Modelling PCB-153 in northern ecosystems across time, space, and species using the nested exposure model†
Received
31st October 2022
, Accepted 1st October 2023
First published on 6th October 2023
Abstract
There is concern over possible effects on ecosystems and humans from exposure to persistent organic pollutants (POPs) and chemicals with similar properties. The main objective of this study was to develop, evaluate, and apply the Nested Exposure Model (NEM) designed to simulate the link between global emissions and resulting ecosystem exposure while accounting for variation in time and space. NEM, using environmental and biological data, global emissions, and physicochemical properties as input, was used to estimate PCB-153 concentrations in seawater and biota of the Norwegian marine environment from 1930 to 2020. These concentrations were compared to measured concentrations in (i) seawater, (ii) an Arctic marine food web comprising zooplankton, fish and marine mammals, and (iii) Atlantic herring (Clupea harengus) and Atlantic cod (Gadus morhua) from large baseline studies and monitoring programs. NEM reproduced PCB-153 concentrations in seawater, the Arctic food web, and Norwegian fish within a factor of 0.1–31, 0.14–3.1, and 0.09–21, respectively. The model also successfully reproduced measured trophic magnification factors for PCB-153 at Svalbard as well as geographical variations in PCB-153 burden in Atlantic cod between the Skagerrak, North Sea, Norwegian Sea, and Barents Sea, but estimated a steeper decline in PCB-153 concentration in herring and cod during the last decades than observed. Using the evaluated model with various emission scenarios showed the important contribution of European and global primary emissions for the PCB-153 load in fish from Norwegian marine offshore areas.
Environmental significance
We have developed, evaluated and applied an integrated, dynamic and spatially resolved fate and bioaccumulation model (NEM) for organic contaminants that enables future model applications of regulatory and scientific interest such as (i) quantifying the contribution of national, regional, or global emission sources to the observed contaminant concentrations in different species and ecosystems, (ii) estimating changes in ecosystem exposure as a result of both changing primary emissions and a changing climate, and (iii) evaluating environmental fate and bioaccumulation for not yet regulated chemicals to assist scientifically sound regulatory decision making.
|
Introduction
Legacy persistent organic pollutants (POPs) are regulated on a global scale due to their persistence, toxicity, and potential for bioaccumulation and long-range transport.1 As a result of these properties, POPs are ubiquitous in the environment, also in Arctic ecosystems far away from emission sources.2,3 For instance, polychlorinated biphenyls (PCBs) reaching high concentrations in Arctic top predators such as seabirds and polar bears,4–6 are still of ecotoxicological concern decades after they were prohibited.7,8 PCBs are of global concern and are found in marine environments and biota across the globe.9–17
Marine ecosystems are a key source of nutrients and contaminants for both marine top predators18–20 and humans,21,22 with fish playing a key role as vectors of contaminant exposure. Hence, understanding contaminant sources, pathways and exposure of marine fish is also vital for understanding and managing contaminant exposure of humans and top predators. The Norwegian marine areas are important commercial fishing grounds,23 as well as feeding areas for numerous seabird, seal, and whale species. These areas range from the North Sea and Skagerrak impacted by local sources in the south, to the remote Barents Sea in the north where long-range environmental transport is the main source. Concentrations of POPs such as PCBs are routinely monitored here in the atmosphere,24 sediments,25 and fish.26–28 In addition, field-based research contributes important insights into POPs exposure across environmental media29,30 and trophic levels.31,32 However, environmental sampling and chemical analysis for POPs are expensive and time-consuming, limiting the availability of field-based measurements across time, space, and species. Moreover, measured concentrations alone are often not enough to provide information on contaminant sources, particularly when exposure may arise from global emissions. As a result, a comprehensive understanding of the full link between global emissions and biotic exposure is difficult to achieve based on measurements alone.
Multimedia physical fate and bioaccumulation models have been developed to further increase our understanding of the environmental fate and bioaccumulation behavior of organic contaminants.33 Objectives of earlier modeling studies on contaminants in fish and biota in Norwegian marine areas included increasing the understanding of bioaccumulation in Arctic marine species and food webs32,34,35 and how this may be impacted by climate change.36 Models have also been used to identify chemicals of concern,37 and to elucidate the role of plastic in organic contaminant bioaccumulation38 in this region. Most of these studies relied on steady-state models not accounting for temporal or spatial variability, even though high latitude ecosystems are highly dynamic due to a strong seasonality in light regimes, temperatures, primary production, etc. An exception is the OMEGA model, which has been used to investigate the seasonality of PCB bioaccumulation in the Barents Sea34 as well as time-trends of a range of contaminants.37 However, this model is not spatially resolved. Environmental fate and bioaccumulation models can greatly benefit from taking into account that emissions and environmental characteristics vary in space and time.39 In fact, increased capability for estimating temporally and spatially variable concentrations has been identified as a key research need to improve exposure estimation in ecological risk assessment in the 21st century.40,41
Furthermore, none of the earlier studies modeling bioaccumulation in Norwegian marine areas included a simulation of chemical fate and transport in the physical environment. They instead relied either on aqueous concentrations as input, taken from measurements in water32,35,38 or derived from measured concentrations in biota,32,35,37 or on hypothetical scenarios.34,36 This approach does not facilitate an understanding of the whole link between chemical emissions to the environment and biotic exposure, which is crucial for enabling scientifically-sound management strategies. Combined models of environmental fate and bioaccumulation have proven fruitful to increase our understanding of contaminant behavior and exposure. An example is the CoZMoMAN model42 which has been used both to evaluate the environmental fate of short-chain chlorinated paraffins in the Nordic environment43 and to increase our understanding of human exposure to PCBs.44,45 The CoZMoMAN model is limited by its lack of spatial resolution and because it cannot realistically model contaminant transport into the model domain from the outside world. The bioaccumulation model ACC-Human46 has been combined with global dynamic and spatially resolved environmental fate models like Globo-POP2,47,48 and BETR-Global49 to quantitatively describe the whole link between global contaminant emissions and exposure to humans and wildlife. For example, BETR-Global and ACC-Human have been used to estimate human exposure to PCB-153 on a global scale.50,51 Also, Globo-POP and an Arctic version of ACC-Human have been used to distinguish the physico-chemical properties of chemicals likely to be Arctic contaminants.3 Furthermore, Globo-POP and an expanded version of the Arctic ACC-Human model have been used in several studies to increase the understanding of PCB exposure in both wildlife52 and Arctic indigenous human populations in the Canadian Arctic.53–55
Recently, the Nested Exposure Model (NEM)56 was introduced for the physical environment as a global dynamic multimedia environmental fate and transport model that can zoom into a user-defined region of interest with increasing spatial resolution. The nested feature of NEM reduces computational demands, while allowing for simulations that are global in scale yet with a high spatial resolution in the region of interest. NEM has been shown to satisfactorily reproduce fate and concentrations of PCB-153 in the European atmosphere, with differences between observations and model estimates comparable to sampling and analytical variability across involved laboratories.56 However, NEM has not yet been evaluated for environmental compartments other than air. Moreover, while Breivik et al.56 noted that “NEM is also integrated with the ACC-Human bioaccumulation model”, this was not further evaluated or explored. Hence, the main objectives of this study are to (i) further develop NEM for northern marine ecosystems, (ii) evaluate NEM's ability to estimate PCB-153 concentrations across time, space, and species, and (iii) apply the model to explore the sources of PCB-153 to northern marine ecosystems. The focus of the evaluation is on an Arctic marine food web in Svalbard, Norway, and on two ecologically and commercially important fish species (Atlantic herring (Clupea harengus) and Atlantic cod (Gadus morhua)). These case-studies were selected because of the availability of consistent measured data across time, space, and species and their relevance to management for protecting northern ecosystems and human health.
Materials and methods
The NEM model structure
The dynamic and spatially resolved fugacity-based NEM model (Fig. 1) consists of two parts: (i) a module that simulates environmental fate and behavior of organic contaminants in the physical environment56 and (ii) a bioaccumulation module based on the ACC-Human,46 Arctic ACC-Human,3 and expanded Arctic ACC-Human models.52,54 NEM uses global emission scenarios to estimate concentrations in air, water, soil, and sediment in a user-defined domain (in time and space) with a user-defined spatial resolution ranging from 30° × 30° latitude/longitude (lat/long) to 0.5° × 0.5° lat/long. The nested feature of NEM means that it is possible to model the entire globe with a coarse resolution, then zoom into an area of interest with increased spatial resolution while accounting for chemical inflow from the outside world into the nested domain. In doing this, the model uses the output from a larger domain with coarser resolution as boundary conditions for a smaller domain with finer resolution.56 Next, estimated concentrations or fugacities in the physical environment are used to simulate bioaccumulation behavior of organic contaminants in a pelagic food web typical for Norwegian marine ecosystems (Fig. 1). The bioaccumulation module can be run for a user-defined spatial domain that is embedded in the domain of the physical module with the same spatial resolution. Currently, organisms cannot move between grid cells, but are assumed to remain within a single grid cell throughout their life. This simplification can lead to errors when assessing contaminant exposure of migratory species. The temporally resolved concentrations or fugacities in the physical environment driving the bioaccumulation module in NEM need not be output of the physical fate module, but can be user-generated files, e.g. based on environmental measurements.
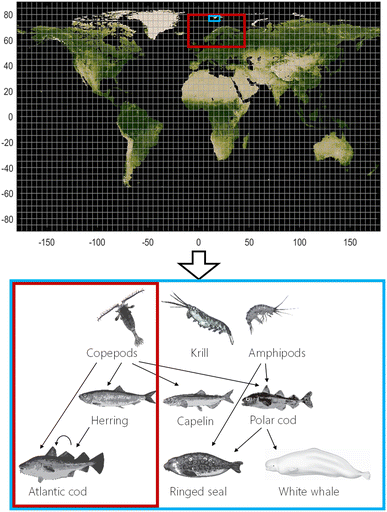 |
| Fig. 1 Illustration of the NEM version used in this study. A global environmental fate module is used to run dynamic simulations with 5° × 5° lat/long resolution, with subdomains used for the Norwegian marine areas (red) and the Svalbard region (blue) with increased spatial resolution (1° × 1°) highlighted. Concentrations are estimated in three (red) and nine (blue) selected species for the subdomain in the same color. Arrows illustrate feeding relationships. | |
Species included and biota parameterization
Nine species of key importance to Norwegian sub-Arctic and Arctic pelagic marine ecosystems make up the food web in the current version of NEM (Fig. 1):57 three pelagic zooplankton groups with different feeding strategies58,59 (copepods, krill, and amphipods, ESI S1.1†), four fish species (Atlantic herring,46 capelin (Mallotus villosus),52 polar cod (Boreogadus saida),3 and Atlantic cod46), and two Arctic marine mammals (ringed seal (Pusa hispida)3,52,60 and white whale (Delphinapterus leucas)52). Existing modules for lichen, caribou, narwhal, and humans are also integrated in NEM but are not included in this study.46,52,54 Contaminant concentrations in all three zooplankton groups are assumed to be in equilibrium with seawater based on bioaccumulation factors measured in King's Bay, Svalbard.59 Age-dependent lipid-normalized concentrations of contaminants in fish and mammals are calculated from mass balance equations comprising key uptake and elimination mechanisms, as described previously.3,46,52,54,60 Details of the parameterization of the organisms, especially updates to earlier versions of the Arctic ACC-HUMAN models,3,52,54,60 can be found in ESI S1.† In particular, seasonal lipid-dynamics have been included for all plankton and fish species, except for Atlantic cod which has a relatively constant lipid content (S1.1 and S1.2†). Parameterization of the two mammals has not been changed.3,52,60
Other input parameters
All environmental input parameters to the physical fate module of NEM have been described in detail previously.56 Most spatially resolved environmental parameters have a resolution of 0.5° × 0.5°, and the model automatically calculates the spatially resolved environmental parameters at the selected resolution based on these parameters at 0.5° × 0.5°.56 The bioaccumulation module uses the same spatially and temporally resolved seawater and air temperatures as direct input as used in the physical fate module of NEM.56
For evaluation and application of the model, we selected PCB-153 (2,2′,4,4′,5,5′-hexachlorobiphenyl) because (i) NEM has already been shown to reproduce PCB-153 concentrations in the European atmosphere within a factor of 1.5 of observations,56 (ii) it is still of toxicological importance in wildlife,7 (iii) empirical data for PCB-153 in marine and Arctic biota is plentiful, and (iv) a global historical emission scenario61,62 is available. The most up-to-date default global emission scenario for PCB-153 from 1930 to 2100 (ref. 63) was used as input, assuming emissions solely to air, as in Breivik et al.56 Physical-chemical property data used as input for PCB-153, including equilibrium partition ratios between air and water (KAW), octanol and water (KOW), and octanol and air (KOA)64 and their temperature dependence were also harmonized with the NEM module for the physical environment and are described in Breivik et al.56 Biotransformation of PCB-153 was assumed negligible in zooplankton and fish. Biotransformation rates and feces-blood partition coefficients (KFB) of PCB-153 in the mammals were used as in Binnington et al.52
Simulations for model evaluation
Simulations for PCB-153 in the physical environment were carried out for the years 1930 to 2020, starting with global simulations at 5° × 5° resolution, followed by additional simulations for the Norwegian marine areas and bordering regions (55°N – 80°N, 10°W – 45°E) at 1° × 1° resolution (Fig. 1). Based on the calculated fugacities of PCB-153 in the atmospheric gas phase and in the dissolved seawater phase, PCB-153 fugacities and concentrations were estimated for zooplankton, cod, and herring with spatial resolutions of both 5° × 5° and 1° × 1° (Fig. S2†). Simulations for all species, including marine mammals, were run for the Svalbard region only (75°N – 80°N, 10°E − 20°E) (Fig. S2†), also at both resolutions. We ran simulations for all species for the Svalbard region only because measurement data for model evaluation are available for this region and to save computing time. All simulations were run with a 12 h time-step, with results stored after every 1752 h (73 days), and results based on both spatial resolutions are presented.
Measurement data for model evaluation
First, we evaluated the model by comparing estimated PCB-153 concentrations in the dissolved seawater phase with measurements in the dissolved phase in surface seawater samples taken from areas within the model domain (including the Baltic Sea, Skagerrak, North Sea, Norwegian Sea, and Svalbard/Barents Sea region) (Fig. S3†).32,59,65–67 When a sample had been collected along ship transects spanning up to several degrees latitude/longitude, the median and range of concentrations estimated at the time of sampling in the grid cells covering the transect were used for comparison.
Next, we used the two case-studies (“Svalbard” and “Norwegian fish”) for more detailed model evaluation. For evaluation of model performance across compartments and species in the Svalbard area (case-study “Svalbard”), we compared estimated concentrations with PCB-153 concentrations measured in bulk air at the Zeppelin observatory (2007–2016),68 in dissolved seawater phase (July 2008)59 and whole-body zooplankton, herring, polar cod and Atlantic cod (May–Oct 2007)31 from King's Bay, Svalbard (Fig. S4†), as well as in blubber of ringed seal (May–Sept 2014) and white whale (July–August 2014–2016) from the larger Svalbard area (Fig. S5†).69 King's Bay falls entirely within one grid cell based on NEM's spatial resolution of 5° × 5°, but is located at the intersection of four cells in a 1° × 1° grid (Fig. S4†). Ringed seal and white whale were sampled across Svalbard, spanning two 5° × 5° grid cells (Fig. S5†). For estimates based on 1° × 1°, the median and range of concentrations estimated for the three grid cells covering most of King's Bay (Fig. S4†) were used. For estimates based on 5° × 5°, median and range of estimated concentrations in the one (air, seawater, plankton, and fish) or two cells (marine mammals) covering King's Bay and the wider Svalbard region, respectively, were used. In all cases, concentrations estimated for the same year and season as when the samples were collected were used for comparison. For fish and white whale, estimated concentrations for all age groups were used for comparison. For ringed seal whose age had been determined,69 only estimates for seal aged 5–20 years (females) and 0–30 years (males) were used to increase comparability to the measured data. For marine mammals, the median estimated concentration in total blubber was used.69
For evaluation of model performance across space and time, we relied on an extensive dataset of PCB-153 concentrations measured in muscle of Norwegian spring-spawning (NSS) herring and liver of Atlantic cod from large baseline and monitoring studies in Norwegian marine areas performed by the Institute of Marine Research (case-study “Norwegian fish”) (Fig. S6 and Table S1†).26,28,70 This dataset includes measured data from the Skagerrak, North Sea, Norwegian Sea and Barents Sea, as well as Norwegian coastal and fjord areas (Fig. S6–S8†) covering the years 1995–2017 for herring (n = 1254) and 2002–2018 for cod (n = 3651). Some of the cod have been sampled in areas impacted by local sources, mostly from historically PCB-contaminated sediment acting as a secondary source to the local marine environment. These samples were flagged as “may be impacted”, “slightly impacted”, or “heavily impacted” (Table S1†), based on the relative vicinity of the sampling location to local sources. Heavily impacted samples (e.g., from the inner Oslofjord and Bergen harbor) (n = 229) were removed from the dataset, as the spatial resolution of NEM is too coarse to resolve concentration gradients at the local scale. Estimated lipid-normalized concentrations (ng per g lipid weight (lw)) of PCB-153 in individual fish were used for comparison, if the measured data had accompanying information about (i) lipid content and (ii) age of the fish, (iii) the time of sampling (date and year), and (iv) the sampling position (in lat/long) (Table S1†). This was the case for more than 3000 fish (n = 783 for herring, n = 2291 for cod). For these, the measured lipid-normalized PCB-153 concentration in individual fish was compared to the estimated lipid-normalized PCB-153 concentration for a cod or herring of the same age at the time and location closest to the time and place of sampling. For sampled fish older than 10 years, the estimated concentration for a 10 year-old fish was used (n = 38 for herring and n = 4 for cod). Because such auxiliary data are missing for fish sampled prior to 2006, earlier data (n = 310 for herring, n = 29 for cod) were only included to evaluate the overall time-trends on a ng per g wet weight (ww) basis. The NEM model was evaluated for its ability to reproduce (i) PCB-153 concentrations in individual fish, (ii) spatial trends of PCB-153 in fish, and (iii) temporal trends of PCB-153 in fish.
Exploration of emission scenarios
Three country- or region-specific emission scenarios for PCB-153 for 1930–2020 were explored to evaluate the impact on concentrations in Norwegian fish: (1) primary emissions within Norway only, (2) primary emissions within the countries that were European Union (EU) members as of 1973 only (Germany, France, Italy, Netherlands, Belgium, Luxembourg, Denmark, Ireland, United Kingdom), and (3) primary emissions within Russia only. The results from these scenarios were compared to the default scenario based on global emissions to investigate the relative contribution of historical primary emissions in Norway, EU, and Russia to PCB-153 contamination in Norwegian fish. For this purpose, estimated lipid-normalized concentrations in a 5 year-old herring were compared between scenarios. For example, the contribution from Norwegian primary emissions to the total body burden of PCB-153 concentrations in a 5 year old herring was calculated as the estimated concentrations in the herring based on primary emissions within Norway only (scenario 1) divided by the estimated concentrations in herring based on global emissions (default scenario). As the model responds linearly to emission changes, results will be identical for cod and herring in the same grid cell.
Evaluation of model performance
To evaluate model performance, several quantitative metrics were used. The ratio between estimated and measured concentrations (prediction-measurement ratio, PMR) can be calculated for individual pairs of estimated and measured concentrations and provides information on both the extent of agreement between estimates and measurements (the closer to 1, the better agreement) and direction of agreement (underprediction (PMR < 1) or overprediction (PMR > 1)).
It has been argued that an agreement between model estimates and field measurements of an order of magnitude can be used as a rule of thumb when evaluating the performance of multimedia models.71 This compares to a PMR between 0.1 and 10.
To evaluate overall model performance, the model bias (MB)72 was calculated as
Hence, MB is the geometric mean of the PMRs for e.g. all species (Svalbard) or all individual cod or herring (Norwegian fish), and provides information on the overall performance of the model, including the extent (the closer to 1, the better agreement) and direction of agreement (underprediction (MB < 1) or overprediction (MB > 1)).72
Similarly, the root mean square error of the log-transformed concentrations39 was calculated as
and summarizes both random error and systematic bias.
39 The smaller RMSE
log is, the better model performance.
In addition, Spearman's coefficient (rs) was calculated for correlations between estimated and measured PCB-153 concentrations in individual organisms. The higher rs is, the better the correlation.
Results and discussion
Model evaluation for seawater
NEM has already been shown to successfully estimate PCB-153 concentrations in air.56 Prior to applying NEM to marine biota, we evaluated its ability to estimate PCB-153 concentrations in seawater. Measured concentrations included in the evaluation ranged from 0.02 pg L−1 (Barents Sea, 1999)32 to 12.6 pg L−1 (Skagerrak, 1989),66 spanning more than two orders of magnitude. This range represents both a temporal decline due to the global phase-out of PCBs as well as spatial variation due to variable distance from source areas (see map in Fig. S3†). Measuring concentrations of PCB-153 dissolved in seawater is difficult due to its low water solubility, and the measurements have relatively high inherent uncertainty.32 Estimated concentrations agreed better with measurements when based on a spatial resolution of 1° × 1° (MB = 1.9, RMSElog = 5.9) than 5° × 5° (MB = 3.2, RMSElog = 6.9) (Fig. S9†). This mirrors the results of the model evaluation for the atmosphere and illustrates the effect of numerical diffusion.56 Based on 1° × 1°, the PMR in seawater ranged from 0.1 to 31, with 64% of estimates within a factor of 4 of measurements (n = 33) (Fig. S9†).
Model evaluation across species (Svalbard)
Estimated concentrations (1° × 1° resolution) of PCB-153 in air and seawater for King's Bay displayed PMRs of 0.89 and 1.1, respectively (Table S2†). Estimated concentrations in plankton, fish and marine mammals from King's Bay and Svalbard are compared to measurements in Fig. 2 (1° × 1° resolution) and S10 (5° × 5° resolution).† As discussed previously for the atmosphere56 and seawater (above), model estimates for Svalbard biota agreed best with measurements based on the spatial resolution of 1° × 1° (Table S2 and Fig. 2, S10†), demonstrating again improved model performance at increased spatial resolution. This is partly due to the good agreement between measured and estimated seawater concentrations in King's Bay (PMR = 1.1), because the estimated concentrations in water-respiring biota scale linearly with the seawater concentrations (i.e., if water concentrations are doubled, so do concentrations in biota). Seen in the context of the overall evaluation of seawater concentrations where PMRs range from 0.1 to 31 (1° × 1°, Fig. S9†), this may be partly fortuitous.
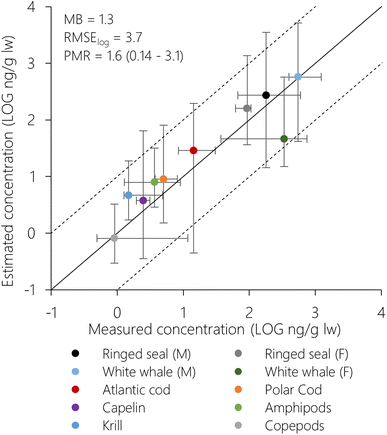 |
| Fig. 2 Model evaluation across species in Svalbard for PCB-153 based on 1° × 1° resolution. Median (point) and range (variability bars) of estimated concentrations are plotted against median (point) and range (variability bars) of measured concentrations. M = male, F = female. The solid diagonal line represents a perfect match between estimated and measured concentrations, while dashed diagonal lines show deviations of one order of magnitude. The ranges for the measured concentrations do not include concentrations below the limit of detection. | |
Model estimates (1° × 1°) across species displayed PMRs between 0.14 and 3.1, an overall MB of 1.3 and RMSElog of 3.7 (Table S2†). Estimated concentrations in female white whale displayed the greatest deviation from measurements and were underpredicted compared to measurements (PMR = 0.14). This was also observed for PCB-153 estimates in female white whales in the Canadian Arctic using the Expanded Arctic ACC-Human model by Binnington et al.,52 who suggested that the model overestimates reproductive loss of PCBs through calving and/or lactation. If female white whale is excluded, the PMR varied between 0.89–3.1 across plankton, fish, and marine mammals. This is deemed acceptable considering all inherent uncertainties in input properties, model assumptions, as well as measurement data. Most importantly, this means that NEM succeeds in simulating the whole continuum of processes linking global historical emissions of PCB-153 with marine ecosystem exposure in the Norwegian Arctic, including bioaccumulation across trophic levels.
To investigate NEMs ability to reproduce bioaccumulation across trophic levels in more detail, we also compared measured and estimated trophic magnification factors (TMF) using the data in Fig. 2. For zooplankton groups and fish species, average trophic positions (TP) from Hallanger et al.31 were used. For mammals, trophic positions were calculated from stable nitrogen ratios (δ15N) in skin,73 measured in the same individuals of ringed seal and white whale as the PCB-153 concentrations.69 The TP of the marine mammals and the TMFs were calculated following the method by Hallanger et al.31 for consistency. Separate TMFs were calculated for poikilotherms only (zooplankton and fish) and for all organisms (including ringed seal and white whale), as including homeotherms can significantly impact calculated TMFs. Linear regressions between log-normalized PCB-153 concentrations and TP showed significant positive relationships for both measurements and estimates (Fig. S11†). The TMFs for poikilotherms were 3.89 and 4.21 for measurements and estimates, respectively. This is within, or close to, the range of the seasonal TMFs for PCB-153 (1.53–4.07) calculated by Hallanger et al.31 based on the same measurement data, but including some more species of fish (haddock and pollock) and zooplankton (arrow worms (Chaetognatha)). When we included the homeotherms in the regressions, the TMFs increased to 8.82 and 6.08 for measurements and estimates, respectively. Increased TMFs when homeotherms are included have also been seen previously.31,74 There was a larger discrepancy between measured and estimated TMF when homeotherms were included. This is partly due to the underprediction of PCB-153 concentrations in female white whale. If female white whale is excluded from the regression of the estimated concentrations, the estimated TMF increase to 7.51.
Model evaluation across space and time (Norwegian fish)
Maps showing the spatial variation in estimated concentrations of PCB-153 in herring and cod are shown in Fig. 3, exemplified for January 2020. Model evaluations for PCB-153 in individual fish based on 1° × 1° and 5° × 5° resolutions are shown in Fig. S13† and 4, respectively, and are summarized in Tables S3 and S4.† For the whole dataset, the median measured PCB-153 concentrations were 10.6 (0.9–102) and 71.5 (3.1–5934) ng per g lw in herring muscle (n = 783) and cod liver (n = 2291), respectively (Table S3†). The estimated median PCB-153 concentrations in herring from NEM were 11.1 (1.2–79) and 17.9 (7.3–109) ng per g lw when using a 1° × 1° or 5° × 5° resolution, respectively (Table S3†), i.e., 1.1–1.7 times higher than the measured median. The estimated median PCB-153 concentrations in cod of 23.2 (2.9–1031, 1° × 1°) and 31.2 (6.5–527, 5° × 5°) ng per g lw were 2.3–3.1 times lower than the measured median concentration (Table S3†).
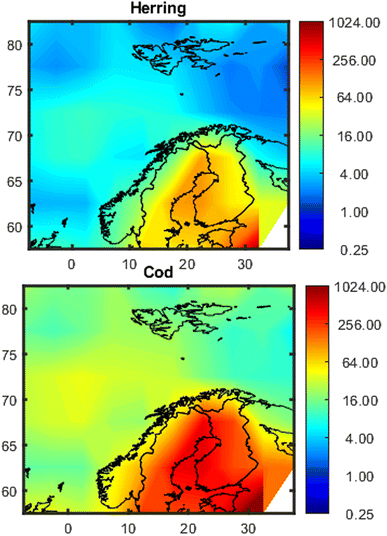 |
| Fig. 3 Maps of estimated concentrations of PCB-153 (ng per g lw) in 5-year-old herring and cod in northern European marine areas in January 2020 based on 5° × 5° resolution. The data has been interpolated with the MATLAB 2023a provided script contourf, because it gives a better impression of the concentration distribution (see Fig. S12†). | |
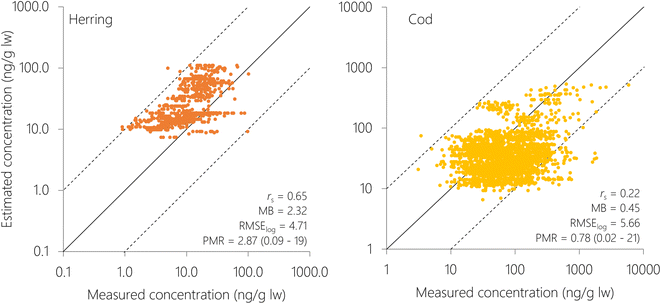 |
| Fig. 4 Model evaluation for individual estimates of PCB-153 in Norwegian spring-spawning herring and Atlantic cod in Norwegian marine areas based on 5° × 5° resolution. The solid diagonal line represents a perfect match between estimated and measured concentrations, while dashed diagonal lines show deviations of one order of magnitude. | |
Herring and cod display migratory behavior to varying degrees and have not spent their entire lives at the sampling position. Hence, the measured concentrations in fish reflect exposure from a wider geographical area than their sampling position. Northeast Atlantic cod migrates seasonally between the Barents Sea and the Norwegian Sea to spawn,75 while coastal cod and North Sea cod are more stationary.76 The NSS herring undertake seasonal migrations between coastal and offshore areas in the Norwegian Sea.77 This is not yet accounted for in NEM, where fish are assumed to reside in individual grid cells for their entire lifecycle. Hence, using a coarser spatial resolution (5° × 5°) may be more representative for their real-life exposure than the finer spatial resolution (1° × 1°). This agrees with the results obtained herein, where all metrics of model performance (PMR, MB, RMSElog and rs) for estimates of PCB-153 in individual fish were better when NEM was run with the coarse resolution (5° × 5°), except for the average PMR and model bias for herring (Table S4†). The improved MB and average PMR for herring at 1° × 1° are because estimates are more spread out with concentrations both lower and higher than measurements (Fig. S13†), whereas there is a clearer tendency for overprediction but less spread at 5° × 5° resolution (Fig. 4). To explore the effect of migration, two simplified migration scenarios for herring and cod, respectively, were constructed to explore how spatial migration impacts estimated concentrations of PCB-153 as well as model performance (5° × 5° resolution, ESI Section S8†). Overall, accounting for migration did not improve estimates for herring and cod (ESI Section S8†). Hence, the remainder of the results and discussion is based on 5° × 5° spatial resolution without accounting for migration between grid cells.
Model performance (5° × 5°) was better for individual herring than for individual cod, with a lower RMSElog (4.71 compared to 5.66), higher spearman rs (0.65 compared to 0.22), and comparable MB (2.32 compared to 0.45) (Fig. 4 and Table S4†). There was a tendency of overpredicting concentrations in herring (MB = 2.32, i.e. 2.32-fold overprediction), and underpredicting concentrations in cod (MB = 0.45, i.e. 2.22-fold underprediction), with 77% and 70% of estimates for herring and cod, respectively, within a factor 4 of measurements. NSS herring is a schooling, pelagic fish stock migrating throughout the Norwegian Sea, and is relatively homogenous in terms of diet, habitat, and geographical distribution.77 Consequently, the dataset used for model evaluation of PCB-153 in herring is also relatively homogenous. On the other hand, cod has a much more diverse diet, and may feed on both pelagic and benthic species.78,79 A benthic diet of cod is not yet accounted for in NEM. Moreover, the dataset for Atlantic cod includes several stocks; both the highly migratory Northeast Atlantic cod, and the more stationary North Sea cod and Norwegian coastal cod.76,78–80 The model does not account for substantial differences in the biology of these cod stocks, including differences in migration, life history, and diet. Furthermore, some of the cod have been sampled in areas suspected, or known, to be influenced by local (secondary) sources of pollution (Tables S1 and S5†). The larger diversity of the cod dataset may explain the poorer model performance when compared to herring, as NEM is currently not able to capture this diversity in diet, ecology, and space-use strategies.
To explore whether there was any spatial variation in the performance of NEM, the logarithm of the PMR was plotted against number, latitude, and longitude of the grid cell for both herring and cod (Fig. S14†). Even though there was variation in the PMR, there was no systematic bias with either grid cell number, latitude, or longitude. This means that there were no particular cells or spatial regions that could explain the overprediction of herring or the underprediction of cod. This also indicates that NEM performs equally well for the whole Norwegian marine area, ranging from the North Sea in the South to the Barents Sea in the North. To explore this in more detail, the Norwegian marine areas were divided into four regions (Skagerrak, North Sea, Norwegian Sea, Barents Sea) as in Nøstbakken et al.,81 and the cod measurements were allocated to these regions depending on their sampling location. Measured concentrations of PCB-153 in cod liver decreased in the sequence: Skagerrak > North Sea > Norwegian Sea > Barents Sea (Table S6, Fig. 5 and S15†), in agreement with spatial patterns observed by Ho et al.28 and Everaert et al.25 NEM reproduced this trend of decreasing concentrations from south to north (Table S6† and Fig. 5), with the exception of slightly higher estimated concentrations in the Norwegian Sea than in the North Sea, contrary to observations. Lower median estimated PCB-153 concentrations in North Sea cod compared to measurements (PMR = 0.25) than in the three other marine regions (PMRs 0.53–0.89) (Table S6 and Fig. S15, S16†) could have several explanations. Broadly, it can be caused by uncertainties in (i) the global emission estimates used as input to the model, (ii) estimated fate and persistence in the physical environment, and/or (iii) estimated bioaccumulation. NEM could reproduce measured temporal trends in air at the Birkenes observatory in southern Norway, close to the North Sea.56 This suggests that the discrepancy by NEM for cod in the North Sea is not likely to be due to the emission estimates. However, seawater and seawater sediments are expected to respond more slowly to emission changes than the atmosphere. Thus, it cannot be excluded that PCB-153 concentrations in the North Sea have responded more slowly to declining primary emissions the last decades than estimated by the model, e.g., due to underestimating secondary sources. Moreover, there could be aspects of the diet or ecology of North Sea cod that is not adequately represented in NEM and underestimates their real-life exposure. For example, North Sea cod grows faster than cod in the Barents Sea.80 As a result, North Sea cod is larger at the same age than cod in the Norwegian Sea, and could possibly be feeding at a higher trophic level. A strong benthic link, particularly in combination with historically contaminated sediment, could also underestimate their real-life exposure as the prey species for cod in NEM is currently only pelagic.
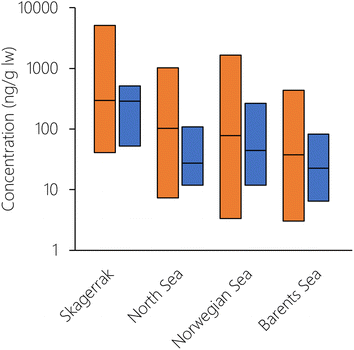 |
| Fig. 5 Comparison of median and range of measured (orange) and estimated (blue) PCB-153 concentrations (ng per g lw) in cod liver of the four different sea regions of the Norwegian marine areas included in the model evaluation. | |
The estimated temporal trends of PCB-153 concentrations in ng per g ww in herring and cod in Norwegian marine areas for 1930–2020 are shown in Fig. 6. Concentrations were not lipid-normalized to allow for the use of data for fish collected before 2006 when lipid content was not recorded. Concentrations were estimated to peak around 1980, followed by a decline, reflecting the global historical emission trend of PCB-153.61–63 The large range in estimated concentrations in Fig. 6, particularly for cod, is caused by combining results for several grid cells to look at the overall results for Norwegian marine areas (see Fig. S17–S19† for selected individual grid cells). The estimated time-trends overlapped with the measured time-trends in both herring and cod (Fig. 6). To investigate the agreement between the estimated and measured time-trends in more detail, we calculated the environmental half-lives (t1/2) of PCB-153 in herring and cod for both measured and estimated concentrations assuming first-order kinetics using eqn (1) and (2):
|  | (1) |
|  | (2) |
where
C is the PCB-153 concentration in herring or cod
t years after the first year of the measured time-series (
t0),
C0 is the median PCB-153 concentration in herring or cod at
t0, and
k is the rate constant of decline. For cod, we calculated separate half-lives for the North Sea, Norwegian Sea, and the Barents Sea, as the number of fish sampled every year in each region varies, and this can impact the calculated half-life since the PCB-153 concentrations vary between the different sea regions (
Fig. 5). Skagerrak was not included in this analysis, as the dataset contained cod sampled in Skagerrak for only two separate years.
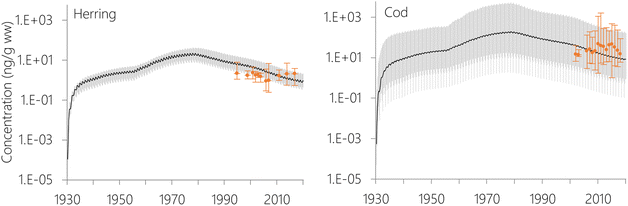 |
| Fig. 6 Evaluation of time-trends for concentrations of PCB-153 (ng per g ww) in herring and cod based on 5° × 5° resolution. The black line and the grey area represent the median and range (min-max), respectively, of estimated concentrations for all ages of fish in all grid cells where samples have been collected after 2006 (n = 10 cells for herring, n = 19 cells for cod). The orange markers and their variability bars are median and range (min-max) of the measured PCB-153 concentrations. | |
The measured concentrations showed a significant decline with time for PCB-153 only in cod liver from the Barents Sea (Fig. S20 and Table S7†), with a calculated half-life of 32.6 years. In contrast, NEM estimated a consistent, significant decline in PCB-153 concentrations for both species and all sea regions with half-lives ranging between 7.5–9.5 years. Measured PCB-153 concentrations showed non-significant declines for herring and cod in the Norwegian Sea, and a significant increase with time for North Sea cod (Fig. S20 and Table S7†). It should be noted that this is not an in-depth time-trend analysis of the measurement data, and any differences between years in size, age, lipid-content, or sampling season for the fish have not been accounted for. Hence, these results should be interpreted with caution. The results nonetheless agree with monitoring for Norwegian marine fish, showing a decline in contaminant concentrations in the 1990s followed by a stabilization of concentrations in the 2000s.82 Independent of the exact half-lives calculated for the measurement data herein, NEM estimates a steeper decline in PCB-153 concentrations in Norwegian marine fish than observed. This was also observed for certain stations in the evaluation of NEM for the atmosphere, including Stórhöfdi (Iceland) and Aspvreten (Sweden).56 Possible explanations for this include (i) uncertainties in the emission estimates, namely an overestimation of the success of efforts to reduce primary PCB emissions, (ii) uncertainties in the estimated fate and persistence of PCB-153 in the physical environment, (iii) uncertainties in estimated bioaccumulation, and (iv) environmental and/or biotic parameters that experienced a temporal trend during the twenty years of sampling, but which the model assumes to be constant. It has been suggested that climate change may perturbate observed time-trends of POPs in the physical environment and biota in the Arctic,83,84 which may also be the case for Norwegian marine areas in general. As the properties of the physical environment and biota in NEM only varies with seasons, and not over longer time scales, NEM is currently not able to capture this.
The model performance of NEM is comparable to, or better than, the performance of other bioaccumulation models that have been applied to Norwegian marine and Arctic areas. Borgå and Di Guardo32 used a mechanistic steady-state bioaccumulation model (without spatial resolution) and measured concentrations in water to estimate PCB concentrations in copepods, krill, amphipods and polar cod in the Barents Sea. Estimated concentrations were 20 to 200 times lower than measured, which was attributed to uncertainty in the measured concentrations in water.32 However, observed food chain biomagnification could be reproduced, also in a follow-up study with a model that had been expanded to include the seabird black-legged kittiwake (Rissa tridactyla).36 Likewise, observed bioaccumulation factors (BAF) of PCBs in capelin and cod in the Barents Sea could be reproduced with the dynamic OMEGA model based on concentrations in water as input.34 This study was followed up by two broader studies using the OMEGA model for a range of POPs and marine species in the Svalbard area that estimated 61% and 87% of concentrations within a factor of 5 of measured concentrations, respectively.35,37 Also here, concentrations in water that had been measured, or derived from measured concentrations in air or biota, were used as input. The main strength of NEM compared to these models, is that NEM enables simulation of the complete link from global emissions to biotic exposure, including temporal and spatial variation in both emissions and environmental factors. To our knowledge, this is the first study to estimate PCB-153 concentrations in marine biota in Norwegian marine areas based on global emissions. Our results demonstrate that NEM performs better in reproducing average measured concentrations, than for individual organisms. This has been observed previously for individual estimates of PCBs in humans made with the ACC-Human model.45,54,85 Individual estimates of PCB-153 in Norwegian women, mothers in the Canadian Arctic, and in American citizens correlated with measured levels with rs of 0.13–0.67, 0.17–0.43, and 0.44, respectively. This is in the same range as our results for herring (rs = 0.65) and cod (rs = 0.22). Unreliable dietary intake data at the individual level was identified as a main contributor to poor model performance for estimates of PCB-153 in individual humans.54,85 Similarly, we believe that failing to represent the diversity in the diet of cod is one of the main reasons for the poorer model performance for cod than for herring in this study. Also, one of the main reasons for the good model performance for individual estimates of PCB-153 in herring is likely attributed to the homogeneity of the NSS herring including its diet.
Exploration of emission scenarios
The estimated contribution from Norwegian primary emissions to the total body burden of PCB-153 concentrations in 5 year-old herring was small for the whole time-period and all grid cells (Fig. S21–S23†), ranging from 0.2% (grid cell 1, Fram Strait/Arctic Ocean) to 0.9% (grid cell 48, along the coast of south-western Norway) in 2020 (see Fig. S2† for location of all individual grid cells). For the whole period 1930–2020, the highest contribution from Norwegian primary emissions was estimated to be in the 1960s (Fig. S23†). Even then, the estimated maximum contribution from Norwegian primary emissions to PCB-153 burden in Norwegian herring was not larger than 3.4% (1963, grid cell 48). This compares to a Norwegian fraction of the total global cumulative PCB-153 emissions (1930–2020) of 0.11% (Fig. S24†), with a yearly maximum of 0.18% in 1965 (Fig. S25†). Hence, even if the contribution from Norwegian primary emissions to the body burden of PCB-153 in Norwegian herring is estimated to be small, it is still larger than the Norwegian fraction of global PCB-153 emissions. Nevertheless, this shows that PCB-153 concentrations in fish in Norwegian off-shore marine areas are mainly a result of historical and/or ongoing primary emissions outside of Norway, and not from historical and/or ongoing primary emissions within Norway. This is an important result for Norwegian environmental management, as this means that the regulatory focus needs to be placed on international measures (e.g. the Stockholm Convention) more than national efforts to control and reduce PCB-153 emissions. This conclusion is not valid for locations known to be impacted by local sources including historically contaminated sediment, such as those excluded from this model evaluation (Tables S1 and S5†).
The estimated contribution of European and Russian primary emissions to the total body burden of PCB-153 concentrations in 5 year-old herring in January 2020 is shown in Fig. 7 and S22,† respectively. The relative contribution of EU emissions to the total PCB-153 load in herring varied with both space (Fig. 7 and S21†) and time (Fig. S23†). The contribution of EU primary emissions decreased further north and further east within the model domain (Fig. 7), ranging in 2020 from 31% (cell 55; White Sea) to 74% (cell 60; Skagerrak/Kattegat area) (Fig. S21†). This compares to a European fraction of the total global cumulative PCB-153 emissions (1930–2020) of 26% (Fig. S24†), with a yearly maximum of 38% in 1977 (Fig. S25†). The temporal trend of the relative contribution of European primary emissions for 1930–2020 was similar for all cells (shown for four selected cells in Fig. S23†). This trend is influenced by the time-trend of European production of PCBs, which increased from the 1950s (Fig. S25†).61 Decreasing contribution from European emissions from the 1980s (Fig. S23†) indicates that emissions ceased faster in the included EU countries than in other countries (Fig. S25†). This is reflected in the temporal trend of the relative contribution of Russian primary emissions, which increases somewhat from the 1980s onward (Fig. S23 and S25†). Russia was the last country to stop production of PCBs.61 The estimated contribution of Russian primary emissions to PCB-153 body burden in herring is for obvious reasons highest along the Russian coast (White Sea area) (max in 2020: 31%, grid cell 55) and into the Barents Sea, while it is small in the southwestern part of the model domain (min in 2020: 3% in grid cell 60). This compares to a Russian fraction of the total global cumulative PCB-153 emissions (1930–2020) of 4.8% (Fig. S24†), with a yearly maximum of 13% in 1953 (Fig. S25†). This means that Russian emissions may be important for e.g., PCB-153 exposure in Northeast Atlantic cod residing in the Barents Sea for large parts of the year, while it is small for fish in the North Sea and Norwegian Sea areas. The contributions of both European and Russian primary emission are smallest in the northwestern part of the model domain, where the total body burden of PCB-153 in 5 year-old herring is mainly explained by emissions from other countries not included in the explored emission scenarios (Fig. S22†). This makes sense considering that this area is furthest away from both the EU countries, Russia, and Norway, and may be more impacted by primary emissions originating from elsewhere. In grid cell 16, which covers the western part of Svalbard, including King's Bay, PCB-153 concentrations in herring in 2020 are estimated to be the result of primary emissions from “other countries” (49%, i.e. from all countries except Norway, Russia, and EU member states as of 1973), EU (39%), Russia (12%), and Norway (0.4%) (Fig. S21 and S23†). This clearly shows the importance of global chemical regulations to protect Arctic ecosystems from persistent organic pollutants like PCB-153.
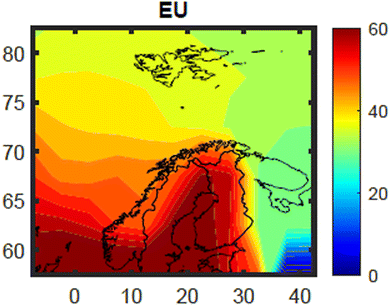 |
| Fig. 7 Fraction (in percent (%)) of total estimated PCB-153 concentrations in herring muscle in January 2020 estimated to originate from historical or ongoing primary emissions in the EU (member countries as of 1973). The data has been interpolated with the MATLAB 2023a provided script contourf, to give a better impression of the spatial distribution (see Fig. 3 and S12†). | |
Conclusions
NEM allows for an integrated, quantitative analysis of the whole continuum of processes linking global emissions of contaminants with exposure of organisms in northern ecosystems. We have shown that NEM is able to reproduce medians and ranges of measured PCB-153 concentrations across compartments, including observed variation across space and trophic levels. Further research is needed to investigate why NEM estimates a steeper decline in PCB-153 concentrations in Norwegian fish with time than those observed in the measurement data. We have used the model to explore how the current PCB-153 burden in Atlantic herring from Norwegian marine areas can largely be rationalized by historical primary emissions from outside Norway. This is just an example of how NEM can be applied to explore the relative role of emissions originating from different countries/regions, and to tease out the most important sources for a given contaminant in a specific species or region over time. This is of key importance for policymakers, as information about emission sources is a prerequisite for scientifically sound regulatory decision making.
A key advantage of NEM is its mechanistic and integrated nature that allows for scenario testing of not only various emission scenarios, but also evaluation of the estimated effects of changes in other input parameters on estimated fate, bioaccumulation, and concentrations. The model can be used to explore how changes in both the physical environment and the food web are estimated to impact contaminant concentrations, and to tease out to which parameters the model estimates are most sensitive. This is particularly relevant in the context of climate change. Climate change has already been identified as a driver of future changes in the distribution and viability of Norwegian and Arctic marine ecosystems.23,86,87 Climate change-induced alterations in the physical environment and food webs are also expected to impact contaminant dynamics in Arctic ecosystems, but the extent and direction of it is still largely unknown.83,84,88,89 As NEM is based on both temporally and spatially resolved emission inventories and environmental and food–chain properties (e.g., temperatures, air and water circulation, organic carbon and lipid contents, dietary relationships etc.), it is well-equipped to evaluate concurrent changes in primary emissions and climate-induced changes in environmental and ecosystem characteristics on contaminant dynamics and exposure. Even if our understanding of future climate-induced changes in the physical and biological environment may still be limited,88 the model can be used for scenario-based hypothesis testing to shed light on processes and parameters that could be important. The model's capability to describe complex climate change scenarios will be restricted to key aspects, such as temperatures or ice cover varying between years/decades, and/or shifting diets and lipid dynamics in the food web.
The NEM bioaccumulation module currently does not allow for animal movement between grid cells, and biota properties are only seasonally and not spatially resolved. Further development is needed to include spatial migrations, differentiated for different species or for different populations within species. It should also be evaluated whether spatial and long-term temporal variation in biota properties should be incorporated in the model. As the physical module of NEM is global in scope, it is possible to apply NEM to other regions of interest. While the NEM bioaccumulation module is currently parameterized for species found in Norwegian marine waters, it is possible to further develop, parameterize, and evaluate it for other species and ecosystems of interest. Moreover, NEM has so far only been evaluated for PCB-153. Further research is needed to evaluate NEM for a range of chemicals with diverse physico-chemical properties, emission scenarios and regulatory status. Based on the good performance of NEM for PCB-153, we are optimistic that the model is also applicable to other POPs and POP-like chemicals. Hence, NEM could represent a future tool for scientists and decision-makers to evaluate the link between emissions and exposure also for chemicals considered for regulation.
Author contributions
Ingjerd S. Krogseth: conceptualization, data curation, formal analysis, funding acquisition, investigation, methodology, project administration, software, visualization, writing – original draft, writing – review & editing. Knut Breivik: conceptualization, data curation, funding acquisition, investigation, methodology, software, writing – review & editing. Sylvia Frantzen: data curation, writing – review & editing. Bente M. Nilsen: data curation, writing – review & editing. Sabine Eckhardt: data curation, software, visualization, writing – review & editing. Therese H. Nøst: conceptualization, funding acquisition, visualization, writing – review & editing. Frank Wania: conceptualization, methodology, supervision, writing – review & editing.
Conflicts of interest
There are no conflicts of interest to declare.
Acknowledgements
This study was funded by the Research Council of Norway (RCN) (#244298, #267574, #287114, #311503), the FRAM Centre Flagship for Hazardous Substances – effects on ecosystems and human health (#132018, #142018, #522018), the FRAM Centre Research Programme SUDARCO, and the Norwegian Ministry of Climate and Environment through the Strategic Institute Programs granted by the RCN. We also thank Drakamöllans Vänner för Kultur och Vetenskap and Sia Gerrard.
References
-
United Nations Environment Programme (UNEP), The Stockholm Convention on Persistent Organic Pollutants (POPs) as amended in 2009. Text and annexes, Stockholm, Sweden, 2009 Search PubMed.
- F. Wania, Assessing the potential of persistent organic chemicals for long-range transport and accumulation in polar regions, Environ. Sci. Technol., 2003, 37, 1344–1351, DOI:10.1021/es026019e.
- G. Czub, F. Wania and M. S. McLachlan, Combining long-range transport and bioaccumulation considerations to identify potential Arctic contaminants, Environ. Sci. Technol., 2008, 42, 3704–3709, DOI:10.1021/es7028679.
- H. Routti, T. C. Atwood, T. Bechshoft, A. Boltunov, T. M. Ciesielski, J.-P. Desforges, R. Dietz, G. W. Gabrielsen, B. M. Jenssen, R. J. Letcher, M. A. McKinney, A. D. Morris, F. F. Rigét, C. Sonne, B. Styrishave and S. Tartu, State of knowledge on current exposure, fate and potential health effects of contaminants in polar bears from the circumpolar Arctic, Sci. Total Environ., 2019, 664, 1063–1083, DOI:10.1016/j.scitotenv.2019.02.030.
- F. Rigét, A. Bignert, B. Braune, J. Stow and S. Wilson, Temporal trends of legacy POPs in Arctic biota, an update, Sci. Total Environ., 2010, 408, 2874–2884, DOI:10.1016/j.scitotenv.2009.07.036.
- J. Verreault, G. Gabrielsen and J. Bustnes, The Svalbard glaucous gull as bioindicator species in the European Arctic: insight from 35 years of contaminants research, Rev. Environ. Contam. Toxicol., 2010, 205, 77–116, DOI:10.1007/978-1-4419-5623-1_2.
- R. Dietz, R. J. Letcher, J.-P. Desforges, I. Eulaers, C. Sonne, S. Wilson, E. Andersen-Ranberg, N. Basu, B. D. Barst, J. O. Bustnes, J. Bytingsvik, T. M. Ciesielski, P. E. Drevnick, G. W. Gabrielsen, A. Haarr, K. Hylland, B. M. Jenssen, M. Levin, M. A. McKinney, R. D. Nørregaard, K. E. Pedersen, J. Provencher, B. Styrishave, S. Tartu, J. Aars, J. T. Ackerman, A. Rosing-Asvid, R. Barrett, A. Bignert, E. W. Born, M. Branigan, B. Braune, C. E. Bryan, M. Dam, C. A. Eagles-Smith, M. Evans, T. J. Evans, A. T. Fisk, M. Gamberg, K. Gustavson, C. A. Hartman, B. Helander, M. P. Herzog, P. F. Hoekstra, M. Houde, K. Hoydal, A. K. Jackson, J. Kucklick, E. Lie, L. Loseto, M. L. Mallory, C. Miljeteig, A. Mosbech, D. C. G. Muir, S. T. Nielsen, E. Peacock, S. Pedro, S. H. Peterson, A. Polder, F. F. Rigét, P. Roach, H. Saunes, M.-H. S. Sinding, J. U. Skaare, J. Søndergaard, G. Stenson, G. Stern, G. Treu, S. S. Schuur and G. Víkingsson, Current state of knowledge on biological effects from contaminants on Arctic wildlife and fish, Sci. Total Environ., 2019, 696, 133792, DOI:10.1016/j.scitotenv.2019.133792.
- P. D. Jepson and R. J. Law, Persistent pollutants, persistent threats, Science, 2016, 352, 1388–1389, DOI:10.1126/science.aaf9075.
- Y. Okumura, Y. Yamashita and S. Isagawa, Sources of polychlorinated dibenzo-p-dioxins (PCDDs), polychlorinated dibenzofurans (PCDFs), and coplanar polychlorinated biphenyls (Co-PCBs), and their bioaccumulation through the marine food web in Sendai Bay, Japan, J. Environ. Monit., 2003, 5, 610–618, 10.1039/B301339C.
- D. Megson, T. Brown, G. R. Jones, M. Robson, G. W. Johnson, G. P. Tiktak, C. D. Sandau and E. J. Reiner, Polychlorinated biphenyl (PCB) concentrations and profiles in marine mammals from the North Atlantic Ocean, Chemosphere, 2022, 288, 132639, DOI:10.1016/j.chemosphere.2021.132639.
- C. Xia, J. C. W. Lam, X. Wu, Z. Xie and P. K. S. Lam, Polychlorinated biphenyls (PCBs) in marine fishes from China: Levels, distribution and risk assessment, Chemosphere, 2012, 89, 944–949, DOI:10.1016/j.chemosphere.2012.06.048.
- A. R. Jafarabadi, A. R. Bakhtiari, S. Mitra, M. Maisano, T. Cappello and C. Jadot, First polychlorinated biphenyls (PCBs) monitoring in seawater, surface sediments and marine fish communities of the Persian Gulf: Distribution, levels, congener profile and health risk assessment, Environ. Pollut., 2019, 253, 78–88, DOI:10.1016/j.envpol.2019.07.023.
- A. C. de Souza, S. Taniguchi, R. C. L. Figueira, R. C. Montone, M. C. Bícego and C. C. Martins, Historical records and spatial distribution of high hazard PCBs levels in sediments around a large South American industrial coastal area (Santos Estuary, Brazil), J. Hazard. Mater., 2018, 360, 428–435, DOI:10.1016/j.jhazmat.2018.08.041.
- S. Tanabe, H. Hidaka and R. Tatsukawa, PCBs and chlorinated hydrocarbon pesticides in Antarctic atmosphere and hydrosphere, Chemosphere, 1983, 12, 277–288, DOI:10.1016/0045-6535(83)90171-6.
- S. Corsolini, T. Romeo, N. Ademollo, S. Greco and S. Focardi, POPs in key species of marine Antarctic ecosystem, Microchem. J., 2002, 73, 187–193, DOI:10.1016/S0026-265X(02)00063-2.
- D. Muir, F. Riget, M. Cleemann, J. Skaare, L. Kleivane, H. Nakata, R. Dietz, T. Severinsen and S. Tanabe, Circumpolar trends of PCBs and organochlorine pesticides in the Arctic marine environment inferred from levels in ringed seals, Environ. Sci. Technol., 2000, 34, 2431–2438, DOI:10.1021/es991245i.
- E. Kampire, G. Rubidge and J. B. Adams, Distribution of polychlorinated biphenyl residues in sediments and blue mussels (Mytilus galloprovincialis) from Port Elizabeth Harbour, South Africa, Mar. Pollut. Bull., 2015, 91, 173–179, DOI:10.1016/j.marpolbul.2014.12.008.
- M.-A. Blanchet, R. Primicerio, A. Frainer, S. Kortsch, M. Skern-Mauritzen, A. V. Dolgov and M. Aschan, The role of marine mammals in the Barents Sea foodweb, ICES J. Mar. Sci., 2019, 76, i37–i53, DOI:10.1093/icesjms/fsz136.
- P. Blévin, J. Aars, M. Andersen, M.-A. Blanchet, L. Hanssen, D. Herzke, R. M. Jeffreys, E. S. Nordøy, M. Pinzone and C. de la Vega, Pelagic vs. coastal—Key drivers of pollutant levels in Barents Sea polar bears with contrasted space-use strategies, Environ. Sci. Technol., 2019, 54, 985–995, DOI:10.1021/acs.est.9b04626.
- K. Borgå, H. Wolkers, J. U. Skaare, H. Hop, D. C. Muir and G. W. Gabrielsen, Bioaccumulation of PCBs in Arctic seabirds: influence of dietary exposure and congener biotransformation, Environ. Pollut., 2005, 134, 397–409, DOI:10.1016/j.envpol.2004.09.016.
- P. O. Darnerud, S. Atuma, M. Aune, R. Bjerselius, A. Glynn, K. P. Grawé and W. Becker, Dietary intake estimations of organohalogen contaminants (dioxins, PCB, PBDE and chlorinated pesticides, e.g. DDT) based on Swedish market basket data, Food Chem. Toxicol., 2006, 44, 1597–1606, DOI:10.1016/j.fct.2006.03.011.
- C. Rylander, E. Lund, L. Frøyland and T. M. Sandanger, Predictors of PCP, OH-PCBs, PCBs and chlorinated pesticides in a general female Norwegian population, Environ. Int., 2012, 43, 13–20, DOI:10.1016/j.envint.2012.02.008.
- M.-A. Blanchet, R. Primicerio, A. Smalås, J. Arias-Hansen and M. Aschan, How vulnerable is the European seafood production to climate warming?, Fish. Res., 2019, 209, 251–258, DOI:10.1016/j.fishres.2018.09.004.
- S. M. Platt, Ø. Hov, T. Berg, K. Breivik, S. Eckhardt, K. Eleftheriadis, N. Evangeliou, M. Fiebig, R. Fisher and G. Hansen, Atmospheric composition in the European Arctic and 30 years of the Zeppelin Observatory, Ny-Ålesund, Atmos. Chem. Phys. Discuss., 2021, 1–80, DOI:10.5194/acp-22-3321-2022.
- G. Everaert, A. Ruus, D. Ø. Hjermann, K. Borgå, N. Green, S. Boitsov, H. Jensen and A. Poste, Additive models reveal sources of metals and organic pollutants in Norwegian marine sediments, Environ. Sci. Technol., 2017, 51, 12764–12773, DOI:10.1021/acs.est.7b02964.
- S. Frantzen, A. Måge, S. A. Iversen and K. Julshamn, Seasonal variation in the levels of organohalogen compounds in herring (Clupea harengus) from the Norwegian Sea, Chemosphere, 2011, 85, 179–187, DOI:10.1016/j.chemosphere.2011.06.034.
- K. Julshamn, A. K. Lundebye, K. Heggstad, M. H. G. Berntssen and B. Boe, Norwegian monitoring programme on the inorganic and organic contaminants in fish caught in the Barents Sea, Norwegian Sea and North Sea, 1994–2001, Food Addit. Contam., 2004, 21, 365–376, DOI:10.1080/02652030310001639512.
- Q. T. Ho, M. S. Bank, A. M. Azad, B. M. Nilsen, S. Frantzen, S. Boitsov, A. Maage, T. Kögel, M. Sanden and L. Frøyland, Co-occurrence of contaminants in marine fish from the North East Atlantic Ocean: Implications for human risk assessment, Environ. Int., 2021, 157, 106858, DOI:10.1016/j.chemosphere.2011.06.034.
- R. Gioia, R. Lohmann, J. Dachs, C. Temme, S. Lakaschus, D. Schulz-Bull, I. Hand and K. C. Jones, Polychlorinated biphenyls in air and water of the North Atlantic and Arctic Ocean, J. Geophys. Res.: Atmos., 2008, 113, D19302, DOI:10.1029/2007JD009750.
- A. Sobek and Ö. Gustafsson, Deep water masses and sediments are main compartments for polychlorinated biphenyls in the Arctic Ocean, Environ. Sci. Technol., 2014, 48, 6719–6725, DOI:10.1021/es500736q.
- I. G. Hallanger, N. A. Warner, A. Ruus, A. Evenset, G. Christensen, D. Herzke, G. W. Gabrielsen and K. Borgå, Seasonality in contaminant accumulation in Arctic marine pelagic food webs using trophic magnification factor as a measure of bioaccumulation, Environ. Toxicol. Chem., 2011, 30, 1026–1035, DOI:10.1002/etc.488.
- K. Borgå and A. Di Guardo, Comparing measured and predicted PCB concentrations in Arctic seawater and marine biota, Sci. Total Environ., 2005, 342, 281–300, DOI:10.1016/j.scitotenv.2004.12.043.
-
D. Mackay, Multimedia Environmental Models. The Fugacity Approach, Lewis publishers, Boca Raton, FL, US, 2nd edn, 2001 Search PubMed.
- F. D. Laender, D. V. Oevelen, S. Frantzen, J. J. Middelburg and K. Soetaert, Seasonal PCB bioaccumulation in an Arctic marine ecosystem: A model analysis incorporating lipid dynamics, food-web productivity and migration, Environ. Sci. Technol., 2010, 44, 356–361, DOI:10.1021/es902625u.
- R. P. Hoondert, N. W. van den Brink, M. J. Van den Heuvel-Greve, A. J. Ragas and A. Jan Hendriks, Implications of trophic variability for modeling biomagnification of POPs in marine food webs in the Svalbard archipelago, Environ. Sci. Technol., 2020, 54, 4026–4035, DOI:10.1021/acs.est.9b06666.
- K. Borgå, T. M. Saloranta and A. Ruus, Simulating climate change induced alterations in bioaccumulation of organic contaminants in an Arctic marine food web, Environ. Toxicol. Chem., 2010, 29, 1349–1357, DOI:10.1002/etc.159.
- F. D. Laender, J. Hammer, A. J. Hendriks, K. Soetaert and C. Janssen, Combining monitoring data and modeling identifies PAHs as emerging contaminants in the Arctic, Environ. Sci. Technol., 2011, 45, 9024–9029, DOI:10.1021/es202423f.
- N. J. Diepens and A. A. Koelmans, Accumulation of plastic debris and associated contaminants in aquatic food webs, Environ. Sci. Technol., 2018, 52, 8510–8520, DOI:10.1021/acs.est.8b02515.
- M. Hauck, M. A. J. Huijbregts, A. Hollander, A. J. Hendriks and D. van de Meent, Modeled and monitored variation in space and time of PCB-153 concentrations in air, sediment, soil and aquatic biota on a European scale, Sci. Total Environ., 2010, 408, 3831–3839, DOI:10.1016/j.scitotenv.2009.11.037.
- A. Di Guardo, T. Gouin, M. MacLeod and M. Scheringer, Environmental fate and exposure models: advances and challenges in 21st century chemical risk assessment, Environ. Sci.: Processes Impacts, 2018, 20, 58–71, 10.1039/C7EM00568G.
- A. Di Guardo and J. L. Hermens, Challenges for exposure prediction in ecological risk assessment, Integr. Environ. Assess. Manage., 2013, 9, e4–e14, DOI:10.1002/ieam.1442.
- K. Breivik, G. Czub, M. S. McLachlan and F. Wania, Towards an understanding of the link between environmental emissions and human body burdens of PCBs using CoZMoMAN, Environ. Int., 2010, 36, 85–91, DOI:10.1016/j.envint.2009.10.006.
- I. S. Krogseth, K. Breivik, J. A. Arnot, F. Wania, A. R. Borgen and M. Schlabach, Evaluating the environmental fate of short-chain chlorinated paraffins (SCCPs) in the Nordic environment using a dynamic multimedia model, Environ. Sci.: Processes Impacts, 2013, 15, 2240–2251, 10.1039/C3EM00407D.
- T. H. Nøst, K. Breivik, O.-M. Fuskevåg, E. Nieboer, J. Ø. Odland and T. M. Sandanger, Persistent organic pollutants in Norwegian men from 1979 to 2007: intraindividual changes, age–period–cohort effects, and model predictions, Environ. Health Perspect., 2013, 121, 1292–1298, DOI:10.1289/ehp.1206317.
- T. H. Nøst, K. Breivik, F. Wania, C. Rylander, J. Ø. Odland and T. M. Sandanger, Estimating time-varying PCB exposures using person-specific predictions to supplement measured values: A comparison of observed and predicted values in two cohorts of Norwegian women, Environ. Health Perspect., 2015, 124, 299–305, DOI:10.1289/ehp.1409191.
- G. Czub and M. S. McLachlan, A food chain model to predict the levels of lipophilic organic contaminants in humans, Environ. Toxicol. Chem., 2004, 23, 2356–2366, DOI:10.1897/03-317.
- F. Wania and D. Mackay, A global distribution model for persistent organic chemicals, Sci. Total Environ., 1995, 160, 211–232, DOI:10.1016/0048-9697(95)04358-8.
- F. Wania, Potential of degradable organic chemicals for absolute and relative enrichment in the Arctic, Environ. Sci. Technol., 2006, 40, 569–577, DOI:10.1021/es051406k.
- M. MacLeod, H. von Waldow, P. Tay, J. M. Armitage, H. Wöhrnschimmel, W. J. Riley, T. E. McKone and K. Hungerbühler, BETR global - A geographically-explicit global-scale multimedia contaminant fate model, Environ. Pollut., 2011, 159, 1442–1445, DOI:10.1016/j.envpol.2011.01.038.
- M. S. McLachlan, E. Undeman, F. Zhao and M. MacLeod, Predicting global scale exposure of humans to PCB 153 from historical emissions, Environ. Sci.: Processes Impacts, 2018, 20, 747–756, 10.1039/C8EM00023A.
- E. Undeman, T. N. Brown, M. S. McLachlan and F. Wania, Who in the world is most exposed to polychlorinated biphenyls? Using models to identify highly exposed populations, Environ. Res. Lett., 2018, 13, 064036, DOI:10.1088/1748-9326/aac5fe.
- M. J. Binnington and F. Wania, Clarifying relationships between persistent organic pollutant concentrations and age in wildlife biomonitoring: Individuals, cross-sections, and the roles of lifespan and sex, Environ. Toxicol. Chem., 2014, 33, 1415–1426, DOI:10.1002/etc.2576.
- M. J. Binnington, M. S. Curren, H. M. Chan and F. Wania, Balancing the benefits and costs of traditional food substitution by indigenous Arctic women of childbearing age: Impacts on persistent organic pollutant, mercury, and nutrient intakes, Environ. Int., 2016, 94, 554–566, DOI:10.1016/j.envint.2016.06.016.
- M. J. Binnington, M. S. Curren, C. L. Quinn, J. M. Armitage, J. A. Arnot, H. M. Chan and F. Wania, Mechanistic polychlorinated biphenyl exposure modeling of mothers in the Canadian Arctic: the challenge of reliably establishing dietary composition, Environ. Int., 2016, 92–93, 256–268, DOI:10.1016/j.envint.2016.04.011.
- F. Wania, M. J. Binnington and M. S. Curren, Mechanistic modeling of persistent organic pollutant exposure among indigenous Arctic populations: motivations, challenges, and benefits, Environ. Rev., 2017, 1–12, DOI:10.1139/er-2017-0010.
- K. Breivik, S. Eckhardt, M. S. McLachlan and F. Wania, Introducing a nested multimedia fate and transport model for organic contaminants (NEM), Environ. Sci.: Processes Impacts, 2021, 23, 1146–1157, 10.1039/D1EM00084E.
- H. Hop and H. Gjøsæter, Polar cod (Boreogadus saida) and capelin (Mallotus villosus) as key species in marine food webs of the Arctic and the Barents Sea, Mar. Biol. Res., 2013, 9, 878–894, DOI:10.1080/17451000.2013.775458.
- I. G. Hallanger, A. Ruus, D. Herzke, N. A. Warner, A. Evenset, E. S. Heimstad, G. W. Gabrielsen and K. Borgå, Influence of season, location, and feeding strategy on bioaccumulation of halogenated organic contaminants in Arctic marine zooplankton, Environ. Toxicol. Chem., 2011, 30, 77–87, DOI:10.1002/etc.362.
- I. G. Hallanger, A. Ruus, N. A. Warner, D. Herzke, A. Evenset, M. Schøyen, G. W. Gabrielsen and K. Borgå, Differences between Arctic and Atlantic fjord systems on bioaccumulation of persistent organic pollutants in zooplankton from Svalbard, Sci. Total Environ., 2011, 409, 2783–2795, DOI:10.1016/j.scitotenv.2011.03.015.
- G. Czub and M. S. McLachlan, Influence of the temperature gradient in blubber on the bioaccumulation of persistent lipophilic organic chemicals in seals, Environ. Toxicol. Chem., 2007, 26, 1600–1605, DOI:10.1897/06-548R.1.
- K. Breivik, A. Sweetman, J. M. Pacyna and K. C. Jones, Towards a global historical emission inventory for selected PCB congeners - a mass balance approach 1. Global production and consumption, Sci. Total Environ., 2002, 290, 181–198, DOI:10.1016/s0048-9697(01)01075-0.
- K. Breivik, A. Sweetman, J. M. Pacyna and K. C. Jones, Towards a global historical emission inventory for selected PCB congeners - a mass balance approach 2. Emissions, Sci. Total Environ., 2002, 290, 199–224, DOI:10.1016/s0048-9697(01)01076-2.
- K. Breivik, J. M. Armitage, F. Wania, A. J. Sweetman and K. C. Jones, Tracking the global distribution of persistent organic pollutants accounting for e-waste exports to developing regions, Environ. Sci. Technol., 2016, 50, 798–805, DOI:10.1021/acs.est.5b04226.
- N. Li, F. Wania, Y. D. Lei and G. L. Daly, A Comprehensive and critical compilation, evaluation, and selection of physical–chemical property data for selected polychlorinated biphenyls, J. Phys. Chem. Ref. Data, 2003, 32, 1545–1590, DOI:10.1063/1.1562632.
- A. Sobek and Ö. Gustafsson, Latitudinal fractionation of polychlorinated biphenyls in surface seawater along a 62° N−89° N transect from the southern Norwegian Sea to the North pole area, Environ. Sci. Technol., 2004, 38, 2746–2751, DOI:10.1021/es0353816.
- D. E. Schulz-Bull, G. Petrick, N. Kannan and J. C. Duinker, Distribution of individual chlorobiphenyls (PCB) in solution and suspension in the Baltic Sea, Mar. Chem., 1995, 48, 245–270, DOI:10.1016/0304-4203(94)00054-H.
- D. Wodarg, P. Kömp and M. S. McLachlan, A baseline study of polychlorinated biphenyl and hexachlorobenzene concentrations in the western Baltic Sea and Baltic Proper, Mar. Chem., 2004, 87, 23–36, DOI:10.1016/j.marchem.2003.12.002.
-
NILU, EBAS, https://ebas.nilu.no/, accessed Dec 6th 2021 Search PubMed.
-
P. Blévin, J. Aars, C. Andvik, M. Biuw, K. Borgå, J. Bytingsvik, A. Fisk, T. Haug, D. Herzke, J. L. Lyche, C. Lydersen, K. Kovacs, A. Rikardsen, E. Vogel and H. Routti, Getting the full picture: persistent organic pollutants in marine mammals from the Norwegian Arctic, In preparation.
- K. Julshamn, A. Duinker, M. Berntssen, B. M. Nilsen, S. Frantzen, K. Nedreaas and A. Maage, A baseline study on levels of polychlorinated dibenzo-p-dioxins, polychlorinated dibenzofurans, non-ortho and mono-ortho PCBs, non-dioxin-like PCBs and polybrominated diphenyl ethers in Northeast Arctic cod (Gadus morhua) from different parts of the Barents Sea, Mar. Pollut. Bull., 2013, 75, 250–258, DOI:10.1016/j.marpolbul.2013.07.017.
- L. Li, C. Chen, D. Li, K. Breivik, G. Abbasi and Y.-F. Li, What do we know about the production and release of persistent organic pollutants in the global environment?, Environ. Sci.: Adv., 2023, 2, 55–68, 10.1039/D2VA00145D.
- J. J. Alava, P. S. Ross and F. A. Gobas, Food web bioaccumulation model for resident killer whales from the Northeastern Pacific Ocean as a tool for the derivation of PBDE-sediment quality guidelines, Arch. Environ. Contam. Toxicol., 2016, 70, 155–168, DOI:10.1007/s00244-015-0215-y.
- K. M. MacKenzie, C. Lydersen, T. Haug, H. Routti, J. Aars, C. M. Andvik, K. Borgå, A. T. Fisk, S. Meier, M. Biuw, A. D. Lowther, U. Lindstrøm and K. M. Kovacs, Niches of marine mammals in the European Arctic, Ecol. Indic., 2022, 136, 108661, DOI:10.1016/j.ecolind.2022.108661.
- H. Hop, K. Borgå, G. W. Gabrielsen, L. Kleivane and J. U. Skaare, Food web magnification of persistent organic pollutants in poikilotherms and homeotherms from the Barents Sea, Environ. Sci. Technol., 2002, 36, 2589–2597, DOI:10.1021/es010231l.
- H. Andrade, P. van der Sleen, B. A. Black, J. A. Godiksen, W. L. Locke, M. L. Carroll, W. G. Ambrose and A. Geffen, Ontogenetic movements of cod in Arctic fjords and the Barents Sea as revealed by otolith microchemistry, Polar Biol., 2020, 43, 409–421, DOI:10.1007/s00300-020-02642-1.
- C. Stransky, H. Baumann, S.-E. Fevolden, A. Harbitz, H. Høie, K. H. Nedreaas, A.-B. Salberg and T. H. Skarstein, Separation of Norwegian coastal cod and Northeast Arctic cod by outer otolith shape analysis, Fish. Res., 2008, 90, 26–35, DOI:10.1016/j.fishres.2007.09.009.
-
J. C. Holst, I. Røttingen and W. Melle, in The Norwegian Sea Ecosystem, ed. H. R. Skjoldal, Tapir Academic Press, Trondheim, Norway, 2004, pp. 203–226 Search PubMed.
- S. E. Enoksen and H. Reiss, Diet of Norwegian coastal cod (Gadus morhua) studied by using citizen science, J. Mar. Syst., 2018, 180, 246–254, DOI:10.1016/j.jmarsys.2017.06.006.
- R. E. Holt, B. Bogstad, J. M. Durant, A. V. Dolgov and G. Ottersen, Barents Sea cod (Gadus morhua) diet composition: long-term interannual, seasonal, and ontogenetic patterns, ICES J. Mar. Sci., 2019, 76, 1641–1652, DOI:10.1093/icesjms/fsz082.
-
IMR, Topic: Atlantic cod - North Sea, Skagerrak and the Eastern English Channel, https://www.hi.no/en/hi/temasider/species/atlantic-cod--north-sea,-skagerrak-and-the-eastern-english-channel, accessed Aug 11th 2022 Search PubMed.
- O. J. Nøstbakken, A. Duinker, J. D. Rasinger, B. M. Nilsen, M. Sanden, S. Frantzen, H. T. Hove, A.-K. Lundebye, M. H. G. Berntssen, R. Hannisdal, L. Madsen and A. Maage, Factors influencing risk assessments of brominated flame-retardants; evidence based on seafood from the North East Atlantic Ocean, Environ. Int., 2018, 119, 544–557, DOI:10.1016/j.envint.2018.04.044.
-
S. Frantzen, S. Boitsov, N. Dehnhard, A. Duinker, B. E. Grøsvik, E. S. Heimstad, D. Hjermann, H. Jensen, L. K. Jensen, Ø. Leiknes, B. M. Nilsen, H. Routti, M. Schøyen and H. K. Skjerdal, Pollution in the Norwegian sea areas - Barents Sea, Norwegian Sea and North Sea, Report from the Monitoring Group 2021, Report 2022-3, Bergen, 2022 Search PubMed.
-
AMAP, AMAP Assessment 2020: POPs and Chemicals of Emerging Arctic Concern: Influence of Climate Change, Tromsø, 2020 Search PubMed.
- C. A. de Wit, K. Vorkamp and D. Muir, Influence of climate change on persistent organic pollutants and chemicals of emerging concern in the Arctic: state of knowledge and recommendations for future research, Environ. Sci.: Processes Impacts, 2022, 24, 1530–1543, 10.1039/D1EM00531F.
- S. A. Wood, J. M. Armitage, M. J. Binnington and F. Wania, Deterministic modeling of the exposure of individual participants in the National Health and Nutrition Examination Survey (NHANES) to polychlorinated biphenyls, Environ. Sci.: Processes Impacts, 2016, 18, 1157–1168, 10.1039/C6EM00424E.
- M.-A. Blanchet, J. Aars, M. Andersen and H. Routti, Space-use strategy affects energy requirements in Barents Sea polar bears, Mar. Ecol.: Prog. Ser., 2020, 639, 1–19, DOI:10.3354/meps13290.
- F. J. Mueter, B. Planque, G. L. Hunt Jr, I. D. Alabia, T. Hirawake, L. Eisner, P. Dalpadado, M. Chierici, K. F. Drinkwater and N. Harada, Possible future scenarios in the gateways to the Arctic for Subarctic and Arctic marine systems: II. prey resources, food webs, fish, and fisheries, ICES J. Mar. Sci., 2021, 78, 3017–3045, DOI:10.1093/icesjms/fsab122.
- K. Borgå, M. A. McKinney, H. Routti, K. J. Fernie, J. Giebichenstein, I. Hallanger and D. C. G. Muir, The influence of global climate change on accumulation and toxicity of persistent organic pollutants and chemicals of emerging concern in Arctic food webs, Environ. Sci.: Processes Impacts, 2022, 24, 1544–1576, 10.1039/D1EM00469G.
- H. Hung, C. Halsall, H. Ball, T. Bidleman, J. Dachs, A. De Silva, M. Hermanson, R. Kallenborn, D. Muir, R. Sühring, X. Wang and S. Wilson, Climate change influence on the levels and trends of persistent organic pollutants (POPs) and chemicals of emerging Arctic concern (CEACs) in the Arctic physical environment – a review, Environ. Sci.: Processes Impacts, 2022, 24, 1577–1615, 10.1039/D1EM00485A.
|
This journal is © The Royal Society of Chemistry 2023 |
Click here to see how this site uses Cookies. View our privacy policy here.