How advances in theoretical chemistry meet industrial expectations in electrocatalysts for water splitting
Received
9th June 2023
, Accepted 11th September 2023
First published on 22nd September 2023
Abstract
Fundamental knowledge about heterogeneous catalysis has significantly advanced in the last few years due to the awareness of the role of non-weakly correlated electrons in open-shell magnetic catalysts, and their degrees of freedom (charge, spin, orbital and structure). Such recognition represents a paradigm shift, because it proves the existence of non-linear oscillations with orbital filling, and also feasible deviations from the Bell–Evans–Polanyi (BEP) principle. By including all the relevant quantum interactions, orbital engineering seeks to identify potentially successful catalysts aprioristically by first principles. The approach does not include nor admit shortcuts. Two steps are needed to narrow down the synthetic quest for optimal catalysts (via orbital configurations), to boost and, concomitantly, fully understand catalytic activities: 1) obtaining the electronic properties, bond topology, populations, magnetic (spin–orbital ordering) structure to infer stability and reactivity, and 2) achieving complete mechanistic insights. Thus, quantum chemistry can be a powerful tool to reinforce traditional industrial developments in water electrolysis and accelerate catalytic designs by implementing physical rationality, while reducing considerably time and waste. This perspective intends to clarify the electrocatalytic challenges in using water electrolysers (WEL), and the advanced computational approaches to tackle them from the perspective of industrial needs.
1. Introduction
The green hydrogen industry is currently governed by the urgency of mass production of high performing catalysts for water electrolysers (WEL) and hydrogen fuel cells (HFC). The main reactions taking place in WELs are the hydrogen evolution reaction (HER) and the oxygen evolution reaction (OER), and there are still unresolved case studies from the perspective of activity and stability.1 Moreover, industry still relies too heavily on the use of precious metals (platinum group) as the catalysts of choice in WEL technology,2,3 whereas, due to value, scarcity and global demand of for Pt group elements, it should rather focus on maximising the use of earth abundant elements, such as Co Cr, iron (Fe), Mn, nickel (Ni) and V. Precious metals/ions have open-shell configurations, and their compositions usually show a large variety of magnetic properties (spin–orbital ordering), being strongly correlated compositions.4 Because spin-dependent interactions can positively impact on oxygen electrochemistry,5–13 our focus in understanding non-weakly correlated open-shell compositions has been steadily growing since 2015. Spin-potentials enhance biological-, homogeneous-, heterogeneous-, electro- and photo-catalysts,14–25 thus any open-shell configuration, whether it is metal, non-metal,26,27 single site,14,19,28–39 clusters40–42 or extended systems.43–48 The spin interactions in open-shell configurations based on some 3d and 4d metals/ions are of particular interest in OER (therefore in WEL) because they can reduce activation barriers, thus enhancing reaction kinetics.8,49
The understanding of interactions between electrons in heterogeneous catalysts has substantially improved in the last few years coinciding with a better interpretation of quantum correlations.4,50–53 We have been able to establish a link between non-linear alterations in catalytic activity, the orbital filling, and the magnetic/spin ordering of materials.5,51,53 All these scientific advances, which demonstrate the significant influence of (cooperative) electronic correlations on the activity and stability of catalysts, triggered mainly by quantum spin exchange interactions (QSEI),4 are the spark that may influence the situation for industrial electrocatalysts. The need for a thorough search for catalysts with specific magnetic/spin properties is an integral part of our electrolyser developments. Industry may benefit greatly from a knowledge-based design approach, a higher degree of control, predictability, and a high success rate generated by theoretical predictions of electrocatalysts. This perspective aims to draw more attention to how modern orbital engineering (an incorporation of complete fundamental quantum principles)54 can be synergistically combined with other methodologies to satisfy industrial needs.
2. Discussion and results
2.1. Brief industrial overview of electrolysers
Fig. 1 shows the most prominent features of water WELs. Alkaline electrolysers (A_EL) are the oldest and most established systems, and they mainly rely on abundant alloys of Ni (NB: open-shell configuration) and molybdenum (Mo) as cathodes for the HER. The Ni and Fe metals (NB: both open-shell configurations) are used as anodes for the OER (NB: oxygen is in the triplet state). The electrolyte normally employed is 6 M KOH and a diaphragm (e.g., Zirfon©) is used to separate the half cells to prevent gas crossover.55–57 The diaphragm is permeable to the liquid electrolyte, thus the hydroxide ions produced at the cathode during proton reduction can diffuse to the anode. Lower (compared with proton exchange membrane electrolysers, PEM_EL) current densities (up to 0.5–1.0 A cm−2, depending on the catalyst used and influenced by the higher resistance of the cell assembly), and a corrosive electrolyte are the main drawbacks.58 In addition, the A_EL suffers from a severe catalyst degradation due to reverse currents in the starting and stopping operations. The integration of durable electrodes and catalysts that are stable under intermittent renewable power is indispensable.59
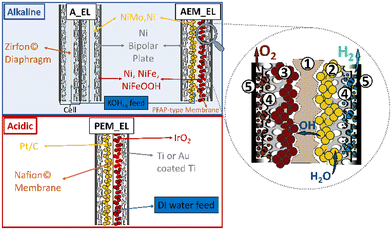 |
| Fig. 1 Left: Comparison of different electrolyser technologies, namely, alkaline (A_EL), proton exchange membrane (PEM_EL), and anion exchange membrane (AEM_EL). Right: Alkaline water electrolyser cell and its components: (1) separator, (2) HER catalyst, (3) OER catalyst, (4) porous electrode substrate and (5) bipolar endplate with gas channels. | |
The PEM_EL have been derived from fuel cell technology. A membrane separates the two compartments, which is typically directly coated with the catalyst. This membrane is a solid electrolyte, conducting protons produced by water oxidation towards the cathode. The pH of the cell is strongly acidic, rendering non-noble metal-based catalysts unstable. Platinum is mainly used at the cathode, and iridium oxide (IrO2) at the anode.60,61 All the peripheral parts (e.g., gas diffusion layers and bipolar plates) are made of corrosion resistant metals. The current density (well above 1 A cm−2) is improved in the PEM_EL when compared to the A_EL and the system exhibits a higher flexibility towards the energy source.62 Still, the expensive rare metals, necessary to enable low degradation in the harsh conditions of PEM_EL, are too scarce for the global demand.
Anion exchange membrane electrolysers (AEM_EL) are in development, and the alkaline electrolytes can be used as medium, enabling the use of abundant catalysts similar to A_EL.63 The membrane in AEM_EL can be coated directly with the catalysts as in the PEM_EL or, alternatively, a coated current collector can be pressed onto the membrane, with the possibility to achieve high currents of up to 1–2 A cm−2. There is a lack of deployment and data for large electrolysers, however, because developments are still ongoing in this premature technology. Instability of the membrane is the main target for improvements. It should also be noted that there is still an opportunity for progress in A_EL technology, thus it remains unclear whether AEM_EL may outperform advanced A_EL and subsequently penetrate the market.
Generally, and independent of the technology, industry aims to operate electrolysers below 1.85 V with current densities of at least 1 A cm−2. This translates to cell efficiencies of 55–65%, with a total overpotential of 0.4–0.7 V, out of which the activation losses at the OER electrode are about 60–80% of the total catalytic losses.63 To become cost competitive, H2 from WEL should be below 2.6 € per kg,64,65 thus, not only the efficiency, but also capital expenditures (CAPEX), must be improved. Nowadays, the cost of hydrogen from electrolysers is about ∼10 € per kg,66,67 and this is waiting to be decreased by the appearance of cheaper renewable sources of electricity and cheaper stack technology. Another important aspect to consider is that a higher CAPEX does not necessarily equate to an optimised WEL system. Only about 50% of the CAPEX in A_EL (ca. 250–1000 € per kW)63 and PEM_EL (ca. 900–2000 € per kW)66 are based on stack cost, and the catalysts make up only ca. 25% of the stack cost in PEM_EL, and ca. 50% of the stack costs in A_EL.67,68 This translates into the fact that the main technology used to produce hydrogen to date is the PEM system,63 due to their higher flexibility and better current densities, even though they have significantly higher CAPEX, and lower efficiencies.59
Another important expenditure factor is the cost/availability of global electrolyser production. The use of cheap, but highly active, materials is unavoidable to achieve the goal of an employed capacity of several hundred gigawatts (GWs) in a sustainable fashion. There are, of course, costs of manufacturing, but they tend to decrease with mass production, so the real problem lies in the cost, and the scarcity of some of the elements used that might make the whole process unsustainable. Many A_ELs, for example, still rely on electrodes coated with platinum-group metals (PGM) to enhance stable performances, despite catalysts based on PGM (e.g., Ir, IrO2, and so on) being very expensive and not optimally suited for the task, but precisely for their high cost and scarcity. In order to replace a large steam reforming plant (180.000 Nm3 per h of H2), a load of about 0.5–1 ton of IrO2 is necessary, if PEM_EL is used, translating to an investment cost of about 50–110 million € for the catalytic coating of the electrodes alone (at 1–2 mg cm−2 and an IrO2 price of about 152.000 € per kg),70 without considering the maintenance and recoating, that has to take place at least every 10 years with recycling losses of PGM of about 50% (−25–−50 million €). It also starts to become apparent that the CAPEX contributions become less important to the overall H2 costs for high annual load hours, see Fig. 2, which are especially targeted at high investment costs. Therefore, durability and efficiency play a key role, and the improved kinetics of open-shell magnetic catalysts directly translates into a reduced CAPEX $ per kg_H2 per hour, Fig. 2. On the other hand, the high costs of electrical power in Germany, for example, are generally the main driving force for the H2 price in the long run, and legislation has to be adapted and translated into a CO2 taxation to make the cost of green hydrogen competitive.68
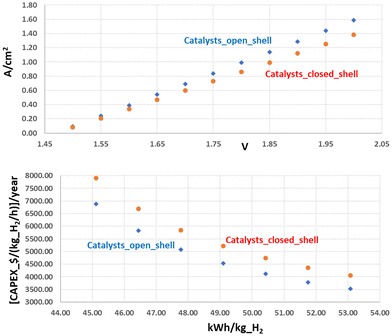 |
| Fig. 2 Top: Catalysts with improved kinetics, influenced by optimised (intra- and inter-atomic) QSEI, show higher current densities. Bottom: Optimum kinetics is one of the most relevant parameters to reduce the investment per kg H2 per hour produced. The graphs extrapolate the data from the average improvement of the current density observed by Xu and co-workers,10,69 and correlating them with the WEL building specifications from manufacturers and market data. | |
From an industrial perspective, materials are chosen based on their proven performance, hence more accurate predictive tools can have a great impact on future catalyst generations, paving the way towards cheaper and better catalysts.
2.2. Predictive models in WEL: complete reaction mechanism with quantum correlations
Theoretical and computational chemistry must still adapt to heterogeneous catalysis to provide improved predictions of highly efficient catalysts based on earth abundance and low-cost materials, thus making the future H2-GW-scale up easier. For example, fast computational approximations for HER based on volcano plots generated only from the adsorption enthalpy of atomic hydrogen are unable to properly rank catalysts by their activity. For example, simplified approximations do not distinguish the low activity of WS2 or MoS2 from the high activity of Pt or the incorrect prediction of a lower activity of NiMo, or the activity of Re from Ni and Co,71 or Pt from Pd and Ir.72 The good news is that these approximations did not consider complete reaction paths and possible deviations from the BEP principle coming from open-shell QSEI.4,73,74 The BEP approximation for a reaction step assumes that the difference in activation energy between two catalysts is proportional to the enthalpy difference of the event at the distinct active sites. Thus, it is assumed that the pre-exponential factor of the Arrhenius equation, and the position of the transition state along the reaction coordinate are the same for all catalysts for common reaction steps.
These considerations can be extended to OER, especially for Fe, Co and Ni based catalysts (NB: the same consideration can be extended to any magnetic 3d, 4d, 4f metals) because the spin-polarisation of open-shell systems and its influence on activation barriers have been frequently disregarded in calculations. It is understood that the spin-potentials are the quantum trigger that industry needs for catalytic design.5,9–12,75 When perusing the literature, on OER, incomplete thermodynamic profiles in WEL are used, frequently including the formation/activation and the adsorption/desorption of the product triplet state 3O2. It is unclear yet whether the total overpotential is always proportional to the over- or under-bonding of certain reactants. Overall, simple linear correlations based on the local density functional theory (DFT) have an error margin of ±0.3 V vs. the experimental data of the oxygen electrocatalysis.76–78 Rendering this approach ineffective, based on the lack distinctions between outstanding catalysts with low overpotentials (i.e., possible cells with efficiencies of 75–85%, operating at 1.6–1.8 V with current densities over 1 A cm−2) and terrible catalysts with efficiencies of 20–40% (operating at 2.0–2.2 V with current densities over 1 A cm−2). However, this is still good news, because we know most likely what is missing, complete reaction profiles with the thermodynamics of all the intermediates, including the transition states to really map the kinetics. This is the best strategy, sufficiently accurate, to properly understand and compare, most of the studied cases dealing with weakly and non-weakly correlated electrons.
However, the main challenge in modern theoretical chemistry for heterogeneous catalysis still lies in the proper description of the electronic structure of for the non-weakly correlated compositions (NWCO).4 For the NWCO, the approximation of independent electrons, which is used in common local-DFT studies, fails.79 It is promising that the scientific literature starts to show a transition towards more accurate models for WEL, OER and HER: from a dozen publications before 2016, we have moved to about a thousand papers related to the significance of spin-/magnetic-potentials in heterogeneous catalysis.
Industry demands a step forward in the rational design80 of catalytic electrodes and to realise this, the magnetic properties of the whole catalytic interphase must also be considered at the development stage.6,10,45,81–96 MagnetoCat's R&D department is currently pushing fundamental computational chemistry towards the proper inclusion of quantum correlations4–6,50 in the description of complete reaction pathways, materials properties and comprehensive physical models. This methodology allows a common treatment and a fair comparison (with similar accuracy) between closed-shell (weakly correlated) and open-shell catalysts alike. The inclusion of quantum correlations in catalytic design is essential if we consider that novel predictors mainly based on spin-potentials from open-shell orbitals have already contributed to identify magnetic material combinations that exhibit OER activities at least one order of magnitude larger than that of IrO2 (usually identified as the state-of-the-art in industrial OER).11,97–99 These advances will reduce the gap between fundamental research and applied technology for clean energy,15,43,100–103 and we are moving towards a ±0.1 V error in the computational accuracy of reaction pathways when comparing and screening active catalysts. Likewise, accurate computational chemistry can also deliver improvements in noble metal based catalysts utilised in PEM_EL, by maximising the efficiency and concomitantly lowering the Pt content, via orbital engineering of magnetic elements, as in the case of Pt3Co.52,53
Because there is no clear indication at the present time of which WEL technology will become dominant in the near future (although PEM_EL might be the technology of choice when coupled to highly volatile renewables)63 specific developments and optimisation methods should not be limited to a single technology, but they should be extended to all six possible (different) catalytic interphases (i.e., three set ups previously seen for HER and OER). There are several factors that come together in the creation of optimal catalytic interphases: there are aspects of fundamental science, such as the design of optimal combinations of current collector and catalyst, and there are technological aspects, such as robustness and performance, that cannot be assessed or identified simply by fundamental research.104 Finally, there are market demands, also not identifiable by fundamental science. While the latter aspects are somewhat routine in industrial operations, the former aspect, the rational design80 and improvement of catalysts (and/or related components), can be successfully carried out by including orbital theory and engineering into the primary designs.
Industrial catalytic design is often slow due to a cumbersome trial-and-error strategy in the synthetic as well as in the testing phase: in fact, every potential catalytic candidate must be proved sufficiently stable for at least 10–20
000 h of testing under operating conditions. Stability of the catalyst is a major factor in the design of industrial scale electrolysers because the investment costs are high, and maintenance and replacement should be minimised as much as possible: catalysts and electrodes from abundant cheap elements would be ideal if highly resistant to operational conditions. If some properties of catalysts could be predicted instead, leading to enhanced efficiency/stability in alkaline and acidic media with minimum use of rare metals, then the scope of the materials could be narrowed down to the decrease R&D costs and wastes associated with stability assessment. Data coming from relatively inexpensive computational rational design can be used to “hint” at suitable catalytic structures with the required characteristics of stability and activity for synthesis and testing. The latter would provide definitive validation or feed into the loop suggestions of improvement for general and/or specific parameters. The models will be updated using these new data and the screening will be improved by re-initiating a screening and re-feeding new potential candidates into the loop, Fig. 3.
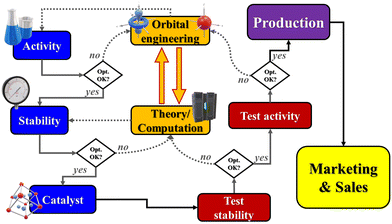 |
| Fig. 3 Schematic integration of theoretical chemistry into our industrial routines. | |
2.3. Oxygen evolution reaction (OER)
The reaction mechanism and steps involved in the OER are still not fully elucidated for most of the catalysts used. The starting substrate of OER is either H2O, in an acid environment, or OH−, in an alkaline environment, and either of them is in a singlet state (↑↓ all electrons paired). Hence, it becomes clear that, in the absence of spin-selection or spin flipping/scattering, the oxygen evolved will be in the singlet state, 1 eV higher in energy than the actual product triplet state ↑O
O↑,9,105 following angular momentum conservation. Thus, for the closed-shell catalysts, the process either pays a considerable energy penalty or undergoes slower non-coherent spin flip events, as shown in Fig. 4 and 5.
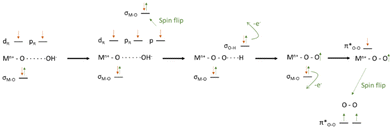 |
| Fig. 4 Schematic representation of OER for the formation of singlet or triplet oxygen via spin flip events. Reprinted and readapted from Wu and Xu, Oxygen evolution in spin-sensitive pathways, volume 30, page no. 100804, Copyright (2021), with permission from Elsevier.105 | |
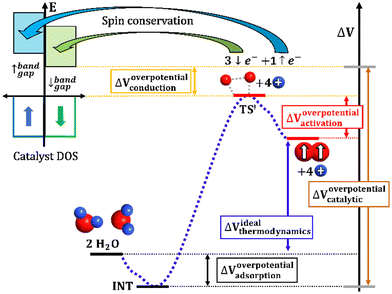 |
| Fig. 5 Illustration of the several factors considered to contribute to the total OER overpotential at the catalytic interphase. | |
The four-electron oxidation process of OER happens at the anode viaeqn (1a) or (1b) (depending on the conditions, see Appendix A) and usually shows high overpotentials for most catalysts.106–109 It is well-known that the overall efficiency of water splitting typically decreases due to the sluggish kinetics in OER.107,110Fig. 5 illustrates every single component of the total overpotential, ΔVoverpotentialcatalytic, for a general OER catalyst. Each component has a different origin, but they must be considered together. ΔVoverpotentialadsorption is the overpotential related to the adsorption of intermediates, where ΔVidealthermodynamics is the ideal thermodynamic value for OER (E0 = 1.23 V in standard conditions). ΔVoverpotentialactivation is the overpotential related to the activation barrier(s) for the formation or cleavage of relevant bonds, and ΔVoverpotentialconduction is the overpotential related to electron transfers from the reactants to the conduction band of the catalyst. Industry should be aware that theoretical chemistry is able to isolate, study and quantify all these contributions in the quest for improved catalytic structures. The observation of such complexity in experiments, in fact, explains the failure of multiple descriptors.61,111 Complete reaction profiles will be employed to have mechanistic studies with the proper description of the ΔVoverpotentialcatalytic.
In modern catalytic design, the first parameter to look for in efficient catalysts for OER (carbides, nitrides, oxides, phosphides, and sulfides, among others) are itinerant spin channels extended through space and associated with ferromagnetic inter-atomic exchange in the catalytic structure.5–8,10,11,17,112–114 The electrons need an excess of degenerate orthogonal orbitals between the inter-atomic bonds to maximise the QSEI4–6,112 in a certain direction of space (NB: spin/orbital ordering adds a directional character to many properties). The metal–ligand–metal double-exchange mechanism, originated from the quantum correlations and creates itinerant ferromagnetic spin channels in the OER catalysts,98,115–118 even in RuO2 shown in Fig. 6. Double exchange needs to be the dominant, at least, in one dimension of the space, in metallic states whether above or below the Curie temperature,11,74,98,119,120 to allow spin transport towards the evolution of the triplet state 3O2 from the singlet H2O. In order to provide industry with a tangible quantification of the effects mentioned previously, we can consider the example of Sr1-XCaXCoO2+δ, which is part of a family of catalysts with exceptional OER activity:121 where the transition state for the formation of 3O2 is reduced by ca. 23% by spin-potentials, and the desorption energies decrease by about 10–15% compared to a non-magnetic system,8 providing an example of deviation from the BEP principle. On the other hand, if there are no extra degenerate empty orbitals available in the species along a particular direction, antiferromagnetic (AFM) ordering might get stabilised in that direction, blocking spin-transport along those bonds.79,112,122–124 Interactions between substrates/intermediates and magnetic catalysts display poorer covalency, opening up the possibility of enhanced catalytic activity with respect to that of the closed-shell non-magnetic catalysts. The nature of QSEI in magnetic bonds, stable but less bonding, can also relate to the Sabatier principle: the concept of magnetic nobility.50–53 The non-covalent stabilisation due to inter-atomic QSEI between atoms with open-shell configurations leads to the decrease of: a) the interatomic electronic repulsions within the catalyst itself, and b) the bond strength of the oxygenated reactants and lowering of the activation barriers.
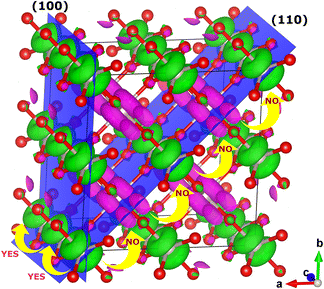 |
| Fig. 6 Itinerant ferromagnetic spin channels, parallel to the c axis, in AFM rutile RuO2. The spin density shows the occupation of the (non-bonding) t2g orbitals. | |
No energy separation between the valence and conduction band occurs for non-magnetic closed-shell or open-shell configurations with metallic density of states (DOS). For these materials there is no contribution to the OER overpotential due to the conduction of the electrons through the catalysts, ∇Voverpotentialconduction = 0. If electrons do not have to change the spin direction to move from one species to another, their movement is also facilitated. Thus, metallic open-shell DOS showing itinerant ferromagnetic spin channels, such as rutile RuO2, with differentiated electronic mobility and chemical potential for each spin, have the potential to offer better catalytic activity (even above the Curie or Néel temperatures). Rutile RuO2 is a C-type AFM metallic conductor125,126 (Néel T > 300 K) and it is considered a reference catalyst for OER in the academic community.11,127–132
Fig. 6 shows the ferromagnetic itinerant spin channels for bulk rutile RuO2 along the (100) plane. Spin polarisation on the bonds of the OER active sites is an important parameter because it can decrease the overpotentials by facilitating the path of intermediates with favourable spin-orientation towards the formation of 3O2 (ref. 11 and 127–132) and, additionally, it facilitates the accumulation of spin-density on the catalyst by oxidation of the reactants, and its release in the form of 3O2.8 So far, in the ferromagnetic paths the majority spin will be parallel to the evolution
, whereas the minority spin will transport most of the charge.
The three parameters previously elucidated are good examples of useful descriptors that can be anticipated by using orbital engineering: they lead to an immediate discard of Mott insulators, such LaCrO3 or LaFeO3, as potential electrocatalysts for OER, which agrees with the experimental data.133,134 The quest is clear, we are looking for good conductive magnetic structures112,135 with optimum occupation of the orbitals, which is also important in many other reactions, e.g., in ammonia synthesis,136 the Fischer–Tropsch process,137 and fuel cells.50Fig. 7 shows the improved OER current density of Ni foams with deposited RuO2 nanoparticles. The work of Yu and co-workers proves independently, how metal foams can serve as substrates to construct high performance WEL OER electrodes.138 This notion agrees with our theoretical model, where spin-channels, as in the case of RuO2, can improve current densities by several orders of magnitude. This effect can be enhanced by doping with RuO2 to make it more (spin-polarised) ferromagnetic.11,127–132
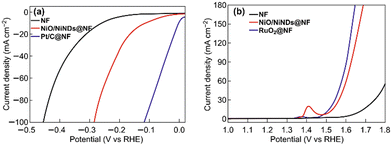 |
| Fig. 7 a) The HER and b) the OER performance of different Ni foam-based electrodes under 1 mg cm−2 loading determined using linear sweep voltammetry with a three-electrode configuration in a 1 M KOH aqueous electrolyte (all of the scan rates were 2 mV s−1). Reprinted and readapted under the terms of the Creative Commons CC BY license from the work of Yu et al.,138 with permission from Springer Nature Publisher. | |
2.4. Hydrogen evolution reaction (HER)
The hydrogen evolution reaction (HER) yields valuable H2 in electrolysers, therefore, a lot of effort is also required to reduce its overpotentials. The Volmer step (eqn (3) in Appendix A) or the Heyrovský step (eqn (4) in Appendix A) dominate HER at low potentials on metals, followed by the Heyrovský step at high overpotentials, whereas the Tafel step (eqn (5) in Appendix A) can be ignored.139 Due to the involvement of oxygenated intermediates in HER (derived from the presence of aqueous or alkaline media), additional factors besides the M–H adsorption and the H2 formation come into play. Studies of the hydrogenation of metal surfaces, dissociative adsorption, and associative desorption of H2 are mostly carried out in vacuum or, at the very least, in a hydrogenated atmosphere (see, for example, studies on Ni,140 Ag,141 and Pt (ref. 142)), but this is not a “real” environment during an electrocatalytic HER process. Species such as H2O, in both acidic and alkaline media, and OH−, particularly in alkaline media, may influence the HER mechanism143 kinetically supported by their overwhelming concentrations with respect to substrates, and participating as Langmuir–Hinshelwood mediators.
The situation for HER closely resembles that of OER from the point of view of the computational models. Approximations based on volcano plots derived from the adsorption enthalpy of atomic hydrogen on metal surfaces are ill-suited to properly rank catalysts in order of activity. The data from Shen et al. show that five metals (Co, Cu, Fe, Ni and Pd) out of a small group of nine metals are out of the linear trend for HER in basic media.144 Simplifications are unable to explain the alterations between Au and Ag, between Cu and Pt, and among Fe, Co and Ni. These studies agree well with results reported in literature,143–149 and the authors generally observed that it was impossible to distinguish between Pd, Ru and Rh in a group of eight metals.145 It was also noticed that antiferromagnetic Mn was absent in most studies, being one of the worst HER catalysts.150
Fig. 8 shows an inconclusive spread of the d-band-center in describing HER. Table 1 includes M–H and M–O bond strengths for a sample of metals51 with their HER activity based experimental currents in acidic and alkaline media (all values are taken from the published literature).148–150 A 2D-scatter plot of the HER activity versus calculated M–H bond enthalpy in Fig. 9a is inadequate to explain the catalytic trends seen among the metals. With such simplification we cannot explain why, for example, Pt3Co and Cu which have very similar M–H bond strengths, but completely dissimilar activities, whereas Pt3Co is a very good catalyst and Cu is not, as for Pt and Ni.
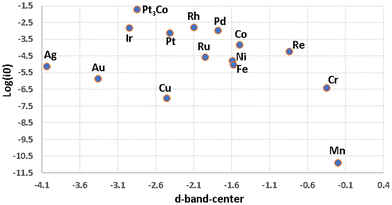 |
| Fig. 8 A 2D-scatter graph plotting experimental exchange current densities for HER in acidic conditions (Table 1) over transition metals versus the d-band-center (Table 1). | |
Table 1 Experimental activities of metals used in HER in acidic148–150 and alkaline media144,148 related to computational M–H and M–O bond strengths
Period |
Metal |
M–H (eV)51,161 (0.5 ML) |
M–O (eV)51,162 (0.5 ML) |
j
0 (10 mA cm−2) (acidic media)148–150 |
j
0 (10 mA cm−2) (alkaline media)144,148 |
Proposed d-band-centre145,163,164 |
3d |
Cr |
0.8 |
−2.19 |
−6.4 |
— |
−0.35 |
3d |
Mn |
1.23 |
−2.21 |
−10.89 |
— |
−0.19 |
3d |
Fe |
−0.8 |
−3.25 |
−4.2 |
−4.9 |
−0.84 |
3d |
Co |
−0.69 |
−2.4 |
−3.8 |
−5.5 |
−1.50 |
3d |
Ni |
−0.66 |
−1.85 |
−4.75 |
−5.1 |
−1.59 |
3d |
Cu |
−0.4 |
−1.45 |
−7.0 |
−5.8 |
−2.46 |
3d |
Zn |
0.5 |
−1.55 |
−10.8 |
— |
— |
4d |
Mo |
−1.23 |
— |
— |
— |
−0.90 |
4d |
Ru |
−0.73 |
−2.8 |
−4.55 |
−3.3 |
−1.95 |
4d |
Rh |
−0.66 |
−1.85 |
−2.75 |
— |
−2.10 |
4d |
Pd |
−0.65 |
−1.0 |
−2.95 |
−3.9 |
−1.78 |
4d |
Ag |
−0.05 |
0.0 |
−5.1 |
−7.3 |
−4.04 |
4d |
Cd |
0.95 |
−0.2 |
−10.77 |
— |
— |
5d |
W |
−1.24 |
−2.4 |
— |
−7.2 |
−0.77 |
5d |
Re |
−0.87 (this work) |
−3.93 (this work) |
−5.0 |
— |
−1.58 |
5d |
Ir |
−0.65 |
−1.5 |
−2.80 |
— |
−2.95 |
5d |
Pt |
−0.61 (ref. 53) |
−0.95 (ref. 53) |
−3.1 |
−3.2 |
−2.42 |
Alloy (5d/3d) |
Pt3Co |
−0.35 (ref. 12) |
−0.52 (ref. 12) |
−1.7 |
— |
−2.85 |
5d |
Au |
0.1 (ref. 53) |
0.5 (ref. 53) |
−5.85 |
−6.2 |
−3.36 |
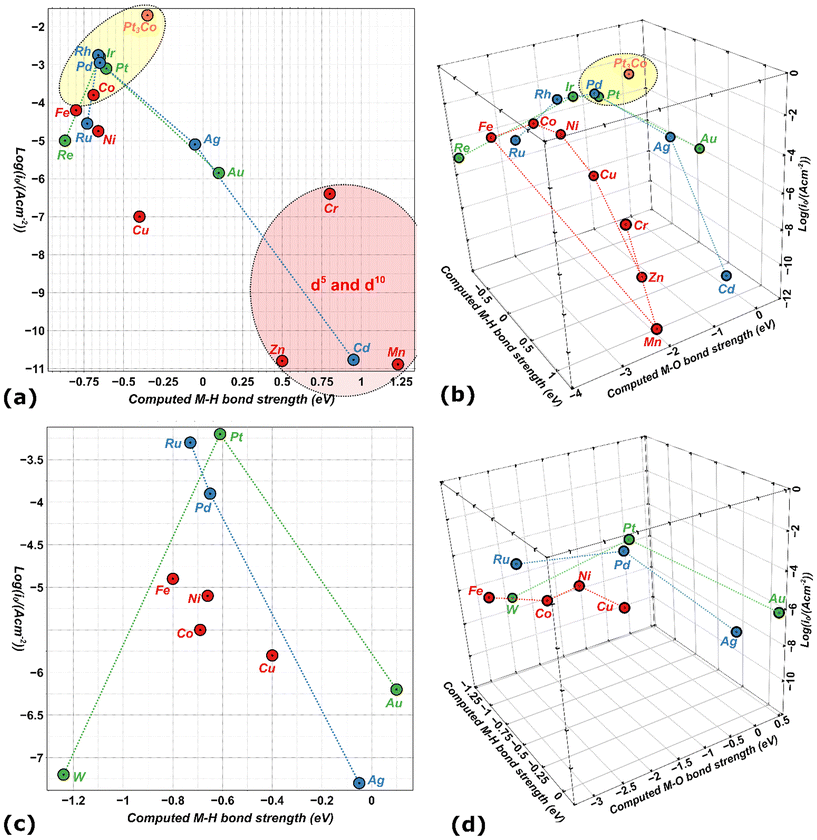 |
| Fig. 9 (a): Experimental exchange current densities for HER in acidic conditions over a selected group of metals 3d (red dots), 4d (cyan dots) and 5d (green dots), and Pt3Co alloy (orange dot) on the y axis, versus the calculated M–H bond energies, on the x axis. (b) The 3D-scatter graph plot in perspective the same experimental exchange current densities for HER in acidic conditions (as before), on the z axis, versus calculated M–H bond energies, on the x axis, and the calculated M–O bond energies, on the y axis. (c) The same 2D-scatter graph as before, but with experimental current densities reported in alkaline conditions. (d) The same 3D-scatter graph as before, but with experimental currents densities reported in alkaline conditions. | |
Overall, fitting linear functions from incomplete reaction profiles and thermodynamics to experimental overpotentials typically does not describe the kinetics of HER, nor does it seem to advance the general understanding of catalysis.28,151–159
The introduction of M–H and M–O bond strengths as minimum indicators renders a better differentiation between metals. Five examples are Ir, Pd, Pt, Pt3Co, and Rh,160 and they exhibit the highest intrinsic activity based on optimum binding energies, for both the hydrogen atom and oxygen intermediates (Fig. 9). Likewise, the low activity of Cu in HER, for example, seems to be based on the overly strong adsorption of oxygenated species, particularly in alkaline media. Nevertheless, it was still observed that the adsorption energies do not seem to describe the activity of all metals satisfactorily. Thus, complete reaction mechanisms with explicit activation barriers are still needed.
In addition, to further elaborate on the key concept of spin-exchange (QSEI) and how it affects reactivity, we can use the intermetallic Pt3Co structure in Table 1. The computed value for Pt3Co has been taken for a non-intermetallic layered model already published in literature.52,53 Magnetic Pt3Co is more active than pure Pt for HER,165,166and shows a decrease in the adsorption energies of the intermediates due to anti-bonding orbitals and spin-potentials.8,9,52,53 Nevertheless, we cannot completely understand the enhanced kinetics of Pt3Co vs. Pt, until the complete reaction mechanism unravelled.
Industry is still far from developing an optimal catalyst for HER catalysis, one that is efficient, stable, and cost-efficient. The PtxCoy alloys display high activity, due to the specific magnetic arrangements of the orbitals and atoms,167–171 but they do not represent a sustainable solution, because of the still elevated cost based on its high content of Pt. Studies in HER catalysis are still incomplete, comprehensive models should be employed to predict and design electrocatalysts for industrial electrolysers taking into consideration all the reaction steps/events and orbital quantum physics. We are looking for highly conductive metals with low adsorption energies for the hydrogen and oxygen atoms. Optimising the use of PGM metals for performance/cost improvements makes sense in terms of economics, particularly for the industrial ‘window of interest’ at 0.8–1.2 A cm−2. To this end, Fig. 7 shows how HER on Ni foams is improved by the deposition of Pt. However, there is still an objective is to find more abundant HER catalysts to deposit on metal foams for the best performances.
3. Conclusions
Explaining the activity and the mechanisms of the most important reactions for sustainable clean energy, HER and OER, is still under development, and it will benefit from more accurate quantum chemistry. We do not know all the limiting factors in the complete reaction pathways that are inherent to active catalysts, but, at least, we acknowledge that the description of non-weakly correlated compositions, properly including QSEI, is a step forward in the general understanding. The first indication for catalytic design in OER is the identification of metallic oxides with suitable ferromagnetic inter-atomic exchange mediated by the ligand atoms in itinerant spin channels. Related principles apply to HER in the search of magnetic inter-metallic structures. The advantage of orbital engineering, particularly when applied in industrial environments, lies in the possibility to significantly reduce the need to incur undesired synthetic steps. Successful a priori predictions can substantially help the industry to overcome material limitations, and the rational development of catalysts can unlock the true potential of electrolysers.
4. Materials and methods
Calculations reported in this paper have been carried out using Quantum ESPRESSO v6.8.172,173 The functionals used in this work are PBEsol for Re(111) and optB86b for bulk RuO2 with ultrasoft and PAW pseudopotentials, respectively, compiled from PSlibrary.174 Computational values in Table 1 are the electronic energies non-corrected for zero-point energy (ZPE) for all metals at the PBEsol level taken from literature,51 except those for Re(111), that have been calculated specifically for this work at the same level of theory. Plots in Fig. 9 were generated with Veusz.175
Appendix A
Eqn (1a) (acid environment), (1b) (alkaline environment) and (2) show the three main reactions taking place in a common electrolyser.
Anode:
| 2H2O → 4H+ + 4e−(↓↓↓↑) + ↑O O↑ 1.23 V vs. RHE | (1a) |
| 4OH− → 2H2O + 4e−(↓↓↓↑) + ↑O O↑ 1.23 V vs. RHE | (1b) |
Cathode:
| 4H+ + 4e− → 2H2 0.00 V vs. RHE | (2) |
Eqn (3)–(5) describe the three fundamental steps of the HER mechanism on a metal electrode in alkaline medium.176,177
| Hads + H2O + e− ⇆ H2 + OH− | (4) |
Author contributions
Conceptualisation by JG and SA. Software and calculations by CB and MF. Writing – original draft preparation by JG. Writing – review and editing by JG, SA and MF. All the authors have read and agreed to the published version of the manuscript.
Conflicts of interest
The authors declare no conflicts of interest.
Acknowledgements
This research was funded by the European Union's Horizon 2020 research and innovation program (Grant No. 964972). The authors thank the European Union for Horizon 2020 research and innovation program (Grant No. 964972).
References
- F. Zeng, C. Mebrahtu, L. Liao, A. K. Beine and R. Palkovits, J. Energy Chem., 2022, 69, 301–329 CrossRef CAS.
- L. M. Salonen, D. Y. Petrovykh and Yu. V. Kolen'ko, Mater. Today Sustain., 2021, 11–12, 100060 CrossRef.
- C. Minke, M. Suermann, B. Bensmann and R. Hanke-Rauschenbach, Int. J. Hydrogen Energy, 2021, 46, 23581–23590 CrossRef CAS.
- C. Biz, M. Fianchini and J. Gracia, ACS Catal., 2021, 11, 14249–14261 CrossRef CAS.
- J. Gracia, Phys. Chem. Chem. Phys., 2017, 19, 20451–20456 RSC.
- J. Gracia, R. Sharpe and J. Munarriz, J. Catal., 2018, 361, 331–338 CrossRef CAS.
- T. Lim, J. W. H. Niemantsverdriet and J. Gracia, ChemCatChem, 2016, 8, 2968–2974 CrossRef CAS.
- L. Zhang, A. Cheruvathur, C. Biz, M. Fianchini and J. Gracia, Phys. Chem. Chem. Phys., 2019, 21, 2977–2983 RSC.
- Y. Sun, S. Sun, H. Yang, S. Xi, J. Gracia and Z. J. Xu, Adv. Mater., 2020, 32, 2003297 CrossRef CAS PubMed.
- X. Ren, T. Wu, Y. Sun, Y. Li, G. Xian, X. Liu, C. Shen, J. Gracia, H.-J. Gao, H. Yang and Z. J. Xu, Nat. Commun., 2021, 12, 2608 CrossRef CAS PubMed.
- L. Li, J. Zhou, X. Wang, J. Gracia, M. Valvidares, J. Ke, M. Fang, C. Shen, J. Chen, Y. Chang, C. Pao, S. Hsu, J. Lee, A. Ruotolo, Y. Chin, Z. Hu, X. Huang and Q. Shao, Adv. Mater., 2023, 35, 35 Search PubMed.
- T. Wu, X. Ren, Y. Sun, S. Sun, G. Xian, G. G. Scherer, A. C. Fisher, D. Mandler, J. W. Ager, A. Grimaud, J. Wang, C. Shen, H. Yang, J. Gracia, H.-J. Gao and Z. J. Xu, Nat. Commun., 2021, 12, 3634 CrossRef CAS PubMed.
- T. Wu, Y. Sun, X. Ren, J. Wang, J. Song, Y. Pan, Y. Mu, J. Zhang, Q. Cheng, G. Xian, S. Xi, C. Shen, H. Gao, A. C. Fisher, M. P. Sherburne, Y. Du, J. W. Ager, J. Gracia, H. Yang, L. Zeng and Z. J. Xu, Adv. Mater., 2023, 35, 2207041 CrossRef CAS PubMed.
- D. Kurokawa, J. S. Gueriba and W. A. Diño, ACS Omega, 2018, 3, 9241–9245 CrossRef CAS PubMed.
- X. Wen, L. Ren, D. Du, Y. Yan, H. Xu, T. Zeng, G. Tian, X. Wang, S. Liu and C. Shu, Energy Fuels, 2023, 37, 735–745 CrossRef CAS.
- J. Hao, S. Xie, Q. Huang, Z. Ding, H. Sheng, C. Zhang and J. Yao, CCS Chem., 2022, 1–13 Search PubMed.
- Y. Jiao, R. Sharpe, T. Lim, J. W. H. Niemantsverdriet and J. Gracia, J. Am. Chem. Soc., 2017, 139, 16604–16608 CrossRef CAS PubMed.
- M. Pápai, Inorg. Chem., 2021, 60, 13950–13954 CrossRef PubMed.
- I. A. Gural'skiy, S. I. Shylin, V. Ksenofontov and W. Tremel, Eur. J. Inorg. Chem., 2017, 2017, 3125–3131 CrossRef.
- Y.-N. Gong, W. Zhong, Y. Li, Y. Qiu, L. Zheng, J. Jiang and H.-L. Jiang, J. Am. Chem. Soc., 2020, 142, 16723–16731 CrossRef CAS PubMed.
- C.-C. Lin, T.-R. Liu, S.-R. Lin, K. M. Boopathi, C.-H. Chiang, W.-Y. Tzeng, W.-H. C. Chien, H.-S. Hsu, C.-W. Luo, H.-Y. Tsai, H.-A. Chen, P.-C. Kuo, J. Shiue, J.-W. Chiou, W.-F. Pong, C.-C. Chen and C.-W. Chen, J. Am. Chem. Soc., 2022, 144, 15718–15726 CrossRef CAS PubMed.
- X. Song and B. Wang, J. Chem. Theory Comput., 2023, 19, 2684–2696 CrossRef CAS PubMed.
- P. J. González, M. G. Rivas, F. M. Ferroni, A. C. Rizzi and C. D. Brondino, Coord. Chem. Rev., 2021, 449, 214202 CrossRef.
- L. Pan, M. Ai, C. Huang, L. Yin, X. Liu, R. Zhang, S. Wang, Z. Jiang, X. Zhang, J.-J. Zou and W. Mi, Nat. Commun., 2020, 11, 418 CrossRef CAS PubMed.
- R. Naaman, Y. Paltiel and D. H. Waldeck, Annu. Rev. Biophys., 2022, 51, 99–114 CrossRef PubMed.
- C. Huang, C. Chen, M. Zhang, L. Lin, X. Ye, S. Lin, M. Antonietti and X. Wang, Nat. Commun., 2015, 6, 7698 CrossRef PubMed.
- Y. Tian, H. Cao, H. Yang, W. Yao, J. Wang, Z. Qiao and A. K. Cheetham, Angew. Chem., Int. Ed., 2023, 62, 10 Search PubMed.
- C. Fu, L. Luo, L. Yang, S. Shen, G. Wei and J. Zhang, Catal. Sci. Technol., 2021, 11, 7764–7772 RSC.
- Y. Shan, R. Xu, Y. Zhu, C.-G. Shi and T. Li, Chem. Phys., 2023, 565, 111730 CrossRef CAS.
- J. Miao, Y. Zhu, J. Lang, J. Zhang, S. Cheng, B. Zhou, L. Zhang, P. J. J. Alvarez and M. Long, ACS Catal., 2021, 11, 9569–9577 CrossRef CAS.
- J. Feng, S. Shaik and B. Wang, Angew. Chem., Int. Ed., 2021, 60, 20430–20436 CrossRef CAS PubMed.
- Z. Li, R. Ma, Q. Ju, Q. Liu, L. Liu, Y. Zhu, M. Yang and J. Wang, Innovation, 2022, 3, 100268 CAS.
- T. Sun, Z. Tang, W. Zang, Z. Li, J. Li, Z. Li, L. Cao, J. S. Dominic Rodriguez, C. O. M. Mariano, H. Xu, P. Lyu, X. Hai, H. Lin, X. Sheng, J. Shi, Y. Zheng, Y.-R. Lu, Q. He, J. Chen, K. S. Novoselov, C.-H. Chuang, S. Xi, X. Luo and J. Lu, Nat. Nanotechnol., 2023, 18, 763–771 CrossRef CAS PubMed.
- Y. Wang, W. Cheng, P. Yuan, G. Yang, S. Mu, J. Liang, H. Xia, K. Guo, M. Liu, S. Zhao, G. Qu, B. Lu, Y. Hu, J. Hu and J. Zhang, Adv. Sci., 2021, 8, 2102915 CrossRef CAS PubMed.
- F. Liu, T. Yang, J. Yang, E. Xu, A. Bajaj and H. J. Kulik, Front. Chem., 2019, 7 Search PubMed.
- Z. Li, Z. Wang, S. Xi, X. Zhao, T. Sun, J. Li, W. Yu, H. Xu, T. S. Herng, X. Hai, P. Lyu, M. Zhao, S. J. Pennycook, J. Ding, H. Xiao and J. Lu, ACS Nano, 2021, 15, 7105–7113 CrossRef CAS PubMed.
- Q. Wang, T. Liu, K. Chen, D. Wu, C. Chen, M. Chen, X. Ma, J. Xu, T. Yao, Y. Li, H. Zhou and Y. Wu, Small, 2022, 18, 2204015 CrossRef CAS PubMed.
- D. Xue, P. Yuan, S. Jiang, Y. Wei, Y. Zhou, C.-L. Dong, W. Yan, S. Mu and J.-N. Zhang, Nano Energy, 2023, 105, 108020 CrossRef CAS.
- Q. Dang, S. Tang, T. Liu, X. Li, X. Wang, W. Zhong, Y. Luo and J. Jiang, J. Phys. Chem. Lett., 2021, 12, 8355–8362 CrossRef CAS PubMed.
- X. Wei, S. Song, W. Cai, X. Luo, L. Jiao, Q. Fang, X. Wang, N. Wu, Z. Luo, H. Wang, Z. Zhu, J. Li, L. Zheng, W. Gu, W. Song, S. Guo and C. Zhu, Chem, 2023, 9, 181–197 CAS.
- N. Li, Z. Wang, P. Zhang, X. Li, A. Arramel, C. Sun, X. Zhou and X. Zhao, J. Mater. Chem. A, 2022, 10, 22760–22770 RSC.
- Y. Gu, X. Wang, M. Humayun, L. Li, H. Sun, X. Xu, X. Xue, A. Habibi-Yangjeh, K. Temst and C. Wang, Chin. J. Catal., 2022, 43, 839–850 CrossRef CAS.
- Y. Rao, S. Chen, Q. Yue and Y. Kang, ACS Catal., 2021, 11, 8097–8103 CrossRef CAS.
- F. Si, J. Liu, Y. Zhang, B. Zhao, Y. Liang, X. Wu, X. Kang, X. Yang, J. Zhang, X. Fu and J. Luo, Small, 2023, 19, 2205257 CrossRef CAS PubMed.
- W. Gao, Y. Zou, Y. Zang, X. Zhao, W. Zhou, Y. Dai, H. Liu, J.-J. Wang, Y. Ma and Y. Sang, Chem. Eng. J., 2023, 455, 140821 CrossRef CAS.
- Y. Wen, J. Liu, F. Zhang, Z. Li, P. Wang, Z. Fang, M. He, J. Chen, W. Song, R. Si and L. Wang, Nano Res., 2023, 16, 4664–4670 CrossRef CAS.
- Y. Li, Z. Wang, Y. Wang, A. Kovács, C. Foo, R. E. Dunin-Borkowski, Y. Lu, R. A. Taylor, C. Wu and S. C. E. Tsang, Energy Environ. Sci., 2022, 15, 265–277 RSC.
- Q. Xue, Y. Wang, M. Jiang, R. Cheng, K. Li, T. Zhao and C. Fu, ACS Appl. Energy Mater., 2023, 6, 1888–1896 CrossRef CAS.
- X. Ren, T. Wu, Z. Gong, L. Pan, J. Meng, H. Yang, F. B. Dagbjartsdottir, A. Fisher, H.-J. Gao and Z. J. Xu, Nat. Commun., 2023, 14, 2482 CrossRef CAS PubMed.
- C. Biz, J. Gracia and M. Fianchini, Int. J. Mol. Sci., 2022, 23, 14768 CrossRef CAS PubMed.
- J. Gracia, C. Biz and M. Fianchini, Mater. Today Commun., 2020, 23, 100894 CrossRef CAS.
- C. Biz, M. Fianchini and J. Gracia, ACS Appl. Nano Mater., 2020, 3, 506–515 CrossRef CAS.
- C. Biz, M. Fianchini, V. Polo and J. Gracia, ACS Appl. Mater. Interfaces, 2020, 12, 50484–50494 CrossRef CAS PubMed.
- E. D. Jemmis and S. Ghorai, Isr. J. Chem., 2022, 62, 1–2 CrossRef.
- I. Vincent and D. Bessarabov, Renewable Sustainable Energy Rev., 2018, 81, 1690–1704 CrossRef CAS.
- M. R. Domalanta, J. N. Bamba, D. D. Matienzo, J. A. del Rosario-Paraggua and J. Ocon, ChemSusChem, 2023, 16, 13 CrossRef PubMed.
- A. Buttler and H. Spliethoff, Renewable Sustainable Energy Rev., 2018, 82, 2440–2454 CrossRef CAS.
- A. Ursua, L. M. Gandia and P. Sanchis, Proc. IEEE, 2012, 100, 410–426 CAS.
- H. Kojima, K. Nagasawa, N. Todoroki, Y. Ito, T. Matsui and R. Nakajima, Int. J. Hydrogen Energy, 2023, 48, 4572–4593 CrossRef CAS.
- K. Ayers, Curr. Opin. Electrochem., 2019, 18, 9–15 CrossRef CAS.
- H.-O. Lee, J. Yesuraj and K. Kim, Appl. Energy, 2022, 314, 118928 CrossRef CAS.
- S. Stiber, H. Balzer, A. Wierhake, F. J. Wirkert, J. Roth, U. Rost, M. Brodmann, J. K. Lee, A. Bazylak, W. Waiblinger, A. S. Gago and K. A. Friedrich, Adv. Energy Mater., 2021, 11, 2100630 CrossRef CAS.
- O. Schmidt, A. Gambhir, I. Staffell, A. Hawkes, J. Nelson and S. Few, Int. J. Hydrogen Energy, 2017, 42, 30470–30492 CrossRef CAS.
- A. Hofrichter, D. Rank, M. Heberl and M. Sterner, Int. J. Hydrogen Energy, 2023, 48, 1651–1663 CrossRef CAS.
- M. I. Zappia, S. Bellani, Y. Zuo, M. Ferri, F. Drago, L. Manna and F. Bonaccorso, Front. Chem., 2022, 10 DOI:10.3389/fchem.2022.1045212.
- G. Bristowe and A. Smallbone, Hydrogen, 2021, 2, 273–300 CrossRef CAS.
- S. Shiva Kumar and V. Himabindu, Mater. Sci. Energy Technol., 2019, 2, 442–454 Search PubMed.
- W. Kuckshinrichs, T. Ketelaer and J. C. Koj, Front. Energy Res., 2017, 5 DOI:10.3389/fenrg.2017.00001.
- X. Ren, T. Wu, Z. Gong, L. Pan, J. Meng, H. Yang, F. B. Dagbjartsdottir, A. Fisher, H.-J. Gao and Z. J. Xu, Nat. Commun., 2023, 14, 2482 CrossRef CAS PubMed.
- M. Carmo, D. L. Fritz, J. Mergel and D. Stolten, Int. J. Hydrogen Energy, 2013, 38, 4901–4934 CrossRef CAS.
- P. Yu, F. Wang, T. A. Shifa, X. Zhan, X. Lou, F. Xia and J. He, Nano Energy, 2019, 58, 244–276 CrossRef CAS.
- J. K. Nørskov, T. Bligaard, A. Logadottir, J. R. Kitchin, J. G. Chen, S. Pandelov and U. Stimming, J. Electrochem. Soc., 2005, 152, J23 CrossRef.
- D. Liu, W. Zhao and Q. Yuan, ChemPhysChem, 2022, 17 DOI:10.1002/cphc.202200147.
- J. Pan, T. Li and Y. Shan, Phys. Status Solidi RRL, 2023 DOI:10.1002/pssr.202300124.
- T. Wu, Y. Sun, X. Ren, J. Wang, J. Song, Y. Pan, Y. Mu, J. Zhang, Q. Cheng, G. Xian, S. Xi, C. Shen, H. Gao, A. C. Fisher, M. P. Sherburne, Y. Du, J. W. Ager, J. Gracia, H. Yang, L. Zeng and Z. J. Xu, Adv. Mater., 2023, 35, 2207041 CrossRef CAS PubMed.
- S. Deshpande, J. R. Kitchin and V. Viswanathan, ACS Catal., 2016, 6, 5251–5259 CrossRef CAS.
- D. Krishnamurthy, V. Sumaria and V. Viswanathan, J. Phys. Chem. Lett., 2018, 9, 588–595 CrossRef CAS PubMed.
- E. Sargeant, F. Illas, P. Rodríguez and F. Calle-Vallejo, Electrochim. Acta, 2022, 426, 140799 CrossRef CAS.
- O. I. Malyi and A. Zunger, Appl. Phys. Rev., 2020, 7, 041310 CAS.
- M. Fianchini, Phys. Sci. Rev., 2017, 2 DOI:10.1515/psr-2017-0134.
- J. Zeng, S. Liao, J. Y. Lee and Z. Liang, Int. J. Hydrogen Energy, 2010, 35, 942–948 CrossRef CAS.
- S. Ekeroth, J. Ekspong, D. K. Perivoliotis, S. Sharma, R. Boyd, N. Brenning, E. Gracia-Espino, L. Edman, U. Helmersson and T. Wågberg, ACS Appl. Nano Mater., 2021, 4, 12957–12965 CrossRef CAS.
- S. Luo, K. Elouarzaki and Z. J. Xu, Angew. Chem., Int. Ed., 2022, 61 DOI:10.1002/anie.202203564.
- W. Kiciński, J. P. Sęk, A. Kowalczyk, S. Turczyniak-Surdacka, A. M. Nowicka, S. Dyjak, B. Budner and M. Donten, J. Energy Chem., 2022, 64, 296–308 CrossRef.
- H. Zheng, Y. Wang, J. Xie, P. Gao, D. Li, E. V. Rebrov, H. Qin, X. Liu and H. Xiao, ACS Appl. Mater. Interfaces, 2022, 14, 34627–34636 CrossRef CAS PubMed.
- Y. Zhang, C. Liang, J. Wu, H. Liu, B. Zhang, Z. Jiang, S. Li and P. Xu, ACS Appl. Energy Mater., 2020, 3, 10303–10316 CrossRef CAS.
- H. Li, S. Liu and Y. Liu, ACS Sustainable Chem. Eng., 2021, 9, 12376–12384 CrossRef CAS.
- F. A. Garcés-Pineda, M. Blasco-Ahicart, D. Nieto-Castro, N. López and J. R. Galán-Mascarós, Nat. Energy, 2019, 4, 519–525 CrossRef.
- X. Qin, J. Teng, W. Guo, L. Wang, S. Xiao, Q. Xu, Y. Min and J. Fan, Catal. Lett., 2023, 153, 673–681 CrossRef CAS.
- J. Guo, H. Jiang, Y. Teng, Y. Xiong, Z. Chen, L. You and D. Xiao, J. Mater. Chem. B, 2021, 9, 9076–9099 RSC.
- L. M. Martinez, J. A. Delgado, C. L. Saiz, A. Cosio, Y. Wu, D. Villagrán, K. Gandha, C. Karthik, I. C. Nlebedim and S. R. Singamaneni, J. Appl. Phys., 2018, 124, 153903 CrossRef.
- W. Zhou, M. Chen, M. Guo, A. Hong, T. Yu, X. Luo, C. Yuan, W. Lei and S. Wang, Nano Lett., 2020, 20, 2923–2930 CrossRef CAS PubMed.
- Y. Zhang, P. Guo, S. Li, J. Sun, W. Wang, B. Song, X. Yang, X. Wang, Z. Jiang, G. Wu and P. Xu, J. Mater. Chem. A, 2022, 10, 1760–1767 RSC.
- X. Lyu, Y. Zhang, Z. Du, H. Chen, S. Li, A. I. Rykov, C. Cheng, W. Zhang, L. Chang, W. Kai, J. Wang, L. Zhang, Q. Wang, C. Huang and E. Kan, Small, 2022, 18, 2204143 CrossRef PubMed.
- Y. Zhang, M. Chen, P. Guo, Y. Du, B. Song, X. Wang, Z. Jiang and P. Xu, Carbon Energy, 2023 DOI:10.1002/cey2.351.
- D. Kim, I. Efe, H. Torlakcik, A. Terzopoulou, A. Veciana, E. Siringil, F. Mushtaq, C. Franco, D. Arx, S. Sevim, J. Puigmartí-Luis, B. Nelson, N. A. Spaldin, C. Gattinoni, X. Chen and S. Pané, Adv. Mater., 2022, 34, 2110612 CrossRef CAS PubMed.
- T. Wu, Y. Sun, X. Ren, J. Wang, J. Song, Y. Pan, Y. Mu, J. Zhang, Q. Cheng, G. Xian, S. Xi, C. Shen, H. Gao, A. C. Fisher, M. P. Sherburne, Y. Du, J. W. Ager, J. Gracia, H. Yang, L. Zeng and Z. J. Xu, Adv. Mater., 2022, 2207041 Search PubMed.
- T. Wu, X. Ren, Y. Sun, S. Sun, G. Xian, G. G. Scherer, A. C. Fisher, D. Mandler, J. W. Ager, A. Grimaud, J. Wang, C. Shen, H. Yang, J. Gracia, H.-J. Gao and Z. J. Xu, Nat. Commun., 2021, 12, 3634 CrossRef CAS PubMed.
- X. Ren, T. Wu, Y. Sun, Y. Li, G. Xian, X. Liu, C. Shen, J. Gracia, H.-J. Gao, H. Yang and Z. J. Xu, Nat. Commun., 2021, 12, 2608 CrossRef CAS PubMed.
- L. Ren, X. Wen, D. Du, Y. Yan, H. Xu, T. Zeng and C. Shu, SSRN Electronic Journal, 2022 DOI:10.2139/ssrn.4306083.
- S. Liu, B. Zhang, Y. Cao, H. Wang, Y. Zhang, S. Zhang, Y. Li, H. Gong, S. Liu, Z. Yang and J. Sun, ACS Energy Lett., 2023, 8, 159–168 CrossRef CAS.
- C. Y. Zhang, C. Zhang, G. W. Sun, J. L. Pan, L. Gong, G. Z. Sun, J. J. Biendicho, L. Balcells, X. L. Fan, J. R. Morante, J. Y. Zhou and A. Cabot, Angew. Chem., Int. Ed. DOI:10.1002/anie.202211570.
- Q. Zhang, R. Ao, R. Gao and H. Yang, Inorg. Chem., 2022, 61, 19780–19789 CrossRef CAS PubMed.
- M. F. Lagadec and A. Grimaud, Nat. Mater., 2020, 19, 1140–1150 CrossRef CAS PubMed.
- T. Wu and Z. J. Xu, Curr. Opin. Electrochem., 2021, 30, 100804 CrossRef CAS.
- E. Fabbri and T. J. Schmidt, ACS Catal., 2018, 8, 9765–9774 CrossRef CAS.
- L. An, C. Wei, M. Lu, H. Liu, Y. Chen, G. G. Scherer, A. C. Fisher, P. Xi, Z. J. Xu and C. Yan, Adv. Mater. DOI:10.1002/adma.202006328.
- J. Song, C. Wei, Z.-F. Huang, C. Liu, L. Zeng, X. Wang and Z. J. Xu, Chem. Soc. Rev., 2020, 49, 2196–2214 RSC.
- Q. Wang, Y. Cheng, H. B. Tao, Y. Liu, X. Ma, D. Li, H. Bin Yang and B. Liu, Angew. Chem., Int. Ed. DOI:10.1002/anie.202216645.
- S. She, Y. Zhu, X. Wu, Z. Hu, A. Shelke, W. Pong, Y. Chen, Y. Song, M. Liang, C. Chen, H. Wang, W. Zhou and Z. Shao, Adv. Funct. Mater., 2022, 32, 2111091 CrossRef CAS.
- W. T. Hong, R. E. Welsch and Y. Shao-Horn, J. Phys. Chem. C, 2016, 120, 78–86 CrossRef CAS.
- J. Gracia, J. Phys. Chem. C, 2019, 123, 9967–9972 CrossRef CAS.
- R. Sharpe, T. Lim, Y. Jiao, J. W. H. Niemantsverdriet and J. Gracia, ChemCatChem, 2016, 8, 3762–3768 CrossRef CAS.
- J. Ge, X. Ren, R. R. Chen, Y. Sun, T. Wu, S. J. H. Ong and Z. J. Xu, Angew. Chem., Int. Ed., 2023, 62 DOI:10.1002/anie.202301721.
- B. Tian, H. Shin, S. Liu, M. Fei, Z. Mu, C. Liu, Y. Pan, Y. Sun, W. A. Goddard and M. Ding, Angew. Chem., Int. Ed., 2021, 60, 16448–16456 CrossRef CAS PubMed.
- L. Lin, R. Xin, M. Yuan, T. Wang, J. Li, Y. Xu, X. Xu, M. Li, Y. Du, J. Wang, S. Wang, F. Jiang, W. Wu, C. Lu, B. Huang, Z. Sun, J. Liu, J. He and G. Sun, ACS Catal., 2023, 13, 1431–1440 CrossRef CAS.
- J. B. Goodenough, Rep. Prog. Phys., 2004, 67, 1915–1993 CrossRef CAS.
- Y. Sun, S. Sun, H. Yang, S. Xi, J. Gracia and Z. J. Xu, Adv. Mater., 2020, 32, 2003297 CrossRef CAS PubMed.
- G. Zhou, P. Wang, B. Hu, X. Shen, C. Liu, W. Tao, P. Huang and L. Liu, Nat. Commun., 2022, 13, 4106 CrossRef CAS PubMed.
- L. Wang, H. Yang, J. Yang, Y. Yang, R. Wang, S. Li, H. Wang and S. Ji, Ionics, 2016, 22, 2195–2202 CrossRef CAS.
- X. Li, H. Wang, Z. Cui, Y. Li, S. Xin, J. Zhou, Y. Long, C. Jin and J. B. Goodenough, Sci. Adv., 2019, 5 DOI:10.1126/sciadv.aav6262.
- G. Trimarchi, Z. Wang and A. Zunger, Phys. Rev. B, 2018, 97, 035107 CrossRef CAS.
- J. Varignon, M. Bibes and A. Zunger, Nat. Commun., 2019, 10, 1658 CrossRef PubMed.
- J. Varignon, O. I. Malyi and A. Zunger, Phys. Rev. B, 2022, 105, 165111 CrossRef CAS.
- Z. Feng, X. Zhou, L. Šmejkal, L. Wu, Z. Zhu, H. Guo, R. González-Hernández, X. Wang, H. Yan, P. Qin, X. Zhang, H. Wu, H. Chen, Z. Meng, L. Liu, Z. Xia, J. Sinova, T. Jungwirth and Z. Liu, Nat. Electron., 2022, 5, 735–743 CrossRef CAS.
- Z. H. Zhu, J. Strempfer, R. R. Rao, C. A. Occhialini, J. Pelliciari, Y. Choi, T. Kawaguchi, H. You, J. F. Mitchell, Y. Shao-Horn and R. Comin, Phys. Rev. Lett., 2019, 122, 017202 CrossRef CAS PubMed.
- Y. Tian, S. Wang, E. Velasco, Y. Yang, L. Cao, L. Zhang, X. Li, Y. Lin, Q. Zhang and L. Chen, iScience, 2020, 23, 100756 CrossRef CAS PubMed.
- Y. Lee, J. Suntivich, K. J. May, E. E. Perry and Y. Shao-Horn, J. Phys. Chem. Lett., 2012, 3, 399–404 CrossRef CAS PubMed.
- Y. Yang, Y. Yu, J. Li, Q. Chen, Y. Du, P. Rao, R. Li, C. Jia, Z. Kang, P. Deng, Y. Shen and X. Tian, Nano-Micro Lett., 2021, 13, 160 CrossRef CAS PubMed.
- H. Jin, X. Liu, P. An, C. Tang, H. Yu, Q. Zhang, H.-J. Peng, L. Gu, Y. Zheng, T. Song, K. Davey, U. Paik, J. Dong and S.-Z. Qiao, Nat. Commun., 2023, 14, 354 CrossRef CAS PubMed.
- Y. Lin, Z. Tian, L. Zhang, J. Ma, Z. Jiang, B. J. Deibert, R. Ge and L. Chen, Nat. Commun., 2019, 10, 162 CrossRef PubMed.
- Z. Shi, J. Li, Y. Wang, S. Liu, J. Zhu, J. Yang, X. Wang, J. Ni, Z. Jiang, L. Zhang, Y. Wang, C. Liu, W. Xing and J. Ge, Nat. Commun., 2023, 14, 843 CrossRef CAS PubMed.
- X. Gao, Z. Sun, J. Ran, J. Li, J. Zhang and D. Gao, Sci. Rep., 2020, 10, 13395 CrossRef CAS PubMed.
- D. Zhang, Y. Song, Z. Du, L. Wang, Y. Li and J. B. Goodenough, J. Mater. Chem. A, 2015, 3, 9421–9426 RSC.
- Y. Sun, J. Wang, Q. Liu, M. Xia, Y. Tang, F. Gao, Y. Hou, J. Tse and Y. Zhao, J. Mater. Chem. A, 2019, 7, 27175–27185 RSC.
- J. Munarriz, V. Polo and J. Gracia, ChemPhysChem, 2018, 19, 2843–2847 CrossRef CAS PubMed.
- J. M. Gracia, F. F. Prinsloo and J. W. Niemantsverdriet, Catal. Lett., 2009, 133, 257–261 CrossRef CAS.
- H. Yu, T. Quan, S. Mei, Z. Kochovski, W. Huang, H. Meng and Y. Lu, Nano-Micro Lett., 2019, 11, 41 CrossRef CAS PubMed.
- F. Bao, E. Kemppainen, I. Dorbandt, R. Bors, F. Xi, R. Schlatmann, R. van de Krol and S. Calnan, ChemElectroChem, 2021, 8, 195–208 CrossRef CAS.
- H. Yang and J. L. Whitten, J. Chem. Phys., 1988, 89, 5329–5334 CrossRef CAS.
- G. Lee, P. T. Sprunger, M. Okada, D. B. Poker, D. M. Zehner and E. W. Plummer, J. Vac. Sci. Technol., A, 1994, 12, 2119–2123 CrossRef CAS.
- J. R. Engstrom, W. Tsai and W. H. Weinberg, J. Chem. Phys., 1987, 87, 3104–3119 CrossRef CAS.
- F. C. Østergaard, A. Bagger and J. Rossmeisl, Curr. Opin. Electrochem., 2022, 35, 101037 CrossRef.
- W. Sheng, M. Myint, J. G. Chen and Y. Yan, Energy Environ. Sci., 2013, 6, 1509 RSC.
- A. Vojvodic, J. K. Nørskov and F. Abild-Pedersen, Top. Catal., 2014, 57, 25–32 CrossRef CAS.
- A. R. Zeradjanin, J.-P. Grote, G. Polymeros and K. J. J. Mayrhofer, Electroanalysis, 2016, 28, 2256–2269 CrossRef CAS.
- G. K. Gebremariam, A. Z. Jovanović, A. S. Dobrota, N. V. Skorodumova and I. A. Pašti, Catalysts, 2022, 12, 1541 CrossRef CAS.
- P. Quaino, F. Juarez, E. Santos and W. Schmickler, Beilstein J. Nanotechnol., 2014, 5, 846–854 CrossRef PubMed.
- M. M. Jakšić, C. Lacnjevac, B. N. Grgur and N. V. Krstajić, J. New Mater. Electrochem. Syst., 2000, 3(2), 169–182 Search PubMed.
- S. Trasatti, J. Electroanal. Chem. Interfacial Electrochem., 1972, 39, 163–184 CrossRef CAS.
- M. T. Gorzkowski and A. Lewera, J. Phys. Chem. C, 2015, 119, 18389–18395 CrossRef CAS.
- B. Zandkarimi and A. N. Alexandrova, J. Phys. Chem. Lett., 2019, 10, 460–467 CrossRef PubMed.
- C. Deng, W. Li, R. He, W. Shen and M. Li, J. Phys. Chem. C, 2020, 124, 19530–19537 CrossRef CAS.
- A. Brito-Ravicini and F. Calle-Vallejo, Exploration, 2022, 2, 20210062 CrossRef PubMed.
- V. Vennelakanti, A. Nandy and H. J. Kulik, Top. Catal., 2022, 65, 296–311 CrossRef CAS.
- L. Yu, Q. Yan and A. Ruzsinszky, Phys. Rev. Mater., 2019, 3, 092801 CrossRef CAS.
- H. Xin and S. Linic, J. Chem. Phys., 2010, 132, 221101 CrossRef PubMed.
- N. Govindarajan, J. M. García-Lastra, E. J. Meijer and F. Calle-Vallejo, Curr. Opin. Electrochem., 2018, 8, 110–117 CrossRef CAS.
- J. Liu, X. Liu, H. Shi, J. Luo, L. Wang, J. Liang, S. Li, L.-M. Yang, T. Wang, Y. Huang and Q. Li, Appl. Catal., B, 2022, 302, 120862 CrossRef CAS.
- M. Plevová, J. Hnát and K. Bouzek, J. Power Sources, 2021, 507, 230072 CrossRef.
- Q. Xu, G. Li, Y. Zhang, Q. Yang, Y. Sun and C. Felser, ACS Catal., 2020, 10, 5042–5048 CrossRef CAS PubMed.
- S. D. Miller, N. İnoğlu and J. R. Kitchin, J. Chem. Phys., 2011, 134, 104709 CrossRef PubMed.
- A. Cao and J. K. Nørskov, ACS Catal., 2023, 13, 3456–3462 CrossRef CAS.
- B. S. Mun, M. Watanabe, M. Rossi, V. Stamenkovic, N. M. Markovic and P. N. Ross, J. Chem. Phys., 2005, 123, 204717 CrossRef PubMed.
- S. Kobayashi, D. A. Tryk and H. Uchida, Electrochem. Commun., 2020, 110, 106615 CrossRef CAS.
- C. Lin, Z. Huang, Z. Zhang, T. Zeng, R. Chen, Y. Tan, W. Wu, S. Mu and N. Cheng, ACS Sustainable Chem. Eng., 2020, 8, 16938–16945 CrossRef CAS.
- Z. Wang, X. Ren, Y. Luo, L. Wang, G. Cui, F. Xie, H. Wang, Y. Xie and X. Sun, Nanoscale, 2018, 10, 12302–12307 RSC.
- Q. Xie, J. Li, K. Wang, S. Li, W. Xu, Y. Wang, L. Lei, S. Li, L. Zhuang and Z. Xu, Mater. Chem. Front., 2023, 7, 1607–1616 RSC.
- S. L. Zhang, X. F. Lu, Z. Wu, D. Luan and X. W. Lou, Angew. Chem., Int. Ed., 2021, 60, 19068–19073 CrossRef CAS PubMed.
- Z. Cao, F. Dong, J. Pan, W. Xia, J.-G. Hu and X. Xu, ACS Appl. Energy Mater., 2022, 5, 1496–1504 CrossRef CAS.
- Y. Zhao, P. V. Kumar, X. Tan, X. Lu, X. Zhu, J. Jiang, J. Pan, S. Xi, H. Y. Yang, Z. Ma, T. Wan, D. Chu, W. Jiang, S. C. Smith, R. Amal, Z. Han and X. Lu, Nat. Commun., 2022, 13, 2430 CrossRef CAS PubMed.
- P. Giannozzi, S. Baroni, N. Bonini, M. Calandra, R. Car, C. Cavazzoni, D. Ceresoli, G. L. Chiarotti, M. Cococcioni, I. Dabo, A. Dal Corso, S. de Gironcoli, S. Fabris, G. Fratesi, R. Gebauer, U. Gerstmann, C. Gougoussis, A. Kokalj, M. Lazzeri, L. Martin-Samos, N. Marzari, F. Mauri, R. Mazzarello, S. Paolini, A. Pasquarello, L. Paulatto, C. Sbraccia, S. Scandolo, G. Sclauzero, A. P. Seitsonen, A. Smogunov, P. Umari and R. M. Wentzcovitch, J. Phys.: Condens. Matter, 2009, 21, 395502 CrossRef PubMed.
- P. Giannozzi, O. Baseggio, P. Bonfà, D. Brunato, R. Car, I. Carnimeo, C. Cavazzoni, S. de Gironcoli, P. Delugas, F. Ferrari Ruffino, A. Ferretti, N. Marzari, I. Timrov, A. Urru and S. Baroni, J. Chem. Phys., 2020, 152 DOI:10.1063/5.0005082.
- A. Dal Corso, Comput. Mater. Sci., 2014, 95, 337–350 CrossRef CAS.
-
J. Sanders, Veusz – a scientific plotting package, 2023, Https://veusz.github.io Search PubMed.
- J. M. Jakšić, M. V. Vojnović and N. V. Krstajić, Electrochim. Acta, 2000, 45, 4151–4158 CrossRef.
- J. O. Bockris and E. C. Potter, J. Chem. Phys., 1952, 20, 614–628 CrossRef CAS.
|
This journal is © The Royal Society of Chemistry 2023 |
Click here to see how this site uses Cookies. View our privacy policy here.