Chemical characterization of urban aerosols in Abidjan and Korhogo (Côte d'Ivoire) from 2018 to 2020 and the identification of their potential emission sources†
Received
4th September 2023
, Accepted 1st November 2023
First published on 17th November 2023
Abstract
As part of the Air Pollution and Health in Urban Environments (PASMU) project, equipment was installed in urban sites of Abidjan and Korhogo (Ivory Coast) in West Africa with the aim of monitoring the chemical composition of PM2.5 aerosols. These installations were used to collect PM2.5 aerosols at weekly intervals for the determination of their PM2.5 mass, EC, OC and water-soluble ions (WSI). This database enabled us to analyse the 2 year trend (2018–2020) of the chemical composition of PM2.5 aerosols in these two cities. In addition, this database was used to assess the sources of these aerosols using both PCA (principal component analysis) and the US Environmental Protection Agency's EPA PMF 5.0 software. The results showed that the PM2.5 concentrations observed during the 2 dry seasons were more than twice than that during the 2 wet seasons. Also, over the 2 year study period, the observed PM2.5 concentrations were above the WHO, 2021 standards. The analysis of the chemical composition of PM2.5 showed that organic matter (OM) was the major fraction in the 2 cities, followed by EC in Abidjan and dust in Korhogo. Similarly, the observed trends showed greater variations in OC concentrations between the dry and wet seasons compared with EC. Also, 5 contributing sources were identified with disproportionate contributions. In Abidjan, these sources included road traffic (44.7%), domestic fires (40%), natural and road dust (11.2%), sea salt (3%), and construction dust (1.2%). In Korhogo, the sources were biomass burning and domestic fires (70.7%), road traffic (16%), road dust and sea salt (8.1%), natural dust (2.6%), and agriculture (2.5%). This study offers vital insights into identifying the primary sources of urban air pollution in West African cities. Consequently, tailored strategies based on these sources can effectively mitigate urban particulate pollution, leading to reduced emissions, enhanced air quality, and improved public health in densely populated urban regions.
Environmental significance
Our paper, entitled “Chemical characterization of urban aerosols in Abidjan and Korhogo (Côte d'Ivoire) from 2018 to 2020 and identification of their potential emission sources”, is based on PM2.5 aerosol samples collected over two years in Abidjan and Korhogo, Côte d'Ivoire, analysing carbon compounds (EC and OC) and water-soluble ions (WSI) to identify their contributing emission sources. Our results show that the picture of contributing sources is different from one city to another, and hence it is necessary to define mitigation scenarios specific to each city. This research is crucial, given that it provides an overview of two West African cities with different urban characteristics, enabling targeted actions in the mitigation of atmospheric pollutant emissions.
|
1. Introduction
Particulate pollution is a significant public health problem worldwide, with an even more dramatic situation in developing countries, where its impacts are still poorly understood.1 Furthermore, it has been the cause of many deaths worldwide, i.e., 4.2 million in 2016 according to the WHO. Cohen et al.2 showed the impact of anthropogenic fine particles in West Africa on premature deaths from cardiovascular and respiratory causes (e.g., lung cancer). The concentration levels of particulate matter (PM) depend on several processes that transform emissions into concentrations, including meteorological, climatic, physicochemical, and urban morphology.
In this case, to understand the impact of particulate pollution on the health of the population, it is necessary to characterise the chemical composition of aerosols. Indeed, the health impact of aerosols is highly related to their composition of chemical species and less on the levels of aerosol mass concentrations.3
In West Africa, Adon et al.4 showed a strong dependence between the inflammatory impact of aerosols and their soluble organic carbon (WSOC). In addition, links between oxidative activity (measured using DTT) and carbon species concentrations (OC, EC, WSOC and WIOC) have been established in areas subject to source road traffic.5 In Abidjan, Kouassi et al.6 established the toxicity induced by the chemical composition of PM2.5 from rural, urban and industrial sites. Furthermore, the chemical composition of PM2.5 is dependent on its contributing sources.4,7–11 Therefore, to limit the health impacts of aerosols, it is necessary to study their chemical composition and identify their contributing sources, enabling better action to be taken. This work is particularly important in West African cities, which are experiencing a real population explosion, resulting in increasing emissions of air pollutants since the 1990s (Keita et al.12). The present study aimed to investigate the chemical characteristics of PM2.5 aerosols in Abidjan and Korhogo in Côte d’Ivoire using a database of several years. It is based on sampling techniques using aerosol collectors placed at 1 site in two cites, i.e., Abidjan (site A1, UFHB) and Korhogo (site K1, UPGC). The collected samples allowed several analyses to be carried out to obtain numerous physical–chemical parameters for the aerosols collected. The analysis of this database will be presented as follows: interannual and seasonal evolution of PM2.5 concentrations at the 2 sites, including the evolution of carbonaceous species, evolution of the mineral composition of PM2.5 aerosols, analysis of the chemical composition (carbonaceous and mineral species) with the aim to explain the masses of PM2.5 aerosols collected on the 2 sites, and finally analysis of the contribution of the sources to the concentrations of PM2.5 aerosols in Abidjan and Korhogo. This work was carried out within the framework of the Air Pollution and Health in Urban Areas, Côte d'Ivoire (PASMU) project.
2. Methodology
2.1. Study areas
Data collection took place jointly in Abidjan and Korhogo, the two cities in the PASMU project study. Fig. 1 shows a map of Côte d'Ivoire with the location of these two cities, where Abidjan is in the south and Korhogo in the north. The morphological, geographical and anthropogenic differences in these cities allowed the study of air pollution in two distinct urban configurations of Côte d'Ivoire.
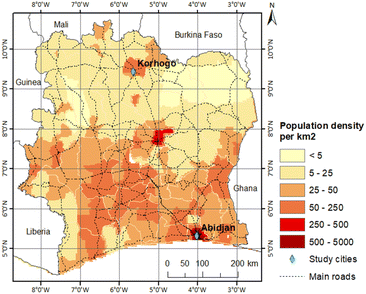 |
| Fig. 1 Population density map of Côte d'Ivoire, with the 2 study towns in green, adapted from Gnamien et al., 2020.20 | |
The city of Abidjan has been the political capital of Côte d'Ivoire for a long time, and to date is still the centre of most of its economic activities. Indeed, with its large coastline, Abidjan shelters the main industrial activities of the country. This city is divided into 10 communes and 3 sub-prefectures (peripheral communes) with a population of 6
321
017 according to the 2021 General Census of Population and Housing or 21.5% of the national population, for an area of 2119 km2. Abidjan is an autonomous district with different levels of urbanization and populations of different social classes. The main sources of anthropogenic pollutant emissions are traffic, domestic fires (use of wood and charcoal), waste combustion and industrial activities.
The city of Korhogo is the third most populous city in the country (440
926 inhabitants, 1.5% of the national population). Much of the city's land area is unbuilt and used for agriculture, with an urbanization rate of 42.9% in 2021.13 In this case, Korhogo is a city marked by agricultural activities with a few factories processing and packaging agricultural products. Consequently, the activities of these factories are strongly linked to local agricultural production. The main sources of emissions in the city of Korhogo are domestic fires and traffic, with intense activity of heavy trucks, intercity buses, and also 2- and 3-wheel motorized vehicles. Moreover, in this city, only the main roads are paved and represent less than 10% of urban roads.14 This implies the important resuspension of aerosols present on the roads, which is also a major source of particulate emissions. The domestic fire source is very important with the use of firewood for economic reasons or convenience because these resources are available in the surrounding villages. In addition to the local anthropogenic sources present in Korhogo, an important impacting source is provided by the harmattan winds. This predominant air mass during the dry season is an important source of dust from the Sahara and aerosols from surrounding or regional savanna fires.
In conclusion, these two cities differ in terms of economic and social activities and infrastructure including the usual means of transportation and types of roads, the fuels used for cooking, the practices of the population, and finally the local weather, as described in paragraph 2.6.
On the map of the population density distribution in Côte d'Ivoire (Fig. 1), it can be seen that the population densities of these two cities are different, where the population density of Abidjan is 10-times higher than that of Korhogo. However, although the population densities in south-central Côte d'Ivoire are generally higher than in the more northern regions, the population density of Korhogo is still high for a northern region. This is due to the existence of industries in this city.
2.2. Measurement sites
As mentioned above, medium-term measurements were carried out at 2 sites, i.e., Abidjan (UFHB) and in Korhogo (UPGC). Measurements were performed at the medium-term sites weekly and/or daily in the 2018 and 2019 dry seasons and in the 2019 wet season.
2.2.1. Abidjan (site A1, University Felix Houphouet-Boigny UFHB).
Site A1 is the main measurement site (reference site) of the PASMU project. It is located within the University Felix Houphouet-Boigny (UFHB) in Abidjan at 5°20′47,58′′N latitude and 3°59′23,96′′W longitude. According to LCSQA,15 Site A1 is an urban site. Indeed, it allows the monitoring of the average exposure of the population to air pollution, which is known as the “background” of urban centers. It is located on the roof of the building of Representation of Institut de Recherche pour le Developpement (IRD) in Côte d'Ivoire at 12 meters from the ground.
2.2.2. Korhogo (site K1, University Peleforo Gon Coulibaly UPGC).
The K1 site is located within the University Peleforo Gon Coulibaly in Korhogo (UPGC). It is located at 9°25′37,09′′N latitude and 5°37′47,17′′W longitude at the entrance of the city, near one of the main roads. The privileged position of this site allows the monitoring of urban background pollution. This site allows the average exposure levels of the population to the atmospheric pollution phenomenon called “background” to be followed at the periphery of the urban centre, thus allowing to better observe the external influences such as road traffic. It also allows the primary pollutants from domestic fires to be followed.
2.3. Sampling methods
Samples were collected on quartz and Teflon filters, depending on the analytical techniques further considered. Quartz filters were used to obtain the mass concentrations and carbonaceous aerosol, especially elemental carbon (EC) and organic carbon (OC), while Teflon filters were used to quantify the content of water-soluble ions (WSI). The measurements were carried out using the aerosol collectors developed in the INDAAF project (https://www.aeris-data.fr/projects/indaaf/), which have been used in the DACCIWA project in Côte d'Ivoire and Benin (https://www.imk-tro.kit.edu/10052.php), notably in the work by Djossou et al.16
These collectors were composed of 2 independent measurement lines and each line was composed of a counter (GALLUS type G4), a ball flowmeter (with a Cole Palmer micrometric valve including flow rate adjustable from 0 to 10 L min−1, accuracy 5%), a pump (KNF, 9 L min−1 N89 KNE-K), a watertight filter holder (47 mm size) and a cut-off head (Rupprecht and Patashnik) to select aerosols by size class, either PM2.5 or PM10 particles. The pump flow rate of 5 L min−1 was necessary for the proper operation of the cut-off head. In addition to this collector, another measurement line with URG cyclone to select PM2.5 particles was used on each site. This line had the same operating principle as the collectors, i.e., atmospheric aerosols are sucked in by a fixed flow pump and collected on filters. For weekly measurements, the measuring line operated for 15 min per hour (i.e., 6 h per day) due to an electric programmer to avoid the overheating of the pumps. This sampling method was used in the DACCIWA project. The filter holders used to expose the filters were cleaned regularly with alcohol. All handling of the filters was done with dedicated tongs. The type of filter used depended on the chemical parameters of interest.
2.3.1. Quartz filters.
Quartz fibre filters were used both in the collector and in the measurement line to collect PM2.5 aerosols at the A1 (UFHB) and K1 (UPGC) sites, respectively. They were from the Whatman brand and required preparation before use. Each quartz filter was burned for 48 h at 400 °C in an oven to remove impurities, and then referenced, weighed on a submicron balance (SARTORIUS) and stored in a Petri dish (single use). These references made it possible to identify the filter throughout its journey from preparation to the various analyses, including exposure at the measurement sites. The analysis carried out on these filters included measuring the aerosol mass and carbonaceous aerosol (EC and OC) concentrations in PM2.5 and PM10 aerosols.
2.3.2. Teflon filters.
Teflon filters or polytetrafluoroethylene (PTFE) were used in the collector to sample PM2.5 aerosols at the A1 (UFHB) and K1 (UPGC) sites. These filters were produced by PALL Corporation, and also used in several other programs and projects. As with the quartz filters, the Teflon filters were stored individually in referenced Petri dishes. The collected Teflon filters were used to analyse the mineral composition of the PM2.5 aerosol.
2.4. Gravimetric and chemical analysis
The analyses of the collected samples were performed at the Laboratoire d'Aerologie (Laero) in Toulouse (France). The analyzers from Laero were regularly tested for certification. In addition, Laero is the reference laboratory for several international programs and projects (such as INDAAF) and its results are published in international journals.4,17–19
2.4.1. Gravimetric analysis.
The mass concentrations were calculated using eqn (1). |  | (1) |
with,
ΔM = Mf − Mi and ΔV = Vf − Vi |
where Mf and Mi are the mass (μg) weighed after and before exposure and Vf and Vi are the volume (in m3) given by the counters after and before exposure, respectively.
For each quartz filter, weighing was performed before and after sampling. The mass difference (ΔM) was used to obtain the mass of aerosols collected. This technique is a reference method and the equipment used has already been used in several studies.4,17,19 Prior to each weighing, the filters were exposed for 24 h in a dedicated chamber installed in a clean room. This allowed the filters to adapt to the ambient air of the room housing the submicron balance. This balance was very sensitive to temperature and humidity. Some filters were weighed twice to evaluate the uncertainty of the weighing.20
The volume of air sampled (ΔV) was given by the difference between the final volume and initial volume given by the counters at the site before and after each filter was exposed. Pump flow checks were regularly performed on each measurement line.
2.4.2. Carbonaceous aerosol analysis.
The carbonaceous aerosol analysis was performed by the two-step thermal method developed by Cachier et al.21 and used in several works including Doumbia et al.9 Keita et al.19 and Adon et al.4Fig. 2 shows the analysis scheme for the carbonaceous species. The sample boat where the filter was installed was first run through an oven at 1000 °C to be cleaned. The machine blank was obtained when the analysis was performed without anything in the oven, whereas the operational blank combined the machine blank with an empty sample boat in the oven. These blank measurements were performed before, in between and after the sample analysis.
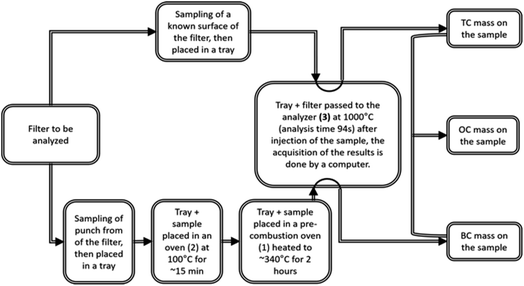 |
| Fig. 2 Scheme of carbon analysis of quartz filters. | |
During the analysis performed using a BRUKER G4 ICARUS analyser, the TC (total carbon) and EC (elemental carbon) were directly obtained. A portion of the filter with a known surface area was first passed directly into the G4 ICARUS analyser to obtain the TC concentrations present on the analysed portion of the filter. In parallel, another portion of the same filter was pre-combusted in an oven heated to ∼340 °C under an oxygen flow for 2 h to remove the organic carbon (OC) fraction. After this step, this portion was analysed by the G4 ICARUS to obtain the amount of EC. Moreover, at the exit of the pre-combustion step, the samples awaiting EC analysis were placed in an oven heated to 100 °C to protect them from impurities.
Finally, the difference in mass between TC and EC (MTC − MEC) gave the mass of OC.
It should be noted that a multi-point calibration of the analyser was performed using tests with a sucrose solution (concentration of 1 μgC μL−1). Indeed, regression lines were drawn for low total carbon and high total carbon conditions, comparing the values of the integrals obtained by the G4 ICARUS and known sucrose concentrations.
2.4.3. Water-soluble ion (WSI) chemical analysis.
Mineral species concentrations (Na+, NH4+, K+, Mg2+, Ca2+, SO42−, NO3−, Cl−, etc.) were assessed by the analysis of the Teflon filters collected at sites A1 and K1. These analyses were performed at the Laboratoire d'Aerologie with Thermo Dionex ion chromatographs (ICS 1000, ICS 1100, and ICS 5000).
Table S1† shows the different species analysed by each of the chromatographs used. Before each series of analyses, 3 standard solutions (standard 1, 2 and 3) with known concentrations were analysed. The results of the analyses of the standard solutions must have a correlation coefficient R2 of greater than 0.99. The concentrations of standard solutions 1 and 3 formed the interval [min, max] containing the concentrations of the solutions to be analysed. The filters to be analysed were first put in solution in vials with 20 mL of ultrapure water, and then passed through an ultrasound tank for 30 min. A further description of this methodology can be found in Adon et al.22
2.4.4. Calculation of uncertainty.
Uncertainty is systematic or statistical. Between them, systematic error is more common, which is attributed to the quality of the measuring instrument or measurement protocol and poor knowledge of the measurement process. Alternatively, statistical or random error occurs when the analysis of a quantity is performed several times. Thus, the error or uncertainty, whether systematic or statistical, must consider these two aspects. For the types of analysis (mass of aerosols, mass of carbonaceous species and mass of mineral species), the uncertainties (Unc) are calculated by the formula given by eqn (2): |  | (2) |
where, ErR is the relative error; Vo is the observed value of the parameter and LoD is the limit of detection.
The relative error (ErR) and the detection limit (LoD) depend on the method of analysis.
Table 1 shows the methods for determining the uncertainties and the values obtained for the 3 types of analyses, i.e., mass of PM, mass of carbonaceous species and mass of mineral species. The uncertainties were used for the analysis of the contribution of the sources.
Table 1 Method for determining uncertainties in sample analysis
Uncertainties |
ErR |
LoD |
PM mass |
Determination: this is the average of the differences between the results of double weighing the filters after exposure. Indeed, one filter out of 10 was weighed twice |
Determination: this is a specific characteristic of the device, given by the manufacturer, i.e., 2 μg, based on an average sampling volume of 12.65 m3 |
ErR = 2% |
Value: 0.16 μg m−3 |
Carbon species mass EC and OC |
Determination: this is the average of the deviations between the double analysis results of 10 blank filters. These values varied between 19.6 and 21.4 μgC. Thus the differences represent 1% to 6% of the mass of carbon obtained |
Determination: this is calculated from the values of the blank filter analyses, which include the uncertainties related to the materials (machine, tray and blank filter). The detection limit is equal to the average of the blanks + 3 × the standard deviation, i.e., 22.01 μgC, which will be related to the average volume of air sampled of 12.65 m3. It was determined on a series of 20 samples |
ErR = 3% |
Value: 1.74 μgC m−3 |
Water-soluble ions (WSI) mass |
Determination: samples are only analysed if the coefficient of determination R2 > 0.99. It would be fair to take ErR ∼0%, but to consider this component, we took ErR = 1%, i.e., 0.01 |
Determination: they are calculated from the white filter values. It was determined on a series of 20 samples |
Thus, for each species, the LoD was determined. However, zero LoDs obtained will be replaced by the lowest LoD |
ErR ∼0% |
Value: Ossohou, 2020 (ref. 23) |
2.5. Analysis of the contribution of the sources
As described in Doumbia et al.,9 two multivariate statistical analysis methods were used to establish the source apportionment of PM2.5 in our study, i.e., The Principal Component Analysis (PCA) and Positive Matrix Factorization (PMF). These methods allowed us to study the dependence between several variables (p). These methods make it possible to reduce the number p of variables to a number k of factors (p > k), while keeping the most information from the variables. These 2 methods are complementary and their combination allowed to obtain quantifiable information on the contribution of the sources to the atmospheric concentrations of PM2.5.
2.5.1. Principal component analysis.
Principal Component Analysis (PCA) is a statistical method for studying the dependencies between multiple variables or parameters. It allows the grouping of variables that present statistical similarities around a factor.
It was developed by Pearson in 1901, and since then its fields of application have continued to grow.24 Today, PCA is applied in several fields for the analysis of large databases.
PCA transforms a large number (p) of variables into factors (k), based on the similarities detected in the analysis of the variables. Consequently, a reduced number of factors will eventually represent almost all the information from the variables.25
Assuming a linear relationship between the variables (which are the species concentrations here) and a number of p factors (which are the number of sources here), the PCA is expressed as follows:
|  | (3) |
where,
Zij is the reduced mass concentration of the species (
i) in the sample (
j), with
k the number of factors (from 1 to
p, which represents the number of species),
gik is the contribution of the species (
i) to the component at the source (
k) and
hkj is the contribution of the component from the source (
k) to the sample (
j).
In the context of air pollution, the variability of observed concentration levels requires the use of normalized PCA. The normalization of values is done through centering and reduction of the series of each variable. Centering allows the impact of extreme values to be reduced by making the mean of the series equal to zero, without changing the nature of the point distribution (point cloud). The reduction process allows to obtain a variance equal to 1. These transformations applied to each of the variables put them in a normalized form, which allowed us to get rid of the dimensions of the variables. Following the normalization, the calculation of the factors allowed us to compose factorial plans from the axes defined by the most significant factors, with the aim to restore almost all the variance of the data set. In the context of particulate pollution, these factors represent a grouping of variables (chemical elements). According to the source profiles (given in the literature), these factors are associated with the different emission sources. The contribution of each variable to each factor gave additional elements of interpretation. Eventually, the application of the PCA upstream of the PMF allowed the identification of the number of factors to retain.
2.5.2. Positive matrix factorization (PMF).
Positive Matrix Factorization (PMF) is a quantitative multivariate analysis method, which was created by Paatero.26,27 The PMF method involves decomposing a database into non-negative matrices, allowing the original data to be explained. Similar to PCA, it is not necessary to know the source profiles of the emission beforehand. As a complement to the PCA results, PMF allows the quantification of the contribution factors of the different sources and requires a large data set and a large time series of variables to work.
PMF was originally developed and used for the study of sources of particulate matter in ambient air based on model input chemical species such as metals, major inorganic species (anions or cations) and specific organic tracers.27–30
Receptor models are mathematical approaches to quantify the contribution of sources to samples based on source composition or speciation. Composition or speciation is determined using analytical methods appropriate to the environment, and associated with key species, where combinations of species are required to separate impacts.
As shown in eqn (4), the X matrix is decomposed into: the matrix F (source), the species profile matrix in each source, and the matrix G, the contribution matrix by each factor (source) to the chemical equilibrium of each sample.31
| 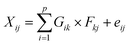 | (4) |
where,
p is the number of factors,
X is the concentration matrix collected at the site, which is composed of the samples (by dates) in rows (
i) and the measured species in columns (
j), with
Xij for each,
G is the contribution matrix of sources (
k) for each sample (
i),
F is the matrix of species profiles (
j)
versus source (
k) and
e is the matrix of residual errors, function of the samples and the species.
Moreover, the elements of the matrices G and F were constrained to be non-negative.
For this, a weighted least squares approach was used to minimize the Q function, which is called the objective function.
To achieve this objective, the Q function (eqn (5)), also called objective function or cost function, must be evaluated and minimized through several randomized simulations.
| 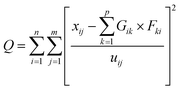 | (5) |
For a species, the sum of the concentrations from the factors (sources) cannot be greater than the concentration.
The PMF analysis uses sample time series, containing the concentrations of each of the chemical species, and the associated uncertainties. This feature allowed us to account for confidence in the measurement. For example, data below the detection limit could be retained for use in the model, with the associated uncertainty adjusted so that these data points have less influence on the solution than measurements above the detection limit.
2.6. Meteorology
Local meteorology (wind direction and intensity, specific humidity, and rainfall) has a proven influence on air pollution.32 It conditions the emissions, transformations, and transport, but also the removal (deposition) of air pollutants. In addition, regional winds carry desert dust and combustion aerosols from, for example, nearby and/or regional biomass burning, which greatly influence the observed concentrations and chemical composition of collected aerosols. The intensity of these parameters varies greatly during the year depending on the season. Moreover, the analysis of some meteorological parameters allowed a seasonal breakdown of the year to better understand the variations in the measured concentrations.
The analysis of specific humidity and rainfall data measured on the synoptic stations managed by the Agence pour la Sécurité de la Navigation Aérienne en Afrique et à Madagascar (ASECNA) at the Félix Houphouet-Boigny airport in Abidjan (Station No. 65578) and at the Korhogo airport (Station No. 65536) was carried out. Daily rainfall was collected at each station and the parameters collected were used to calculate specific humidity.
In Abidjan, the specific humidity was almost constant over the three years (Fig. 3). This is due to the position of this city on the coast of the Atlantic Ocean. Alternatively, the rainfall showed differences over the year, but similarities from one year to another. We noted that the months of January and February have very low rainfall. This observation was also valid for the month of August.
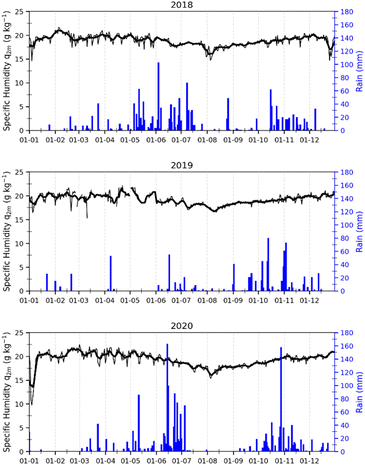 |
| Fig. 3 Variations in specific humidity (black) and rainfall (blue) in Abidjan from 2018, 2019 and 2020. | |
In Korhogo, Fig. 4 reveals another picture, i.e., specific humidity is low from December to February of the following year, and rainfall is concentrated between March and November over the three years. In Korhogo, the observation of these two parameters allowed us to clearly define the seasons. Indeed, the low values of specific humidity revealed an atmosphere poor in water, and therefore a dry season from December to February. Conversely, from March to November, the atmosphere was more humid with high rainfall, and hence a wet season.
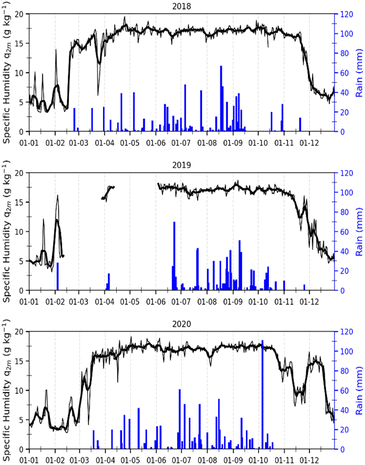 |
| Fig. 4 Variations in specific humidity (black) and rainfall (blue) in Korhogo from 2018, 2019 and 2020. | |
Given the marine influences on Abidjan, we could not establish specific seasons for Abidjan. Thus, we used the following division of seasons: a dry season from December to February and a major wet season from March to November. In accordance with our study period, we defined dry season 1 (DS1) from December 2018 to February 2019 and dry season 2 (DS2) from December 2019 to February 2020. Regarding the periods of March to November of 2018 and 2019, they were wetter, and thus wet seasons 1 (HS1) and 2 (HS2), respectively.
The dry seasons were recognized as periods of high pollution because during these periods, the phenomenon of wet deposition of atmospheric particles is low, or even zero, which increases their concentrations in the atmosphere and promotes the aging of aerosols. Moreover, biomass burning is recurrent, as well as the strong resuspension of aerosols due to the dryness of the soils and desert dust transported.
3. Results
3.1. Temporal variations in PM2.5 aerosol concentrations and their EC and OC content in Abidjan (site A1, UFHB) and Korhogo (site K1, UPGC)
Fig. 5(a and b) show the interannual evolution of the monthly average PM2.5 concentrations in Abidjan (a) and Korhogo (b), as well as their content in EC and OC over the period of December 2018 to March 2020.
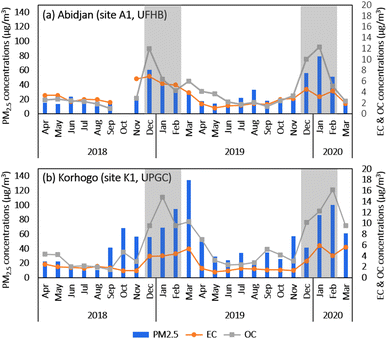 |
| Fig. 5 Monthly average concentrations of PM2.5 aerosols and their EC and OC contents at (a) Abidjan (site A1, UFHB) and (b) Korhogo (site K1, UPGC). The gray-shaded areas represent the dry seasons (DS), from left to right, DS1 and DS2. | |
In Abidjan, the PM2.5 concentrations ranged from 7.5 to 127.6 μg m−3, with an average of 29.4 ± 22.9 μg m−3 over the entire period. This average concentration is above the respective WHO standards (2021). The median of the PM2.5 concentrations is 20.5 μg m−3. As a reminder, the median gives statistical information on the data set, i.e., the value for which we have the most samples of higher and lower concentrations. Here, according to the median values, it was concluded that almost half of the year, the concentrations were higher than the daily standards of the WHO33 (15 μg m−3). The concentrations of EC and OC in PM2.5 varied from 0.3 to 9.3 μg m−3 and 0.2 to 23.9 μg m−3, respectively. On average, the concentrations of EC and OC were 3.2 μg m−3 and 4.3 μg m−3, respectively. The TC content of PM2.5 was 27 ± 13% on average. The OC/EC ratio was 1.6 over the study period. The concentrations during the two dry seasons DS1 (Dec 2018, Jan 2019, and Feb 2019) and DS2 (Dec 2019, Jan 2020, and Feb 2020) and that for the rest of the year (wet season and shoulder season) are shown in Fig. 3 and Table 2. The mean PM2.5 concentrations were 44.9 ± 26.5 μg m−3 for DS1 and 61.8 ± 31.3 μg m−3 for DS2. Thus, the concentrations during DS2 were almost twice that during DS1. It is interesting to note that the OC concentrations also increased from DS1 to DS2, whereas EC decreased.
Table 2 Average concentrations in μg m−3 of PM2.5, EC, OC and OC/EC ratio over the whole study period (04/2018–03/2020), the wet seasons HS1 (04/2018–11/2019) and HS2 (03/2019–11/2019), and the dry seasons DS1 (12/2018–02/2019) and DS2 (12/2019–02/2020)
City (site id, site name) |
Period |
PM2.5 |
EC |
OC |
OC/EC |
Abidjan (site A1, UFHB) |
Study period |
29.6 ± 22.9 |
3.2 ± 1.9 |
4.3 ± 4.1 |
1.6 ± 2.1 |
HS1 |
17.5 ± 6.8 |
3.2 ± 1.7 |
2.3 ± 1.2 |
0.7 ± 0.4 |
DS1 |
44.9 ± 26.5 |
6.0 ± 1.2 |
7.9 ± 5.7 |
1.3 ± 0.7 |
HS2 |
21.3 ± 7.3 |
2.0 ± 0.8 |
2.8 ± 1.4 |
1.7 ± 1.8 |
DS2 |
61.8 ± 31.3 |
4.0 ± 1.8 |
9.2 ± 5.5 |
3.5 ± 4 |
Korhogo (site K1, UPGC) |
Study period |
46.8 ± 36.5 |
2.6 ± 1.8 |
6.1 ± 5.3 |
2.3 ± 1.4 |
HS1 |
27.0 ± 22.9 |
1.8 ± 0.5 |
2.7 ± 1.7 |
1.5 ± 1.0 |
DS1 |
71.9 ± 33.4 |
4.1 ± 1.3 |
11.2 ± 4.3 |
2.8 ± 1.2 |
HS2 |
40.7 ± 35.2 |
1.7 ± 1.0 |
4.1 ± 3.1 |
2.5 ± 1.4 |
DS2 |
82.0 ± 42.0 |
4.2 ± 2.4 |
12.6 ± 7.1 |
3.2 ± 1.9 |
Consequently, the OC/EC ratio in PM2.5 was approximately 1.3 in DS1 and 3.5 in DS2. This increase in the OC/EC ratio in DS2 can be attributed to the higher contribution of secondary organic aerosols, as suggested by Satsangi et al.34 This can be associated with weather conditions that are more conducive to the formation of secondary aerosols, such as reduced rainfall and lower cloud cover during DS2 in comparison to DS1. Therefore, there was a greater contribution from incomplete combustion sources during DS2. The analysis of the back-trajectories to Abidjan, in conjunction with regional active fires in DS1 and DS2 (Fig. 3 and 4), did not provide evidence linking the increase in OC/EC to the impact of biomass burning.
In the case of PM2.5, its concentrations during HS1 and HS2 were 17.5 ± 6.8 μg m−3 and 21.3 ± 7.3 μg m−3, respectively, which are 2–3-times lower than the concentrations during the following dry season. The difference in higher concentrations during the dry seasons than the wet seasons is mainly due to the low rainfall observed during the dry seasons, limiting the removal processes of particles by wet deposition.
In Korhogo (Fig. 5(b) and Table 2), the mean concentration of PM2.5 μg m−3 was 46.8 ± 36.5 μg m−3, in the range of 6.9 to 165.3 μg m−3, with a median concentration of 36 μg m−3. We noted that the average concentrations observed were 2- to 3-times higher than the standards and recommendations of the WHO33 and Côte d'Ivoire, respectively. The concentrations of EC and OC are on average 2.6 ± 1.8 μg m−3 and 6.1 ± 5.3 μg m−3, respectively. On average, TC represents 23 ± 14% of PM2.5 with an average OC/EC ratio of 2.3. This OC/EC ratio value is higher than that observed in Abidjan. This could be due to both the greater contribution of incomplete sources such as the domestic fire source and two-wheeled vehicles, which are more important in Korhogo than in Abidjan, but also to more important secondary aerosol formation. The mean concentration of PM2.5 during DS1 was 71.9 ± 33.4 μg m−3 against 82 ± 42 μg m−3 during DS2, representing a 20% increase, whereas the OC/PM2.5 and OC/EC ratios remained in the same order of magnitude. The back-trajectories to Korhogo (Fig. S7†) during DS1 originated from the African continent, while that of DS2 originated from the Atlantic Ocean. The influence of regional continental (Fig. S7 and S9†) sources on Korhogo could explain the differences observed between DS1 and DS2. This hypothesis was confirmed by the analysis of mineral species. The PM2.5 concentrations during HS1 (27.0 ± 22.9 μg m−3) and HS2 (40.7 ± 35.2 μg m−3) were 2- to 3-times lower than during the dry seasons. It is interesting to note that the concentrations were generally higher in Korhogo than in Abidjan either in the dry seasons or in wet seasons, in agreement with Gnamien et al.20
In Abidjan, the OC/EC ratio values (Table 2) were generally between 0.7 and 3.5 in all the seasons, with an average of 1.6, suggesting a link with the road traffic source,35–37 as on the road traffic site of Djossou et al.,17 which had a value of 2. However, the standard deviations of this ratio were very large, reflecting the wide variability of the ratio values, and therefore the significant influences of various sources. In Korhogo, the OC/EC ratios were generally greater than 2 (with the exception of HS1), with smaller standard deviations from the ratio values. This may reflect the predominance of biomass combustion sources at this site. In fact, an OC/EC ratio greater than 2 indicates both a strong contribution from biofuel and biomass burning38–40 and the presence of secondary organic aerosols (AOS).34
The average PM2.5 concentrations in this study in Abidjan were in the same order as that obtained with the same procedure by Djossou et al.17 at traffic sites in Abidjan (32 ± 32 μg m−3) and Cotonou (Benin) (32 ± 24 μg m−3) and at a landfill fire site in Abidjan (28 ± 19 μg m−3), whereas they were higher in Korhogo. However, both of them remained lower than that at a domestic fire site in Abidjan (149 ± 69 μg m−3), which was a site very close to the source. In addition, other studies have been conducted in West African cities. Thus, in Accra, Dionisio et al.41 obtained 21 μg m−3 and 39 μg m−3 for PM2.5 at sites located in areas with a high and low socioeconomic status, respectively. These concentration levels, as well as that observed by Arku et al.,42 Boman et al.,43 Dieme et al.,7 Doumbia et al.9,44 and Garrison et al.,45 in Accra (Ghana), Ouagadougou (Burkina Faso), Dakar (Senegal) and Bamako (Mali) are in the same order of magnitude as that obtained at our sites, respectively.
3.2. Variations in the concentrations of water-soluble ion (WSI) species in PM2.5 aerosols
At Abidjan site A1, the sum of the water-soluble ions (WSI) represents 10.6 ± 8.8% of the PM2.5 mass (Fig. 6(a)), with sulfate (1.16 ± 1.25 μg m−3) and potassium (0.41 ± 0.52 μg m−3) being the most abundant anion and cation, respectively. Fig. 6(a) shows that sulfate, nitrate, and ammonium, also called SNA, are the major species of WSI.46 The average level of SNA was 1.87 μg m−3, contributing to 7% of the PM2.5 mass. SNA (SO42−, NO3−, and NH4+) accounted for 4.1%, 1.5%, and 1.4% of the PM2.5 mass, respectively. The proportions of K+ (1.3%), Na+ (0.7%), Ca2+ (0.5%), oxalate ions (0.4%), Cl− (0.3%), and Mg2+ (0.2%) were relatively small compared to SNA. The rest of the ions, i.e., acetate ions (0.09 μg m−3) and formate ions (0.05 μg m−3), had contributions of less than 0.1%.
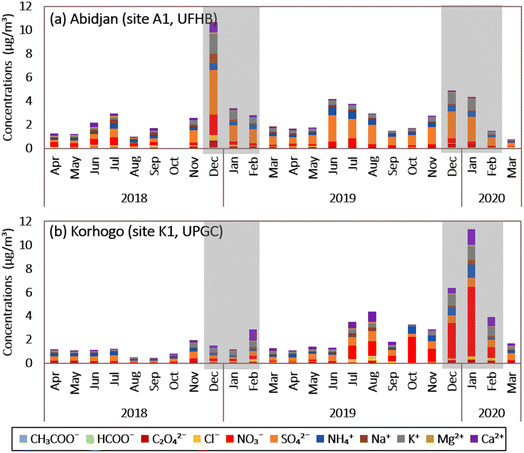 |
| Fig. 6 Monthly mean concentrations of soluble ions (CH3COO−, HCOO−, C2O42−, Cl−, NO3−, SO42−, NH4+, Na+, K+, Mg2+ and Ca2+) contained in PM2.5 aerosols collected in (a) Abidjan (site A1, UFHB) and (b) Korhogo (site K1, UPGC). The gray-shaded areas represent the dry seasons (DS), from left to right, DS1 and DS2. | |
The highest peak was observed for the month of December 2018, with a WSI concentration (excluding acetate and formate ions) of 10.6 μg m−3, or 12.8% of the PM2.5 mass. These high values appeared in 3 of the 4 weeks of December 2018. Also, we observed lower WSI concentrations (mainly sulfate, nitrate and potassium) during DS2 compared to DS1. This high value in December 2018 was reflected in the average observed during DS1, i.e., 13.5 ± 12.6% (caused mainly by SNA, which represents 4.1%, 1.5% and 1.4% of PM2.5, respectively) compared to 6.3 ± 4.1% of WSI in PM2.5 during DS2. SNA alone accounted for 6.51% and 4.13% of WSI during DS1 and DS2, respectively.
As shown earlier, the analysis of the December 2018 back trajectories in A1 showed a difference compared to December 2019. Indeed, in December 2018, the back trajectories (Fig. S6†) and wind roses (Fig. S8†) all originated from the west coast of South Africa, in the Atlantic Ocean, whereas in December 2019, 2 origins were observed, i.e., some of them is from the Atlantic Ocean as in 2018 when the other part originated on the African continent, more precisely in West and Central Africa. These observations allowed us to link the variations in the WS1 concentrations to the greater contribution of marine source in DS1 than in DS2.
During the wet seasons (HS1 and HS2), the WSI had the same order of magnitude, i.e., 11.2 ± 9.6% and 11.4 ± 7.9% of PM2.5, respectively. In contrast to EC and OC, the WSI concentrations were significant in the summer period, for the months of June, July and August (Fig. 6(a)) compared to the other months of the wet season, with a predominance of SNA followed by K+, Ca2+ and Na+. These variations suggest the influence of meteorology on the chemical composition of aerosols.
Fig. 6(b) shows the interannual evolution of the WSI concentration at Korhogo (site K1). The sum of water-soluble ions (WSI) represents 6 ± 8.7% of the PM2.5 mass collected at site K1, with nitrate (0.77 ± 1.81 μg m−3) and K+ (0.34 ± 0.33 μg m−3) being the most abundant anion and cation, respectively.
The annual average level of SNA (SO42−, NO3− and NH4+) was 1.51 μg m−3, contributing 3.16% of the PM2.5 mass in Korhogo. SNA accounted for 1.4%, 1.5% and 0.69% of PM2.5 aerosols, respectively, and 60% of WSI. K+, Ca2+, oxalate, Na+, Cl− and Mg2+ ions accounted for 0.78%, 0.70%, 0.32%, 0.27%, 0.25% and 0.1%, respectively. Acetate (0.02 μg m−3) and formate (0.02 μg m−3) ions had contributions of less than 0.1%, as for the Abidjan A1 site.
The largest peak was observed in January 2020, and more generally during DS2. In January 2020, the WSI concentration was 11.3 μg m−3, or 10.8% of PM2.5. During DS1, WSI accounted for 2.6 ± 1.7% of PM2.5versus 8 ± 7.8% during DS2, with SNA contributing 0.83% and 4.91% of WSI during DS1 and DS2, respectively. This agrees with our earlier results. The back-trajectories (Fig S7†) and wind roses (Fig. S9†) to Korhogo during DS1 originated from the African continent, while that of DS2 originated from the Atlantic Ocean. Then, in DS2, a greater contribution of marine sources was expected with larger WSI concentrations. Also, to the best of our knowledge, it is difficult to perform any comparison with previous studies here, given that previous studies on WSI in West Africa focused on intensive campaigns results. These chemical compounds, combined with carbonaceous species, can be used to quantify the various chemical fractions included in PM2.5 aerosols at the Abidjan and Korhogo sites.
3.3. Analysis of chemical composition of PM2.5 aerosol
Carbonaceous aerosol and soluble ion concentrations from the different analyses allowed us to reconstruct the different aerosol type fractions included in the PM2.5 aerosol. Thus, the concentrations of WSI, EC and OC allowed us to determine 5 main type fractions and evaluate the fraction not determined from our chemical and mass analyses of PM2.5. These fractions are:
Elemental carbon: EC obtained directly from the thermal analysis used in this work developed by Cachier et al.21 and applied by Keita et al.19 and Adon et al.4
Organic matter: OM = 1.8 × [OC] from Temesi et al.47 and Maenhaut et al.48 and [OC] analysed in this study followed the methodology of Cachier et al.21
Dust = 10.96 × [nss-Ca2+], avec [nss-Ca2+] = [Ca2+] − 0.038 × [Na+] from Sciare et al.49 for all the ions analysed in this study.
Sea salt = [Cl−] + [Na+] + [Mg2+] + [ss-K+] + [ss-Ca2+] + [ss-SO42-], avec [ss-K+] = 0.036 × [Na+]; [ss-Ca2+] = 0.038 × [Na+]; [ss-SO42-] = 0.252 × [Na+] from Sciare et al.49 for all the ions analysed in this study.
nss-Ions = [NH4+] + [NO3−] + [Mg2+] + [nss-SO42-] + [nss-K+] + [CH3COO−] + [HCOO−] + [C2O42−] for all the ions analysed in this study and,
n.d. = [PM2.5] − ([EC] + [OM]+ [dust] + [sea salt] + [nss-ions]), where [PM2.5] is the PM2.5 concentration obtained in this study.
Fig. 7(a and b) show the evolution of the chemical composition of the PM2.5 aerosols at sites A1 and K1, respectively. The 5 identified types of fractions explained on average 56.7% and 50.5% of the PM2.5 aerosol mass at Abidjan (site A1, UFHB) and Korhogo (site K1, UPGC), respectively. In decreasing order of contribution, at Abidjan, 26.7 ± 13.2%; 13.2 ± 8.4; 8.2 ± 7.1%; 5.6 ± 5.9% and 1.4 ± 1.6% were calculated for organic matter (OM), EC, nss-ions, dust and sea salt, respectively (Fig. 7(a)).
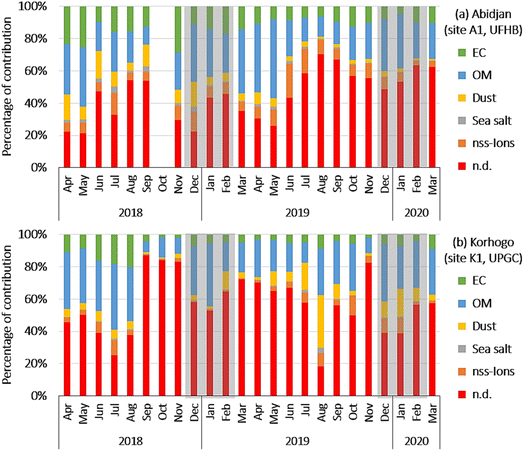 |
| Fig. 7 Main chemical fractions included in PM2.5 aerosol from (a) Abidjan (site A1, UFHB) and (b) Korhogo (site K1, UPGC). The gray-shaded areas represent the dry seasons (DS), from left to right, DS1 and DS2. | |
In Korhogo, 28.2 ± 18.1%, 9.4 ± 18.4%, 7.1 ± 6.1%, 4.4 ± 6% and 0.8 ± 1.6% were the contributions of OM, dust, EC, nss-ions and sea-salt, respectively (Fig. 7(b)).
The major fraction was organic matter in both the Abidjan and Korhogo sites, with close contributions. EC was the 2nd most important fraction in Abidjan (13.2%), but 3rd in Korhogo (7.7%), given that it was supplanted by Dust, with 6.1% and 9.4% in Abidjan and Korhogo, respectively. The nss-ions, with 9.1% and 4.4% in Abidjan and Korhogo, respectively, were in 4th position. The contribution of sea salt was the lowest with 1.5% in Abidjan and 0.8% in Korhogo. The carbonaceous fractions (EC and OM) contributed 40% and 36% of the chemical composition of PM2.5 in Abidjan and Korhogo, respectively, underlining the importance of the contribution of combustion sources to PM2.5 aerosols. Also, as expected, the dust contribution was more important in Korhogo than in Abidjan.20 However, the unexplained fractions were very large, sometimes exceeding 50%. The absence of trace elements and metals from the analyses underestimates the dust fraction, according to Guinot et al.50
In this case, multivariate analyses can identify and quantify the contributions of the different sources.
3.4. Analysis of source apportionment of PM2.5 aerosol
3.4.1. Number of contributing sources.
Principal component analysis (PCA) is one of the most common methods of multivariate analysis. It is applied to different types of data to find dependencies between variables to reduce their number by grouping them. In this study, the variables were the chemical species (EC, OC and WSI). In addition, given that EC and OC were considered independently, TC was not included in the analysis to avoid double counting. From April 2018 to March 2020 (i.e., 105 weeks), the 84- and 83 weeks samples were retained in Abidjan and Korhogo, respectively. Indeed, when the concentrations of a species or a group of species was missing, that week was excluded from the study.
Subsequently, the species were grouped around the principal components, which are also called factors. Here, we use the term factor as a linear combination of species with specific coefficients for each variable and within each factor. These coefficients represent the degrees of correlation between the variables and the factor.9 Thus, the stronger a species correlates with a factor, the more it influences that factor, which could be used to identify the source associated with the factor. In this type of study, a small number of factors that can explain most of the variance is desirable. To determine the number of factors to retain, several methods (criteria) are available. Doumbia et al.9 proposed the following criteria: criterion 1 retains as many axes as necessary to reach the desired threshold of explained variance, a threshold to be defined; criterion 2 retains eigenvalues greater than the value of 1 (following the Kaiser–Guttman criterion, commonly called Kaiser criterion) and criterion 3 is based on the scree plot criterion using the curve of eigenvalue decay, with the number of factors to be retained corresponding to the first inflection point detected on the curve.
Fig. 8, illustrating criterion 2, shows the eigenvalue curve as a function of the number of factors (in blue), with the Kaiser criterion in red. This figure reveals the difficulty of applying criterion 2 in both Abidjan and Korhogo because the inflection point was not always clearly identifiable.
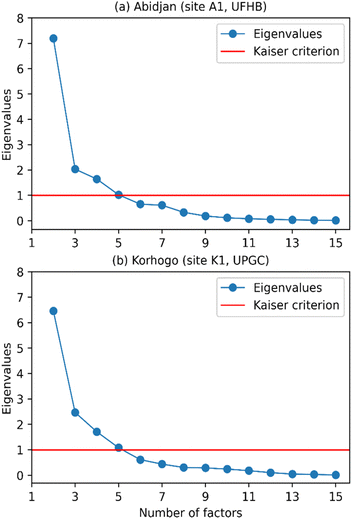 |
| Fig. 8 Eigenvalue decay curves as a function of the number of factors for (a) Abidjan (site A1, UFHB) and (b) Korhogo (site K1, UPGC), with criterion 2 (Kaiser criterion) in red. | |
Moreover, the application of criterion 1 poses a problem in the threshold to choose. Indeed, the choice of the threshold could appear subjective, i.e., made according to the number of factors sought. Pires et al.51 chose a threshold of 90% of variance explained. In our case, this threshold led to 6 sources (factors) in Abidjan and Korhogo. Alternatively, a threshold of 80% led to 4 sources in Abidjan and Korhogo. As a reminder, the choice of the number of factors must allow for the restitution of almost all the information in the database. To avoid grouping of sources, we used criterion 1, with a maximum threshold of 90%. However, we noted that 88% and 89% of the variance explained in Abidjan and Korhogo, respectively, led to 5 factors. This choice seemed appropriate because it seemed inappropriate to add a 6th factor that represents less than 4% of the variance to reach 90%. Indeed, in the absence of trace elements, the identification of certain sources was difficult. Thus, we retained 5 factors (contributing sources) in Abidjan and Korhogo.
3.4.2. Sources contributing to PM2.5 aerosols.
The application of PMF (Positive Matrix Factorization) is based on the 5 factors identified by PCA, both in Abidjan and Korhogo. In this study, version 5 of the EPA PMF software, developed by the US Environmental Protection Agency (US EPA), was used. This software uses the time series of weekly concentrations of chemical species and the associated uncertainties as the input. Thus, the weekly concentrations each represent an independent sample. It is possible to replace the missing data by the median of the series, but this option was not available because we had a significant number of validated samples. Indeed, in the dataset, 84 weeks were retained out of 91 weeks actually sampled in Abidjan. Alternatively, in Korhogo, all 83 weeks sampled were retained. As shown above, the concentrations of the chemical species did not allow the reconstruction of the whole mass of the collected PM2.5 aerosols. To account for the undetermined mass, and as recommended, PM2.5 was added to the species for PMF, with an uncertainty equal to 4 times the observed PM2.5 concentration.52–54
The uncertainties were calculated based on the recommendations provided by the U.S. EPA (2014) software user manual. If the species concentration was below the limit of detection (LoD), the concentration was replaced by half the LoD, and its uncertainty was 5 × LoD/6. According to the species, at site A1, the number of samples involved was 0 for C2O42−, Cl−, NO3−, Na+, NH4+, K+, Mg2+, Ca2+ and PM2.5, 3 for SO42−, 17 for OC, 21 for EC, 34 for HCOO−, and 39 for CH3COO−, out of 84 samples included in the analysis. Alternatively, for site K1, the number of samples involved was 0 for PM2.5, 1 for Cl−, NH4+, K+, Mg2+ and Ca2+, 2 for C2O42− and NO3−, 4 for SO42−, 6 for Na+, 12 for OC, 35 for HCOO−, 36 for EC and 46 for CH3COO−, out of 83 samples.
The application of PMF successively to the Abidjan (site A1) and Korhogo (site K1) datasets allowed us to quantify the contribution of the different sources to PM2.5. Thus, according to the tracers and species that contribute to each factor, a source was identified and attributed to each factor, which was 5 factors here. It often happens that the profile of the contributing species to a factor does not allow the identification of a source, but rather an association of source.
3.4.2.1 Abidjan (site A1, UFHB).
The simulations of the PMF analysis at Abidjan considering 5 factors give coefficients of determination (R2) between the concentrations predicted by the PMF and the observed concentrations of each species. We observed 3 classes, as follows: R2 < 0.4 for EC, CH3COO−, HCOO−, 0.4 < R2 < 0.7 for PM2.5, Na+, Mg2+, OC and R2 > 0.7 for C2O42−, Cl−, NH4+, NO3−, K+, Ca2+ and SO42. Species with R2 < 0.4 correspond to samples for which the concentrations were often below the respective LoD. In this case, as mentioned above, it is recommended to replace the concentration by LoD/2 and the uncertainty by 5 × LoD/6 by the U.S. EPA.55Fig. 9(a) presents the relative contribution to PM2.5 of the 5 sources obtained by our receptor model at A1 site of Abidjan.
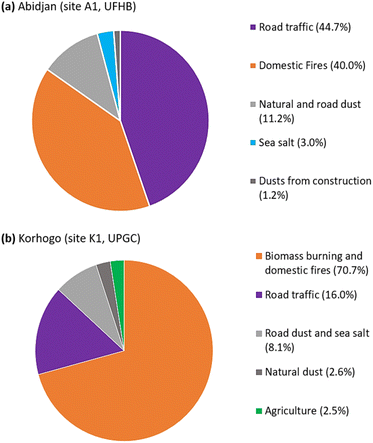 |
| Fig. 9 Apportionment of sources contributing to the PM2.5 aerosol mass identified at (a) Abidjan (site A1, UFHB) and (b) Korhogo (site K1, UPGC). | |
3.4.2.2 Abidjan source 1 (factor 2).
It contributed 44.7% of PM2.5 and 60.6% and 38.1% of EC and OC, respectively. According to Gupta et al.54 and Watson et al.,56 this source is road traffic. The OC/EC ratio in this source was 0.9 less than 2, which according to Guinot et al.,50 Pio et al.57 and Sandradewi et al.58 is consistent with that of the traffic source.17,59,60 This value is close to that of the sites heavily influenced by the traffic source.35–37,61 The high contribution of NH4+ (69.6%), which is associated with that of NO3− (21.3%) and SO42− (24.2%), to this factor highlights the presence of secondary aerosols. Indeed, NH4+, after its formation, remained present in the atmosphere as NH4NO3 and (NH4)2SO4.62 This process was confirmed by the correlation coefficient of 0.75 (p-value <0.05) between NH4+ and the sum of SO42− and NO3−. The presence of carboxylic acids (CH3COO− with 22.7% and HCOO− with 29.2%) confirmed the enrichment of this source by secondary aerosols according to Wang et al.63
3.4.2.3 Abidjan source 2 (factor 4).
It corresponds to 40.5% of the PM2.5 mass, contributing to 57.4% and 26.5% of the OC and EC concentrations, respectively, which are both tracers of domestic fire sources from biofuels according to Cachier and Ducret,64 Chow and Watson,65 Rogge et al.66 and Fine et al.67 The domestic fire source was confirmed by the good correlations between K+ and OC (0.72) and K+ and NH4+ (0.71), with p-values of <0.05, which are all tracers of combustion sources. Also, this source contributed to 61.5% of the mass of K+, which is tracer for this source according to Adon et al.,4 Sharma et al.62 and Wu et al.68
3.4.2.4 Abidjan source 3 (factor 1).
It consists of 11% of the PM2.5 mass, contributing to 42.53% and 18% of the concentrations of Ca2+ and Mg2+, respectively, which are natural dust tracers according to Pant and Harrison.69 In addition, source 3 contributed concentrations of 84.7% for C2O42−, 41.38% for CH3COO−, 40.9% for Na+, 12.9% for EC, 12.1% for Cl− and 4.5% for OC. These contributions can be explained by the resuspension of road dust. According to Gupta et al.,54 Ca2+, Cl− and OC can be considered as tracers of the latter source.
3.4.2.5 Abidjan source 4 (factor 3).
It contributed 3% of the PM2.5 mass, with only soluble ion (WSI) contributions. Thus, it contributed 50.2%, 23.8%, 19.2%, 17.7% and 13.4% to NO3−, SO42−, Na+, Cl− and K+, respectively, which highlight the sea salt source.70 Also, the lack of contribution to EC and OC (0% contribution) helped to confirm that they are marine salts. The absence of a contribution by Mg2+ highlights the inaccuracy of the receptor models in dealing with species that are lower in mass, but have multiple sources.
3.4.2.6 Abidjan source 5 (factor 5).
With 1% of the PM2.5 mass, this source contributed mostly Mg2+ (78.5%) and Ca2+ (15.4%), which are both dust tracers. In addition, this source contributed to 59.8% and 15.4% of the Cl− and Na+ concentrations, respectively, which are tracers of the marine source. Considering the marine origin of the construction sand,71 this factor represents the dust from construction,72 showing its importance in some of the species studied.
In Abidjan, the profile of the road traffic source (Fig. S3†) did not show any seasonal variation, as expected, because this source was not seasonally dependent. However, the profile of the biomass and domestic fire sources showed peaks during the dry seasons, with larger peaks during DS1 than DS2. The natural dust and road dust source profile showed larger contributions during year 1, with large peaks during DS1, but smaller contributions during the 2nd year of sampling. The marine salt source profile also showed a larger contribution in year 1 than in year 2. Finally, the profile of the construction dust source was similar to that of the natural and road dust sources. The analysis of the contribution profiles revealed seasonal specificities of some sources, particularly for OC. However, the large peaks observed during DS1 compared to DS2 should be put into perspective, given that this analysis was based on species that can only explain 56.7% of the collected aerosol mass. Thus, it will be important to reduce the undetermined fraction of the aerosol, a fraction that includes a large part of the trace elements, to better explain these seasonal variations.
3.4.2.7 Korhogo (site K1, UPGC).
The application of the PMF model to the K1 site of Korhogo resulted in R2 coefficients of determination that can be grouped into 2 classes. These were species with R2 < 0.4 for PM2.5, EC, OC, CH3COO−, SO42− and NO3−, and that with R2 > 0.6 for HCOO−, C2O42, Cl−, Na+, NH4+, K+, Mg2+ and Ca2+. The species with R2 < 0.4 showed high values related to the seasonal trends, which peaked likely due to localized events in time. However, because the uncertainties are a function of the concentration of the species in each sample, they increased with concentration. Fig. 9(b) and S1† show the contributions of the 5 different sources determined in our PMF study to the carbonaceous and inorganic species contained in the PM2.5 sampled at site K1 of Korhogo.
3.4.2.8 Korhogo source 1 (factor 4).
Source 1 contributed to 70.7% of the PM2.5 mass in Korhogo, making it the main source in Korhogo. This source was responsible for 79.9% and 62.3% of OC and EC, respectively, according to Cachier and Ducret64 and Chow and Watson,65 which are tracers of biomass burning source. In addition, it provided 77.3% of K+, which is also recognized as a tracer of this source (Wu et al.68 and Sharma et al.62). However, these tracers are also associated with the domestic fire source.67,73–75 Thus, this source combines both biomass burning and domestic fires source, which can be local or regional (driven by regional winds). The observation of the evolution of this source (Fig. S4†) showed higher contributions during the dry seasons (DS1 and DS2) compared to the wet seasons (HS1 and HS2).
3.4.2.9 Korhogo source 2 (factor 2).
This source consisted of 16% of the PM2.5 mass and contributed 22.7% and 13.2% of the EC and OC, respectively, which are tracers of the road traffic source. The OC/EC ratio of 1.46 in the source profile is close to the values for urban areas (influenced by the same source) in Europe76,77 and Morocco.78 In addition, it is responsible for the emissions of 82.5% NH4+; 43.4% NO3− and 32.9% SO42−, which are secondary aerosol tracers according to Sharma et al.62 The presence of sulphate, a tracer of the secondary aerosol component of this factor, could have resulted from photochemical interactions with atmospheric SO2, and the improved photochemistry during the warm season would promote these reactions.78,79 Fig. S3† shows very little seasonality in the contribution of this source to PM2.5 concentrations, which is typical of the traffic source, as is the case for Abidjan.
3.4.2.10 Korhogo source 3 (factor 1).
It contributed 8.1% of the PM2.5 mass, with contributions of 30% and 11.5% to the dust tracers Mg2+ and Ca+, respectively.69 Its low contribution to carbonaceous species (EC, OC) excluded the combustion source, but revealed an indirect link, i.e., it is a dust source from the resuspension of road dust.54 In addition, the 41.4% contribution of Na+, 21.8% of Cl− and 15.7% of K+ revealed the presence of sea salt.70 The association of these 2 sources was confirmed by Fig. S4,† where we observed a contribution of this factor, which increased both in the dry season (dust) and in the wet season (in sea salt).
3.4.2.11 Korhogo source 4 (factor 5).
It consisted of 2.6% of the PM2.5 mass, with contributions of 67.5% and 57.2% of Ca2+ and Mg2+, respectively, which are tracers of desert dust, i.e., natural dust.69 The 9.6% contribution to EC but 0% to OC, and also 27.1% and 21.1% to C2O42− and CH3COO−, respectively, highlighted the remote origin of a carbonaceous particle source. Indeed, only the inert fraction (EC) was observed and the reactive fraction (OC) seemed to have given way to carboxylic acids.
3.4.2.12 Korhogo source 5 (factor 3).
This source, which contributed 2.5% of the PM2.5 mass, consisted of 78.2% and 42.7% Cl− and NO3−, respectively, which according to Nyilitya et al.80 are tracers of the agriculture source, through the use of pesticides, animal manure and fertilizers. Furthermore, chlorine (Cl−), nitrogen (NO3− and NH4+) and potassium (K+) are the main constituents of pesticides, representing 98.9% of this source, confirming the link with agriculture, which is a very preponderant activity in the area. Indeed, Korhogo is the main cotton and mango production area of Côte d'Ivoire. These crops are grown with the use of fertilizers and pesticides. It should be noted that Koh et al.81 recommend an isotopic study to confirm the choice of the source agriculture from these results.
In Korhogo, the profile for the biomass burning and domestic fire source (Fig. S4†) showed a contribution of equal magnitude for DS1 and DS2 for the existing samples (indeed, note the absence of data during DS2), which was much larger than in the wet season. Therefore, this source does not clearly explain the variations in PM2.5 concentrations observed between DS1 and DS2. The traffic source profile showed no seasonal or interannual variation, despite some significant peaks. The road dust and sea salt source is a grouping of sources that could not be separated. However, according to the figure, it can be assumed that (1) the highest contributions to PM2.5 during DS1 and DS2 were due to road dust, a source increasing in the dry season, and (2) the high contributions to PM2.5 during the wet season (HS2) are associated with marine salts. The natural dust source had a larger contribution during DS1 than during DS2, which explains the higher particle concentrations during DS1. However, the larger contributions during HS2 suggested the influence of a third, unidentified source, which may be related to a dust event. The agriculture source had a larger contribution during the 2nd studied year than during the 1st. Thus, the appearance of this source in the second year may be attributed to the start of new agricultural activity or a change in agricultural practice near the study site.
4. Conclusions
This study presented the temporal trends in the chemical composition of PM2.5 aerosols in two cities, i.e., Abidjan in the south and Korhogo in the north in Côte d'Ivoire (West-Africa). The PM2.5 concentrations consistently exceeded both the WHO recommendations and Côte d'Ivoire's air quality standards, with the dry seasons (DS1 and DS2) showing concentrations more than twice that as the wet seasons (HS1 and HS2). Carbonaceous species (EC and OC) accounted for 27% in Abidjan and 23% in Korhogo, with Abidjan indicating a significant influence of traffic emissions and domestic fires (OC/EC ratios <2), while Korhogo reflected biomass and domestic fires (OC/EC ratios >2). Chemical composition analysis explained 56.7% and 50.5% of PM2.5 at the A1 and K1 sites, respectively. Five contributing sources were identified at each site. In Abidjan, they were road traffic (44.7%), domestic fires (40%), natural and road dust (11.2%), sea salt (3%), and dust from construction (1.2%). In Korhogo, is the factors were biomass burning and domestic fires (70.7%), road traffic (16%), road dust and sea salt (8.1%), natural dust (2.6%), and agricultural activities (2.5%). The PM2.5 concentrations in Abidjan and Korhogo exhibited strong links to rainfall and regional sources (dusts and biomass fires) in the dry seasons in Korhogo. The observed seasonal variations emphasized the importance of long-term aerosol composition monitoring to track the effectiveness of pollution source reduction efforts.
In conclusion, this study provides crucial information for identifying the main sources contributing to air pollution in each of the cities studied. By understanding the main sources of these emissions, it becomes possible to put in place targeted strategies aimed at mitigating the impact of urban particulate pollution, which is specific to each city. This approach will not only reduce atmospheric emissions, but also improve air quality, and consequently public health in these highly urbanised areas.
Author contributions
S. G. directed the work on this study, C. L. was the scientific leader, S. K., S. S. and J. B. took part in the design and analysis of the results, E. G. carried out the chemical analyses, K-D. M. and A. O. were involved in data collection in Abidjan and V. Y. was the P. I. of the PASMU project.
Conflicts of interest
There are no conflicts of interest to declare.
Acknowledgements
This study was funded by the Ministry of Education and Research of Côte d'Ivoire, within the framework of the Debt Reduction and Development Contracts (C2D) managed by the French National Research Institute for Sustainable Development (IRD). The authors would like to thank the AMRUGE-CI grant (Appui à la Modernisation et à la Réforme des Universités et Grandes Ecoles de Côte d'Ivoire). The authors thank all the members of the Aerosols and Pollution team of Abidjan (Laboratory of Material, Environmental and Solar Energy Sciences, LASMES of University Felix Houphouët-Boigny, UFHB) and Korhogo (University Péléforo Gon Coulibaly, UPGC) and the scientific and technical administrative staffs of the Laboratoire d'Aérologie (Laero, France).
References
- B. Kirenga, Q. Meng, F. van Gemert, H. Aanyu-Tukamuhebwa, N. Chavannes, A. Katamba, G. Obai, T. Molen, S. Schwander and V. Mohsenin, The State of Ambient Air Quality in Two Ugandan Cities : A Pilot Cross-Sectional Spatial Assessment, nt. J. Environ. Res. Public Health, 2015, 12, 8075–8091 CrossRef CAS PubMed.
- A. J. Cohen, H. Ross Anderson, B. Ostro, K. D. Pandey, M. Krzyzanowski, N. Künzli, K. Gutschmidt, A. Pope, I. Romieu, J. M. Samet and K. Smith, The Global Burden of Disease Due to Outdoor Air Pollution, J. Toxicol. Environ. Health, Part A, 2005, 68, 1301–1307 CrossRef CAS PubMed.
- X. Zhang, X.-M. Zhao, X.-J. Meng, X.-Y. Wang, S. Yang, X.-P. Xu, S.-T. Wang, C. Gu, M.-L. Wang, H. Ren, Z.-Y. Zhang, G.-X. Yan, Z.-G. Cao and Y.-S. Wang, Particle Size Distribution and Human Health Risk Assessment of Heavy Metals in Atmospheric Particles from Beijing and Xinxiang During Summer, Huanjing Kexue, 2018, 39, 997–1003 Search PubMed.
- A. J. Adon, C. Liousse, E. T. Doumbia, A. Baeza-Squiban, H. Cachier, J.-F. Léon, V. Yoboue, A. B. Akpo, C. Galy-Lacaux, C. Zoutien, H. Xu, E. Gardrat and S. Keita, Physico-chemical characterization of urban aerosols from specific combustion sources in West Africa at Abidjan in Côte d'Ivoire and Cotonou in Benin in the frame of DACCIWA program, Atmos. Chem. Phys., 2020, 1–69 Search PubMed.
- A. Saffari, N. Daher, M. M. Shafer, J. J. Schauer and C. Sioutas, Seasonal and spatial variation in dithiothreitol (DTT) activity of quasi-ultrafine particles in the Los Angeles Basin and its association with chemical species, J. Environ. Sci. Health Part A, 2014, 49, 441–451 CrossRef CAS PubMed.
- K. S. Kouassi, S. Billet, G. Garçon, A. Verdin, A. Diouf, F. Cazier, J. Djaman, D. Courcot and P. Shirali, Oxidative damage induced in A549 cells by physically and chemically characterized air particulate matter (PM 2.5) collected in Abidjan, Côte d’Ivoire, J. Appl. Toxicol., 2009, 310–320 Search PubMed.
- D. Dieme, M. Cabral-Ndior, G. Garçon, A. Verdin, S. Billet, F. Cazier, D. Courcot, A. Diouf and P. Shirali, Relationship between physicochemical characterization and toxicity of fine particulate matter (PM2.5) collected in Dakar city (Senegal), Environ. Res., 2012, 113, 1–13 CrossRef CAS PubMed.
- S. Val, C. Liousse, E. H. T. Doumbia, C. Galy-Lacaux, H. Cachier, N. Marchand, A. Badel, E. Gardrat, A. Sylvestre and A. Baeza-Squiban, Physico-chemical characterization of African urban aerosols (Bamako in Mali and Dakar in Senegal) and their toxic effects in human bronchial epithelial cells: description of a worrying situation, Part. Fibre Toxicol., 2013, 10, 10 CrossRef CAS PubMed.
- T. Doumbia, C. Liousse, M.-R. Ouafo-Leumbe, S. A. Ndiaye, E. Gardrat, C. Galy-Lacaux, C. Zouiten, V. Yoboué and C. Granier, Source Apportionment of Ambient Particulate Matter (PM) in Two Western African Urban Sites (Dakar in Senegal and Bamako in Mali), Atmosphere, 2023, 14, 684 CrossRef CAS.
- Y. Luo, Y. Zeng, H. Xu, D. Li, T. Zhang, Y. Lei, S. Huang and Z. Shen, Connecting oxidative potential with organic carbon molecule composition and source-specific apportionment in PM2.5 in Xi’an, China, Atmos. Environ., 2023, 306, 119808 CrossRef CAS.
- Z. Luo, L. Zhang, G. Li, W. Du, Y. Chen, H. Cheng, S. Tao and G. Shen, Evaluating co-emissions
into indoor and outdoor air of EC, OC, and BC from in-home biomass burning, Atmos. Res., 2021, 248, 105247 CrossRef CAS.
- S. Keita, C. Liousse, E.-M. Assamoi, T. Doumbia, E. T. N'Datchoh, S. Gnamien, N. Elguindi, C. Granier and V. Yoboué, African anthropogenic emissions inventory for gases and particles from 1990 to 2015, Earth Syst. Sci. Data, 2021, 13, 3691–3705 CrossRef.
-
R. F. K. Andih, Urbanisation de la Côte d'Ivoire : analyse spatiale de la dynamique urbaine des origines à nos jours, DaloGéo Search PubMed.
-
AGEROUTE, Etendue du Réseau Routier Ivoirien, https://ageroute.ci/index.php/gestion-du-reseau/reseau-routier/repartition-cartographie, (accessed 14 March 2020) Search PubMed.
-
LCSQA, Evolution de la classification et des critères d’implantation des stations de mesure de la qualité de l’air - participation a la réactualisation du guide de classification des stations, Laboratoire Central de Surveillance de la Qualité de l'Air, Ecole Des Mines De Douai Departement Chimie Et Environnement, 2010 Search PubMed.
-
J. Djossou, Analyse de la pollution atmosphérique en zones urbaines en Côte d’Ivoire et au Bénin dans le cadre du programme DACCIWA : Pollution particulaire, University of Abomey-Calavi, Benin, 2018.
- J. Djossou, J.-F. Léon, A. B. Akpo, C. Liousse, V. Yoboué, M. Bedou, M. Bodjrenou, C. Chiron, C. Galy-Lacaux, E. Gardrat, M. Abbey, S. Keita, J. Bahino, E. Touré N'Datchoh, M. Ossohou and C. N. Awanou, Mass concentration, optical depth and carbon composition of particulate matter in the major southern West African cities of Cotonou (Benin) and Abidjan (Côte d'Ivoire), Atmos. Chem. Phys., 2018, 18, 6275–6291 CrossRef CAS.
- J. Bahino, V. Yoboué, C. Galy-Lacaux, M. Adon, A. Akpo, S. Keita, C. Liousse, E. Gardrat, C. Chiron, M. Ossohou, S. Gnamien and J. Djossou, A pilot study of gaseous pollutants' measurement (NO2, SO2, NH3, HNO3 and O3) in Abidjan, Côte d'Ivoire: contribution to an overview of gaseous pollution in African cities, Atmos. Chem. Phys., 2018, 18, 5173–5198 CrossRef CAS.
- S. Keita, C. Liousse, V. Yoboué, P. Dominutti, B. Guinot, E.-M. Assamoi, A. Borbon, S. L. Haslett, L. Bouvier, A. Colomb, H. Coe, A. Akpo, J. Adon, J. Bahino, M. Doumbia, J. Djossou, C. Galy-Lacaux, E. Gardrat, S. Gnamien, J. F. Léon, M. Ossohou, E. T. N'Datchoh and L. Roblou, Particle and VOC emission factor measurements for anthropogenic sources in West Africa, Atmos. Chem. Phys., 2018, 18, 7691–7708 CrossRef CAS.
- S. Gnamien, V. Yoboué, C. Liousse, M. Ossohou, S. Keita, J. Bahino, S. Siélé and L. Diaby, Particulate Pollution in Korhogo and Abidjan (Cote d’Ivoire) during the Dry Season, Aerosol Air Qual. Res., 2020, 20, 1–19 CrossRef.
- H. Cachier, M.-P. Bremond and P. Buat-Ménard, Determination of atmospheric soot carbon with a simple thermal method, Tellus B, 1989, 41, 379–390 CrossRef.
- M. Adon, C. Galy-Lacaux, V. Yoboué, C. Delon, J. P. Lacaux, P. Castera, E. Gardrat, J. Pienaar, H. Al Ourabi and D. Laouali,
et al., Long term measurements of sulfur dioxide, nitrogen dioxide, ammonia, nitric acid and ozone in Africa using passive samplers, Atmos. Chem. Phys., 2010, 10, 7467–7487 CrossRef CAS.
-
M. Ossohou, Etude des tendances des concentrations atmosphériques de gaz azotés en Afrique - Bilan de dépots atmosphériques secs et humides d'azote de l'écosystème de savane humide de Lamto (Côte d'ivoire), Université Félix Houphouët-Boigny, 2020.
- K. Pearson, On Lines and Planes of Closest Fit to Systems of Points in Space, Philos. Mag., 1901, 2, 559–572 Search PubMed.
- P. K. Hopke, An introduction to receptor modeling, Chemom. Intell. Lab. Syst., 1991, 10, 21–43 CrossRef CAS.
- P. Paatero and U. Tapper, Positive matrix factorization: A non-negative factor model with optimal utilization of error estimates of data values, Environmetrics, 1994, 5, 111–126 CrossRef.
- P. Anttila, P. Paatero, U. Tapper and O. Jarvinen, Source identification of bulk wet deposition in Finland by positive matrix factorization, Atmos. Environ., 1995, 29, 1705–1718 CrossRef CAS.
- M. Xie, R. Piedrahita, S. J. Dutton, J. B. Milford, J. G. Hemann, J. L. Peel, S. L. Miller, S.-Y. Kim, S. Vedal, L. Sheppard and M. P. Hannigan, Positive matrix factorization of a 32-month series of daily PM2.5 speciation data with incorporation of temperature stratification, Atmos. Environ., 2013, 65, 11–20 CrossRef CAS PubMed.
- B. R. Larsen, S. Gilardoni, K. Stenström, J. Niedzialek, J. Jimenez and C. A. Belis, Sources for PM air pollution in the Po Plain, Italy: II. Probabilistic uncertainty characterization and sensitivity analysis of secondary and primary sources, Atmos. Environ., 2012, 50, 203–213 CrossRef CAS.
-
A. Albinet and J. Balbiani, Surveillance des HAP – Mise en œuvre d’une méthodologie d’estimation des sources de HAP par modèle récepteur. Application de la Positive Matrix Factorization (PMF), Laboratoire Central de Surveillance de la Qualité de l'Air, France, 2013 Search PubMed.
- P. Paatero, Least squares formulation of robust non-negative factor analysis, Chemom. Intell. Lab. Syst., 1997, 37, 23–35 CrossRef CAS.
- B. Zhang, L. Jiao, G. Xu, S. Zhao, X. Tang, Y. Zhou and C. Gong, Influences of wind and precipitation on different-sized particulate matter concentrations (PM2.5, PM10, PM2.5–10), Meteorol. Atmos. Phys., 2018, 130, 383–392 CrossRef.
-
WHO, Lignes directrices OMS relatives à la qualité de l’air : particules, ozone, dioxyde d’azote et dioxyde de soufre, Organisation mondiale de la Santé, 2021 Search PubMed.
- A. Satsangi, T. Pachauri, V. Singla, A. Lakhani and K. M. Kumari, Indian J. Radio Space Phys., 2010, 39, 218–222 CAS.
- I. El Haddad, N. Marchand, J. Dron, B. Temime-Roussel, E. Quivet, H. Wortham, J. L. Jaffrezo, C. Baduel, D. Voisin, J. L. Besombes and G. Gille, Comprehensive primary particulate organic characterization of vehicular exhaust emissions in France, Atmos. Environ., 2009, 43, 6190–6198 CrossRef CAS.
- C. Hung-Lung and H. Yao-Sheng, Particulate matter emissions from on-road vehicles in a freeway tunnel study, Atmos. Environ., 2009, 43, 4014–4022 CrossRef.
- J. A. Gillies, A. W. Gertler, J. C. Sagebiel and W. A. Dippel, On-Road Particulate Matter (PM 2.5 and PM 10) Emissions in the Sepulveda Tunnel, Los Angeles, California, Environ. Sci. Technol., 2001, 35, 1054–1063 CrossRef CAS PubMed.
- X. Li, S. Wang, L. Duan, J. Hao and Y. Nie, Carbonaceous Aerosol Emissions from Household Biofuel Combustion in China, Environ. Sci. Technol., 2009, 43, 6076–6081 CrossRef CAS PubMed.
- J. Tian, H. Ni, J. Cao, Y. Han, Q. Wang, X. Wang, L.-W. A. Chen, J. C. Chow, J. G. Watson, C. Wei, J. Sun, T. Zhang and R. Huang, Characteristics of carbonaceous particles from residential coal combustion and agricultural biomass burning in China, Atmos. Pollut. Res., 2017, 8, 521–527 CrossRef.
- G. Cao, X. Zhang, S. Gong and F. Zheng, Investigation on emission factors of particulate matter and gaseous pollutants from crop residue burning, J. Environ. Sci., 2008, 20, 50–55 CrossRef CAS PubMed.
- K. L. Dionisio, M. S. Rooney, R. E. Arku, A. B. Friedman, A. F. Hughes, J. Vallarino, S. Agyei-Mensah, J. D. Spengler and M. Ezzati, Within-Neighborhood Patterns and Sources of Particle Pollution: Mobile Monitoring and Geographic Information System Analysis in Four Communities in Accra, Ghana, Environ. Health Perspect., 2010, 118, 607–613 CrossRef CAS PubMed.
- R. E. Arku, J. Vallarino, K. L. Dionisio, R. Willis, H. Choi, J. G. Wilson, C. Hemphill, S. Agyei-Mensah, J. D. Spengler and M. Ezzati, Characterizing air pollution in two low-income neighborhoods in Accra, Ghana, Sci. Total Environ., 2008, 402, 217–231 CrossRef CAS PubMed.
- J. Boman, J. Lindén, S. Thorsson, B. Holmer and I. Eliasson, A tentative study of urban and suburban fine particles (PM2.5) collected in Ouagadougou, Burkina Faso, Xray Spectrom, 2009, 38, 354–362 CrossRef CAS.
-
E. H. T. Doumbia, Caractérisation physico-chimique de la pollution atmosphérique urbaine en Afrique de l'Ouest et étude d'impact sur la santé, Université Toulouse III – Paul Sabatier, 2012 Search PubMed.
- V. H. Garrison, M. S. Majewski, L. Konde, R. E. Wolf, R. D. Otto and Y. Tsuneoka, Inhalable desert dust, urban emissions, and potentially biotoxic metals in urban Saharan–Sahelian air, Sci. Total Environ., 2014, 500–501, 383–394 CrossRef CAS PubMed.
- Y. Xie, H. Lu, A. Yi, Z. Zhang, N. Zheng, X. Fang and H. Xiao, Characterization and source analysis of water–soluble ions in PM2.5 at a background site in Central China, Atmos. Res., 2020, 239, 104881 CrossRef CAS.
- D. Temesi, A. Molnár, E. Mészáros, T. Feczkó, A. Gelencsér, G. Kiss and Z. Krivácsy, Size resolved chemical mass balance of aerosol particles over rural Hungary, Atmos. Environ., 2001, 35, 4347–4355 CrossRef CAS.
- W. Maenhaut, N. Raes, X. Chi, J. Cafmeyer and W. Wang, Chemical composition and mass closure for PM2.5 and PM10 aerosols at K-puszta, Hungary, in summer 2006, X-Ray Spectrom., 2008, 37, 193–197 CrossRef CAS.
- J. Sciare, K. Oikonomou, H. Cachier, N. Mihalopoulos, M. O. Andreae, W. Maenhaut and R. Sarda-Estève, Aerosol mass closure and reconstruction of the light scattering coefficient over the Eastern Mediterranean Sea during the MINOS campaign, Atmos. Chem. Phys., 2005, 5, 2253–2265 CrossRef CAS.
- B. Guinot, H. Cachier and K. Oikonomou, Geochemical perspectives from a new aerosol chemical mass closure, Atmos. Chem. Phys., 2007, 7, 1657–1670 CrossRef CAS.
- J. C. M. Pires, M. C. Pereira, M. C. M. Alvim-Ferraz and F. G. Martins, Identification of redundant air quality measurements through the use of principal component analysis, Atmos. Environ., 2009, 43, 3837–3842 CrossRef CAS.
- N. J. Pekney, C. I. Davidson, A. Robinson, L. Zhou, P. Hopke, D. Eatough and W. F. Rogge, Major Source Categories for PM 2.5 in Pittsburgh using PMF and UNMIX, Aerosol Sci. Technol., 2006, 40, 910–924 CrossRef CAS.
- S. G. Bhanuprasad, C. Venkataraman and M. Bhushan, Positive matrix factorization and trajectory modelling for source identification: A new look at Indian Ocean Experiment ship observations, Atmos. Environ., 2008, 42, 4836–4852 CrossRef CAS.
- I. Gupta, A. Salunkhe and R. Kumar, Source apportionment of PM10 by positive matrix factorization in urban area of Mumbai, India, Sci. World J., 2012, 2012 DOI:10.1100/2012/585791.
-
U.S. EPA, EPA Positive Matrix Factorization (PMF) 5.0 Fundamentals and User Guide, 2014 Search PubMed.
- J. G. Watson, J. C. Chow, D. H. Lowenthal, L.-W. Antony Chen, S. Shaw, E. S. Edgerton and C. L. Blanchard, PM2.5 source apportionment with organic markers in the Southeastern Aerosol Research and Characterization (SEARCH) study, J. Air Waste Manage. Assoc., 2015, 65, 1104–1118 CrossRef CAS PubMed.
- C. Pio, M. Cerqueira, R. M. Harrison, T. Nunes, F. Mirante, C. Alves, C. Oliveira, A. Sanchez de la Campa, B. Artíñano and M. Matos, OC/EC ratio observations in Europe: Re-thinking the approach for apportionment between primary and secondary organic carbon, Atmos. Environ., 2011, 45, 6121–6132 CrossRef CAS.
- J. Sandradewi, A. S. H. Prévôt, S. Szidat, N. Perron, M. R. Alfarra, V. A. Lanz, E. Weingartner and U. Baltensperger, Using Aerosol Light Absorption Measurements for the Quantitative Determination of Wood Burning and Traffic Emission Contributions to Particulate Matter, Environ. Sci. Technol., 2008, 42, 3316–3323 CrossRef CAS PubMed.
- M. I. Manousakas, K. Florou and S. N. Pandis, Source Apportionment of Fine Organic and Inorganic Atmospheric Aerosol in an Urban Background Area in Greece, Atmosphere, 2020, 11, 330 CrossRef CAS.
- C. Samara, D. Voutsa, A. Kouras, K. Eleftheriadis, T. Maggos, D. Saraga and M. Petrakakis, Organic and elemental carbon associated to PM10 and PM2.5 at urban sites of northern Greece, Environ. Sci. Pollut. Res., 2014, 21, 1769–1785 CrossRef CAS PubMed.
- M. Handler, C. Puls, J. Zbiral, I. Marr, H. Puxbaum and A. Limbeck, Size and composition of particulate emissions from motor vehicles in the Kaisermühlen-Tunnel, Vienna, Atmos. Environ., 2008, 42, 2173–2186 CrossRef CAS.
- S. K. Sharma, T. K. Mandal, S. Jain, S. Saraswati, A. Sharma and M. Saxena, Source Apportionment of PM2.5 in Delhi, India Using PMF Model, Bull. Environ. Contam. Toxicol., 2016, 97, 286–293 CrossRef CAS PubMed.
- Y. Wang, G. Zhuang, S. Chen, Z. An and A. Zheng, Characteristics and sources of formic, acetic and oxalic acids in PM2.5 and PM10 aerosols in Beijing, China, Atmos. Res., 2007, 84, 169–181 CrossRef CAS.
- H. Cachier and J. Ducret, Influence of biomass burning on equatorial African rains, Nature, 1991, 352, 228–230 CrossRef.
-
J. C. Chow and J. G. Watson, Guideline on speciated particulate monitoring, 1998 Search PubMed.
- W. F. Rogge, L. M. Hildemann, M. A. Mazurek and G. R. Cass, Sources of Fine Organic Aerosol. 9. Pine, Oak, and Synthetic Log Combustion in Residential Fireplaces, Environ. Sci. Technol., 1998, 32, 13–22 CrossRef CAS.
- P. M. Fine, G. R. Cass and B. R. Simoneit, Chemical characterization of fine particle emissions from the fireplace combustion of wood types grown in the Midwestern and Western United States, Environ. Eng. Sci., 2004, 21, 387–409 CrossRef CAS.
- C. Wu, T. V. Larson, S. Wu, J. Williamson, H. H. Westberg and L.-J. S. Liu, Source apportionment of PM2.5 and selected hazardous air pollutants in Seattle, Sci. Total Environ., 2007, 386, 42–52 CrossRef CAS PubMed.
- P. Pant and R. M. Harrison, Critical review of receptor modelling for particulate matter: A case study of India, Atmos. Environ., 2012, 49, 1–12 CrossRef CAS.
- D. Mooibroek, M. Schaap, E. P. Weijers and R. Hoogerbrugge, Source apportionment and spatial variability of PM2.5 using measurements at five sites in the Netherlands, Atmos. Environ., 2011, 45, 4180–4191 CrossRef CAS.
- I. Kassi-Djodjo, Rôle des transports populaires dans le processus d’urbanisation à Abidjan, Les. Cah. d’Outre-Mer, 2010, 391–402 CrossRef.
- D. Zhang and Y. Iwasaka, Nitrate and sulfate in individual Asian dust-storm particles in Beijing, China in spring of 1995 and 1996, Atmos. Environ., 1999, 33, 3213–3223 CrossRef CAS.
- P. M. Fine, G. R. Cass and B. R. Simoneit, Chemical characterization of fine particle emissions from the fireplace combustion of woods grown in the southern United States, Environ. Sci. Technol., 2002, 36, 1442–1451 CrossRef CAS PubMed.
- J. D. McDonald, B. Zielinska, E. M. Fujita, J. C. Sagebiel, J. C. Chow and J. G. Watson, Fine particle and gaseous emission rates from residential wood combustion, Environ. Sci. Technol., 2000, 34, 2080–2091 CrossRef CAS.
- C. G. Nolte, J. J. Schauer, G. R. Cass and B. R. Simoneit, Highly polar organic compounds present in wood smoke and in the ambient atmosphere, Environ. Sci. Technol., 2001, 35, 1912–1919 CrossRef CAS PubMed.
- G. Lonati, M. Giugliano, P. Butelli, L. Romele and R. Tardivo, Major chemical components of PM2.5 in Milan (Italy), Atmos. Environ., 2005, 39, 1925–1934 CrossRef CAS.
- J.-E. Petit, C. Pallarès, O. Favez, L. Y. Alleman, N. Bonnaire and E. Rivière, Sources and Geographical Origins of PM10 in Metz (France) Using Oxalate as a Marker of Secondary Organic Aerosols by Positive Matrix Factorization Analysis, Atmosphere, 2019, 10, 370 CrossRef CAS.
- A. Benchrif, M. Tahri, B. Guinot, E. M. Chakir, F. Zahry, B. Bagdhad, M. Bounakhla, H. Cachier and F. Costabile, Aerosols in Northern Morocco-2: Chemical Characterization and PMF Source Apportionment of Ambient PM2.5, Atmosphere, 2022, 13, 1701 CrossRef CAS.
- S. Nava, G. Calzolai, M. Chiari, M. Giannoni, F. Giardi, S. Becagli, M. Severi, R. Traversi and F. Lucarelli, Source Apportionment of PM2.5 in Florence (Italy) by PMF Analysis of Aerosol Composition Records, Atmosphere, 2020, 11, 484 CrossRef CAS.
- B. Nyilitya, S. Mureithi, M. Bauters and P. Boeckx, Nitrate source apportionment in the complex Nyando tropical river basin in Kenya, J. Hydrol., 2021, 594, 125926 CrossRef CAS.
- D.-C. Koh, B. Mayer, K.-S. Lee and K.-S. Ko, Land-use controls on sources and fate of nitrate in shallow groundwater of an agricultural area revealed by multiple environmental tracers, J. Contam. Hydrol., 2010, 118, 62–78 CrossRef CAS PubMed.
|
This journal is © The Royal Society of Chemistry 2023 |
Click here to see how this site uses Cookies. View our privacy policy here.