GHGI = greenhouse gas intensity and GWP = global warming potential.
Eco-efficiency index (EEI) is defined as the economic gain per unit of environmental impact in terms of GHG emissions and energy use. Sustainable production systems have higher eco-efficiency by reducing environmental impacts while enhancing economic gain (Babu et al. 2020).15 In the present investigation, the ecological impact of different production systems was estimated in terms of total energy consumption (MJ) or total GHG emission (kg CO2 eq. per year). Eco-efficiency index (EEI) interms of energy use and GHG emission were calculated with eqn (49) and (50), respectively.
|  | (49) |
|  | (50) |
2.6 Statistical analysis
The general linear model56 was used to test the statistical significance of data obtained from diverse production systems. The least significant difference (LSD) and standard error of the mean were employed for valid comparison of data. Furthermore, the Pearson correlation was used to find out the degree of relationship between the studied parameters. Principal component analysis (PCA) was used to reduce dimensionality while maintaining the most variation in the examined dataset by analyzing data on energy indicators from various production systems. Variables with factor loadings and PCs with multiple Eigenvalues were judged to be variables that best described system attributes. Therefore, PCs with Eigenvalues of more than 1.0 were considered for further analysis as these PCs were considered more informative than the others (Kaiser 1960).57 The first PC explained maximum variability and the rest of the PCs explained the remaining lion's share of the residual variability.
3. Results
3.1 Input–output relationship in different integrated farming/production systems
Input costs varied significantly among the various production systems. Man-days employed in different production systems varied from 106–270 (ESI Table 1†). Diversified crop production concurrently with livestock, poultry, and piggery provided 2.6 times higher employment than PS-I (usual business). All the designed systems incurred higher water, electricity, and diesel consumption than PS-I (ESI Table 1†). Concerning the food production potential of different production systems, all commodities were converted into the same unit (rice equivalent yield) on the price scale and expressed as system productivity. The system productivity of all the designed systems had significantly increased compared to that of PS-I. Among the different production systems, the system productivity of PS-IV (19.1 Mg ha−1) was significantly higher than that of other systems. The designed integrated organic farming system (IOFS) models had 3.24 to 4.54 times higher food production than PS-I (Fig. 1). The system production efficiency (per day food productive ability of a system) was also significantly higher in designed systems than that in traditional practice. Among the designed systems PS-IV recorded the highest system production efficiency. It recorded 4.58 times higher system production efficiency than PS-I (Table 1). Concerning waste recycling and the nutrient generation potential of different systems, all the designed systems utilized all the waste generated in the form of composting. However, in traditional systems, the residue recycling potential was very low compared to the designed systems. Among the designed systems, PS III and PS-IV recycled the maximum solid waste generated in the systems. These systems recycled 73.6 and 72.68 kg of nitrogen per annum, respectively into the soil. 100% of crop demand was met out from the waste recycling in the designed systems (ESI Table 3†).
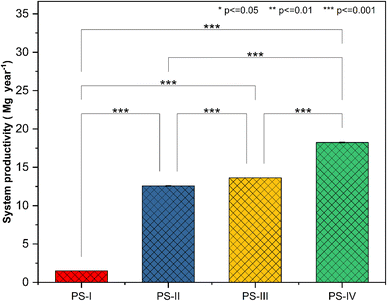 |
| Fig. 1 System productivity of different production/farming systems. PS-I: mono-cropping cereals + livestock; PS-II: diversified cropping + livestock; PS-III: diversified cropping + livestock + poultry; PS-IV: diversified cropping + livestock + poultry + piggery. | |
Table 1 Financial budgeting of different designed production systemsa
Production systems |
PE (kg day−1) |
COP (US$ per y) |
GR (US$ per y) |
NR (US$ per y) |
B : C ratio |
MR |
EE (US$ per y) |
REE |
PS-I: mono-cropping cereals + livestock; PS-II: diversified cropping + livestock; PS-III: diversified cropping + livestock + poultry; PS-IV: diversified cropping + livestock + poultry + piggery; COP: cost of production; GR: gross returns; B : C ratio: benefit cost ratio; MR: marginal returns; EE: economic efficiency; REE: relative economic efficiency; PE: production efficiency; different letters show the standard error of difference between the treatment mean.
|
PS-I |
4.08 ± 0.06d |
719.29 ± 0d |
988.19 ± 5.42d |
268.91 ± 5.42c |
1.38 ± 0.01d |
— |
0.74 ± 0.02c |
— |
PS-II |
34.45 ± 0.21c |
2144.84 ± 0c |
3336.96 ± 19.78c |
1192.12 ± 19.78b |
1.56 ± 0.01b |
0.66 ± 0.02c |
3.27 ± 0.06b |
3.59 ± 0.08b |
PS-III |
37.34 ± 0.09b |
2442.15 ± 0b |
3616.5 ± 8.32b |
1174.35 ± 8.32b |
1.49 ± 0.01c |
0.54 ± 0.01b |
3.22 ± 0.03b |
3.53 ± 0.04b |
PS-IV |
49.95 ± 0.24a |
2950.07 ± 0a |
4813.31 ± 19.64a |
1863.24 ± 19.64a |
1.64 ± 0.01a |
0.72 ± 0.01a |
5.11 ± 0.06a |
6.18 ± 0.08a |
3.2 Financial budgeting
Among the production systems, the maximum production cost (2950.07 US$ per y) was incurred in PS-IV while PS-I had the least cost of production (719.29 US$ per y). Designed production systems had ∼3 times higher marginal returns than the traditional system (Table 1). Similarly, the system returns also varied significantly among the production systems. The gross and net returns per annum obtained from the designed IOFS model were significantly higher than those from the traditional system. Among the different production systems, PS-IV recorded significantly higher gross (4813.31 US$ per year) and net (1864.24 US$ per year) returns followed by PS-II. PS-IV recorded ∼7-fold higher net returns than PS-I (usual business). The designed systems had a significantly higher B
:
C ratio (1.49–1.64) than the traditional ones. PS-IV recorded a significantly higher B
:
C ratio than PS-I. System economic efficiency is the economic return ability of the designed systems compared to the prevailing production system. All the designed systems outperformed the existing systems. The system economic efficiency (SEE) of PS-IV (5.11 US$ per day) was the highest among different IOFS models. The designed system had ∼3–6 times higher relative economic efficiency (REE) than the usual business. Minimum improvements in the net return (1174.35 US$ per year), economic efficiency (3.22 US$ per day), and B
:
C ratio (1.49) were observed in PS-III. Similarly, PS-IV registered the highest additional returns per unit of monetary expenditure marginal returns (MR 0.72) and REE (6.18) followed by PS-III. However, PS-I had the least marginal retruns (MR) and REE (Table 1).
3.2 Energy dynamics under different production systems
Energy budgeting is crucial for designing an environmentally clean production system. The highest energy investment (86
349.94 MJ per year) was recorded in PS-IV while PS-I had the lowest energy input (48
887.39 MJ per year). In the current investigation, all the designed systems generated expressively greater energy returns than PS-I. Designed systems produced ∼13-18-fold higher net energy output than PS-I. Among all the designed systems, PS-III recorded considerably higher gross (188
362.39 MJ per year) and net energy returns (116
944.22 MJ per year). This system had 93.69% higher net energy returns than PS-I (Table 2).
Table 2 Energy dynamics under different production systemsa
Production systems |
Energy input (MJ per year) |
GER (MJ per year) |
NER (MJ per year) |
Human energy use efficiency |
Specific energy (MJ kg−1) |
PS-I: mono-cropping cereals + livestock; PS-II: diversified cropping + livestock; PS-III: diversified cropping + livestock + poultry; PS-IV: diversified cropping + livestock + poultry + piggery; GER: Gross energy returns; NER: net energy returns.
|
PS-I |
48 887.39 ± 4.43d |
56 274.32 ± 460.59c |
7380.94 ± 460.59c |
270.87 ± 2.22c |
32.9 ± 0.46a |
PS-II |
68 251.19 ± 12c |
183 954.8 ± 430.91b |
115 725.61 ± 430.91a |
399.39 ± 0.94a |
5.43 ± 0.04b |
PS-III |
71 431.78 ± 9.46b |
188 362.39 ± 1006.92a |
116 944.22 ± 1006.92a |
392.26 ± 2.1a |
5.25 ± 0.02b |
PS-IV |
86 349.94 ± 8a |
184 615.71 ± 1794.82a,b |
98 247.78 ± 1794.82b |
348.86 ± 3.4b |
4.74 ± 0.03b |
Concerning specific energy (SE), the total energy demand for unit food production, was significantly less (4.72 MJ kg−1) in PS-IV, while PS-I had the maximum SE (32.89 MJ kg−1) (Table 2). Moreover, all the designed systems had less specific energy than the usual business. Designed systems required ∼6 times less energy per unit of food production than the existing system of the region. The energy productivity, energy efficiency ratio, human energy efficiency, and profitability significantly varied among the production systems. Concurrent production of diversified crops and livestock under PS-II gave the highest energy efficiency ratio (2.70) (Fig. 2) and human energy use efficiency (399.38) (Table 2) followed by simultaneous cultivation of diversified cropping, livestock, and poultry under PS-III. But induction of piggery reduced the energy efficiency ratio. PS-I had the lowest energy efficiency ratio (1.16). Moreover, all the designed systems recorded a higher energy efficiency ratio than the existing production practices (Fig. 2). In the case of energy productivity, PS-IV registered the highest energy productivity (0.21 kg MJ−1), however, this was statistically on par with PS-III but significantly superior to PS-II and PS- I (Fig. 3). Energy profitability has been expressed as the energy gain per unit of energy investment. PS-II recorded the maximum energy profitability (1.70 MJ MJ−1) while PS-I had the least energy profitability (0.15 MJ MJ−1) (Fig. 4).
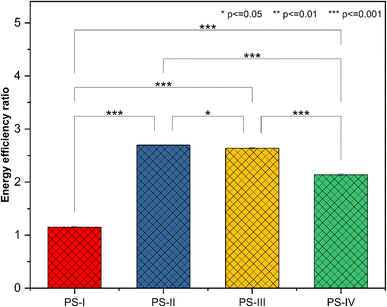 |
| Fig. 2 Energy efficiency ratio of different production systems. PS-I: mono-cropping cereals + livestock; PS-II: diversified cropping + livestock; PS-III: diversified cropping + livestock + poultry; PS-IV: diversified cropping + livestock + poultry + piggery. | |
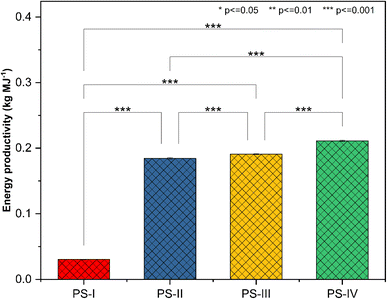 |
| Fig. 3 Energy productivity of different production systems. PS-I: mono-cropping cereals + livestock; PS-II: diversified cropping + livestock; PS-III: diversified cropping + livestock + poultry; PS-IV: diversified cropping + livestock + poultry + piggery. | |
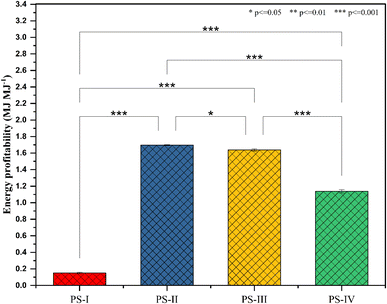 |
| Fig. 4 Energy profitability of different production systems. PS-I: mono-cropping cereals + livestock; PS-II: diversified cropping + livestock; PS-III: diversified cropping + livestock + poultry; PS-IV: diversified cropping + livestock + poultry + piggery. | |
3.4 Environmental indicators
The ecological sustainability of the intended systems was assessed in terms of GWP, greenhouse gas intensity (GHGI), and eco-efficiency index. The CO2e emission (GWP) varied considerably among the production systems (Table 3). The intensified system had more GWP than the less intensified system. It indicates that the enterprise intensification amplified the GWP as the intensification incurred more energy and other input to manage the different components. A wide variation (2313.6 to 6202.1) in CO2 eq. per y among the different tested production systems was detected in the current investigation (Table 3). PS–IV registered the highest CF (6202.1 kg CO2e) while PS-I had the lowest CF (2313.6 kg CO2e). Contrary to GWP on a spatial scale, PS-III had significantly lower GHGI (0.34 kg CO2e per kg food production) followed by PS-IV. The designed systems had 37.5–44.6% lower GHGI than the existing production systems.
Table 3 Environmental foot prints of different production systemsa
Production systems |
GWP (US$ CO2e per y) |
GHGI (kg CO2 e per kg product) |
EEIe (US$ per MJ) |
EEIGHG (US$ per kg CO2e) |
PS-I: mono-cropping cereals + livestock; PS-II: diversified cropping + livestock; PS-III: diversified cropping + livestock + poultry; PS-IV: diversified cropping + livestock + poultry + piggery. Different letters (a–d) indicate the least significant difference at p = 0.05%. GWP: global warming potential; GHGI: greenhouse gass intensity; EEIe: eco-efficiency index in terms of energy consumption; EEIGHG: eco-efficiency index in terms of greenhouse gass emission.
|
PS-I |
2313.6d |
1.00a |
0.03d |
0.12c |
PS-II |
4526.2c |
0.37b |
0.05c |
0.27b |
PS-III |
4593.1b |
0.34b |
0.06b |
0.26b |
PS-IV |
6202.1a |
0.35b |
0.06a |
0.31a |
Eco-efficiency indicates the financial gain concerning environmental destruction in term of energy investment and GHG emission. The eco-efficiency index (EII) was expressed in terms of monetary gain per unit of energy use (US$ per MJ) and CO2e emission (US$ per kg CO2e). PS-I had the lowest eco-efficiency (0.03 US$ per MJ and 0.12 US$ per kg Co2e) (Table 3). PS-IV registered the maximum EEI both concerning energy investment and GHG release followed by PS-III and PS-II, respectively. It implied that the existing production systems in Eastern Himalayas are not a profitable venture both in terms of economic gain and environmental quality. Concurrent rearing of livestock, poultry, and piggery along with diversified cropping recorded 50.0% and 61.29% higher eco-efficiency concerning energy use and GHG emission than crop mono-cropping + livestock (PS–I), respectively (Table 3).
3.5 Nexus between system productivity, economic returns, energy dynamics, and GHG emission
Pearson correlation was calculated among the selected variables. The legend indicates that the two different colors are representing positive and negative correlations. The degree of bend in the eclipse indicates a degree of correlation in the core plot. Strong nexus was observed between food production, economic gain, energy dynamics, and GWP (Fig. 5). In the present study, net returns and energy productivity were positively correlated with system productivity. However, system productivity was negatively correlated with specific energy and GHGI. GHGI was positively correlated with specific energy and GWP was positively associated with energy investment. Principal component analysis (PCA) was done. PCA biplot variables represented by arms showed unidirectional orthogonality with wide variation in length and angle. This implies that all energy variables increased with intensified production systems. Clustering in biplot was done between the production systems which clustered in different quadrants indicating the clear difference between production systems in terms of energy parameters (Fig. 6).
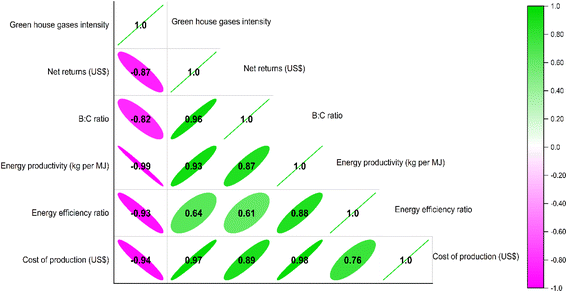 |
| Fig. 5 Correlation between food production-energy dynamics and GHG emission under different production systems. PS-I: mono-cropping cereals + livestock; PS-II: diversified cropping + livestock; PS-III: diversified cropping + livestock + poultry; PS-IV: diversified cropping + livestock + poultry + piggery. | |
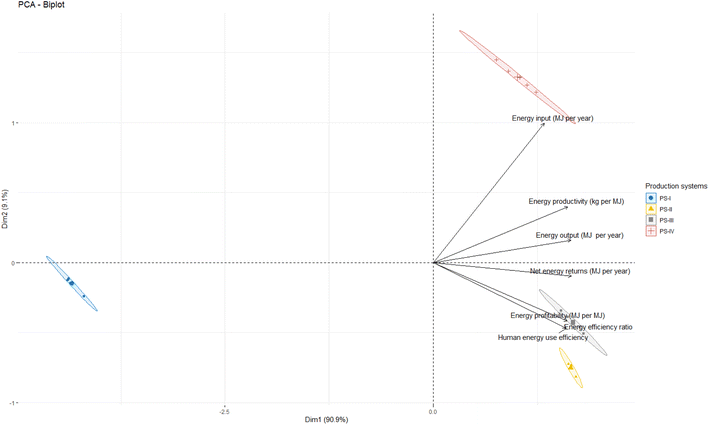 |
| Fig. 6 PCA analysis of energy dynamics under different production systems. PS-I: mono-cropping cereals + livestock; PS-II: diversified cropping + livestock; PS-III: diversified cropping + livestock + poultry; PS-IV: diversified cropping + livestock + poultry + piggery. | |
4. Discussion
4.1 Waste recycling and system production efficiency
An integrated production/farming system encompasses diversified cropping, livestock, piggery, and poultry at a single point.15 In integrated production systems, each enterprise is linked with the other in a closed loop. In the designed system, waste was recycled very efficiently than the usual business. In PS-I, most of the crop residue was removed from the field and at the same time, the animal waste was not properly utilized. The animal waste was dumpped in PS-I without handling resulted in nutrient losses. However, the closed-linked system followed a designed systems that efficiently utilized farm waste and reduced external input use.3,58 These efficiently recycled wastes meet the total nutrient requirement of the diversified crop grown under designed production systems.
Closed-loop nutrient recycling reduced the production cost and increased farm profitability. System integration of diversified cropping with livestock, poultry, and piggery is a major contribution to the revenue generation in designed intensified production systems compared to the existing system. The designed system promoted efficient waste recycling and reduced energy use and greenhouse gas emissions and followed the circular economy principles. Hence, the reintegration of crops with animal systems at the field level could minimize environmental pollution and increase farm productivity and profitability.59,60 Farm-based crop animal integration enhances nutrient recycling61 which favors soil fertility build-up and carbon sequestration. Appropriate crop-livestock integration increases land productivity and use efficiency.62 An effective IOFS model comprising suitable livestock, poultry, piggery, and other components minimizes farmers' risks due to climatic and market uncertainties and provides constant income and employment to the weaker section of the rural society.63,64 Furthermore, diversified commodities help growers to market volatility.65 System productivity and production efficiency varied among the production systems and it was maximum with the integration of diverse enterprises whereas lower system productivity was recorded with PS-I. It is pertinent to mention here that even a simple integration of diversified cropping + livestock has the potential to enhance total system productivity as compared to mono-cropping with livestock. Bio-intensification with complementary integration of different enterprises including crops, horticultural, livestock, fishery, poultry, agroforestry, etc. could lead to higher system productivity.66,67 A many-fold increase in the system productivity under diverse integration compared to existing systems in the Bihar region of India was observed over the existing farming practice.68 Appropriate integration of diversified cropping along with livestock increased productivity by 3 times compared to cropping alone in the Tamil Nadu region of India.69 However, farm productivity under integrated production systems depends largely on the type and degree of integration between different enterprises on the same unit of land.66 Integration of rice with fishery and other compatible enterprises has better productivity and energy credentials compared to crop-based production systems alone in the coastal region of India.27
4.2 Financial budgeting
Financial budgeting is crucial for determining the practical utility of any designed system as the financial well-being of the end-user is the ultimate target for designing any production system. The production cost was maximum in PS-IV while the lowest was in PS-I. The production cost increased with an increase in the level of integration.58 Among the designed systems PS-IV had the maximum economic returns. Complementary interaction of crops, livestock, piggery, and poultry resulted in higher marginal returns than PS-I. This indicates that there is great potential in the Himalayan region to integrate piggery with other enterprises. Moreover, piggery and poultry are the preferred enterprises in integrated production.8 Thus, the results indicate that in terms of unit investment PS-IV was the most productive IOFS system among other systems. Continuous egg, meat, and milk production along with a constant supply of food, feed, and vegetable crops further augments the economic returns of the designed system, particularly of PS-IV. The designed production system had a 7–15% more financial return per unit investment, i.e., the B
:
C ratio compared to the existing farming practice. Diversification of cereal-based systems further augments the farm profitability compared to the existing system.70 The economic returns had a positive correlation with energy productivity but a negative correlation with GWP and specific energy.23 Growers' livelihoods are largely determined by the per day economic gain. In the present study, the per day economic gain was measured from economic efficiency to assess the economic viability and growing prosperity. All the designed systems performed better in terms of economic efficiency. PS-IV recorded 85% higher economic efficiency than the existing system. This indicates that the existing farming system needs to be redesigned to make farming a profitable venture in the Himalayan region.
4.3 Energy dynamics under different production systems
Food production is responsible for ∼30% of the global energy consumption, hence, energy auditing is very imperative to select an energy-efficient production system.71 Reducing input energy and improving energy use efficiency are crucial for achieving energy sustainability in agricultural production. The energy input–output relationship depends on the level of integration and spatial arrangement. In the present study, the inclusion of crops with cattle + poultry + piggery in PS-IV had led to exceedingly energy-intensive systems compared to other production systems. Efficient recycling of residues takes place within a large number of enterprises with diverse kinds of management.27 Co-culturing of rice-based cropping systems with mushroom and poultry required more energy whereas sole rice cropping incurred the least energy investment in the eastern plain zone of India.72 In the present study, the designed production system registered 13–18 times higher energy returns than the existing farming practice of the region. It indicates that the traditional farming business is not an energy-efficient affair, hence, needs to be substituted with a more diverse system. This was due to the less productive capacity of PS-I than that of the designed farming systems, as the energy returns of production systems largely depend upon quantity and quality of the output.71,73 Complementary enterprise integration can efficiently utilize scarce and costly resources.74
The energy productivity, profitability, and efficiency ratio were also higher in designed production systems with more enterprises. This inferred higher energy saving in the designed system than in the existing practice. Among the designed systems, PS-II had registered the maximum energy efficiency ratio, energy profitability, and human energy use efficiency. PS-II had recorded a 2.3, 1.4, and 11 times higher energy efficiency ratio, human energy use efficiency, and energy profitability than existing systems, respectively. The energy productivity was higher in PS-IV and other systems having diverse enterprises since there was synergism among the diverse enterprises and the output of one enterprise served as the input for another. In designed systems, better waste recycling might improve crop productivity which resulted in better economic returns and ultimately improved energy productivity. Hence, it is pertinent to mention that the recycling of waste, the use of a more organic source of nutrients, the conservation of effective soil, and crop management can enhance the energy use efficiency of integrated production systems.75 The compatible integration of livestock, poultry, and piggery with diversified cropping facilitates efficient waste recycling which significantly increased the net energy gain and energy efficiency. The energy productivity further increased with the integration of poultry and piggery since the purchased feed (off-farm) was not given and the by-products available at the farm were used as feed. Thus, it can be emphasized that the integration of complementary enterprises ensures that strategic integration in diverse production systems is energy-efficient.72,76 Concerning specific energy (energy requirement per unit of food production), all the designed systems had a lower specific energy than the existing system. The PS-IV system had the lowest energy requirement per unit of food production. This system needs ∼7 times less energy for unit food production as compared to PS-I. This indicated that all the designed systems are energy-saving and environmentally friendly compared to the existing system of the Eastern Himalayan region of India. The findings of the present study highlighted that the less energy-requiring system doesn't need to be energy efficient; the energy productivity and profitability are unswervingly connected with the quantity of economic returns per unit of energy investment.15 Moreover, technology and methods having higher energy use efficiency can effectively minimize the energy-related negative environmental outcomes.18,77 Hence, the existing farming systems must be characterized to capture the farming system diversity.78 The reintegration of crops with livestock will reduce energy consumption and increase food production.27,60 Hence, redesigning of the contemporary agriculture production system must be the priority to achieve an energy-smart and environmentally clean production system in the fragile hill ecosystems.
4.4 Environmental/ecological indicators
Environmental footprints such as global warming potential (GWP) and eco-efficacy determine the ecological robustness of a production system.15,71 The designed system must focus on multiple ecosystem gains, as one ecosystem service-oriented production system potentially harms other ecosystem services.79 GHG emissions under an integrated production system primarily depend on the level and degree of integration.80 In the present study, GWP varied significantly across the production systems. It has been presumed that enterprise intensification increases the GWP as intensified production systems consumed more energy which resulted in higher GWP. A positive correction for energy use of GWP is potentially testified by several investigators.18,71 In this study, PS-IV (concurrent cultivation of diversified cropping along with livestock, piggery, and poultry) had the highest GWP primarily due to higher resource consumption and energy use. Furthermore, there is a marked increase in the GWP in PS-IV on account of higher CH4 and N2O emissions from livestock, poultry, and piggery component. In contrast, the greenhouse gas intensity (GHGI) substantially reduces when the number of enterprises increases. The GHGI of PS-III with more number of complementary enterprises was lower suggesting that the adoption of integrated production systems with a higher number of complementary enterprises can potentially reduce the GHGI. In the present study, concurrent cultivation of diversified cropping along with livestock and poultry had 2.9 times less GHGI than PS-I (mono-cropping along with livestock). Designed production systems resulted in lower GHG emissions per unit of food production and land due to more system productivity and less energy consumption per unit of production. In PS-I, higher GHGI may be attributed due to higher reliance on imported feed, which requires a lot of energy investment to reach the farm gate. Diversified cropping, livestock, poultry, and piggery integration has slightly higher GHGI than PS-III but is significantly lower than PS-I. Higher GHGI under PS-IV might be due to piggery integration as it accounted for ∼68% of the total GHGI in PS-IV. The lower GHGI in designed intensified production systems than that in PS-I is primarily due to higher system productivity. Hence, enterprises must be selected very wisely when designing integrated production systems. Therefore, low input demanding and more productive enterprises which contribute less to GHG emissions and have high resource conversion efficiency should be selected. The system productivity and GHGI had an inverse relation.15,71 The results of this study are with the common view that intensified production systems had lower GHGI than mono-culturing. Eco-efficiency index (EEI) estimation is imperative when evaluating the environmental performance of a designed system. The EEI is used to express the efficiency of a system concerning environmental destruction. The EEI encompasses the financial as well as ecological magnitudes of a production structure.81,82 Hence, it is considered one of the best parameters to judge the economic and environmental credentials of the designed system. In this study, eco-efficiency in energy use and GHG emission concerning economic returns were measured. PS-I had the lowest EEI, this indicates that the existing production system of the Indian Himalayan region is not environmentally robust and needs to be shifted towards more sustainable systems. Integration of diversified cropping in complementary mode with livestock (PS-II), poultry (PS-III), and piggery (PS-IV) substantially increases the eco-efficiency index compared to that of the usual business. In the present study, the designed system had 40–50% and 53.8–61.30% higher EEI concerning energy consumption and GHG emission than PS-I, respectively. The system having diverse enterprises recorded a higher eco-efficiency index when compared to the existing system and crop enterprise signifying that the integrated production systems were environmentally efficient with more economic returns per unit of GHG emission. Farming practices that increase eco-efficiency and reduce energy use and GHG emissions can achieve environmentally robust and economically feasible production systems.83 Thus, it can be emphasized that the adoption of integrated production systems with diverse complementary enterprises can lead the way toward sustainable production systems.
5 Policy implications of the study
Indian agriculture is negatively impacted by climate change adversities. The agriculture sector contributes ∼14% of the total GHG emission out of which 54.3% is by enteric fermentation, 17.5% is by rice cultivation, 19.1% by fertilizers applied to agricultural soils, 6.7% by manure management, and 2.2% due to field residue burning.84 Hence, there is a dire need to develop and design the appropriate technologies that can potently achieve food security and environmental sustainability objectives. The Indian Himalayan region (IHR) is spread over 13 Indian states/Union territories (Arunachal Pradesh, Nagaland, Mizoram, Manipur, Meghalaya, Tripura, Sikkim part of Assam and West Bengal in the northeast and Jammu and Kashmir, Ladakh, Uttarakhand and Himachal Pradesh in the northwest) and covers ∼53 Mha area (16.2% of the total geographical area of India) . The IHR is the habitat of ∼50 million population. Subsistence farming is in vogue in the IHR which is not able to support the livelihood security of the hill farmers. The IHR enjoys very good rainfall during the monsoon period but, in contrast, during the winter season, it suffers from tremendous water scarcity. Agricultural food production systems in the IHR suffered from multipronged challenges of soil erosion, moisture scarcity, poor seed replacement ratio, mono-cropping, etc. Furthermore, ∼65% of the agricultural land in the region is rainfed which needs appropriate soil and water conservation measures. Hence, the sustainability of hill agriculture is highly questioned and the contemporary agriculture production systems are not a profitable and environmentally friendly affair. Food and nutritional security is essential for a healthy and productive life. But many people living in the mountain regions are deprived of food and nutritional sufficiency. Food-related insecurity is increasing day-by-day in the Himalayan region. In the rural mountain population, food insecurity increased by 12% in five years (2012–17).85 There is a tremendous scope of organic farming in the hilly regions due to the congenial climate and abundant availability of organic nutritional sources and poor use of inorganic fertilizers. Integrated organic farming system (IOFS), a multi-agri-food production technology based on the complementary interaction among the different enterprises favored the complete recycling of farm waste. The IOFS follows the concept of the circular economy by closed-loop recycling of agricultural waste. The interlinked resource use and by-product recycling reduce the cost of production which can potentially reverse the trend of declining farm profits. The findings of the current study showed that the food production and economic gain potential of PS-IV were ∼4.5 and 6.9 times higher than those of traditional farming practices, respectively. Overall all the designed systems are energy efficient and environmentally friendly compared to PS-1 (usual business of the region). PS-IV had 18 times high energy returns and ∼3 times less greenhouse gas intensity than PS-I. The findings suggested that large-scale adoption of PS-IV can be an innovative tool for ensuring food and nutritional security besides minimizing the negative environmental outcomes. But it is important to mention here that enterprises must be optimized as per the location-specific requirement and financial budgeting. The results of the current study also help to achieve some targets of sustainable development goals (SDGs) and COP-26 commitment to India. However, these targets can only be strengthened by the joint efforts of farmers, scientists, and policymakers. Location-specific research for IOFS model refinement, awareness among the different stockholders, policy formulations, and market regulations are warranted for achieving the different SDGs related to agricultural production systems.
6. Conclusions
Thus, the finding proved the hypothesis that wise integration of crops along with livestock and other components sustainably harnesses the food-energy-carbon nexus in the Indian Himalayas by improving food production, energy, economic returns, and eco-efficiency, and by reducing GHG emission. Among the designed systems, PS-IV (diversified cropping + livetsock + poultry + piggery) regenerated and recycled maximum solid waste and maintains 100% crop nutrient demand followed by PS-III and PS-II, respectively. PS-I had the lowest waste recycling potential. Furthermore, PS-IV had the maximum net returns (US$ 4813.31 per annum), B
:
C ratio (1.64), and marginal returns (0.72). However, PS-II recorded the highest energy efficiency ratio (2.70) and energy profitability (1.70), while PS-IV recorded maximum energy productivity (0.21) and the least specific energy (4.74). Concerning the environmental footprint, PS-III had the least GHGI (0.34 kg CO2e per kg food production) while PS-IV had the highest eco-efficiency. It is inferred that PS-IV had the least negative impact on the environment with the highest economic gain.
Thus, considering food production, energy dynamics, economic returns, GHGI, and eco-efficiency, PS-IV and PS-II were considered to be the most sustainable production systems, which can be potentially recommended for food, energy, and environmental securities in the Himalayan region. Hence, to reinforce India's CoP-26 commitments, circular economy targets, and SDGs location specific integrated produtcion/farming system models could be recommended for agricultural planning in the fragile hill and mountain ecosystems of India and other similar ecoregions.
Conflicts of interest
There are no conflicts to declare.
Acknowledgements
The authors express their sincere thanks to the Director, ICAR Research Complex, Umiam, Meghalaya, and the Director, ICAR-IIFSR, Modipuram for providing the essential funding and amenities to conduct this research under the All India Coordinator Research Project on Integrated Farming Systems (AICRP-IFS) under the Tribal Sub Plan. The author also acknowledges the support of Project staff of AICRP-IFS and the farmers for helping in data recording and providing tireless support to complete this study.
References
-
E. M. Biggs, B. Boruff, E. Bruce, et al., Environmental livelihood security in Southeast Asia and Oceania: a water-energy-food-livelihoods nexus approach for spatially assessing change, White paper, IWMI, 2014, DOI: DOI:10.5337/2014.231.
- R. Lal, Soil degradation as a reason for inadequate human nutrition, Food Secur., 2009, 1(1), 45–57, DOI:10.1007/s12571-009-0009-z.
- A. Das, D. Datta and T. Samajdar,
et al., Livelihood security of smallholder farmers in eastern Himalayas, India: pond based integrated farming system a sustainable approach, Curr. Res. Environ. Sustain., 2021, 3, 100076, DOI:10.1016/j.crsust.2021.100076.
- O. A. Ogbolumani and N. I. Nwulu, A food-energy-water nexus meta-model for food and energy security, Sustain. Prod. Consum., 2022, 30, 438–453, DOI:10.1016/j.spc.2021.12.019.
- S. K. Jaiswal, F. Y. I. Ibny, M. Mohammed and F. D. Dakora, Rhizobia as a Source of Plant Growth-Promoting Molecules: Potential Applications and Possible Operational Mechanisms, Front. Sustain. Food Syst., 2021, 4, 619676, DOI:10.3389/fsufs.2020.619676.
- B. Lozano-García, R. Francaviglia and G. Renzi,
et al., Land use change effects on soil organic carbon store. An opportunity to soils regeneration in Mediterranean areas: implications in the 4p1000 notion, Ecol. Indicat., 2020, 119, 106831, DOI:10.1016/j.ecolind.2020.106831.
- E. J. Mantoam, G. Angnes, M. M. Mekonnen and T. L. Romanelli, Energy, carbon and water footprints on agricultural machinery, Biosyst. Eng., 2020, 198, 304–322, DOI:10.1016/j.biosystemseng.2020.08.019.
- S. Babu, R. Singh and R. Avasthe,
et al., Impact of land configuration and organic nutrient management on productivity, quality and soil properties under baby corn in Eastern Himalayas, Sci. Rep., 2020, 10(1), 1–14, DOI:10.1038/s41598-020-73072-6.
- N. Singh, Y. Tang, Z. Zhang and C. Zheng, COVID-19 waste management: Effective and successful measures in Wuhan, China, Resour. Conserv. Recycl., 2020, 163, 105071, DOI:10.1016/j.resconrec.2020.105071.
- J. F. Velasco-Muñoz, J. M. F. Mendoza, J. A. Aznar-Sáncheza and A. Gallego-Schmid, Circular economy implementation in the agricultural sector: definition, strategies and indicators, Resour. Conserv. Recycl., 2021, 170, 105618, DOI:10.1016/j.resconrec.2021.105618.
- K. Lorenz and R. Lal, Environmental impact of organic agriculture, Adv. Agron., 2016, 139, 99–152, DOI:10.1002/9780470015902.a0026341.
- G. Schwilch, L. Bernet and L. Fleskens,
et al., Operationalizing ecosystem services for the mitigation of soil threats: a proposed framework, Ecol. Indicat., 2016, 67, 586–597, DOI:10.1016/j.ecolind.2016.03.016.
- L. Boone, I. Roldán-Ruiz, H. Muylle and J. Dewulf, Environmental sustainability of conventional and organic farming: accounting for ecosystem services in life cycle assessment, Sci. Total Environ., 2019, 695, 133841, DOI:10.1016/j.scitotenv.2019.133841.
-
P. Yadav, D. K. Jaiswal and R. K. Sinha, Climate change: impact on agricultural production and sustainable mitigation, Glob. Clim. Change, Elsevier, 2021, pp. 151–174, DOI:10.1016/B978-0-12-822928-6.00010-1.
- S. Babu, R. Singh and R. Avasthe,
et al., Soil carbon dynamics in Indian Himalayan intensified organic rice-based cropping sequences, Ecol. Indicat., 2020, 114, 106292, DOI:10.1016/j.ecolind.2020.106292.
- J. Sherwood, The significance of biomass in a circular economy, Bioresour. Technol., 2020, 300, 122755, DOI:10.1016/j.biortech.2020.122755.
- U. De Corato, Soil microbiome manipulation gives new insights in plant disease- suppressive soils from the perspective of a circular economy: a critical review, Sustainability, 2020, 13(1) DOI:10.3390/su13010010.
- P. Rathore, S. Chakraborty, M. Gupta and S. Sarmah, Towards a sustainable organic waste supply chain: a comparison of centralized and decentralized systems, J. Environ. Manage., 2022, 315, 115141, DOI:10.1016/j.jenvman.2022.115141.
- M. Kuisma and H. Kahiluoto, Biotic resource loss beyond food waste: agriculture leaks worst, Resour. Conserv. Recycl., 2017, 124, 129–140, DOI:10.1016/j.resconrec.2017.04.008.
- M. V. Barros, R. Salvador, A. C. de Francisco and C. M. Piekarski, Mapping of research lines on circular economy practices in agriculture: from waste to energy, Renew. Sustain. Energy Rev., 2020, 131, 109958, DOI:10.1016/j.rser.2020.109958.
- P. Stegmann, M. Londo and M. Junginger, The circular bioeconomy: its elements and role in European bioeconomy clusters, Resour. Conserv. Recycl., 2020, 6, 100029, DOI:10.1016/j.rcrx.2019.100029.
- M. Dziedzic, P. R. Gomes and M. Angilella,
et al., International circular economy strategies and their impacts on agricultural water use, Clean. Eng. Technol., 2022, 8, 100504 CrossRef
https://hdl.handle.net/20.500.12552/6430
.
- T. G. Morais, C. Tufik and A. E. Rato,
et al., Estimating soil organic carbon of sown biodiverse permanent pastures in Portugal using near-infrared spectral data and artificial neural networks, Geoderma, 2021, 404, 115387, DOI:10.1016/j.geoderma.2021.115387.
- D. Taufik, J. Bolderdijk and L. Steg, Acting green elicits a literal warm glow, Nat. Clim. Change, 2015, 5, 37–40, DOI:10.1038/nclimate2449.
- K. Yrjälä, M. Ramakrishnanand and E. Salo, Agricultural waste streams as a resource in the circular economy for biochar production towards carbon neutrality, Curr. Opin. Environ. Sci. Health, 2022, 26, 100339, DOI:10.1016/j.coesh.2022.100339.
- S. Babu, S. S. Rathore, R. Singh, S. Kumar, V. K. Singh, S. K. Yadav, V. Yadav, R. Raj, D. Yadav, S. Kapila and O. A. Wani, Exploring agricultural waste biomass for energy, food and feed production and pollution mitigation: a review, Bioresour. Technol., 2022, 360 DOI:10.1016/j.biortech.2022.127566.
- V. Paramesh, R. Parajuli and E. Chakurkar,
et al., Sustainability, energy budgeting, and life cycle assessment of crop-dairy-fish-poultry mixed farming system for coastal lowlands under humid tropic condition of India, Energy, 2019, 188, 116101, DOI:10.1016/j.energy.2019.116101.
- S. Bhuvaneshwari, H. Hettiarachchi and J. N. Meegoda, Crop residue burning in India: policy challenges and potential solutions, Int. J. Environ. Res. Publ. Health, 2019, 16(5), 832, DOI:10.3390/ijerph16050832.
- G. S. Yadav, S. Babu, A. Das, K. P. Mohapatra, R. Singh, R. K. Avasthe and S. Roy, No till and mulching enhance energy use efficiency and reduce carbon footprint of a direct-seeded upland rice production system, J. Clean. Prod., 2020 DOI:10.1016/j.jclepro.122700.
- J. Song, Y. Wang and S. Zhang,
et al., Coupling biochar with anaerobic digestion in a circular economy perspective: a promising way to promote sustainable energy, environment and agriculture development in China, Renew. Sustain. Energy Rev., 2021, 144, 110973, DOI:10.1016/j.rser.2021.110973.
- N. S. Bentsen, C. Felby and B. J. Thorsen, Agricultural residue production and potentials for energy and materials services, Prog. Energy Combust. Sci., 2014, 40, 59–73, DOI:10.1016/j.pecs.2013.09.003.
-
D. Gielen, R. Gorini, N. Wagner, et al., Global Energy Transformation: a Roadmap to 2050, 2019 Search PubMed.
- R. Ulucak and F. Bilgili, A reinvestigation of EKC model by ecological footprint measurement for high middle and low-income countries, J. Clean. Prod., 2018, 188, 144–157, DOI:10.1016/j.jclepro.2018.03.191.
- C. Folke, R. Biggs and A. V. Norström,
et al., Social-ecological resilience and biosphere-based sustainability science, Ecol. Soc., 2016, 21(3) DOI:10.5751/ES-08748-210341.
-
J. Bullock, G. D. Haddow and D. P. Coppola, Introduction to Emergency Management, Butterworth-Heinemann, eBook, 2017, ISBN: 9780128171400 Search PubMed.
- T. Garnett, M. C. Appleby and A. Balmford,
et al., Sustainable intensification in agriculture: premises and policies, Science, 2013, 341, 33–34, DOI:10.1126/science.123448.
- Q. Chai, T. Nemecek and C. Liang,
et al., Integrated farming with intercropping increases food production while reducing environmental footprint, Proc. Natl. Acad. Sci. U.S.A., 2021, 118(38), e2106382118 CrossRef CAS PubMed.
- M. L. Martínez, A. Intralawan and G. Vázquez,
et al., The coasts of our world: ecological, economic and social importance, Ecol. Econ., 2007, 63, 254–272, DOI:10.1016/j.ecolecon.2006.10.022.
- S. Lu, X. Bai, W. Li and N. Wang, Impacts of climate change on water resources and grain production, Technol. Forecast. Soc. Change, 2019, 143, 76–84, DOI:10.1016/j.techfore.2019.01.015.
- R. Lal, Food security impacts of the “4 per Thousand” initiative, Geoderma, 2020, 374, 114427, DOI:10.1016/j.geoderma.2020.114427.
- L. Hoagland, L. Hodges and G. Helmers,
et al., Labor availability in an integrated agricultural system, J. Sustain. Agric., 2010, 34(5), 532–548, DOI:10.1080/10440046.2010.484697.
- L. L. Kjaer, D. C. Pigosso and M. Niero,
et al., Product/service-systems for a circular economy: the route to decoupling economic growth from resource consumption?, J. Ind. Ecol., 2019, 23(1), 22–35, DOI:10.1111/jiec.12747.
- S. Babu, R. Singh and R. Avasthe,
et al., Intensification of maize (Zea mays)-based cropping sequence in rainfed ecosystem of Sikkim Himalayas for improving system productivity, profitability, employment generation and energy-use efficiency under organic management conditions, Indian J. Agric. Sci., 2016, 86(6), 778–784 Search PubMed.
- G. Pratibha, I. Srinivas and K. Rao,
et al., Net global warming potential and greenhouse gas intensity of conventional and conservation agriculture system in rainfed semi arid tropics of India, Atmos. Environ., 2016, 145, 239–250, DOI:10.1016/j.atmosenv.2016.09.039.
- G. Sreenivas, P. Mahesh and J. Subin,
et al., Influence of Meteorology and interrelationship with greenhouse gases (CO2 and CH4) at a suburban site of India, Atmos. Chem. Phys., 2016, 16, 3953–3967, DOI:10.5194/acp-16-3953-2016.
- G. S. Yadav, R. Lal and R. S. Meena,
et al., Energy budgeting for designing sustainable and environmentally clean/safer cropping systems for rainfed rice fallow lands in India, J. Clean. Prod., 2017, 158, 29–37, DOI:10.1016/j.jclepro.2017.04.170.
- R. Lal, Reducing carbon footprints of agriculture and food systems, Carbon Footprints, 2022, 1, 3, DOI:10.20517/cf.2022.05.
-
T. F. Stocker, D. Qin, G. K. Plattner, et al., The Physical Science Basis. Contribution of Working Group I to the Fifth Assessment Report of IPCC the Intergovernmental Panel on Climate Change. 2014, 2013 Search PubMed.
- S. S. Rathore, S. Babu and K. Shekhawat,
et al., Designing energy cum carbon-efficient environmentally clean production system for achieving green economy in agriculture, Sustain. Energy Technol. Assessments, 2022, 52(Part B) DOI:10.1016/j.seta.2022.102190.
- F. N. Tubiello, M. Salvatore and A. F. Ferrara,
et al., The contribution of agriculture, forestry and other land use activities to global warming, 1990–2012, Glob. Change Biol., 2015, 21, 2655–2660, DOI:10.1111/gcb.1286.
-
H. Eggleston, L. Buendia, K. Miwa, T. Ngara and K. Tanabe, 2006 IPCC Guidelines for National Greenhouse Gas Inventories, 2006 Search PubMed.
-
E. C. Buendia, S. Guendehou, B. Limmeechokchai, R. Pipatti, Y. Rojas, R. Sturgiss, K. Tanabe and T. Wirth, Refinement to the 2006, IPCC Guidelines for National Greenhouse Gas Inventories, 2019, https://www.ipcc.ch/site/assets/uploads/2019/12/19R_V0_01_Overview.pdf Search PubMed.
-
IPCC, 2006 IPCC Guidelines for National Greenhouse Gas Inventories, IPCC, Geneva, Switzerland, 2006 Search PubMed.
-
N. Alexandratos, World Agriculture: towards 2010: an FAO Study, 1995 Search PubMed.
- FAO, FAOSTAT, Food and Agriculture Organization of the United Nations, 2010.
-
SAS, Statistical Analysis System, SAS Release 9.1 for Windows, SAS Institute Inc, Cary, NC, USA, 2003 Search PubMed.
- H. F. Kaiser, The application of electronic computers to factor analysis, Edu. Psychol. Meas., 1960, 20(1), 141–151, DOI:10.1177/001316446002000116.
- J. Das, V. Poonia, S. Jha and M. K. Goyal, Understanding the climate
change impact on crop yield over Eastern Himalayan Region: ascertaining GCM and scenario uncertainty, Theor. Appl. Climatol., 2020, 142(1), 467–482, DOI:10.1007/s00704-020-03332-y.
- K. Sartor, Y. Restivo, P. Ngendakumana and P. Dewallef, Prediction of SOx and NOx emissions from a medium size biomass boiler, Biomass Bioenergy, 2014, 65, 91–100, DOI:10.1016/j.biombioe.2014.04.013.
- M. Martins, S. Sant'Anna and M. Zaman,
et al., Strategies for the use of urease and nitrification inhibitors with urea: impact on N2O and NH3 emissions, fertilizer-15N recovery and maize yield in a tropical soil, Agric. Ecosyst. Environ., 2017, 247, 54–62, DOI:10.1016/j.agee.2017.06.021.
- J. F. Soussana and G. Lemaire, Coupling carbon and nitrogen cycles for environmentally sustainable intensification of grasslands and crop-livestock systems, Agric. Ecosyst. Environ., 2014, 190, 9–17, DOI:10.1016/j.agee.2013.10.012.
- L. Domiciano, M. Mombach and P. Carvalho,
et al., Performance and behavior of Nellore steers on integrated systems, Anim. Prod. Sci., 2016, 58, 920–929, DOI:10.1071/AN16351.
- M. A. Sanderson, D. Archer and J. Hendrickson,
et al., Diversification and ecosystem services for conservation agriculture: outcomes from pastures and integrated crop-livestock systems, Renew. Agric. Food Syst., 2013, 28(2), 129–144, DOI:10.1017/S1742170513000124.
- L. Bell, W. A. D. Moore and J. A. Kirkegaard, Evolution in crop-livestock integration systems that improve farm productivity and environmental performance in Australia, Eur. J. Agron., 2014, 57, 10–20, DOI:10.1016/j.eja.2013.04.007.
- L. W. Bell and A. D. Moore, Integrated crop-livestock systems in Australian agriculture: trends, drivers and implications, Agric. Syst., 2012, 111, 1–12, DOI:10.1016/j.agsy.2012.04.003.
- R. Singh, G. Chung and R. Nelson, Landmark research in legumes, Genome, 2007, 50(6), 525–537, DOI:10.1139/G07-037.
- S. Dhyani and M. Thummarukuddy, Ecological engineering for disaster risk reduction and climate change adaptation, Environ. Sci. Pollut. Res., 2016, 23(19), 20049–20052, DOI:10.1007/s11356-016-7517-0.
- M. Kumar, S. Hazarika and B. Choudhury,
et al., Liming and integrated nutrient management for enhancing maize productivity on acidic soils of northeast India, Indian J. Hill Farm, 2012, 25(1), 36–38 Search PubMed.
- C. Jayanthi, P. Malarvizhi, K. A. Fazullah and C. Chinnusamy, Integrated nutrient management in forage oat (Avena sativa), Indian J. Agron., 2002, 47(1), 130–133 Search PubMed.
- N. Verhulst, F. Kienle and K. D. Sayre,
et al., Soil quality as affected by tillage-residue management in a wheat-maize irrigated bed planting system, Plant Soil, 2011, 340(1), 453–466, DOI:10.1007/s11104-010-0618-5.
- G. S. Yadav, A. Das and B. Kandpal,
et al., The food-energy-water-carbon nexus in a maize-maize- mustard cropping sequence of the Indian Himalayas: an impact of tillage-cum-live mulching, Renew. Sustain. Energy Rev., 2021, 151, 111602, DOI:10.1016/j.rser.2021.111602.
- M. Kumar, S. Dahiya and P. Ratwan, Backyard poultry farming in India: a tool for nutritional security and women empowerment, Biol. Rhythm. Res., 2021, 52(10), 1476–1491, DOI:10.1080/09291016.2019.1628396.
- I. Gelfand, S. S. Snapp and G. P. Robertson, Energy efficiency of conventional, organic, and alternative cropping systems for food and fuel at a site in the US Midwest, Environ. Sci. Technol., 2010, 44(10), 4006–4011, DOI:10.1021/es903385g.
- V. Korikanthimath and B. Manjunath, Integrated farming systems for sustainability in agricultural production, Indian J. Agron., 2009, 54(2), 140–148 Search PubMed.
- A. Mohammadi, S. Rafiee and A. Jafari,
et al., Energy use efficiency and greenhouse gas emissions of farming systems in north Iran, Renew. Sustain. Energy Rev., 2014, 30, 724–733, DOI:10.1016/j.rser.2013.11.012.
- Y. Assefa, S. Yadav and K. Mondal,
et al., Crop diversification in rice-based systems in the polders of Bangladesh: yield stability, profitability, and associated risk, Agric. Syst., 2021, 187, 102986, DOI:10.1016/j.agsy.2020.102986.
- K. Esengun, G. Erdal, O. Gündüz and H. Erdal, An economic analysis and energy use in stake-tomato production in Tokat province of Turkey, Renew. Energy, 2007, 32(11), 1873–1881, DOI:10.1016/j.renene.2006.07.005.
- C. Daugbjer and P. H. Feindt, Post-exceptionalism in public policy: transforming food and agricultural policy, J. Eur. Publ. Pol., 2017, 24(11), 1565–1584, DOI:10.1080/13501763.2017.1334081.
- R. Seppelt, S. Lautenbach and M. Volk, Identifying trade-offs between ecosystem services, land use, and biodiversity: a plea for combining scenario analysis and optimization on different spatial scales, Curr. Opin. Environ. Sustain., 2013, 5(5), 458–463, DOI:10.1016/j.cosust.2013.05.002.
- G. Lemaire, A. Franzluebbers, P. C. de Faccio Carvalho and B. Dedieu, Integrated crop-livestock systems: strategies to achieve synergy between agricultural production and environmental quality, Agric. Ecosyst. Environ., 2014, 190, 4–8, DOI:10.1016/j.agee.2013.08.009.
-
P. Saling, Sustainability management in strategic decision-making processes, Uwf UmweltWirtschaftsForum|Sustainability Management Forum, Springer, 2017, DOI:10.1007/s00550-017-0461-8.
- D. Maye, H. Chiswell, M. Vigani and J. Kirwan, Present realities and the need for a lived experience perspective in Brexit agri-food governance, Space Polity, 2018, 22(2), 270–286, DOI:10.1080/13562576.2018.1519390.
- H. Cicek, J. R. T. Martens, K. C. Bamford and M. H. Entz, Late-season catch crops reduce nitrate leaching risk after grazed green manures but release N slower than wheat demand, Agric. Ecosyst. Environ., 2015, 202, 31–41, DOI:10.1016/j.agee.2014.12.007.
-
MoEFCC, India: Third Biennial Update Report to the United Nations Framework Convention on Climate Change, Ministry of Environment, Forest and Climate Change, Government of India, 2021, https://unfccc.int/sites/default/files/resource/INDIA_%20BUR-3_20.02.2021_High.pdf Search PubMed.
-
The Wire, Climate Change: People of Asia's Mountains Face Severe Food Insecurity, https://thewire.in/environment/climate-change-cop14-hindu-kush-himalaya-land-degratation, accessed on 31st October 2022 Search PubMed.
|
This journal is © The Royal Society of Chemistry 2023 |
Click here to see how this site uses Cookies. View our privacy policy here.