Overcoming the lack of authentic standards for the quantification of biogenic secondary organic aerosol markers†
Received
22nd June 2022
, Accepted 27th November 2022
First published on 14th December 2022
Abstract
Liquid chromatography coupled to electrospray ionisation high resolution mass spectrometry is an extremely powerful technique for both targeted and non-targeted analysis of organic aerosol. However, quantification of biogenic secondary organic aerosol species (BSOA) is hindered by a lack of commercially available authentic standards. To overcome the lack of authentic standards, this study proposes a quantification method based on the prediction of relative ionisation efficiency (RIE) factors to correct concentrations obtained via calibration using a proxy standard. RIE measurements of 89 commercially available standards were made relative to cis-pinonic acid and coupled to structural descriptors. A regularised random forest predictive model was developed using the authentic standards (R2 = 0.66, RMSE = 0.59). The model was then used to predict the RIE’s of 87 biogenic organic acid markers from α-pinene, limonene and β-caryophyllene without available authentic standards. The predicted RIE’s ranged from 0.27 to 13.5, with a mean ± standard deviation of 4.2 ± 3.9. 25 markers were structurally identified in chamber samples and ambient aerosol filter samples collected in summertime Beijing. The markers were quantified using a cis-pinonic acid calibration and then corrected using the predicted RIE factors. This resulted in the average BSOA concentration decreasing from 146 ng m−3 to 51 ng m−3, respectively. This change in concentration is highlighted to have an impact on the types of average aerosol metrics commonly used to describe bulk composition. This study is the first of its kind to use predicted ionisation efficiency factors to overcome known differences in BSOA concentrations due to the inherent lack of authentic standards in aerosol chemistry.
Environmental significance
Organic aerosol is a major contributor to particulate matter concentrations and is made up of an extremely complex mixture of thousands of different compounds. Due to this complexity and an inherent lack of authentic standards, understanding aerosol composition and individual compound concentrations is extremely challenging. Significant differences in ionisation efficiencies have been observed between biogenic secondary organic aerosol compounds, when using electrospray ionisation techniques. These differences lead to significant uncertainties in organic aerosol composition and quantification. A predictive relative ionisation efficiency model allows for these differences to be considered, in turn leading to more reliable composition and concentration results.
|
1 Introduction
PM2.5 (particulate matter less than 2.5 μm in diameter) is considered to be one of the most dangerous forms of air pollution with respect to human health, made up of a complex mixture of different compounds from a range of natural and anthropogenic sources.1 Organic aerosol (OA) makes up a significant portion of PM2.5,2,3 and consists of thousands of individual compounds, comprised of multiple chemical functionalities from a variety of sources.1 A key subset of OA is biogenic secondary organic aerosol (BSOA) which can be formed during the oxidation of biogenic volatile organic compounds (BVOCs) emitted from vegetation.4–9 In less polluted regions BSOA can represent between 23 and 50% of organic aerosol mass,10–14 and has been shown to contribute significantly even in urban areas.15,16
One of the challenges in analysing OA is the number of chemical degradation pathways available for precursor VOCs and the subsequent range of products that can be formed, with one precursor having the potential to create 10 s of different compounds.17,18 High resolution mass spectrometry (HRMS) with electrospray ionisation (ESI) sources have become an extremely versatile technique for improving our understanding of complex environmental samples.19 ESI is a soft ionisation technique allowing for the molecular identification of thousands of individual species.20,21 However a species' ability to be ionised is highly structurally specific,22 meaning the relative contribution of sample is hard to determine. Many previous studies have used direct injection techniques, without prior separation by liquid chromatography.23–26 Direct injection allows for the identification of 1000 s of different molecular formulae within one sample. However, due to a lack of isomer identification, quantification of individual compounds is not possible. Most studies use data visualisation techniques such as van Krevelen and Kendrick mass diagrams, and other chemical metrics such as average O
:
C and H
:
C ratios to draw conclusions about the aerosol composition, ageing and sources.18,21,26–31
However, these chemical metrics are based on signal response, not quantified concentrations, and as such assume all species ionise with equal efficiency. While semi-quantitative information can be obtained for samples of similar chemical speciation, ionisation efficiencies can vastly differ resulting in data bias and misinterpretation as shown by Pereira et al., 2021 and references therein. Targeted analysis and quantification using authentic standards overcomes these issues. However, due to the sheer number of compounds present in OA and inherent lack of authentic standards, proxy standards are routinely used where equivalent analyte ionisation efficiencies are assumed.32,33,70 However, the use of proxy standards still assumes all species in one functionality group or retention time window have the same ionisation efficiency.
Ideally, all species would have their own authentic standard for quantification, and recently groups have started to synthesise compounds such as organosulfates from isoprene and monoterpenes,34–37 nitrooxy organosulfates from monoterpenes38 and organic acids from a range of monoterpenes.39–42 Kenseth et al., 2020 recently synthesised 6 α-pinene derived carboxylic and dimer ester species and found large differences between their ionisation efficiencies.39 The measured relative response (RF) to cis-pinonic acid ranged from 0.46 to 35.65. The large differences in response factors observed by this study highlight the need to consider these differences in the quantification of species with similar functionalities and retention time windows. However, two issues arise with this approach; firstly, the time and expenses to synthesise different standards limits the work to larger laboratories with synthesis facilities, and secondly the sheer number of standards that would need to be synthesised for the hundreds of identified compounds makes this approach impractical.
A species' ability to ionise in the ESI source, both in the negative and positive modes, is highly dependent on its functionality and structure as well as the ionisation conditions.22,43,44 This has led to the development of models that can predict how well a compound can ionise based on structural descriptors or properties relative to a standard compound.43,45–47 The RF, i.e. how well a compound ionises in comparison to a reference compound, is calculated as shown in eqn (1), where log
RF(C1,C2) is the log value of the ratio of the gradients for compounds C1 and C2 across a concentration–response curve.43,46
| 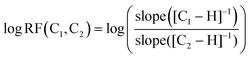 | (1) |
This type of RF scale has been used to investigate the structural or chemical features which affect a species' ability to ionise in the ESI. Early studies focussed on measured or calculated physical properties such as log
P or pKa.48–52 Recent studies have focussed on using computationally calculated molecular fingerprints or structural descriptors to assess and predict a species RF.46,47 Mayhew et al., 2020 measured RF's of 51 carboxylic acids which combined with structural fingerprints were used to develop a Bayesian ridge regression model.47 The model showed R2 and RMSE values in line with comparable studies, without the need to measure or predict physical properties of compounds. Liigand et al., 2020 recently developed a predictive machine learning model, which can predict the RF's of species relative to benzoic acid based on their structure, both in the positive and negative ionisation modes across a range of solvent compositions.46 Their model used data collected over a decade and contains RF measurements of 3139 and 1286 compound–solvent combinations in the positive and negative modes respectively. Previously, to our knowledge only one study has predicted RF factors for BSOA species.53 Zhang et al., 2015 estimated the RF's of a range of α-pinene derived organic acids54 based on a linear model developed by Kruve et al., 2014.45 The predicted RF's ranged from 0.54 to 51.64, with dimer species such as C16H26O6 having the largest predicted RF values, in-line with the observations in Kenseth et al., 2020.
In this study we aim to establish an RF model and apply it to improving our ability to reliably quantify BSOA markers. RF measurements of 89 authentic organic compounds relative to cis-pinonic acid were conducted in the negative ionisation mode. These measurements were then coupled to predicted, easily obtained chemical descriptors of molecular structure from ChemDes55 as well as pKa and log
P values to develop a random forest model for the prediction of BSOA RF factors. RF's were then predicted for previously identified BSOA markers which were used to correct concentrations calculated from a proxy cis-pinonic acid calibration of ambient samples collected in summertime Beijing. Overall, this study is the first to apply a method for the prediction of BSOA ESI response factors based on RF measurements, and as such provides a basis for future studies to establish more reliable quantification methods.
2 Materials and methods
2.1 Instrument and data analysis
Samples were analysed using an Ultimate 3000 ultra-high pressure liquid chromatography system (UHPLC, Thermo Scientific, USA) coupled to a Q Exactive Orbitrap MS (Thermo Fisher Scientific, USA) using data dependent tandem mass spectrometry (ddMS2) with heated electrospray ionization source (HESI). The UHPLC method uses a reverse phase, 2.6 μm, 100 × 2.1 mm, Accucore column (Thermo Scientific, UK), held at 40 °C. The mobile phase consisted of water (A) and methanol (B) both Optima grade (Thermo Fisher Scientific) with 0.1% (v/v) of formic acid (98% purity, Acros Organics). The injection volume was 4 μL. The solvent gradient was held for one minute at 90
:
10H2O
:
MeOH, then changed linearly to 10
:
90 over 24 minutes, returning to 90
:
10 over 2 minutes and then held for 2 minutes, with a flow rate of 300 μL min−1. The MS was operated in negative mode, using full scan data dependant MS2. The scan range was set between 50 and 750 m/z, with a mass resolution of 120
000. The capillary and auxiliary gas temperatures were 320 °C. The sheath and auxiliary gas flow rates were 45 arb. and 10 arb respectively. The spray voltage was set to 4 kV. The number of most abundant precursors for MS2 fragmentation was set to 10. Data was analysed using TraceFinder 4.1 General Quan software (Thermo Fisher Scientific) using a targeted compound library of both standards and BSOA species, with a mass accuracy of 3 ppm for marker identification. All isotopic peaks were corrected with the theoretical isotope correction factor within the software.
2.2 Commercially available standards
RF measurements of 89 authentic standards relative to cis-pinonic acid were conducted, as shown in Table S1.† All commercial standards were of high purity (>95%) to reduce the effect of purity on measured RF values. The standards were prepared in mixtures of 50
:
50 MeOH
:
H2O, where no compound had the same retention time (RT) in order to reduce matrix effects which would affect the measured RF. The mixtures were prepared across a 7-point concentration gradient (5, 2.5, 1, 0.5, 0.25, 0.125, 0.0625 ppm, R2 > 0.95), with 3 replicate measurements per concentration. However, some compounds reached limit of detection before the lowest concentration. A 9-point cis-pinonic acid calibration was run alongside the ambient PM2.5 samples which was used for quantification (R2 > 0.99). 35 compounds were common between this study and that conducted in Mayhew et al., 2020, using the same mass spectrometer but via direct infusion. The 35 compounds showed a high correlation (R2 = 0.83) across the two methods, with an average difference in the measured log
RF's of 0.24 ± 0.42, highlighting the reliability of these measurements. The errors in the measured log
RF values were small, on average 3.6% across the 89 standards based on the standard error of the calibration slopes.
2.3 Pinic acid synthesis
Pinic acid (purity > 99%) was synthesised adapted from the method outlined by Kenseth et al., 2020. The full experimental procedure is given in the ESI.†
2.4 Chamber samples
To identify the retention time of BSOA tracers from specific precursors, BSOA was generated from α-pinene, limonene and β-caryophyllene using an aerosol flow reactor as outlined in Table 1 of Pereira et al., 2019.56 The generated BSOA was collected using an electrical low-pressure impactor onto foil-lined impactor plates and dissolved in 50
:
50 MeOH
:
H2O (optima, LC-MS grade, Fisher Scientific, UK). Individual α-pinene markers were isolated and collected based on their retention times from generated BSOA mass using a HPLC-ion-trap mass spectrometer coupled to an automated fraction collector, using the method described in Finessi et al., 2014.57
Table 1 Comparison between marker concentrations quantified by a standard cis-pinonic acid calibration and corrected by the predicted RF's in Table S3. cis-Pinonic acid is Pinene_183
Tag |
Molecular formula |
PA concentration (ng m−3) |
RF concentration (ng m−3) |
Lim_173a |
C7H10O5 |
71.9 |
14.4 |
Lim_187a |
C8H12O5 |
39.8 |
7.8 |
Pinene_185a |
C9H14O4 |
10.4 |
1.4 |
Pinene_183 |
C10H16O3 |
8.9 |
8.8 |
Bcary_253b |
C14H22O4 |
5.4 |
0.4 |
Bcary_197 |
C11H18O3 |
3.2 |
5.9 |
Lim_183 |
C10H16O3 |
2.3 |
8.4 |
Pinene_171a |
C8H12O4 |
2.1 |
3.6 |
Bcary_255a |
C13H20O5 |
2.0 |
0.2 |
Total |
|
146.0 |
51.0 |
2.5 Ambient samples: collection and extraction
Ambient PM2.5 samples were collected in the summer of 2017 (24/05/2017–30/05/2017) at the Institute of Atmospheric Physics (IAP) in Beijing, China (Table S4†). This sampling was part of the Sources and Emissions of Air Pollutants in Beijing (AIRPOLL-Beijing) project, as part of the wider Atmospheric Pollution and Human Health in a Chinese Megacity (APHH-Beijing) programme.57 Quartz filters (Whatman QMA, 10′′ by 8′′) were pre-baked at 500 °C for 5 hours and wrapped in foil before use. The samples were collected using a HiVol sampler at a flow rate of 1.33 m3 min−1. The samples were then stored at −20 °C before use. A 38.44 cm2 cutting was taken from the filter and cut into roughly 1 cm2 pieces. 8 mL of MeOH (Optima LC-MS grade) was then added to the filter pieces and sonicated for 45 min under ice. The extract was then removed and filtered through a 0.22 μm syringe filter (Millipore) into a new vial. 2 mL (2 × 1 mL) of MeOH was then added to the filter pieces and extracted through the 0.22 μm filter and combined with the rest of the extract. The combined extract was then reduced to near dryness using a solvent evaporator, before being reconstituted in 50
:
50 MeOH
:
H2O. Triplicate recovery tests showed an almost complete recovery of cis-pinonic acid (99 ± 15.6%, n = 3) from the filter.
2.6 Model development
Commercially available authentic standards were analysed alongside cis-pinonic acid within mixtures for RF calculation and to allow correction for inter-day variability in instrument sensitivity. All standards contained a carboxylic acid or alcohol functional group but spanned a wide range of structures and other functionalities. The species eluted across a wide range of retention times, from highly polar species such as malic acid eluting within the first minute, to less polar species such as dodecanoic acid eluting at the end of the elution gradient. cis-Pinonic acid, the reference compound eluted around 8 minutes. The gradient of calibration curves of the 89 standards were determined by linear regression. The calculated log
RF values of the species, given in Table S1† ranged from −2.84 to 1.75, covering four orders of magnitude. Several basic parameters were correlated with the measured log
RF including mass, RT, number of carbon and oxygen atoms as well as the O
:
C and H
:
C ratios, however no correlation (R < 0.1) was observed. On average, the lowest RF values were observed for species eluting before 6 minutes, and after 15 minutes, with the highest between 9 and 12 minutes. Matrix effects were investigated using the same method as in Bryant et al., 2021 using cis-pinonic acid to determine if signal suppression was occurring due to the highly complex nature of the samples.32 However, no significant matrix effect was observed for cis-pinonic acid, but further work is required for a range of different acid species. These measured log
RF values were then combined with over 3000 predicted chemical structural descriptors predicted from the ChemDes platform for computing molecular descriptors and fingerprints (molecular descriptors were taken from the Chemopy, CDK, RDKit, Pybel and PaDEL open-source packages).55
Several data cleaning steps were undertaken before model development. Firstly, non-numeric descriptors and descriptors containing only one value were removed resulting in 1766 descriptors. Descriptors with a pairwise correlation greater than R2 = 0.8 were then removed, in-line with previous studies,46 resulting in 224 descriptors using the “findCorrelation” function from the Caret R package.58 The remaining descriptors were then correlated to the log
RF values of the standards, and those with an R greater than 0.3 were selected (Table S2†). Two descriptors were removed (“fr_nitro_arom_nonortho” and “fr_phenol”), due to their lack of applicability to functionalities of the organic acid markers being studied here. pKa and log
P were also predicted using ChemDraw Prime 18.1 software, based on previous studies highlighting their importance to the ionisation efficiencies of compounds.47 The pKa had a correlation of R = 0.32 towards log
RF, but limited correlations were observed for log
P (R < 0.1), however a more accurate model was obtained with the inclusion of log
P. The predicted pKa and log
P values were combined with the remaining descriptors, giving 18 descriptors for model development. Several predictive models were developed using the Caret R package58 including random forest, Bayesian ridge regression and linear regression, with regularised random forest (RRF) being the best performing based on the lowest RMSE. Regularised random forest models work in the same way as random forest models but reduce model complexity by disregarding features that share information. The number of trees used in the random forest was optimised to 100 trees, and mtry (the number of variables available for splitting at each tree node) was optimised to 10. The RMSE and R2 values were calculated by default by the built-in functionality of the Caret R package. The final model was chosen based on minimising the RMSE.
Owing to the small dataset size, leave one out cross validation (LOOCV) was used to test the predictive capabilities of the model. LOOCV uses each compound in the data set once as a test set, with the other (n − 1) compounds as the training set. Full details of the model development and the dataset containing the predicted descriptors can be found at https://github.com/djb96/Response_factor_model.
As shown in Table 2, the 18 descriptors for model development were those of structural descriptors surrounding acidity and polarisation. Of the 18 descriptors, the most influential descriptors were MLFER_A and SpMAD_Dzp. MLFER_A provides a description of the overall solute hydrogen bond acidity and SpMAD_Dzp is a measure of a compound's polarizability. These specific descriptors were not identified as important in Liigand et al., 2020, but other descriptors for acidity/basicity were.
Table 2 Comparison of average aerosol metrics weighted by the number of markers, cis-pinonic acid calibration derived concentrations and RF corrected concentrations. O
:
C – oxygen to carbon ration, H
:
C – hydrogen to carbon ratio, DBE – double bond equivalent, C – number of carbons, H = number of hydrogens, O – number of oxygens, MF – average molecular formula
Metric |
Number |
cis-Pinonic acid calibration |
RF calibration |
O : C |
0.43 |
0.61 |
0.48 |
H : C |
1.55 |
1.48 |
1.53 |
DBE |
3.22 |
3.05 |
3.01 |
C |
10 |
8.1 |
8.84 |
H |
15.6 |
12.1 |
13.65 |
O |
4.0 |
4.7 |
4.0 |
MF |
C10H15.6O4 |
C8.1H12.1O4.7 |
C8.8H13.7O4.0 |
3 Results and discussion
3.1 Development of a RF predictive model
Fig. 1 shows the correlation between the measured and predicted log
RF values for the 89 readily available standards. The optimised model shows similar accuracy and linearity to previous studies43,45–47,59,60 with an R2 of 0.66 and RMSE of 0.59. The RMSE error means that if compound A is predicted to have an RF 10 times higher (log
RF = 1) than cis-pinonic acid (log
RF = 0), the actual RF would be in the range 2.6–38.9 (log
RF = 1.0 ± 0.59). Overall, the model performed similarly to previous studies, although starts to perform poorly for compounds with log
RF's less than −1, as seen previously, likely due to the lack of observations.46,47 Further work is needed to increase the RF measurement database for more accurate model development and optimisation.
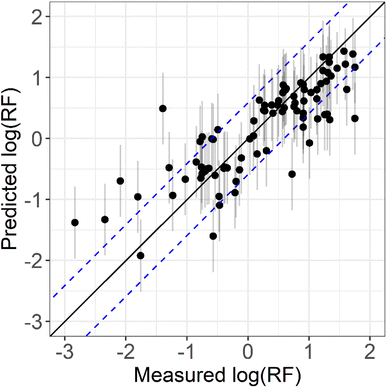 |
| Fig. 1 Comparison between measured log RF (log RFM) and predicted log RF (log1 RFp) produced by a RRF model. All log RFM and log RFP values are given in Table S1† for the standards used in this study. The solid black line is 1 : 1 i.e. would represent perfect predictions of the measured values. The blue dotted lines represent 2× RMSE from the 1 : 1 line. The grey vertical lines represent predicted log RF ± RMSE. | |
Liigand et al., 2020 has previously developed this type of machine learning quantitative ESI-LC-MS approach, using to date, the largest compiled dataset of RF measurements. This is a complex dataset, spanning an array of different solvent compositions, ionisation modes and instruments. The model presented here is the first to predict BSOA RF factors based on an experimentally derived predictive model. The model was built for the purpose of quantifying BSOA compounds in a set solvent mixture, and only on one instrument, meaning the dataset could be less complex. This study therefore highlights a method for quantification of BSOA species without authentic standards, without the need of large datasets which take a long time to accumulate, using commercially available, low-cost standards. This method also negates the need to perform numerous standard calibrations for component quantification, leading to faster throughput of samples. However, more authentic BSOA standards are needed to further develop the model and to validate the predicted values. Due to the lack of commercially available authentic BSOA standards, predicted RF values could not be compared to numerous measured RF's of authentic standards. However, pinic acid was synthesised as part of this study adapted from the procedure developed by Kenseth et al., 2020. The RF of pinic acid was analysed across the same concentration range as part of a mixture with cis-pinonic acid. The measured log
RF of pinic acid was 0.46, considering the purity of the synthesised compound compared to a predicted value of 0.86 ± 0.59 highlighting the relative accuracy of the model and its ability to predict reliable RF values. Further BSOA authentic standards are needed to fully validate the predictive RF model.
Furthermore, Liigand et al., 2020 shows that these models can be transferred between instruments, while each instrument and method would produce a specific RF value for a compound, specific compounds have been shown to be effective at moving the model across instruments. This suggests an aerosol community model could be developed but more work is needed. An open-source database which has now been developed by the Kruve group allowing for large amounts of RF measurements to be compiled across instruments and laboratories.22 This would allow for a generalised RF model to be produced for standardised RF factors of SOA species based on a set of defined authentic standards.
3.2 Predicted BSOA RF factors
Several studies have previously investigated the formation of organic acids in SOA derived from α-pinene, limonene and β-caryophyllene.8,39,53,61–63 Table S3† contains the SMILES formulae of 87 organic acid structures across 60 unique molecular formulae previously proposed in these studies. One of the main challenges of the quantification approach used in this study is the need for structure elucidation. Where multiple isomeric species are present, tandem mass spectrometry data is essential to elucidate chemical structure although similar precursor spectra may still be obtained.
The chemical descriptors for these structures were obtained and using the optimised model described in Section 3.1 and the log
RF values were predicted. The predicted RF's ranged from 0.27 to 13.5, with an average of 4.2 ± 3.9 (mean ± SD). These values are of similar magnitude to those measured by Kenseth et al., 2020 and proposed by Zhang et al., 2015, however are notably smaller.71 Due to the lack of intercomparison studies, the cause of this deviation is unknown, but is likely due to different instrument set ups and analysis. The α-pinene and β-caryophyllene markers had similar average RF values of 5.2 ± 4.0 and 5.6 ± 4.5 respectively, while limonene markers had an average of 2.4 ± 2.3. It should be noted that multiple isomers are likely for most of the markers, however only selected isomers which had previously proposed structures were used in this study. For example, 10 isomeric structures of Lim_199 (C10H16O4) were proposed by Hammes et al., 2019.62 The average RF of these 10 structures was 1.4 ± 1.7, with a range of 0.49–4.80, highlighting the importance of structure confirmation for quantification. The highest predicted RF value for the α-pinene markers was 12.7 for Pinene_353a (C19H30O6) and, Pinene_353b (C20H34O5), both of which are dimer species. This is in-line with high RF values measured via authentic standards of 35.6 and 21.1 for Pinene 353a and 353b respectively by Kenseth et al., 2020. Measured and predicted RIE values were not expected to be the same due to the method specific nature of the values as discussed earlier, but the RF values are in-line with one another.
The species in Table S3† were then targeted in the SOA chamber samples generated from α-pinene, limonene and β-caryophyllene precursors. Most of the α-pinene marker structures were confirmed via comparison to either authentic standards56 or matching product ion mass spectra with previous studies.39,53 Comparatively less MS2 data was available for the limonene markers, with far more isomers identified and structures proposed. Several markers were authenticated via matching MS2 peaks to Witkowski et al., 2017.63 For the β-caryophyllene markers, only one species (β-caryophyllinic acid) was authenticated via MS2. Several of the β-caryophyllene markers identified in the chamber samples only had one previously proposed structure, as such markers with only one isomer were assumed to be the same structure. 25 markers were added into a database containing accurate masses and retention times for targeted identification in the ambient samples.
3.3 Quantification of BSOA in summertime Beijing
To investigate the impact of using these predicted RF factors has on the quantification of BSOA markers in the real atmosphere, 26 markers, including cis-pinonic acid, were targeted in ambient PM2.5 filter extracts collected in summertime Beijing, China in 2017 as part of the APHH campaign.57 Of the 26 targeted markers, 18 were identified in at least one of the samples, while only 9 were identified in more than 40% of the samples, with these used for further analysis (as shown in Table 1 and Fig. 2). These markers were then quantified using a 9-point cis-pinonic acid calibration (R2 > 0.99), with the time averaged concentrations shown in Table 1. The total average concentration of the markers was 146 ng m−3, with cis-pinonic acid contributing on average 6% of the mass. Using this method, Lim_173a (C7H10O5) (Table 1) had the highest predicted concentration, with a mean concentration of 71.8 ng m−3, contributing 49% of the mass. The marker concentrations were then corrected using the predicted RF factors as shown in Fig. 2. The total marker concentration decreased to 51 ng m−3, representing a decrease of a factor of 3 compared to quantification by cis-pinonic acid. The ionisation efficiency of cis-pinonic acid is low compared to many of the other BSOA compounds, most likely because of it having a single isolated carboxylic acid and carbonyl functionalities. This highlights that using cis-pinonic acid as a proxy for quantification can lead to significant overestimations in marker concentrations. Using the RF method, Lim_173a was still the most abundant marker, but now only contributed 28.2% (down from 49%) to the mass, while the contribution from cis-pinonic acid increased to 17.4% up from 6%. This change in contributions could have important implications on conclusions from chamber and ambient studies. For example, Thomsen et al., 2021 identified and quantified organic acids formed from the oxidation of Δ3-carene and α-pinene using proxy standards of cis-pinic acid, cis-pinonic acid and diaterpenylic acid acetate.72 They found large contributions of caric acid from Δ3-carene oxidation and large contributions of dimer species from α-pinene oxidation. These large contributions however could be due to the differing ionisation efficiencies of the markers vs. comparatively lower ionisation efficiencies of the proxy standards.
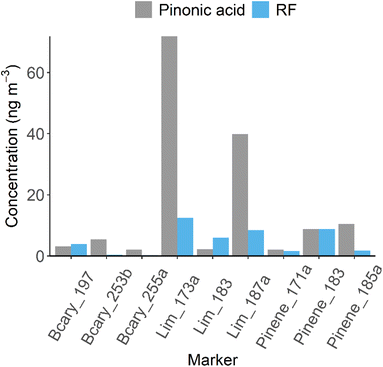 |
| Fig. 2 Concentration comparison for the 9 BSOA species identified in the ambient samples, quantified by cis-pinonic acid, and then “corrected” using the predicted RF's. The concentration of cis-pinonic acid stayed the same due to having an RF of 1 (log RF = 0). | |
High resolution MS studies of aerosol composition generally employ mass spectral data evaluation methods such as Van Krevelen diagrams, double bond equivalents (DBE), average oxidation states and average molecular formulae (MF) based on the number of detected molecular formulae.18,20,27,64 For example, Kundu et al., 2012 investigated the relative abundance of compounds with different O
:
C and H
:
C ratios and found a high abundance of high molecular weight functionalised aliphatic compounds. These relative abundances when corrected for by RF factors could be drastically different to that proposed using the raw signal.65
To investigate the effect of RF factors on these evaluation methods, the hydrogen to carbon (H
:
C) and oxygen to carbon (O
:
C) ratios, DBE and average MF were standardised by number, proxy concentration (i.e. proportional to peak area) and RF corrected concentrations as summarised in Table 2. First, the average O
:
C and H
:
C ratios were calculated for the 9 markers. O
:
C was calculated to be 0.43 based on the number of markers but increased to 0.61 when the average was weighted by the cis-pinonic acid derived concentrations and 0.48 when weighted by the RF corrected concentrations. This is a significant difference considering relatively small differences in O
:
C ratios between different grouped MF based on mass ranges65,66 and different sources.23,26,67–69 A significant shift in average MF was seen when using the number of unique formulae identified (C10H15.6O4), weighted by cis-pinonic acid calibration concentrations (C8.1H12.1O4.7) and weighted by the RF corrected concentrations (C8.8H13.7O4.0). Overall, this shows that even with a small number of markers, the average MF can change, moving from C10 species to C8/C9 depending on the weighting of the average. More work is needed to understand the impact of different RFs when many hundreds of compounds are used to calculate these metrics.
4 Conclusion
This study has outlined a preliminary method for the semi-quantification of multi-functional biogenic organic acid markers using electrospray ionisation, attempting to overcome the lack of commercially available authentic standards. Based on a method developed by Liigand et al., 2020, a predictive random forest model was developed to predict the RF's of a range of atmospherically important BSOA markers. The model was validated by using a synthesised authentic pinic acid standard, with a measured log
RF value of 0.62 compared to the predicted value of 0.86. RF values for the BSOA markers ranged from 0.27 to 13.5, meaning that by using cis-pinonic acid as a proxy calibrant, concentrations of individual BSOA components could be underpredicted by a factor of 3.7 or over predicted by 13.5. Nine BSOA markers, including cis-pinonic acid, were then quantified in 25 ambient Beijing PM2.5 samples. Time averaged quantified compound concentrations decreased from 146.0 ng m−3 to 51 ng m−3 when calibrating using a standard cis-pinonic acid calibration and then correcting using the model predicted RF factors. The effect of these factors was then investigated on common aerosol evaluation methods, with differences in O
:
C ratios of 0.61 vs. 0.48 for cis-pinonic acid calibrated and RF corrected weighted average concentrations. A geometric mean average RF value was calculated to be 4.2 ± 3.9, highlighting the large variability in the predicted RFs, and therefore its lack of reliability if used as a generalised RF. We feel it is important to highlight the issues when assuming a single response factor, whether that be from cis-pinonic acid, or a “corrected” RF. Overall, this study highlights a need to account for the differences in ionisation efficiencies when investigating organic aerosol composition, due to the significant differences in calculated aerosol evaluation metrics, which could influence source contributions. Further work is needed to develop this method to predict RF's without the need of structure elucidation and expand to include newly synthesised organic compounds and the range of functional groups and gas phase precursors. Previous studies have suggested the applicability of transferring the predictive model between instruments, suggesting an open-source aerosol community model could be developed in the future.
Conflicts of interest
There are no conflicts to declare.
References
- M. Hallquist, J. C. Wenger, U. Baltensperger, Y. Rudich, D. Simpson, M. Claeys, J. Dommen, N. M. Donahue, C. George, A. H. Goldstein, J. F. Hamilton, H. Herrmann, T. Hoffmann, Y. Iinuma, M. Jang, M. E. Jenkin, J. L. Jimenez, A. Kiendler-Scharr, W. Maenhaut, G. McFiggans, Th. F. Mentel, A. Monod, A. S. H. Prévôt, J. H. Seinfeld, J. D. Surratt, R. Szmigielski and J. Wildt, Atmos. Chem. Phys., 2009, 9, 5155–5236 CrossRef CAS.
- Q. Zhang, J. L. Jimenez, M. R. Canagaratna, I. M. Ulbrich, N. L. Ng, D. R. Worsnop and Y. Sun, Anal. Bioanal. Chem., 2011, 401, 3045–3067 CrossRef CAS PubMed.
- Y. Sun, W. Xu, Q. Zhang, Q. Jiang, F. Canonaco, A. S. H. Prévôt, P. Fu, J. Li, J. Jayne, D. R. Worsnop and Z. Wang, Atmos. Chem. Phys., 2018, 18, 8469–8489 CrossRef CAS.
- Y. Gómez-González, J. D. Surratt, F. Cuyckens, R. Szmigielski, R. Vermeylen, M. Jaoui, M. Lewandowski, J. H. Offenberg, T. E. Kleindienst, E. O. Edney, F. Blockhuys, C. Van Alsenoy, W. Maenhaut and M. Claeys, J. Mass Spectrom., 2008, 43, 371–382 CrossRef PubMed.
- J. D. Surratt, Y. Gómez-González, A. W. H. Chan, R. Vermeylen, M. Shahgholi, T. E. Kleindienst, E. O. Edney, J. H. Offenberg, M. Lewandowski, M. Jaoui, W. Maenhaut, M. Claeys, R. C. Flagan and J. H. Seinfeld, J. Phys. Chem. A, 2008, 112, 8345–8378 CrossRef CAS PubMed.
- J. D. Surratt, S. M. Murphy, J. H. Kroll, N. L. Ng, L. Hildebrandt, A. Sorooshian, R. Szmigielski, R. Vermeylen, W. Maenhaut, M. Claeys, R. C. Flagan and J. H. Seinfeld, J. Phys. Chem. A, 2006, 110, 9665–9690 CrossRef CAS PubMed.
- Y. Iinuma, O. Böge, A. Kahnt and H. Herrmann, Phys. Chem. Chem. Phys., 2009, 11, 7985–7997 RSC.
- M. N. Chan, J. D. Surratt, A. W. H. Chan, K. Schilling, J. H. Offenberg, M. Lewandowski, E. O. Edney, T. E. Kleindienst, M. Jaoui, E. S. Edgerton, R. L. Tanner, S. L. Shaw, M. Zheng, E. M. Knipping and J. H. Seinfeld, Atmos. Chem. Phys., 2011, 11, 1735–1751 CrossRef CAS.
- M. S. Shalamzari, R. Vermeylen, F. Blockhuys, T. E. Kleindienst, M. Lewandowski, R. Szmigielski, K. J. Rudzinski, G. Spólnik, W. Danikiewicz, W. Maenhaut and M. Claeys, Atmos. Chem. Phys., 2016, 16, 7135–7148 CrossRef CAS.
- N. H. Robinson, J. F. Hamilton, J. D. Allan, B. Langford, D. E. Oram, Q. Chen, K. Docherty, D. K. Farmer, J. L. Jimenez, M. W. Ward, C. N. Hewitt, M. H. Barley, M. E. Jenkin, A. R. Rickard, S. T. Martin, G. McFiggans and H. Coe, Atmos. Chem. Phys., 2011, 11, 1039–1050 CrossRef CAS.
- Q. Chen, D. K. Farmer, L. V. Rizzo, T. Pauliquevis, M. Kuwata, T. G. Karl, A. Guenther, J. D. Allan, H. Coe, M. O. Andreae, U. Pöschl, J. L. Jimenez, P. Artaxo and S. T. Martin, Atmos. Chem. Phys., 2015, 15, 3687–3701 CrossRef CAS.
- S. H. Budisulistiorini, M. R. Canagaratna, P. L. Croteau, W. J. Marth, K. Baumann, E. S. Edgerton, S. L. Shaw, E. M. Knipping, D. R. Worsnop, J. T. Jayne, A. Gold and J. D. Surratt, Environ. Sci. Technol., 2013, 47, 5686–5694 CrossRef CAS PubMed.
- S. H. Budisulistiorini, X. Li, S. T. Bairai, J. Renfro, Y. Liu, Y. J. Liu, K. A. McKinney, S. T. Martin, V. F. McNeill, H. O. T. Pye, A. Nenes, M. E. Neff, E. A. Stone, S. Mueller, C. Knote, S. L. Shaw, Z. Zhang, A. Gold and J. D. Surratt, Atmos. Chem. Phys., 2015, 15, 8871–8888 CrossRef CAS.
- W. Rattanavaraha, K. Chu, S. H. Budisulistiorini, M. Riva, Y.-H. Lin, E. S. Edgerton, K. Baumann, S. L. Shaw, H. Guo, L. King, R. J. Weber, M. E. Neff, E. A. Stone, J. H. Offenberg, Z. Zhang, A. Gold and J. D. Surratt, Atmos. Chem. Phys., 2016, 16, 4897–4914 CrossRef CAS PubMed.
- X. Ding, Q.-F. He, R.-Q. Shen, Q.-Q. Yu and X.-M. Wang, J. Geophys. Res.: Atmos., 2014, 119, 11877–11891 CrossRef CAS.
- Q. Wang, X. He, X. H. H. Huang, S. M. Griffith, Y. Feng, T. Zhang, Q. Zhang, D. Wu and J. Z. Yu, ACS Earth Space Chem., 2017, 1, 562–571 CrossRef CAS.
- P. O. Wennberg, K. H. Bates, J. D. Crounse, L. G. Dodson, R. C. McVay, L. A. Mertens, T. B. Nguyen, E. Praske, R. H. Schwantes, M. D. Smarte, J. M. St Clair, A. P. Teng, X. Zhang and J. H. Seinfeld, Chem. Rev., 2018, 118, 3337–3390 CrossRef CAS PubMed.
- I. Kourtchev, J.-F. Doussin, C. Giorio, B. Mahon, E. M. Wilson, N. Maurin, E. Pangui, D. S. Venables, J. C. Wenger and M. Kalberer, Atmos. Chem. Phys., 2015, 15, 5683–5695 CrossRef CAS.
- M. Glasius and A. H. Goldstein, Environ. Sci. Technol., 2016, 50, 2754–2764 CrossRef CAS PubMed.
- I. Kourtchev, R. H. M. Godoi, S. Connors, J. G. Levine, A. T. Archibald, A. F. L. Godoi, S. L. Paralovo, C. G. G. Barbosa, R. A. F. Souza, A. O. Manzi, R. Seco, S. Sjostedt, J. H. Park, A. Guenther, S. Kim, J. Smith, S. T. Martin and M. Kalberer, Atmos. Chem. Phys., 2016, 16, 11899–11913 CrossRef CAS.
- K. R. Daellenbach, I. Kourtchev, A. L. Vogel, E. A. Bruns, J. Jiang, T. Petäjä, J.-L. Jaffrezo, S. Aksoyoglu, M. Kalberer, U. Baltensperger, I. El Haddad and A. S. H. Prévôt, Atmos. Chem. Phys., 2019, 19, 5973–5991 CrossRef CAS.
- P. Liigand, J. Liigand, K. Kaupmees and A. Kruve, Anal. Chim. Acta, 2021, 1152, 238117 CrossRef CAS PubMed.
- S. S. Steimer, D. J. Patton, T. V. Vu, M. Panagi, P. S. Monks, R. M. Harrison, Z. L. Fleming, Z. Shi and M. Kalberer, Atmos. Chem. Phys., 2020, 20, 13303–13318 CrossRef CAS.
- C. Giorio, C. Bortolini, I. Kourtchev, A. Tapparo, S. Bogialli and M. Kalberer, Chemosphere, 2019, 224, 786–795 CrossRef CAS PubMed.
- J. Cai, X. Zeng, G. Zhi, S. Gligorovski, G. Sheng, Z. Yu, X. Wang and P. Peng, Atmos. Chem. Phys., 2020, 20, 6115–6128 CrossRef CAS.
- I. Kourtchev, S. J. Fuller, C. Giorio, R. M. Healy, E. Wilson, I. O'Connor, J. C. Wenger, M. McLeod, J. Aalto, T. M. Ruuskanen, W. Maenhaut, R. Jones, D. S. Venables, J. R. Sodeau, M. Kulmala and M. Kalberer, Atmos. Chem. Phys., 2014, 14, 2155–2167 CrossRef.
- I. Kourtchev, I. P. O'Connor, C. Giorio, S. J. Fuller, K. Kristensen, W. Maenhaut, J. C. Wenger, J. R. Sodeau, M. Glasius and M. Kalberer, Atmos. Environ., 2014, 89, 525–532 CrossRef CAS.
- X. K. Wang, S. Rossignol, Y. Ma, L. Yao, M. Y. Wang, J. M. Chen, C. George and L. Wang, Atmos. Chem. Phys., 2016, 16, 2285–2298 CrossRef CAS.
- X. Wang, N. Hayeck, M. Brüggemann, L. Yao, H. Chen, C. Zhang, C. Emmelin, J. Chen, C. George and L. Wang, J. Geophys. Res.: Atmos., 2017, 122, 11703–11722 CrossRef CAS.
- A. G. Rincón, A. I. Calvo, M. Dietzel and M. Kalberer, Environ. Chem., 2012, 9, 298 CrossRef.
- S. S. Steimer, D. J. Patton, T. V. Vu, M. Panagi, P. S. Monks, R. M. Harrison, Z. L. Fleming, Z. Shi and M. Kalberer, Atmos. Chem. Phys., 2020, 20, 13303–13318 CrossRef CAS.
- D. J. Bryant, W. J. Dixon, J. R. Hopkins, R. E. Dunmore, K. L. Pereira, M. Shaw, F. A. Squires, T. J. Bannan, A. Mehra, S. D. Worrall, A. Bacak, H. Coe, C. J. Percival, L. K. Whalley, D. E. Heard, E. J. Slater, B. Ouyang, T. Cui, J. D. Surratt, D. Liu, Z. Shi, R. Harrison, Y. Sun, W. Xu, A. C. Lewis, J. D. Lee, A. R. Rickard and J. F. Hamilton, Atmos. Chem. Phys., 2020, 20, 7531–7552 CrossRef CAS.
- W. Rattanavaraha, K. Chu, S. H. Budisulistiorini, M. Riva, Y. H. Lin, E. S. Edgerton, K. Baumann, S. L. Shaw, H. Guo, L. King, R. J. Weber, M. E. Neff, E. A. Stone, J. H. Offenberg, Z. Zhang, A. Gold and J. D. Surratt, Atmos. Chem. Phys., 2016, 16, 4897–4914 CrossRef CAS PubMed.
- M. Brüggemann, D. van Pinxteren, Y. Wang, J. Z. Yu and H. Herrmann, Environ. Chem., 2019, 16, 333 CrossRef.
- Y. Wang, J. Ren, X. H. H. Huang, R. Tong and J. Z. Yu, Environ. Sci. Technol., 2017, 51, 6791–6801 CrossRef CAS PubMed.
- R.-J. Huang, J. Cao, Y. Chen, L. Yang, J. Shen, Q. You, K. Wang, C. Lin, W. Xu, B. Gao, Y. Li, Q. Chen, T. Hoffmann, C. D. O'Dowd, M. Bilde and M. Glasius, Atmos. Meas. Tech., 2018, 11, 3447–3456 CrossRef CAS.
- Y. Wang, Y. Ma, X. Li, B. Y. Kuang, C. Huang, R. Tong and J. Z. Yu, Environ. Sci. Technol., 2019, 53, 12278–12290 CrossRef CAS PubMed.
- Y. Wang, R. Tong and J. Z. Yu, Environ. Sci. Technol., 2021, 55(13), 8573–8582 CrossRef CAS PubMed.
- C. M. Kenseth, N. J. Hafeman, Y. Huang, N. F. Dalleska, B. M. Stoltz and J. H. Seinfeld, Environ. Sci. Technol., 2020, 54(20), 12829–12839 CrossRef CAS PubMed.
- R. E. O'Brien, A. Laskin, J. Laskin, C. L. Rubitschun, J. D. Surratt and A. H. Goldstein, J. Geophys. Res.: Atmos., 2014, 119, 12706–12720 Search PubMed.
- A. G. Bé, M. A. Upshur, P. Liu, S. T. Martin, F. M. Geiger and R. J. Thomson, ACS Cent. Sci., 2017, 3, 715–725 CrossRef PubMed.
- J. Parshintsev, T. Hyötyläinen, K. Hartonen, M. Kulmala and M. L. Riekkola, Talanta, 2010, 80, 1170–1176 CrossRef CAS PubMed.
- M. Oss, A. Kruve, K. Herodes and I. Leito, Anal. Chem., 2010, 82, 2865–2872 CrossRef CAS PubMed.
- A. Kruve, Anal. Chem., 2020, 92(7), 4691–4699 CrossRef CAS PubMed.
- A. Kruve, K. Kaupmees, J. Liigand and I. Leito, Anal. Chem., 2014, 86, 4822–4830 CrossRef CAS PubMed.
- J. Liigand, T. Wang, J. Kellogg, J. Smedsgaard, N. Cech and A. Kruve, Sci. Rep., 2020, 10, 1–10 CrossRef PubMed.
- A. W. Mayhew, D. O. Topping and J. F. Hamilton, ACS Omega, 2020, 5(16), 9510–9516 CrossRef CAS PubMed.
- C. M. Alfaro, A.-O. Uwakweh, D. A. Todd, B. M. Ehrmann and N. B. Cech, Anal. Chem., 2014, 86, 10639–10645 CrossRef CAS PubMed.
- T. Henriksen, R. K. Juhler, B. Svensmark and N. B. Cech, J. Am. Soc. Mass Spectrom., 2005, 16, 446–455 CrossRef CAS PubMed.
- B. M. Ehrmann, T. Henriksen and N. B. Cech, J. Am. Soc. Mass Spectrom., 2011, 19, 719–728 CrossRef PubMed.
- C. I. Gioumouxouzis, M. G. Kouskoura and C. K. Markopoulou, J. Chromatogr. B: Anal. Technol. Biomed. Life Sci., 2015, 998–999, 97–105 CrossRef CAS PubMed.
- V. J. Mandra, M. G. Kouskoura and C. K. Markopoulou, Rapid Commun. Mass Spectrom., 2015, 29, 1661–1675 CrossRef CAS PubMed.
- J. Liigand, T. Wang, J. Kellogg, J. Smedsgaard, N. Cech and A. Kruve, Sci. Rep., 2020, 10, 5808 CrossRef CAS PubMed.
- X. Zhang, R. C. McVay, D. D. Huang, N. F. Dalleska, B. Aumont, R. C. Flagan and J. H. Seinfeld, Proc. Natl. Acad. Sci. U. S. A., 2015, 112, 14168–14173 CrossRef CAS PubMed.
- J. Dong, D. S. Cao, H. Y. Miao, S. Liu, B. C. Deng, Y. H. Yun, N. N. Wang, A. P. Lu, W. Bin Zeng and A. F. Chen, J. Cheminf., 2015, 7, 60 Search PubMed.
- K. L. Pereira, G. Rovelli, Y. C. Song, A. W. Mayhew, J. P. Reid and J. F. Hamilton, Atmos. Meas. Tech., 2019, 12, 4519–4541 CrossRef CAS.
- E. Finessi, R. T. Lidster, F. Whiting, T. Elliott, M. R. Alfarra, G. B. McFiggans and J. F. Hamilton, Anal. Chem., 2014, 86, 11238–11245 CrossRef CAS PubMed.
- Z. Shi, T. Vu, S. Kotthaus, R. M. Harrison, S. Grimmond, S. Yue, T. Zhu, J. Lee, Y. Han, M. Demuzere, R. E. Dunmore, L. Ren, D. Liu, Y. Wang, O. Wild, J. Allan, W. J. Acton, J. Barlow, B. Barratt, D. Beddows, W. J. Bloss, G. Calzolai, D. Carruthers, D. C. Carslaw, Q. Chan, L. Chatzidiakou, Y. Chen, L. Crilley, H. Coe, T. Dai, R. Doherty, F. Duan, P. Fu, B. Ge, M. Ge, D. Guan, J. F. Hamilton, K. He, M. Heal, D. Heard, C. N. Hewitt, M. Hollaway, M. Hu, D. Ji, X. Jiang, R. Jones, M. Kalberer, F. J. Kelly, L. Kramer, B. Langford, C. Lin, A. C. Lewis, J. Li, W. Li, H. Liu, J. Liu, M. Loh, K. Lu, F. Lucarelli, G. Mann, G. McFiggans, M. R. Miller, G. Mills, P. Monk, E. Nemitz, F. O'Connor, B. Ouyang, P. I. Palmer, C. Percival, O. Popoola, C. Reeves, A. R. Rickard, L. Shao, G. Shi, D. Spracklen, D. Stevenson, Y. Sun, Z. Sun, S. Tao, S. Tong, Q. Wang, W. Wang, X. Wang, X. Wang, Z. Wang, L. Wei, L. Whalley, X. Wu, Z. Wu, P. Xie, F. Yang, Q. Zhang, Y. Zhang, Y. Zhang and M. Zheng, Atmos. Chem. Phys., 2019, 19, 7519–7546 CrossRef CAS.
- M. Kuhn, J. Stat. Software, 2008, 28, 1–26 CrossRef.
- A. Kruve and K. Kaupmees, Anal. Chem., 2017, 89, 5079–5086 CrossRef CAS PubMed.
- T. Wang, J. Liigand, H. L. Frandsen, J. Smedsgaard and A. Kruve, Food Chem., 2020, 318, 126460 CrossRef CAS PubMed.
- J. Hammes, A. Lutz, T. Mentel, C. Faxon and M. Hallquist, Atmos. Chem. Phys., 2019, 19, 13037–13052 CrossRef CAS.
- B. Witkowski and T. Gierczak, Atmos. Environ., 2017, 154, 297–307 CrossRef CAS.
- M. R. Alfarra, J. F. Hamilton, K. P. Wyche, N. Good, M. W. Ward, T. Carr, M. H. Barley, P. S. Monks, M. E. Jenkin, A. C. Lewis and G. B. McFiggans, Atmos. Chem. Phys., 2012, 12, 6417–6436 CrossRef CAS.
- H. Tong, I. Kourtchev, P. Pant, I. J. Keyte, I. P. O'Connor, J. C. Wenger, F. D. Pope, R. M. Harrison and M. Kalberer, Faraday Discuss., 2016, 189, 51–68 RSC.
- S. Kundu, R. Fisseha, A. L. Putman, T. A. Rahn and L. R. Mazzoleni, Atmos. Chem. Phys., 2012, 12, 5523–5536 CrossRef CAS.
- A. L. Putman, J. H. Offenberg, R. Fisseha, S. Kundu, T. A. Rahn and L. R. Mazzoleni, Atmos. Environ., 2012, 46, 164–172 CrossRef CAS.
- I. Kourtchev, S. Fuller, J. Aalto, T. M. Ruuskanen, M. W. McLeod, W. Maenhaut, R. Jones, M. Kulmala and M. Kalberer, Environ. Sci. Technol., 2013, 47, 4069–4079 CrossRef CAS PubMed.
- S. S. Steimer, D. J. Patton, T. V. Vu, M. Panagi, P. S. Monks, R. M. Harrison, Z. L. Fleming, Z. Shi and M. Kalberer, Atmos. Chem. Phys., 2020, 20, 13303–13318 CrossRef CAS.
- K. L. Pereira, M. W. Ward, J. L. Wilkinson, J. B. Sallach, D. J. Bryant, W. J. Dixon, J. F. Hamilton and A. C. Lewis, Environ. Sci. Technol., 2021, 55(11), 7365–7375 CrossRef CAS PubMed.
- X. Zhang, R. C. McVay, D. D. Huang and J. H. Seinfeld, PNAS, 2015, 112(46), 14168–14173 CrossRef CAS PubMed.
- D. Thomsen, J. Elm, B. Rosati, J. T. Skønager, M. Bilde and M. Glasius, ACS Earth Space Chem., 2021, 5(3), 632–644 CrossRef CAS.
Footnotes |
† Electronic supplementary information (ESI) available. See DOI: https://doi.org/10.1039/d2ea00074a |
‡ Present address: Faculty of Science and Technology, Bournemouth University, BH12 5BB, UK. |
|
This journal is © The Royal Society of Chemistry 2023 |